Spare Parts Management Forecasting Research and Extensions John
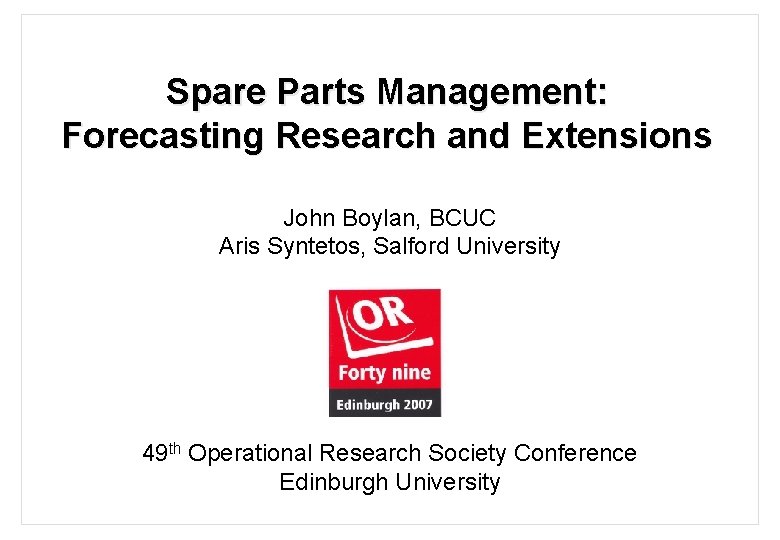
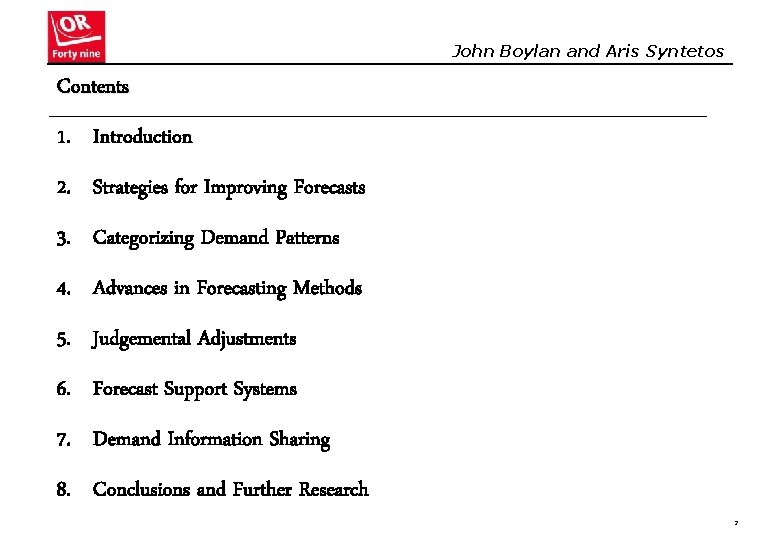
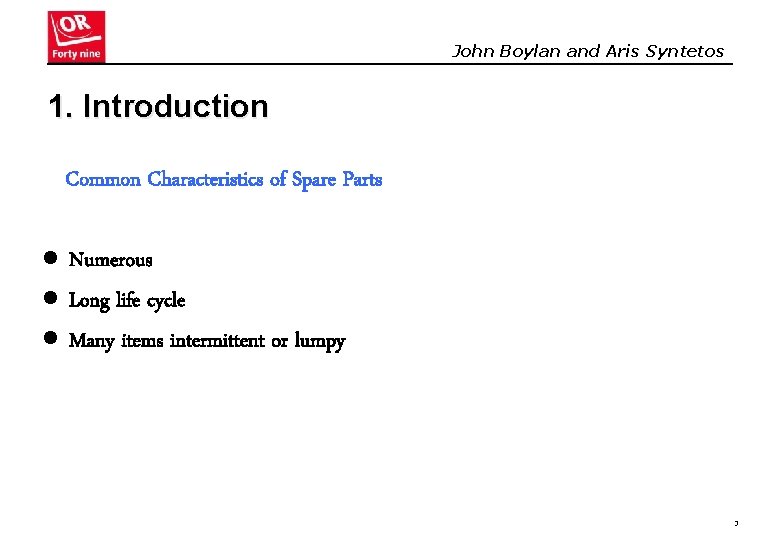
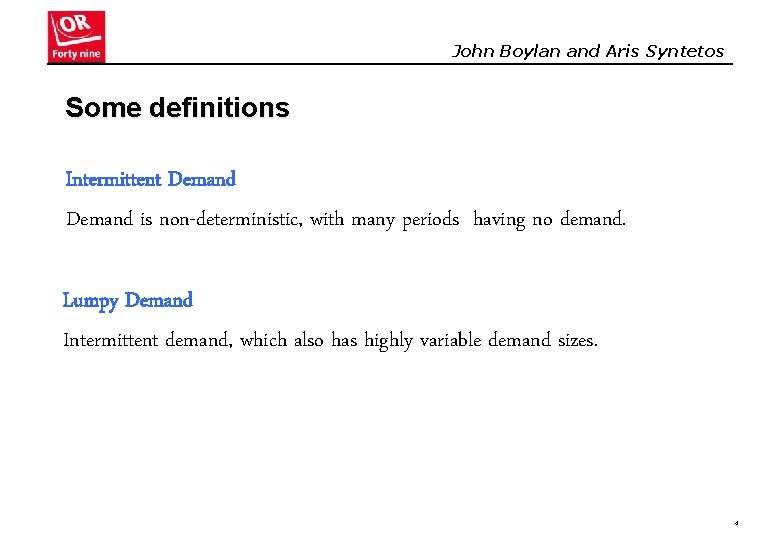
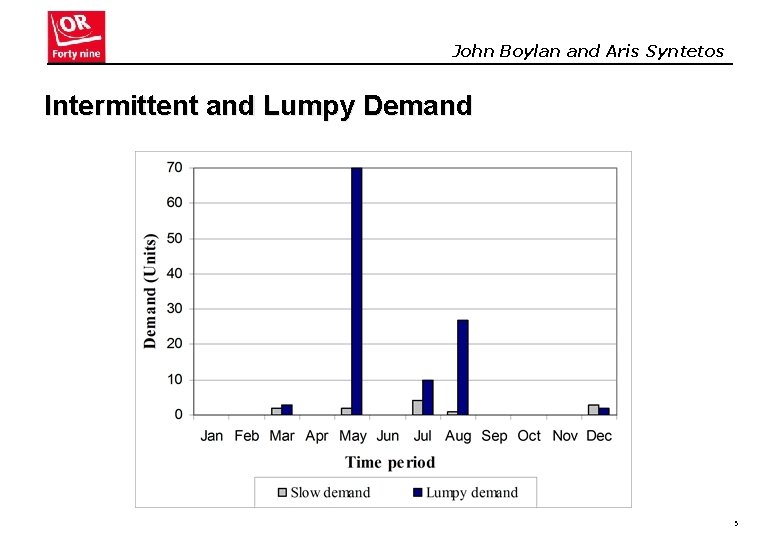
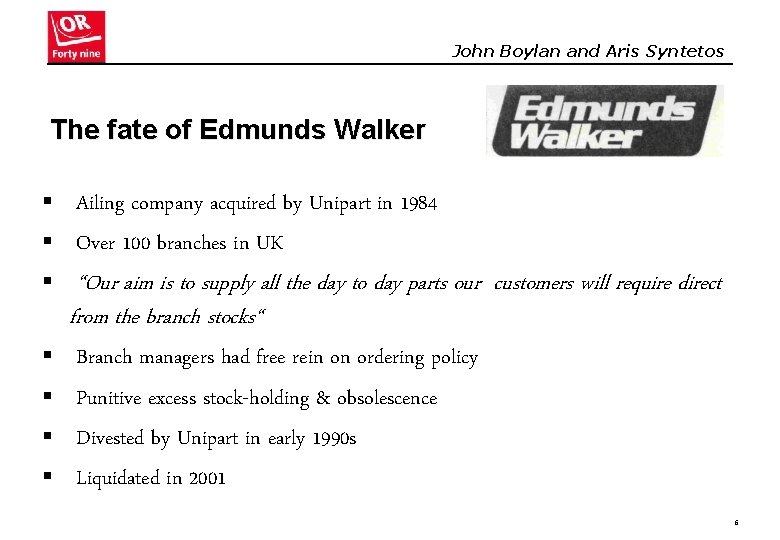
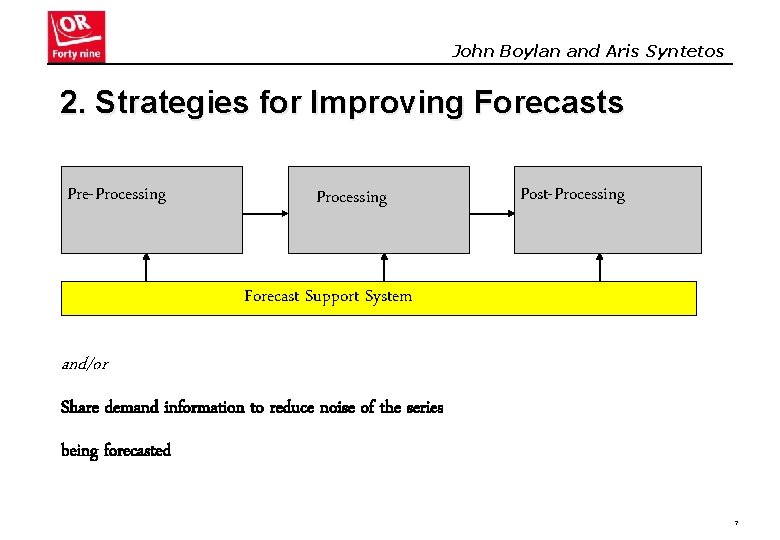
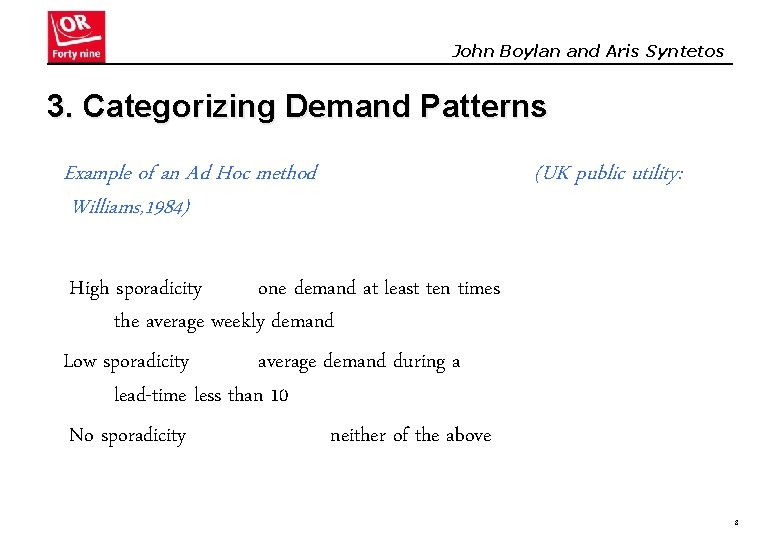
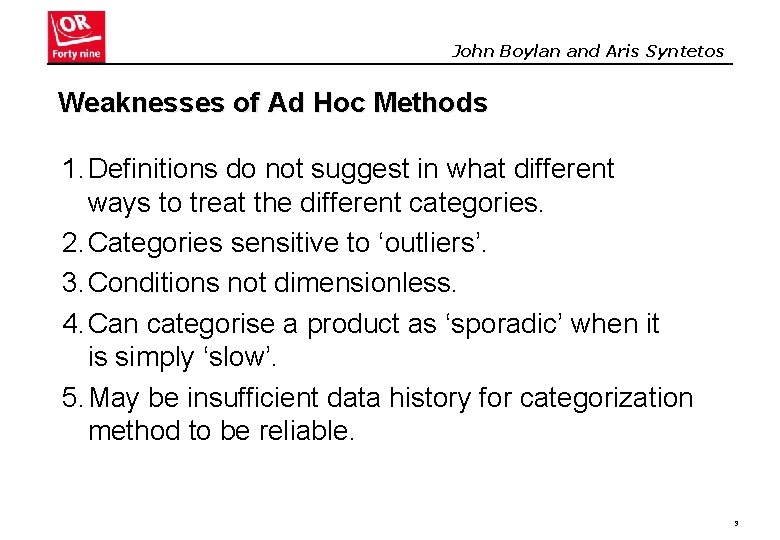
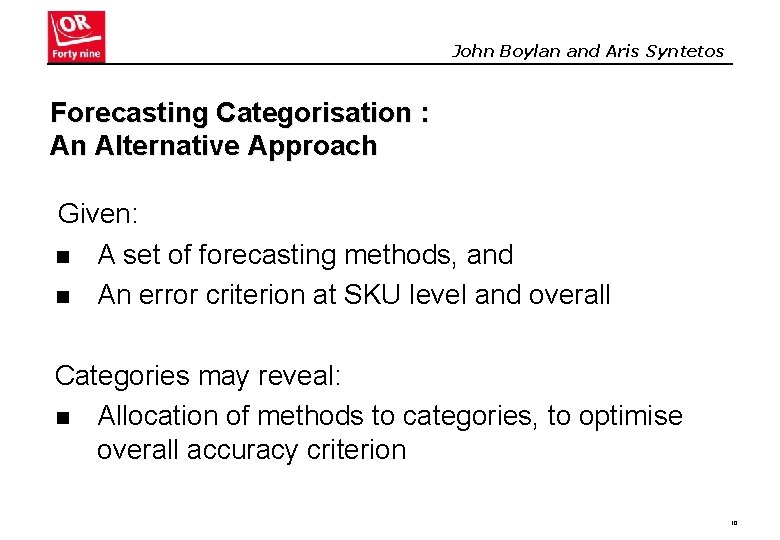
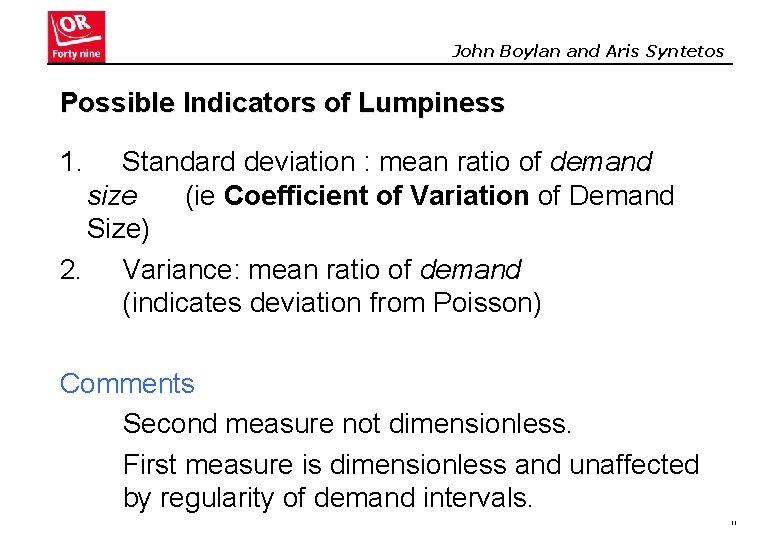
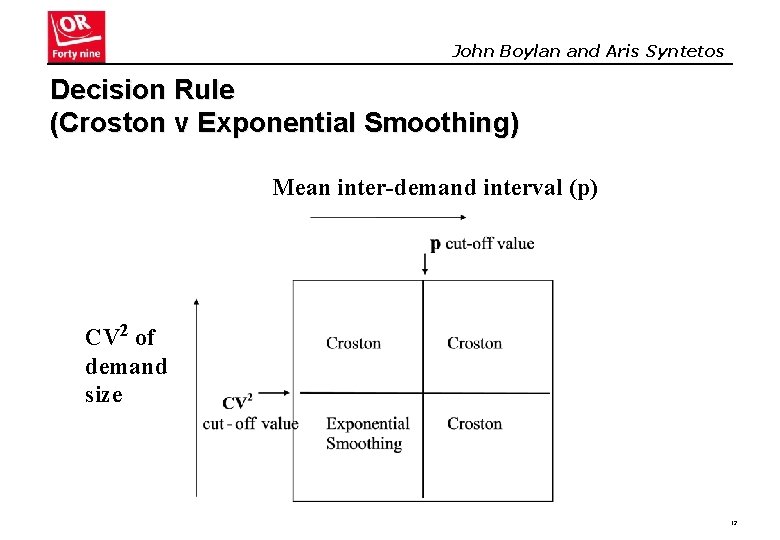
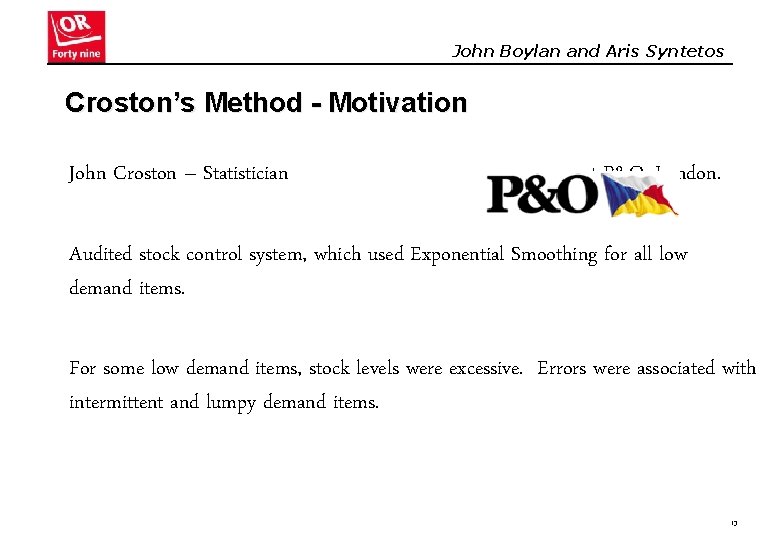
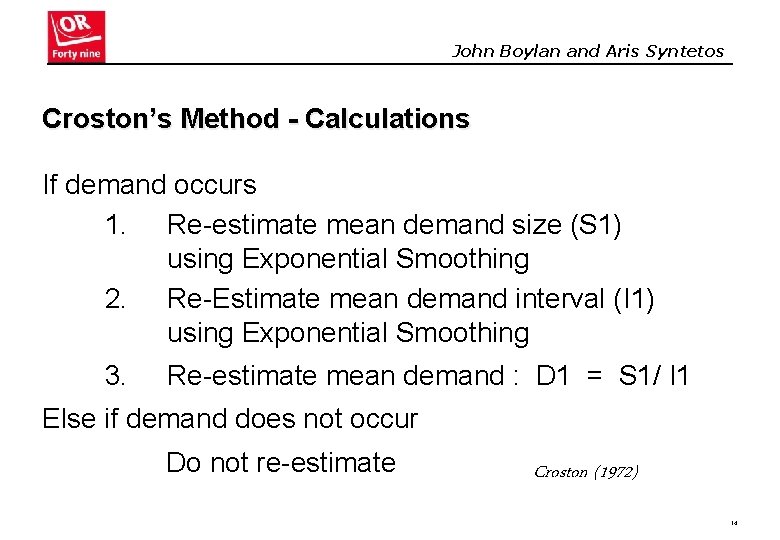
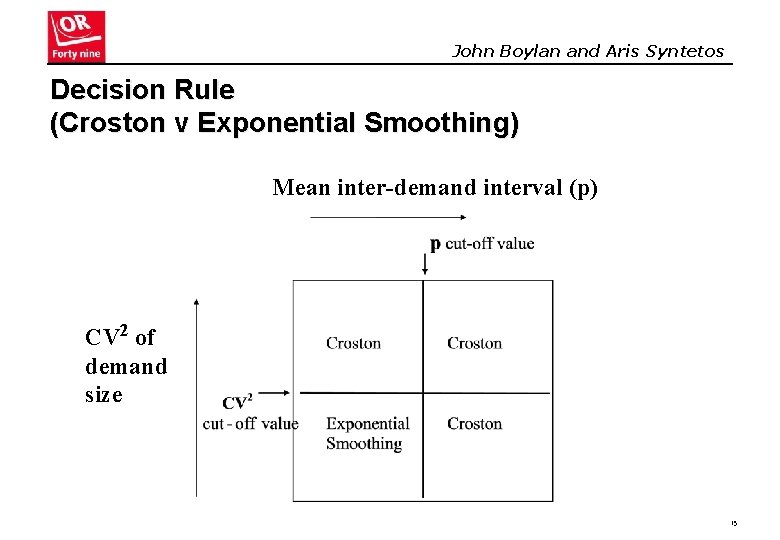
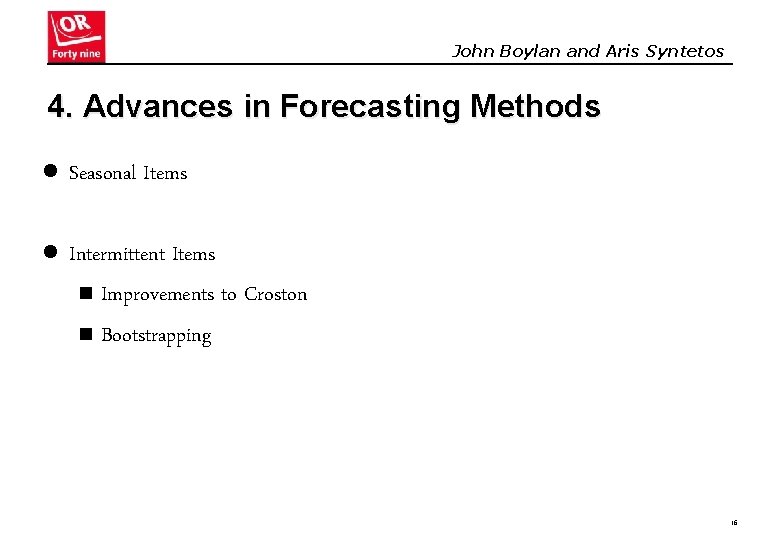
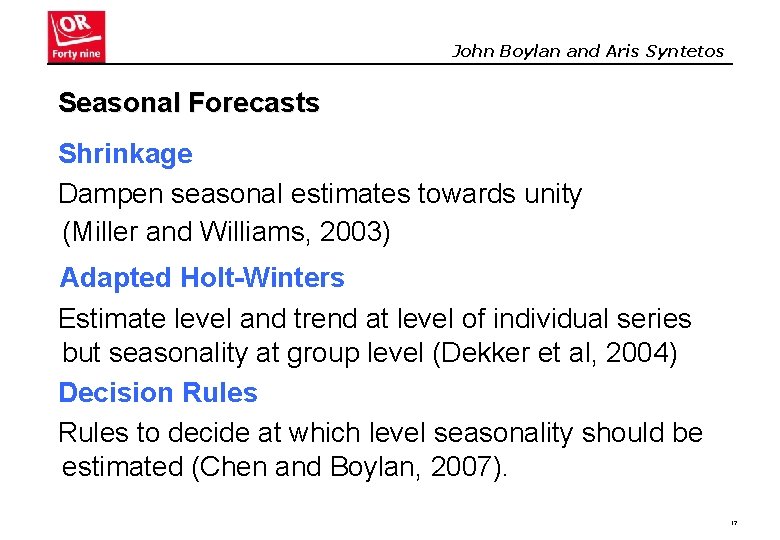
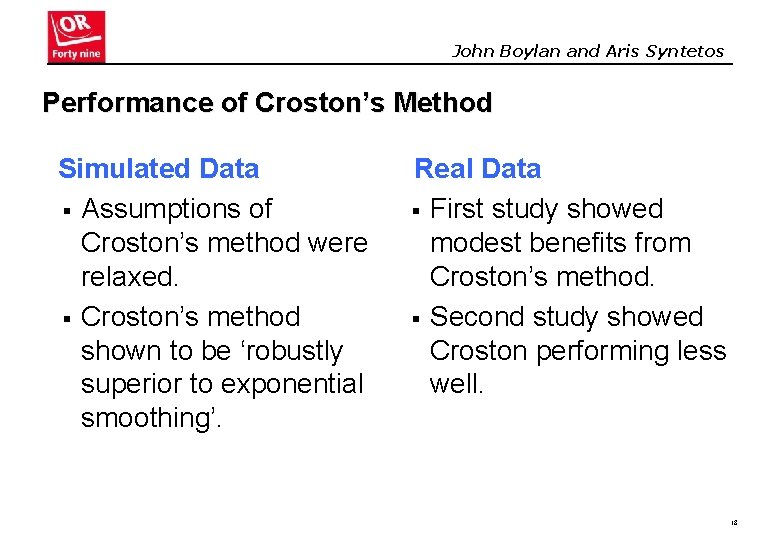
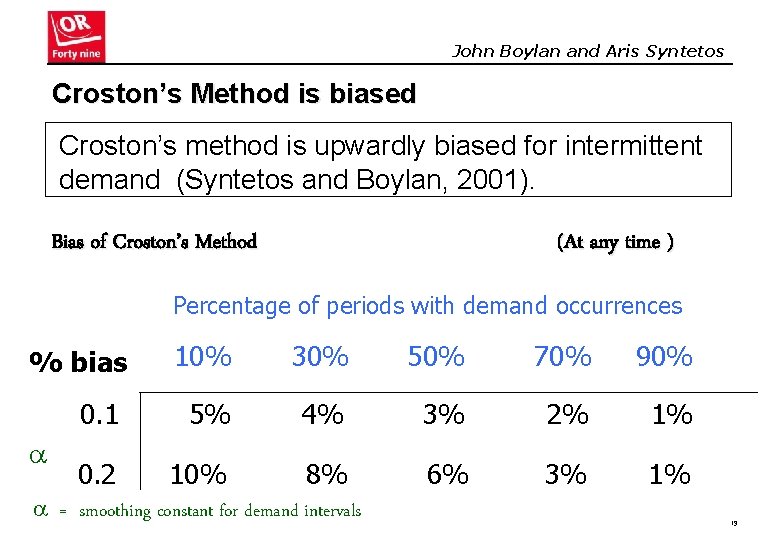
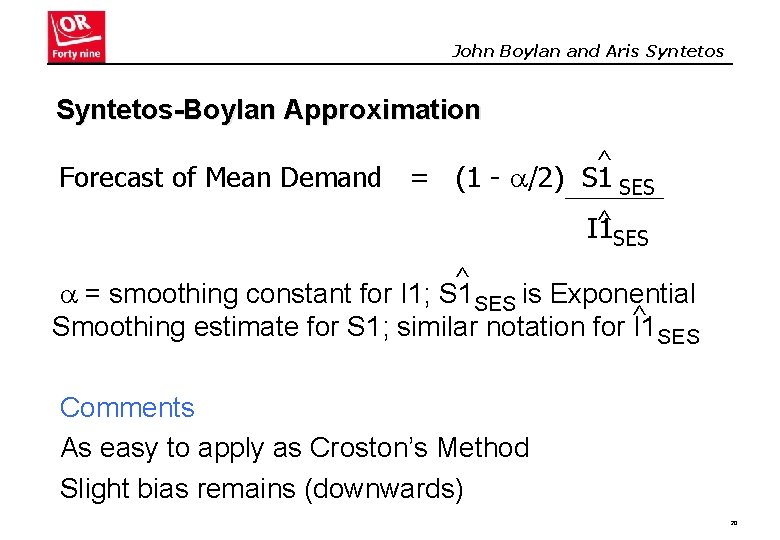
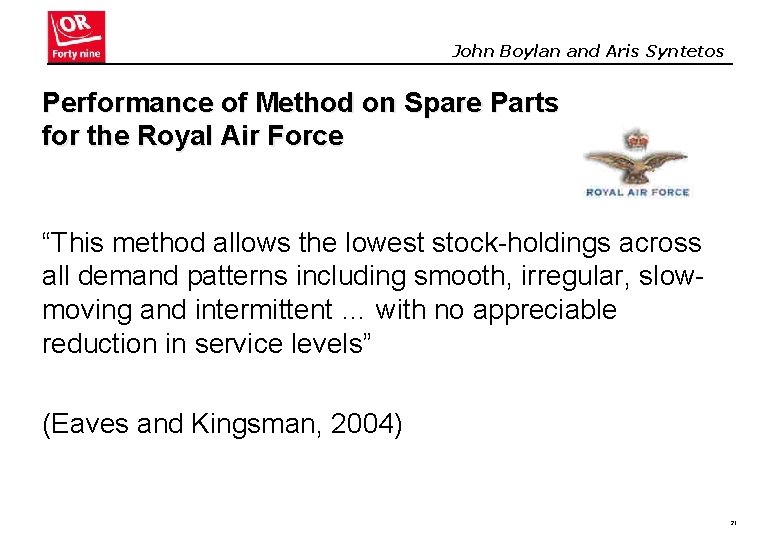
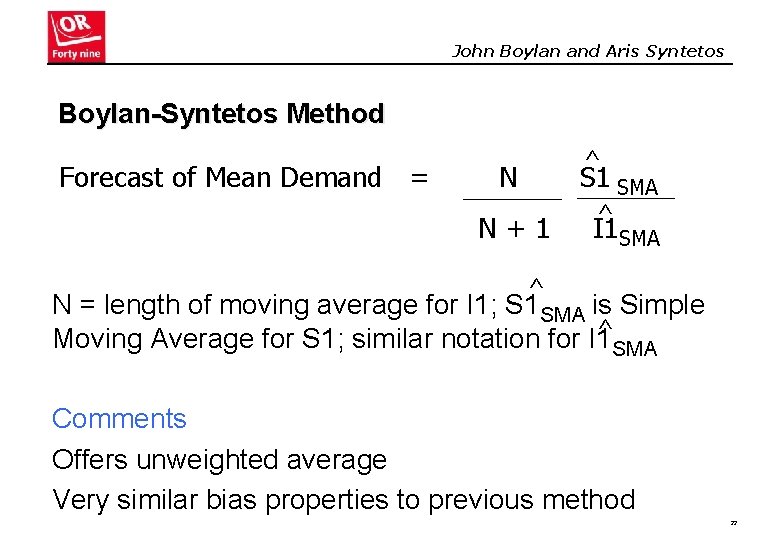
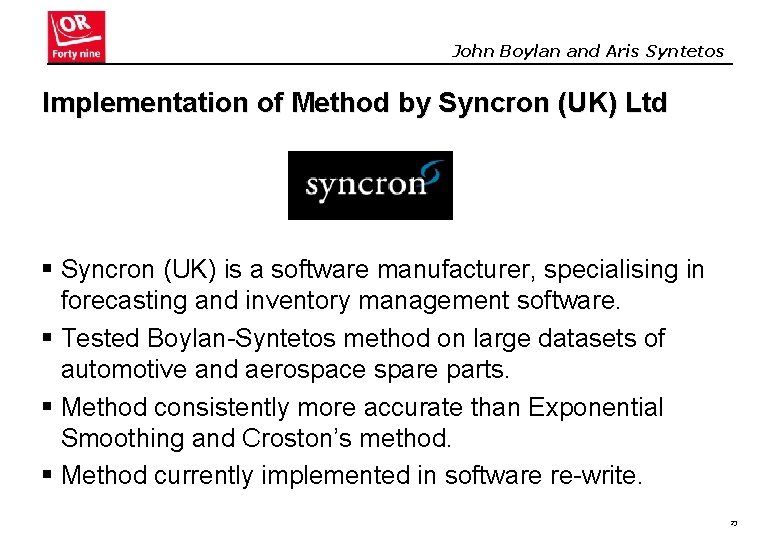
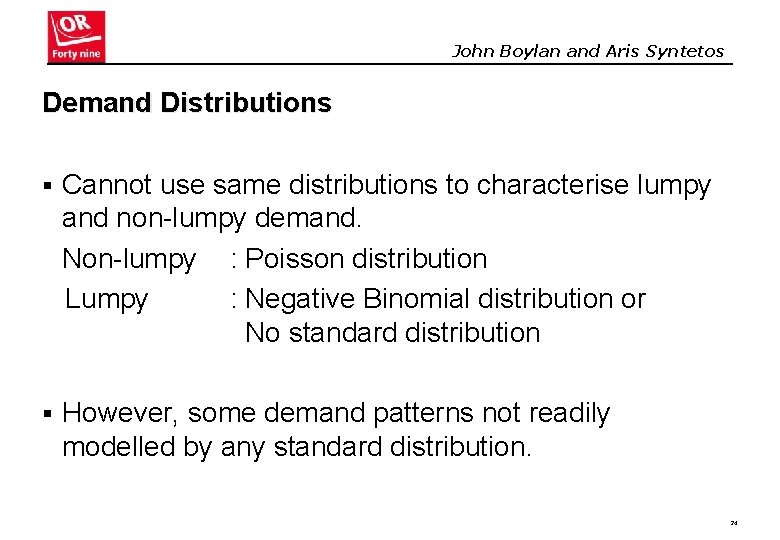
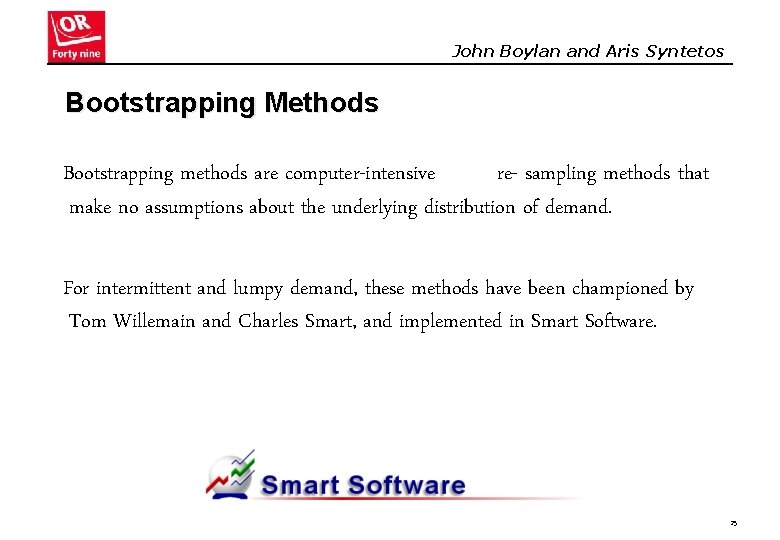
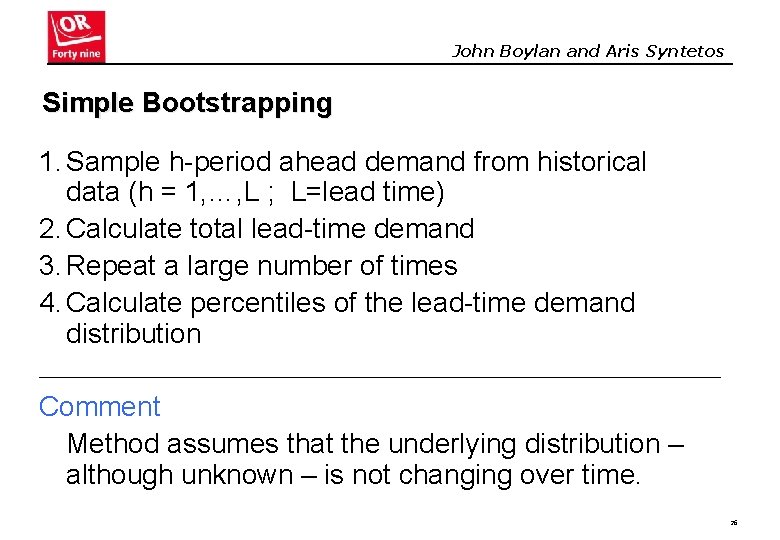
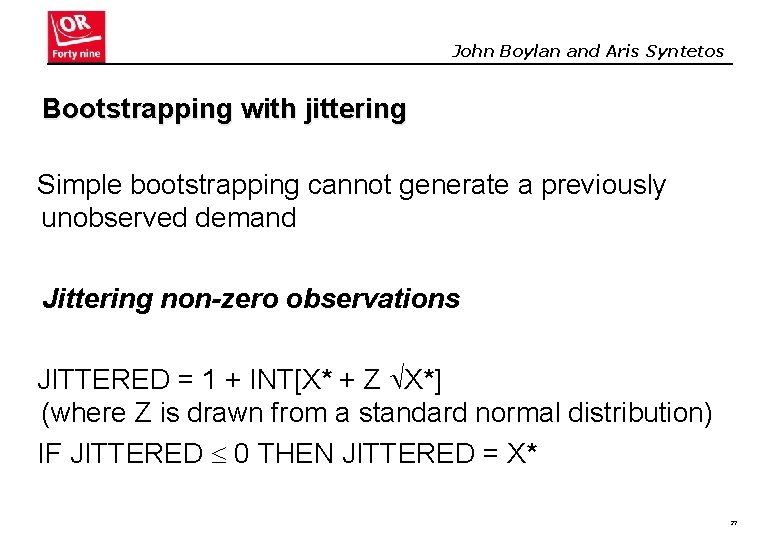
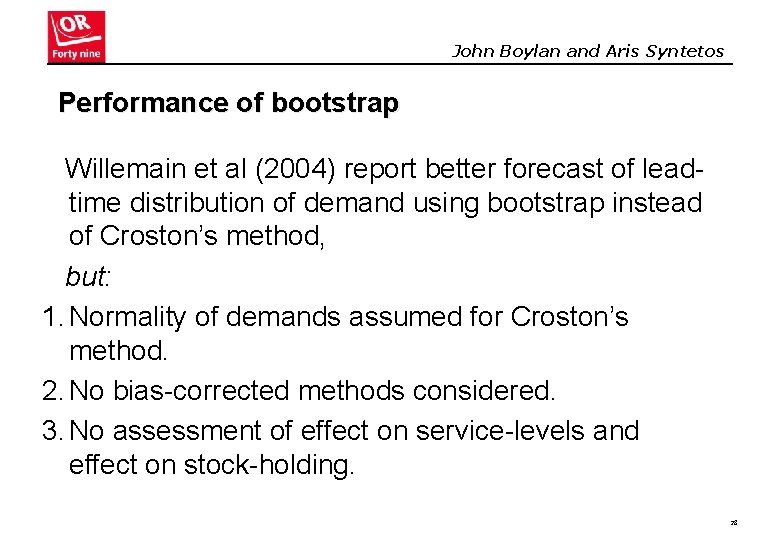
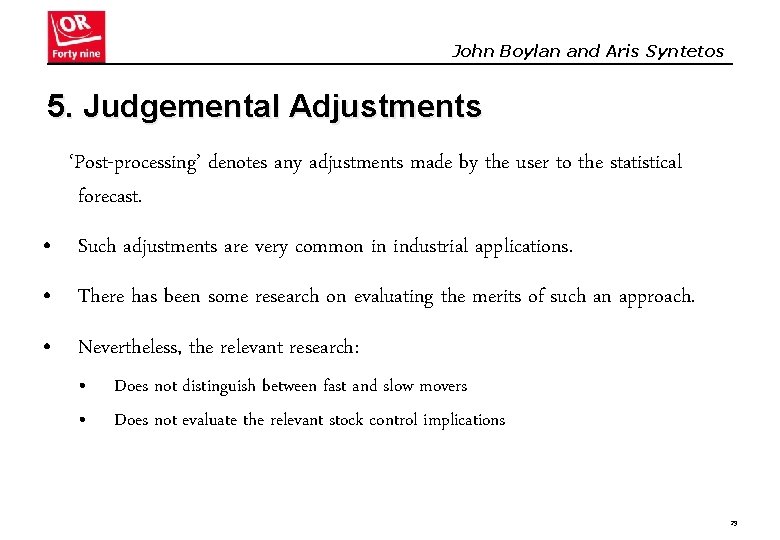
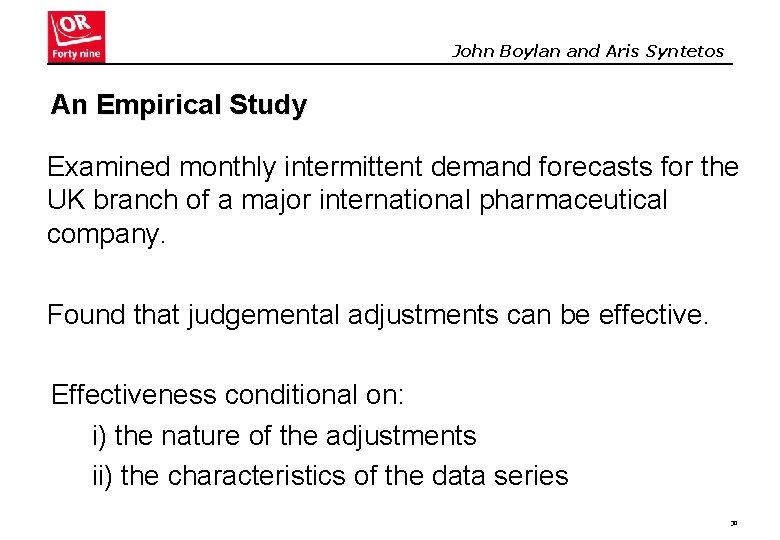
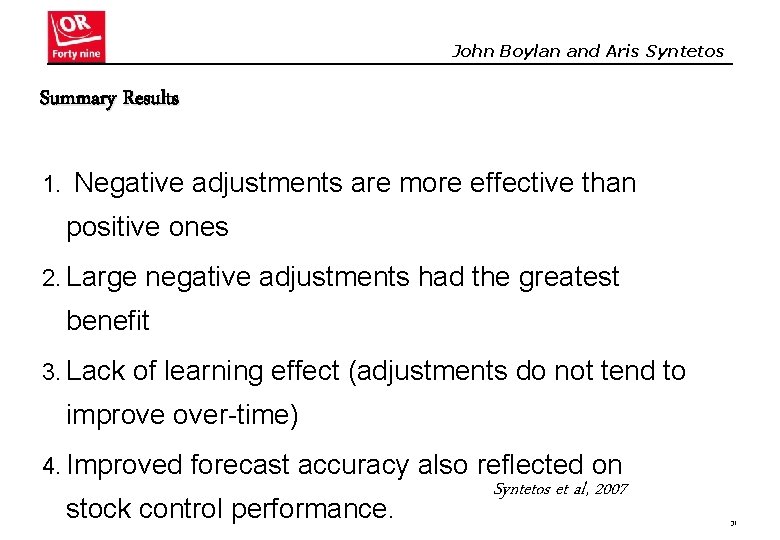
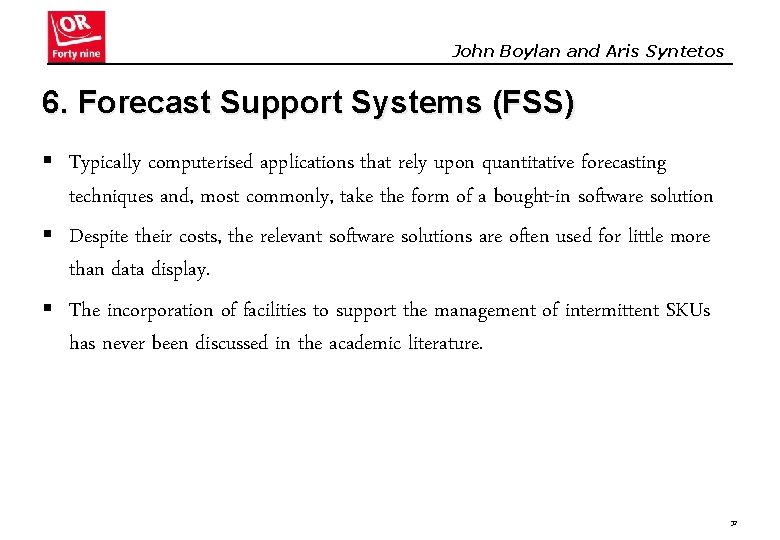
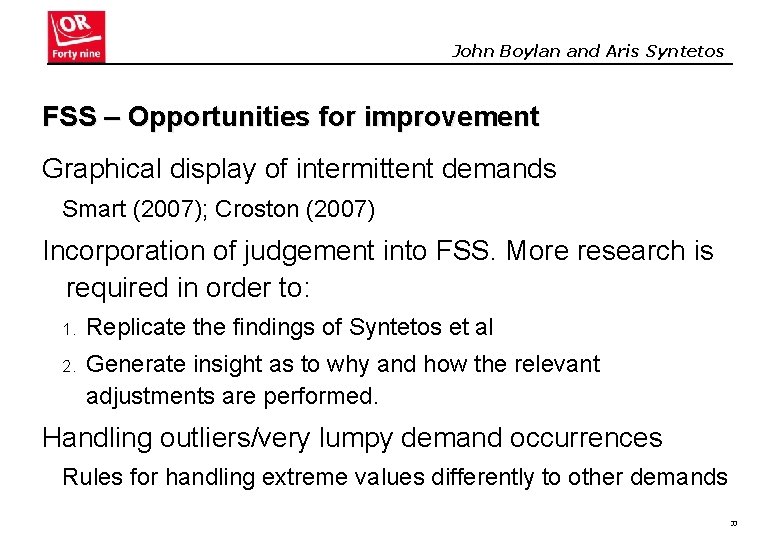
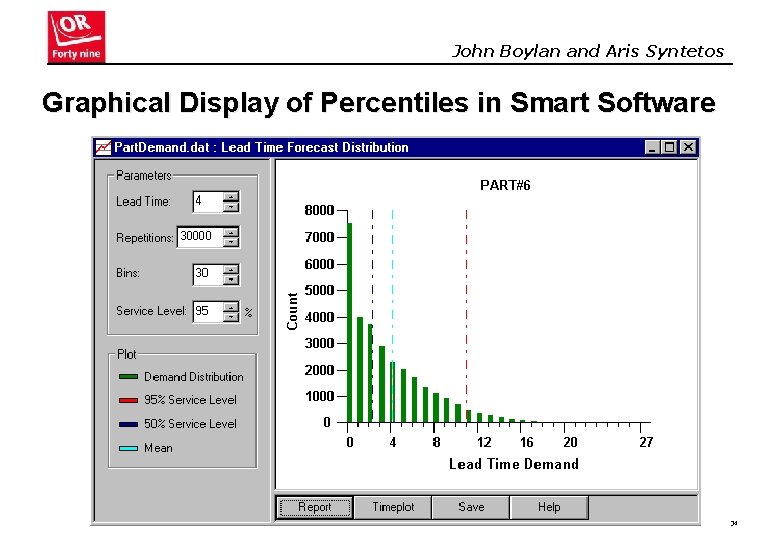
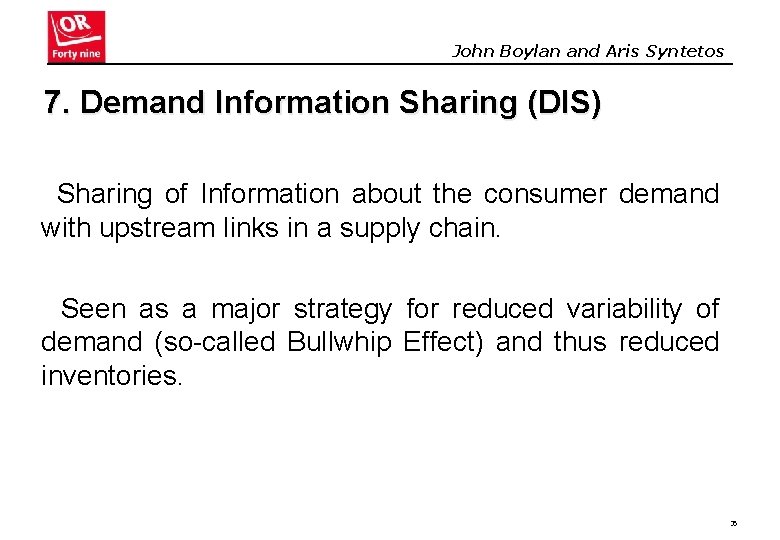
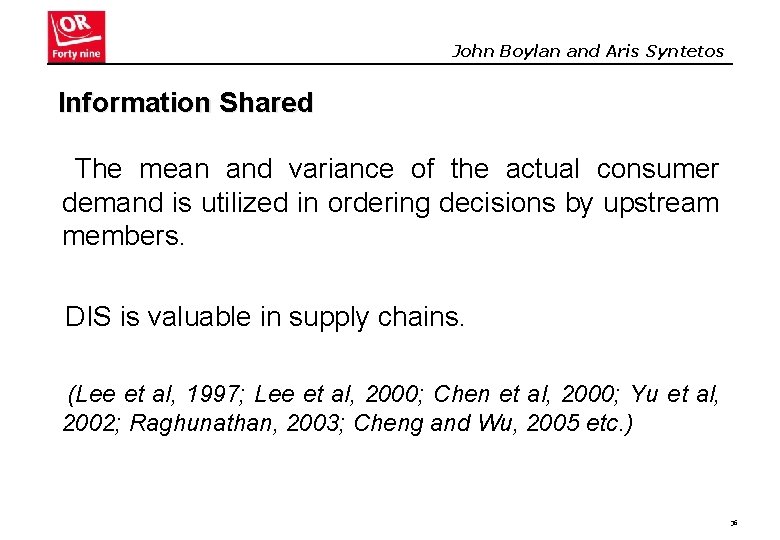
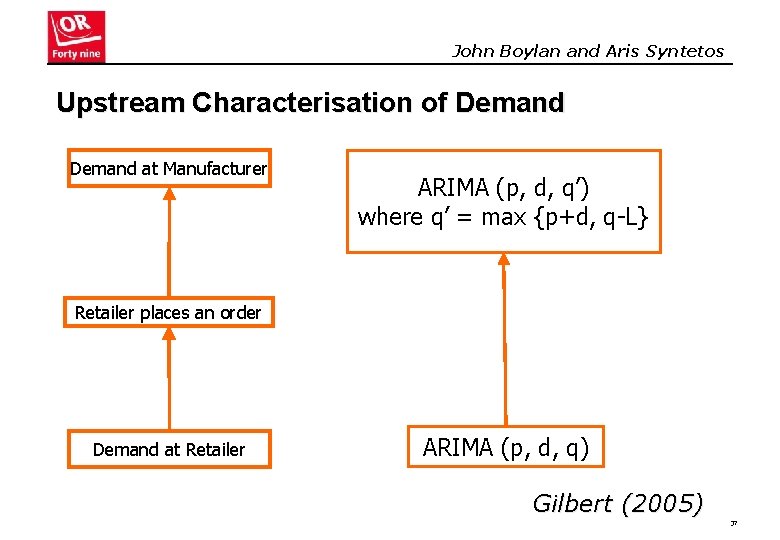
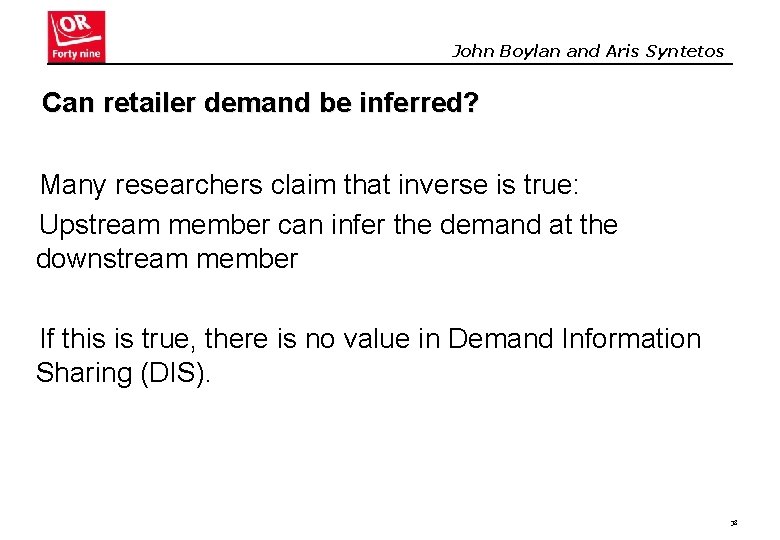
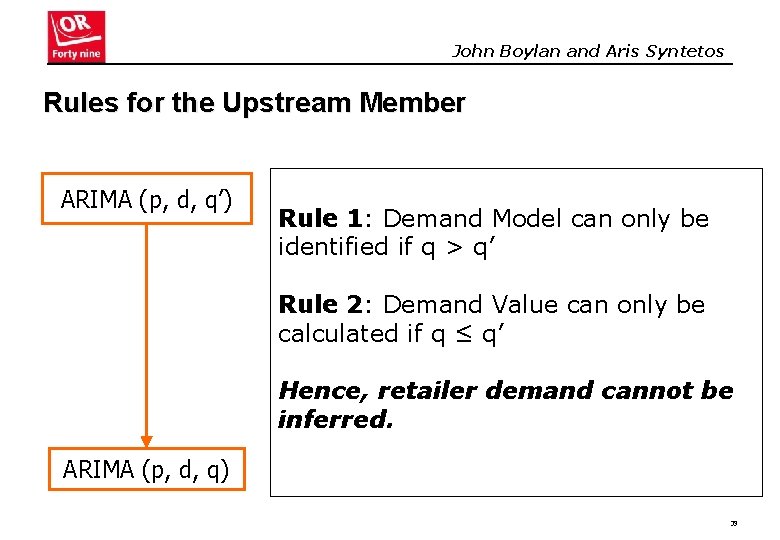
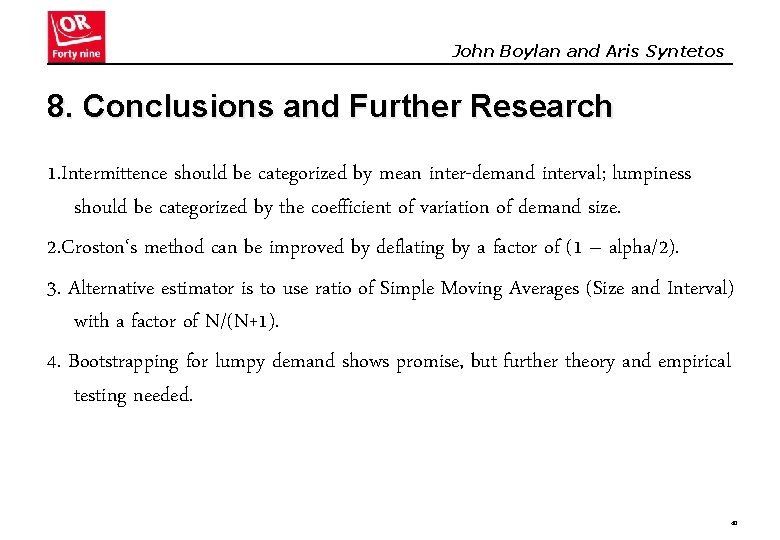
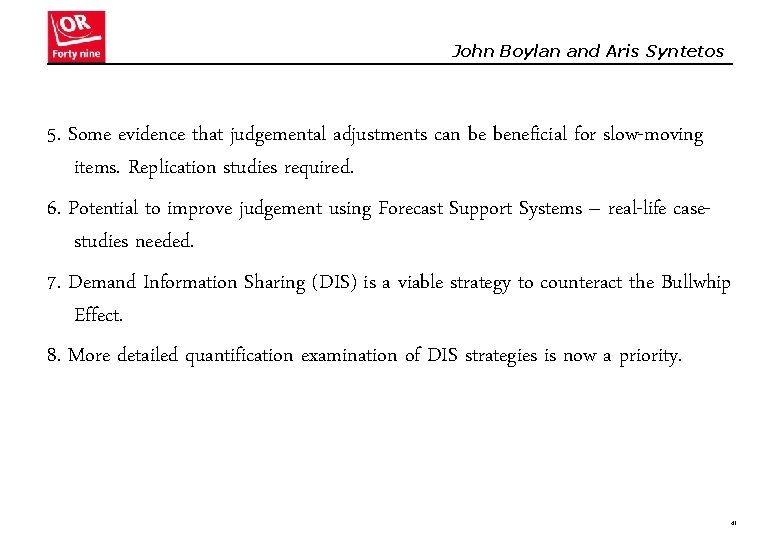
- Slides: 41
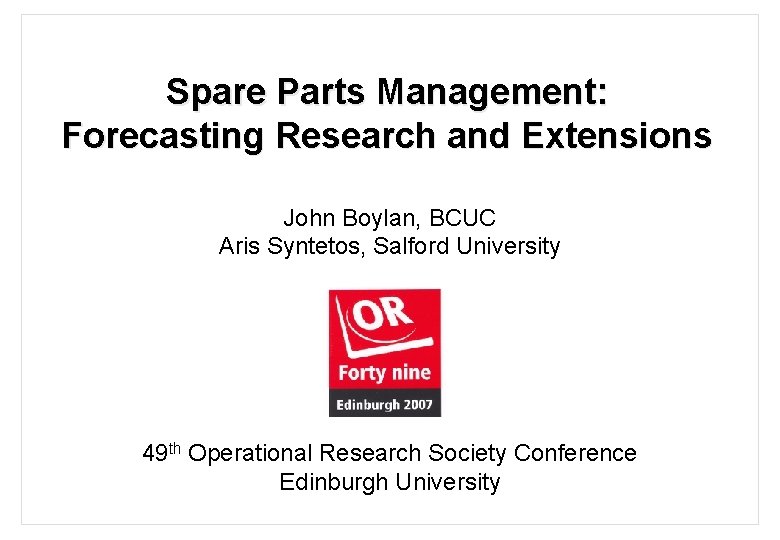
Spare Parts Management: Forecasting Research and Extensions John Boylan, BCUC Aris Syntetos, Salford University 49 th Operational Research Society Conference Edinburgh University
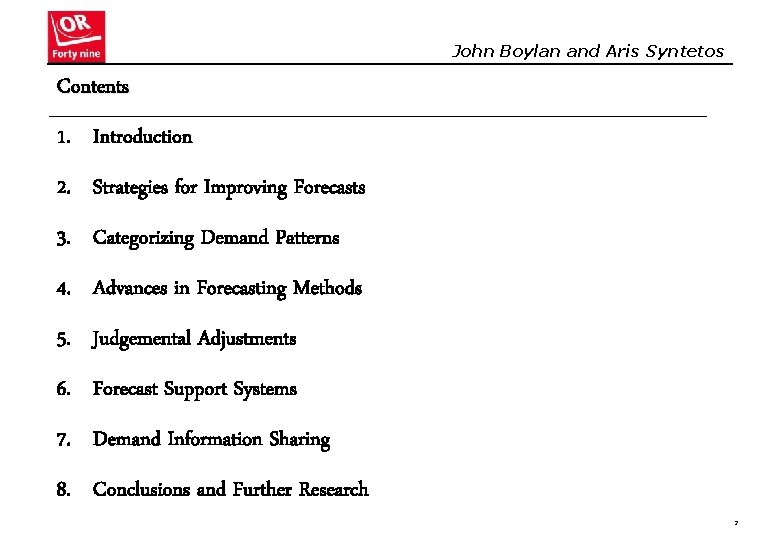
John Boylan and Aris Syntetos Contents 1. Introduction 2. Strategies for Improving Forecasts 3. Categorizing Demand Patterns 4. Advances in Forecasting Methods 5. Judgemental Adjustments 6. Forecast Support Systems 7. Demand Information Sharing 8. Conclusions and Further Research 2
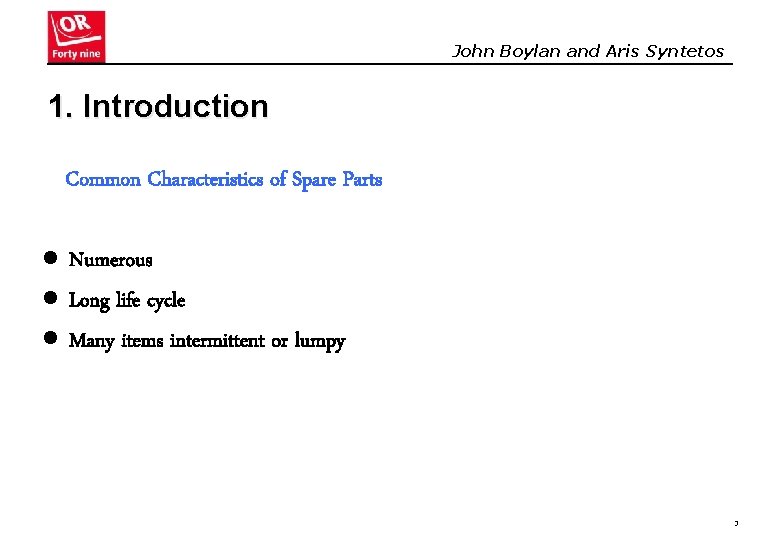
John Boylan and Aris Syntetos 1. Introduction Common Characteristics of Spare Parts l Numerous l Long life cycle l Many items intermittent or lumpy 3
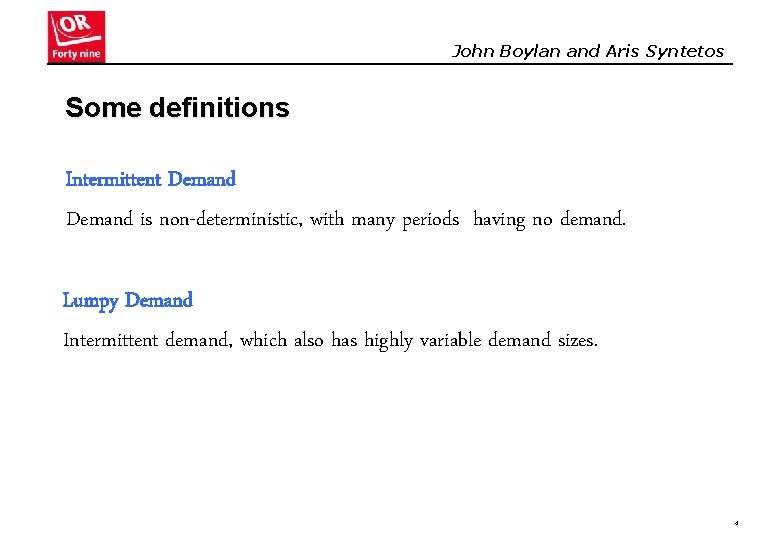
John Boylan and Aris Syntetos Some definitions Intermittent Demand is non-deterministic, with many periods having no demand. Lumpy Demand Intermittent demand, which also has highly variable demand sizes. 4
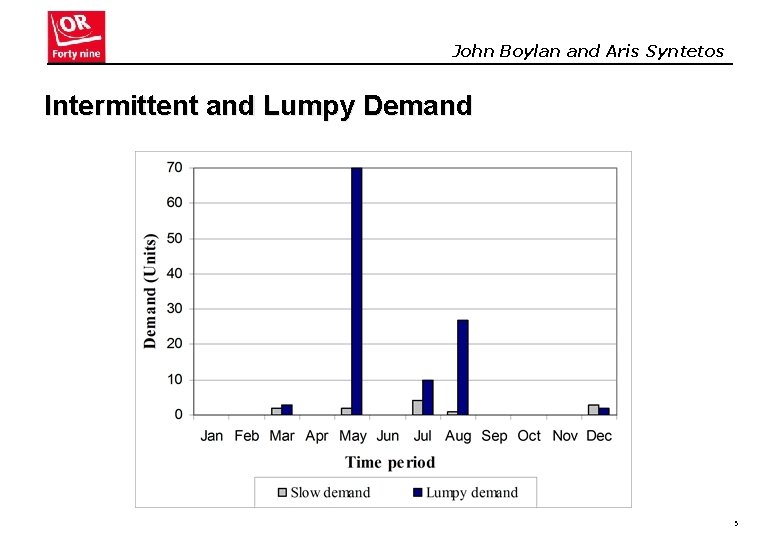
John Boylan and Aris Syntetos Intermittent and Lumpy Demand 5
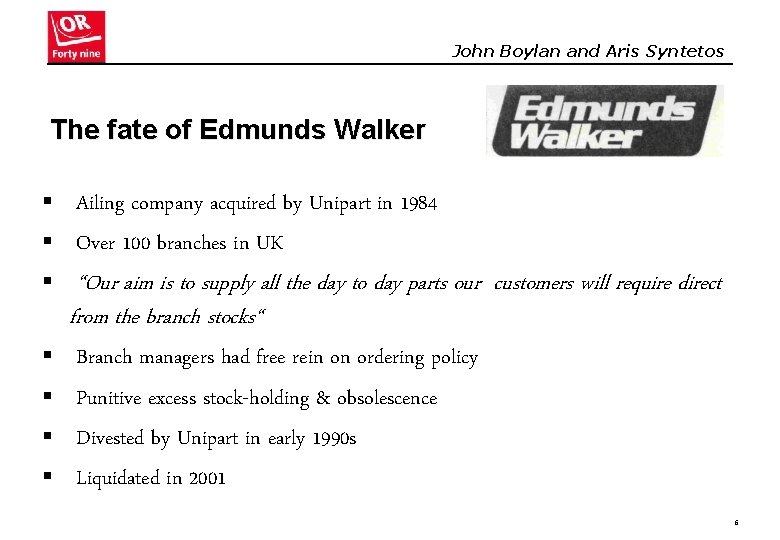
John Boylan and Aris Syntetos The fate of Edmunds Walker § Ailing company acquired by Unipart in 1984 § Over 100 branches in UK § “Our aim is to supply all the day to day parts our customers will require direct from the branch stocks“ § Branch managers had free rein on ordering policy § Punitive excess stock-holding & obsolescence § Divested by Unipart in early 1990 s § Liquidated in 2001 6
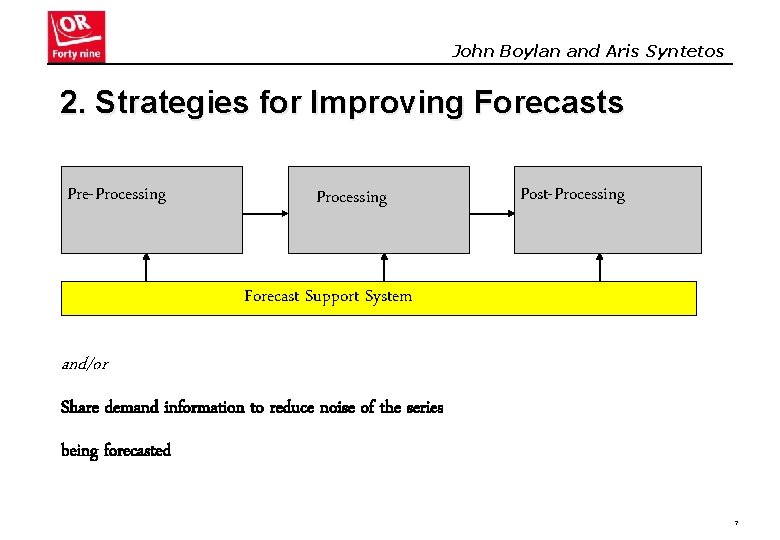
John Boylan and Aris Syntetos 2. Strategies for Improving Forecasts Pre-Processing Post-Processing Forecast Support System and/or Share demand information to reduce noise of the series being forecasted 7
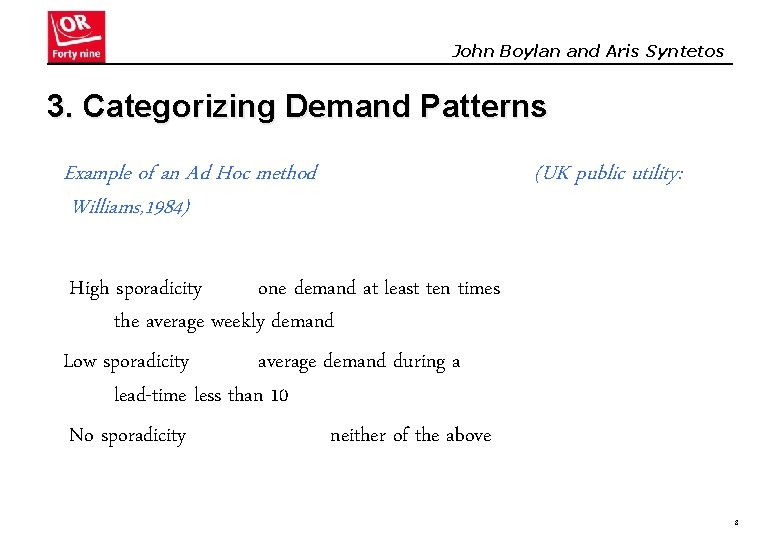
John Boylan and Aris Syntetos 3. Categorizing Demand Patterns Example of an Ad Hoc method Williams, 1984) (UK public utility: High sporadicity one demand at least ten times the average weekly demand Low sporadicity average demand during a lead-time less than 10 No sporadicity neither of the above 8
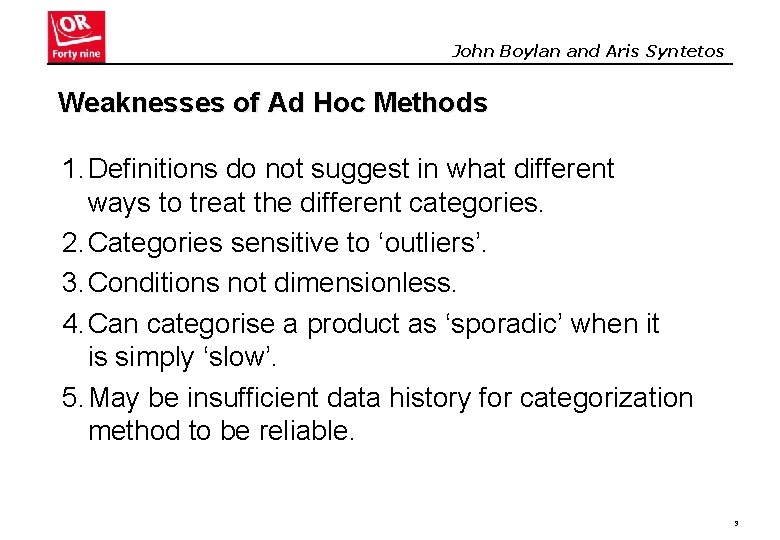
John Boylan and Aris Syntetos Weaknesses of Ad Hoc Methods 1. Definitions do not suggest in what different ways to treat the different categories. 2. Categories sensitive to ‘outliers’. 3. Conditions not dimensionless. 4. Can categorise a product as ‘sporadic’ when it is simply ‘slow’. 5. May be insufficient data history for categorization method to be reliable. 9
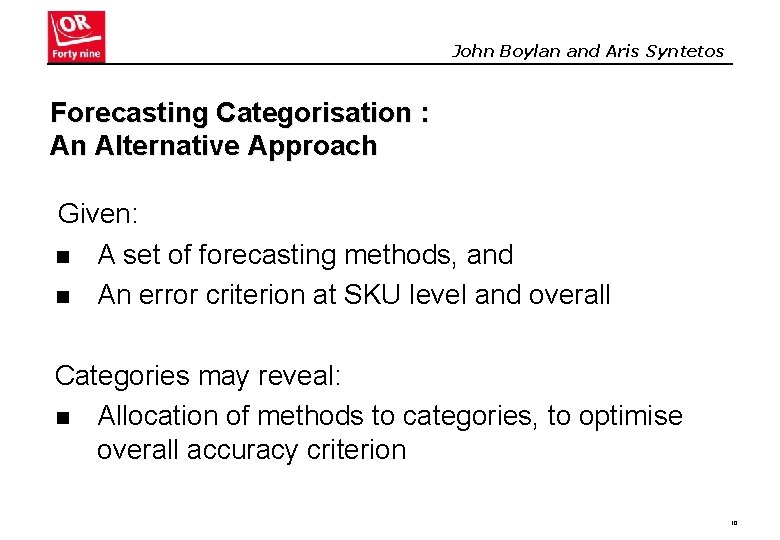
John Boylan and Aris Syntetos Forecasting Categorisation : An Alternative Approach Given: n A set of forecasting methods, and n An error criterion at SKU level and overall Categories may reveal: n Allocation of methods to categories, to optimise overall accuracy criterion 10
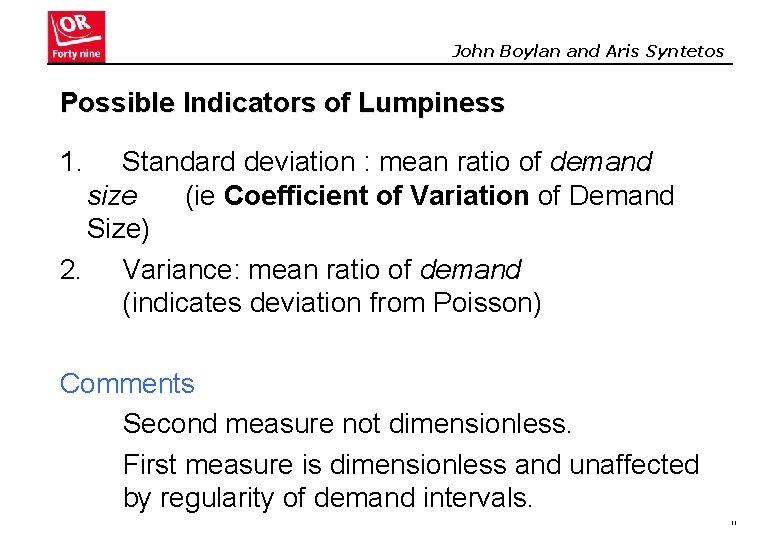
John Boylan and Aris Syntetos Possible Indicators of Lumpiness 1. Standard deviation : mean ratio of demand size (ie Coefficient of Variation of Demand Size) 2. Variance: mean ratio of demand (indicates deviation from Poisson) Comments Second measure not dimensionless. First measure is dimensionless and unaffected by regularity of demand intervals. 11
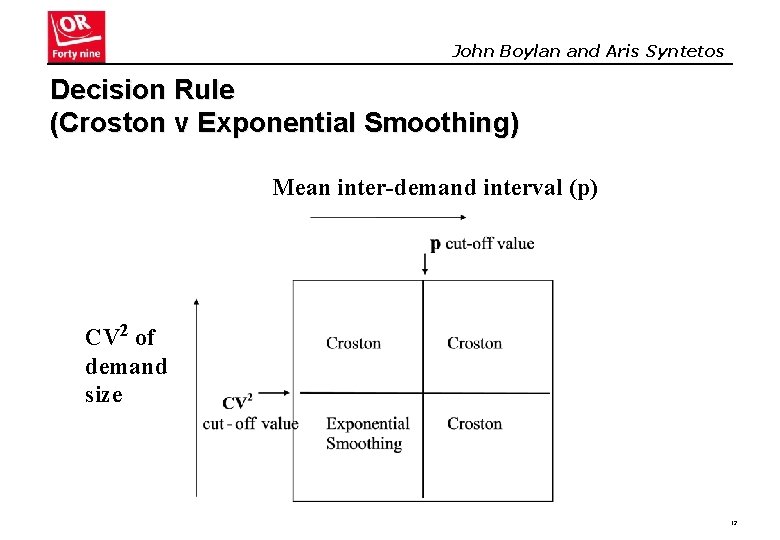
John Boylan and Aris Syntetos Decision Rule (Croston v Exponential Smoothing) Mean inter-demand interval (p) CV 2 of demand size 12
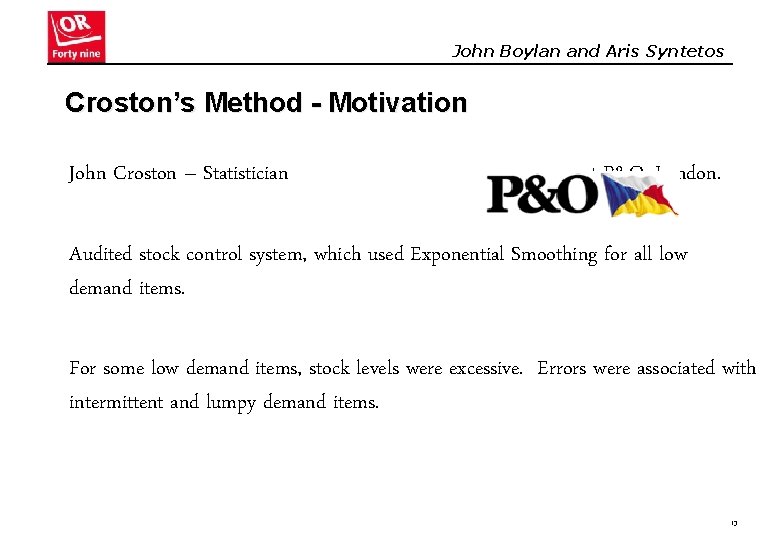
John Boylan and Aris Syntetos Croston’s Method - Motivation John Croston – Statistician at P&O, London. Audited stock control system, which used Exponential Smoothing for all low demand items. For some low demand items, stock levels were excessive. Errors were associated with intermittent and lumpy demand items. 13
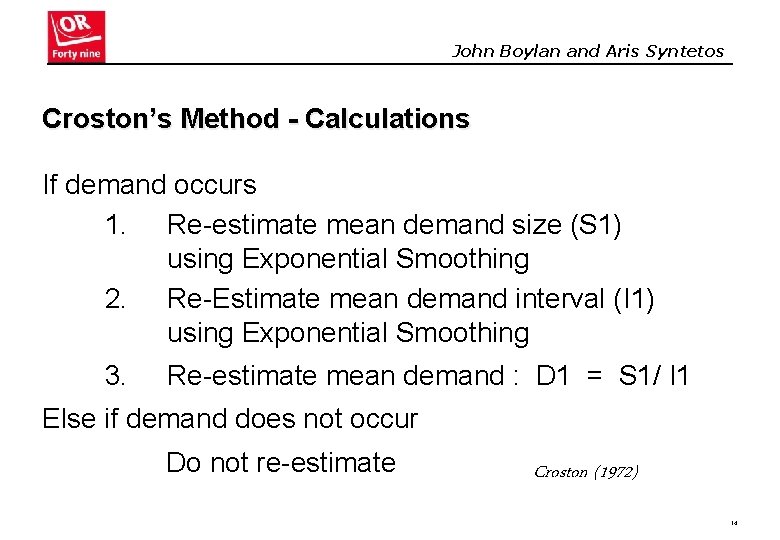
John Boylan and Aris Syntetos Croston’s Method - Calculations If demand occurs 1. Re-estimate mean demand size (S 1) using Exponential Smoothing 2. Re-Estimate mean demand interval (I 1) using Exponential Smoothing 3. Re-estimate mean demand : D 1 = S 1/ I 1 Else if demand does not occur Do not re-estimate Croston (1972) 14
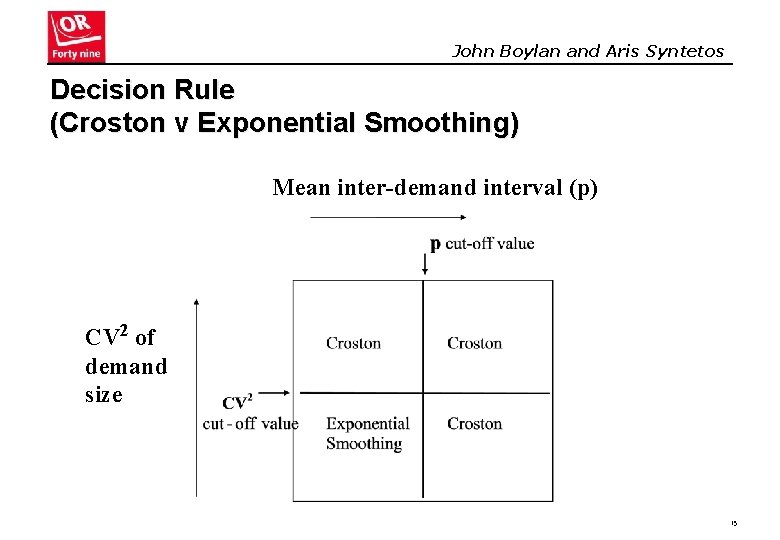
John Boylan and Aris Syntetos Decision Rule (Croston v Exponential Smoothing) Mean inter-demand interval (p) CV 2 of demand size 15
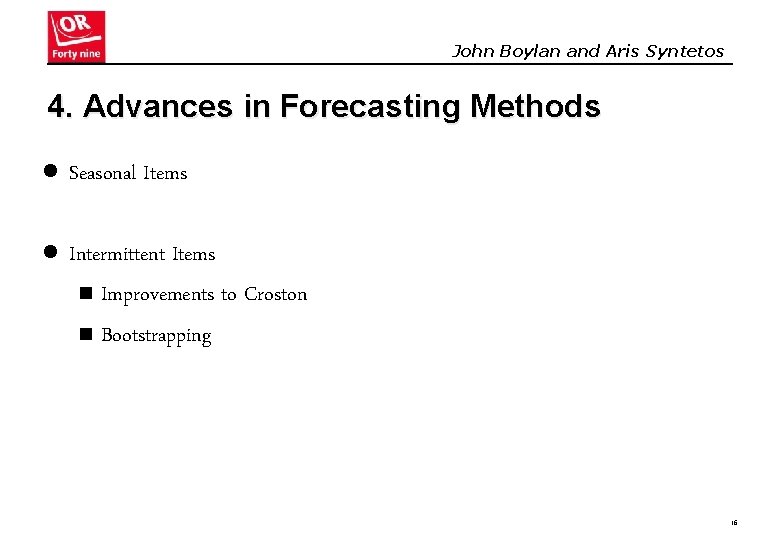
John Boylan and Aris Syntetos 4. Advances in Forecasting Methods l Seasonal Items l Intermittent Items n Improvements to Croston n Bootstrapping 16
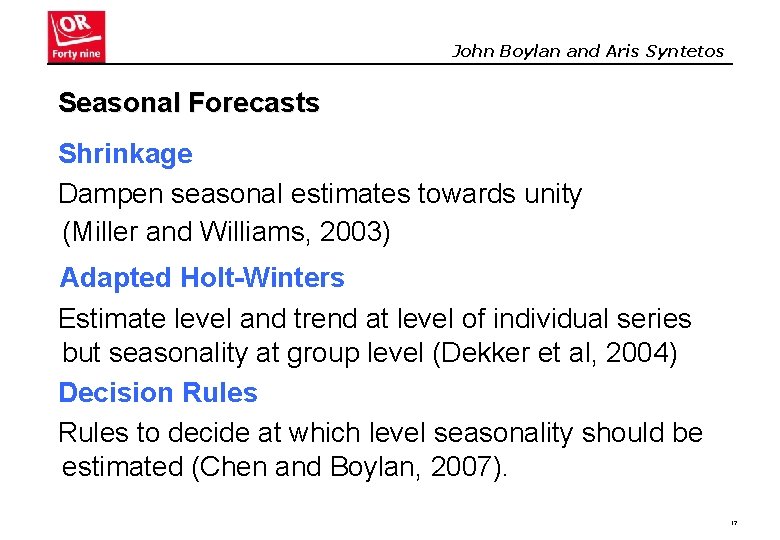
John Boylan and Aris Syntetos Seasonal Forecasts Shrinkage Dampen seasonal estimates towards unity (Miller and Williams, 2003) Adapted Holt-Winters Estimate level and trend at level of individual series but seasonality at group level (Dekker et al, 2004) Decision Rules to decide at which level seasonality should be estimated (Chen and Boylan, 2007). 17
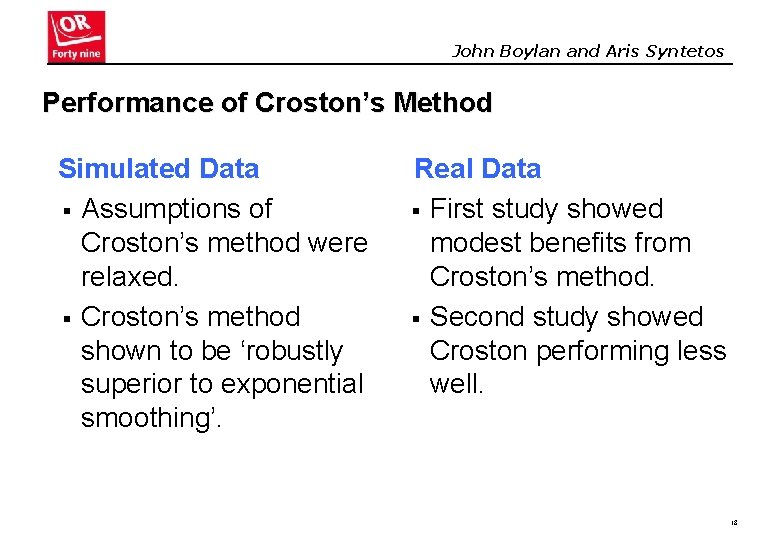
John Boylan and Aris Syntetos Performance of Croston’s Method Simulated Data § Assumptions of Croston’s method were relaxed. § Croston’s method shown to be ‘robustly superior to exponential smoothing’. Real Data § First study showed modest benefits from Croston’s method. § Second study showed Croston performing less well. 18
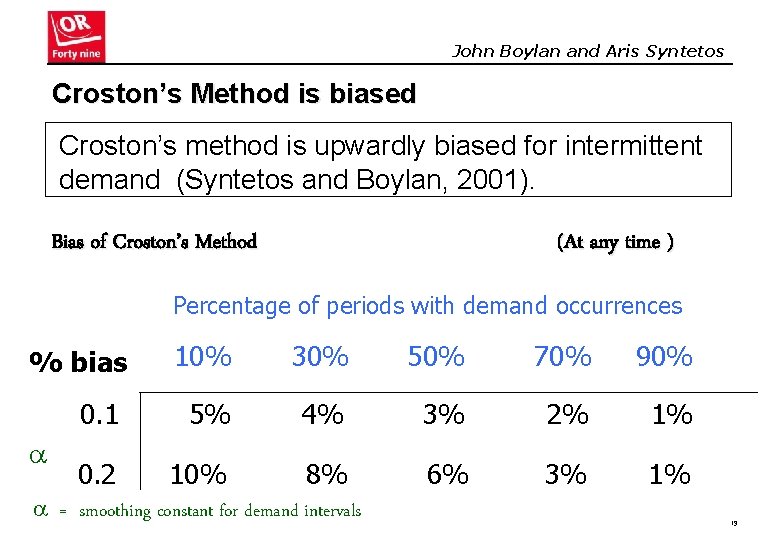
John Boylan and Aris Syntetos Croston’s Method is biased Croston’s method is upwardly biased for intermittent demand (Syntetos and Boylan, 2001). Bias of Croston’s Method (At any time ) Percentage of periods with demand occurrences 10% 30% 50% 70% 90% 0. 1 5% 4% 3% 2% 1% 0. 2 10% 8% 6% 3% 1% % bias = smoothing constant for demand intervals 19
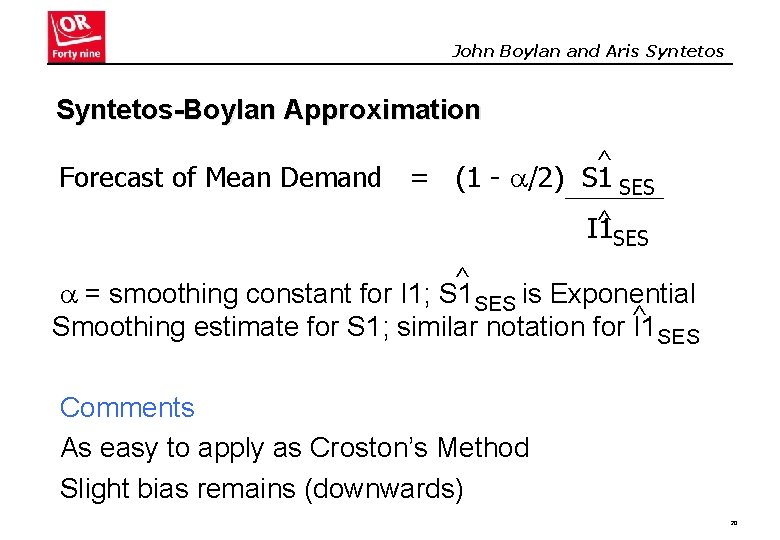
John Boylan and Aris Syntetos-Boylan Approximation ^ Forecast of Mean Demand = (1 - /2) S 1 SES ^ I 1 SES ^ = smoothing constant for I 1; S 1 SES is Exponential ^ Smoothing estimate for S 1; similar notation for I 1 SES Comments As easy to apply as Croston’s Method Slight bias remains (downwards) 20
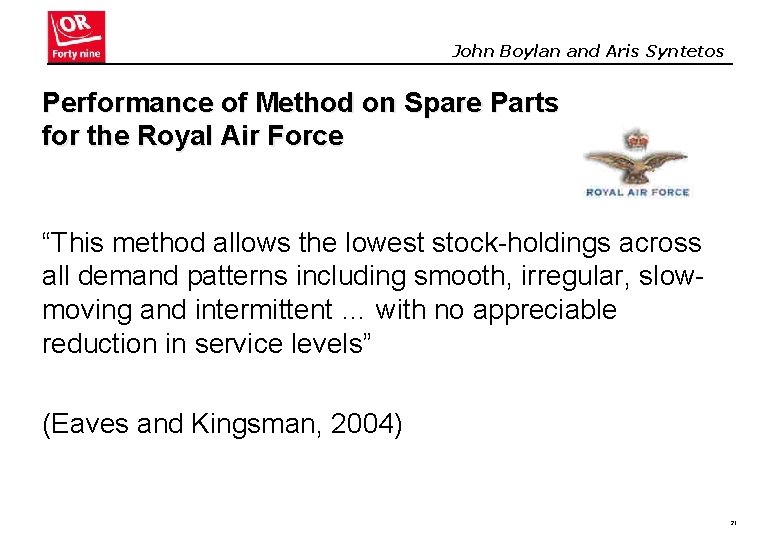
John Boylan and Aris Syntetos Performance of Method on Spare Parts for the Royal Air Force “This method allows the lowest stock-holdings across all demand patterns including smooth, irregular, slowmoving and intermittent … with no appreciable reduction in service levels” (Eaves and Kingsman, 2004) 21
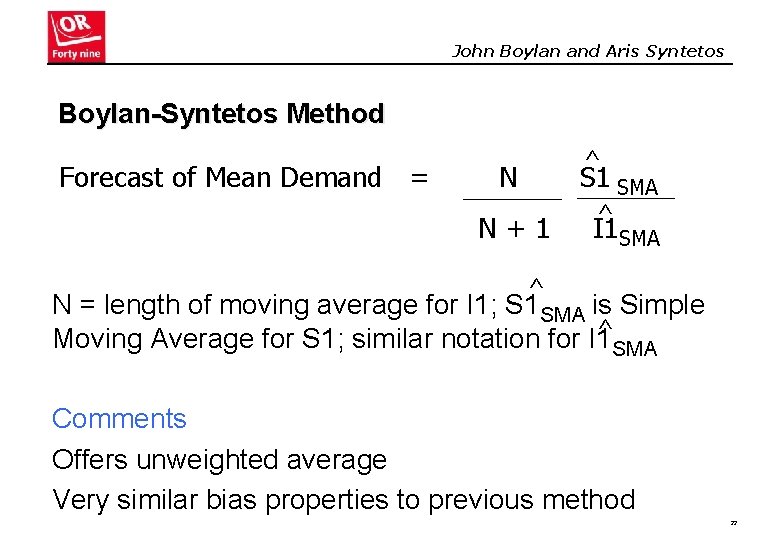
John Boylan and Aris Syntetos Boylan-Syntetos Method Forecast of Mean Demand = N N+1 ^ S 1 SMA ^ I 1 SMA ^ N = length of moving average for I 1; S 1 SMA is Simple ^ Moving Average for S 1; similar notation for I 1 SMA Comments Offers unweighted average Very similar bias properties to previous method 22
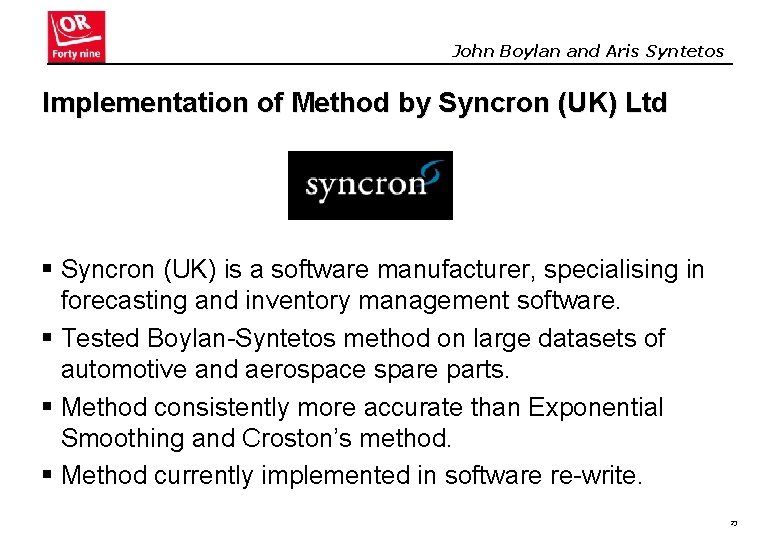
John Boylan and Aris Syntetos Implementation of Method by Syncron (UK) Ltd § Syncron (UK) is a software manufacturer, specialising in forecasting and inventory management software. § Tested Boylan-Syntetos method on large datasets of automotive and aerospace spare parts. § Method consistently more accurate than Exponential Smoothing and Croston’s method. § Method currently implemented in software re-write. 23
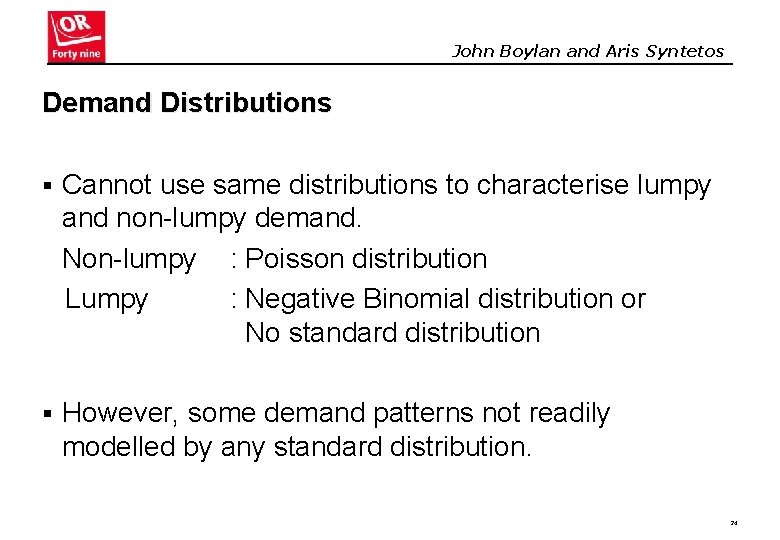
John Boylan and Aris Syntetos Demand Distributions § Cannot use same distributions to characterise lumpy and non-lumpy demand. Non-lumpy : Poisson distribution Lumpy : Negative Binomial distribution or No standard distribution § However, some demand patterns not readily modelled by any standard distribution. 24
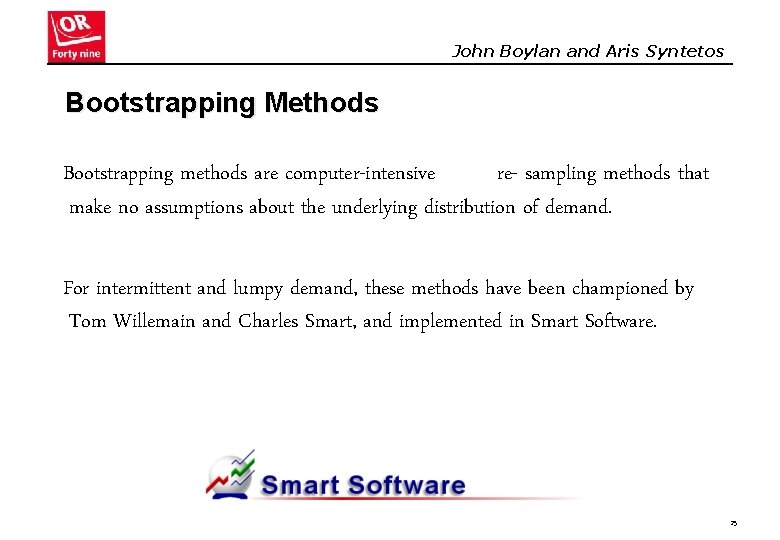
John Boylan and Aris Syntetos Bootstrapping Methods Bootstrapping methods are computer-intensive re- sampling methods that make no assumptions about the underlying distribution of demand. For intermittent and lumpy demand, these methods have been championed by Tom Willemain and Charles Smart, and implemented in Smart Software. 25
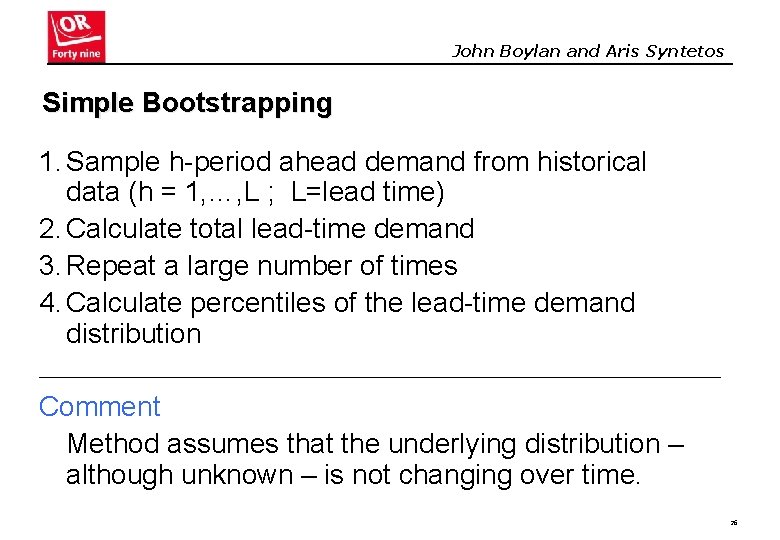
John Boylan and Aris Syntetos Simple Bootstrapping 1. Sample h-period ahead demand from historical data (h = 1, …, L ; L=lead time) 2. Calculate total lead-time demand 3. Repeat a large number of times 4. Calculate percentiles of the lead-time demand distribution Comment Method assumes that the underlying distribution – although unknown – is not changing over time. 26
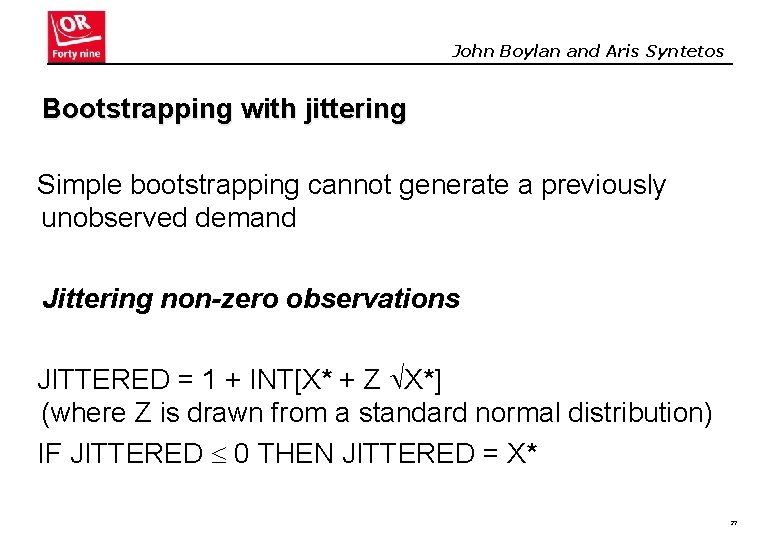
John Boylan and Aris Syntetos Bootstrapping with jittering Simple bootstrapping cannot generate a previously unobserved demand Jittering non-zero observations JITTERED = 1 + INT[X* + Z X*] (where Z is drawn from a standard normal distribution) IF JITTERED 0 THEN JITTERED = X* 27
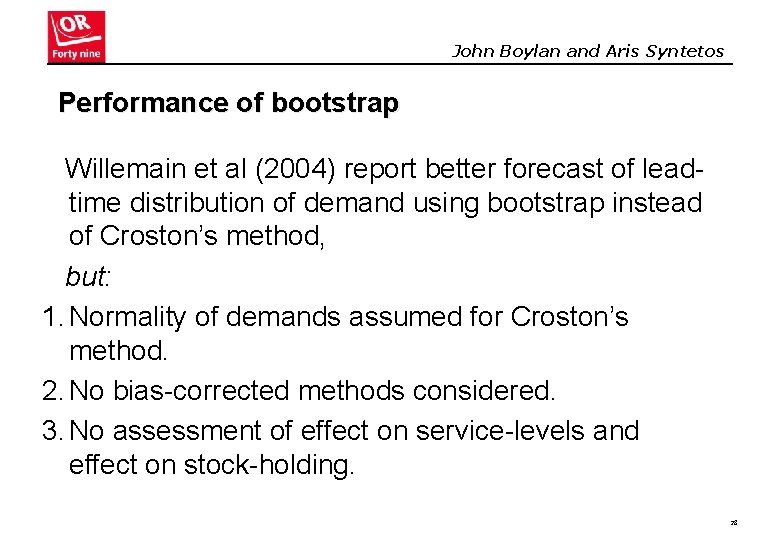
John Boylan and Aris Syntetos Performance of bootstrap Willemain et al (2004) report better forecast of leadtime distribution of demand using bootstrap instead of Croston’s method, but: 1. Normality of demands assumed for Croston’s method. 2. No bias-corrected methods considered. 3. No assessment of effect on service-levels and effect on stock-holding. 28
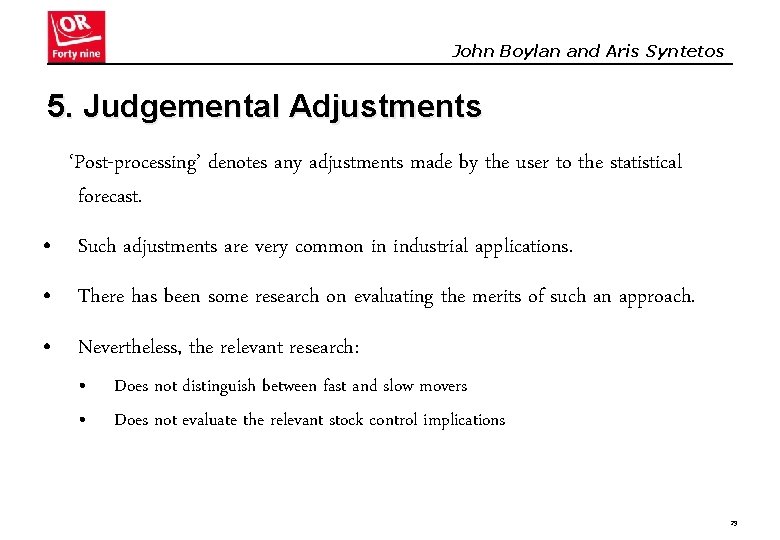
John Boylan and Aris Syntetos 5. Judgemental Adjustments ‘Post-processing’ denotes any adjustments made by the user to the statistical forecast. • Such adjustments are very common in industrial applications. • There has been some research on evaluating the merits of such an approach. • Nevertheless, the relevant research: • • Does not distinguish between fast and slow movers Does not evaluate the relevant stock control implications 29
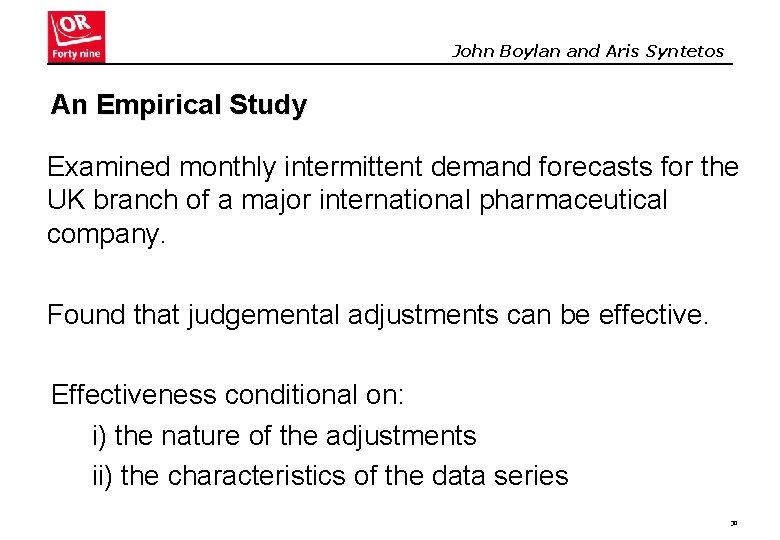
John Boylan and Aris Syntetos An Empirical Study Examined monthly intermittent demand forecasts for the UK branch of a major international pharmaceutical company. Found that judgemental adjustments can be effective. Effectiveness conditional on: i) the nature of the adjustments ii) the characteristics of the data series 30
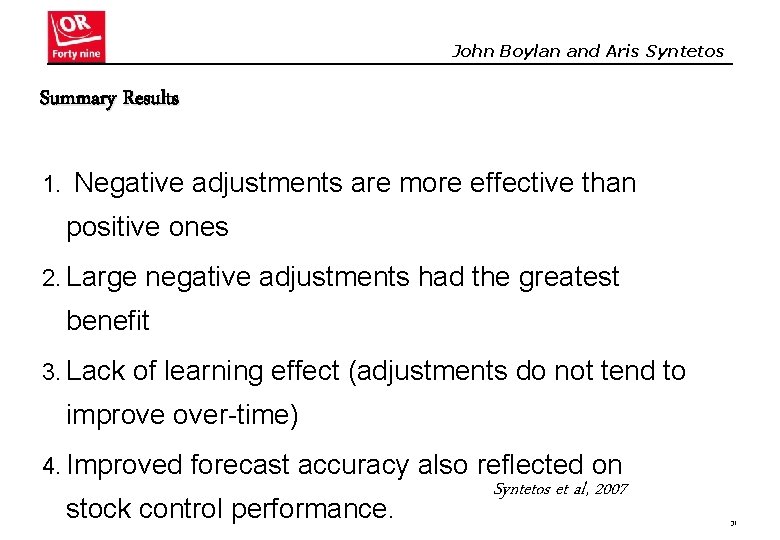
John Boylan and Aris Syntetos Summary Results 1. Negative adjustments are more effective than positive ones 2. Large negative adjustments had the greatest benefit 3. Lack of learning effect (adjustments do not tend to improve over-time) 4. Improved forecast accuracy also reflected on stock control performance. Syntetos et al, 2007 31
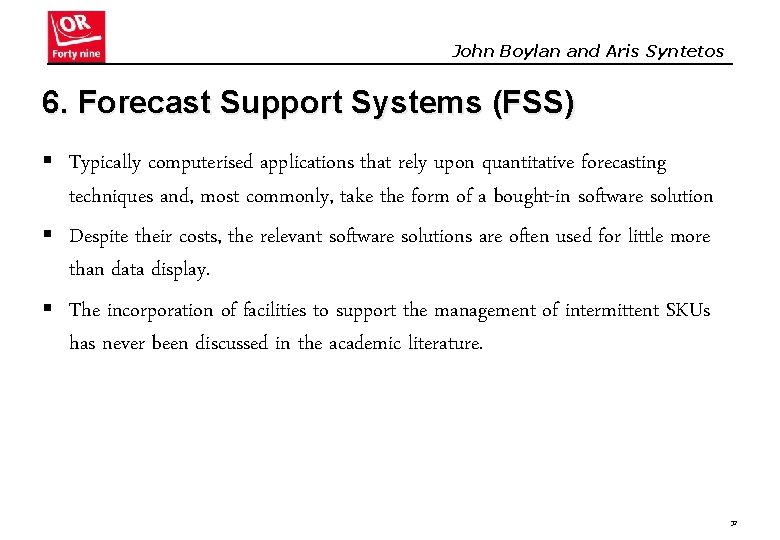
John Boylan and Aris Syntetos 6. Forecast Support Systems (FSS) § Typically computerised applications that rely upon quantitative forecasting techniques and, most commonly, take the form of a bought-in software solution § Despite their costs, the relevant software solutions are often used for little more than data display. § The incorporation of facilities to support the management of intermittent SKUs has never been discussed in the academic literature. 32
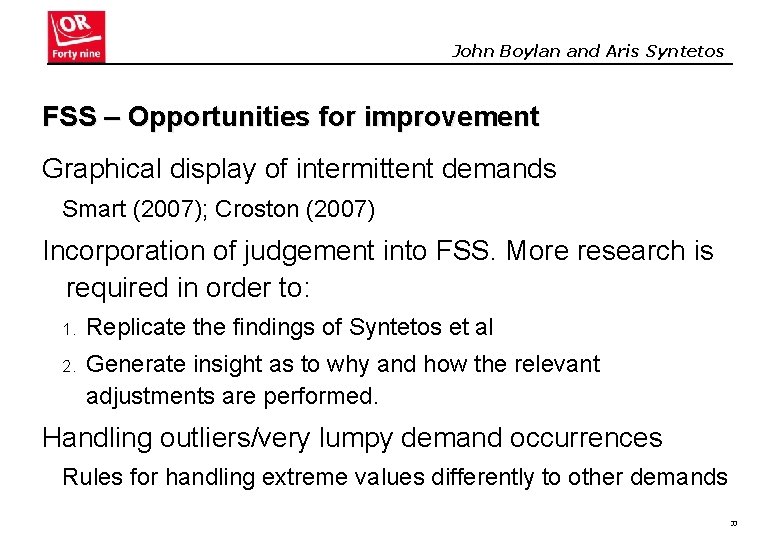
John Boylan and Aris Syntetos FSS – Opportunities for improvement Graphical display of intermittent demands Smart (2007); Croston (2007) Incorporation of judgement into FSS. More research is required in order to: 1. Replicate the findings of Syntetos et al 2. Generate insight as to why and how the relevant adjustments are performed. Handling outliers/very lumpy demand occurrences Rules for handling extreme values differently to other demands 33
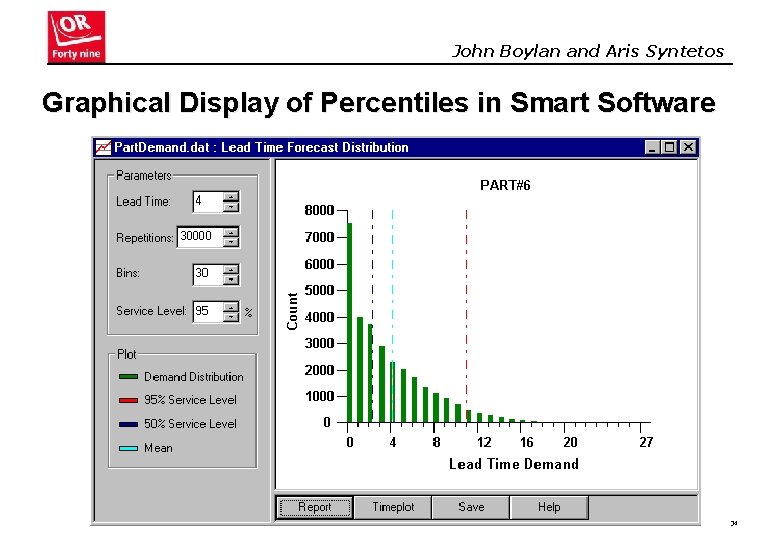
John Boylan and Aris Syntetos Graphical Display of Percentiles in Smart Software 34
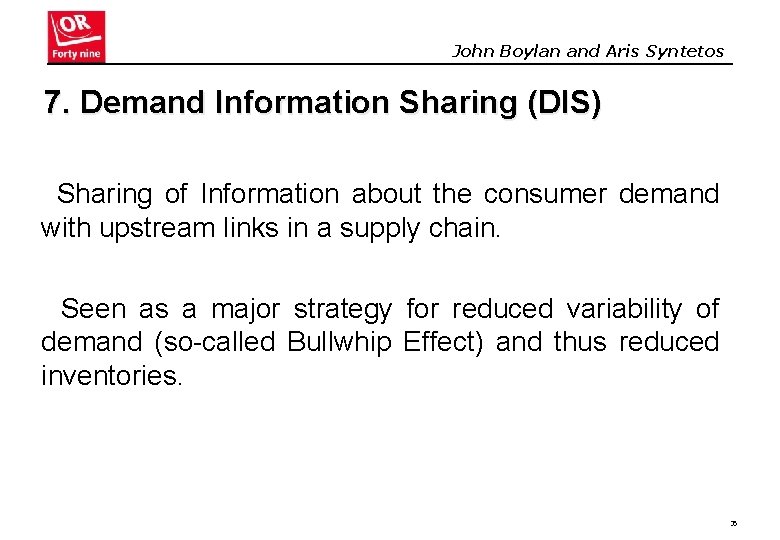
John Boylan and Aris Syntetos 7. Demand Information Sharing (DIS) Sharing of Information about the consumer demand with upstream links in a supply chain. Seen as a major strategy for reduced variability of demand (so-called Bullwhip Effect) and thus reduced inventories. 35
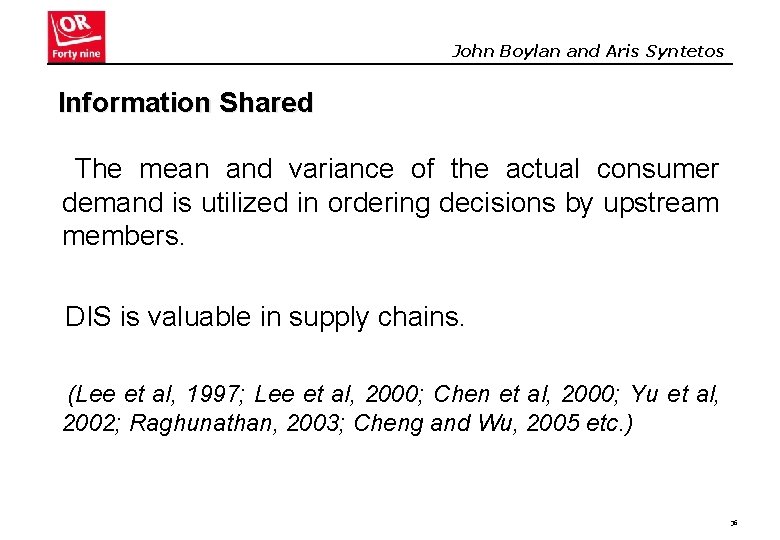
John Boylan and Aris Syntetos Information Shared The mean and variance of the actual consumer demand is utilized in ordering decisions by upstream members. DIS is valuable in supply chains. (Lee et al, 1997; Lee et al, 2000; Chen et al, 2000; Yu et al, 2002; Raghunathan, 2003; Cheng and Wu, 2005 etc. ) 36
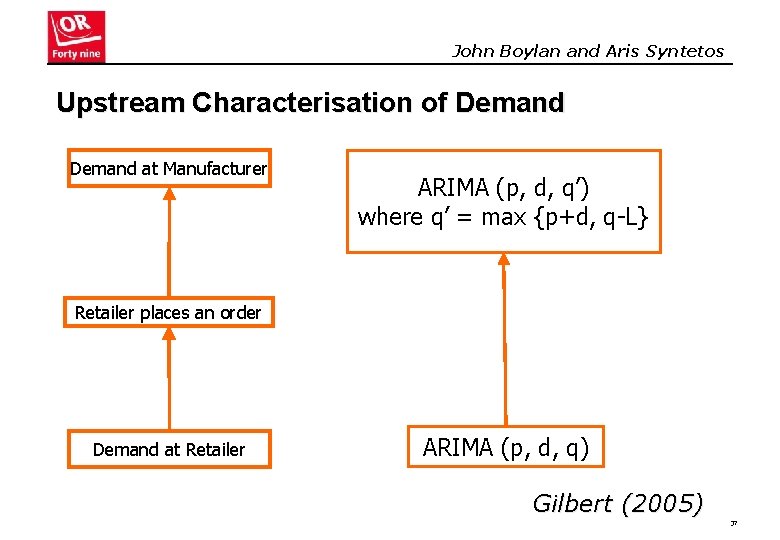
John Boylan and Aris Syntetos Upstream Characterisation of Demand at Manufacturer ARIMA (p, d, q’) where q’ = max {p+d, q-L} Retailer places an order Demand at Retailer ARIMA (p, d, q) Gilbert (2005) 37
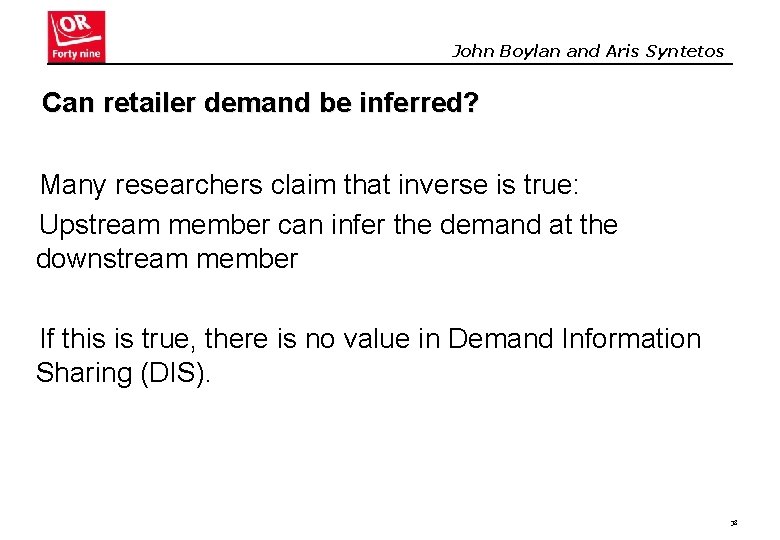
John Boylan and Aris Syntetos Can retailer demand be inferred? Many researchers claim that inverse is true: Upstream member can infer the demand at the downstream member If this is true, there is no value in Demand Information Sharing (DIS). 38
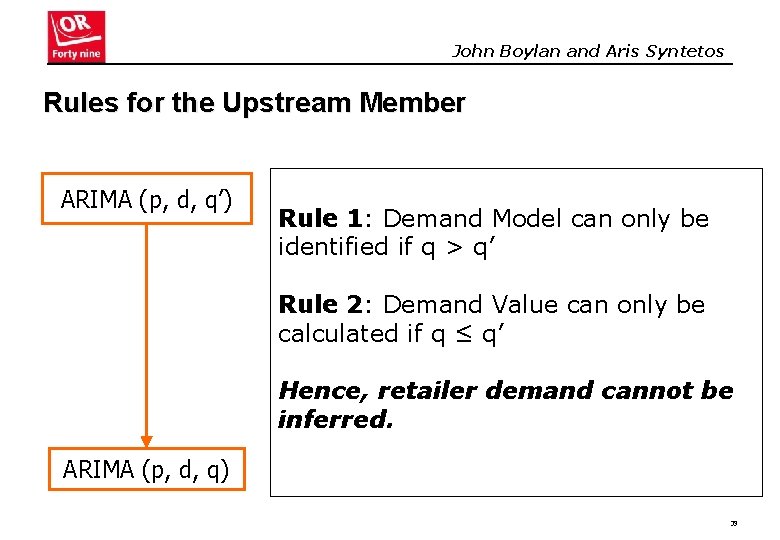
John Boylan and Aris Syntetos Rules for the Upstream Member ARIMA (p, d, q’) Rule 1: Demand Model can only be identified if q > q’ Rule 2: Demand Value can only be calculated if q ≤ q’ Hence, retailer demand cannot be inferred. ARIMA (p, d, q) 39
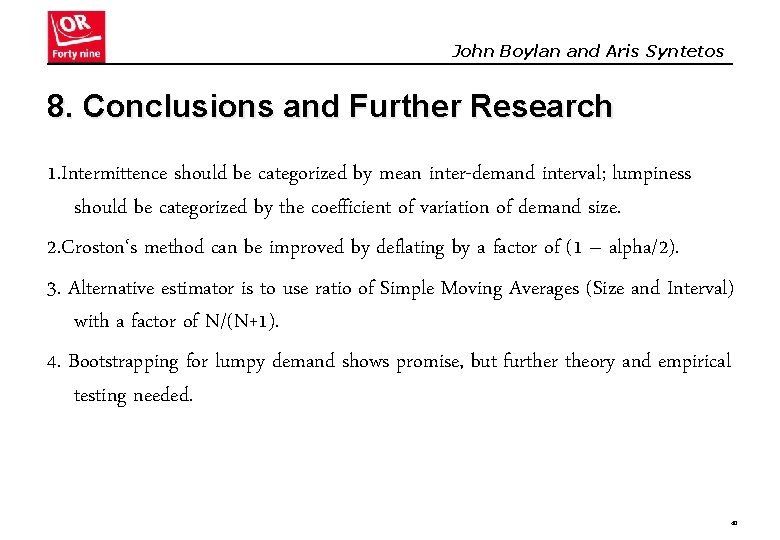
John Boylan and Aris Syntetos 8. Conclusions and Further Research 1. Intermittence should be categorized by mean inter-demand interval; lumpiness should be categorized by the coefficient of variation of demand size. 2. Croston‘s method can be improved by deflating by a factor of (1 – alpha/2). 3. Alternative estimator is to use ratio of Simple Moving Averages (Size and Interval) with a factor of N/(N+1). 4. Bootstrapping for lumpy demand shows promise, but further theory and empirical testing needed. 40
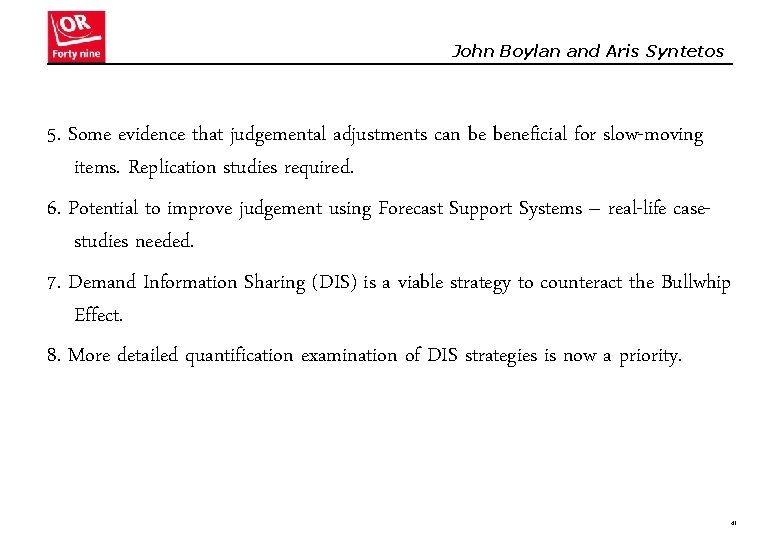
John Boylan and Aris Syntetos 5. Some evidence that judgemental adjustments can be beneficial for slow-moving items. Replication studies required. 6. Potential to improve judgement using Forecast Support Systems – real-life casestudies needed. 7. Demand Information Sharing (DIS) is a viable strategy to counteract the Bullwhip Effect. 8. More detailed quantification examination of DIS strategies is now a priority. 41
Dynamitron
Swot analysis of tata group
Tower crane anatomy
Introducing and naming new products and brand extensions
Introducing and naming new products and brand extensions
Conducting marketing research and forecasting demand
Conducting marketing research and forecasting demand
Short term financial management
Collecting information and forecasting demand summary
Iontropic
Spare receptors
Paul ehrlich
3 6 9 spare system chart
Spare change physical versus chemical change
Spare or execute alba
Active listening vs hearing
All star somebody once told me
Picaxe 14m2 pinout
Bowling techniques
The famous spare tire problem is the example of
The famous spare tire problem is the example of
Spare time hobby
Chapter 18 braiding and braid extensions
Extensions of demand and supply analysis
Technology life cycle
University of kent extensions
Hybris modules
Oracle procedural language extensions to sql
Can't pickle psycopg2.extensions.connection objects
Simd extensions
Extensions of recurrent neural network language model
Point to point correspondence and formal similarity
_mm_add_ss
Data mining extensions
Restoration electrolysis
Reactive programming for net developers
Mapping extensions
Tall pad extensions are used
Intel isa extensions
Extensions of turing machine
Sw guard extensions
Shirt collar extensions