Spam 2 0 Workshop on Digital Social Networks
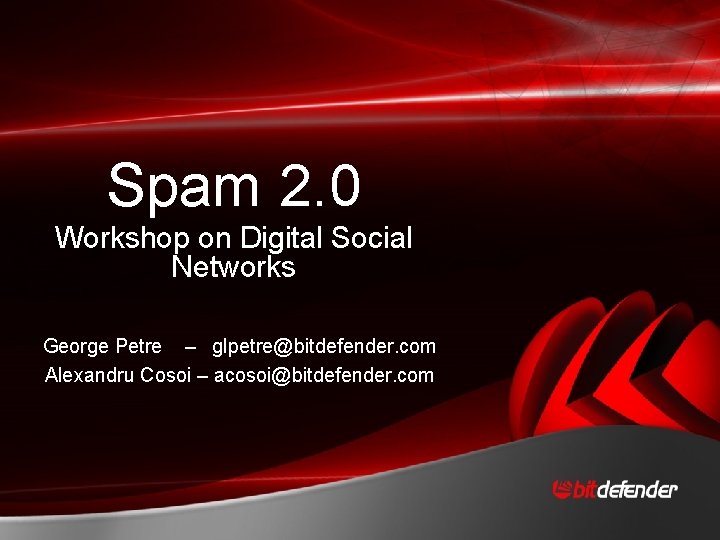
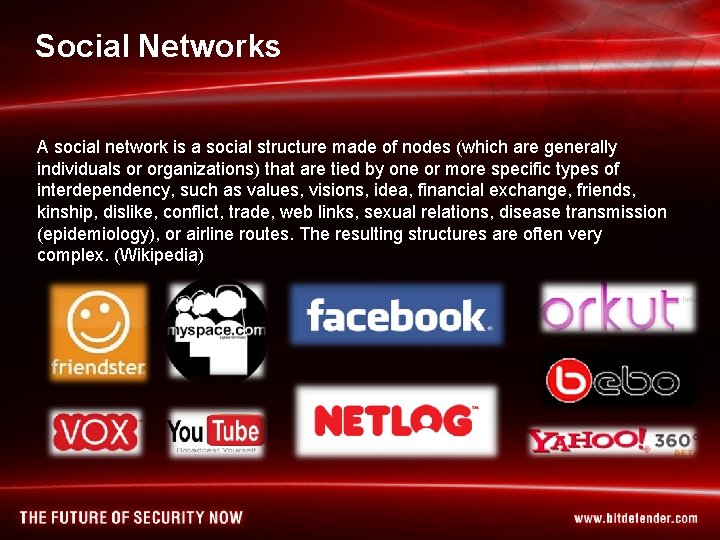
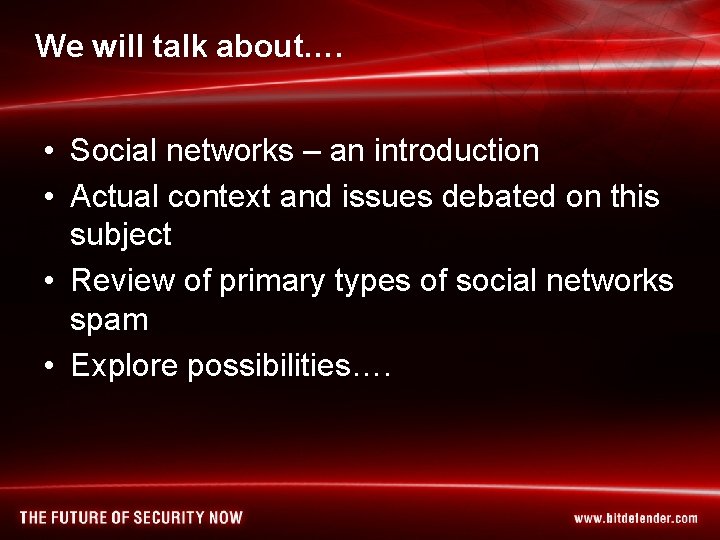
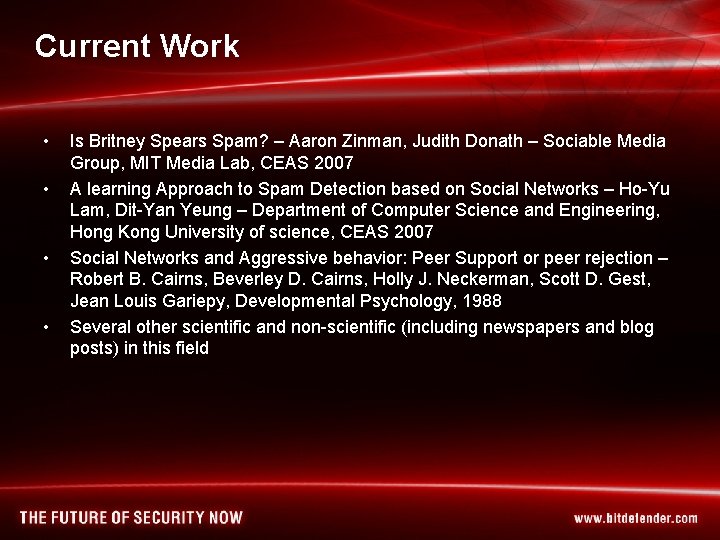
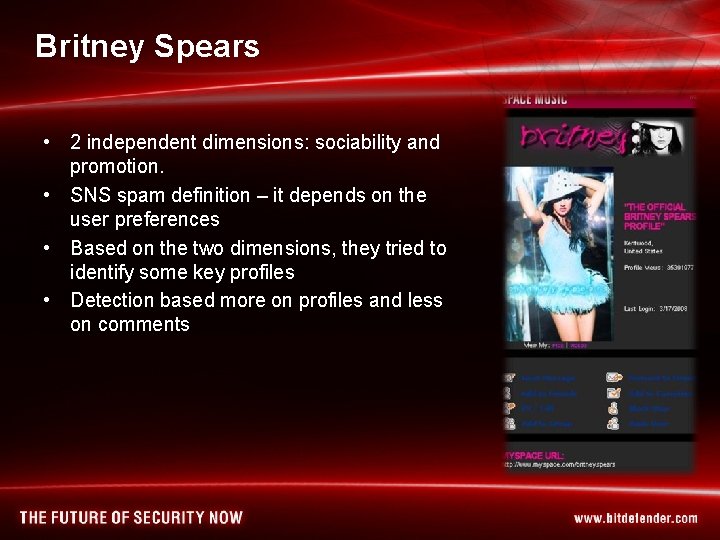
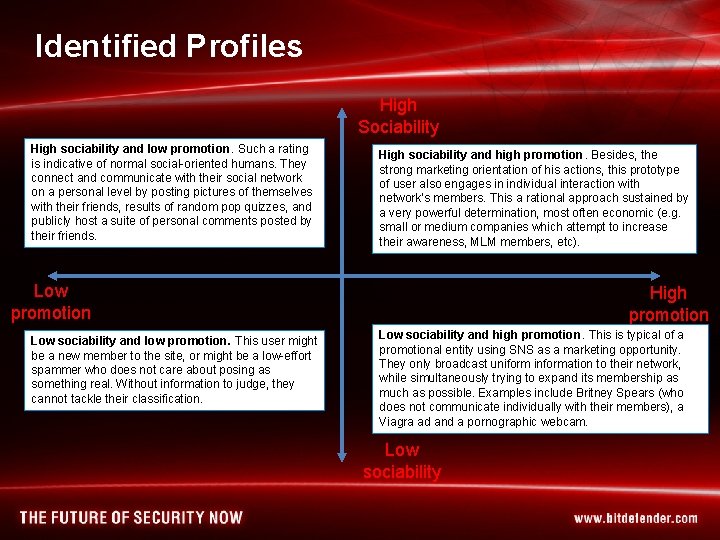
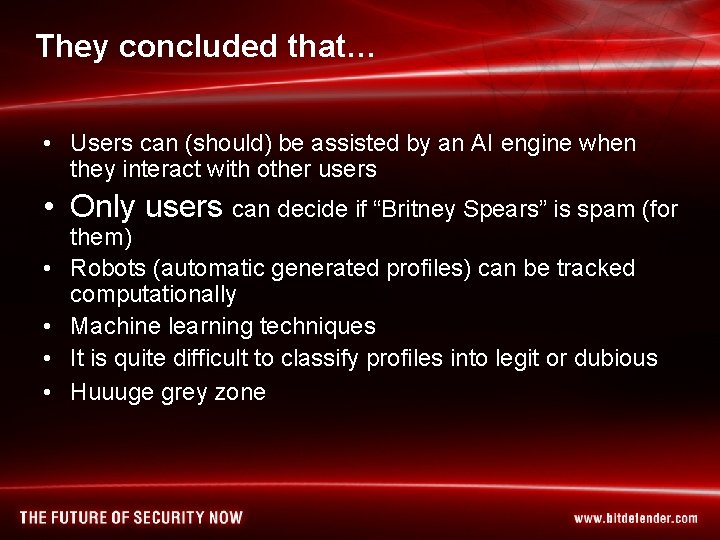
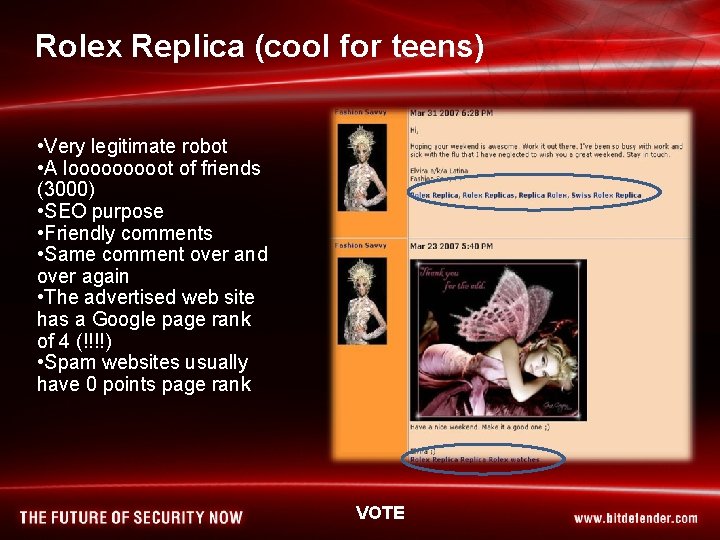
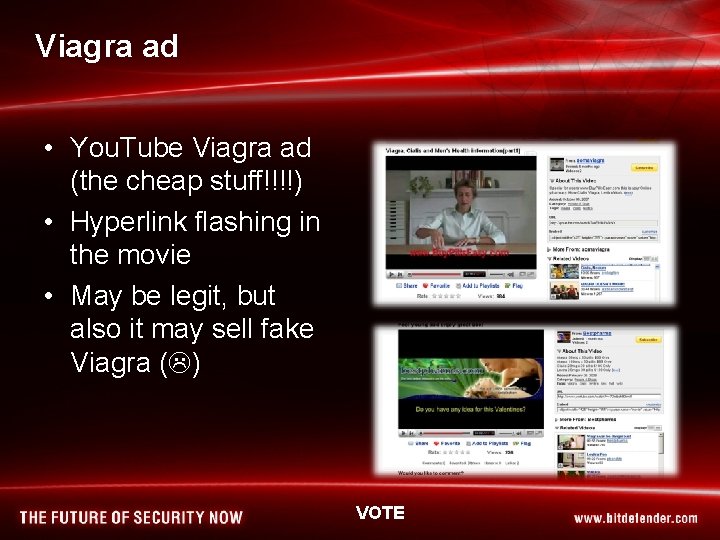
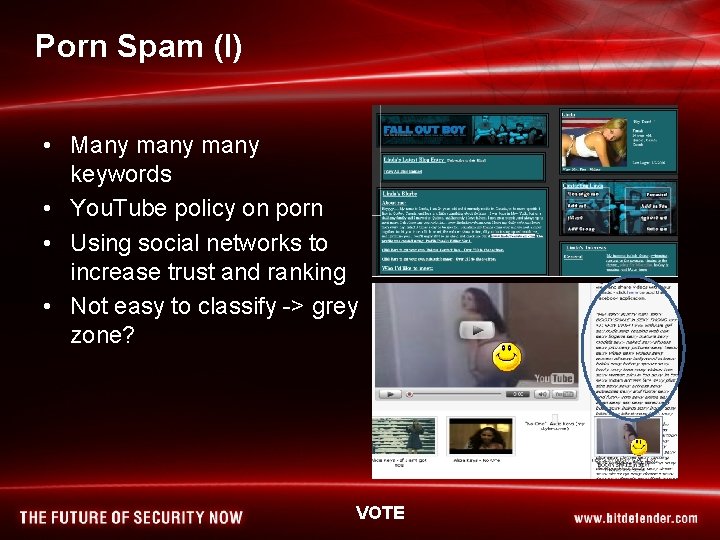
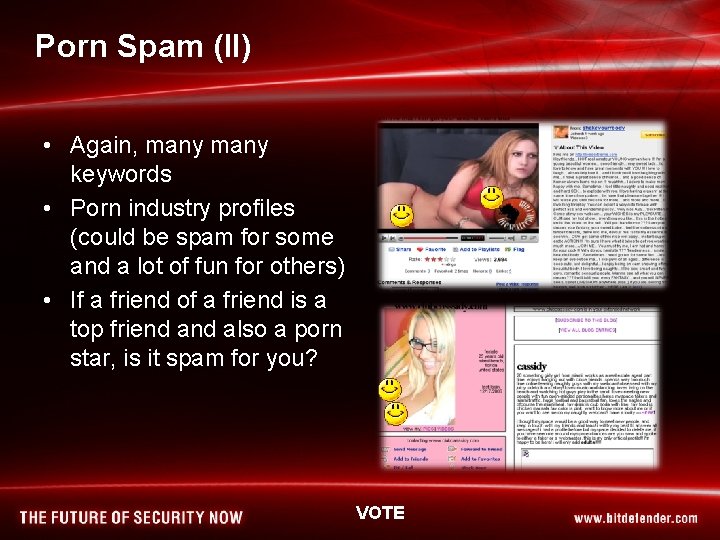
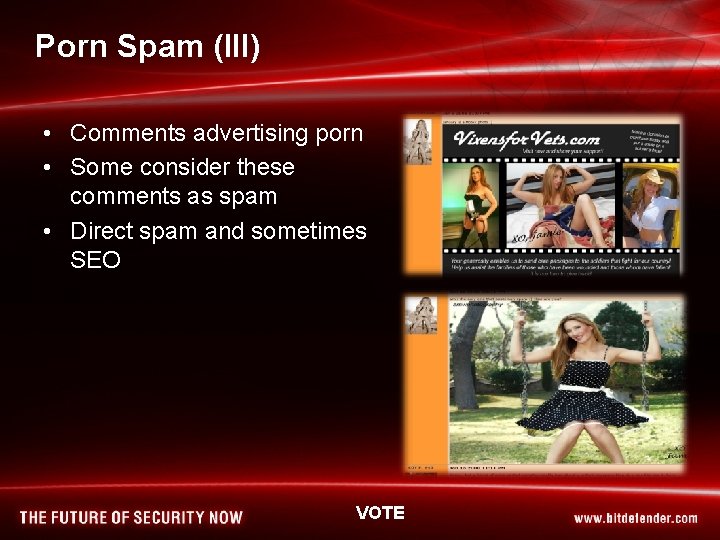
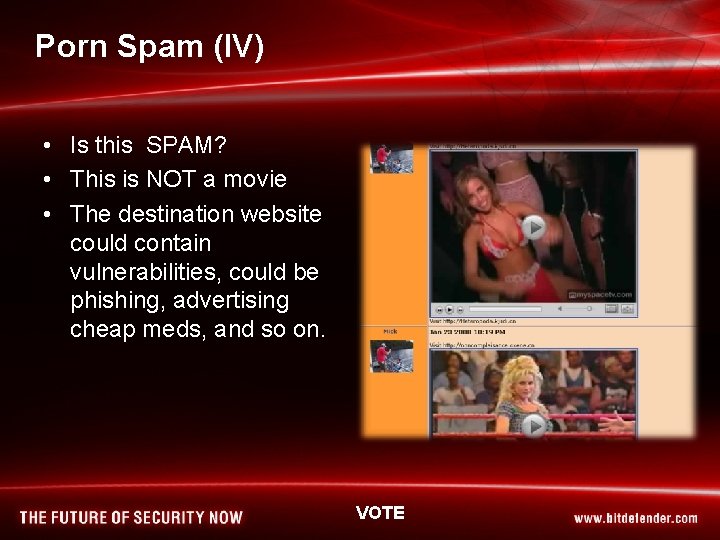
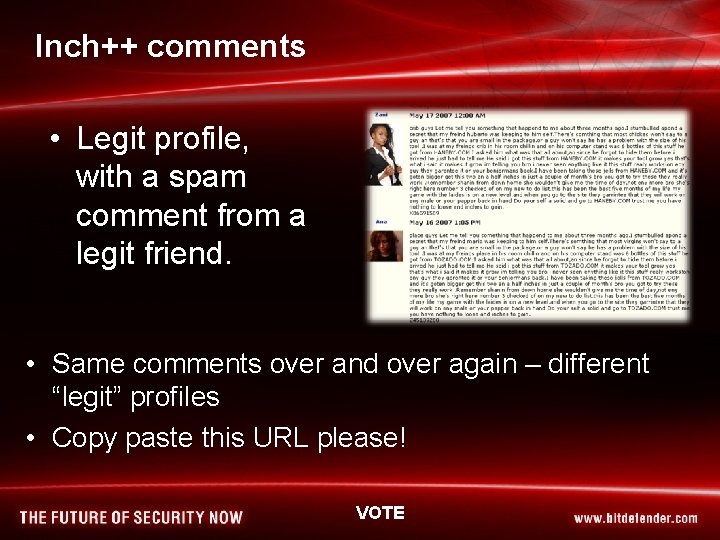
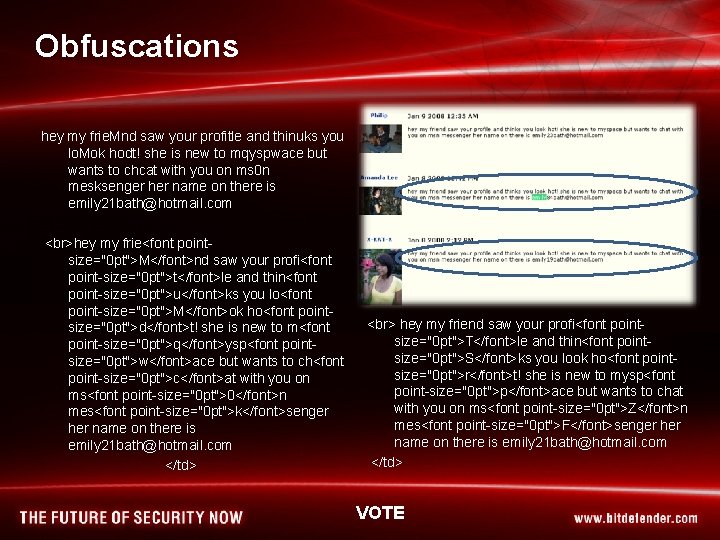
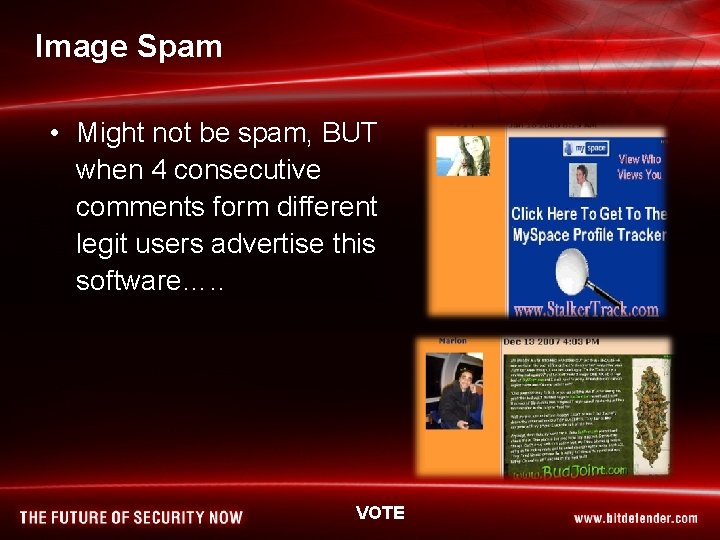
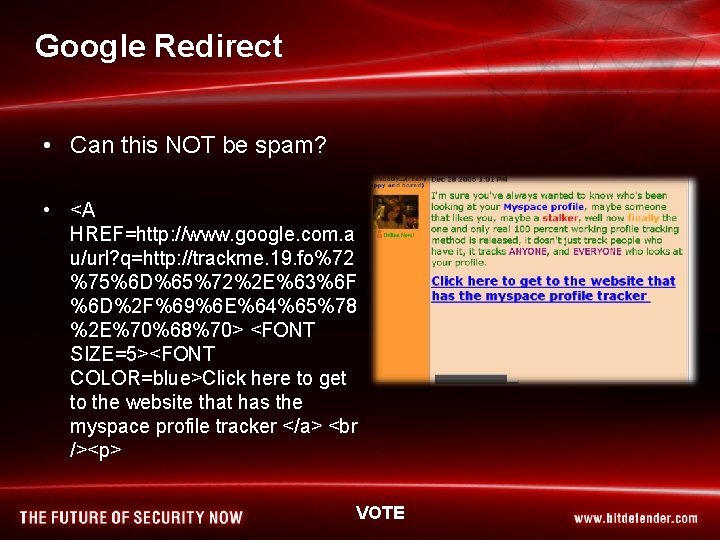
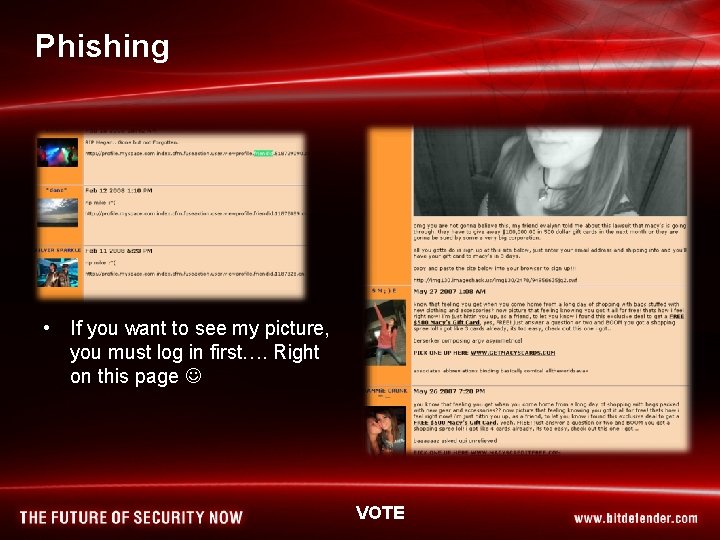
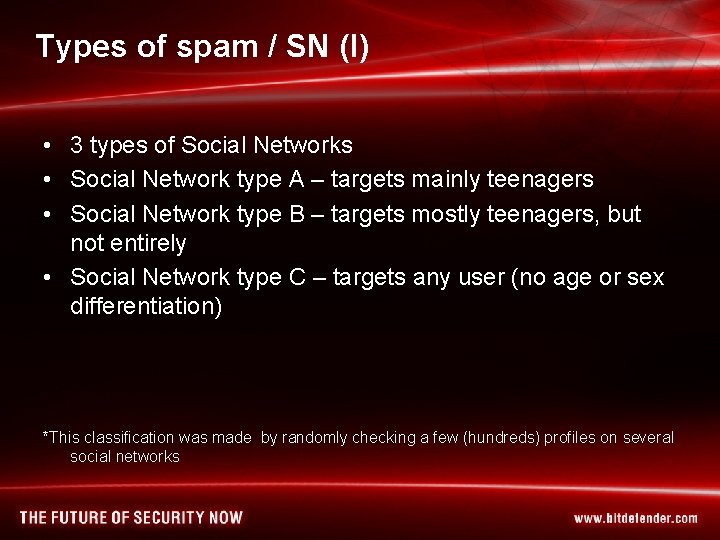
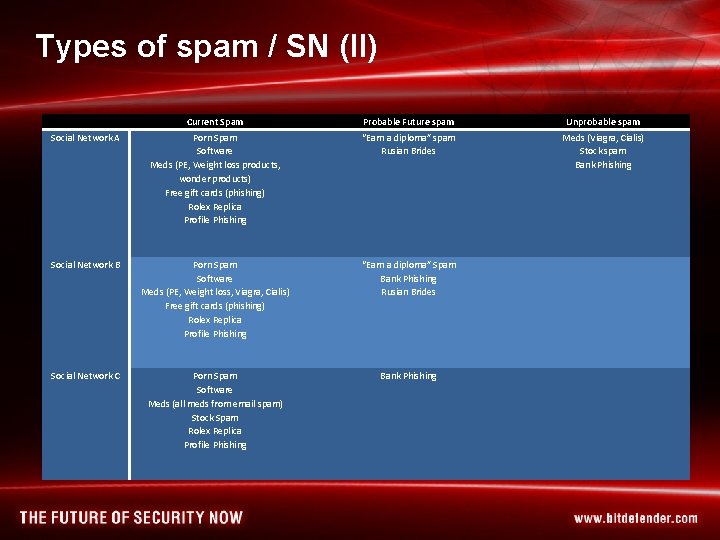
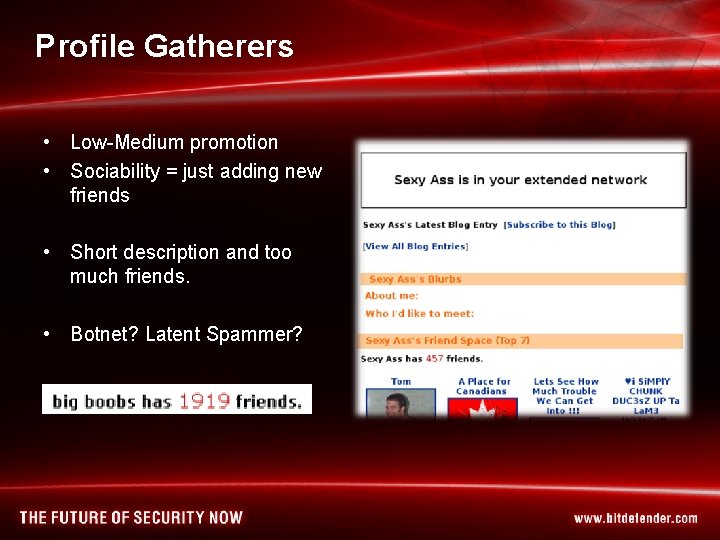
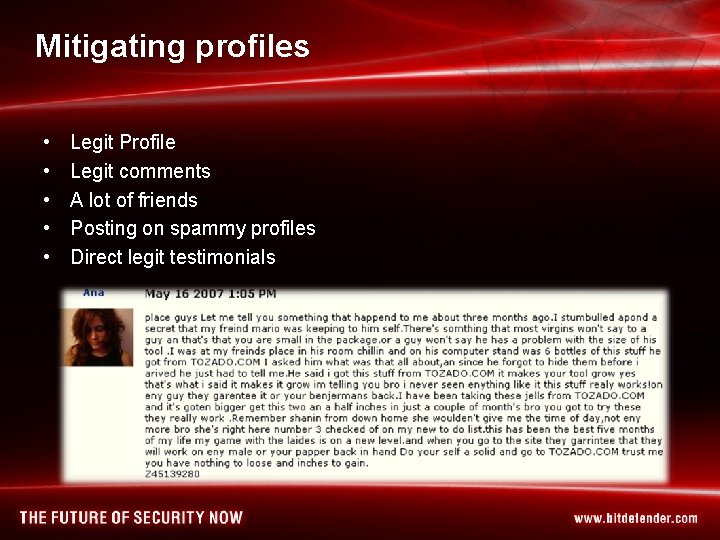
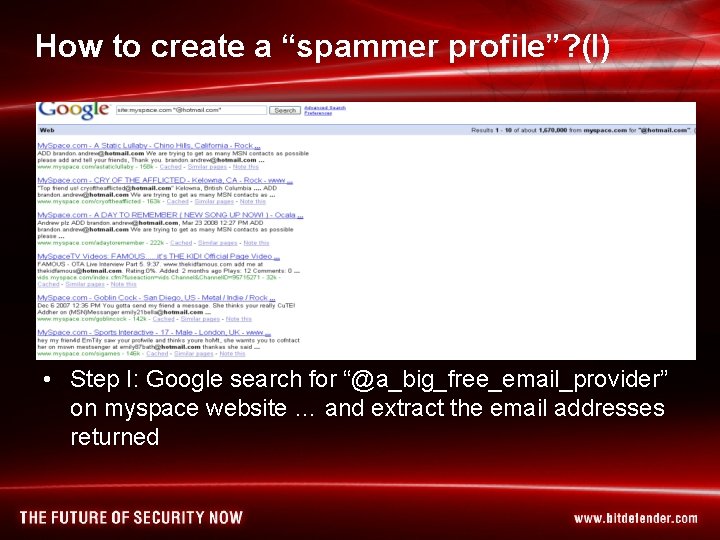
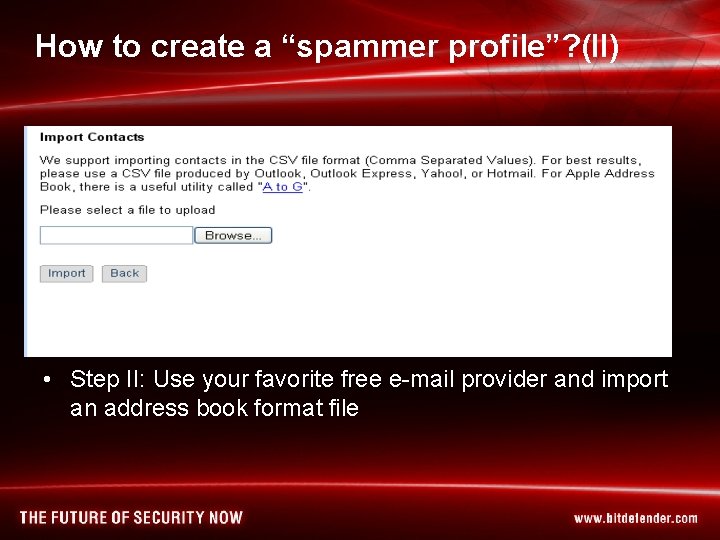
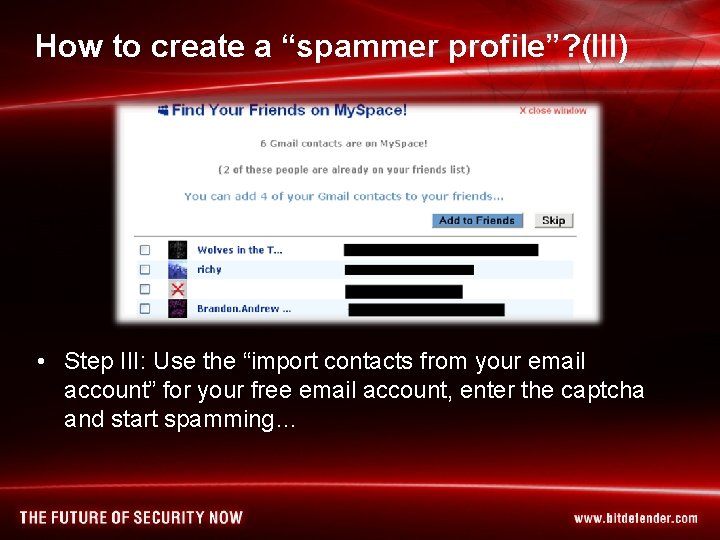
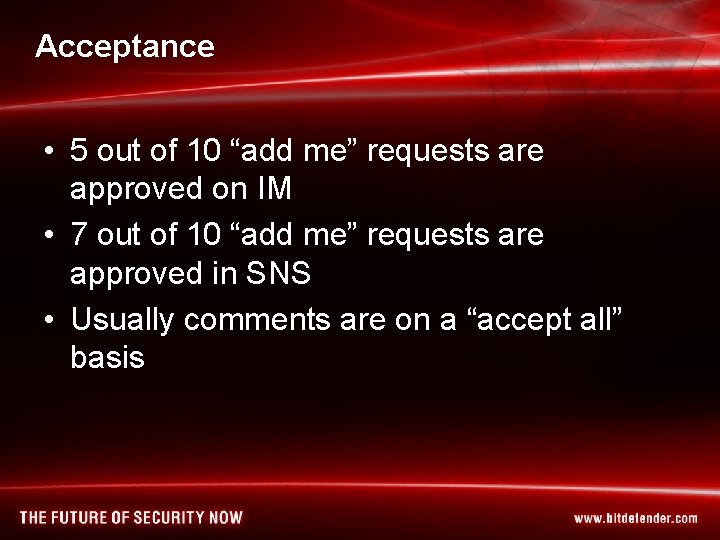
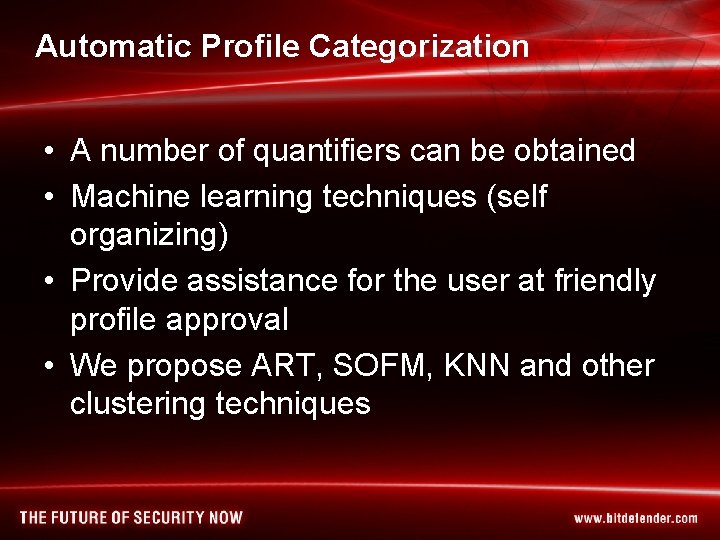
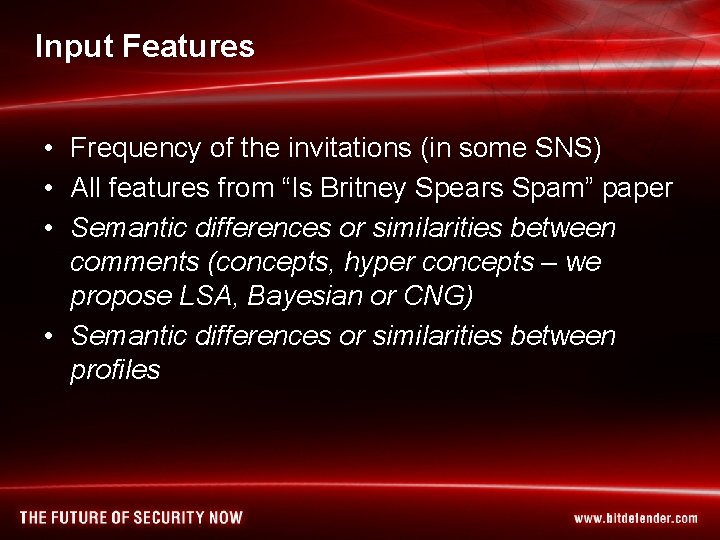
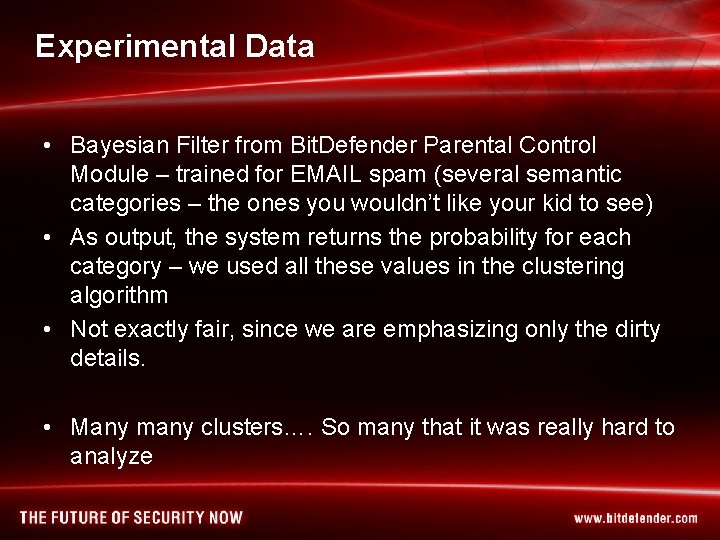
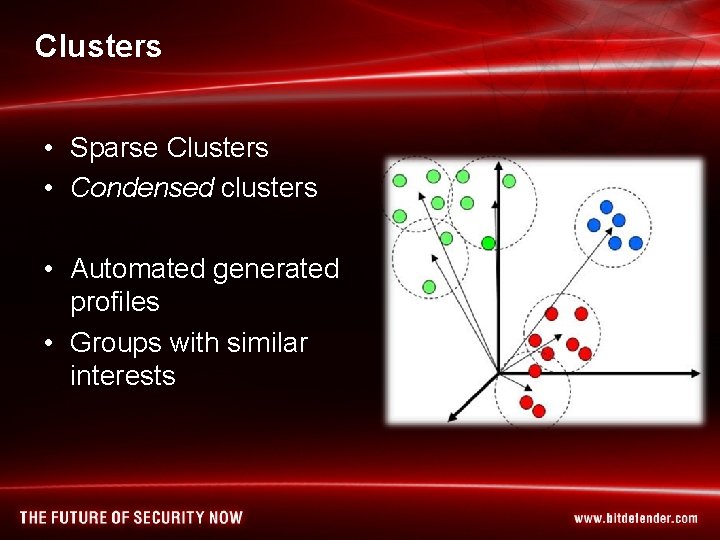
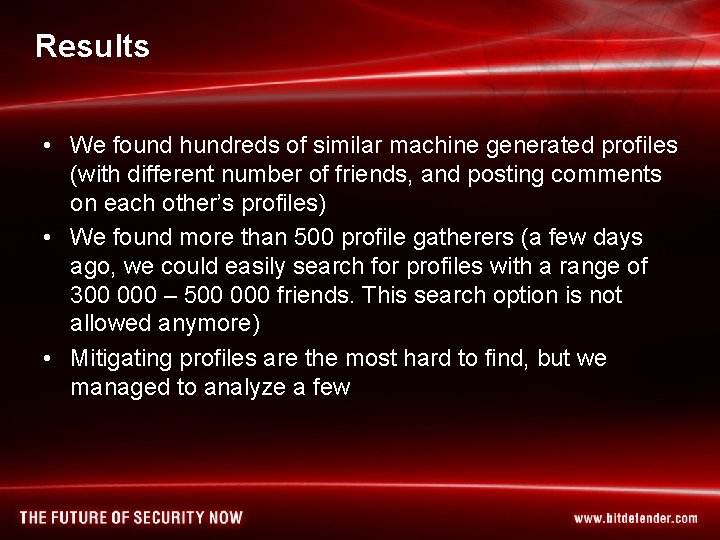
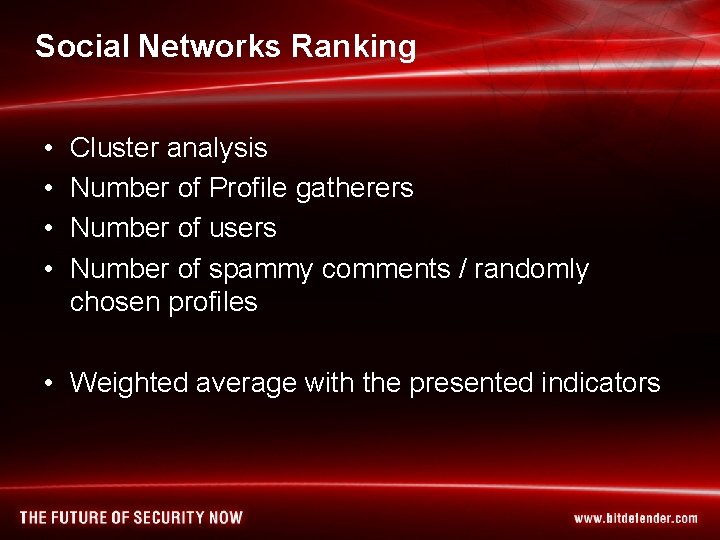
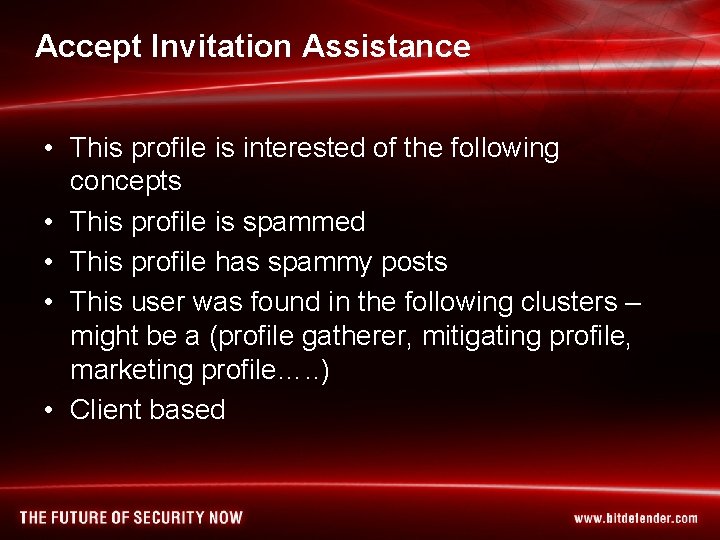
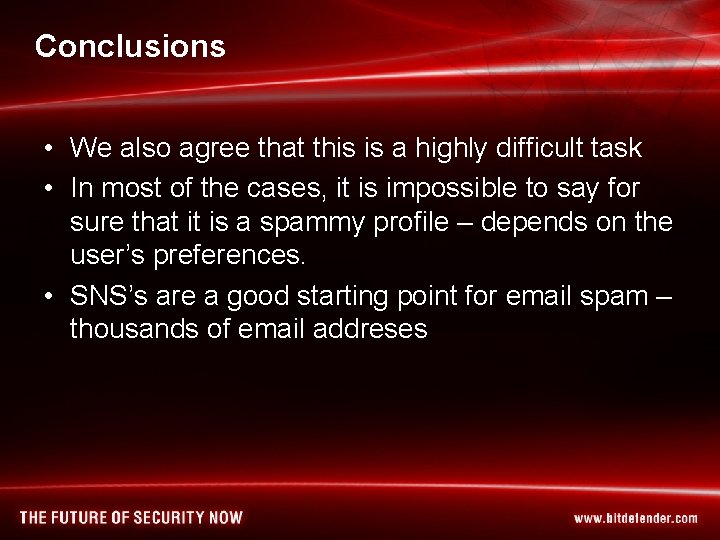
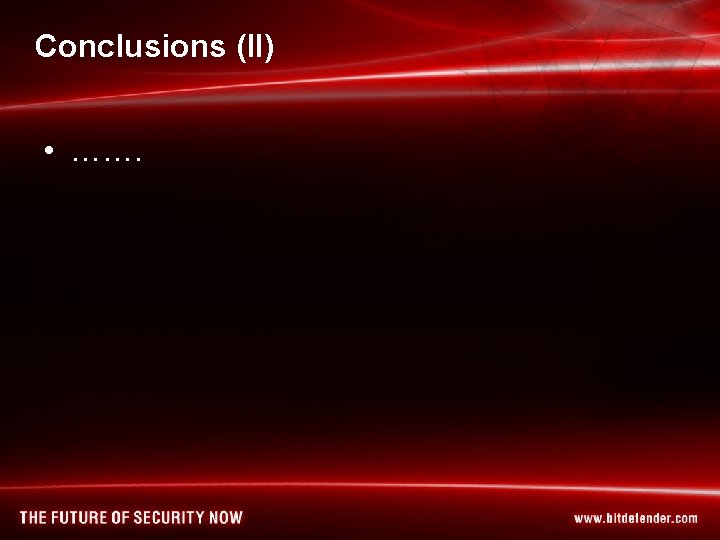
- Slides: 35
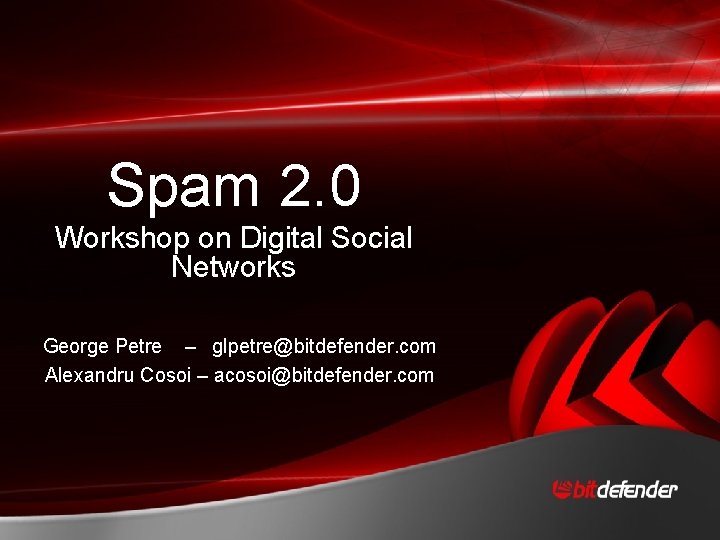
Spam 2. 0 Workshop on Digital Social Networks George Petre – glpetre@bitdefender. com Alexandru Cosoi – acosoi@bitdefender. com
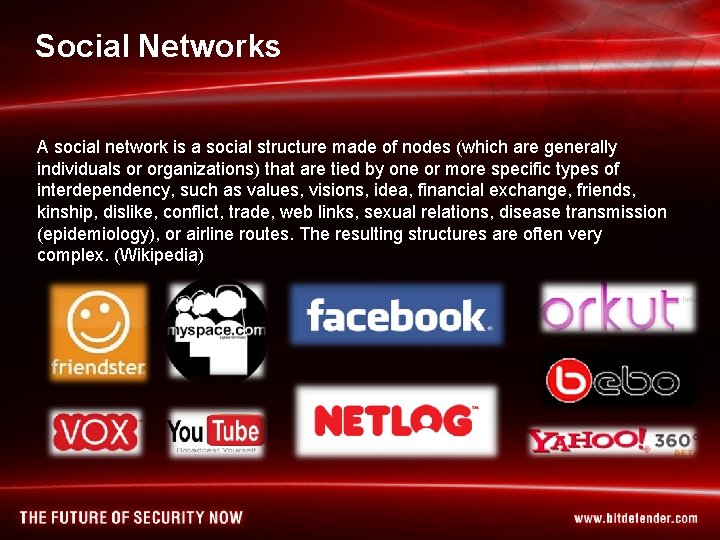
Social Networks A social network is a social structure made of nodes (which are generally individuals or organizations) that are tied by one or more specific types of interdependency, such as values, visions, idea, financial exchange, friends, kinship, dislike, conflict, trade, web links, sexual relations, disease transmission (epidemiology), or airline routes. The resulting structures are often very complex. (Wikipedia)
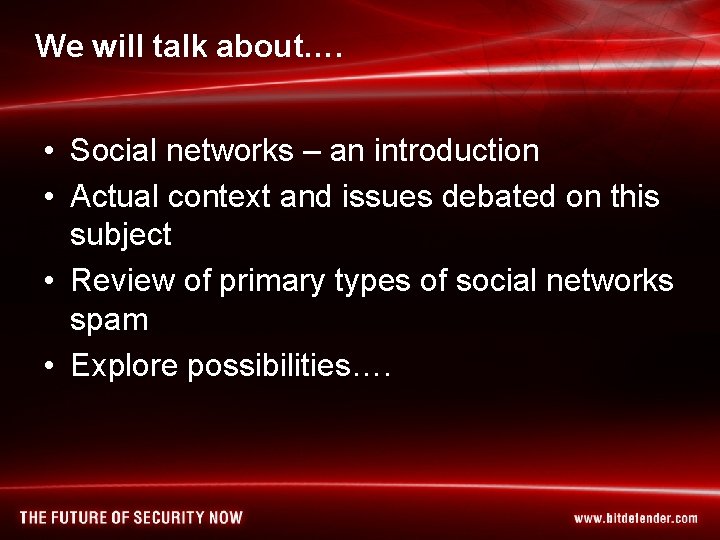
We will talk about…. • Social networks – an introduction • Actual context and issues debated on this subject • Review of primary types of social networks spam • Explore possibilities….
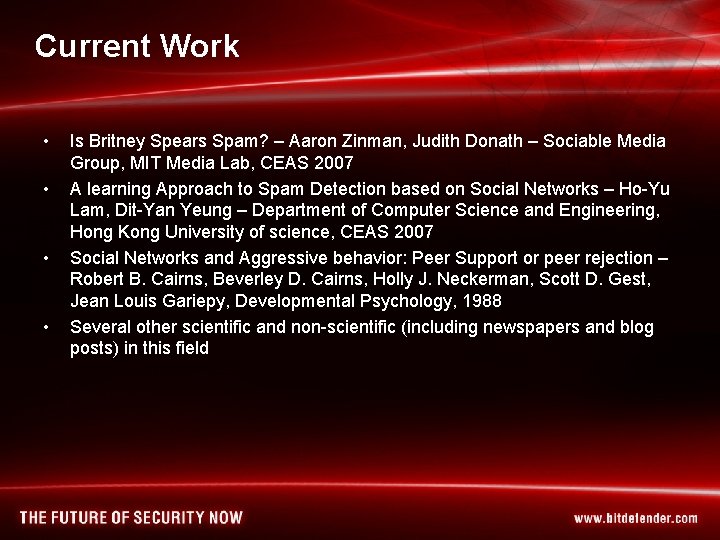
Current Work • • Is Britney Spears Spam? – Aaron Zinman, Judith Donath – Sociable Media Group, MIT Media Lab, CEAS 2007 A learning Approach to Spam Detection based on Social Networks – Ho-Yu Lam, Dit-Yan Yeung – Department of Computer Science and Engineering, Hong Kong University of science, CEAS 2007 Social Networks and Aggressive behavior: Peer Support or peer rejection – Robert B. Cairns, Beverley D. Cairns, Holly J. Neckerman, Scott D. Gest, Jean Louis Gariepy, Developmental Psychology, 1988 Several other scientific and non-scientific (including newspapers and blog posts) in this field
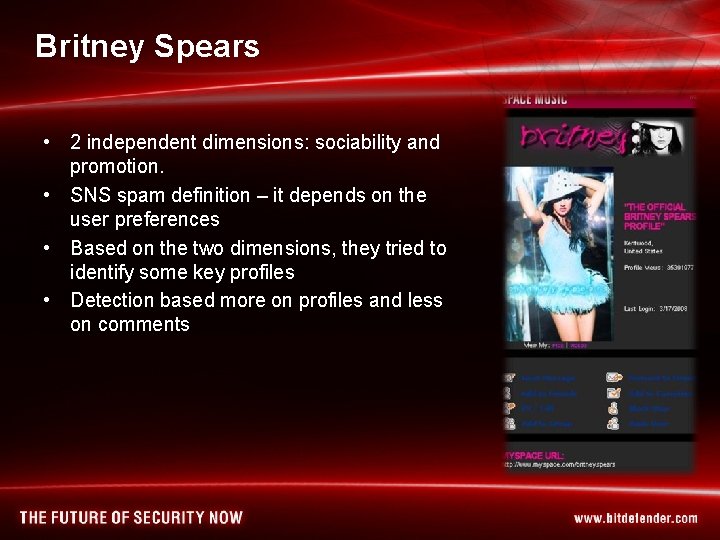
Britney Spears • 2 independent dimensions: sociability and promotion. • SNS spam definition – it depends on the user preferences • Based on the two dimensions, they tried to identify some key profiles • Detection based more on profiles and less on comments
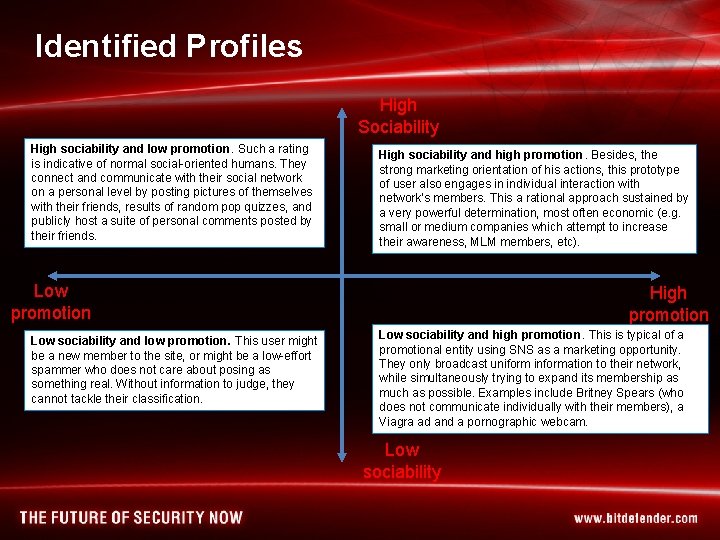
Identified Profiles High Sociability High sociability and low promotion. Such a rating is indicative of normal social-oriented humans. They connect and communicate with their social network on a personal level by posting pictures of themselves with their friends, results of random pop quizzes, and publicly host a suite of personal comments posted by their friends. High sociability and high promotion. Besides, the strong marketing orientation of his actions, this prototype of user also engages in individual interaction with network’s members. This a rational approach sustained by a very powerful determination, most often economic (e. g. small or medium companies which attempt to increase their awareness, MLM members, etc). Low promotion Low sociability and low promotion. This user might be a new member to the site, or might be a low-effort spammer who does not care about posing as something real. Without information to judge, they cannot tackle their classification. High promotion Low sociability and high promotion. This is typical of a promotional entity using SNS as a marketing opportunity. They only broadcast uniform information to their network, while simultaneously trying to expand its membership as much as possible. Examples include Britney Spears (who does not communicate individually with their members), a Viagra ad and a pornographic webcam. Low sociability
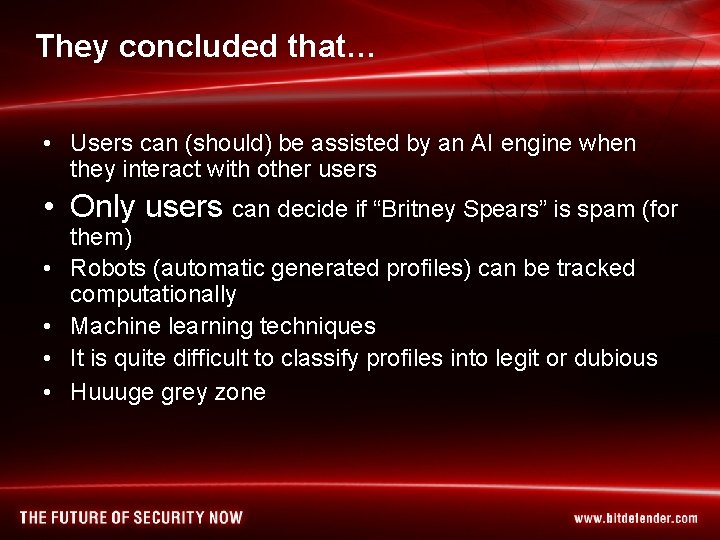
They concluded that… • Users can (should) be assisted by an AI engine when they interact with other users • Only users can decide if “Britney Spears” is spam (for • • them) Robots (automatic generated profiles) can be tracked computationally Machine learning techniques It is quite difficult to classify profiles into legit or dubious Huuuge grey zone
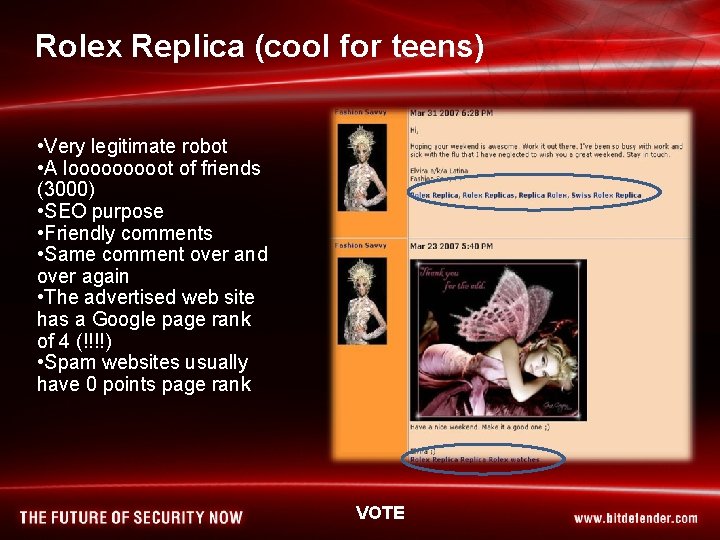
Rolex Replica (cool for teens) • Very legitimate robot • A looooot of friends (3000) • SEO purpose • Friendly comments • Same comment over and over again • The advertised web site has a Google page rank of 4 (!!!!) • Spam websites usually have 0 points page rank VOTE
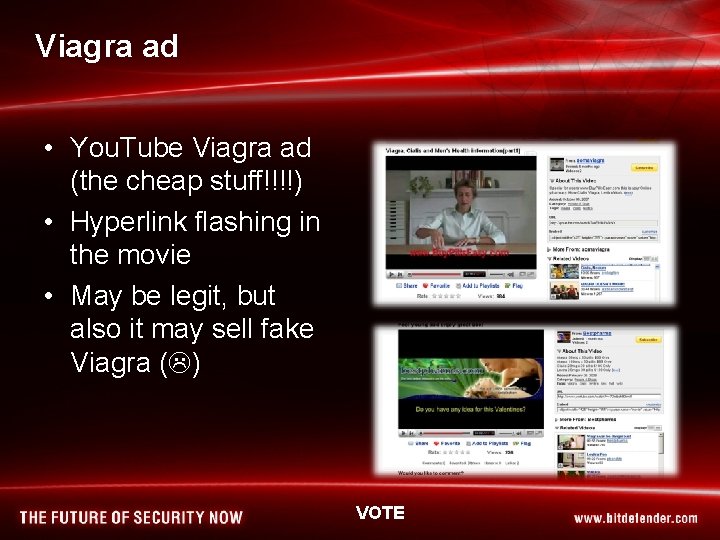
Viagra ad • You. Tube Viagra ad (the cheap stuff!!!!) • Hyperlink flashing in the movie • May be legit, but also it may sell fake Viagra ( ) VOTE
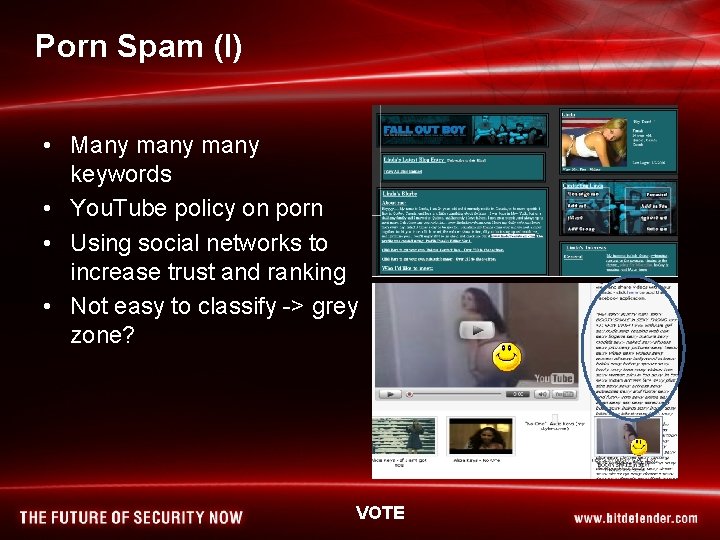
Porn Spam (I) • Many many keywords • You. Tube policy on porn • Using social networks to increase trust and ranking • Not easy to classify -> grey zone? VOTE
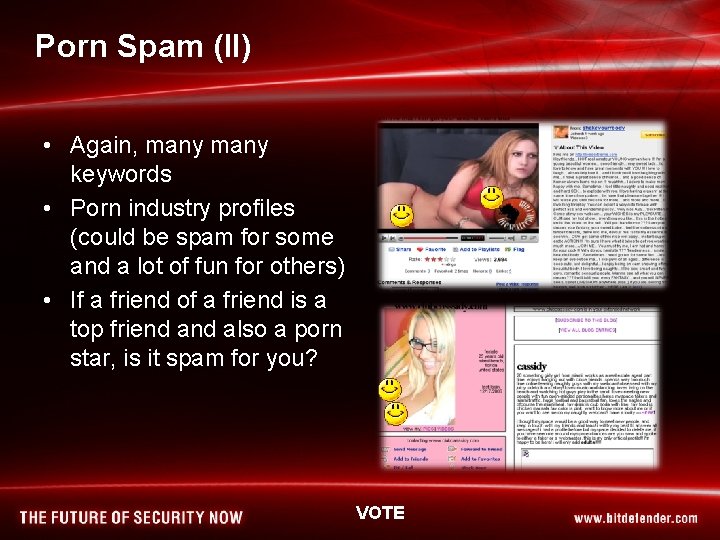
Porn Spam (II) • Again, many keywords • Porn industry profiles (could be spam for some and a lot of fun for others) • If a friend of a friend is a top friend also a porn star, is it spam for you? VOTE
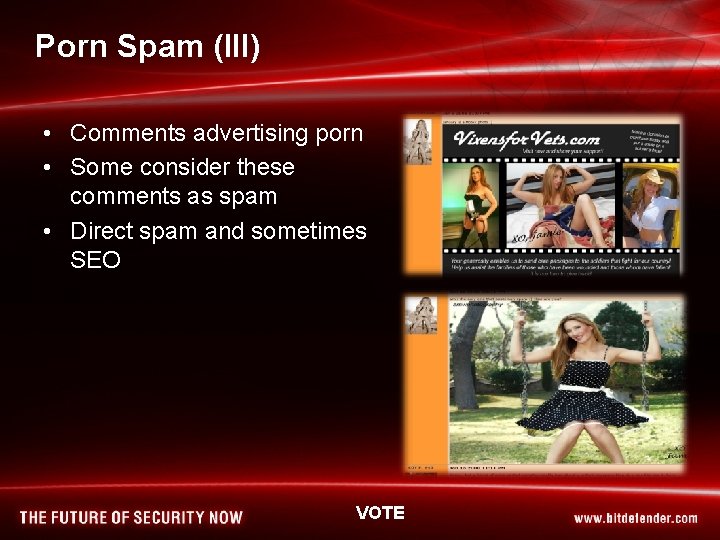
Porn Spam (III) • Comments advertising porn • Some consider these comments as spam • Direct spam and sometimes SEO VOTE
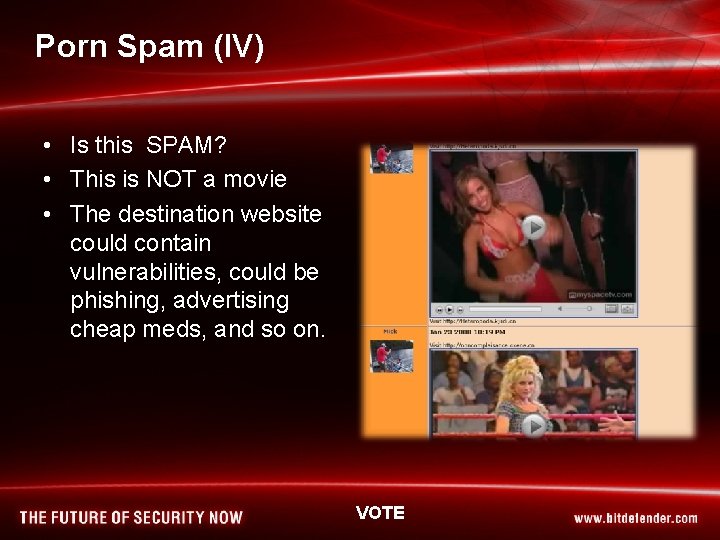
Porn Spam (IV) • Is this SPAM? • This is NOT a movie • The destination website could contain vulnerabilities, could be phishing, advertising cheap meds, and so on. VOTE
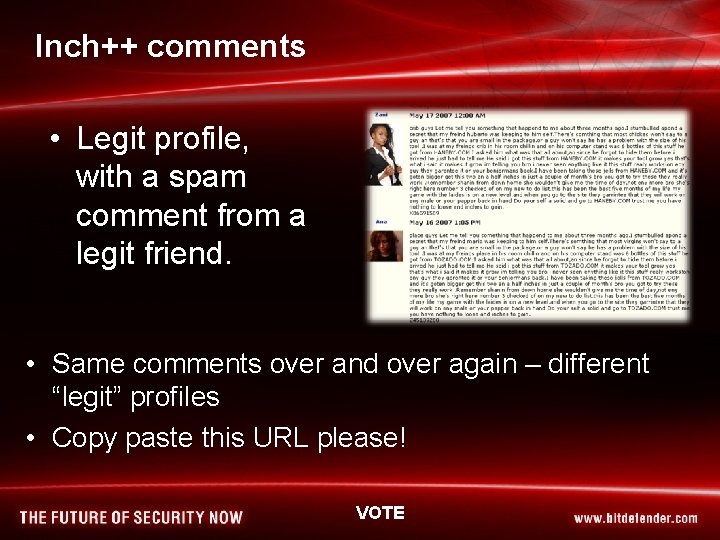
Inch++ comments • Legit profile, with a spam comment from a legit friend. • Same comments over and over again – different “legit” profiles • Copy paste this URL please! VOTE
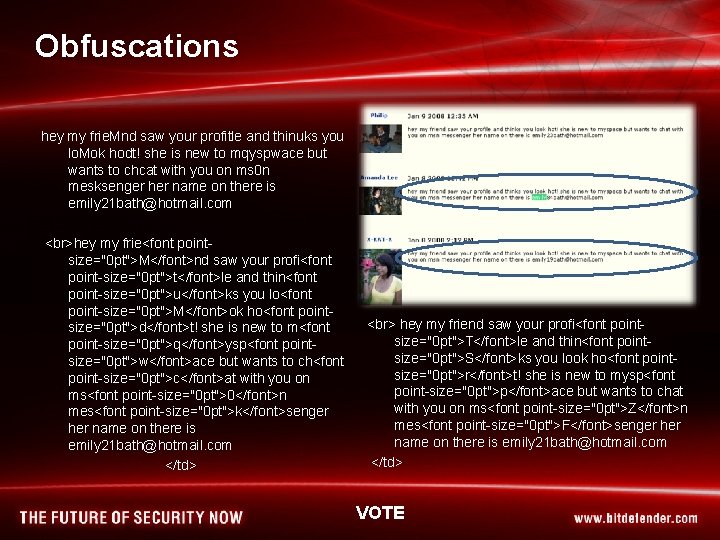
Obfuscations hey my frie. Mnd saw your profitle and thinuks you lo. Mok hodt! she is new to mqyspwace but wants to chcat with you on ms 0 n mesksenger her name on there is emily 21 bath@hotmail. com hey my frie<font pointsize="0 pt">M</font>nd saw your profi<font point-size="0 pt">t</font>le and thin<font point-size="0 pt">u</font>ks you lo<font point-size="0 pt">M</font>ok ho<font pointsize="0 pt">d</font>t! she is new to m<font point-size="0 pt">q</font>ysp<font pointsize="0 pt">w</font>ace but wants to ch<font point-size="0 pt">c</font>at with you on ms<font point-size="0 pt">0</font>n mes<font point-size="0 pt">k</font>senger her name on there is emily 21 bath@hotmail. com </td> hey my friend saw your profi<font pointsize="0 pt">T</font>le and thin<font pointsize="0 pt">S</font>ks you look ho<font pointsize="0 pt">r</font>t! she is new to mysp<font point-size="0 pt">p</font>ace but wants to chat with you on ms<font point-size="0 pt">Z</font>n mes<font point-size="0 pt">F</font>senger her name on there is emily 21 bath@hotmail. com </td> VOTE
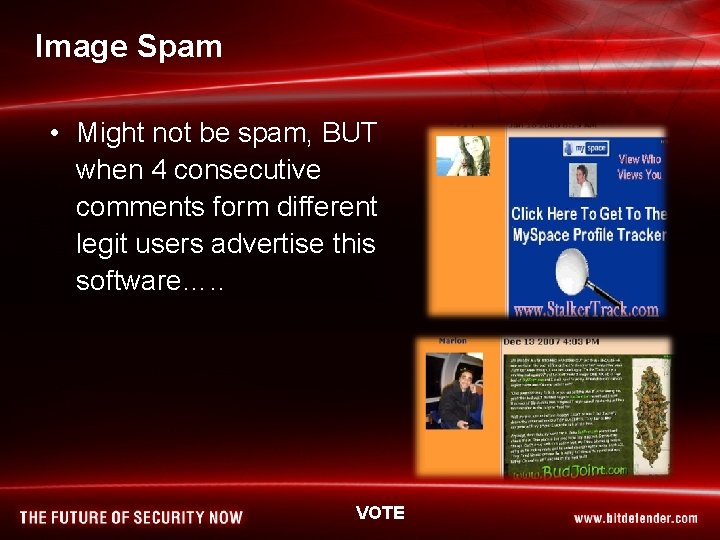
Image Spam • Might not be spam, BUT when 4 consecutive comments form different legit users advertise this software…. . VOTE
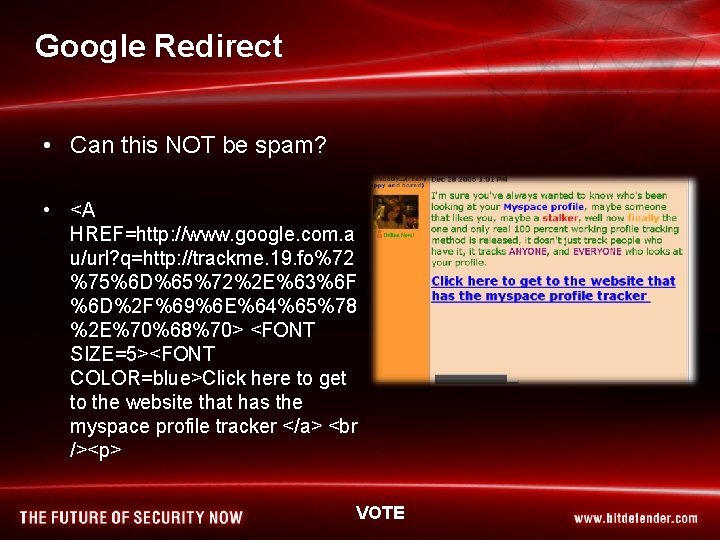
Google Redirect • Can this NOT be spam? • <A HREF=http: //www. google. com. a u/url? q=http: //trackme. 19. fo%72 %75%6 D%65%72%2 E%63%6 F %6 D%2 F%69%6 E%64%65%78 %2 E%70%68%70> <FONT SIZE=5><FONT COLOR=blue>Click here to get to the website that has the myspace profile tracker </a> <br /><p> VOTE
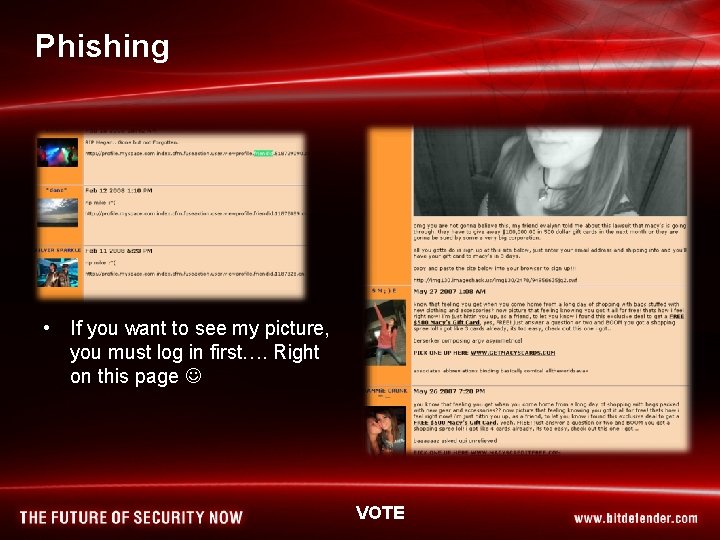
Phishing • If you want to see my picture, you must log in first…. Right on this page VOTE
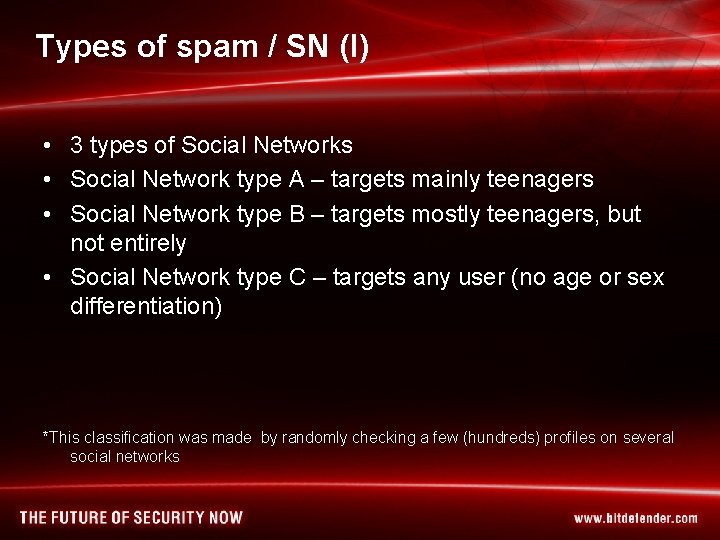
Types of spam / SN (I) • 3 types of Social Networks • Social Network type A – targets mainly teenagers • Social Network type B – targets mostly teenagers, but not entirely • Social Network type C – targets any user (no age or sex differentiation) *This classification was made by randomly checking a few (hundreds) profiles on several social networks
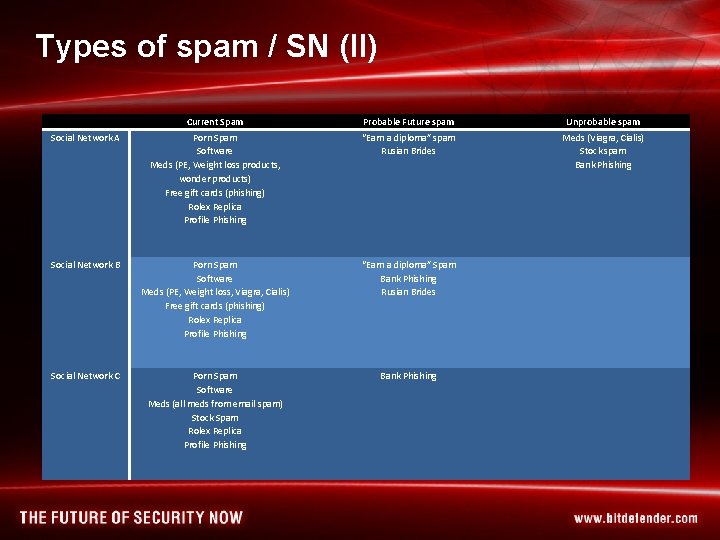
Types of spam / SN (II) Current Spam Probable Future spam Unprobable spam Social Network A Porn Spam Software Meds (PE, Weight loss products, wonder products) Free gift cards (phishing) Rolex Replica Profile Phishing “Earn a diploma” spam Rusian Brides Meds (Viagra, Cialis) Stock spam Bank Phishing Social Network B Porn Spam Software Meds (PE, Weight loss, Viagra, Cialis) Free gift cards (phishing) Rolex Replica Profile Phishing “Earn a diploma” Spam Bank Phishing Rusian Brides Social Network C Porn Spam Software Meds (all meds from email spam) Stock Spam Rolex Replica Profile Phishing Bank Phishing
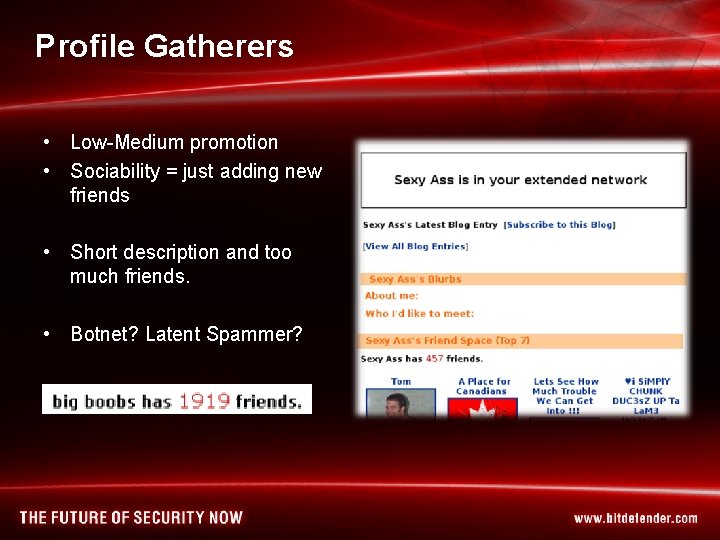
Profile Gatherers • Low-Medium promotion • Sociability = just adding new friends • Short description and too much friends. • Botnet? Latent Spammer?
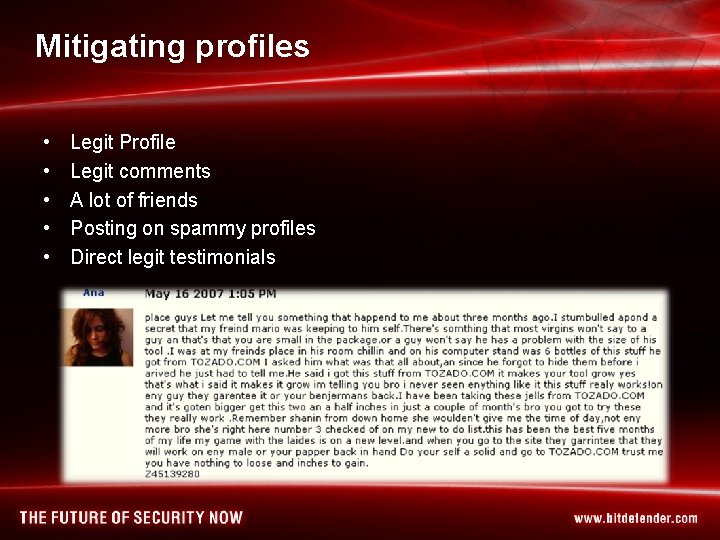
Mitigating profiles • • • Legit Profile Legit comments A lot of friends Posting on spammy profiles Direct legit testimonials
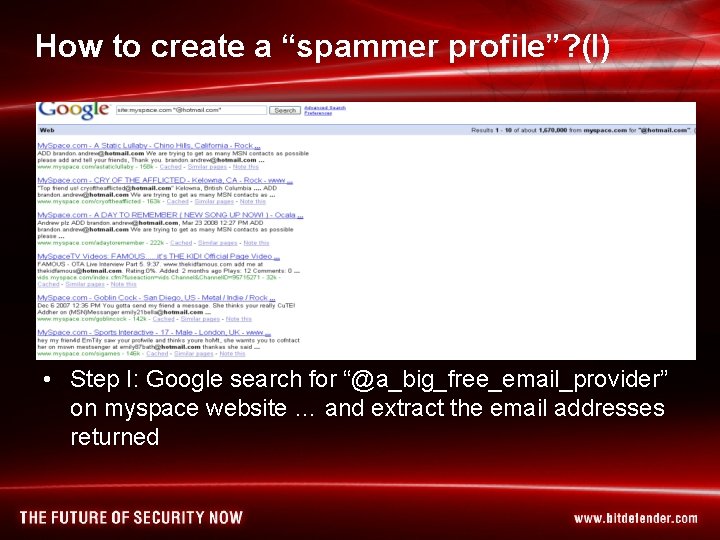
How to create a “spammer profile”? (I) • Step I: Google search for “@a_big_free_email_provider” on myspace website … and extract the email addresses returned
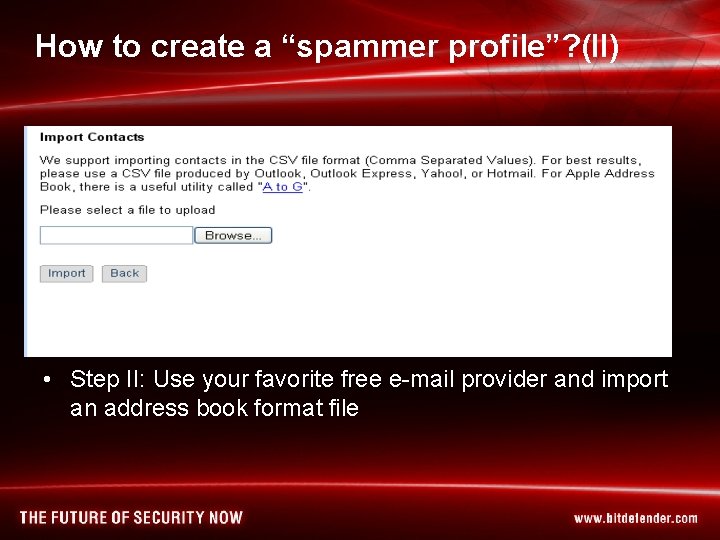
How to create a “spammer profile”? (II) • Step II: Use your favorite free e-mail provider and import an address book format file
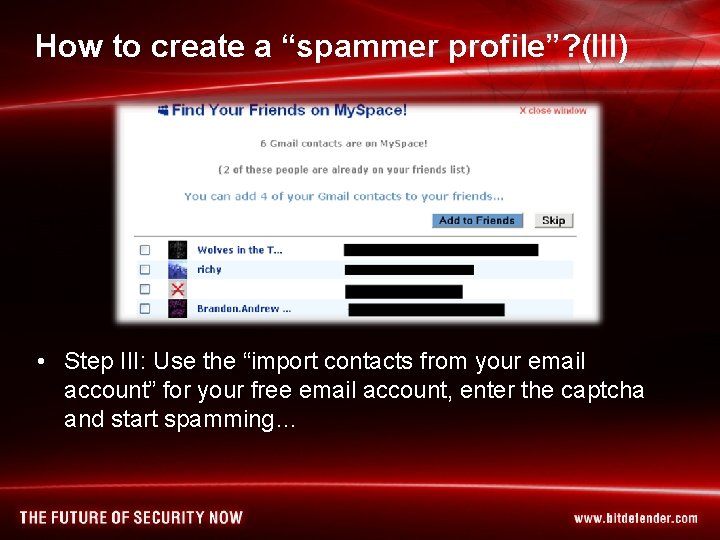
How to create a “spammer profile”? (III) • Step III: Use the “import contacts from your email account” for your free email account, enter the captcha and start spamming…
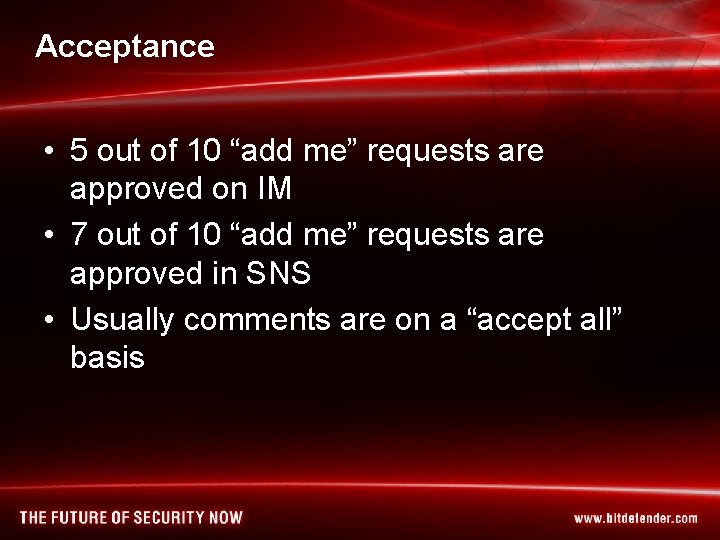
Acceptance • 5 out of 10 “add me” requests are approved on IM • 7 out of 10 “add me” requests are approved in SNS • Usually comments are on a “accept all” basis
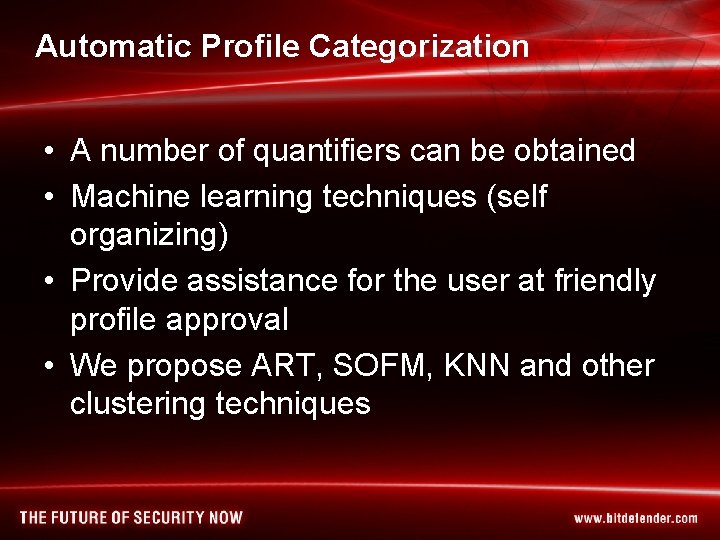
Automatic Profile Categorization • A number of quantifiers can be obtained • Machine learning techniques (self organizing) • Provide assistance for the user at friendly profile approval • We propose ART, SOFM, KNN and other clustering techniques
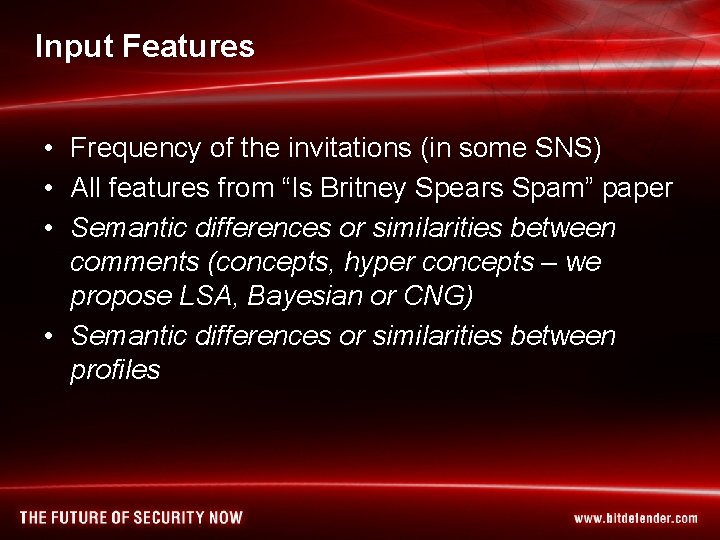
Input Features • Frequency of the invitations (in some SNS) • All features from “Is Britney Spears Spam” paper • Semantic differences or similarities between comments (concepts, hyper concepts – we propose LSA, Bayesian or CNG) • Semantic differences or similarities between profiles
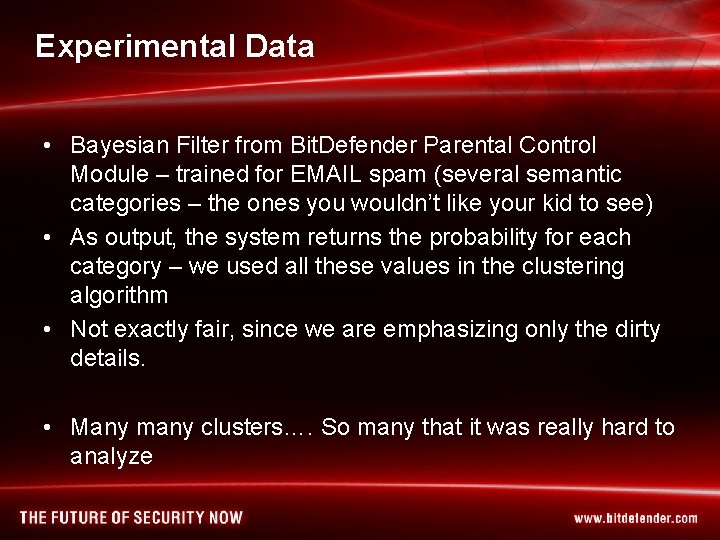
Experimental Data • Bayesian Filter from Bit. Defender Parental Control Module – trained for EMAIL spam (several semantic categories – the ones you wouldn’t like your kid to see) • As output, the system returns the probability for each category – we used all these values in the clustering algorithm • Not exactly fair, since we are emphasizing only the dirty details. • Many many clusters…. So many that it was really hard to analyze
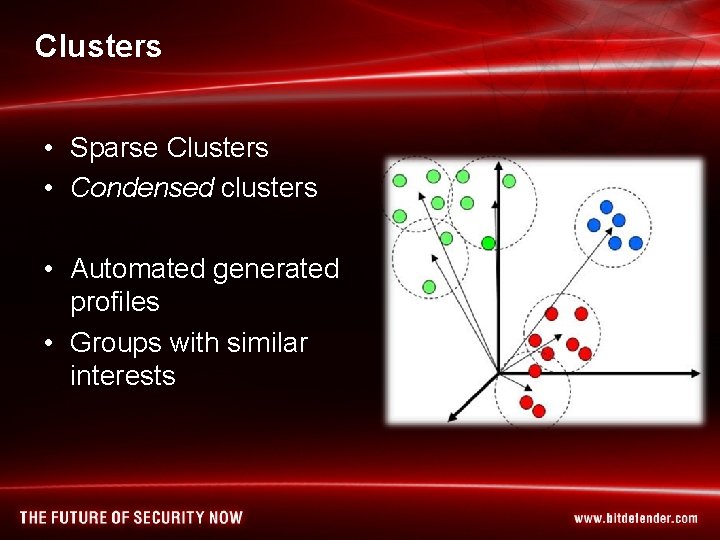
Clusters • Sparse Clusters • Condensed clusters • Automated generated profiles • Groups with similar interests
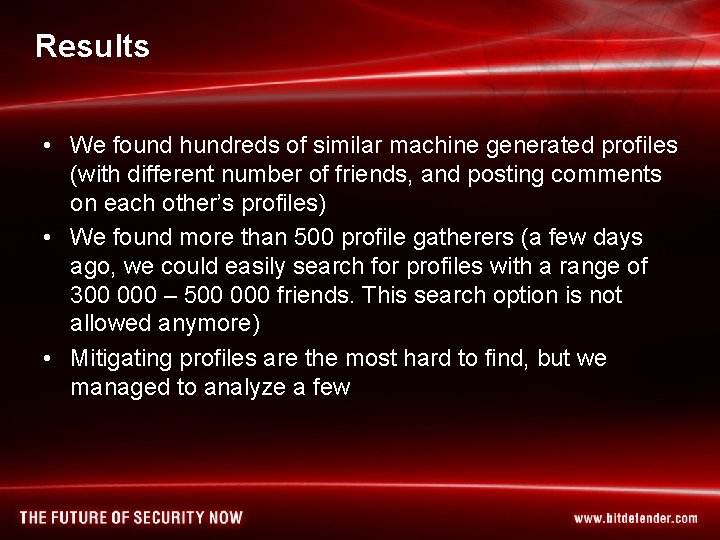
Results • We found hundreds of similar machine generated profiles (with different number of friends, and posting comments on each other’s profiles) • We found more than 500 profile gatherers (a few days ago, we could easily search for profiles with a range of 300 000 – 500 000 friends. This search option is not allowed anymore) • Mitigating profiles are the most hard to find, but we managed to analyze a few
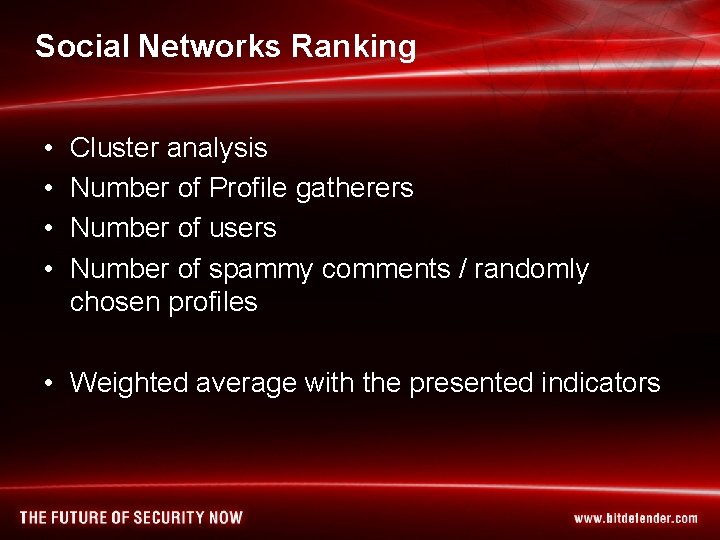
Social Networks Ranking • • Cluster analysis Number of Profile gatherers Number of users Number of spammy comments / randomly chosen profiles • Weighted average with the presented indicators
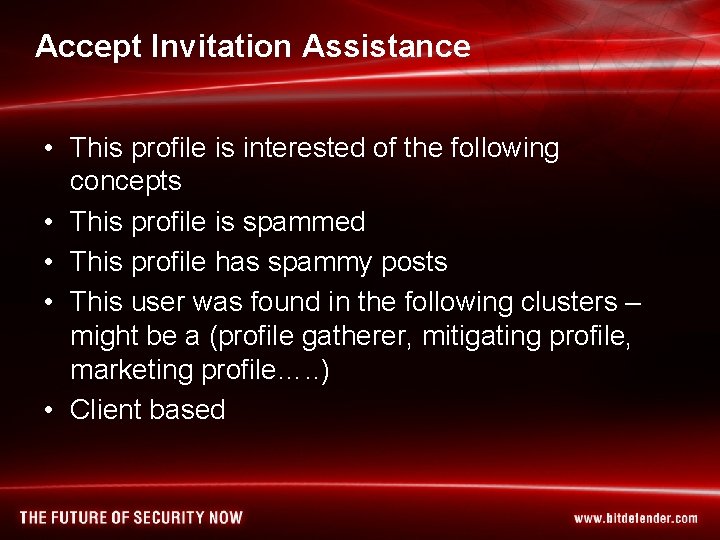
Accept Invitation Assistance • This profile is interested of the following concepts • This profile is spammed • This profile has spammy posts • This user was found in the following clusters – might be a (profile gatherer, mitigating profile, marketing profile…. . ) • Client based
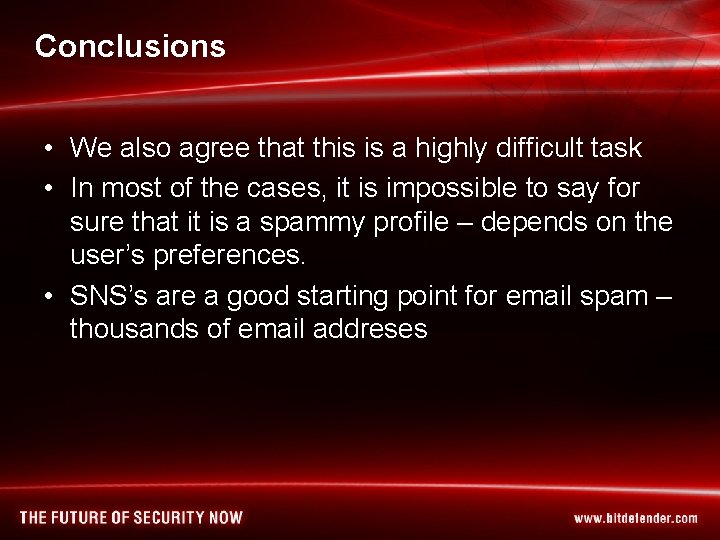
Conclusions • We also agree that this is a highly difficult task • In most of the cases, it is impossible to say for sure that it is a spammy profile – depends on the user’s preferences. • SNS’s are a good starting point for email spam – thousands of email addreses
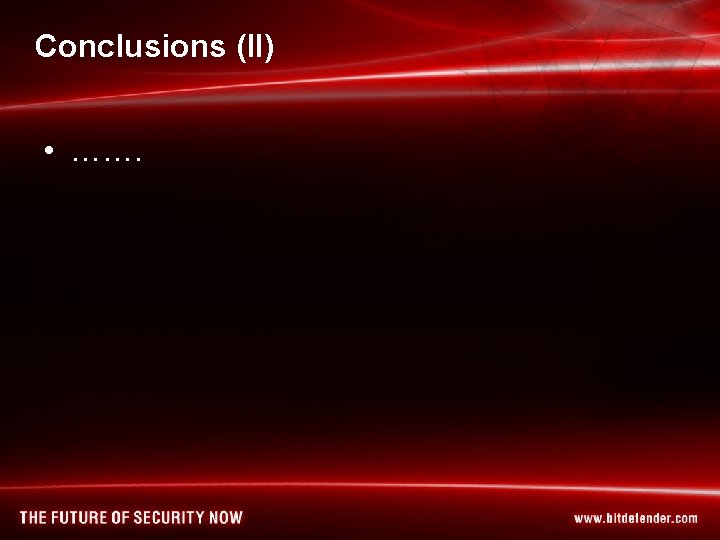
Conclusions (II) • …….
Difference between datagram and virtual circuit
Basestore iptv
Digital signage workshop
Digital communications and networks impact factor
Analog and digital signals in computer networking
What id spam
Komponen spam
Nie spam
Spam porn
"spam"
Spam bukan jaringan perpipaan
Standar kedalaman galian pipa gas
Spam
Nie spam
Perencanaan teknis spam
Max ciccotosto
Spam engineering
Nie spam
Spam
Spam
Spamato
Spam
Neogolism
Smsc anti spam
No te creas los chollos y los regalos
Mailcleaner-anti-spam-antivirus
Wait but why
Honey spam
Spam dosenfleisch
Spam filter
Spam
Spamproxy
Spam text 313131
Nevyžiadaná pošta spam
Nevyžiadaná pošta spam
Cox spam blocker