Representative Population the entire group of people or
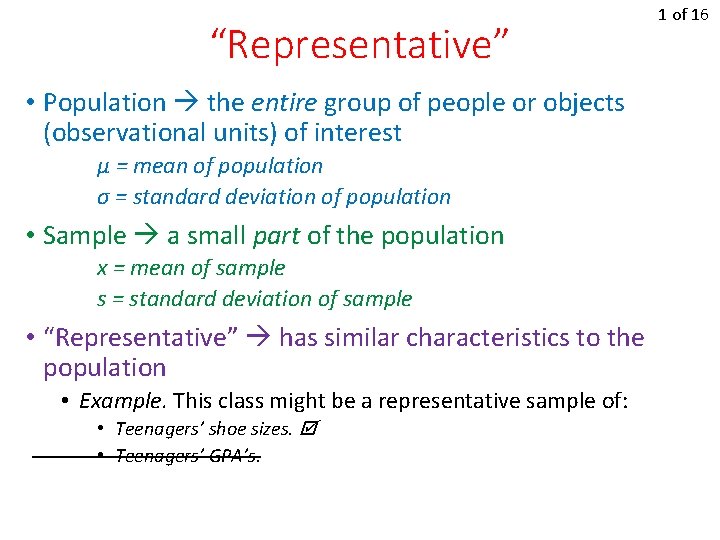
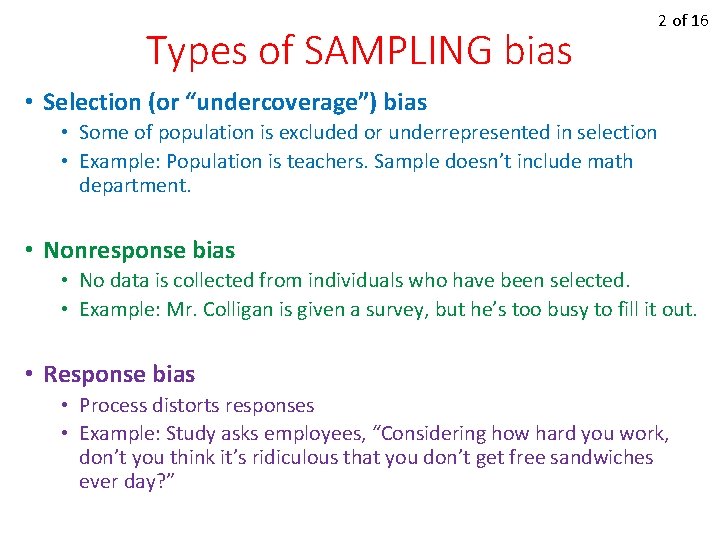
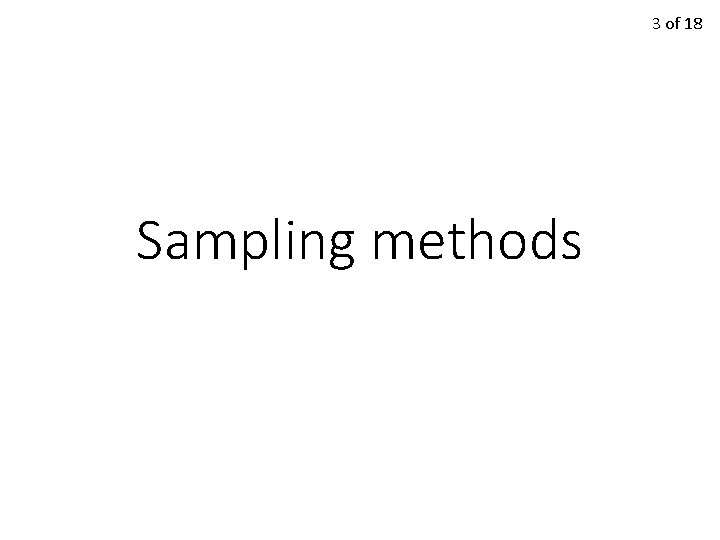
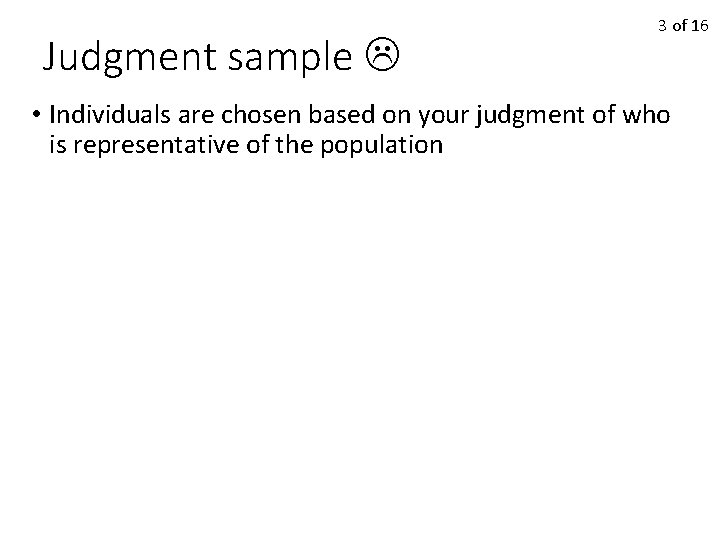
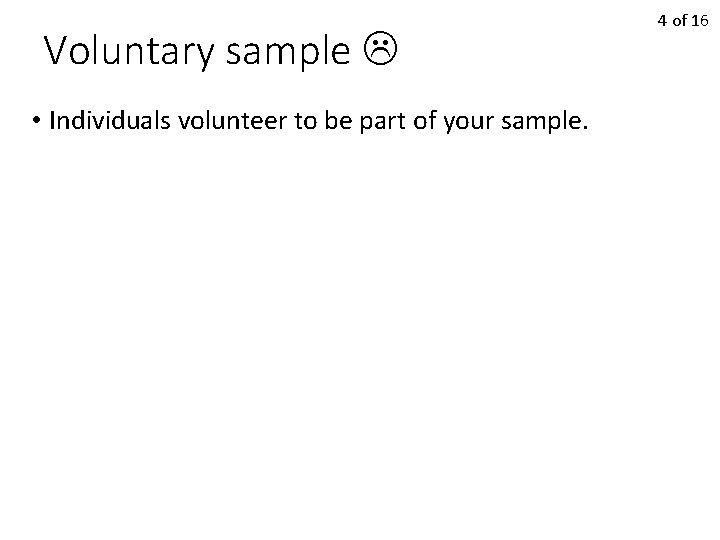
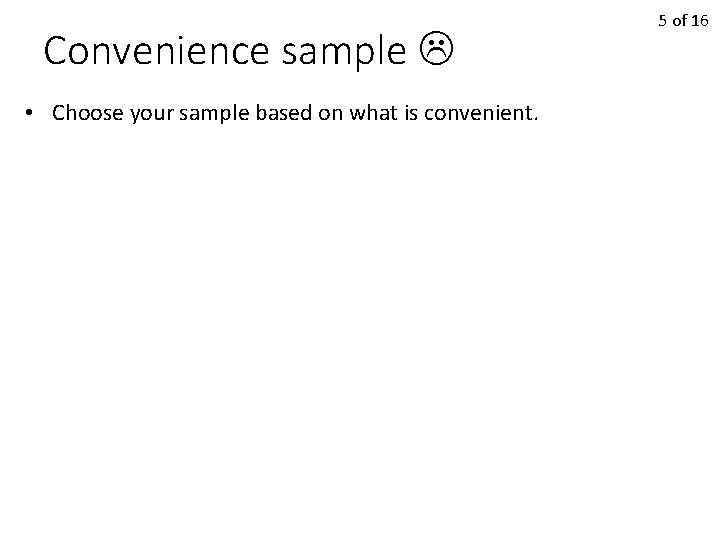
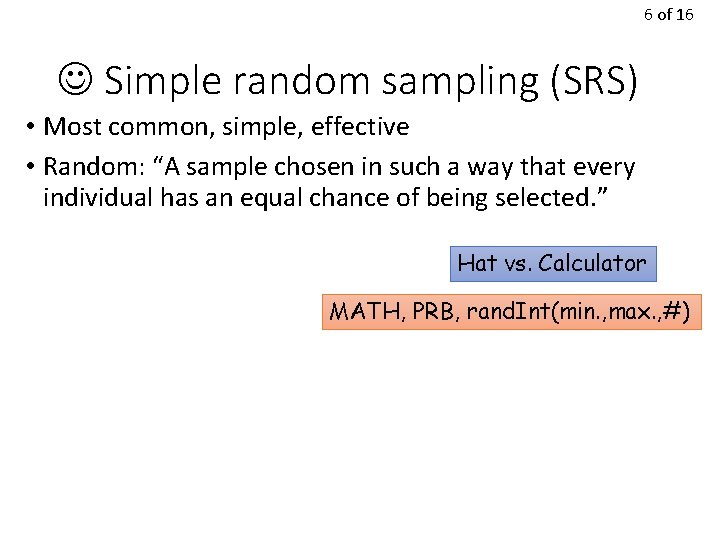
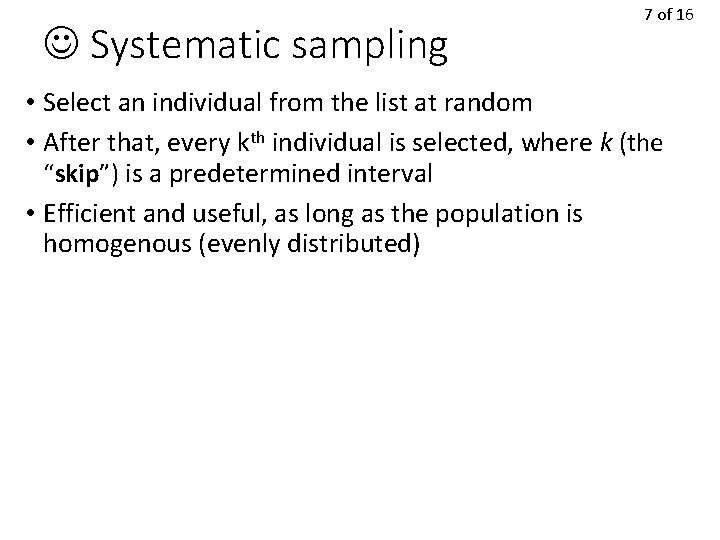
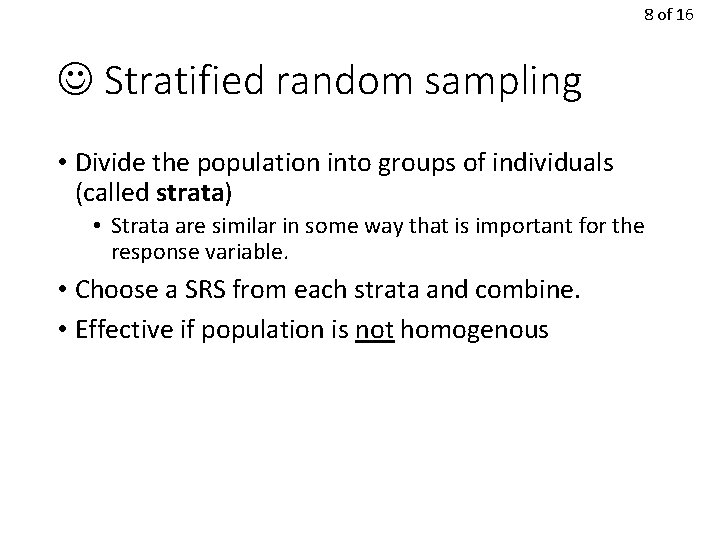
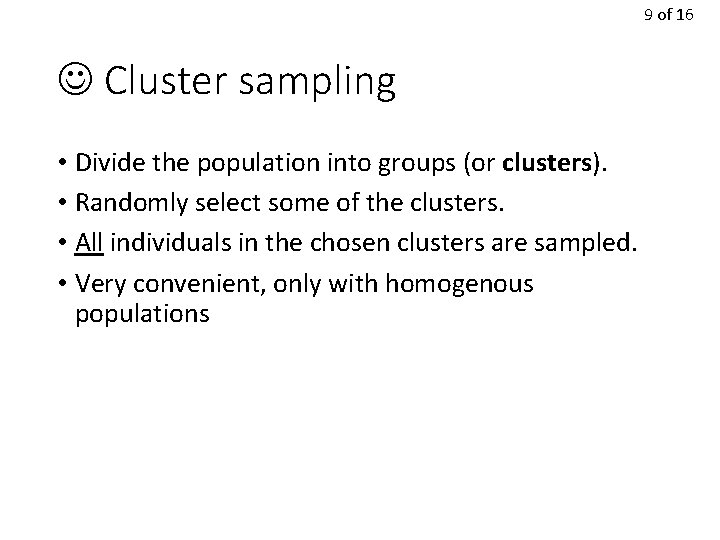
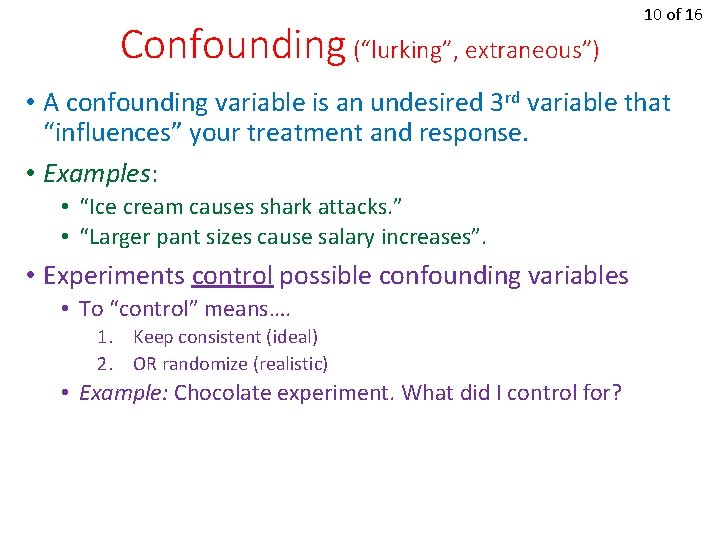
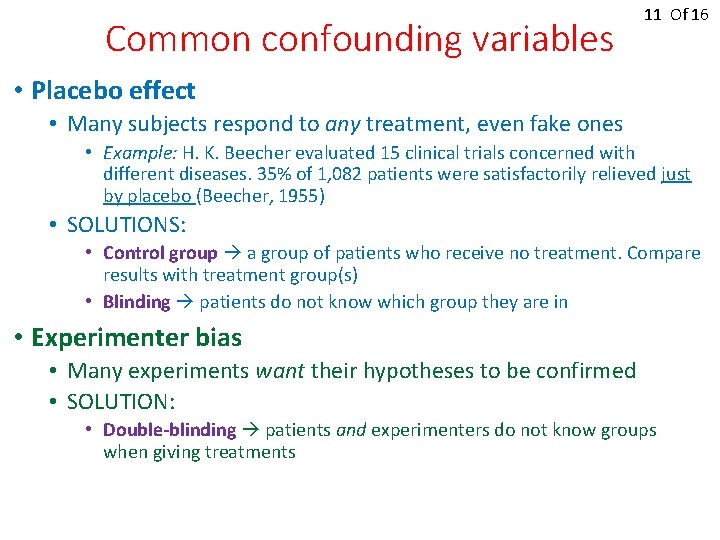
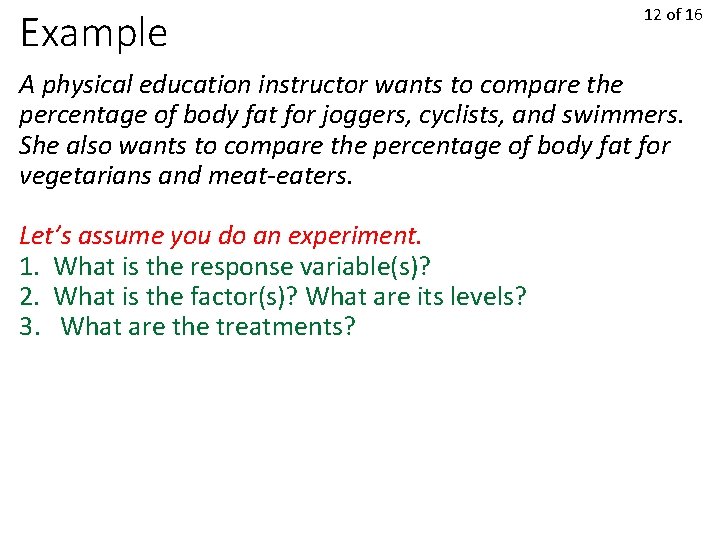
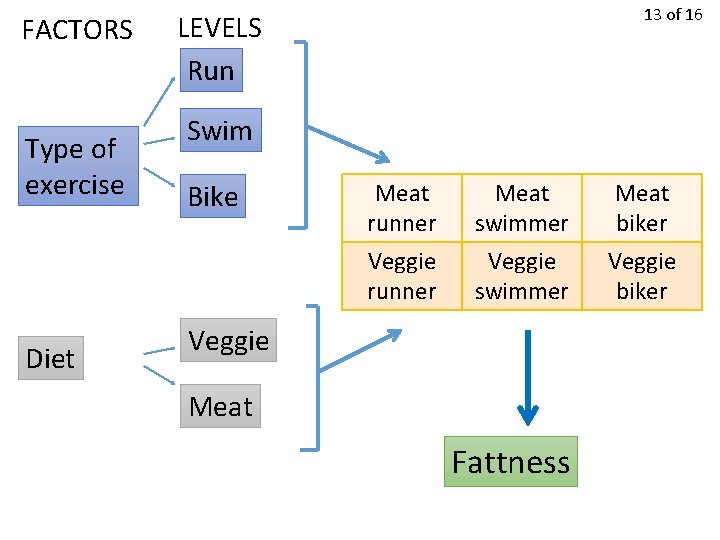
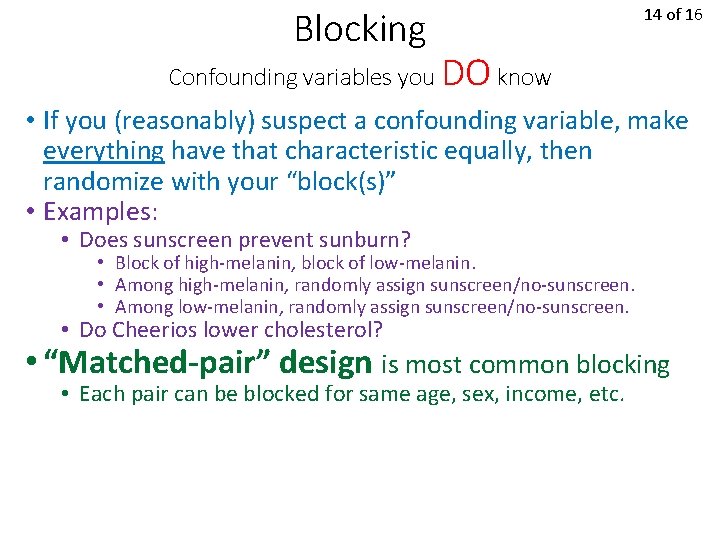
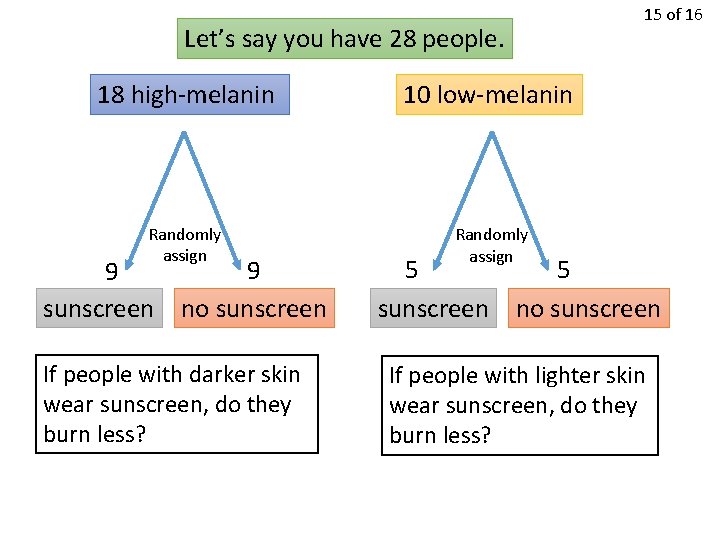
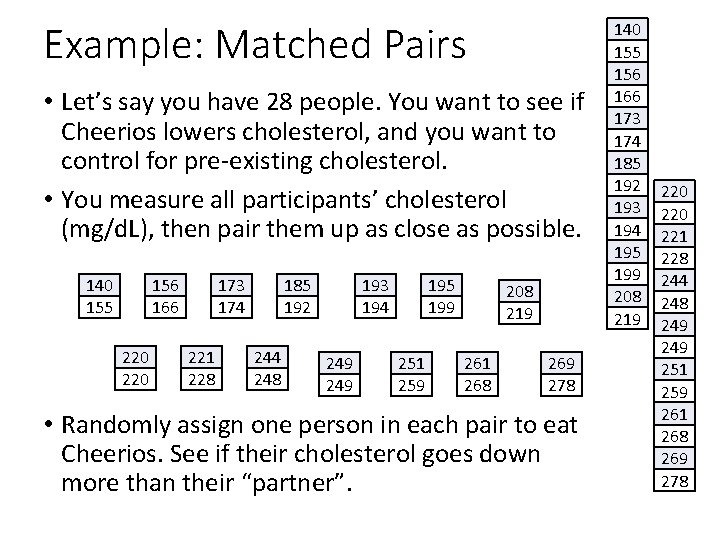
- Slides: 17
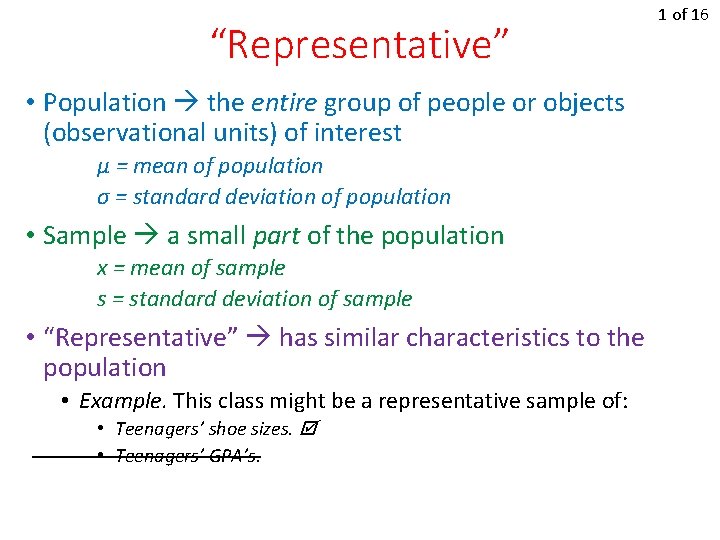
“Representative” • Population the entire group of people or objects (observational units) of interest µ = mean of population σ = standard deviation of population • Sample a small part of the population x = mean of sample s = standard deviation of sample • “Representative” has similar characteristics to the population • Example. This class might be a representative sample of: • Teenagers’ shoe sizes. • Teenagers’ GPA’s. 1 of 16
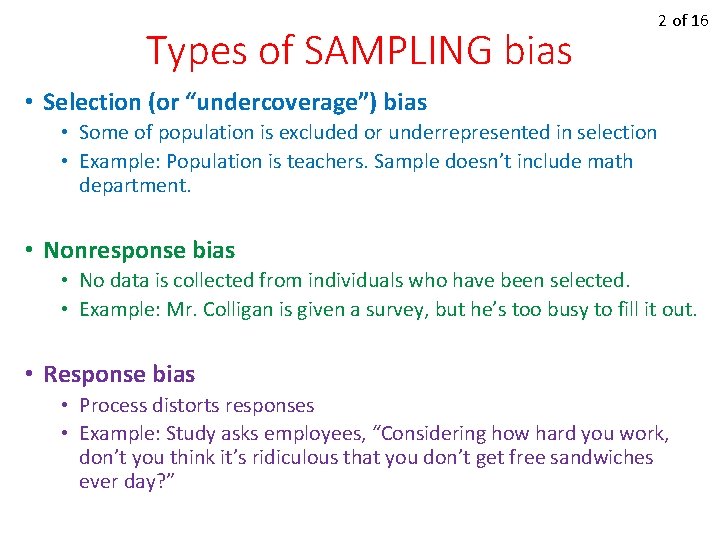
Types of SAMPLING bias 2 of 16 • Selection (or “undercoverage”) bias • Some of population is excluded or underrepresented in selection • Example: Population is teachers. Sample doesn’t include math department. • Nonresponse bias • No data is collected from individuals who have been selected. • Example: Mr. Colligan is given a survey, but he’s too busy to fill it out. • Response bias • Process distorts responses • Example: Study asks employees, “Considering how hard you work, don’t you think it’s ridiculous that you don’t get free sandwiches ever day? ”
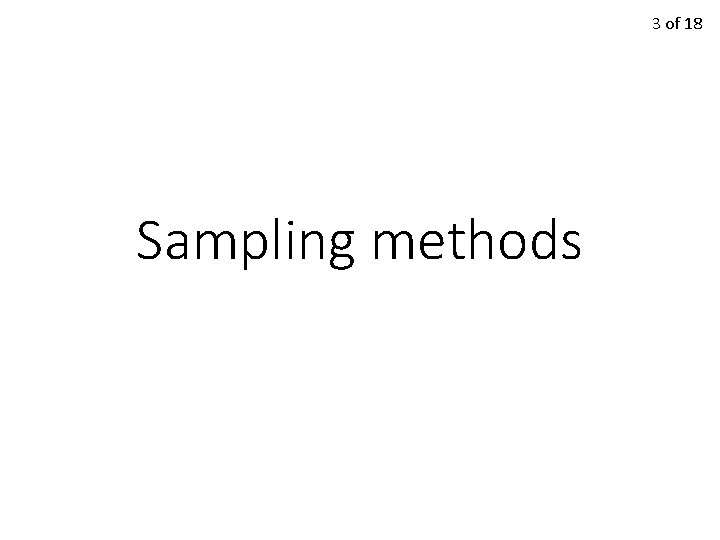
3 of 18 Sampling methods
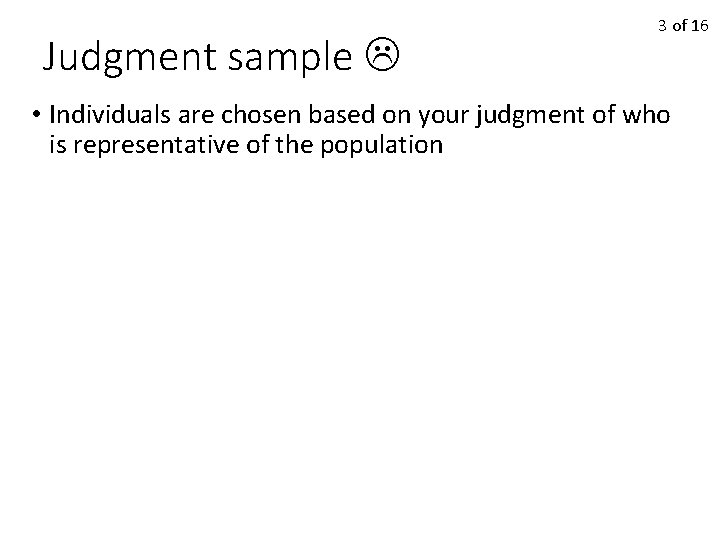
Judgment sample 3 of 16 • Individuals are chosen based on your judgment of who is representative of the population
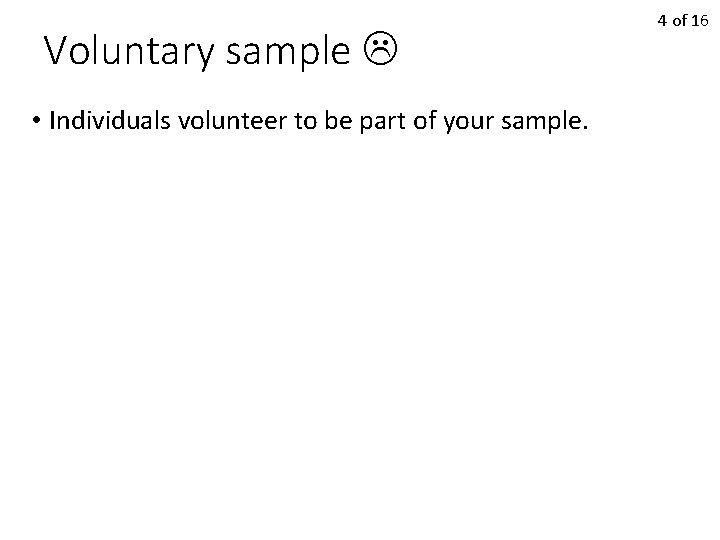
Voluntary sample • Individuals volunteer to be part of your sample. 4 of 16
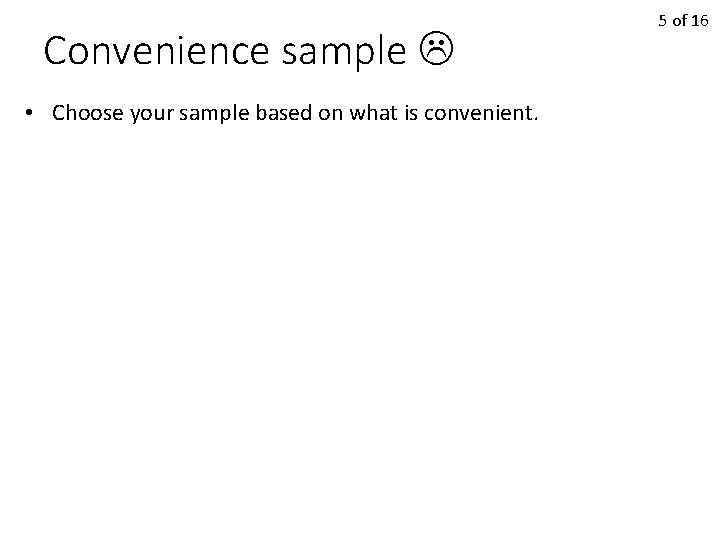
Convenience sample • Choose your sample based on what is convenient. 5 of 16
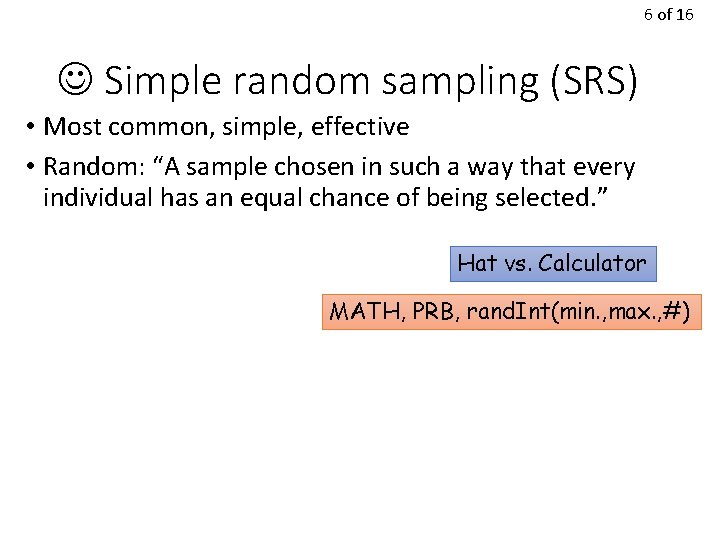
6 of 16 Simple random sampling (SRS) • Most common, simple, effective • Random: “A sample chosen in such a way that every individual has an equal chance of being selected. ” Hat vs. Calculator MATH, PRB, rand. Int(min. , max. , #)
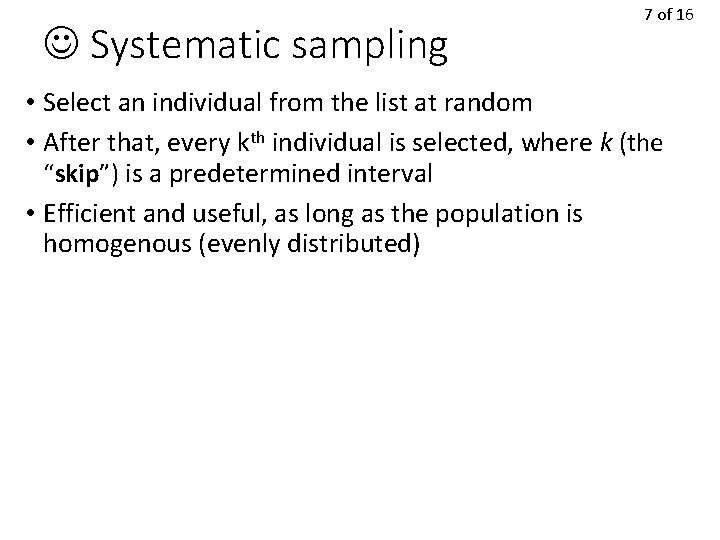
Systematic sampling 7 of 16 • Select an individual from the list at random • After that, every kth individual is selected, where k (the “skip”) is a predetermined interval • Efficient and useful, as long as the population is homogenous (evenly distributed)
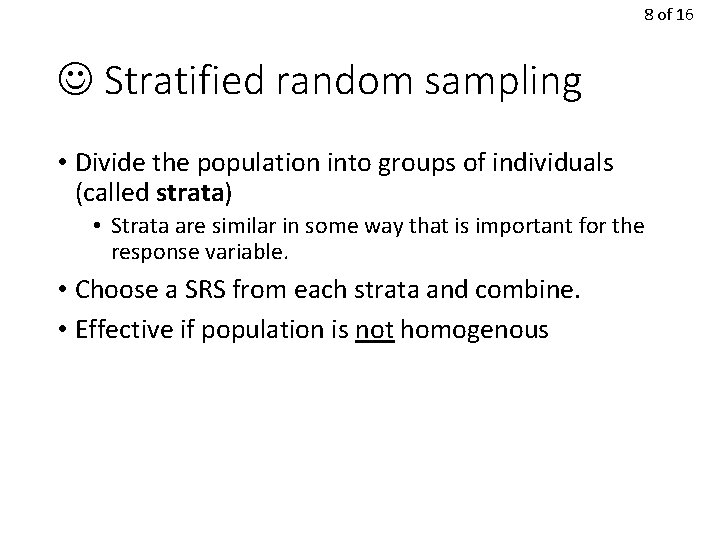
8 of 16 Stratified random sampling • Divide the population into groups of individuals (called strata) • Strata are similar in some way that is important for the response variable. • Choose a SRS from each strata and combine. • Effective if population is not homogenous
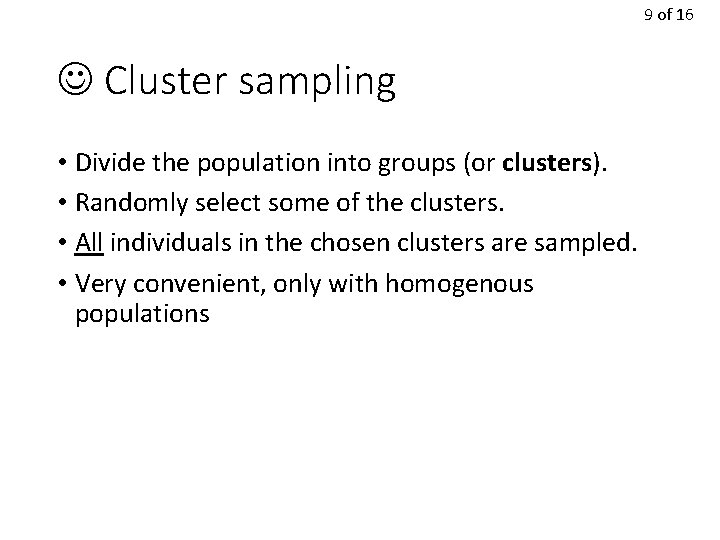
9 of 16 Cluster sampling • Divide the population into groups (or clusters). • Randomly select some of the clusters. • All individuals in the chosen clusters are sampled. • Very convenient, only with homogenous populations
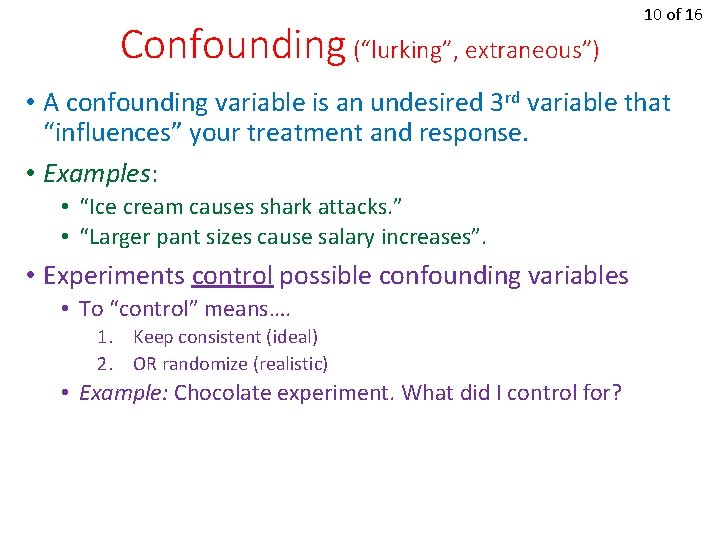
Confounding (“lurking”, extraneous”) 10 of 16 • A confounding variable is an undesired 3 rd variable that “influences” your treatment and response. • Examples: • “Ice cream causes shark attacks. ” • “Larger pant sizes cause salary increases”. • Experiments control possible confounding variables • To “control” means…. 1. Keep consistent (ideal) 2. OR randomize (realistic) • Example: Chocolate experiment. What did I control for?
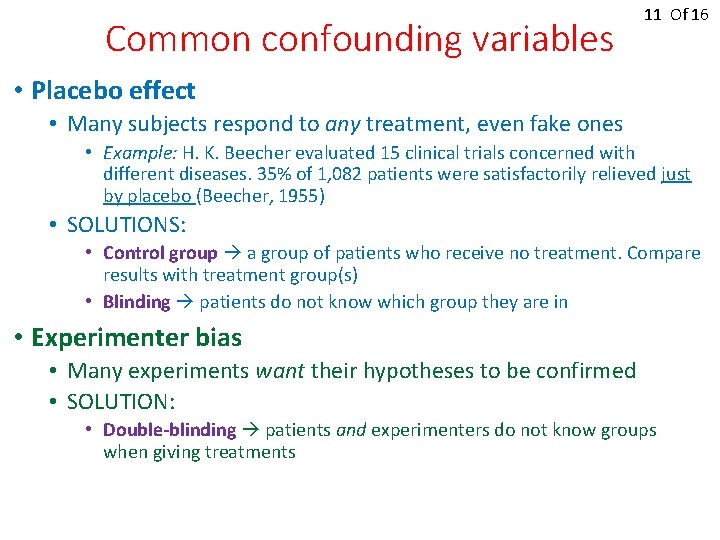
Common confounding variables 11 Of 16 • Placebo effect • Many subjects respond to any treatment, even fake ones • Example: H. K. Beecher evaluated 15 clinical trials concerned with different diseases. 35% of 1, 082 patients were satisfactorily relieved just by placebo (Beecher, 1955) • SOLUTIONS: • Control group a group of patients who receive no treatment. Compare results with treatment group(s) • Blinding patients do not know which group they are in • Experimenter bias • Many experiments want their hypotheses to be confirmed • SOLUTION: • Double-blinding patients and experimenters do not know groups when giving treatments
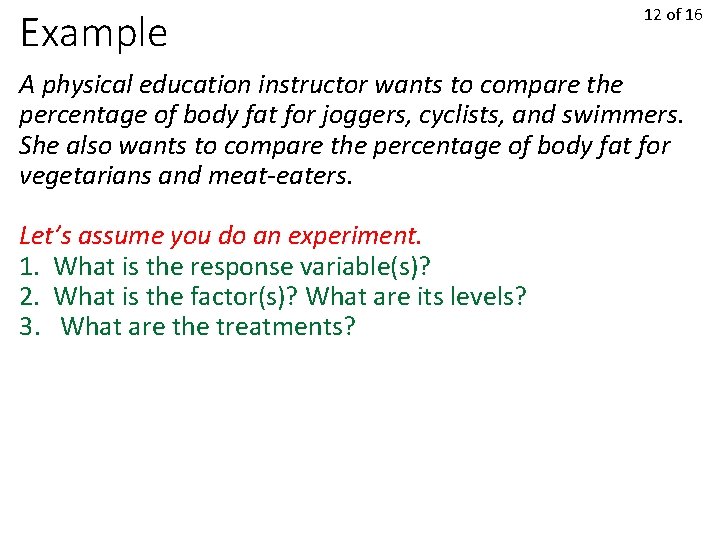
Example 12 of 16 A physical education instructor wants to compare the percentage of body fat for joggers, cyclists, and swimmers. She also wants to compare the percentage of body fat for vegetarians and meat-eaters. Let’s assume you do an experiment. 1. What is the response variable(s)? 2. What is the factor(s)? What are its levels? 3. What are the treatments?
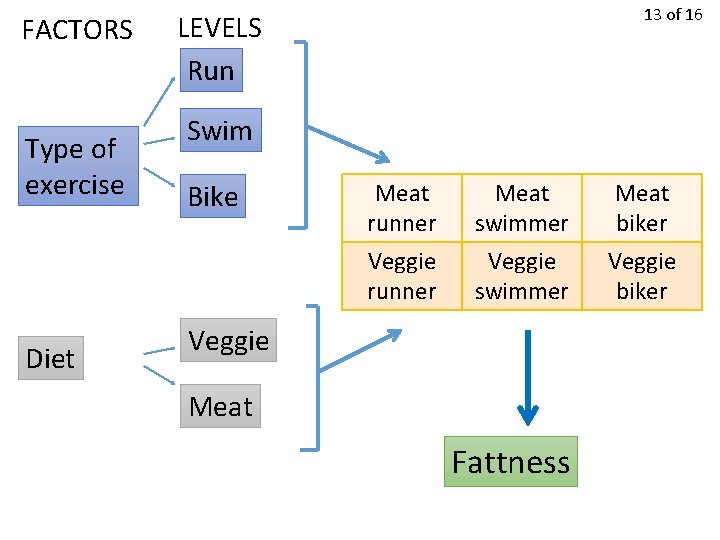
FACTORS Type of exercise Diet 13 of 16 LEVELS Run Swim Bike Meat runner Meat swimmer Meat biker Veggie runner Veggie swimmer Veggie biker Veggie Meat Fattness
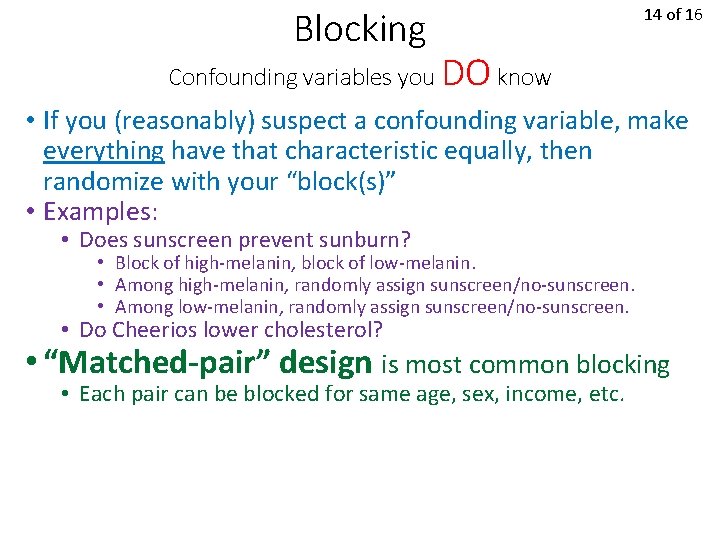
Blocking Confounding variables you 14 of 16 DO know • If you (reasonably) suspect a confounding variable, make everything have that characteristic equally, then randomize with your “block(s)” • Examples: • Does sunscreen prevent sunburn? • Block of high-melanin, block of low-melanin. • Among high-melanin, randomly assign sunscreen/no-sunscreen. • Among low-melanin, randomly assign sunscreen/no-sunscreen. • Do Cheerios lower cholesterol? • “Matched-pair” design is most common blocking • Each pair can be blocked for same age, sex, income, etc.
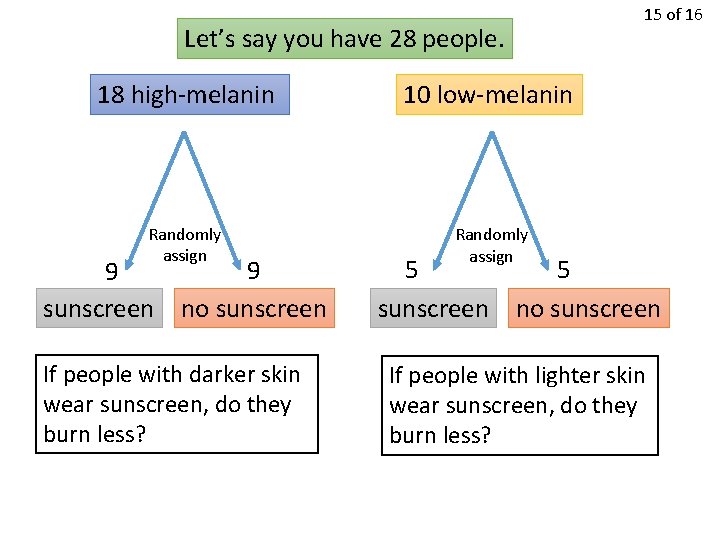
Let’s say you have 28 people. 18 high-melanin 10 low-melanin Randomly assign 9 9 sunscreen no sunscreen If people with darker skin wear sunscreen, do they burn less? 15 of 16 5 5 sunscreen no sunscreen If people with lighter skin wear sunscreen, do they burn less?
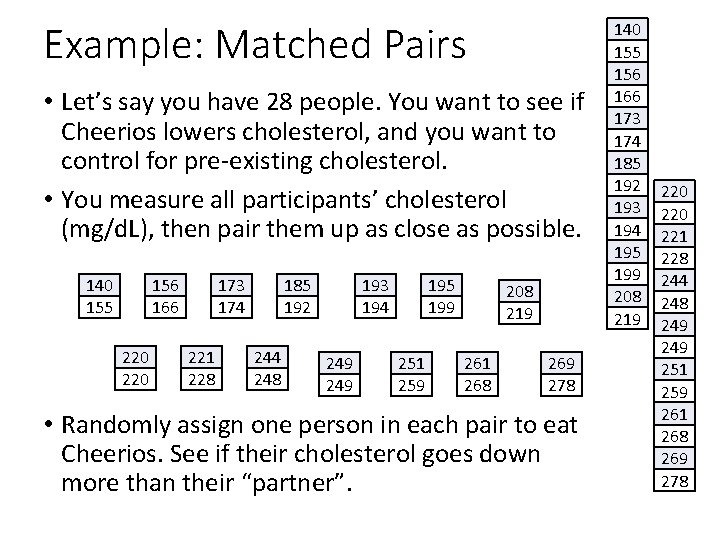
Example: Matched Pairs • Let’s say you have 28 people. You want to see if Cheerios lowers cholesterol, and you want to control for pre-existing cholesterol. • You measure all participants’ cholesterol (mg/d. L), then pair them up as close as possible. 140 155 156 166 220 173 174 221 228 185 192 244 248 193 194 249 195 199 251 259 208 219 261 268 269 278 • Randomly assign one person in each pair to eat Cheerios. See if their cholesterol goes down more than their “partner”. 140 155 156 166 173 174 185 192 193 194 195 199 208 219 220 221 228 244 248 249 251 259 261 268 269 278
Undercoverage bias
Demerits of cluster sampling
Halogens group number
Chapter 4 population ecology answer key
Section 1 population dynamics answer key
Population ecology section 1 population dynamics
Study guide chapter 4 section 1 population dynamics
Hát kết hợp bộ gõ cơ thể
Ng-html
Bổ thể
Tỉ lệ cơ thể trẻ em
Gấu đi như thế nào
Glasgow thang điểm
Hát lên người ơi
Các môn thể thao bắt đầu bằng tiếng bóng
Thế nào là hệ số cao nhất
Các châu lục và đại dương trên thế giới
Công thức tính thế năng