730 Lecture 11 Todays lecture 9162021 730 Lecture
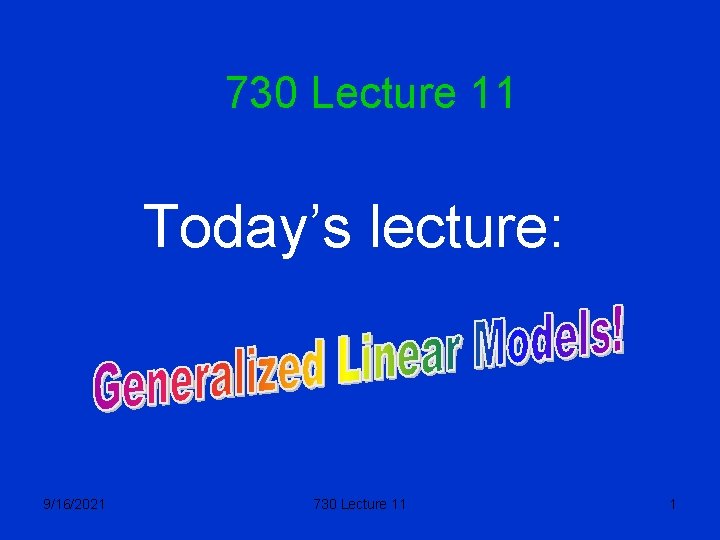
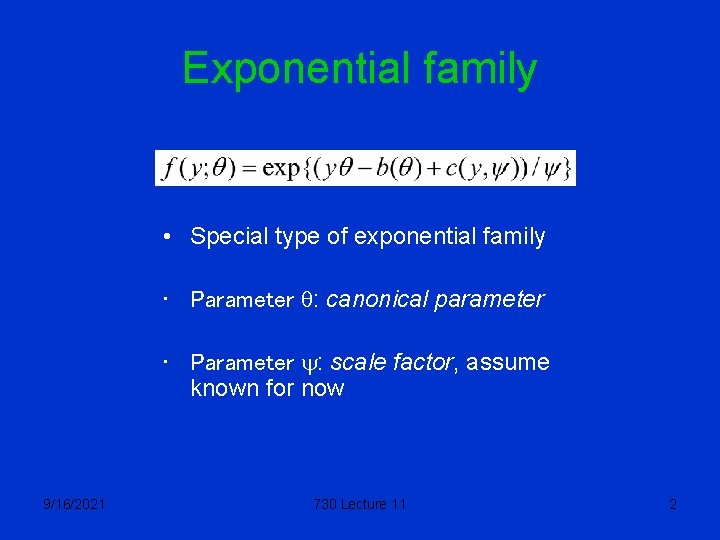
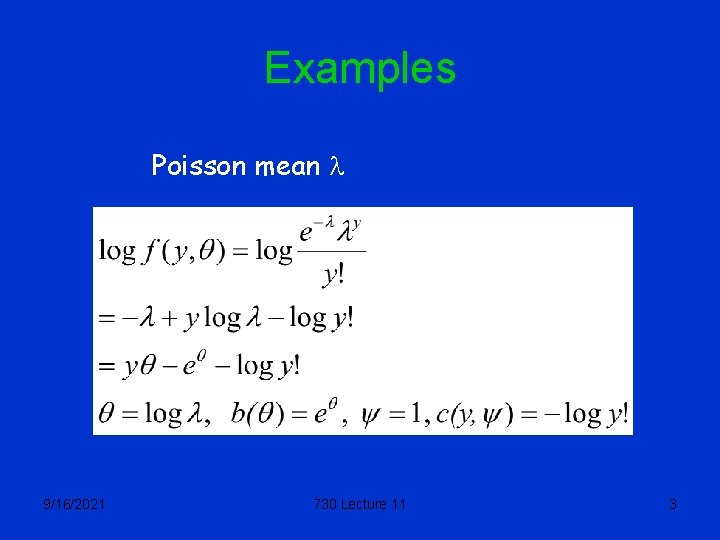
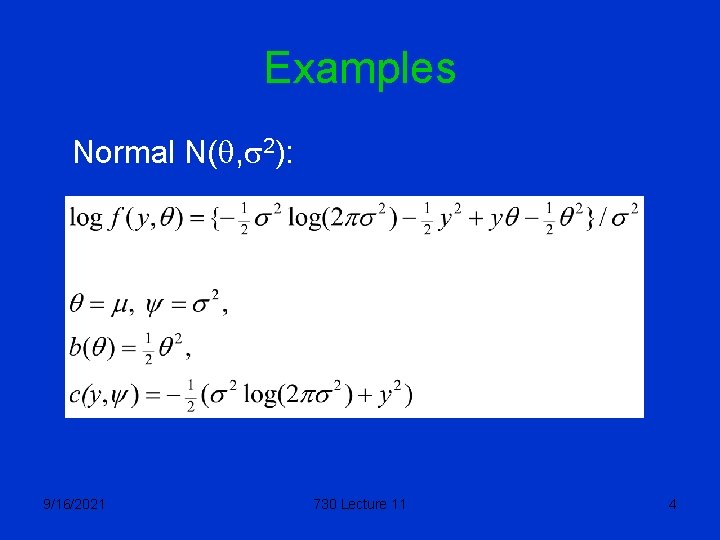
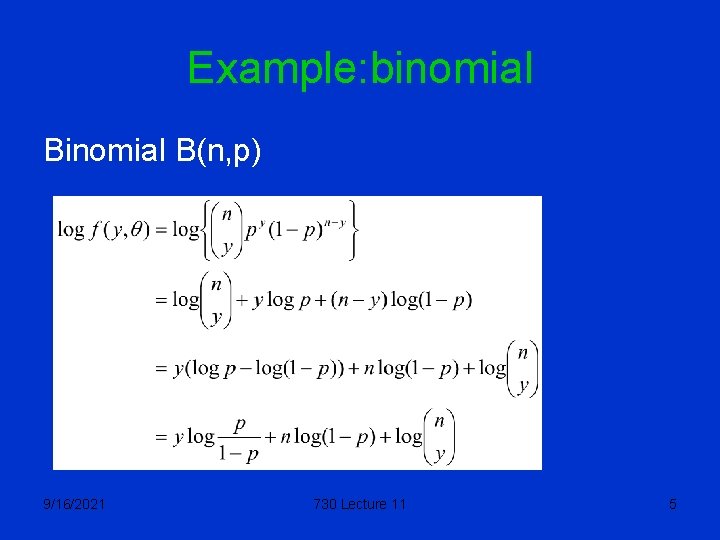
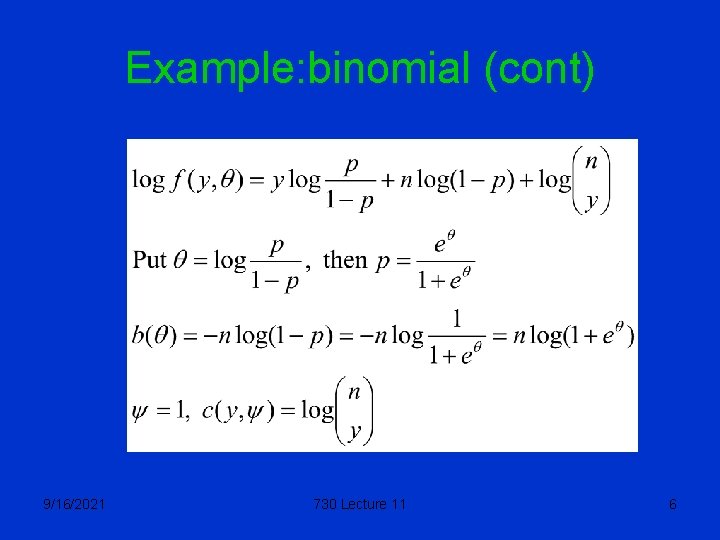
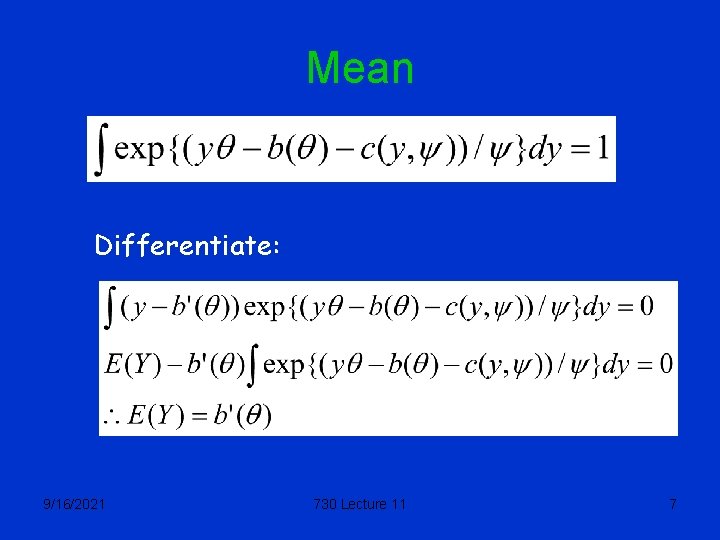
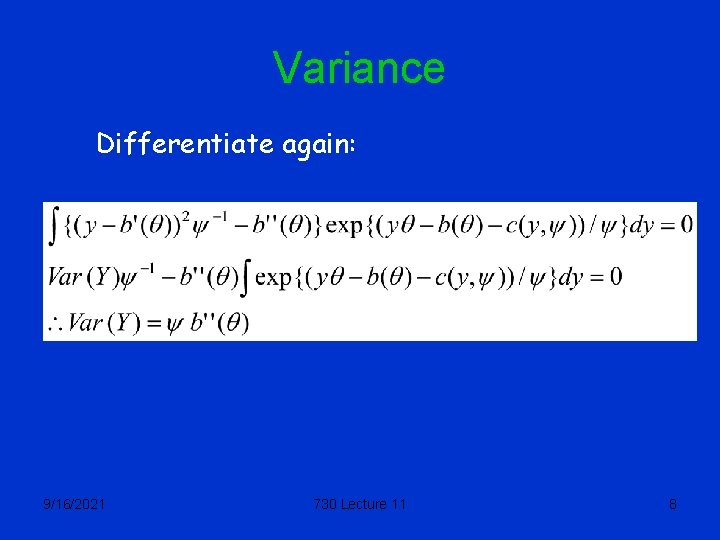
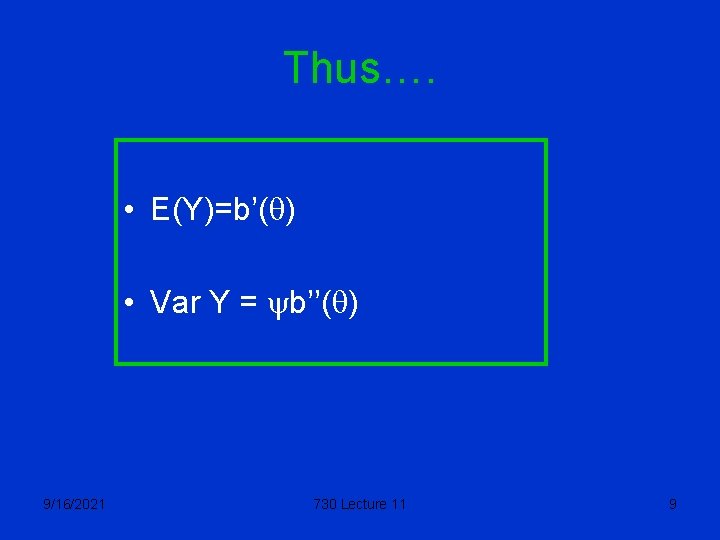
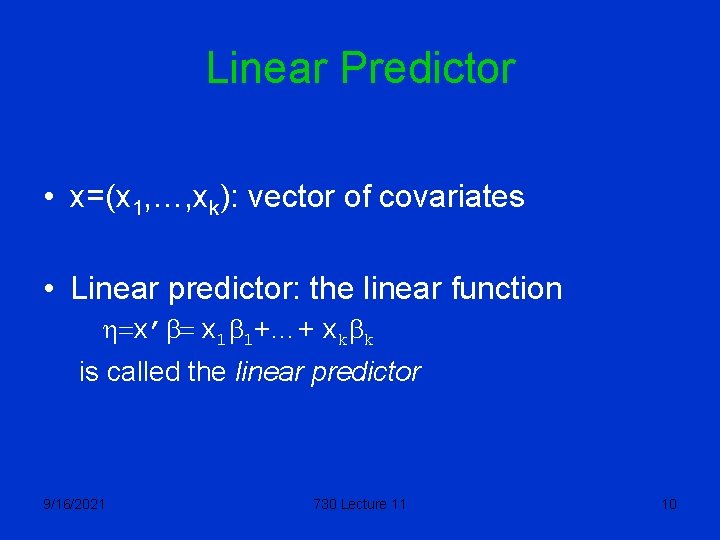
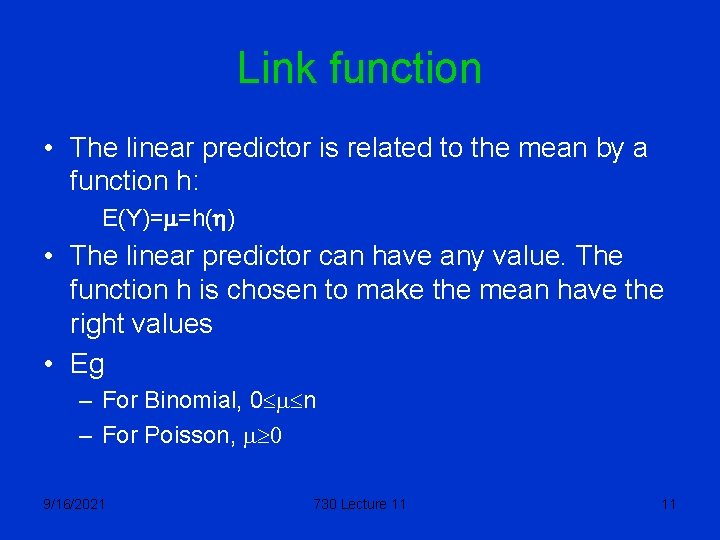
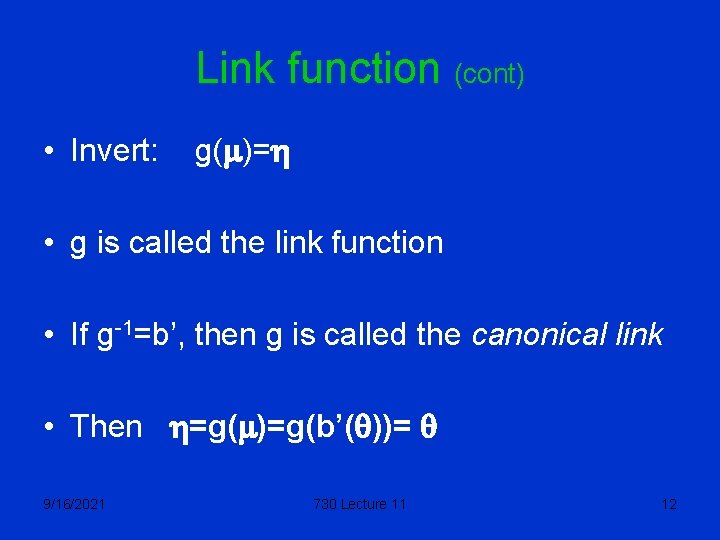
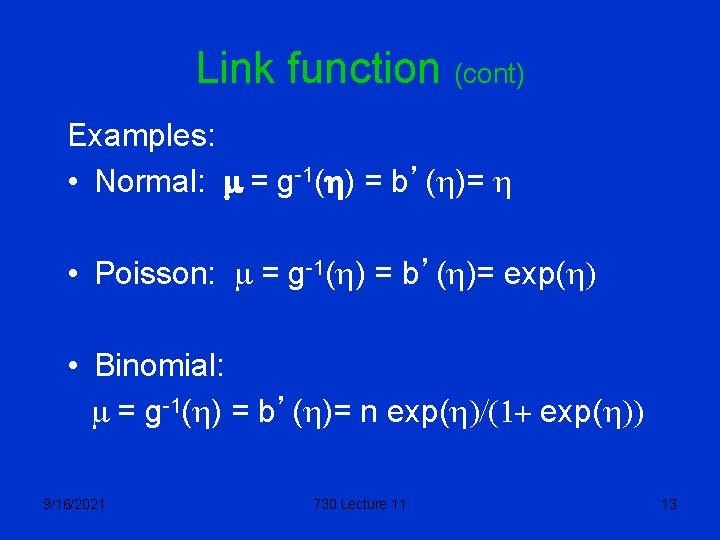
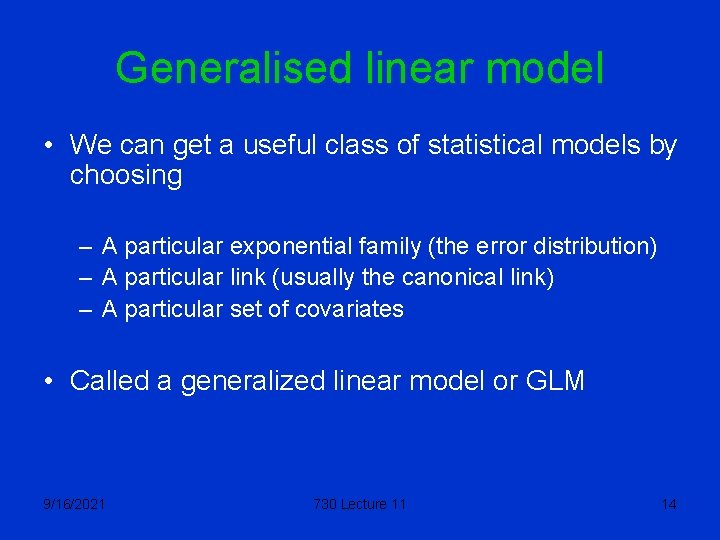
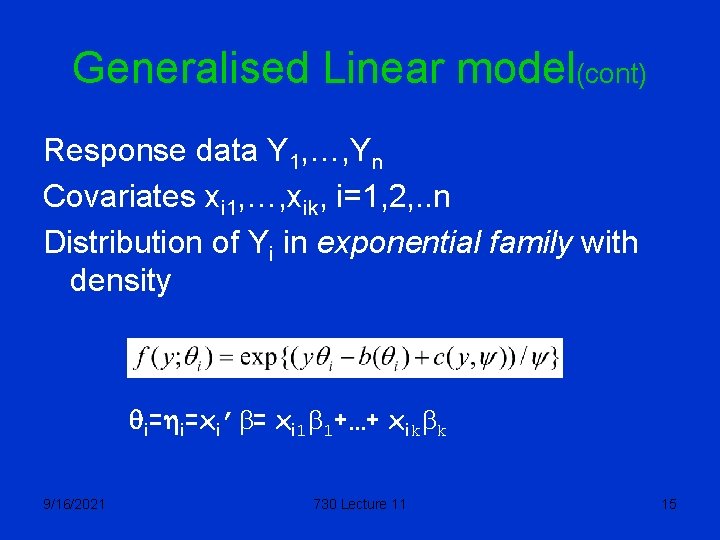
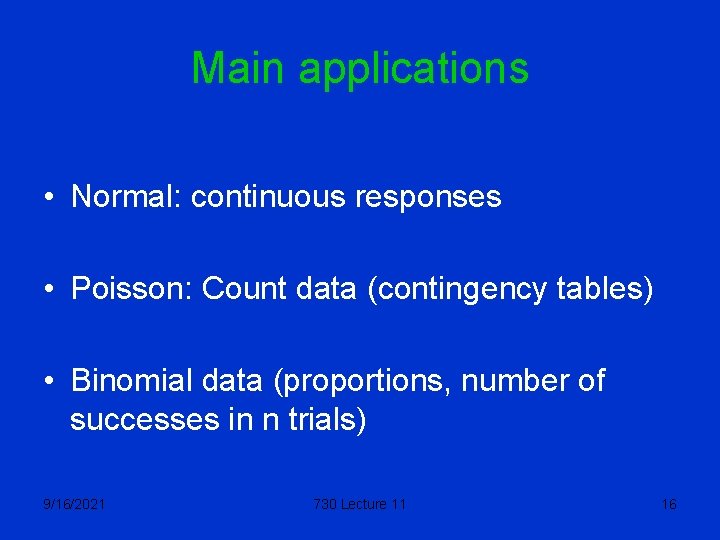
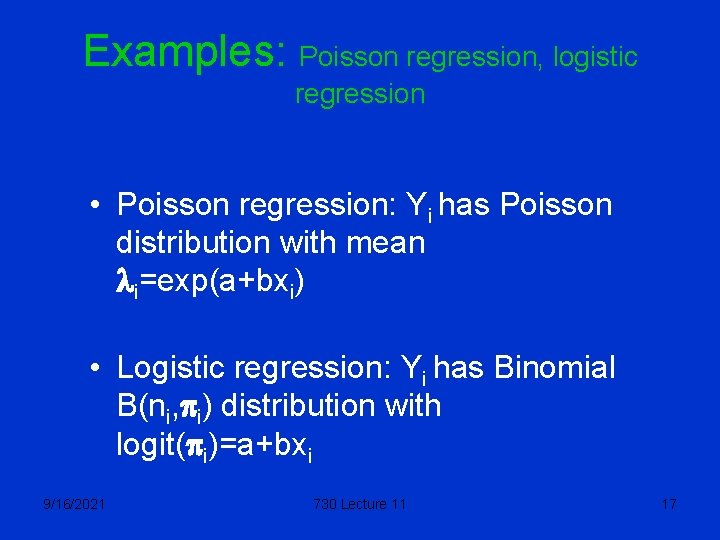
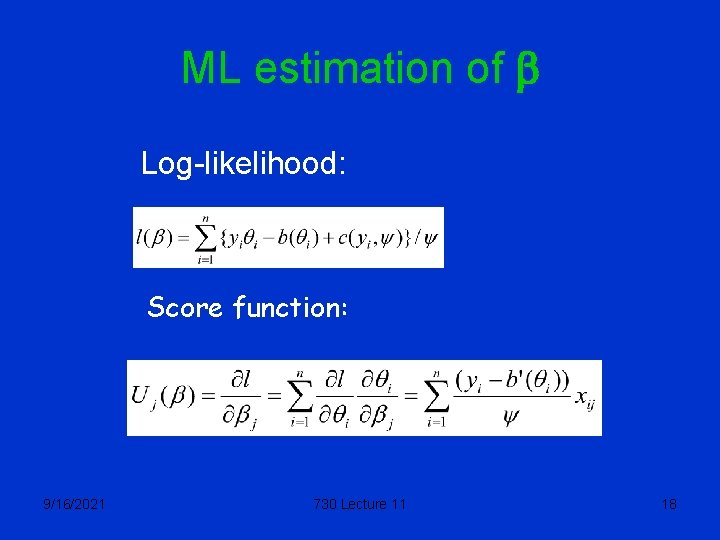
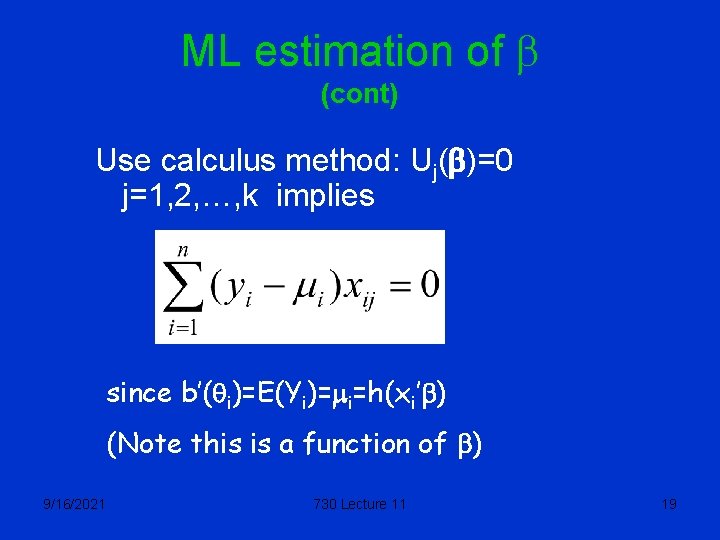
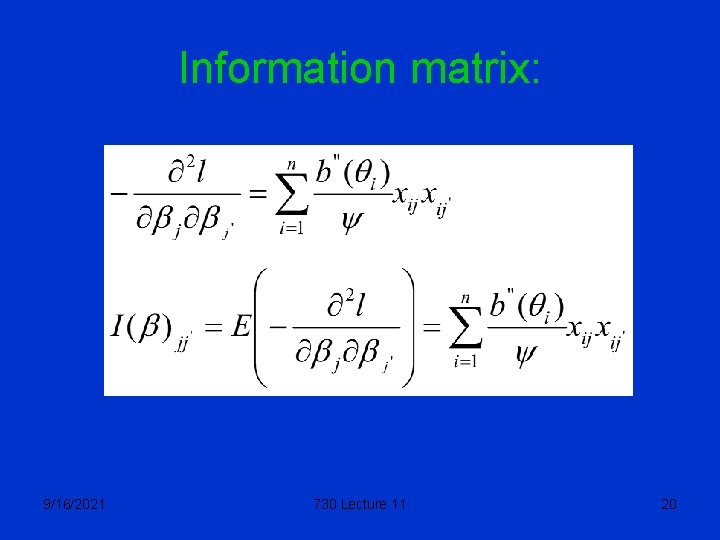
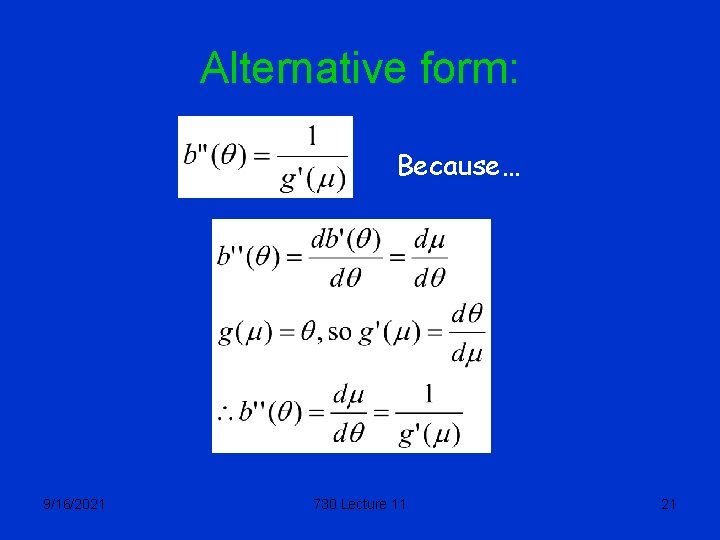
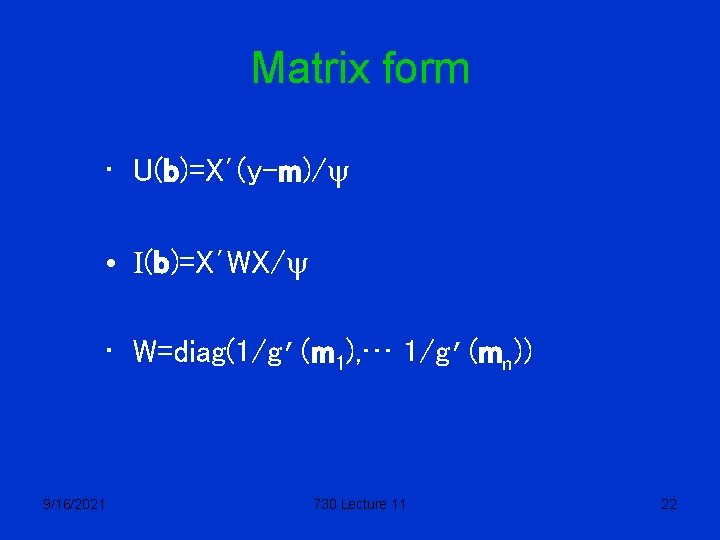
- Slides: 22
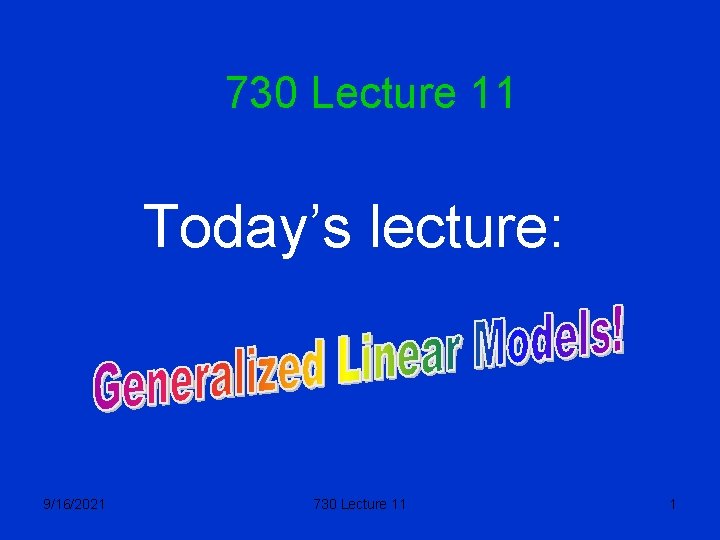
730 Lecture 11 Today’s lecture: 9/16/2021 730 Lecture 11 1
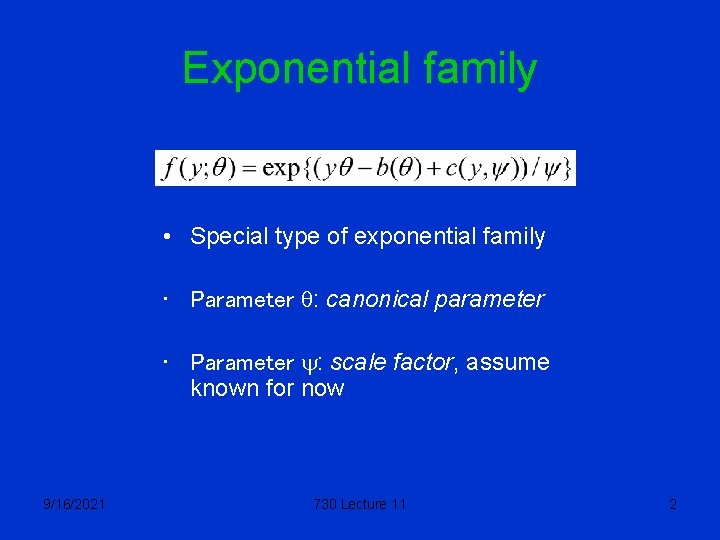
Exponential family • Special type of exponential family • Parameter q: canonical parameter • Parameter y: scale factor, assume known for now 9/16/2021 730 Lecture 11 2
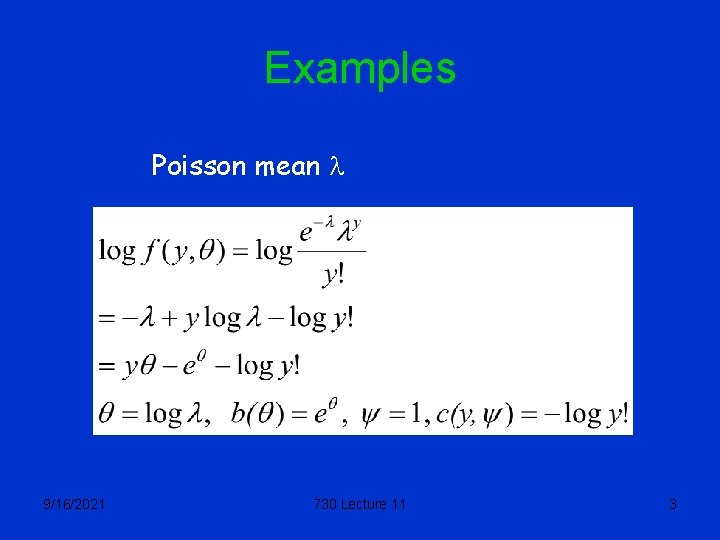
Examples Poisson mean l 9/16/2021 730 Lecture 11 3
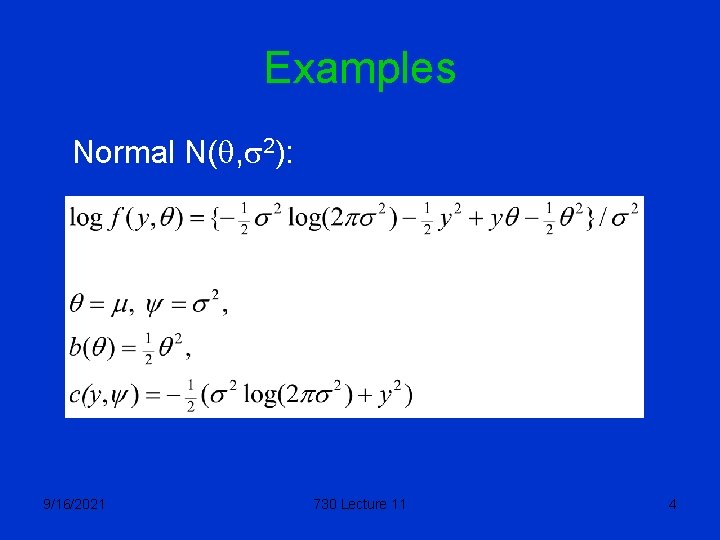
Examples Normal N(q, s 2): 9/16/2021 730 Lecture 11 4
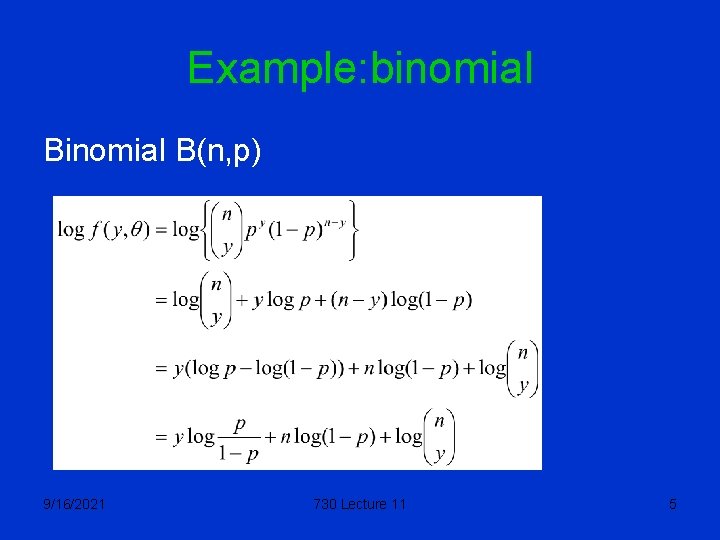
Example: binomial B(n, p) 9/16/2021 730 Lecture 11 5
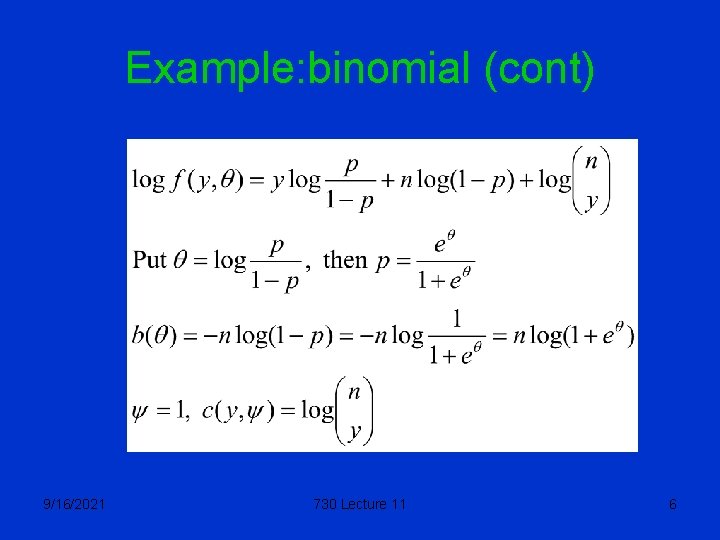
Example: binomial (cont) 9/16/2021 730 Lecture 11 6
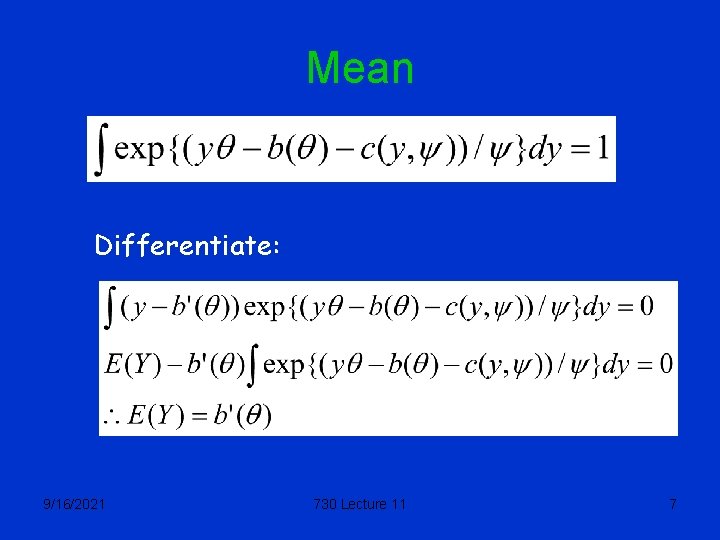
Mean Differentiate: 9/16/2021 730 Lecture 11 7
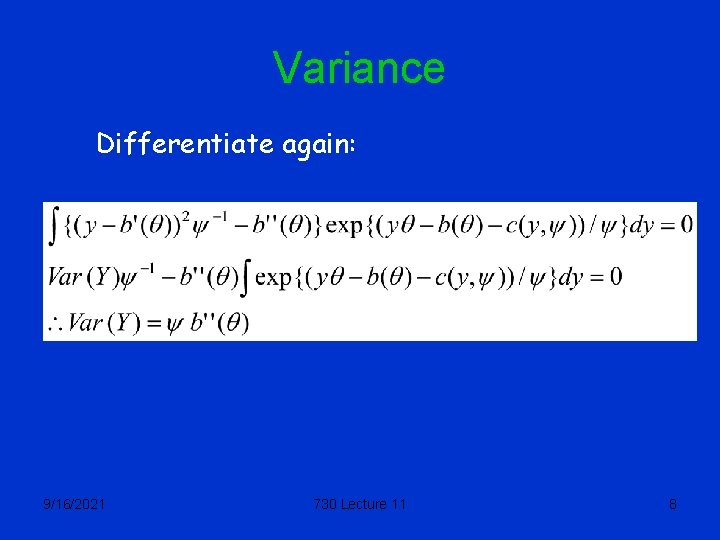
Variance Differentiate again: 9/16/2021 730 Lecture 11 8
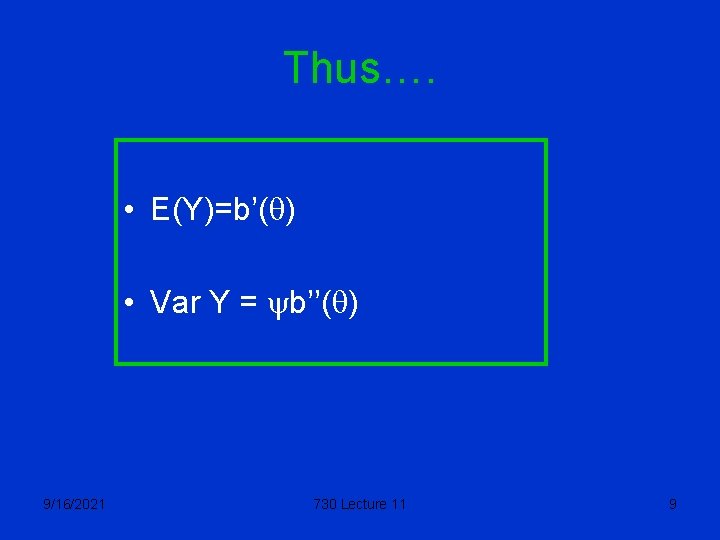
Thus…. • E(Y)=b’(q) • Var Y = yb’’(q) 9/16/2021 730 Lecture 11 9
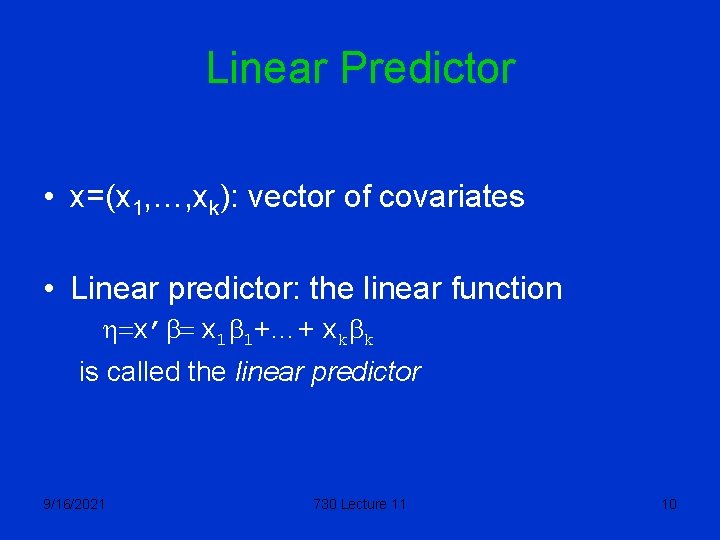
Linear Predictor • x=(x 1, …, xk): vector of covariates • Linear predictor: the linear function h=x’b= x 1 b 1+…+ xkbk is called the linear predictor 9/16/2021 730 Lecture 11 10
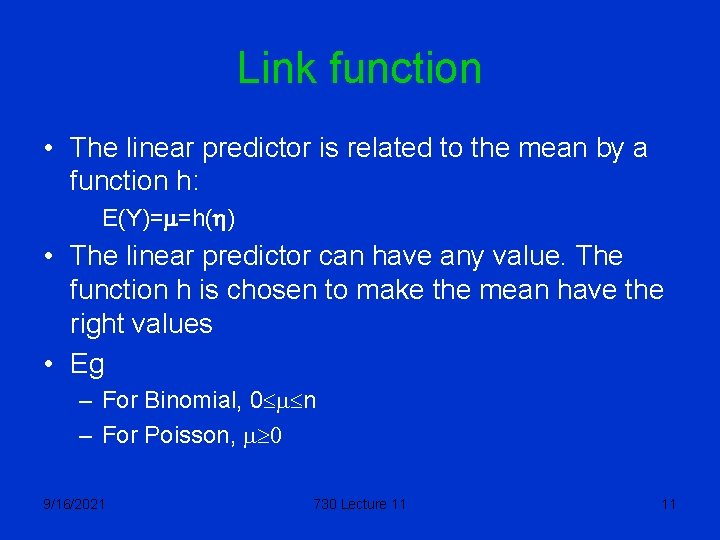
Link function • The linear predictor is related to the mean by a function h: E(Y)=m=h(h) • The linear predictor can have any value. The function h is chosen to make the mean have the right values • Eg – For Binomial, 0£m£n – For Poisson, m³ 0 9/16/2021 730 Lecture 11 11
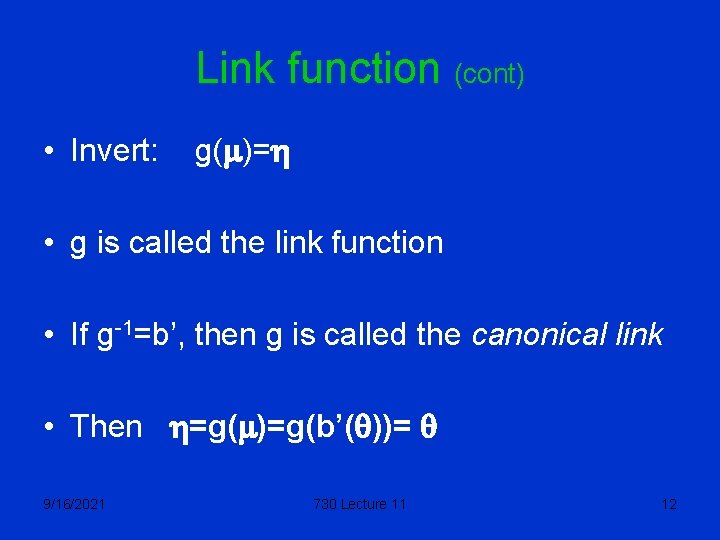
Link function (cont) • Invert: g(m)=h • g is called the link function • If g-1=b’, then g is called the canonical link • Then h=g(m)=g(b’(q))= q 9/16/2021 730 Lecture 11 12
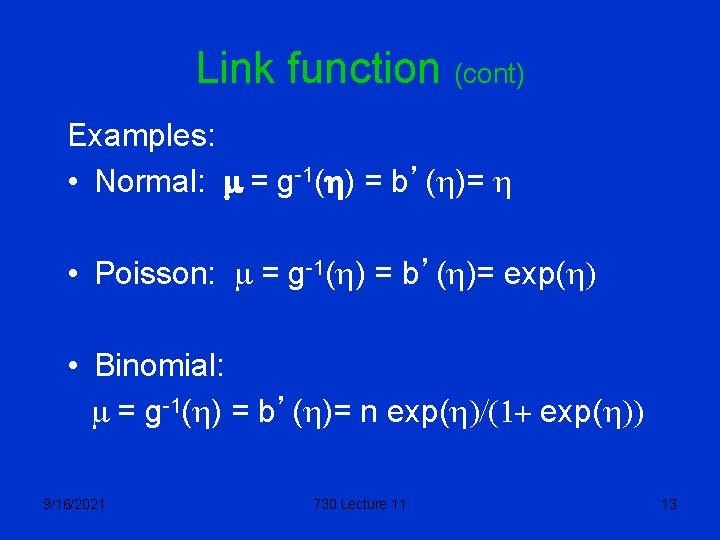
Link function (cont) Examples: • Normal: m = g-1(h) = b’(h)= h • Poisson: m = g-1(h) = b’(h)= exp(h) • Binomial: m = g-1(h) = b’(h)= n exp(h)/(1+ exp(h)) 9/16/2021 730 Lecture 11 13
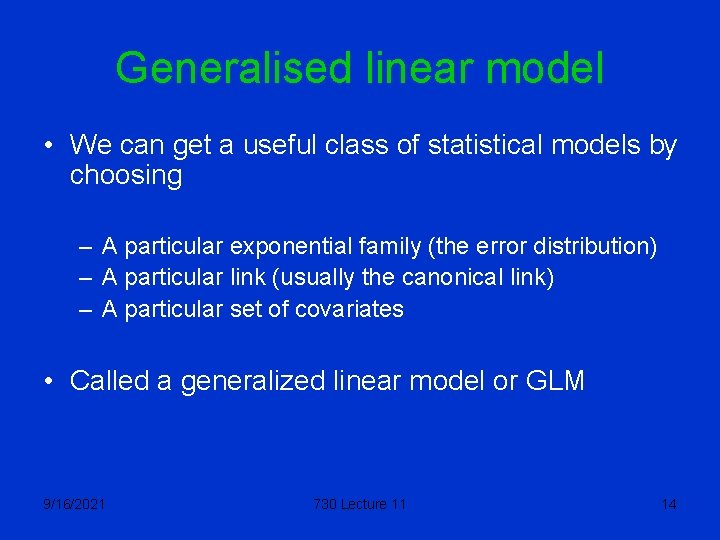
Generalised linear model • We can get a useful class of statistical models by choosing – A particular exponential family (the error distribution) – A particular link (usually the canonical link) – A particular set of covariates • Called a generalized linear model or GLM 9/16/2021 730 Lecture 11 14
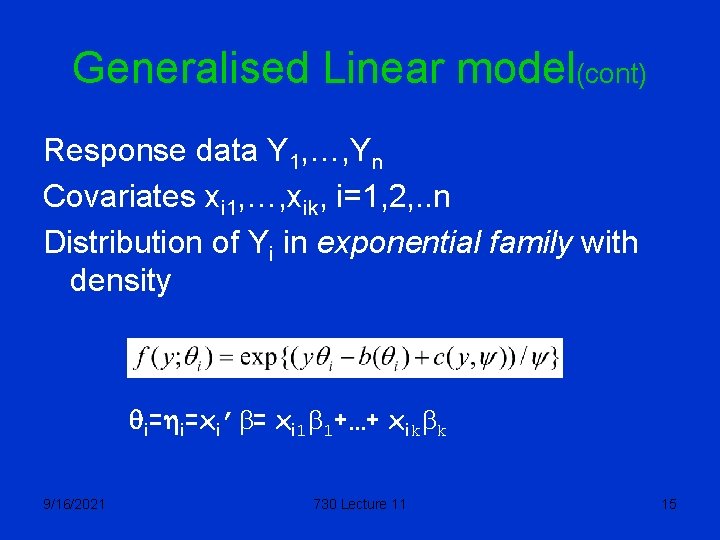
Generalised Linear model(cont) Response data Y 1, …, Yn Covariates xi 1, …, xik, i=1, 2, . . n Distribution of Yi in exponential family with density qi=hi=xi’b= xi 1 b 1+…+ xikbk 9/16/2021 730 Lecture 11 15
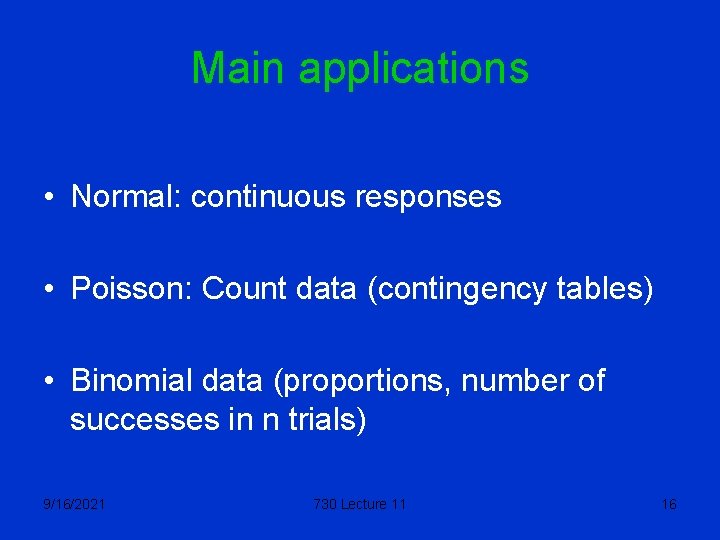
Main applications • Normal: continuous responses • Poisson: Count data (contingency tables) • Binomial data (proportions, number of successes in n trials) 9/16/2021 730 Lecture 11 16
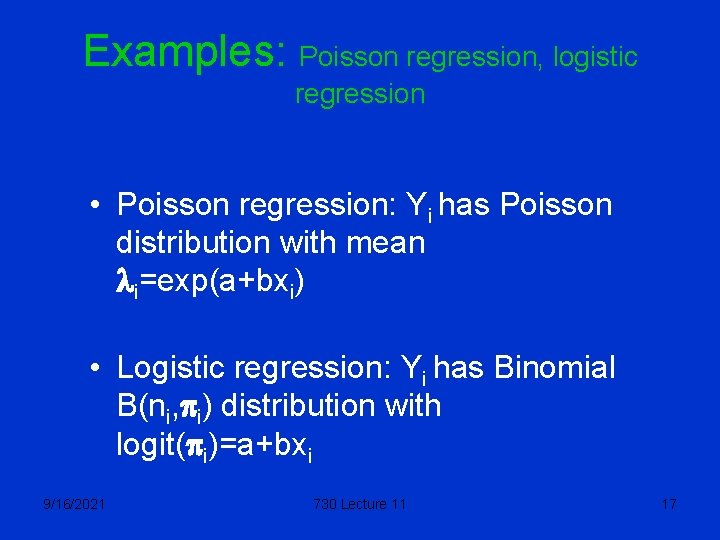
Examples: Poisson regression, logistic regression • Poisson regression: Yi has Poisson distribution with mean li=exp(a+bxi) • Logistic regression: Yi has Binomial B(ni, pi) distribution with logit(pi)=a+bxi 9/16/2021 730 Lecture 11 17
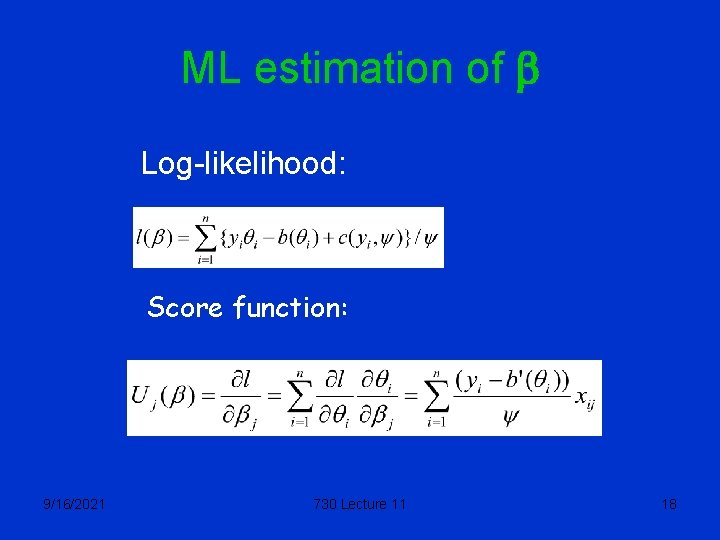
ML estimation of b Log-likelihood: Score function: 9/16/2021 730 Lecture 11 18
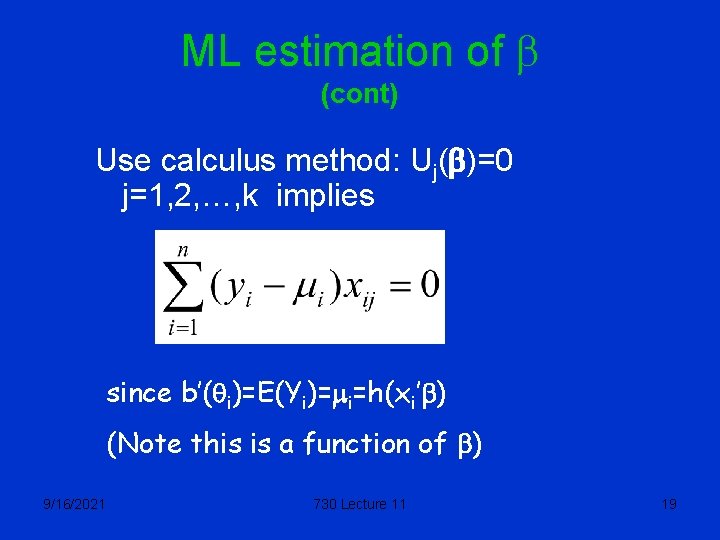
ML estimation of b (cont) Use calculus method: Uj(b)=0 j=1, 2, …, k implies since b’(qi)=E(Yi)=mi=h(xi’b) (Note this is a function of b) 9/16/2021 730 Lecture 11 19
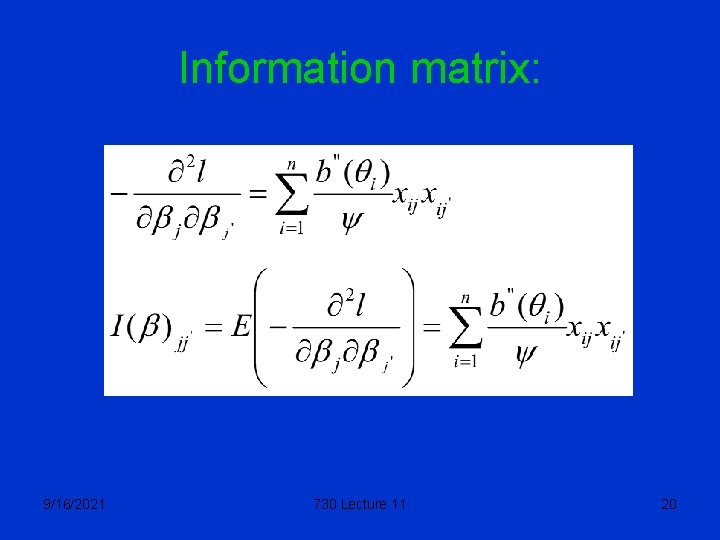
Information matrix: 9/16/2021 730 Lecture 11 20
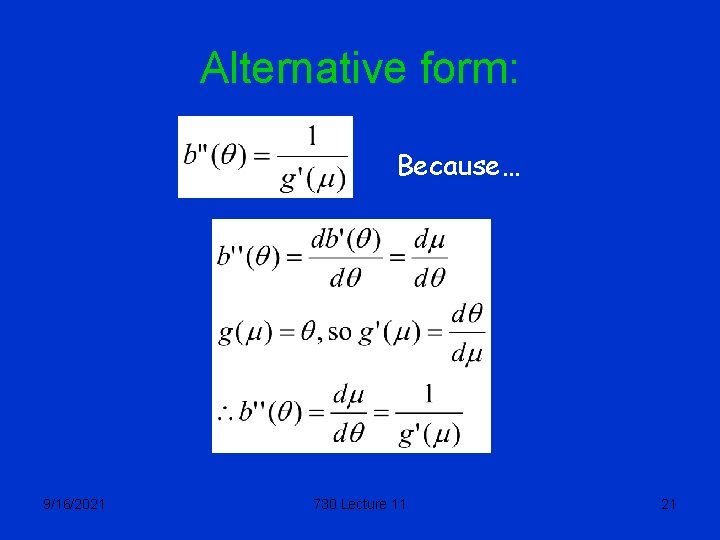
Alternative form: Because… 9/16/2021 730 Lecture 11 21
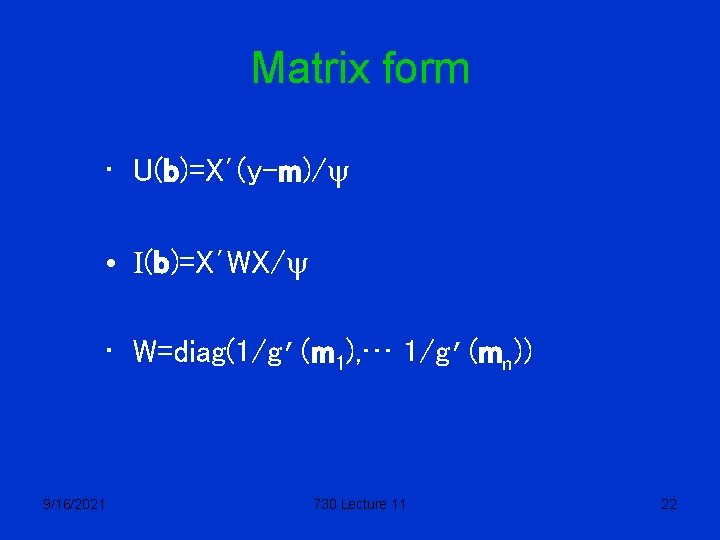
Matrix form • U(b)=X’(y-m)/y • I(b)=X’WX/y • W=diag(1/g’(m 1), … 1/g’(mn)) 9/16/2021 730 Lecture 11 22
Ieee 730
170 f a c
Sps 730
Arlon 25fr
400 en yakın onluğa yuvarlama
Ars 28-730
Welcome to today's class
Good morning class! how are you today?
Todays plan
Todays weather hull
Todays objective
Are you going to class today
Todays whether
Todays sabbath lesson
Whats todays temperature
Todays objective
Todays class
Sample of objective in resume
Mla first page example
Todays globl
Todays objective
Objective of cyberbullying
Bo jackson stutter