Research Methodology Dr Unnikrishnan P C Professor EEE
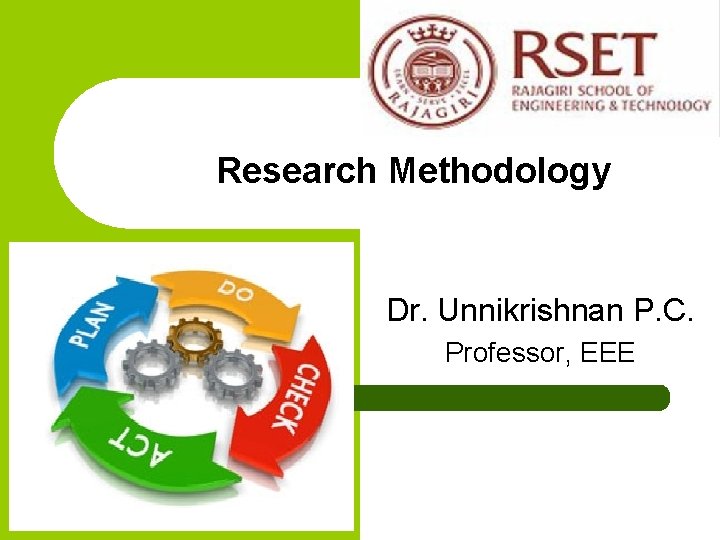
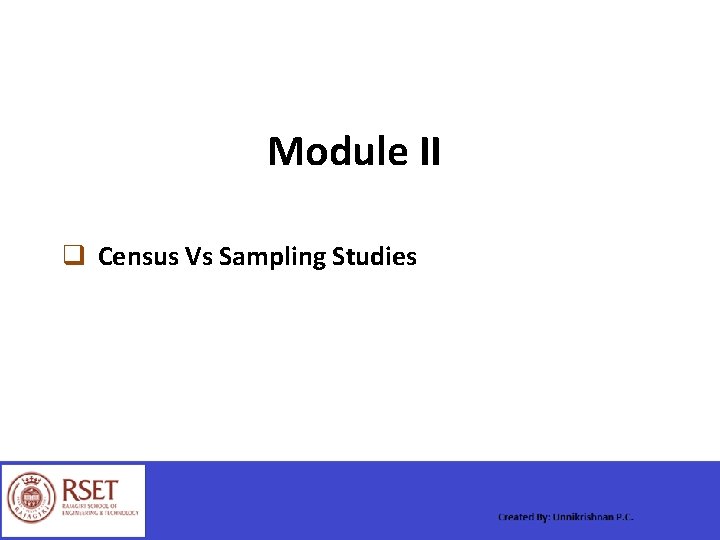
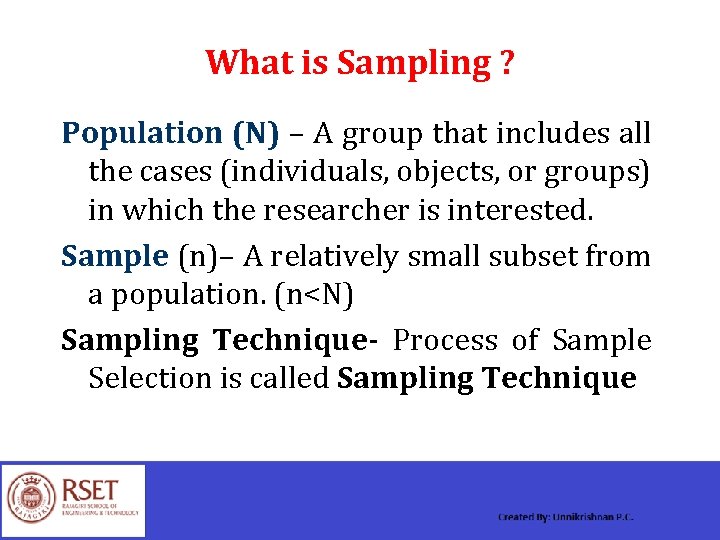
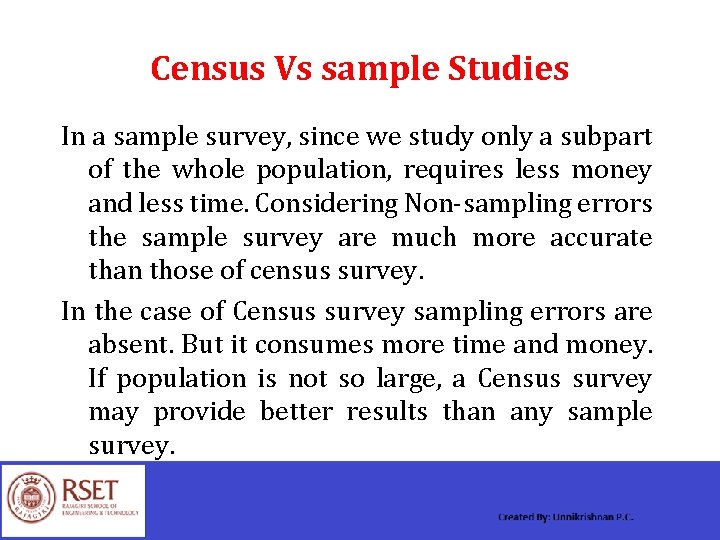
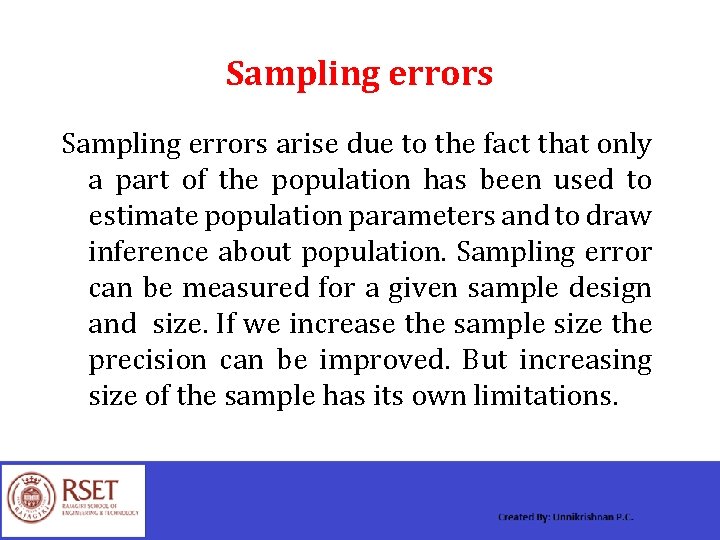
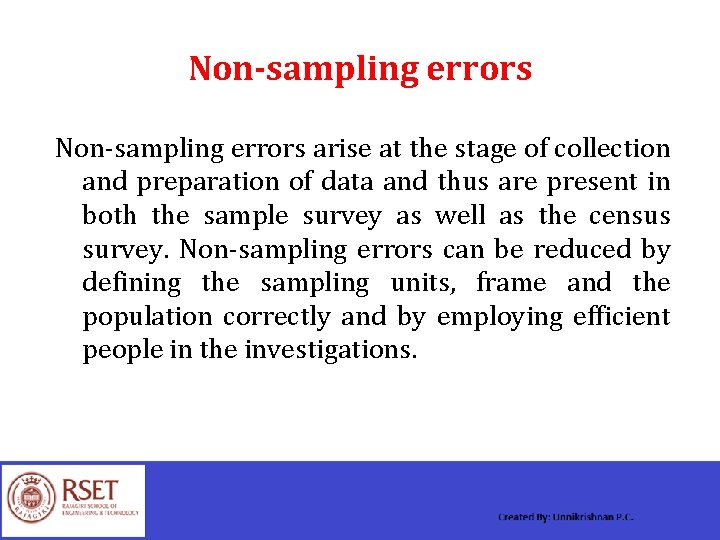
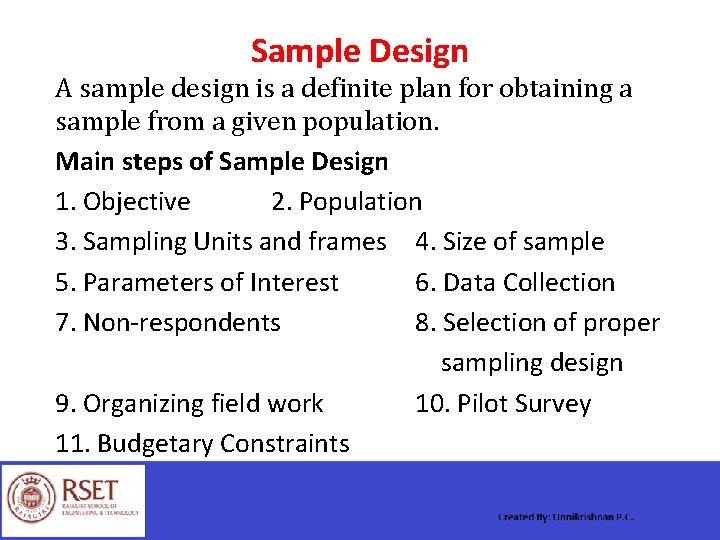
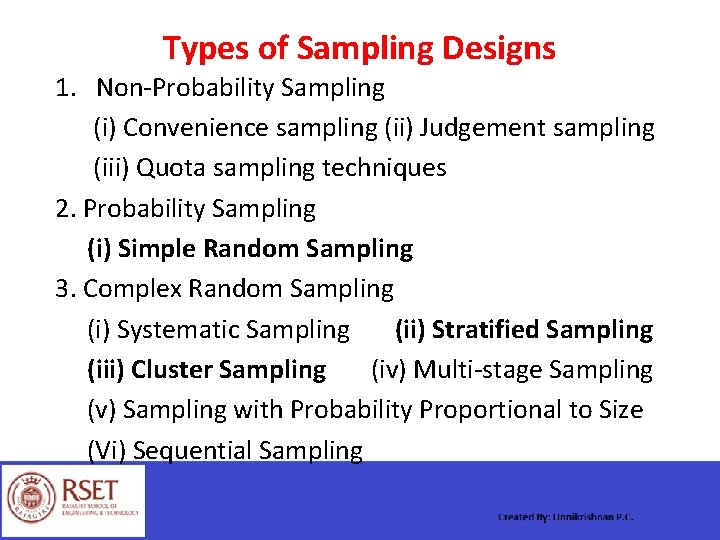
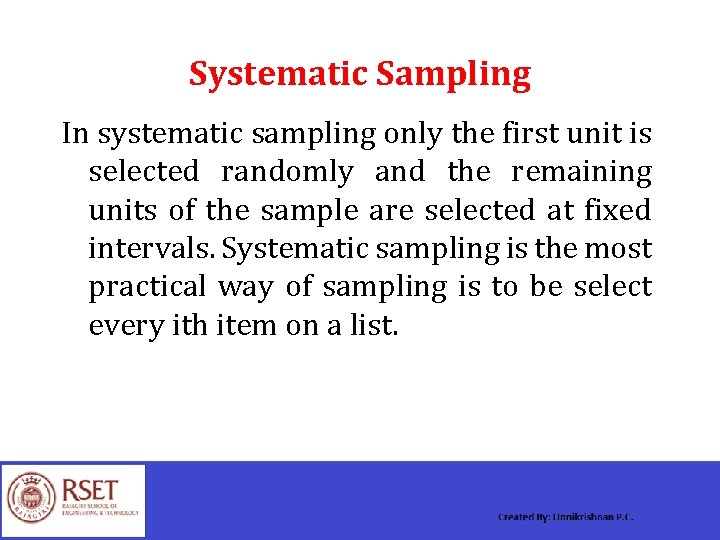
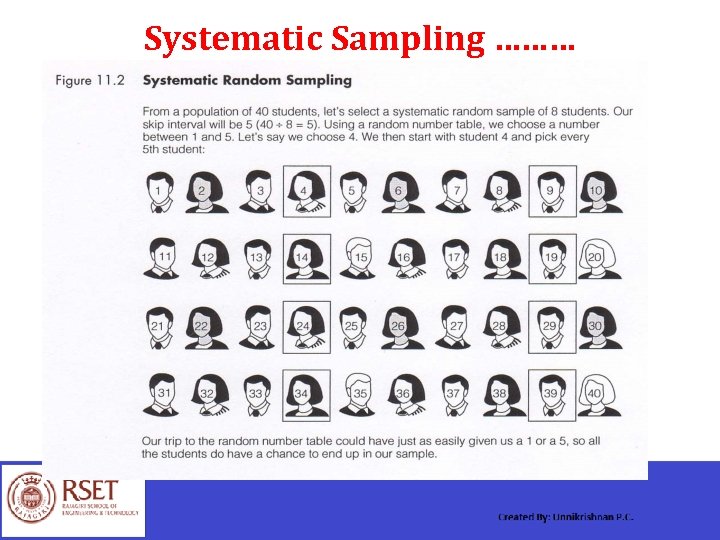
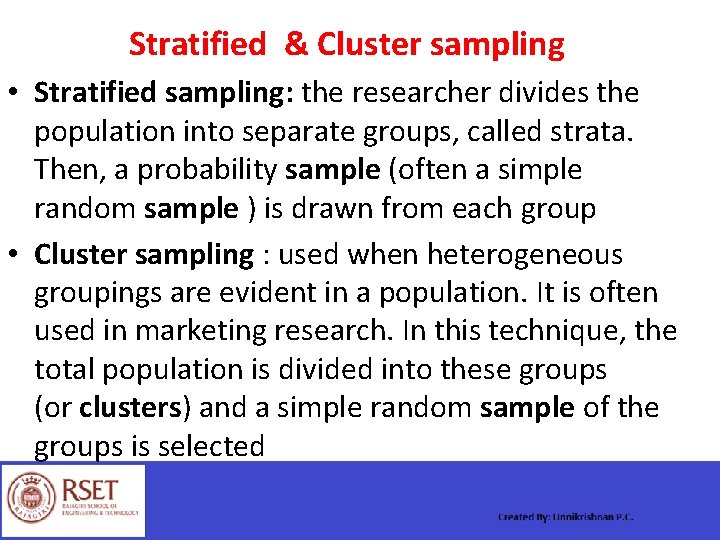
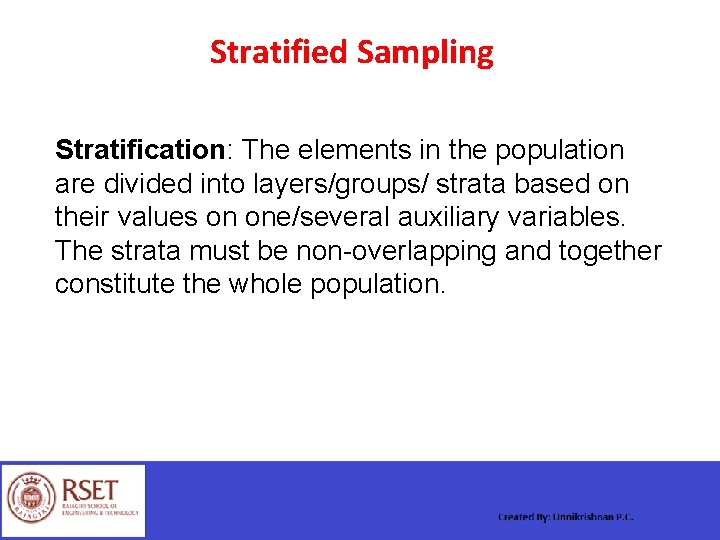
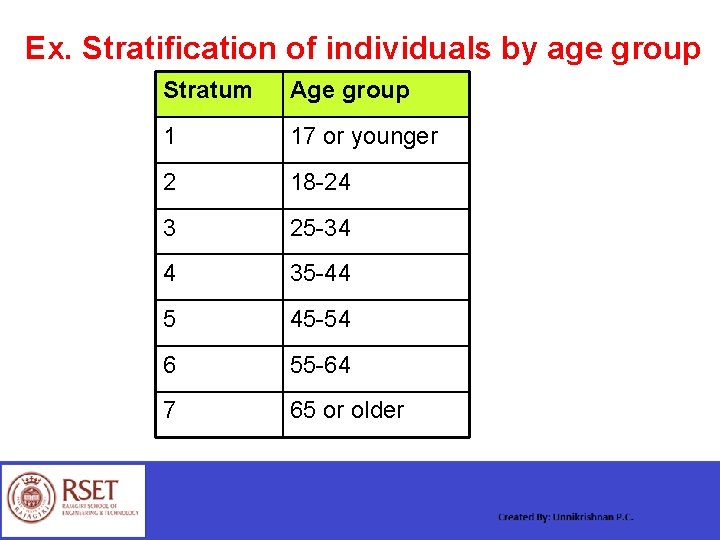
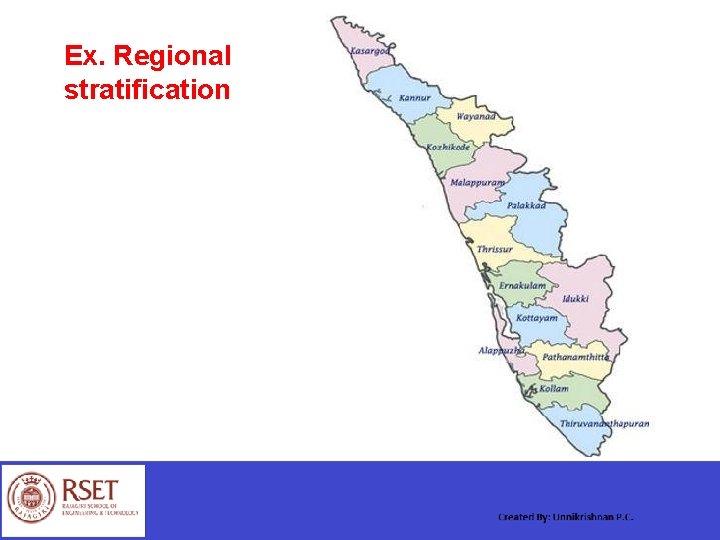
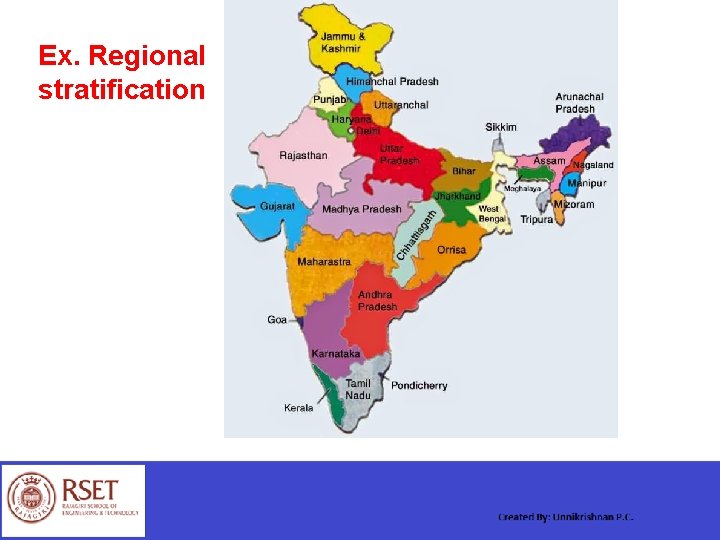
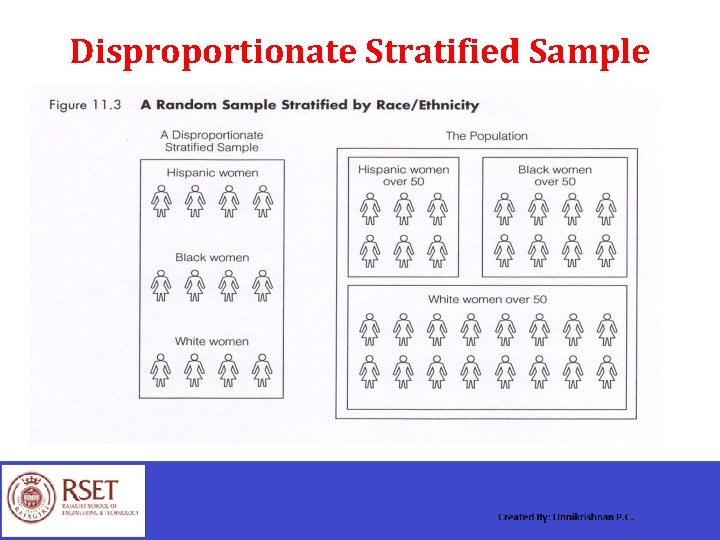
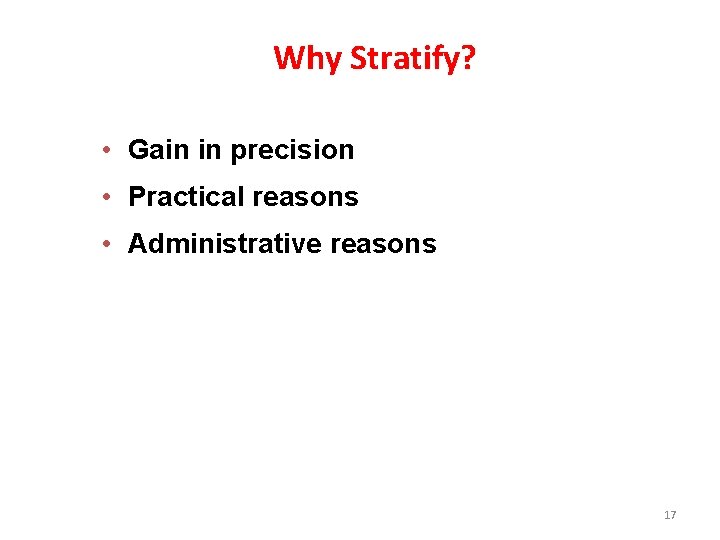
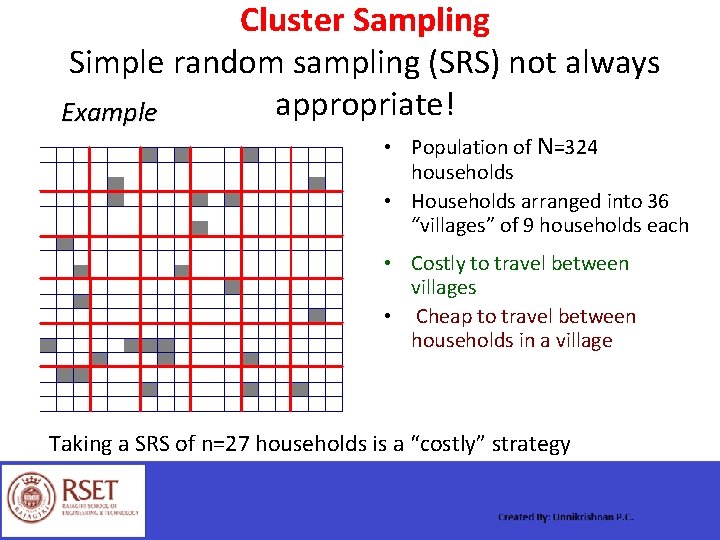
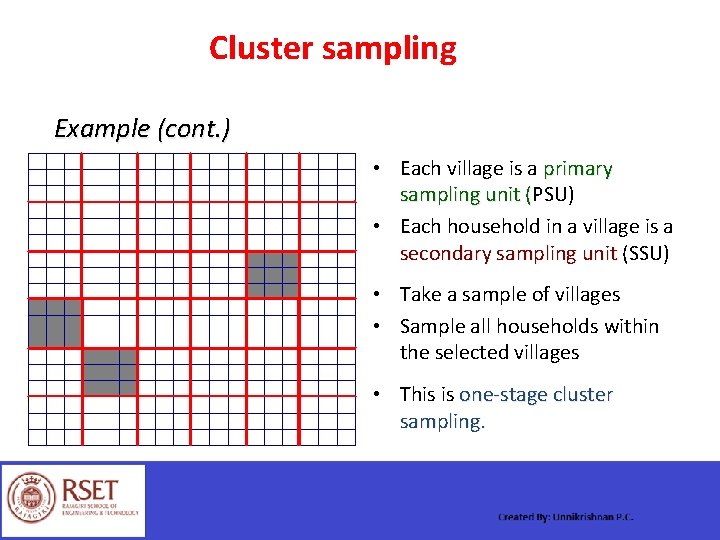
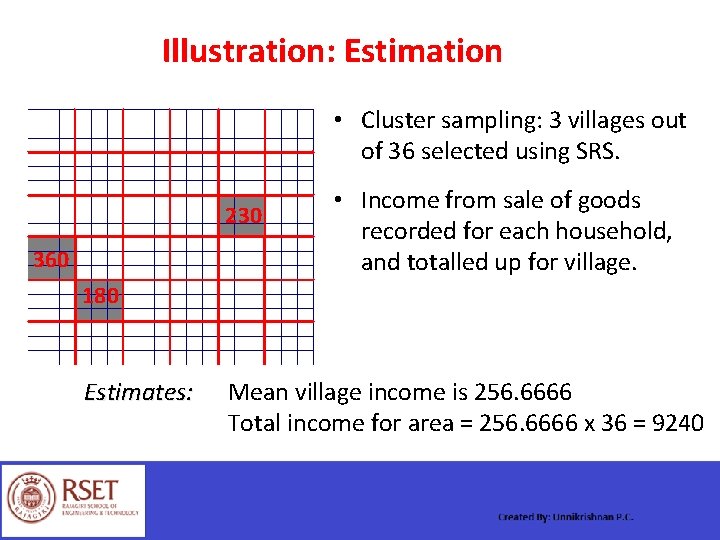
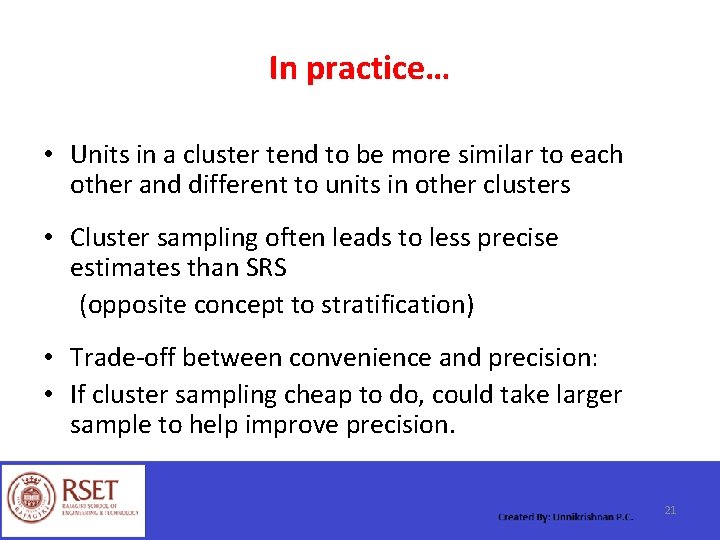
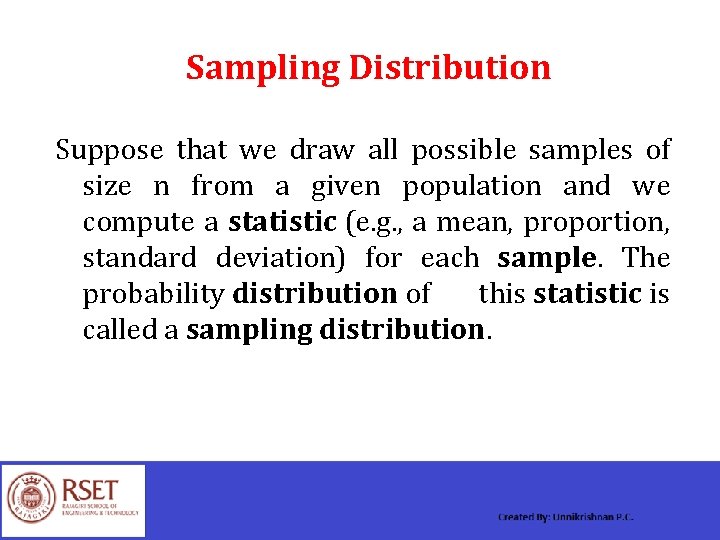
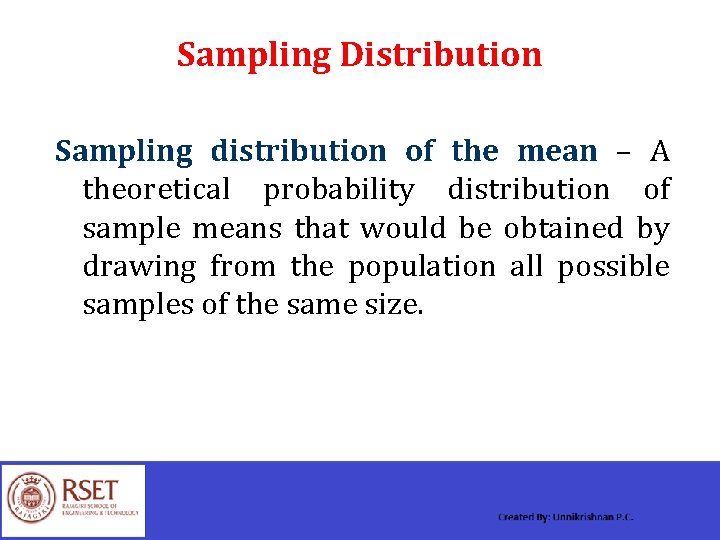
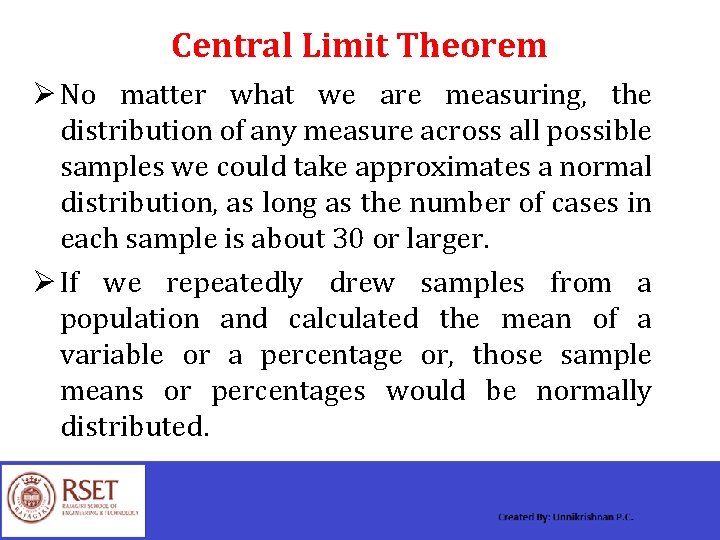
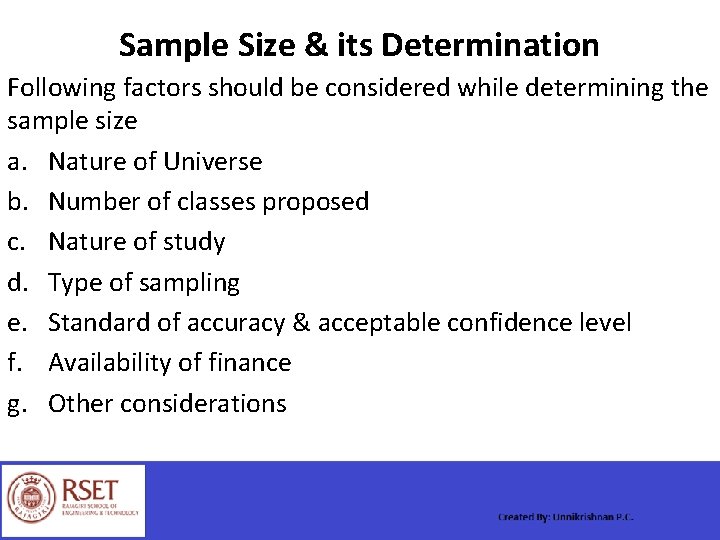
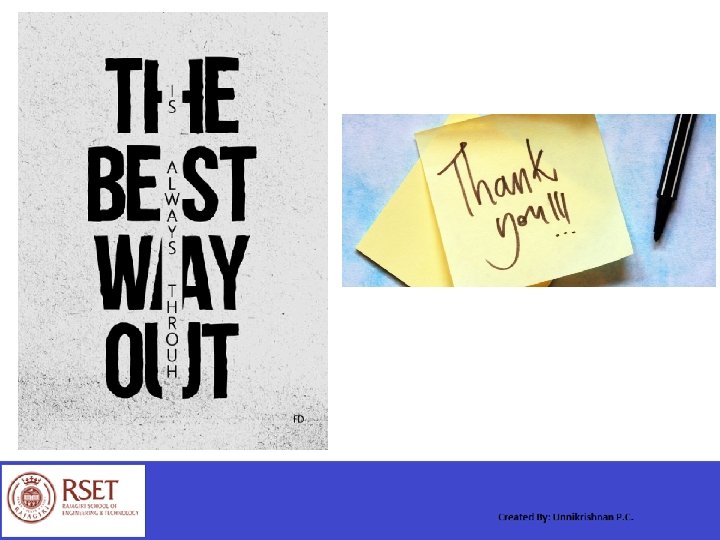
- Slides: 26
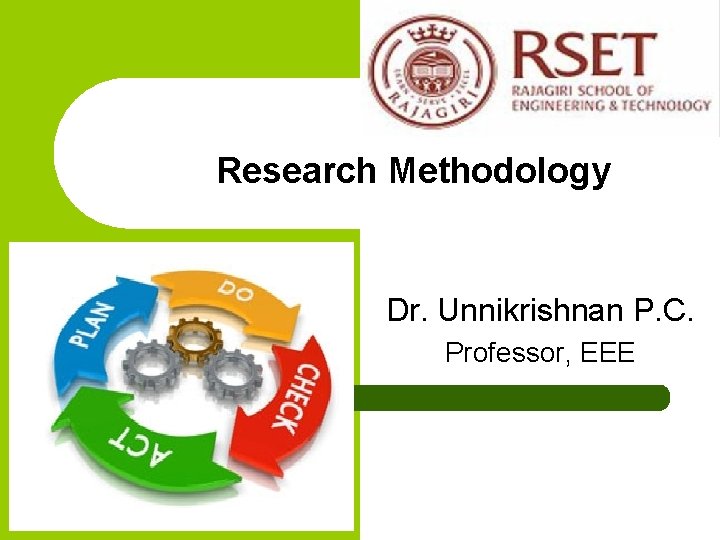
Research Methodology Dr. Unnikrishnan P. C. Professor, EEE
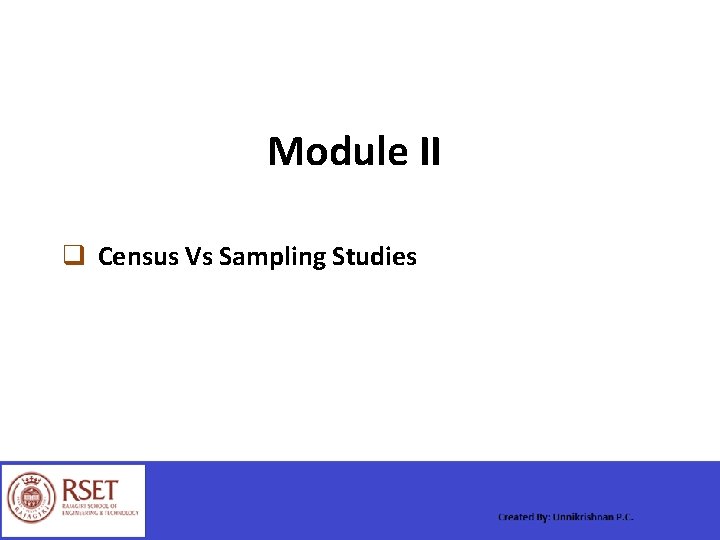
Module II q Census Vs Sampling Studies
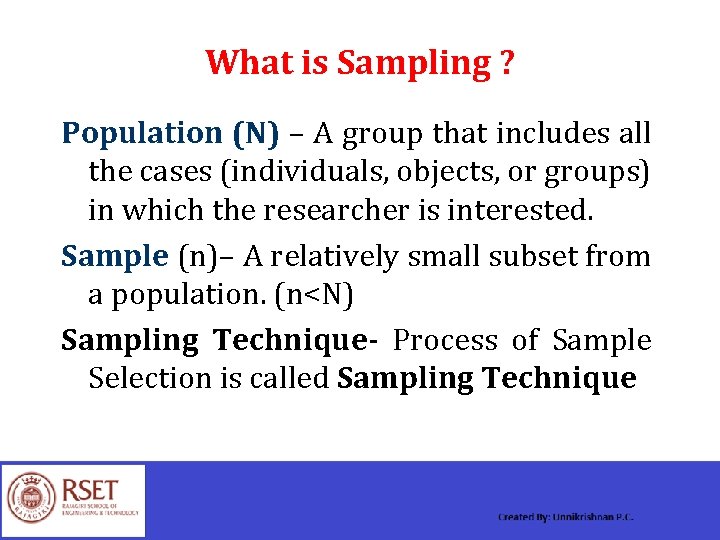
What is Sampling ? Population (N) – A group that includes all the cases (individuals, objects, or groups) in which the researcher is interested. Sample (n)– A relatively small subset from a population. (n<N) Sampling Technique- Process of Sample Selection is called Sampling Technique
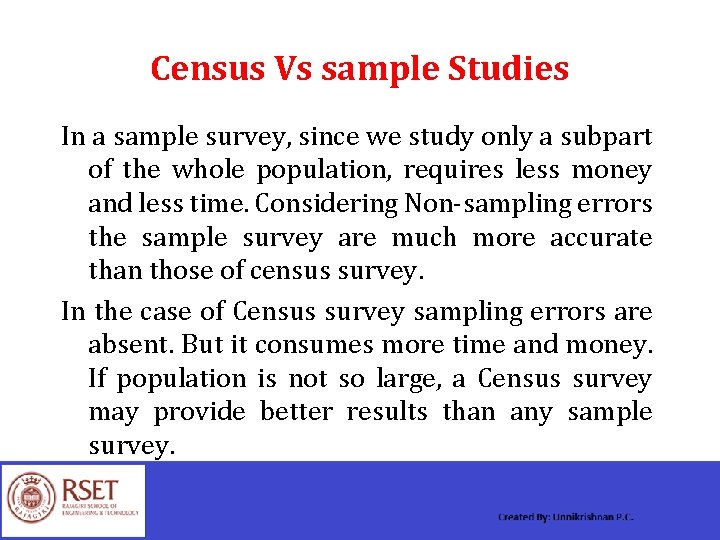
Census Vs sample Studies In a sample survey, since we study only a subpart of the whole population, requires less money and less time. Considering Non-sampling errors the sample survey are much more accurate than those of census survey. In the case of Census survey sampling errors are absent. But it consumes more time and money. If population is not so large, a Census survey may provide better results than any sample survey.
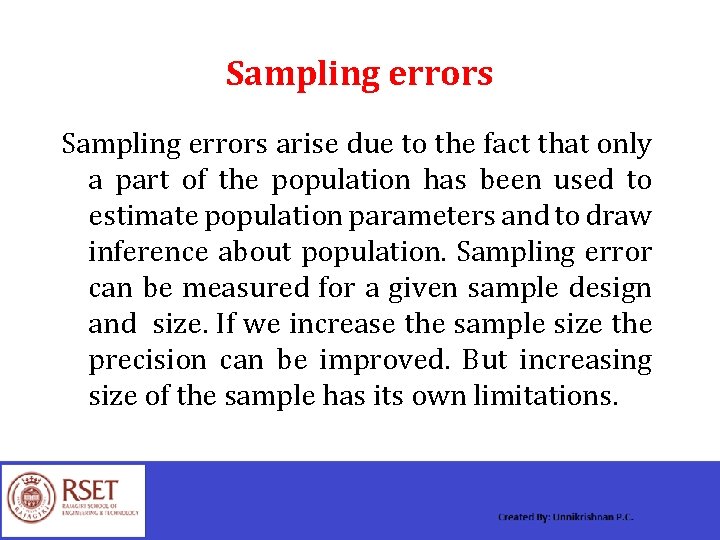
Sampling errors arise due to the fact that only a part of the population has been used to estimate population parameters and to draw inference about population. Sampling error can be measured for a given sample design and size. If we increase the sample size the precision can be improved. But increasing size of the sample has its own limitations.
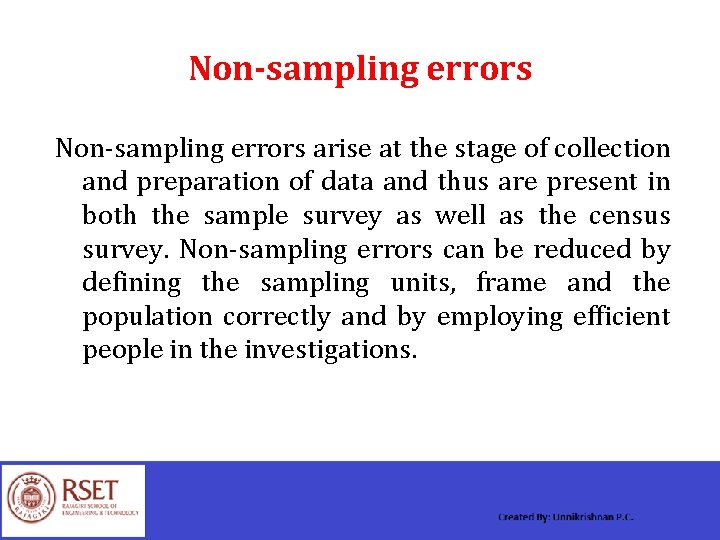
Non-sampling errors arise at the stage of collection and preparation of data and thus are present in both the sample survey as well as the census survey. Non-sampling errors can be reduced by defining the sampling units, frame and the population correctly and by employing efficient people in the investigations.
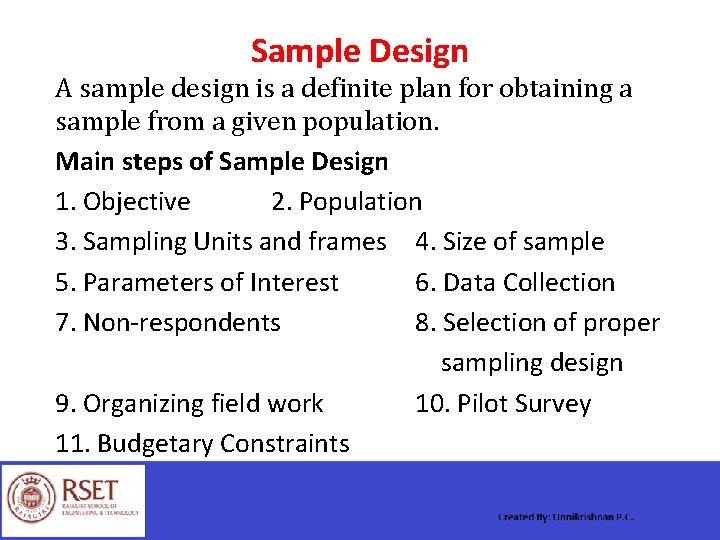
Sample Design A sample design is a definite plan for obtaining a sample from a given population. Main steps of Sample Design 1. Objective 2. Population 3. Sampling Units and frames 4. Size of sample 5. Parameters of Interest 6. Data Collection 7. Non-respondents 8. Selection of proper sampling design 9. Organizing field work 10. Pilot Survey 11. Budgetary Constraints
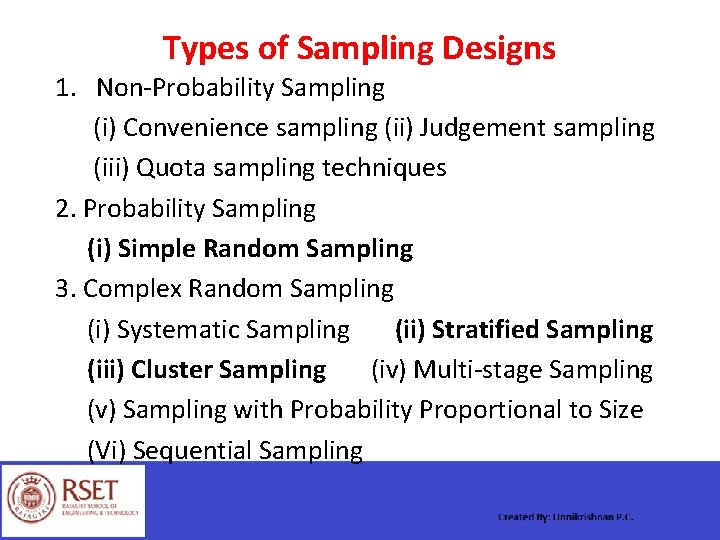
Types of Sampling Designs 1. Non-Probability Sampling (i) Convenience sampling (ii) Judgement sampling (iii) Quota sampling techniques 2. Probability Sampling (i) Simple Random Sampling 3. Complex Random Sampling (i) Systematic Sampling (ii) Stratified Sampling (iii) Cluster Sampling (iv) Multi-stage Sampling (v) Sampling with Probability Proportional to Size (Vi) Sequential Sampling
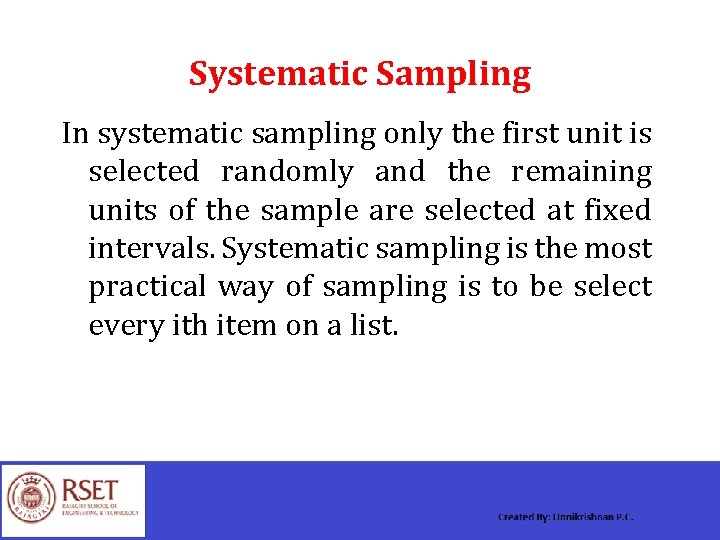
Systematic Sampling In systematic sampling only the first unit is selected randomly and the remaining units of the sample are selected at fixed intervals. Systematic sampling is the most practical way of sampling is to be select every ith item on a list.
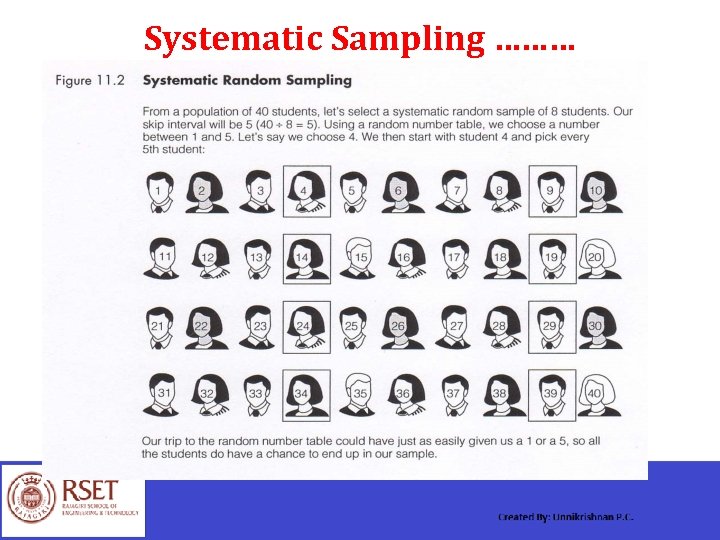
Systematic Sampling ………
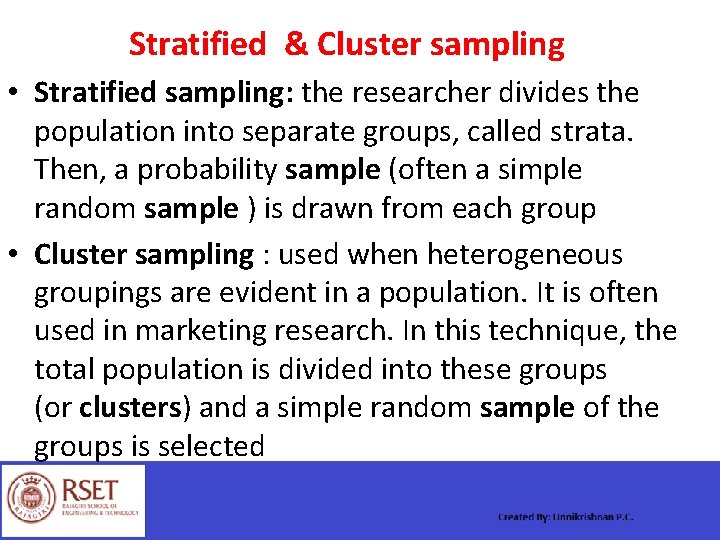
Stratified & Cluster sampling • Stratified sampling: the researcher divides the population into separate groups, called strata. Then, a probability sample (often a simple random sample ) is drawn from each group • Cluster sampling : used when heterogeneous groupings are evident in a population. It is often used in marketing research. In this technique, the total population is divided into these groups (or clusters) and a simple random sample of the groups is selected
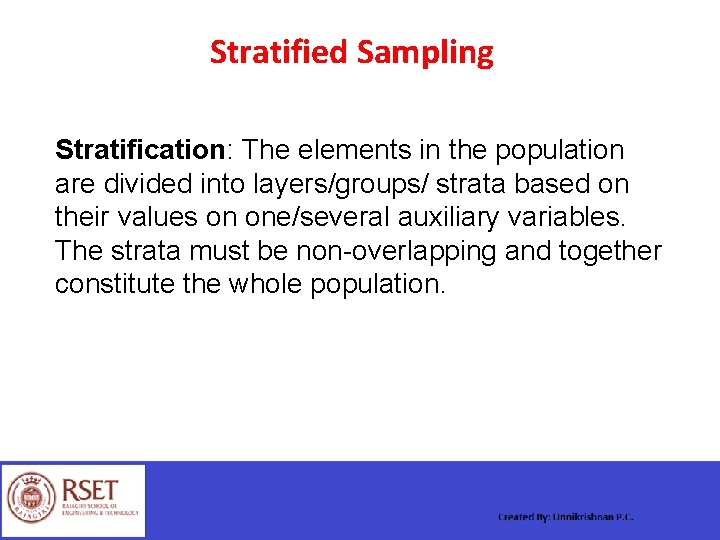
Stratified Sampling Stratification: The elements in the population are divided into layers/groups/ strata based on their values on one/several auxiliary variables. The strata must be non-overlapping and together constitute the whole population.
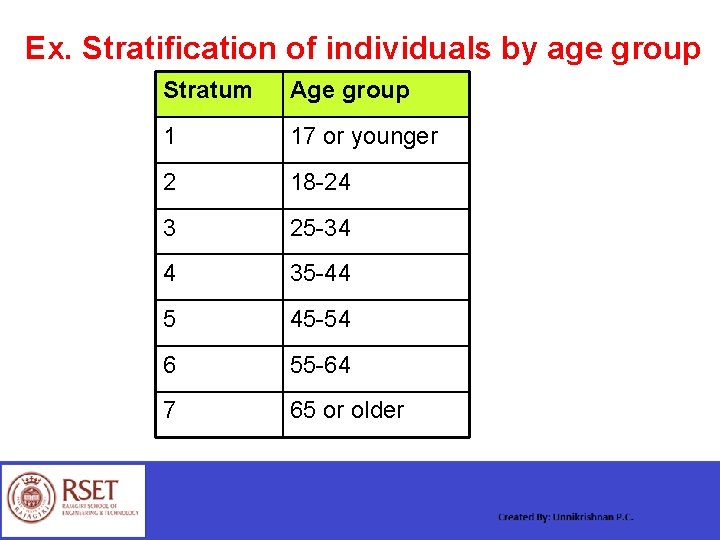
Ex. Stratification of individuals by age group Stratum Age group 1 17 or younger 2 18 -24 3 25 -34 4 35 -44 5 45 -54 6 55 -64 7 65 or older
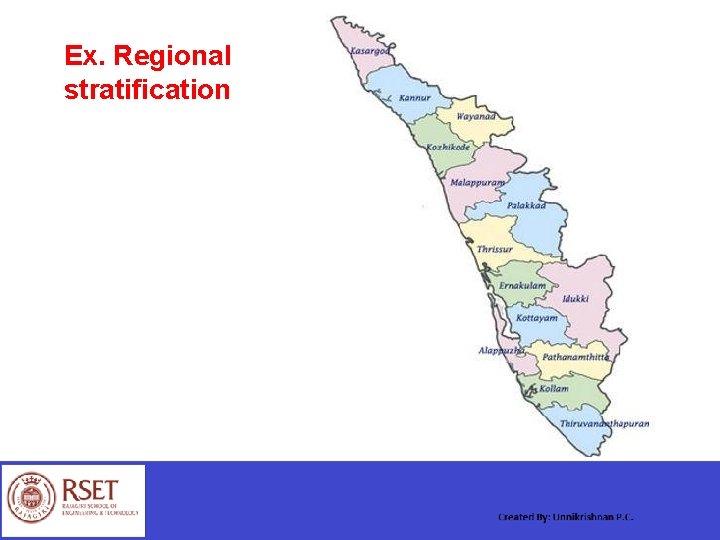
Ex. Regional stratification
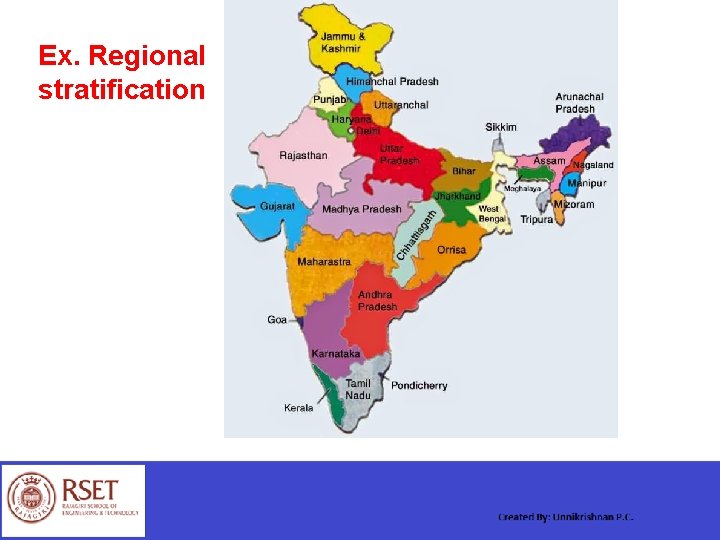
Ex. Regional stratification
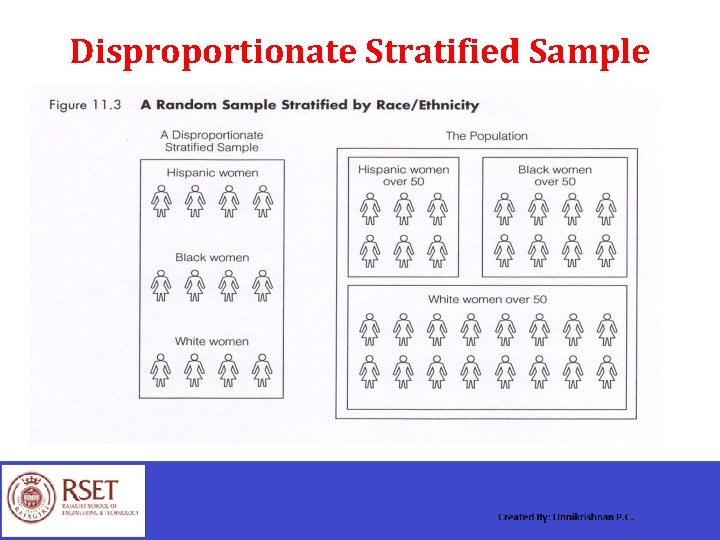
Disproportionate Stratified Sample
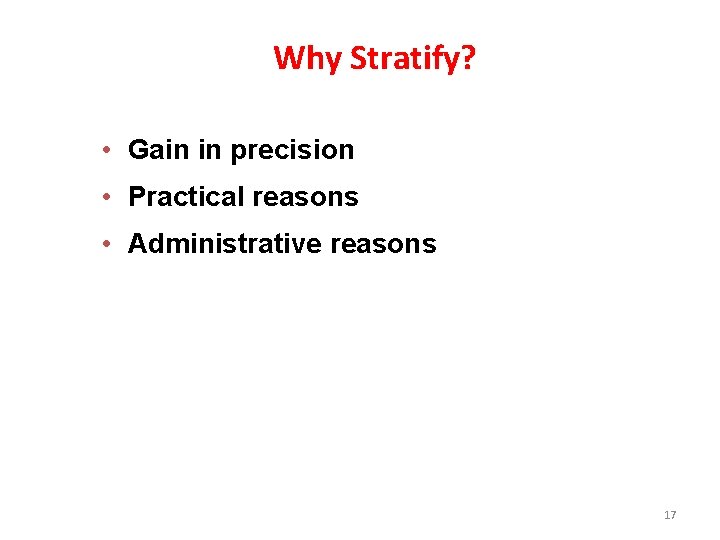
Why Stratify? • Gain in precision • Practical reasons • Administrative reasons 17
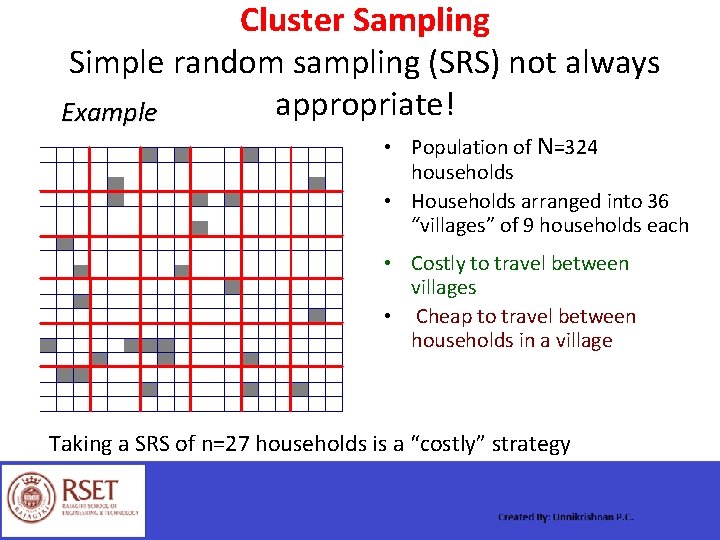
Cluster Sampling Simple random sampling (SRS) not always appropriate! Example • Population of N=324 households • Households arranged into 36 “villages” of 9 households each • Costly to travel between villages • Cheap to travel between households in a village Taking a SRS of n=27 households is a “costly” strategy
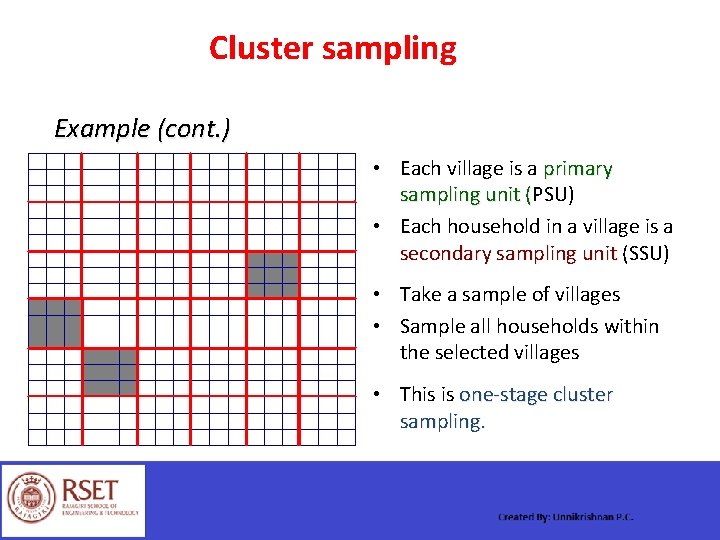
Cluster sampling Example (cont. ) • Each village is a primary sampling unit (PSU) sampling unit ( • Each household in a village is a secondary sampling unit (SSU) secondary sampling unit • Take a sample of villages • Sample all households within the selected villages • This is one-stage cluster sampling.
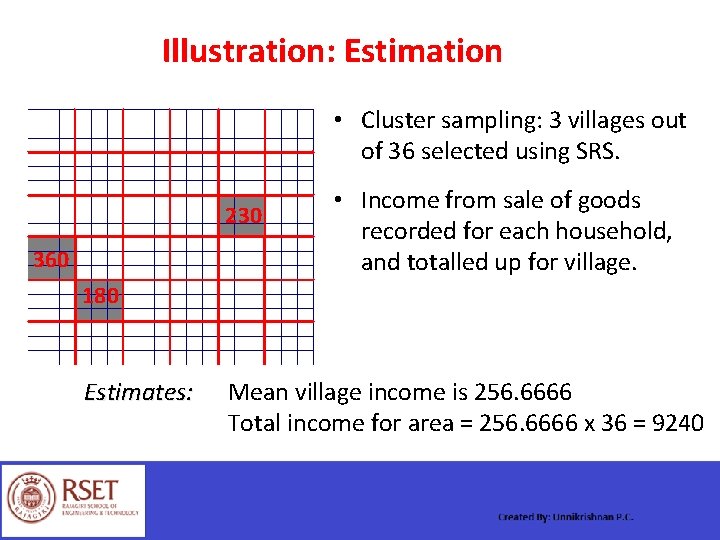
Illustration: Estimation • Cluster sampling: 3 villages out of 36 selected using SRS. 230 360 • Income from sale of goods recorded for each household, and totalled up for village. 180 Estimates: Mean village income is 256. 6666 Total income for area = 256. 6666 x 36 = 9240
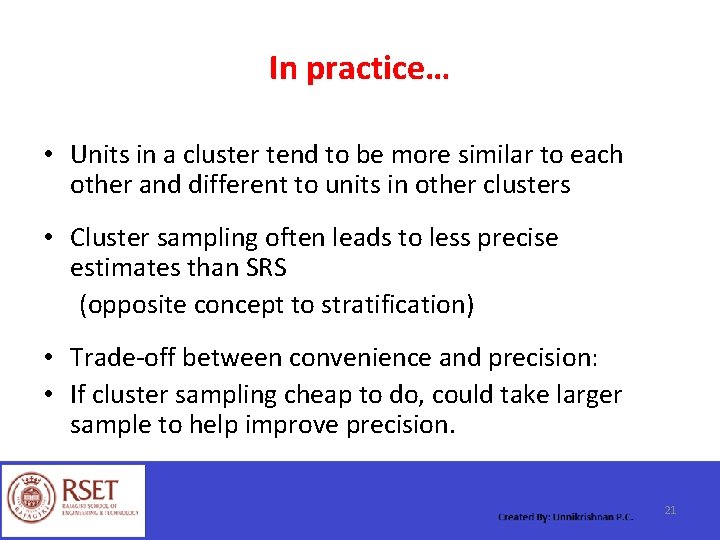
In practice… • Units in a cluster tend to be more similar to each other and different to units in other clusters • Cluster sampling often leads to less precise estimates than SRS (opposite concept to stratification) • Trade-off between convenience and precision: • If cluster sampling cheap to do, could take larger sample to help improve precision. 21
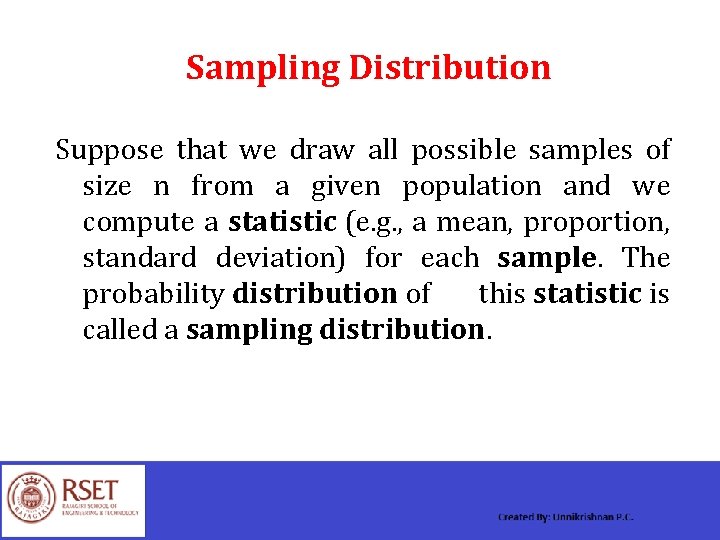
Sampling Distribution Suppose that we draw all possible samples of size n from a given population and we compute a statistic (e. g. , a mean, proportion, standard deviation) for each sample. The probability distribution of this statistic is called a sampling distribution.
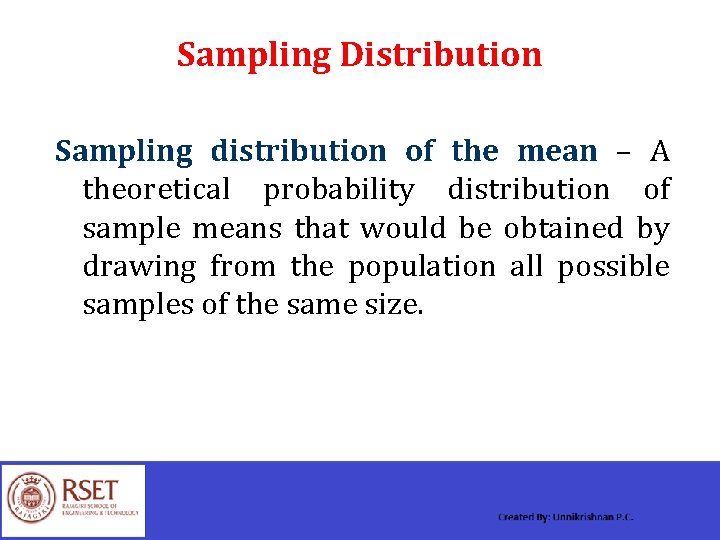
Sampling Distribution Sampling distribution of the mean – A theoretical probability distribution of sample means that would be obtained by drawing from the population all possible samples of the same size.
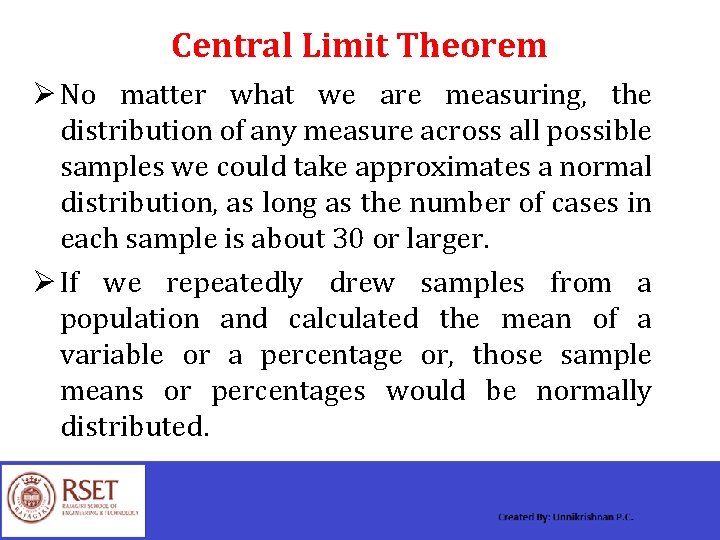
Central Limit Theorem Ø No matter what we are measuring, the distribution of any measure across all possible samples we could take approximates a normal distribution, as long as the number of cases in each sample is about 30 or larger. Ø If we repeatedly drew samples from a population and calculated the mean of a variable or a percentage or, those sample means or percentages would be normally distributed.
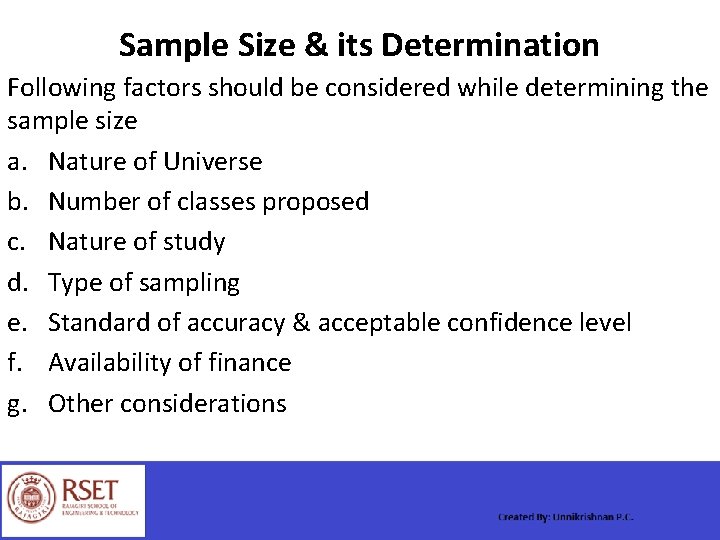
Sample Size & its Determination Following factors should be considered while determining the sample size a. Nature of Universe b. Number of classes proposed c. Nature of study d. Type of sampling e. Standard of accuracy & acceptable confidence level f. Availability of finance g. Other considerations
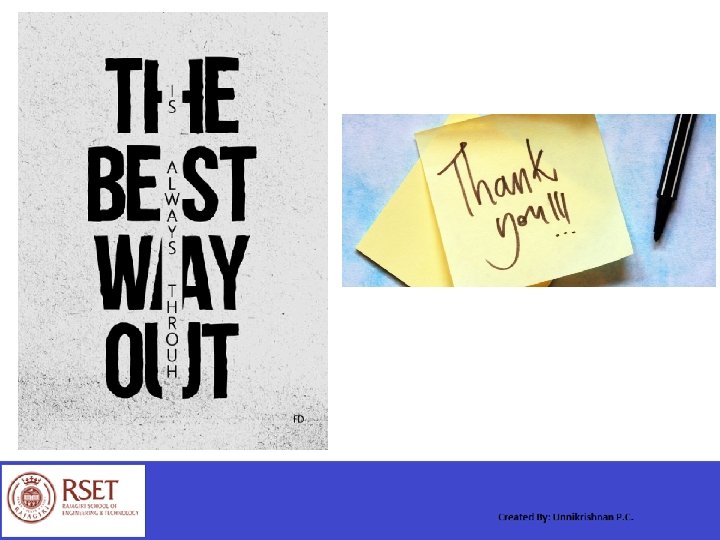