QUANTITATIVE RESEARCH METHODOLOGIES A SAMPLER Ophelia M Mendoza
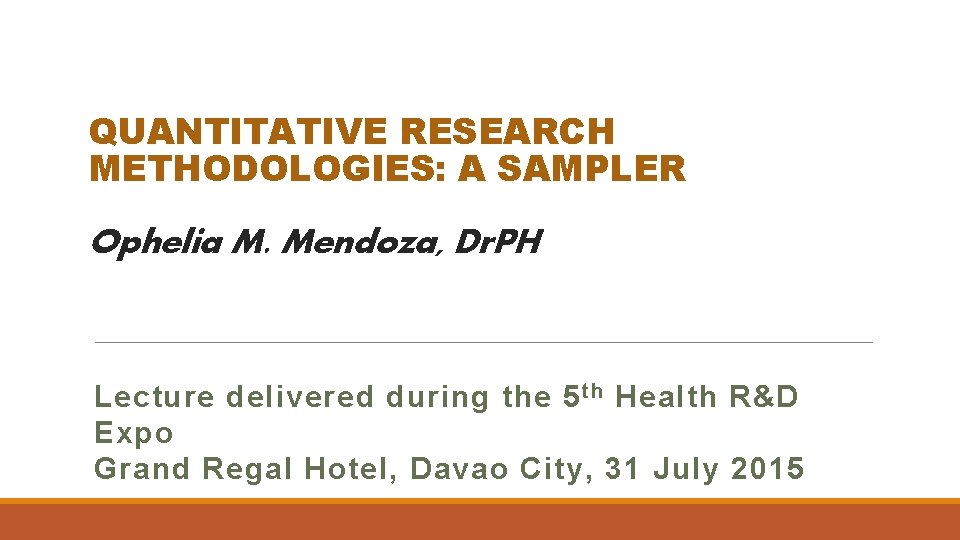
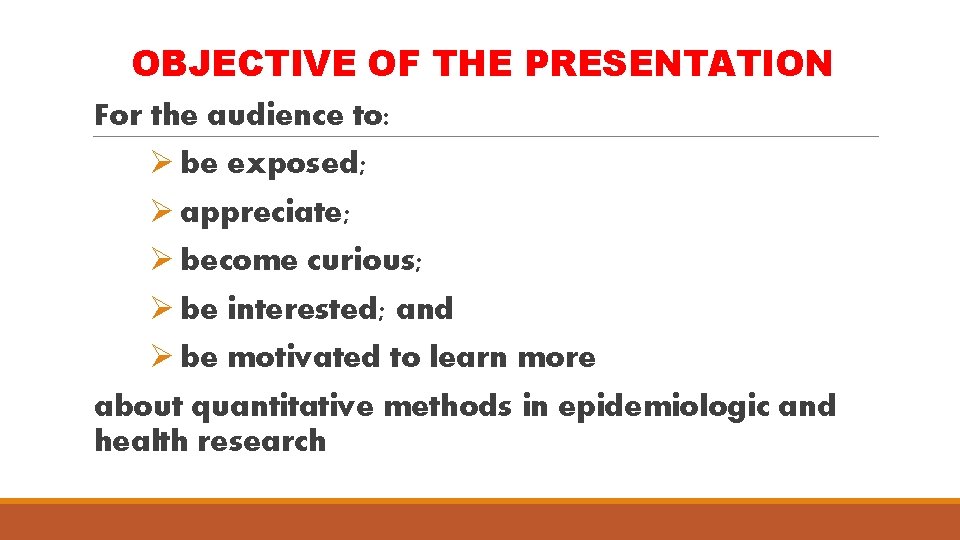
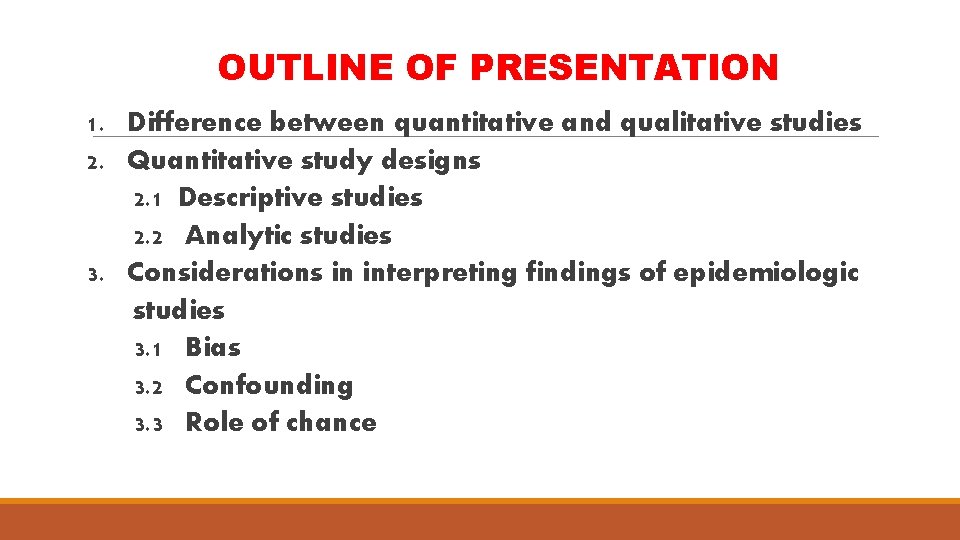
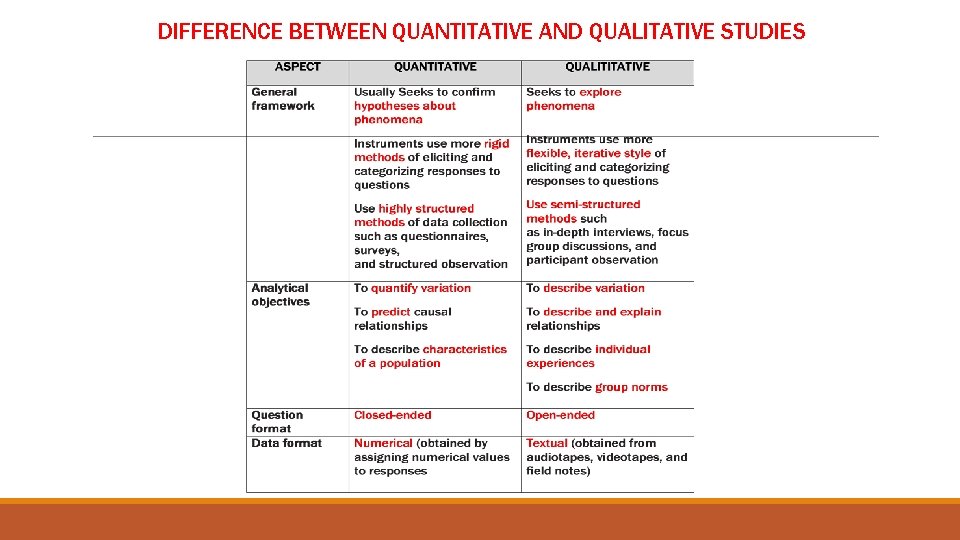
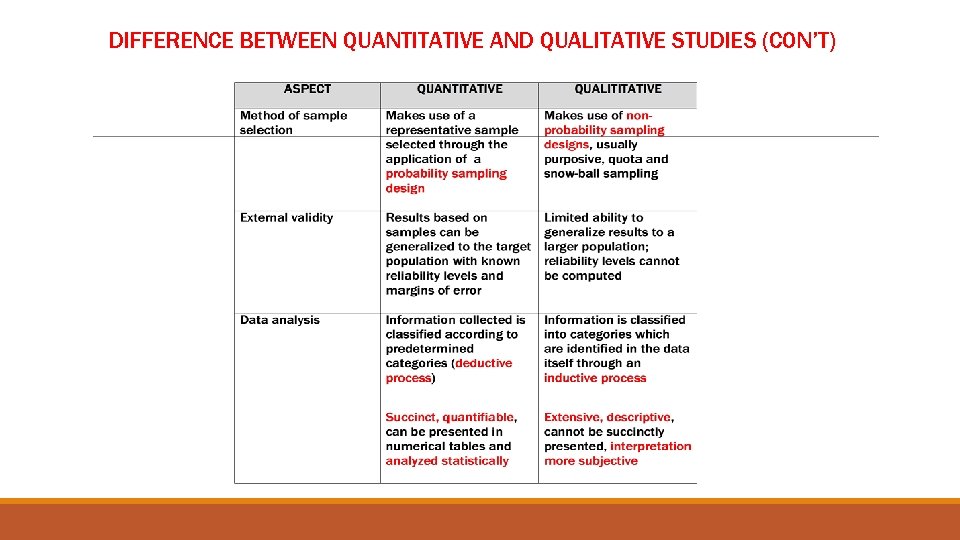
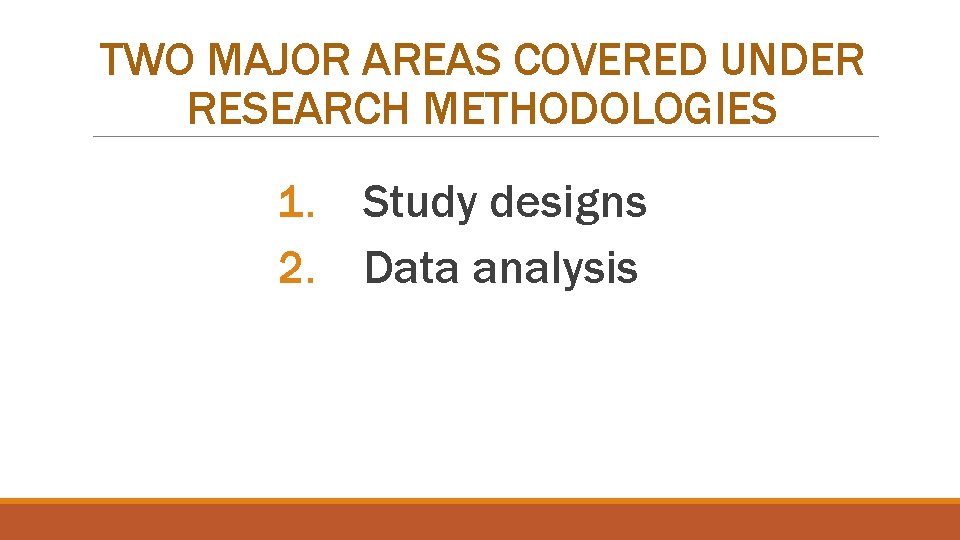
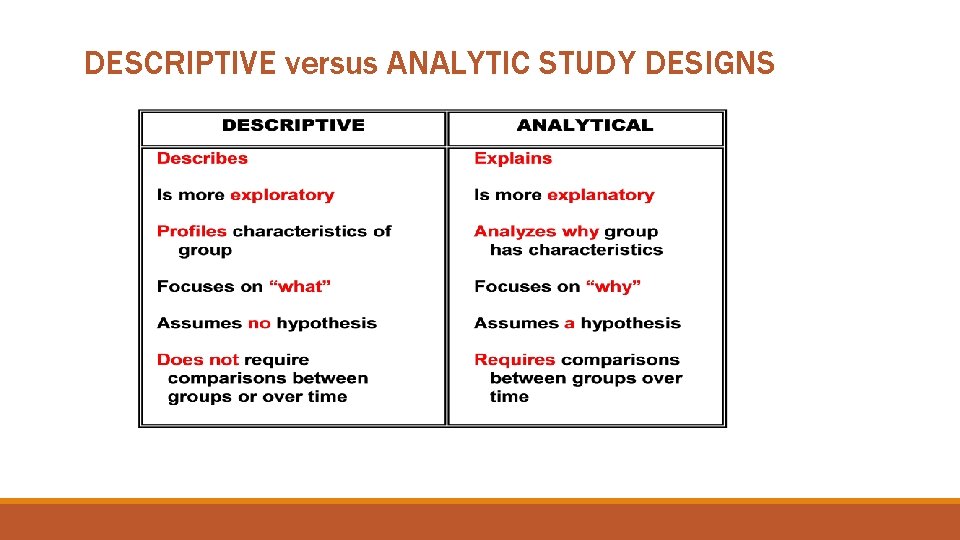
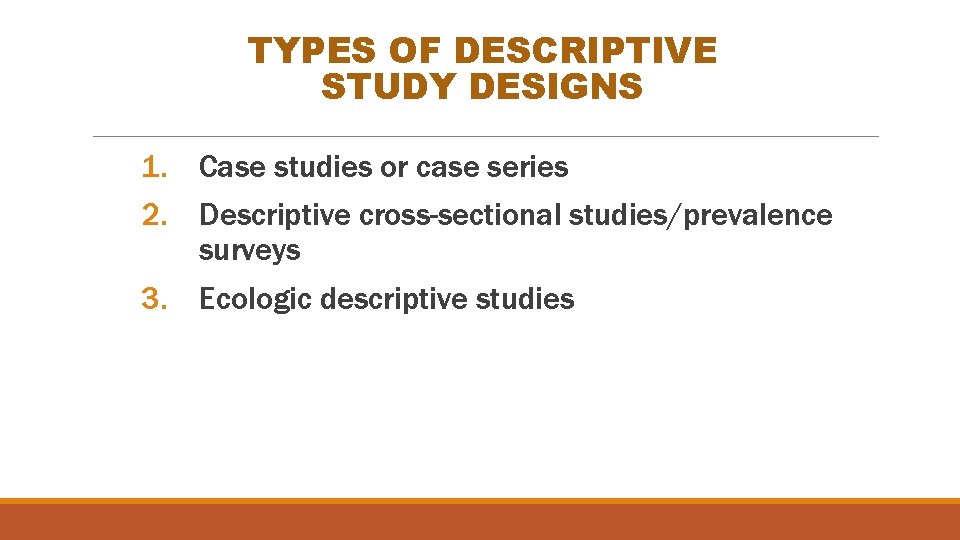
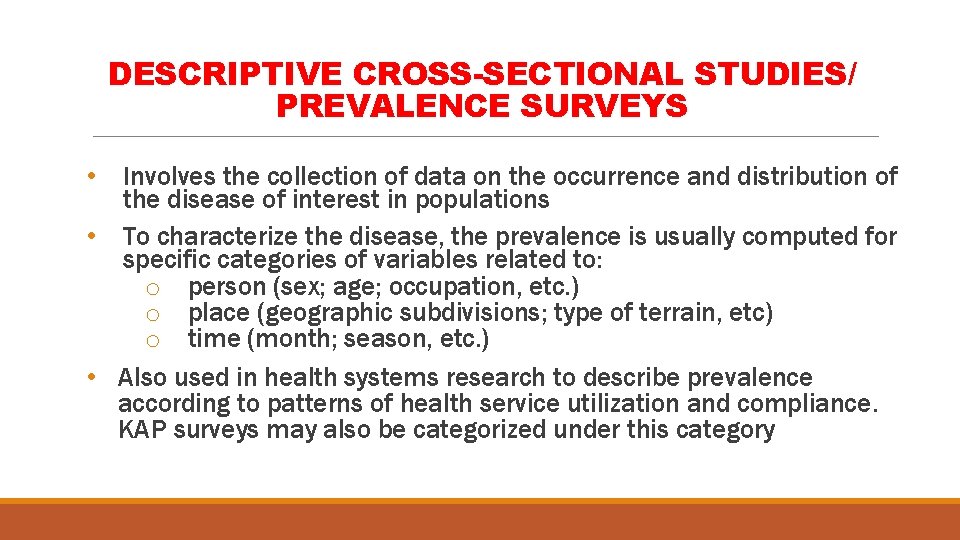
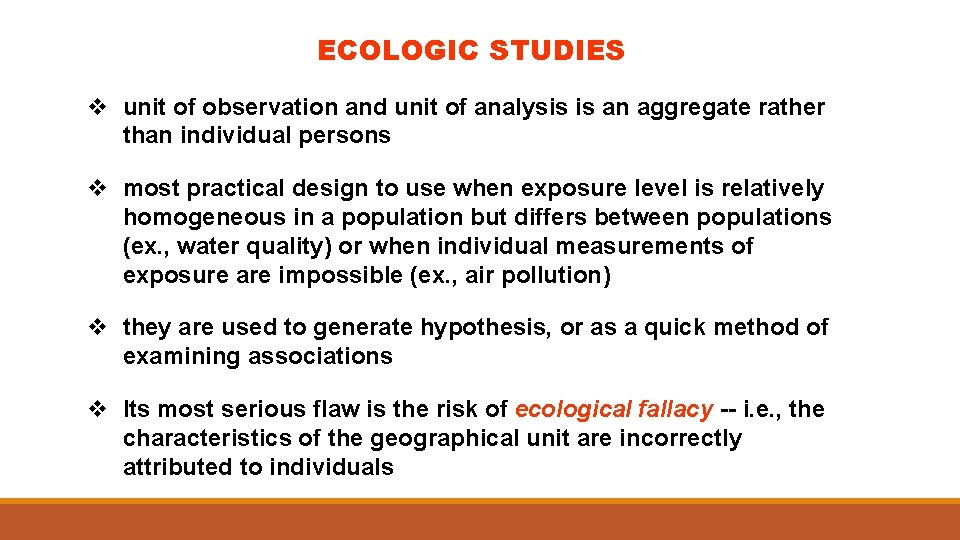
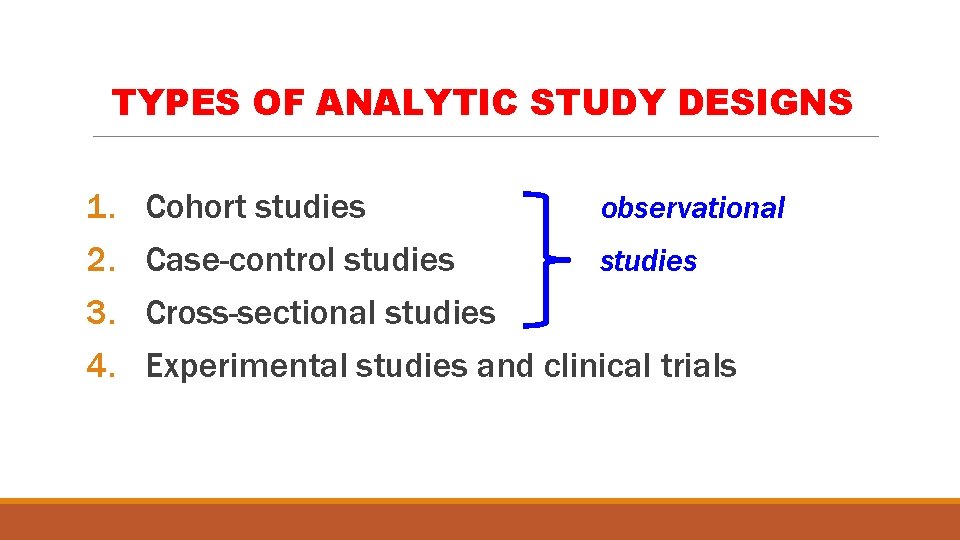
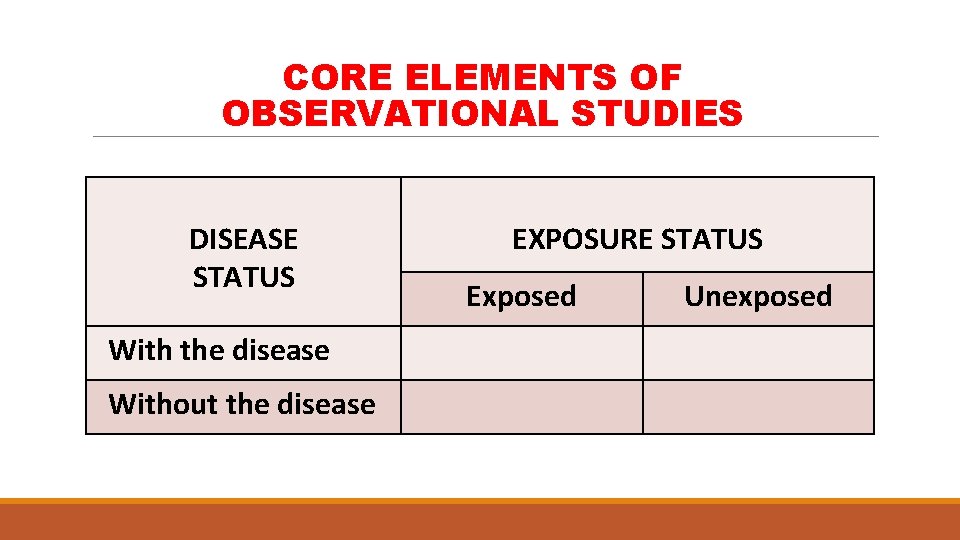
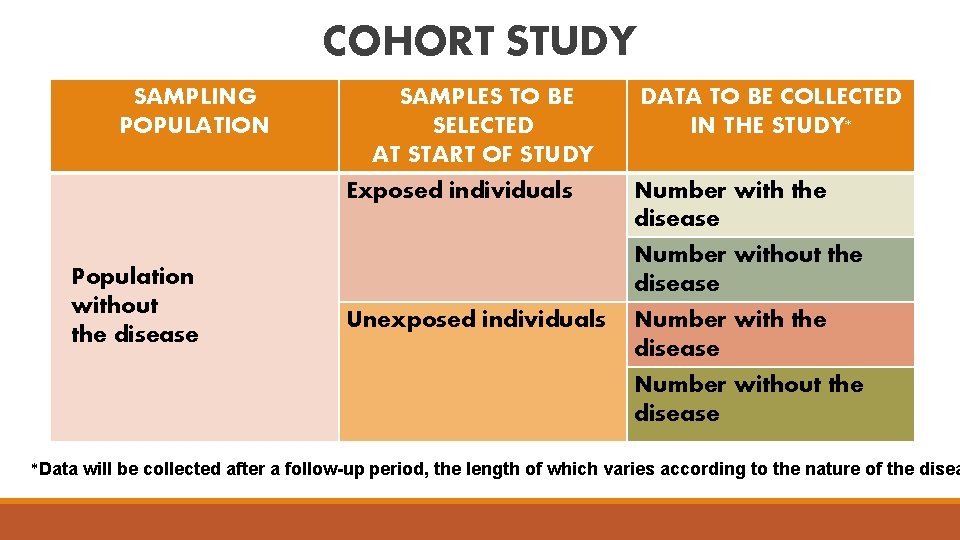
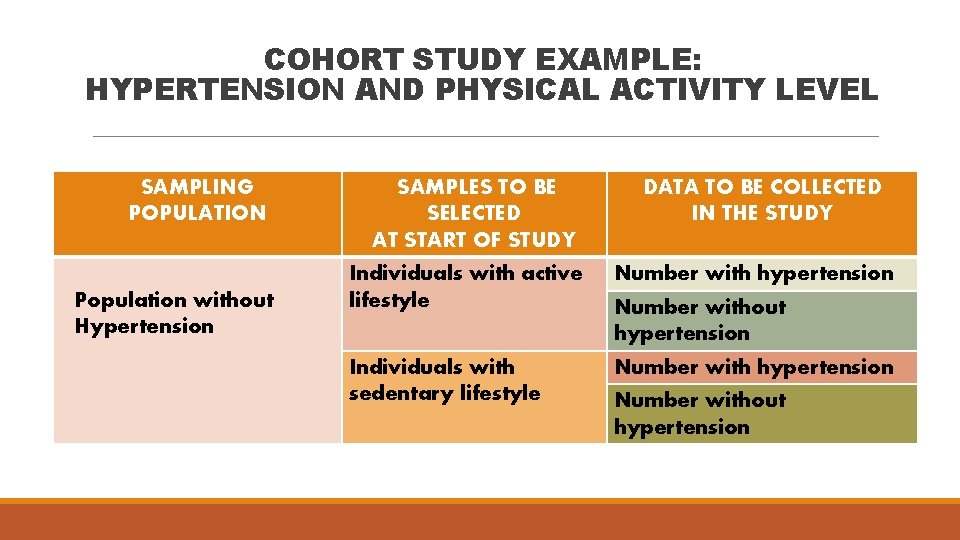
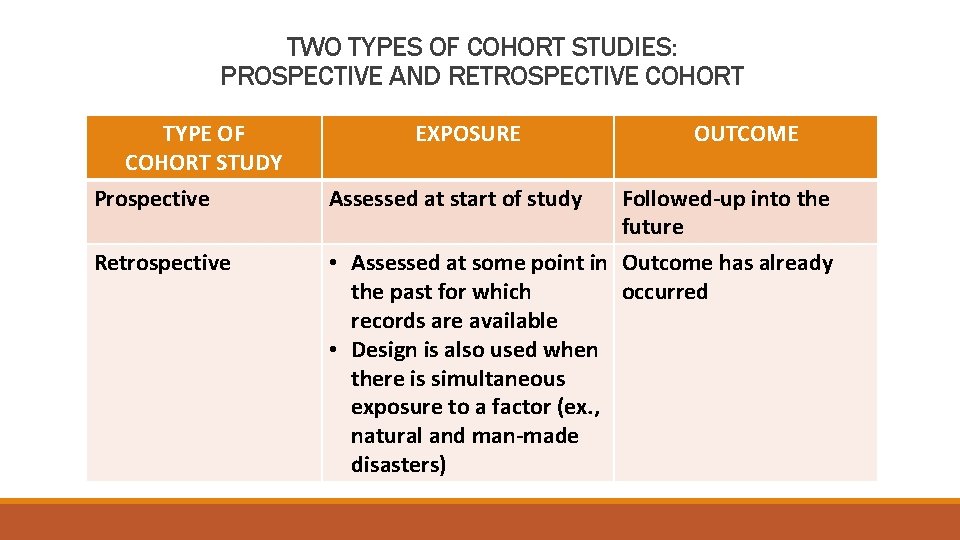
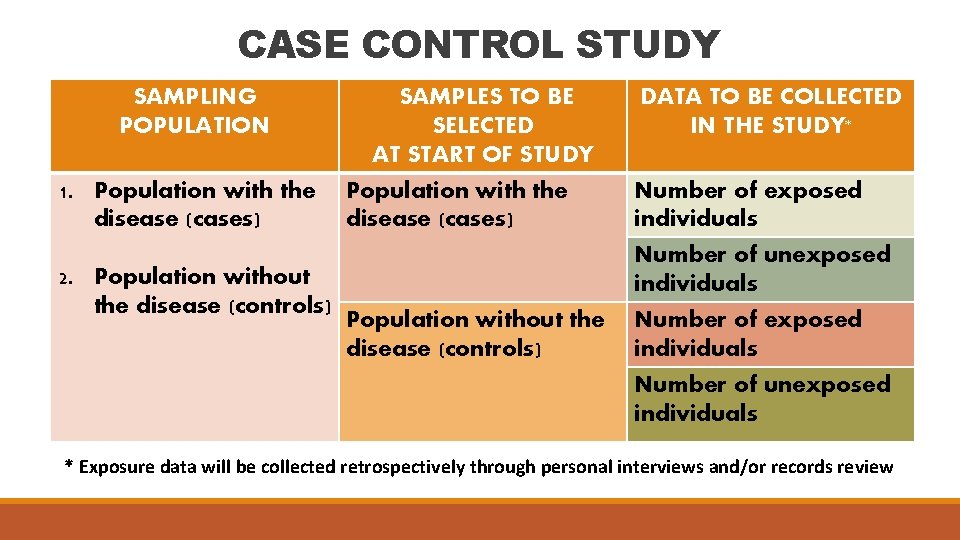
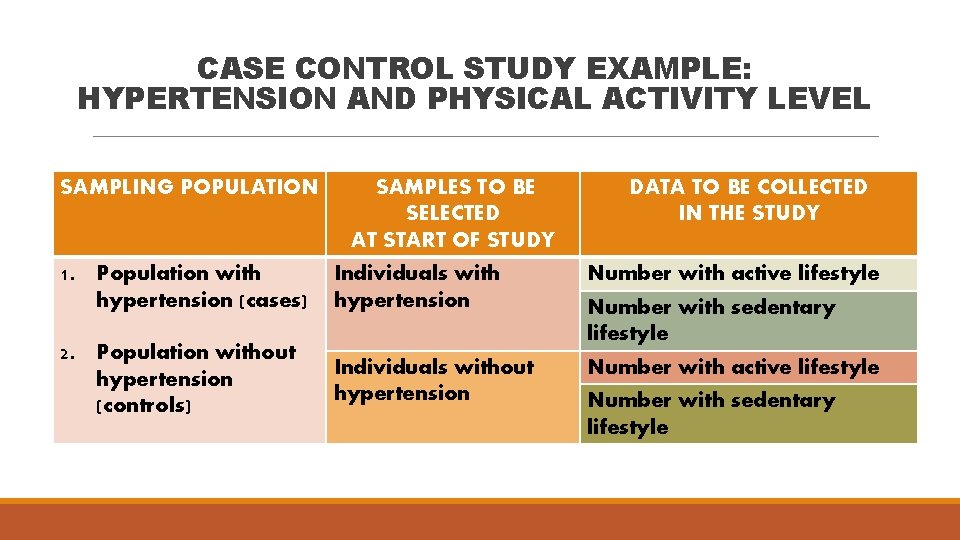
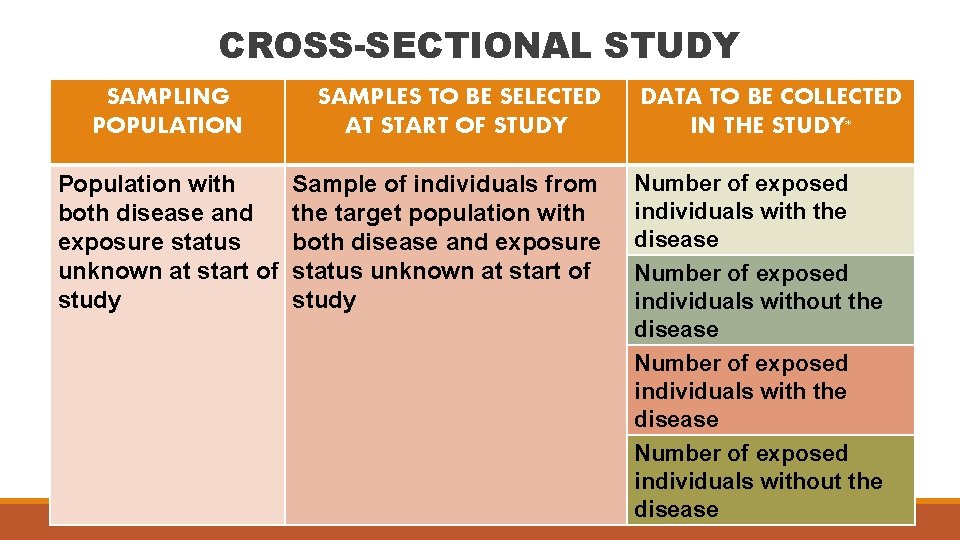
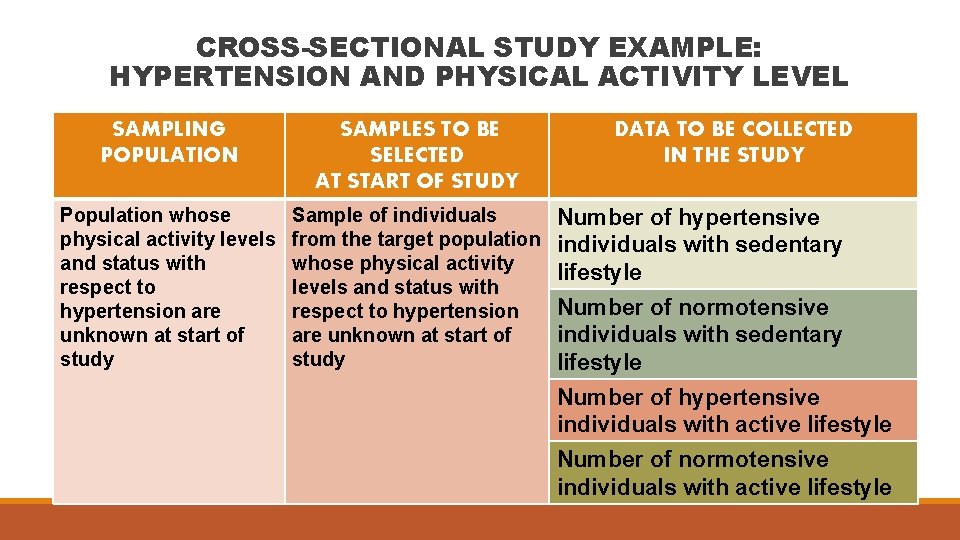
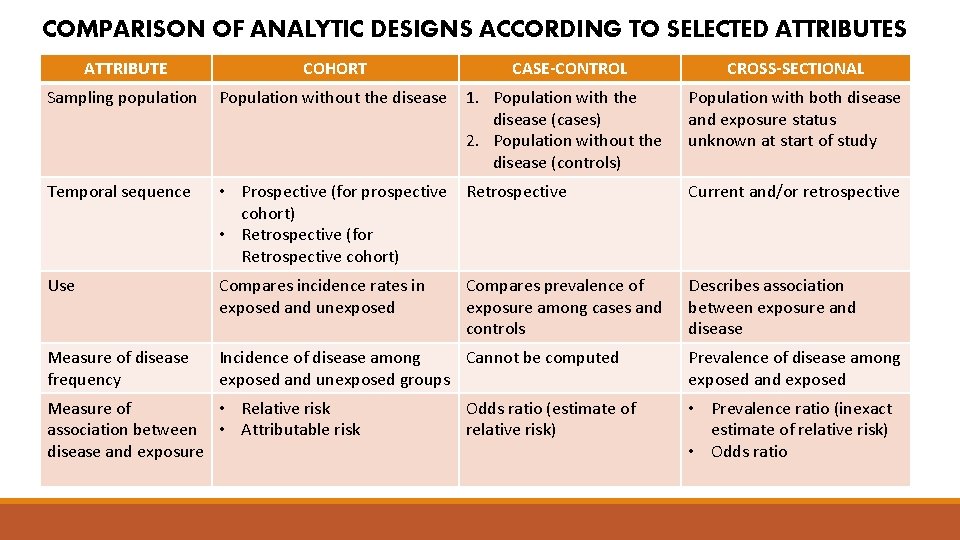
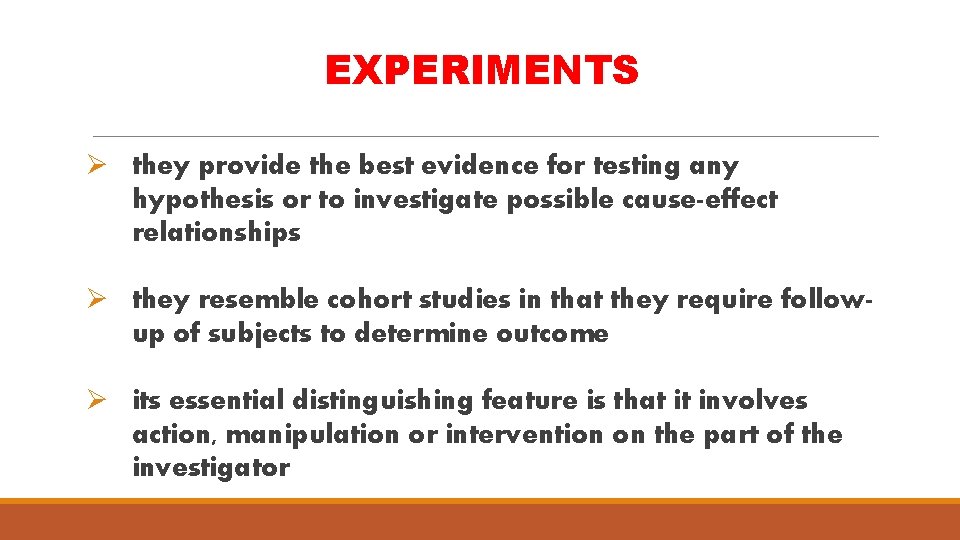
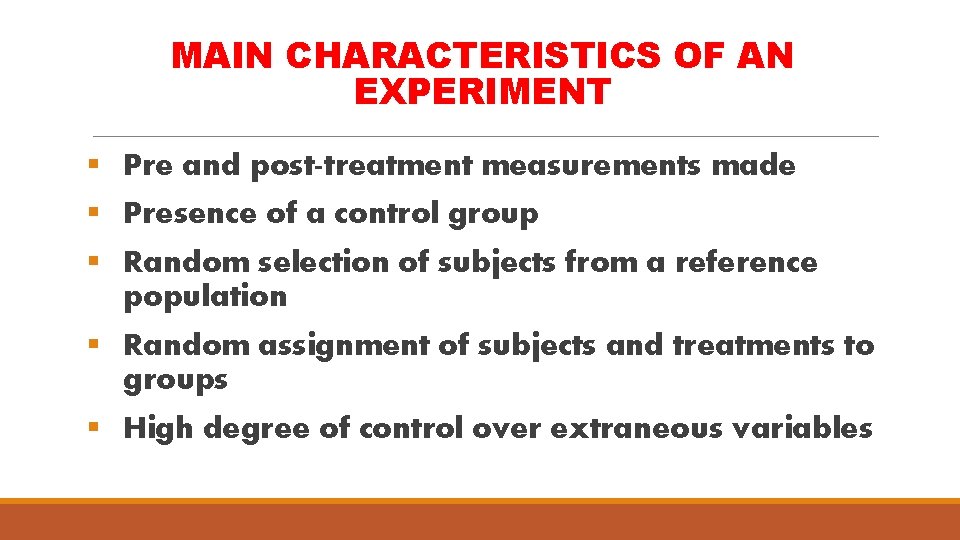
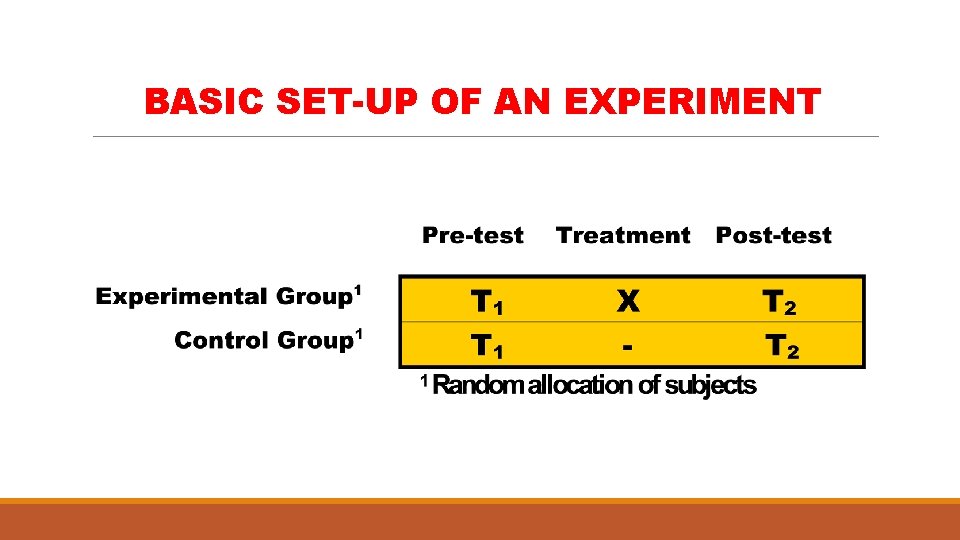
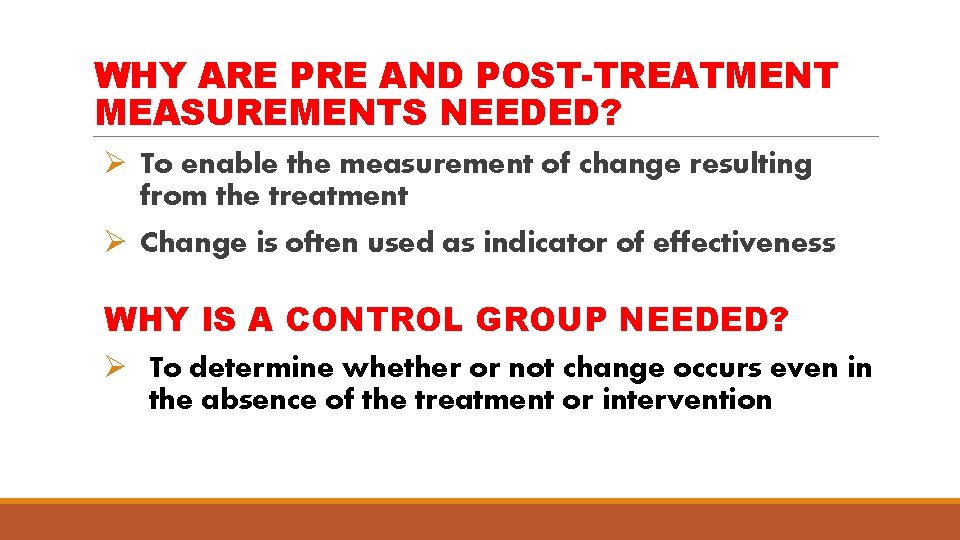
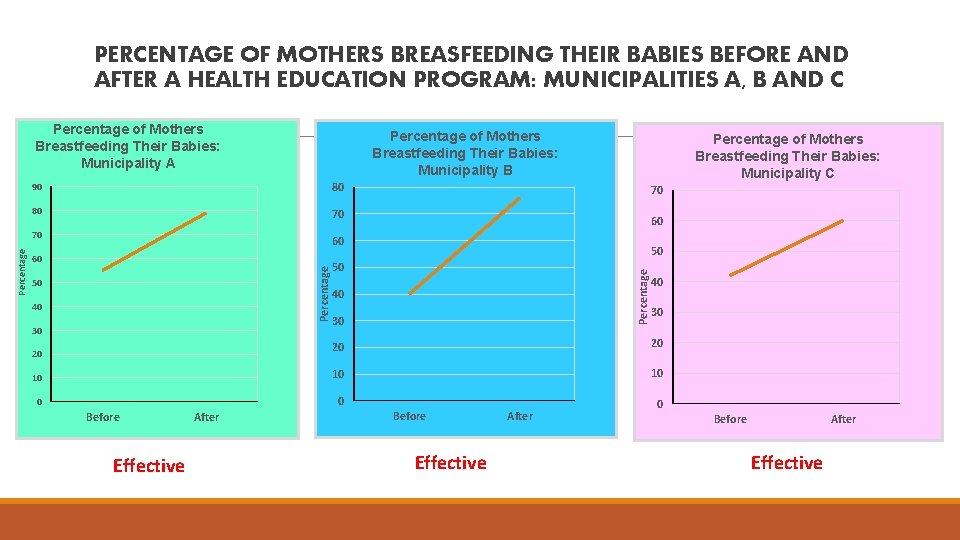
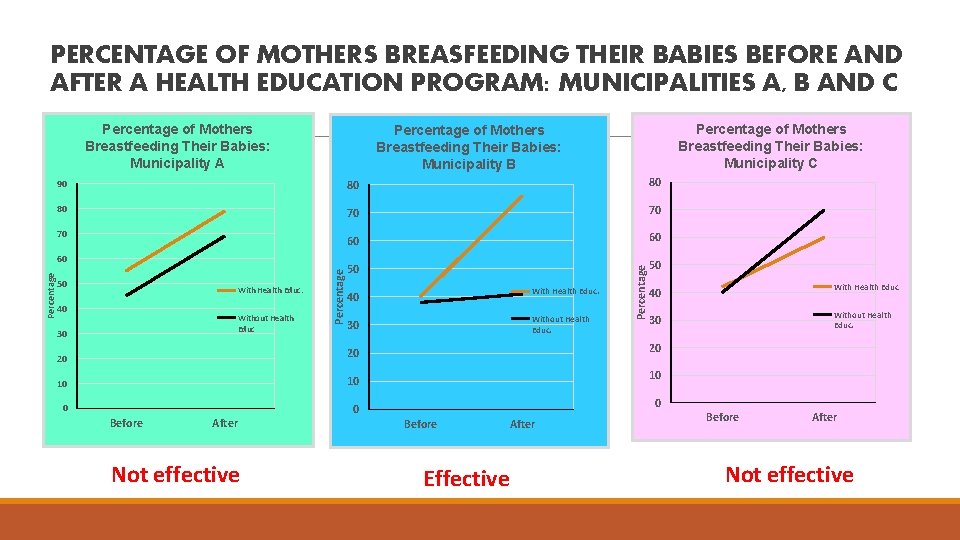
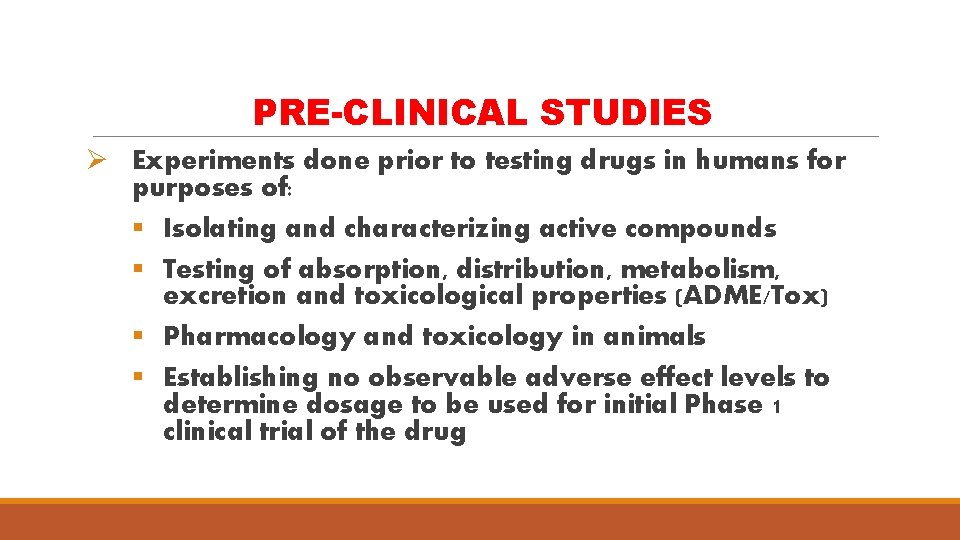
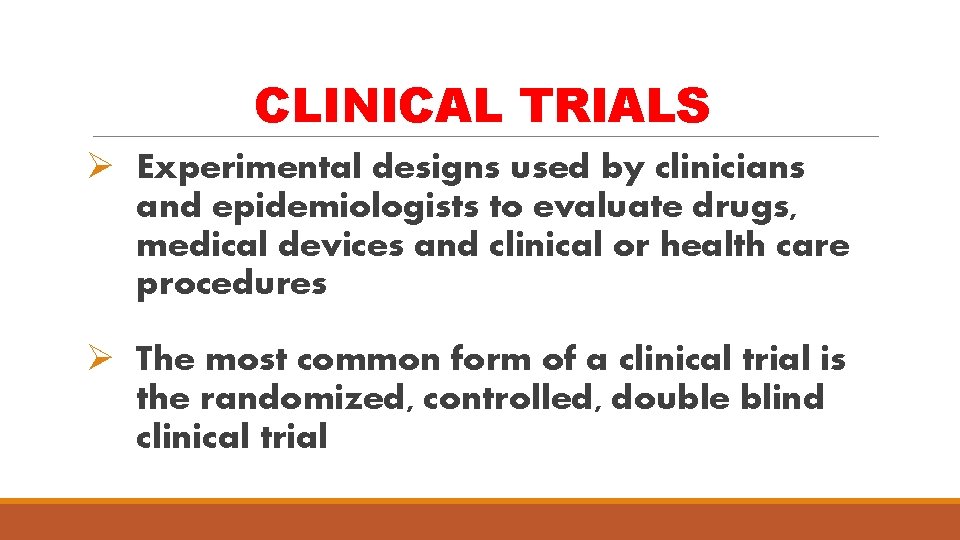
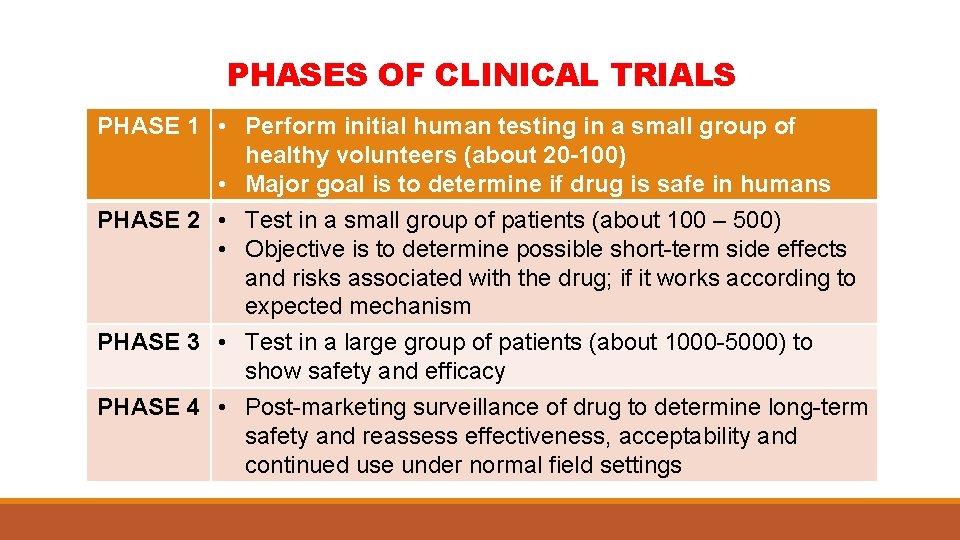
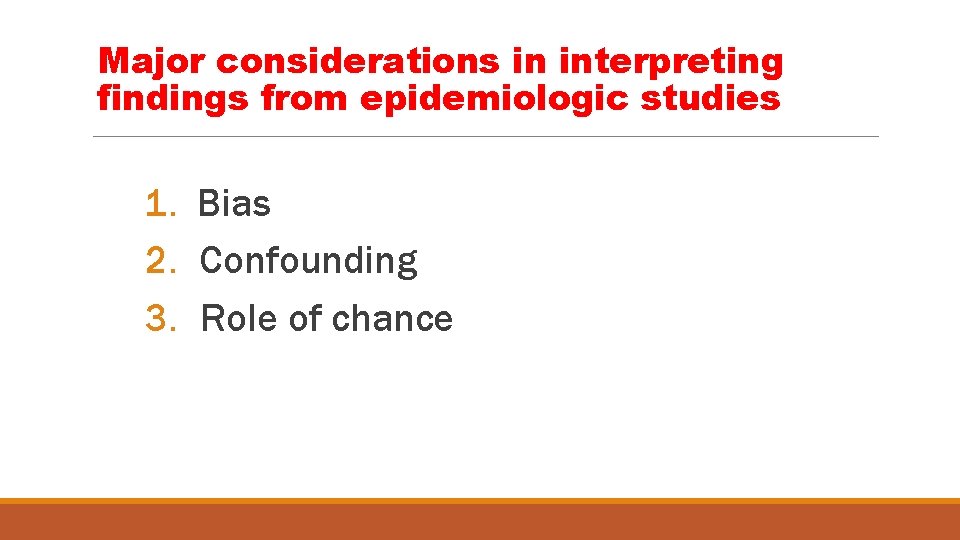
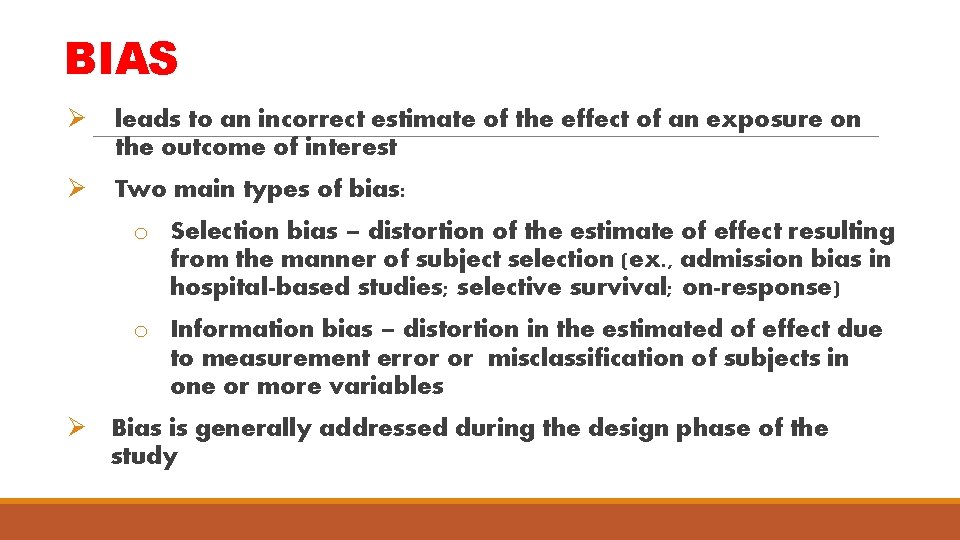
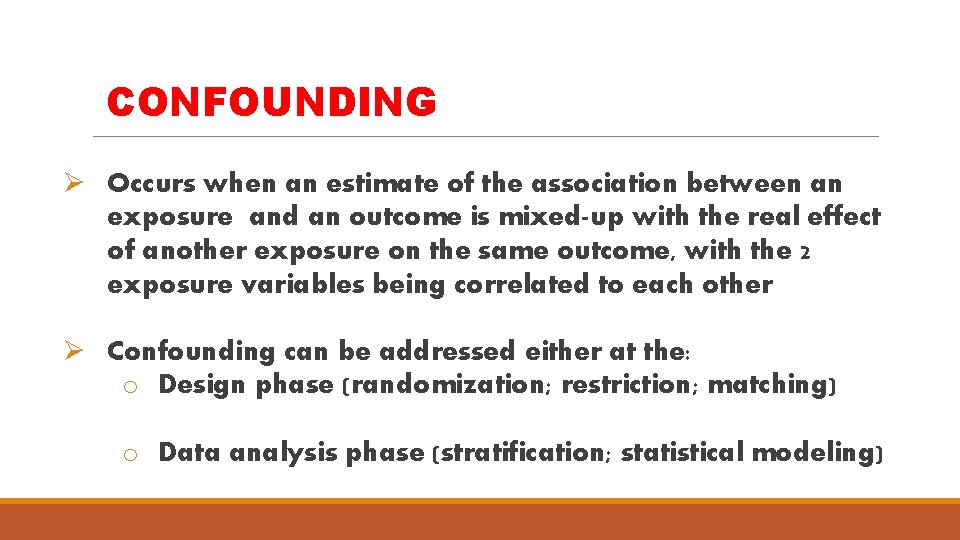
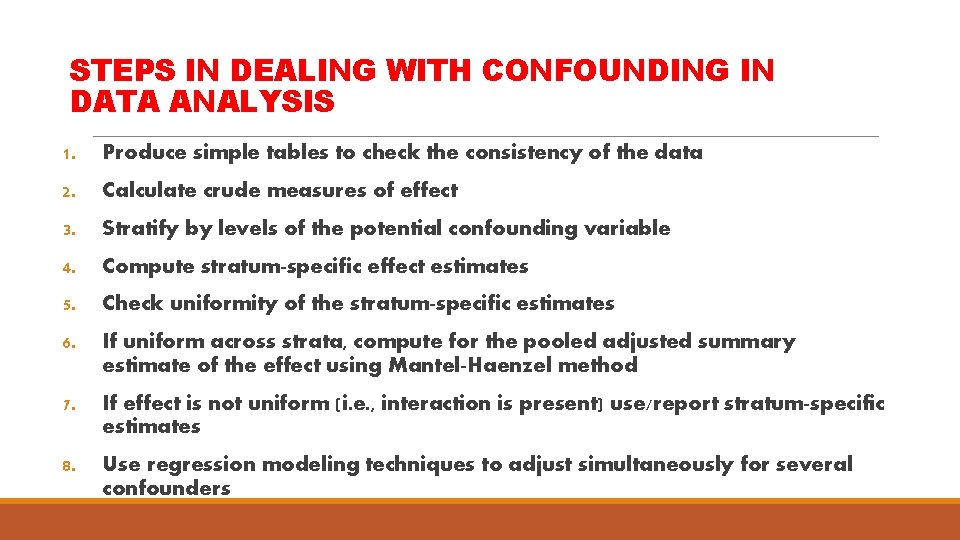
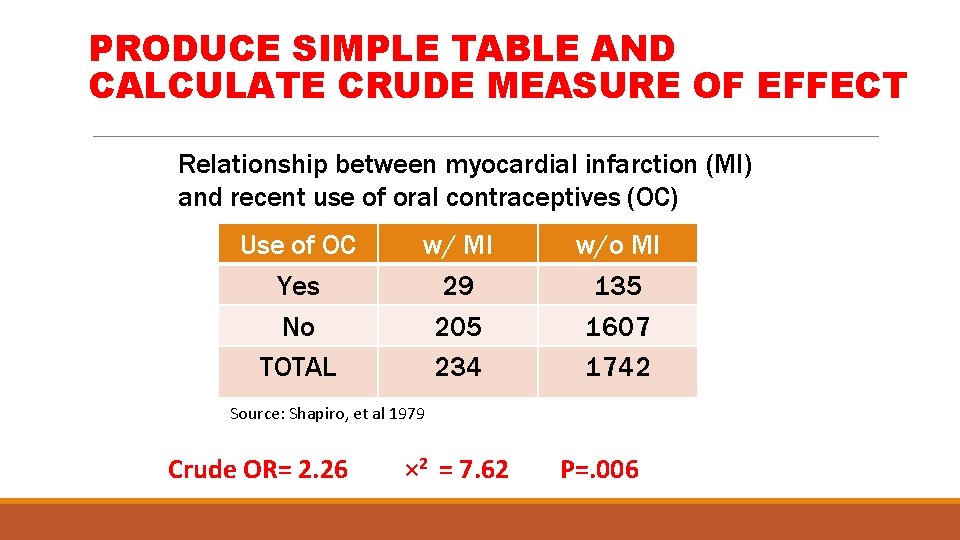
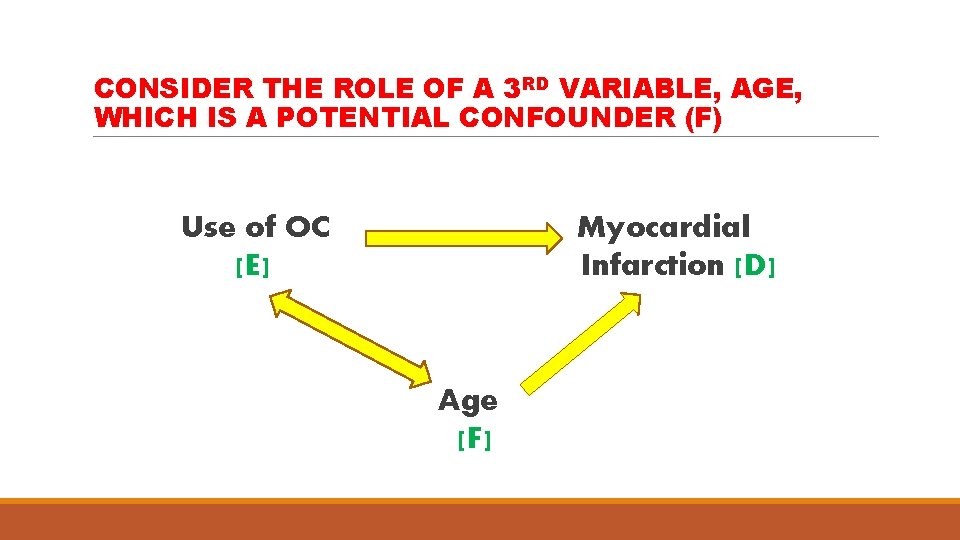
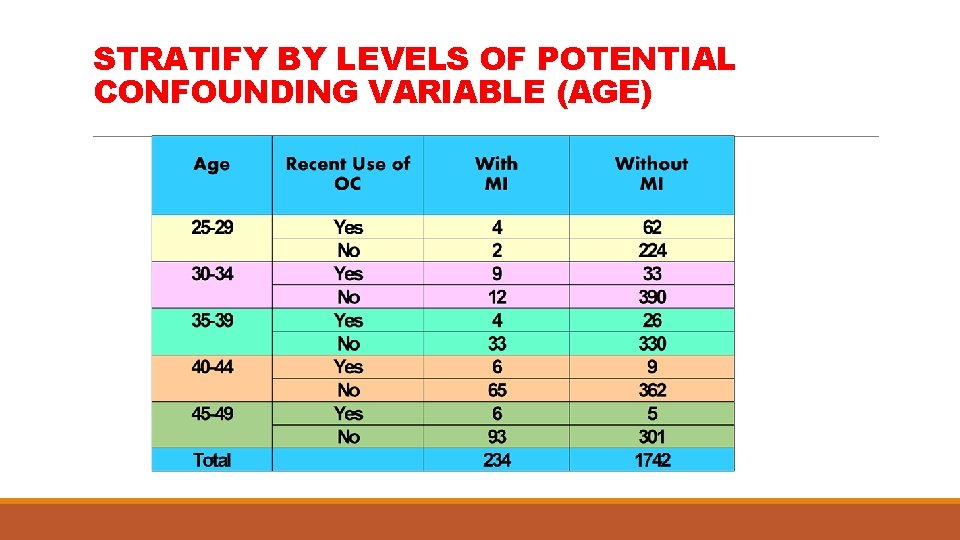
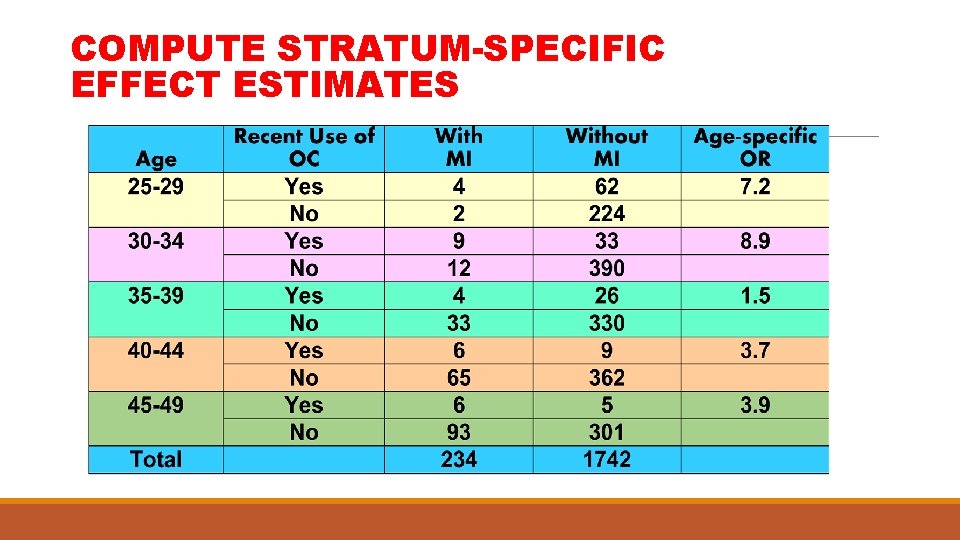
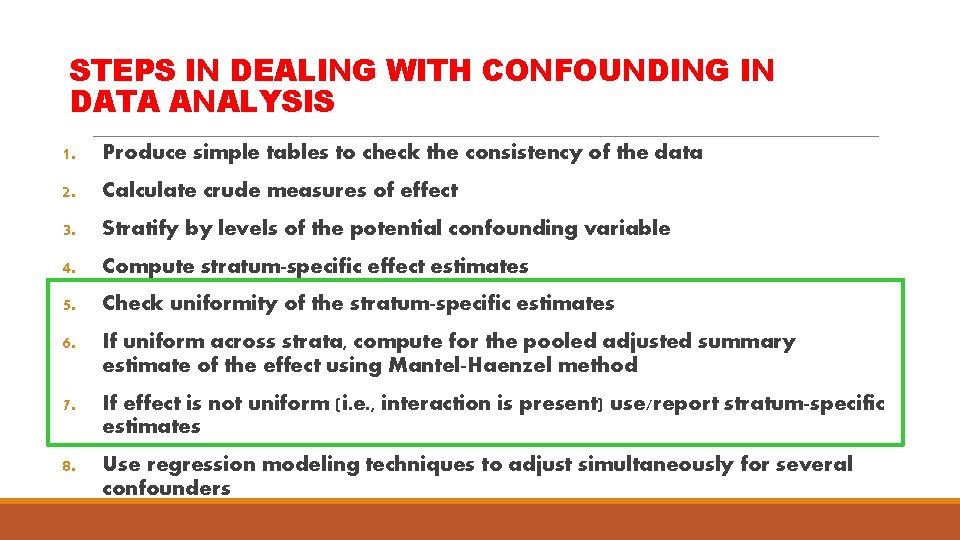
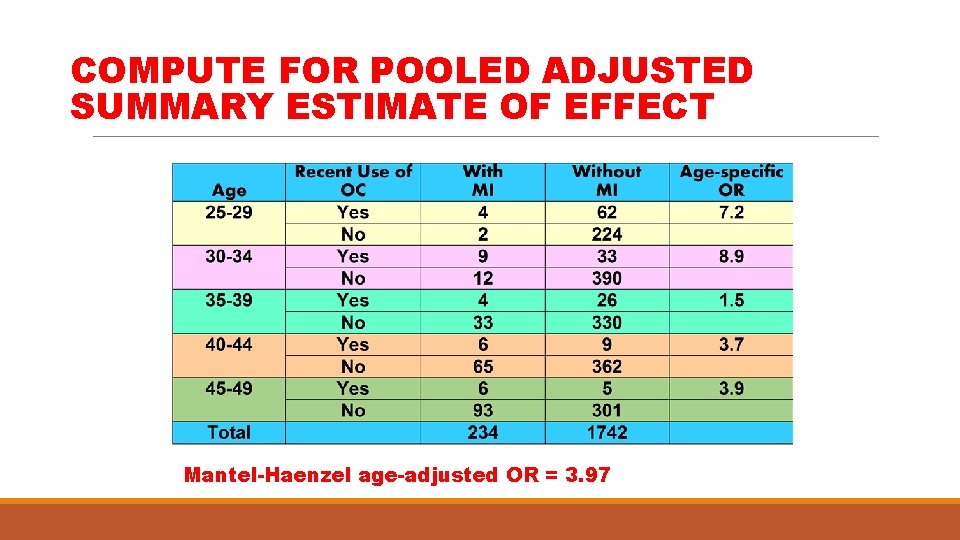
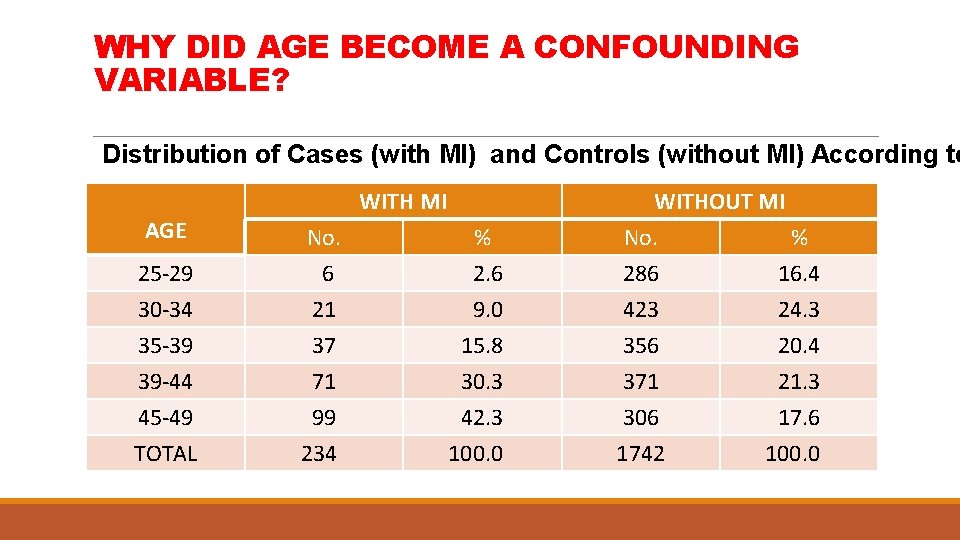
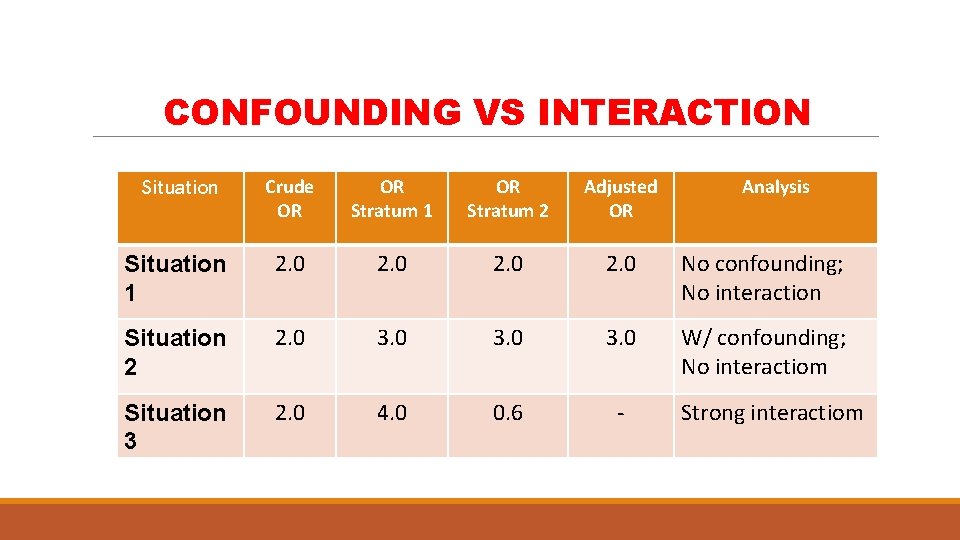
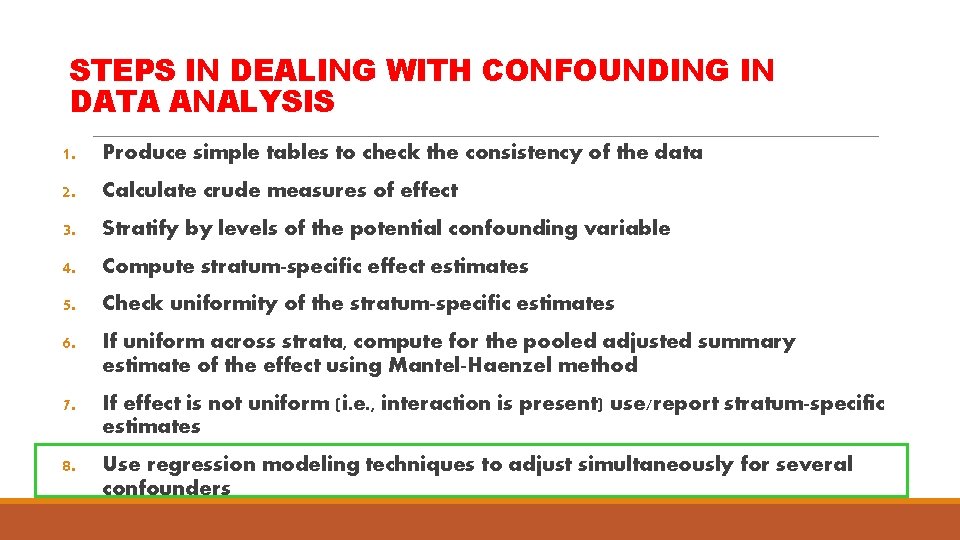
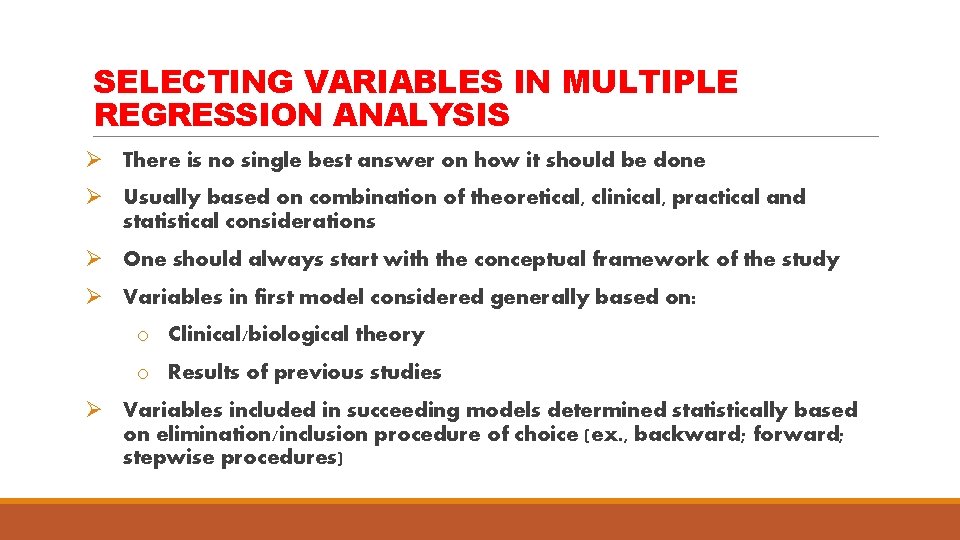
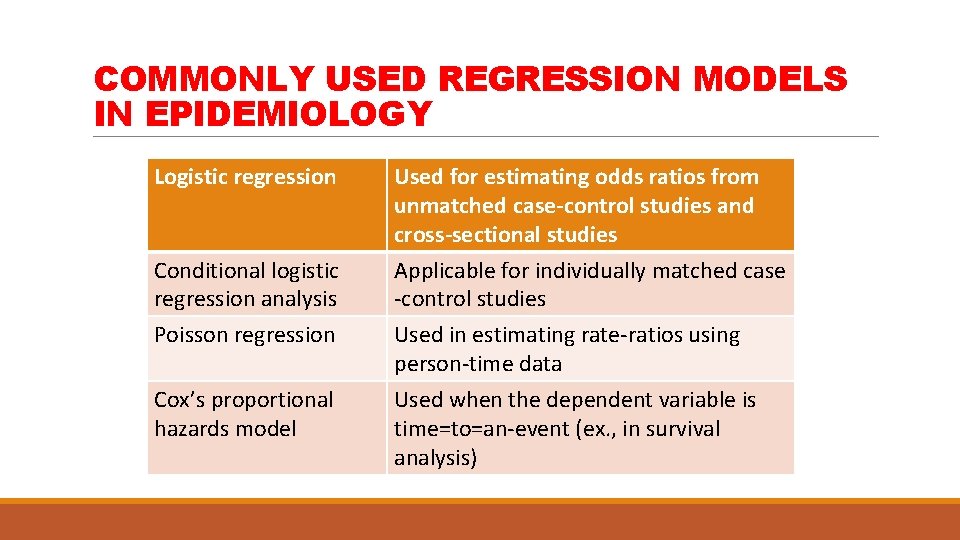
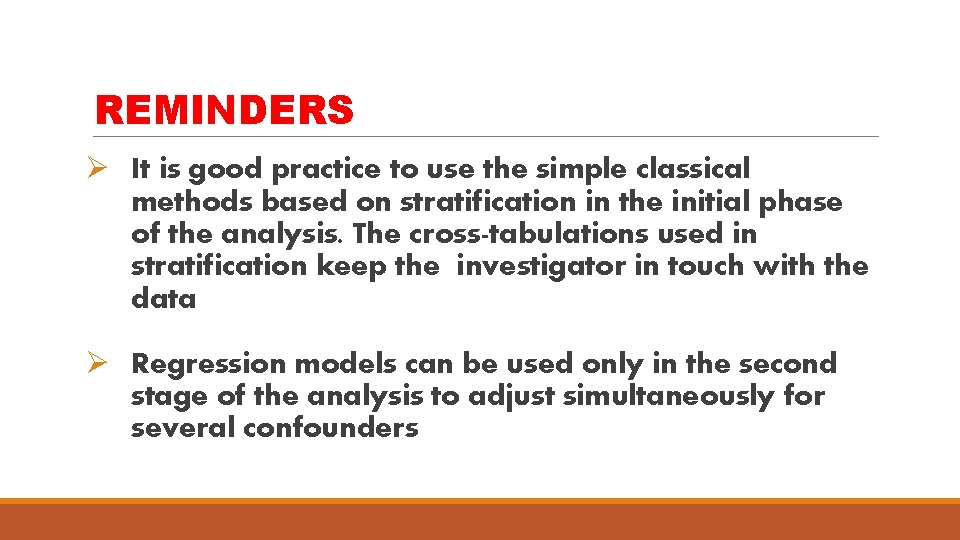
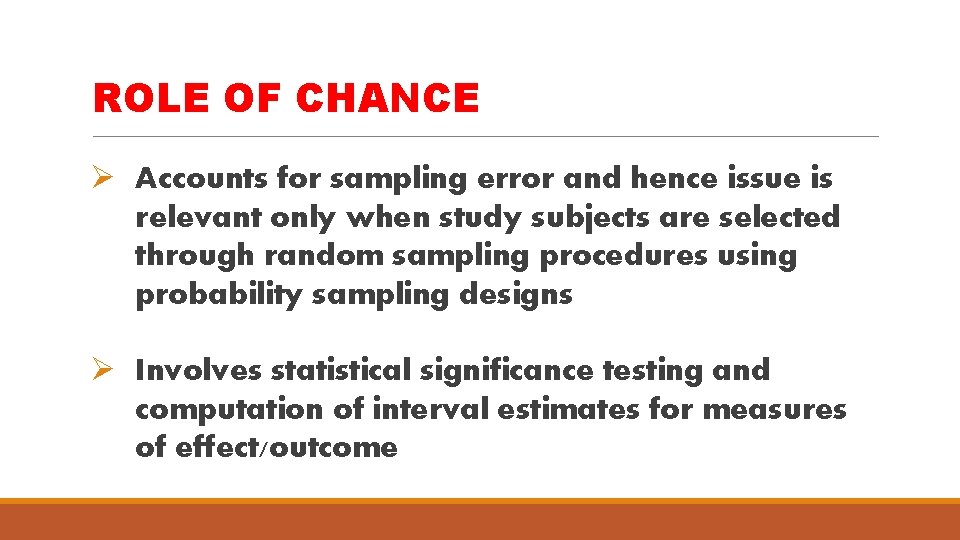
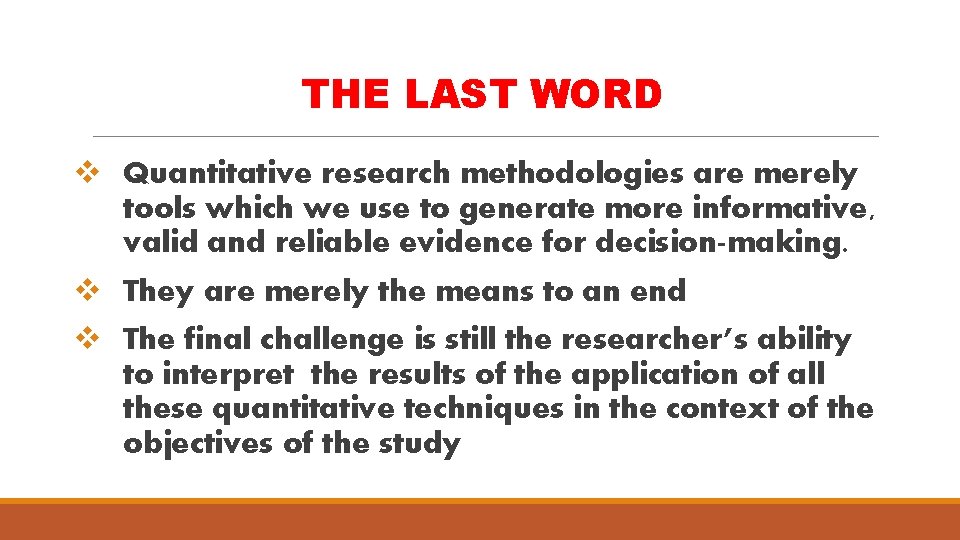
- Slides: 47
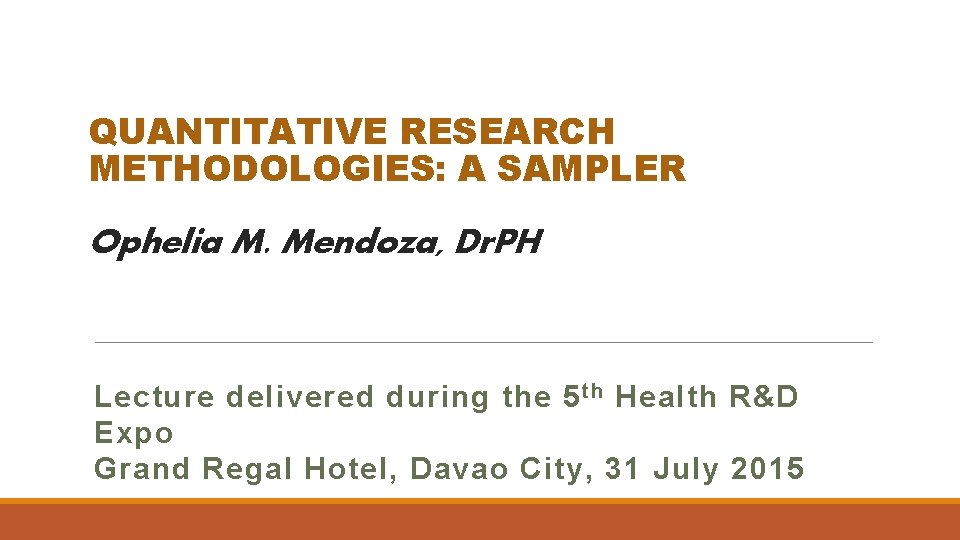
QUANTITATIVE RESEARCH METHODOLOGIES: A SAMPLER Ophelia M. Mendoza, Dr. PH Lecture delivered during the 5 t h Health R&D Expo Grand Regal Hotel, Davao City, 31 July 2015
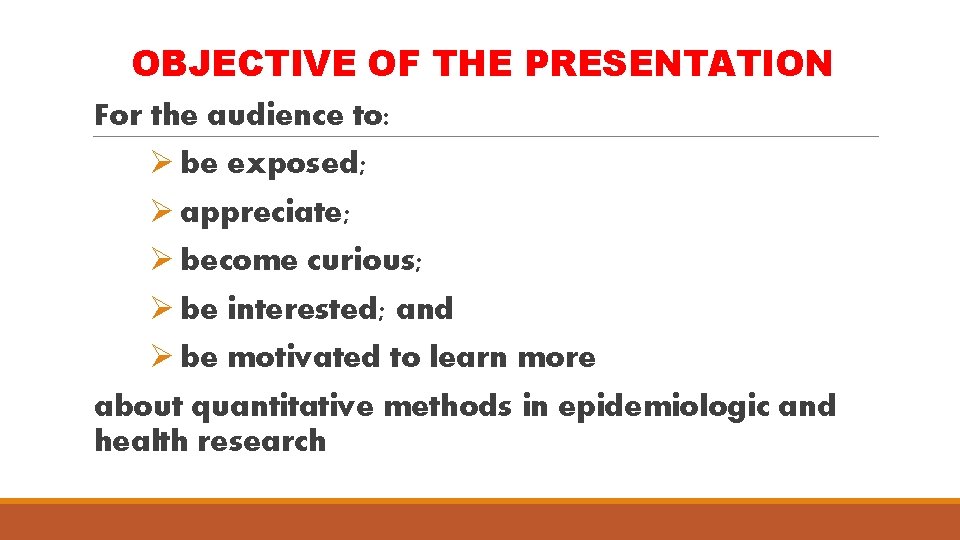
OBJECTIVE OF THE PRESENTATION For the audience to: Ø be exposed; Ø appreciate; Ø become curious; Ø be interested; and Ø be motivated to learn more about quantitative methods in epidemiologic and health research
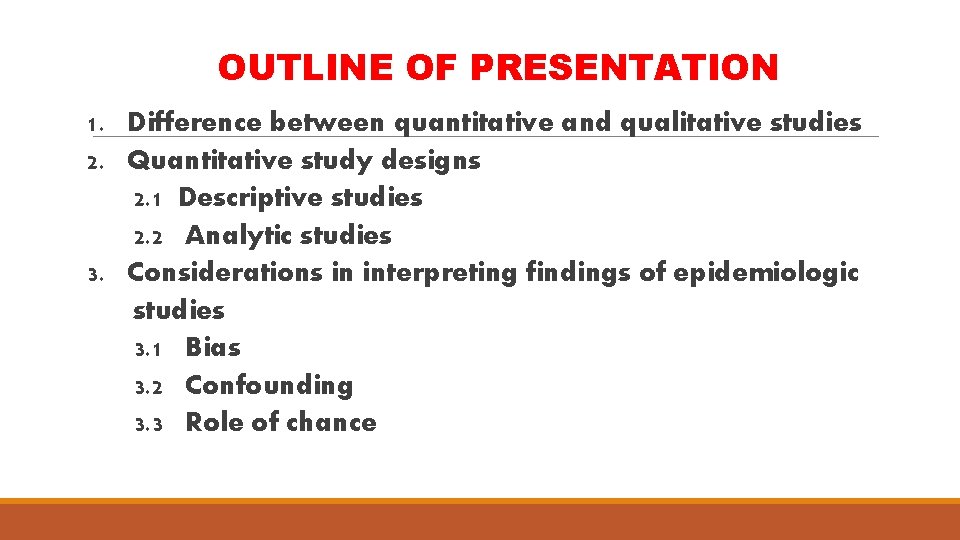
OUTLINE OF PRESENTATION 1. Difference between quantitative and qualitative studies 2. Quantitative study designs 2. 1 Descriptive studies 2. 2 Analytic studies 3. Considerations in interpreting findings of epidemiologic studies 3. 1 Bias 3. 2 Confounding 3. 3 Role of chance
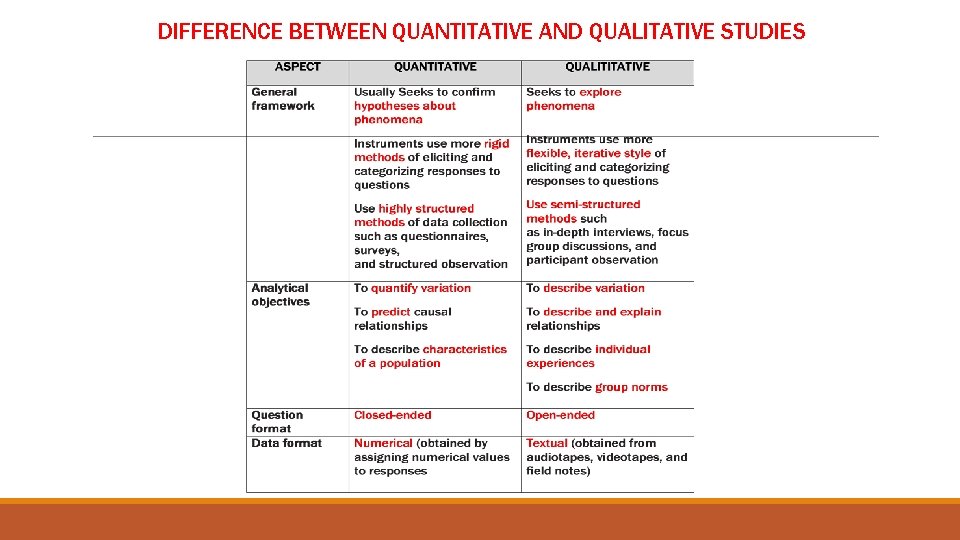
DIFFERENCE BETWEEN QUANTITATIVE AND QUALITATIVE STUDIES
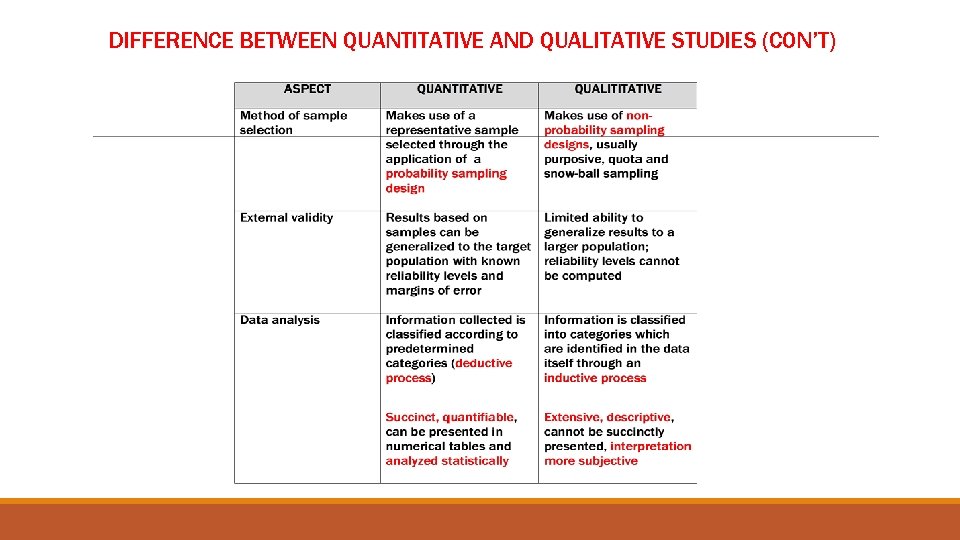
DIFFERENCE BETWEEN QUANTITATIVE AND QUALITATIVE STUDIES (CON’T)
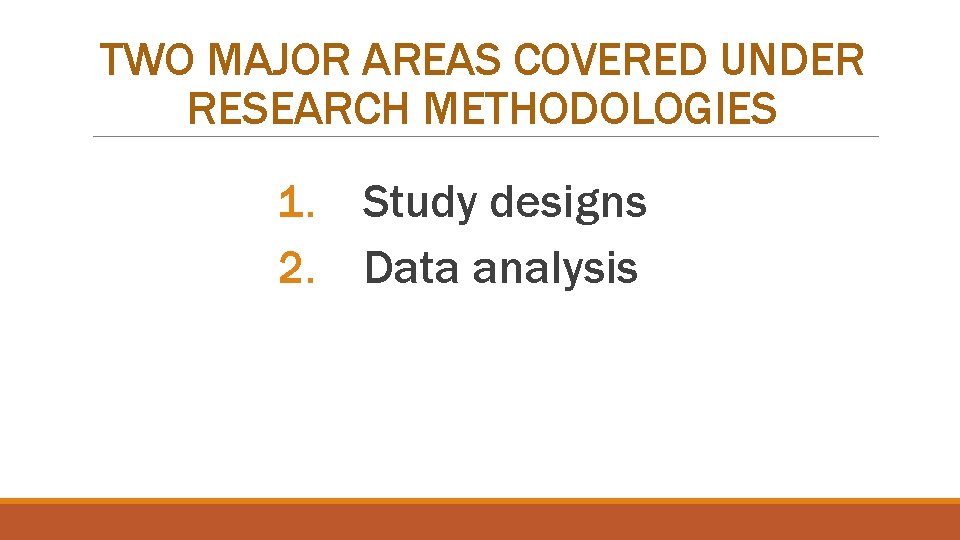
TWO MAJOR AREAS COVERED UNDER RESEARCH METHODOLOGIES 1. Study designs 2. Data analysis
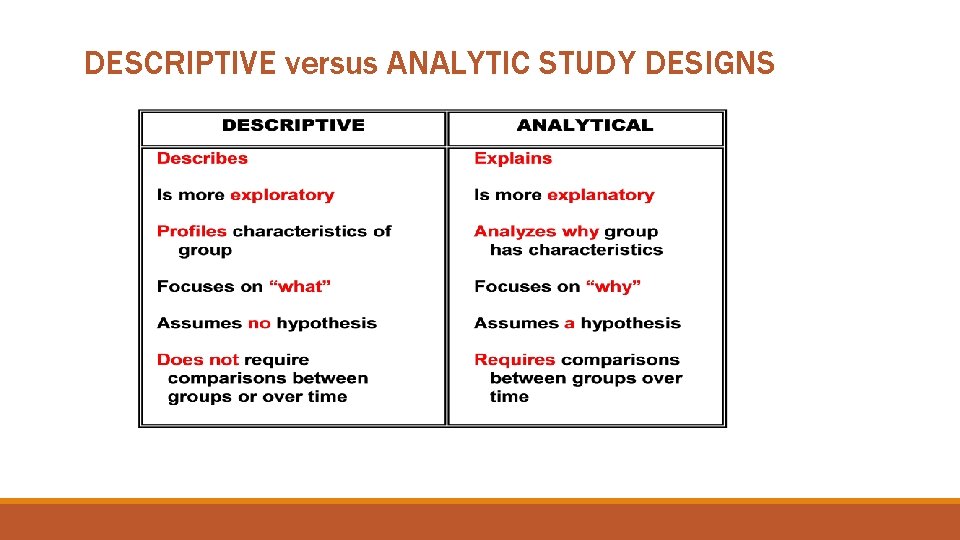
DESCRIPTIVE versus ANALYTIC STUDY DESIGNS
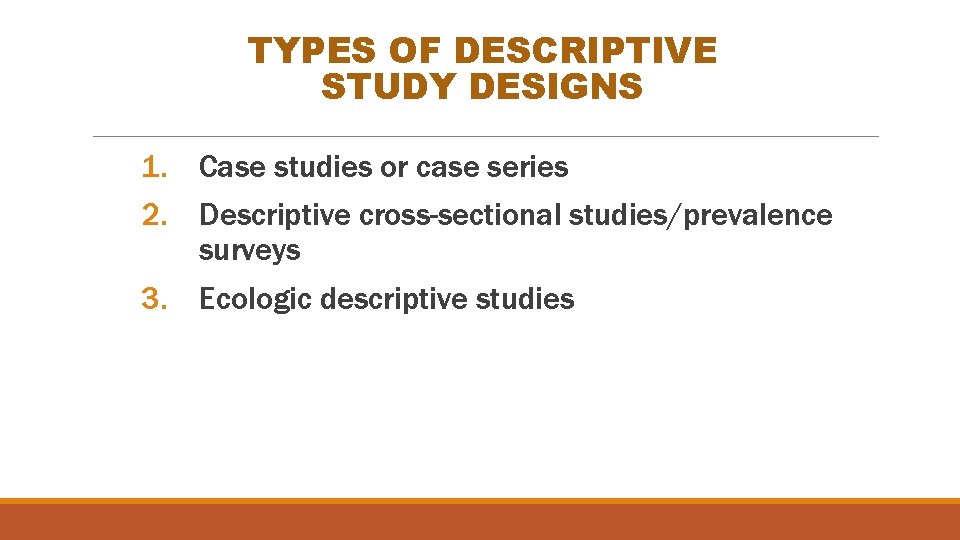
TYPES OF DESCRIPTIVE STUDY DESIGNS 1. Case studies or case series 2. Descriptive cross-sectional studies/prevalence surveys 3. Ecologic descriptive studies
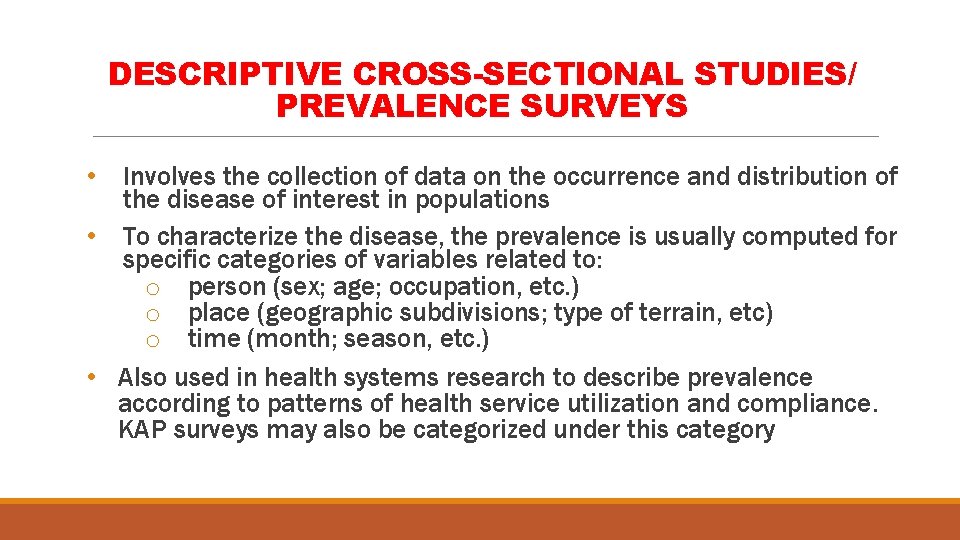
DESCRIPTIVE CROSS-SECTIONAL STUDIES/ PREVALENCE SURVEYS • Involves the collection of data on the occurrence and distribution of the disease of interest in populations • To characterize the disease, the prevalence is usually computed for specific categories of variables related to: o person (sex; age; occupation, etc. ) o place (geographic subdivisions; type of terrain, etc) o time (month; season, etc. ) • Also used in health systems research to describe prevalence according to patterns of health service utilization and compliance. KAP surveys may also be categorized under this category
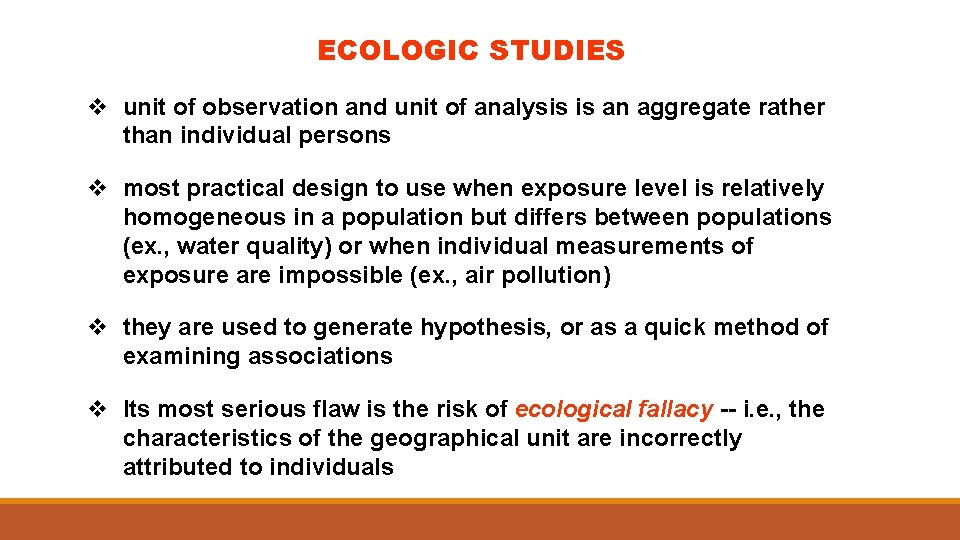
ECOLOGIC STUDIES v unit of observation and unit of analysis is an aggregate rather than individual persons v most practical design to use when exposure level is relatively homogeneous in a population but differs between populations (ex. , water quality) or when individual measurements of exposure are impossible (ex. , air pollution) v they are used to generate hypothesis, or as a quick method of examining associations v Its most serious flaw is the risk of ecological fallacy -- i. e. , the characteristics of the geographical unit are incorrectly attributed to individuals
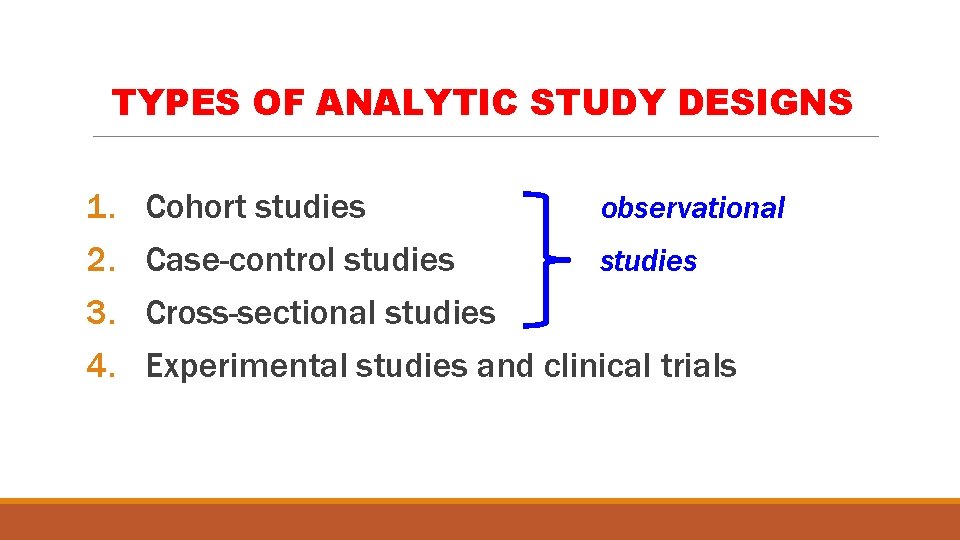
TYPES OF ANALYTIC STUDY DESIGNS 1. 2. 3. 4. Cohort studies observational Case-control studies Cross-sectional studies Experimental studies and clinical trials
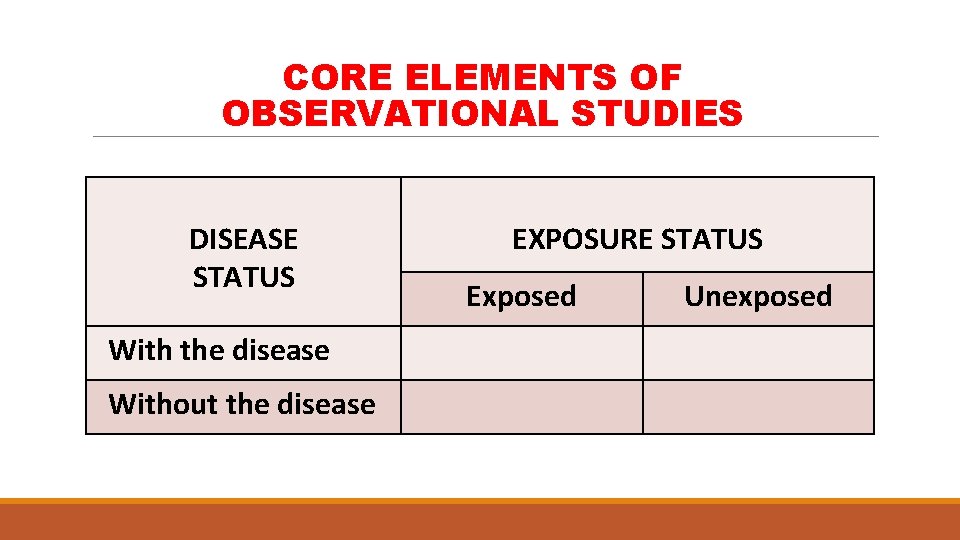
CORE ELEMENTS OF OBSERVATIONAL STUDIES DISEASE STATUS With the disease Without the disease EXPOSURE STATUS Exposed Unexposed
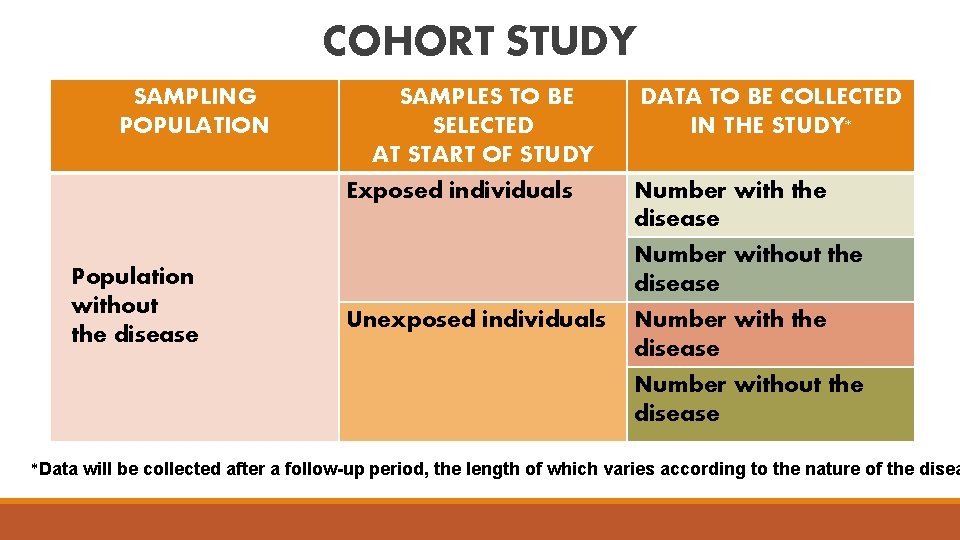
COHORT STUDY SAMPLING POPULATION Population without the disease SAMPLES TO BE SELECTED AT START OF STUDY Exposed individuals Unexposed individuals DATA TO BE COLLECTED IN THE STUDY* Number with the disease Number without the disease *Data will be collected after a follow-up period, the length of which varies according to the nature of the disea
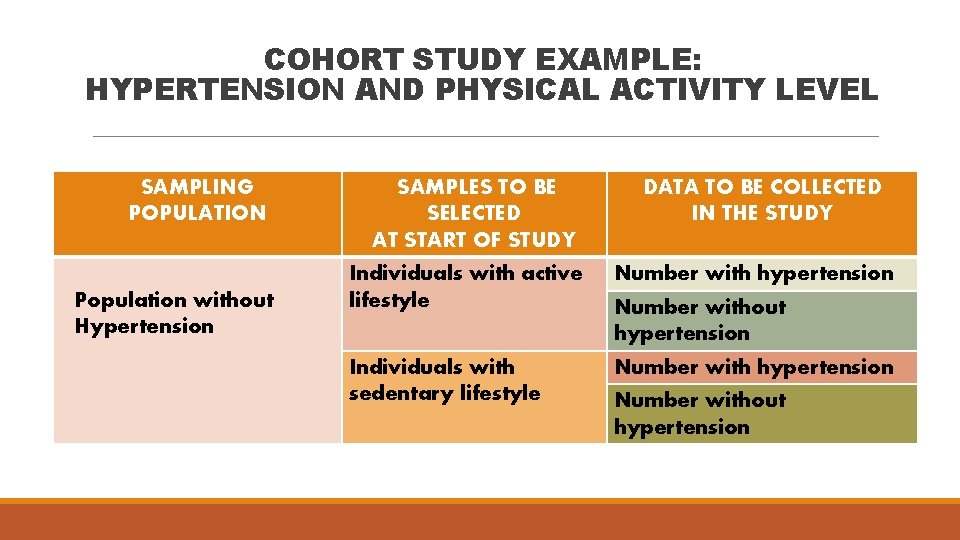
COHORT STUDY EXAMPLE: HYPERTENSION AND PHYSICAL ACTIVITY LEVEL SAMPLING POPULATION Population without Hypertension SAMPLES TO BE SELECTED AT START OF STUDY DATA TO BE COLLECTED IN THE STUDY Individuals with active lifestyle Number with hypertension Individuals with sedentary lifestyle Number with hypertension Number without hypertension
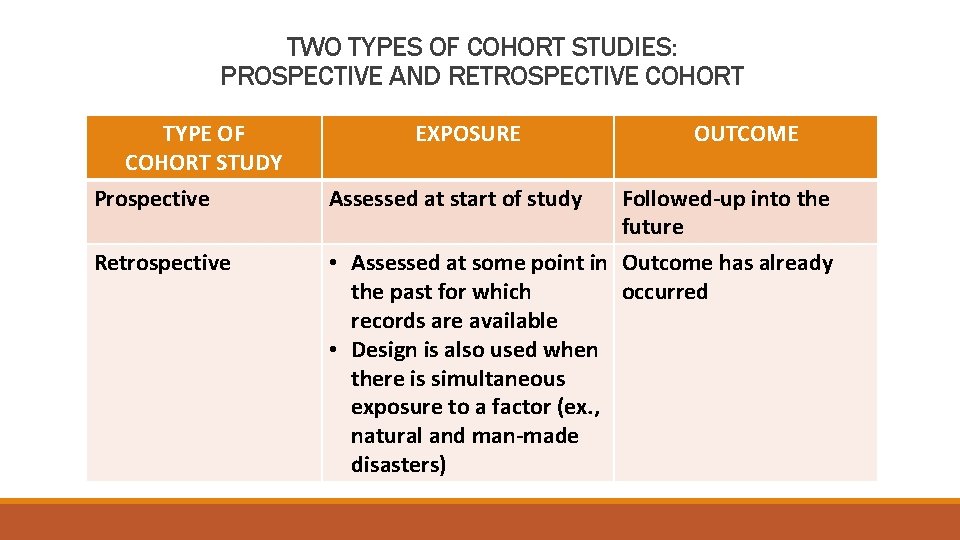
TWO TYPES OF COHORT STUDIES: PROSPECTIVE AND RETROSPECTIVE COHORT TYPE OF COHORT STUDY Prospective Retrospective EXPOSURE Assessed at start of study OUTCOME Followed-up into the future • Assessed at some point in Outcome has already the past for which occurred records are available • Design is also used when there is simultaneous exposure to a factor (ex. , natural and man-made disasters)
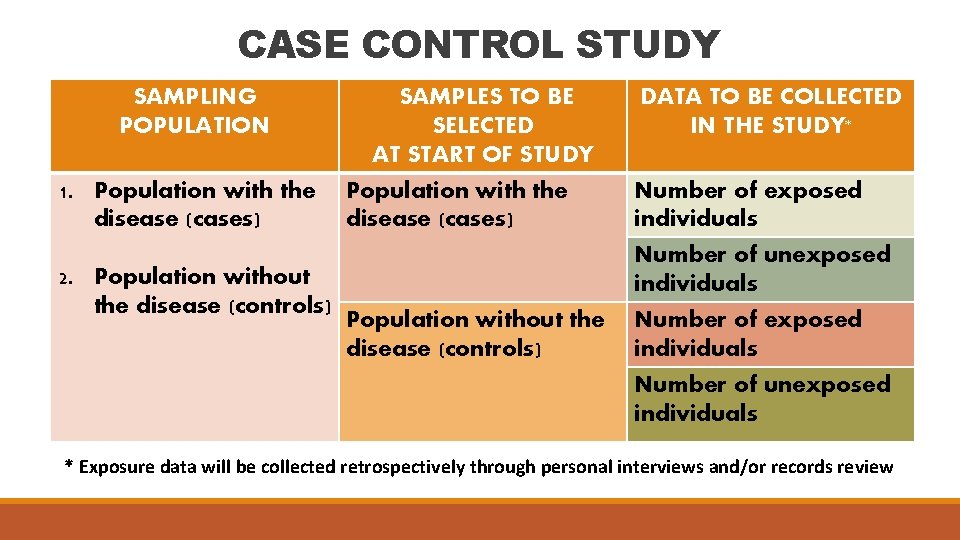
CASE CONTROL STUDY SAMPLING POPULATION 1. Population with the disease (cases) 2. Population without the disease (controls) SAMPLES TO BE SELECTED AT START OF STUDY Population with the disease (cases) Population without the disease (controls) DATA TO BE COLLECTED IN THE STUDY* Number of exposed individuals Number of unexposed individuals * Exposure data will be collected retrospectively through personal interviews and/or records review
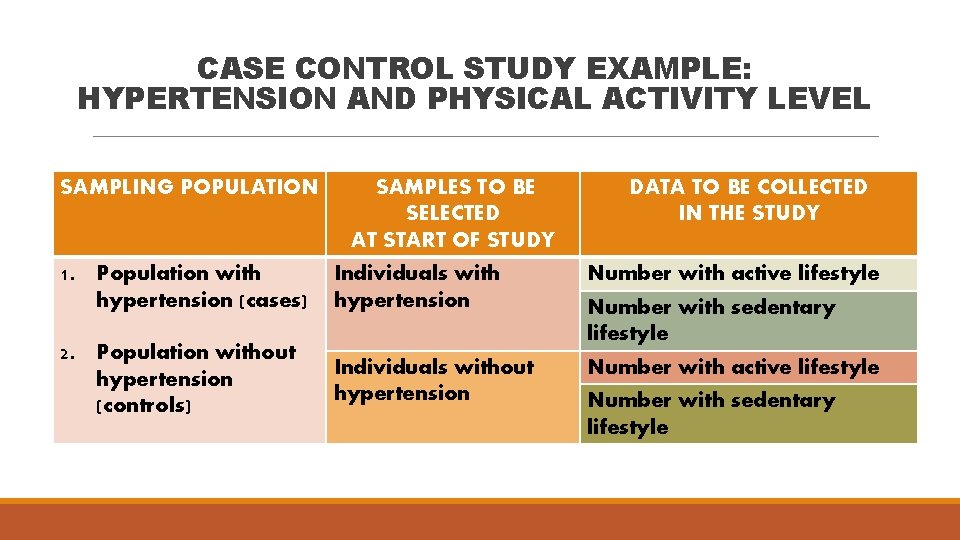
CASE CONTROL STUDY EXAMPLE: HYPERTENSION AND PHYSICAL ACTIVITY LEVEL SAMPLING POPULATION SAMPLES TO BE SELECTED AT START OF STUDY DATA TO BE COLLECTED IN THE STUDY 1. Population with hypertension (cases) Individuals with hypertension Number with active lifestyle 2. Population without hypertension (controls) Individuals without hypertension Number with active lifestyle Number with sedentary lifestyle
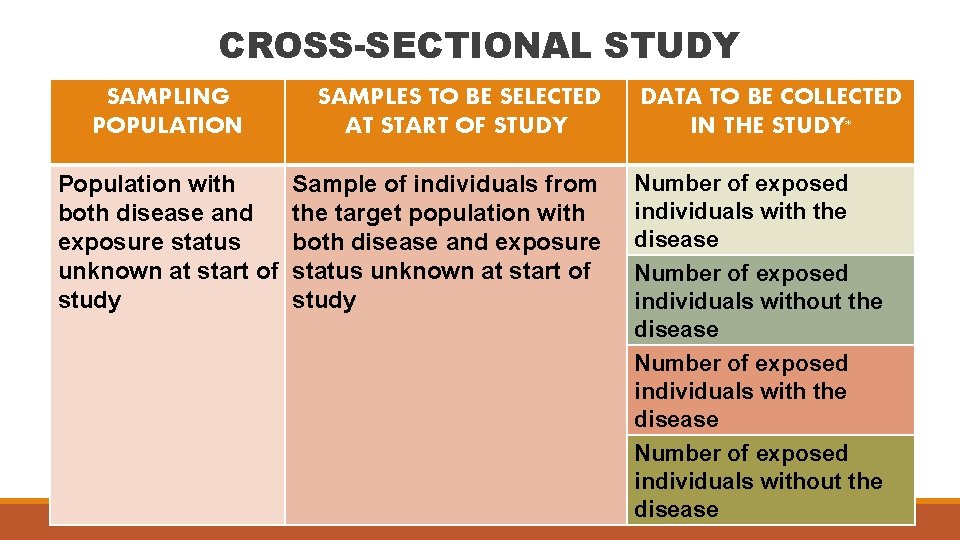
CROSS-SECTIONAL STUDY SAMPLING POPULATION Population with both disease and exposure status unknown at start of study SAMPLES TO BE SELECTED AT START OF STUDY Sample of individuals from the target population with both disease and exposure status unknown at start of study DATA TO BE COLLECTED IN THE STUDY* Number of exposed individuals with the disease Number of exposed individuals without the disease
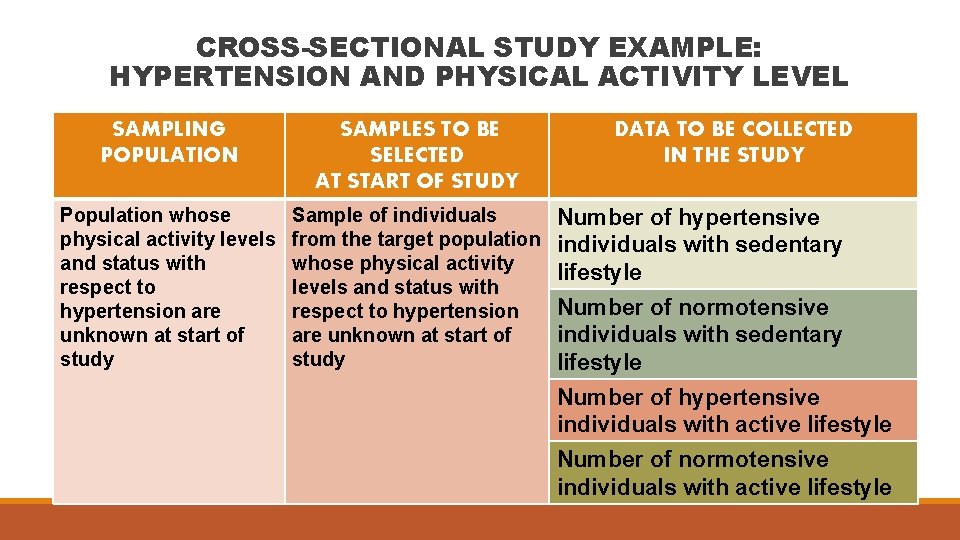
CROSS-SECTIONAL STUDY EXAMPLE: HYPERTENSION AND PHYSICAL ACTIVITY LEVEL SAMPLING POPULATION SAMPLES TO BE SELECTED AT START OF STUDY Population whose physical activity levels and status with respect to hypertension are unknown at start of study Sample of individuals from the target population whose physical activity levels and status with respect to hypertension are unknown at start of study DATA TO BE COLLECTED IN THE STUDY Number of hypertensive individuals with sedentary lifestyle Number of normotensive individuals with sedentary lifestyle Number of hypertensive individuals with active lifestyle Number of normotensive individuals with active lifestyle
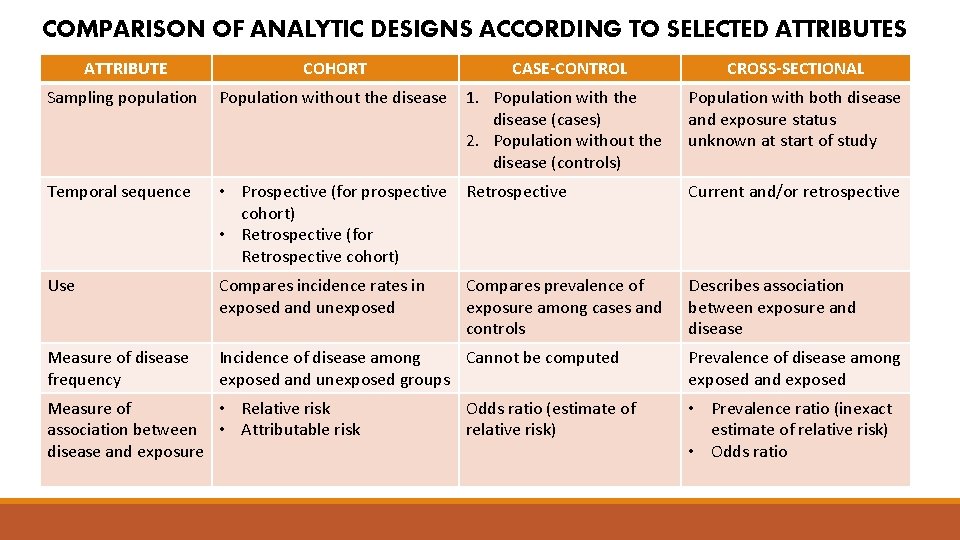
COMPARISON OF ANALYTIC DESIGNS ACCORDING TO SELECTED ATTRIBUTES ATTRIBUTE COHORT Sampling population Population without the disease 1. Population with the disease (cases) 2. Population without the disease (controls) Population with both disease and exposure status unknown at start of study Temporal sequence • Prospective (for prospective cohort) • Retrospective (for Retrospective cohort) Retrospective Current and/or retrospective Use Compares incidence rates in exposed and unexposed Compares prevalence of exposure among cases and controls Describes association between exposure and disease Measure of disease frequency Incidence of disease among Cannot be computed exposed and unexposed groups Measure of • Relative risk association between • Attributable risk disease and exposure CASE-CONTROL Odds ratio (estimate of relative risk) CROSS-SECTIONAL Prevalence of disease among exposed and exposed • Prevalence ratio (inexact estimate of relative risk) • Odds ratio
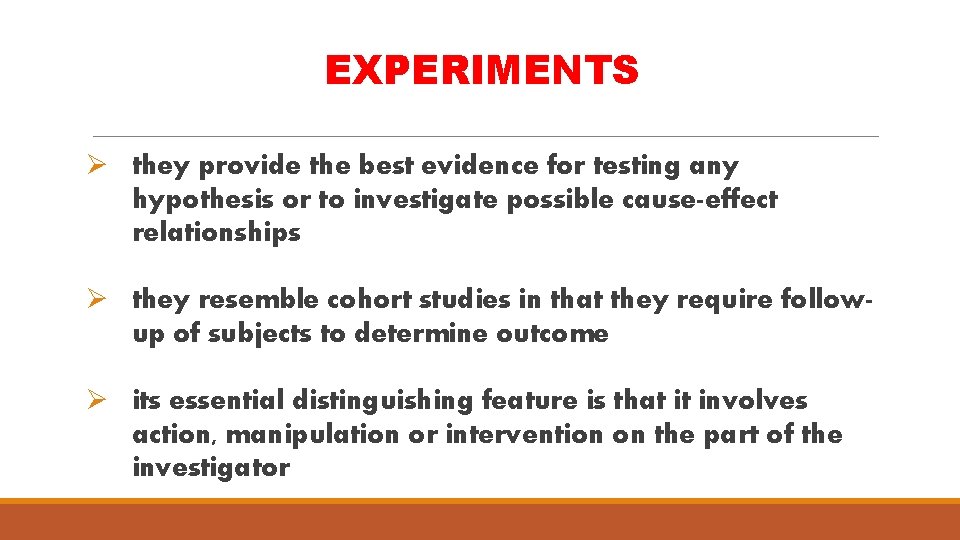
EXPERIMENTS Ø they provide the best evidence for testing any hypothesis or to investigate possible cause-effect relationships Ø they resemble cohort studies in that they require followup of subjects to determine outcome Ø its essential distinguishing feature is that it involves action, manipulation or intervention on the part of the investigator
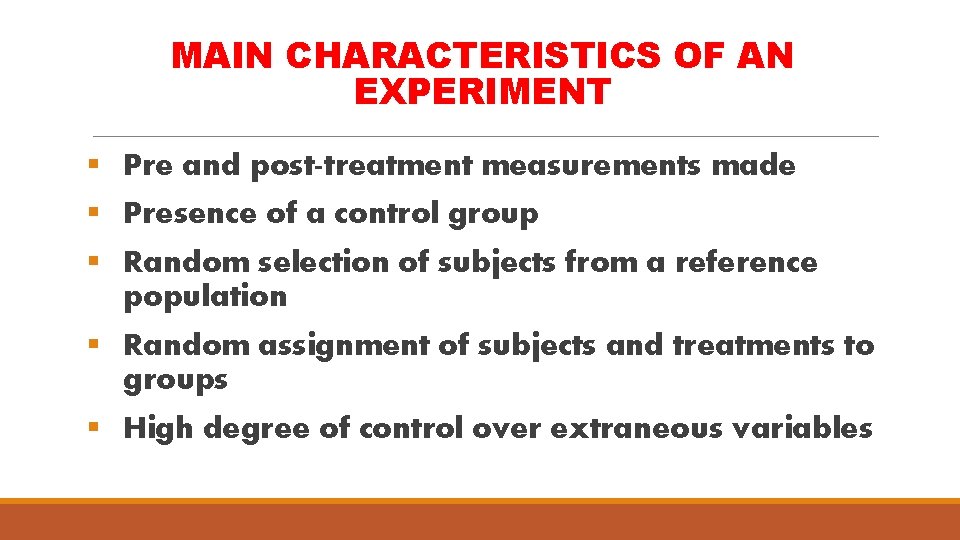
MAIN CHARACTERISTICS OF AN EXPERIMENT § Pre and post-treatment measurements made § Presence of a control group § Random selection of subjects from a reference population § Random assignment of subjects and treatments to groups § High degree of control over extraneous variables
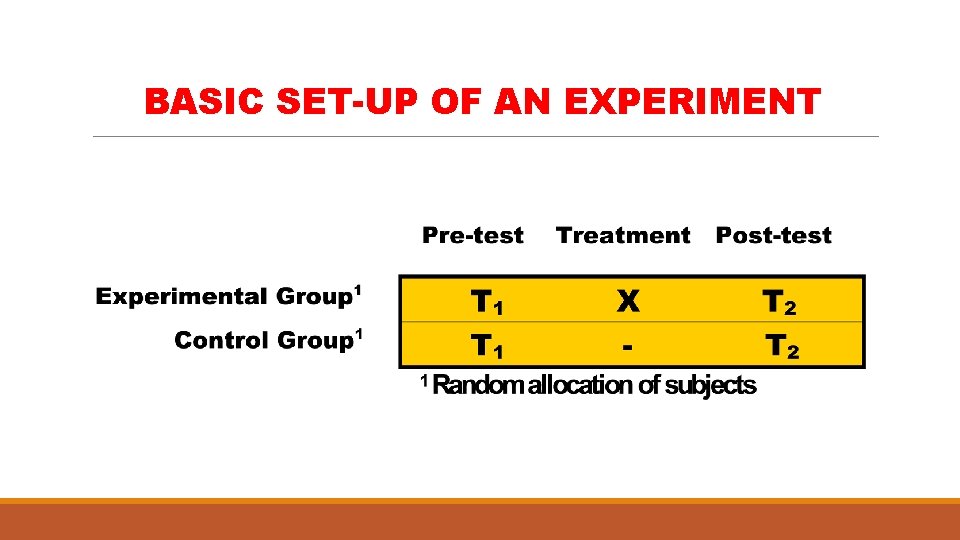
BASIC SET-UP OF AN EXPERIMENT
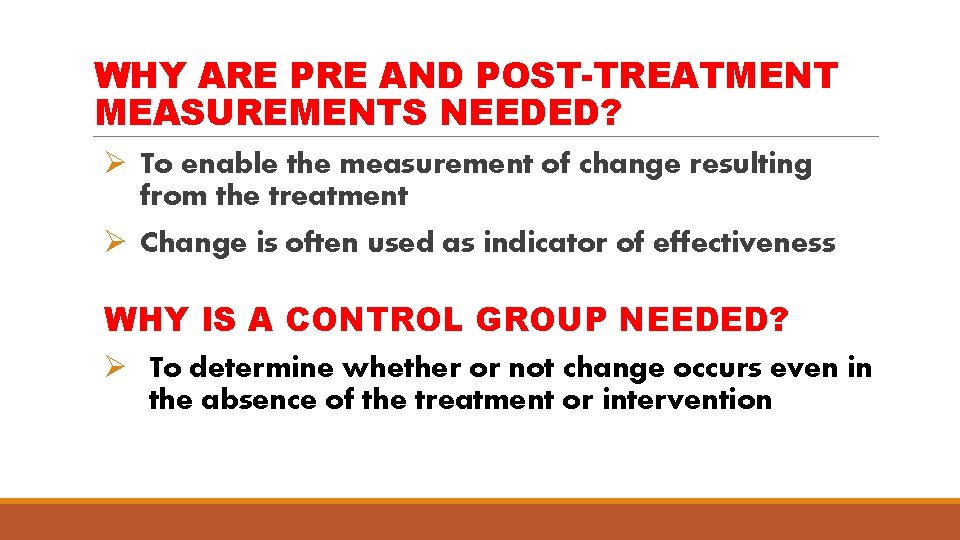
WHY ARE PRE AND POST-TREATMENT MEASUREMENTS NEEDED? Ø To enable the measurement of change resulting from the treatment Ø Change is often used as indicator of effectiveness WHY IS A CONTROL GROUP NEEDED? Ø To determine whether or not change occurs even in the absence of the treatment or intervention
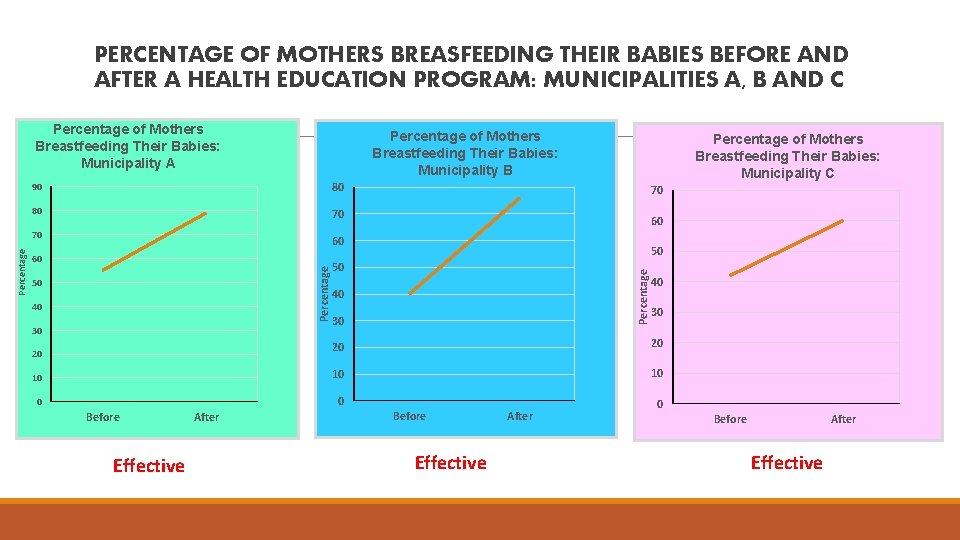
PERCENTAGE OF MOTHERS BREASFEEDING THEIR BABIES BEFORE AND AFTER A HEALTH EDUCATION PROGRAM: MUNICIPALITIES A, B AND C Percentage of Mothers Breastfeeding Their Babies: Municipality B 90 80 80 70 70 60 50 40 30 Percentage of Mothers Breastfeeding Their Babies: Municipality C 70 60 50 50 Percentage 60 Percentage of Mothers Breastfeeding Their Babies: Municipality A 40 30 20 20 10 10 10 0 0 20 Before Effective After 0 Before After Effective
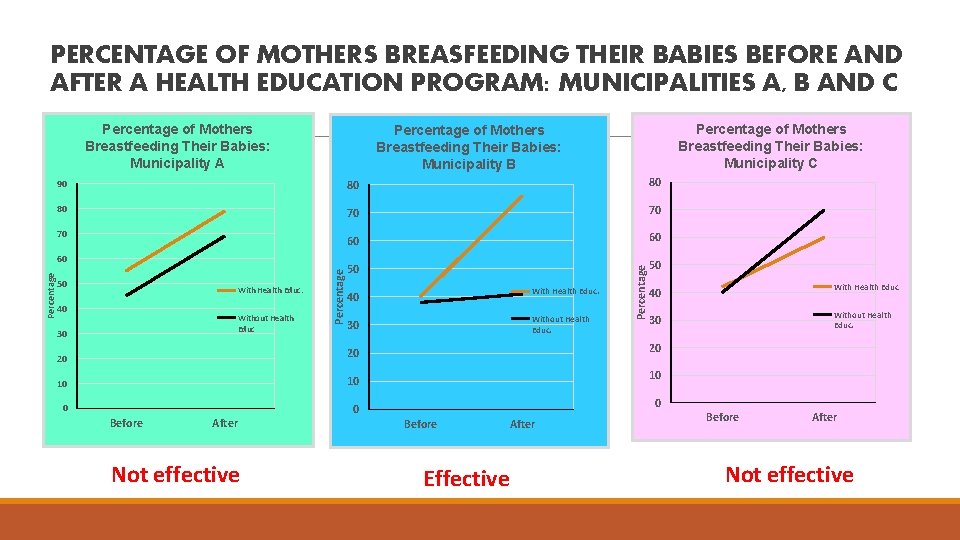
PERCENTAGE OF MOTHERS BREASFEEDING THEIR BABIES BEFORE AND AFTER A HEALTH EDUCATION PROGRAM: MUNICIPALITIES A, B AND C Percentage of Mothers Breastfeeding Their Babies: Municipality A 80 80 80 70 70 60 60 50 50 50 With Health Educ. 40 Without Health Educ 30 Percentage 60 40 With Health Educ. 30 Without Health Educ. Percentage 90 70 Percentage of Mothers Breastfeeding Their Babies: Municipality C Percentage of Mothers Breastfeeding Their Babies: Municipality B 40 With Health Educ 30 Without Health Educ. 20 20 20 10 10 10 0 0 Before After Not effective 0 Before After Effective Before After Not effective
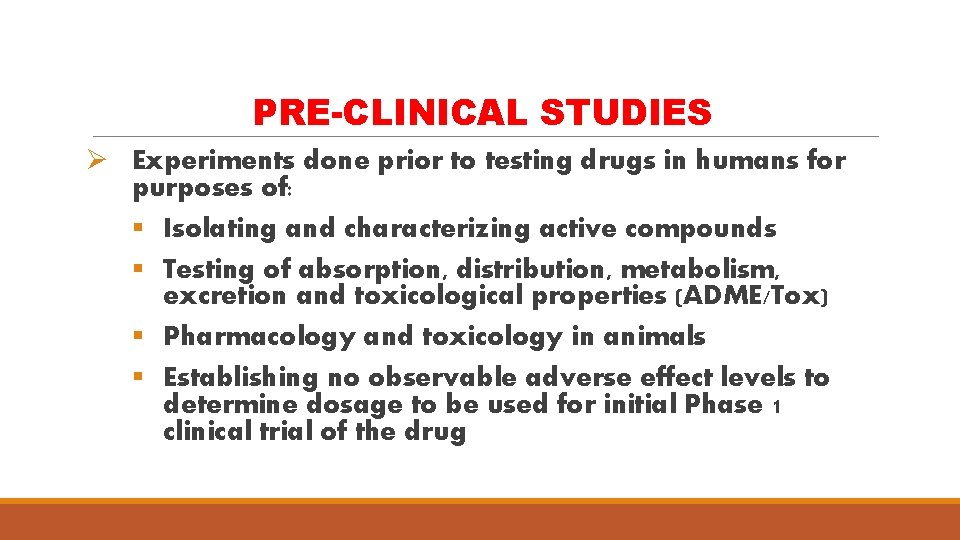
PRE-CLINICAL STUDIES Ø Experiments done prior to testing drugs in humans for purposes of: § Isolating and characterizing active compounds § Testing of absorption, distribution, metabolism, excretion and toxicological properties (ADME/Tox) § Pharmacology and toxicology in animals § Establishing no observable adverse effect levels to determine dosage to be used for initial Phase 1 clinical trial of the drug
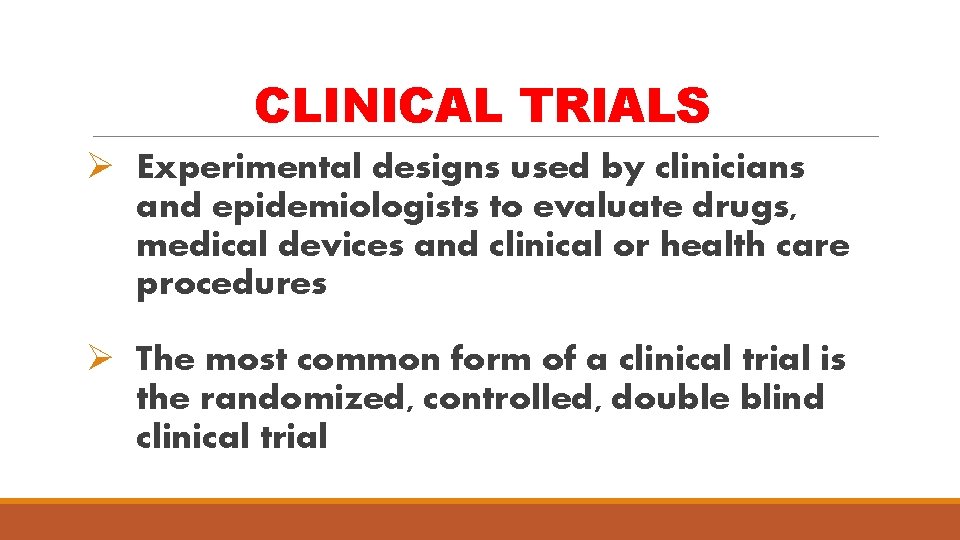
CLINICAL TRIALS Ø Experimental designs used by clinicians and epidemiologists to evaluate drugs, medical devices and clinical or health care procedures Ø The most common form of a clinical trial is the randomized, controlled, double blind clinical trial
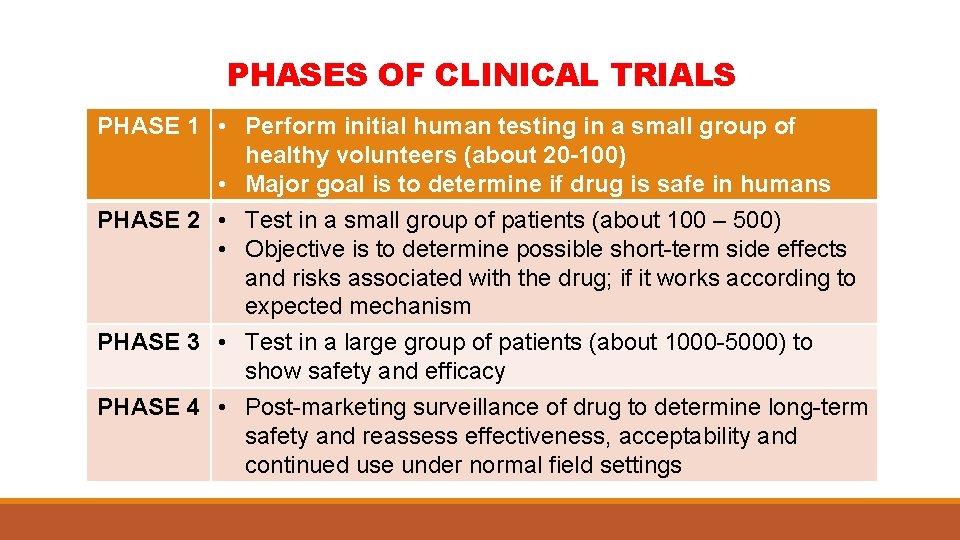
PHASES OF CLINICAL TRIALS PHASE 1 • Perform initial human testing in a small group of healthy volunteers (about 20 -100) • Major goal is to determine if drug is safe in humans PHASE 2 • Test in a small group of patients (about 100 – 500) • Objective is to determine possible short-term side effects and risks associated with the drug; if it works according to expected mechanism PHASE 3 • Test in a large group of patients (about 1000 -5000) to show safety and efficacy PHASE 4 • Post-marketing surveillance of drug to determine long-term safety and reassess effectiveness, acceptability and continued use under normal field settings
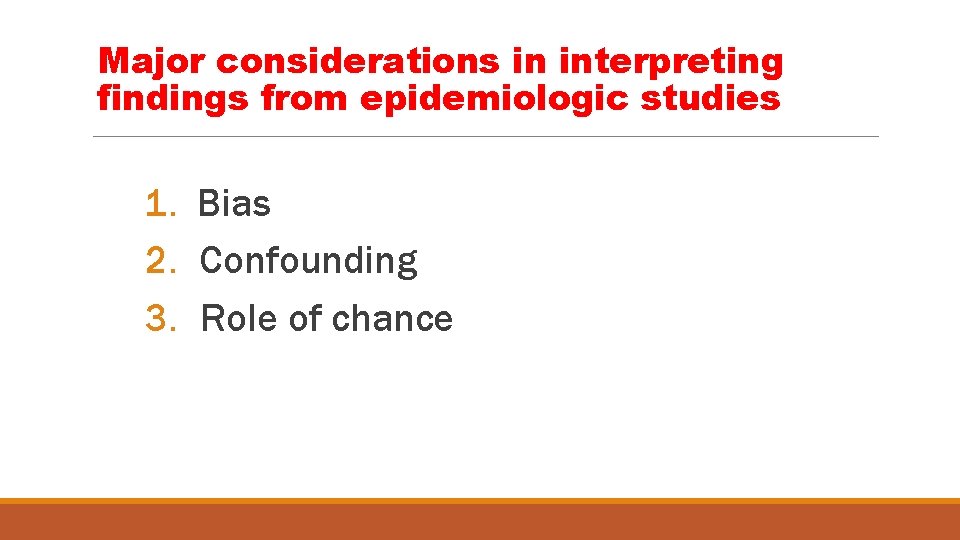
Major considerations in interpreting findings from epidemiologic studies 1. Bias 2. Confounding 3. Role of chance
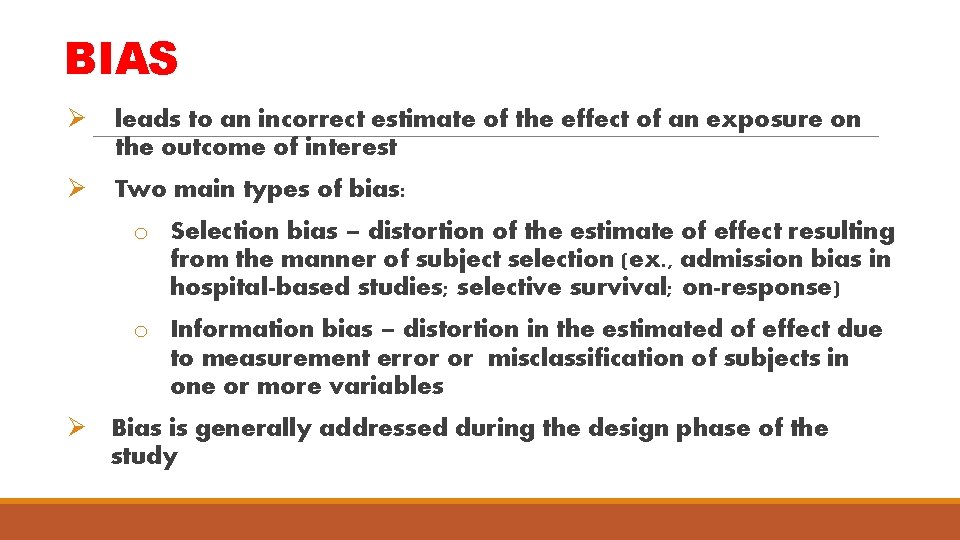
BIAS Ø leads to an incorrect estimate of the effect of an exposure on the outcome of interest Ø Two main types of bias: o Selection bias – distortion of the estimate of effect resulting from the manner of subject selection (ex. , admission bias in hospital-based studies; selective survival; on-response) o Information bias – distortion in the estimated of effect due to measurement error or misclassification of subjects in one or more variables Ø Bias is generally addressed during the design phase of the study
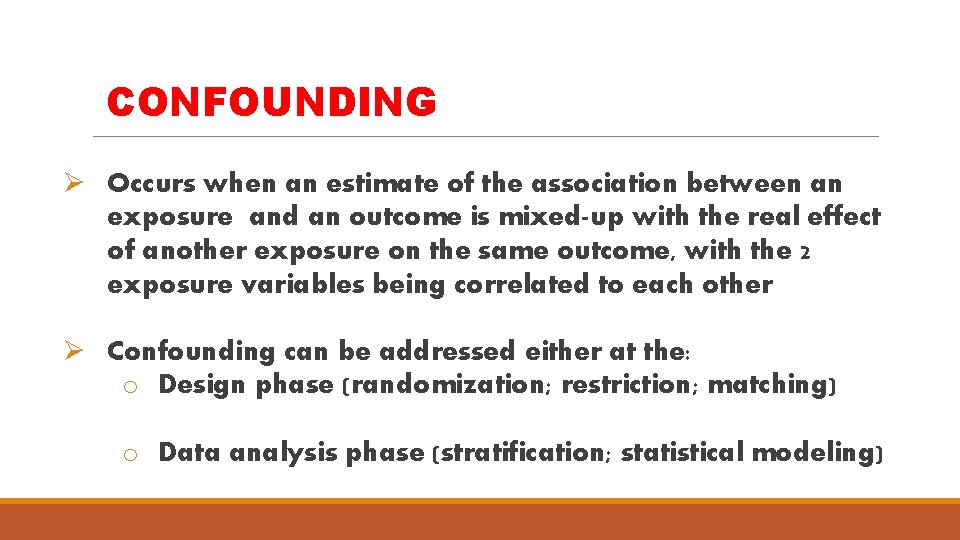
CONFOUNDING Ø Occurs when an estimate of the association between an exposure and an outcome is mixed-up with the real effect of another exposure on the same outcome, with the 2 exposure variables being correlated to each other Ø Confounding can be addressed either at the: o Design phase (randomization; restriction; matching) o Data analysis phase (stratification; statistical modeling)
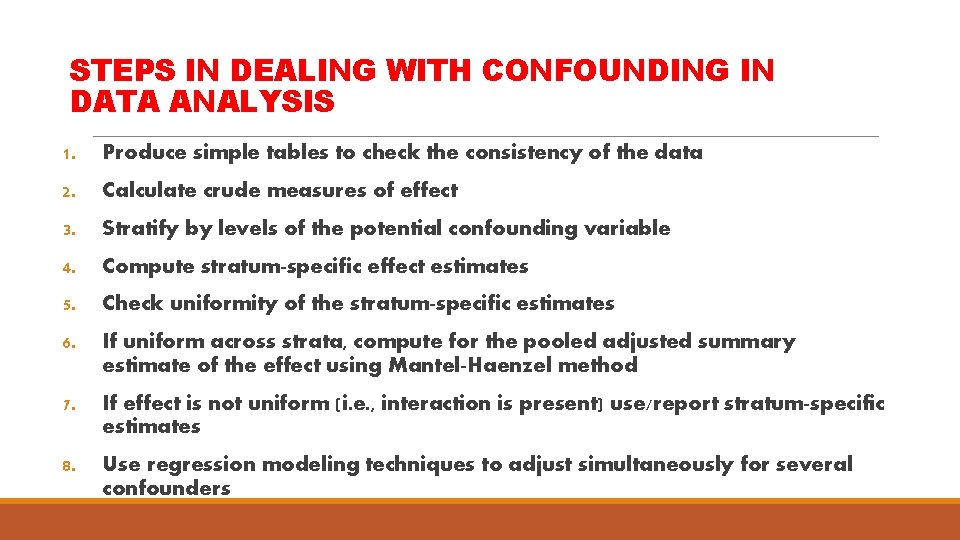
STEPS IN DEALING WITH CONFOUNDING IN DATA ANALYSIS 1. Produce simple tables to check the consistency of the data 2. Calculate crude measures of effect 3. Stratify by levels of the potential confounding variable 4. Compute stratum-specific effect estimates 5. Check uniformity of the stratum-specific estimates 6. If uniform across strata, compute for the pooled adjusted summary estimate of the effect using Mantel-Haenzel method 7. If effect is not uniform (i. e. , interaction is present) use/report stratum-specific estimates 8. Use regression modeling techniques to adjust simultaneously for several confounders
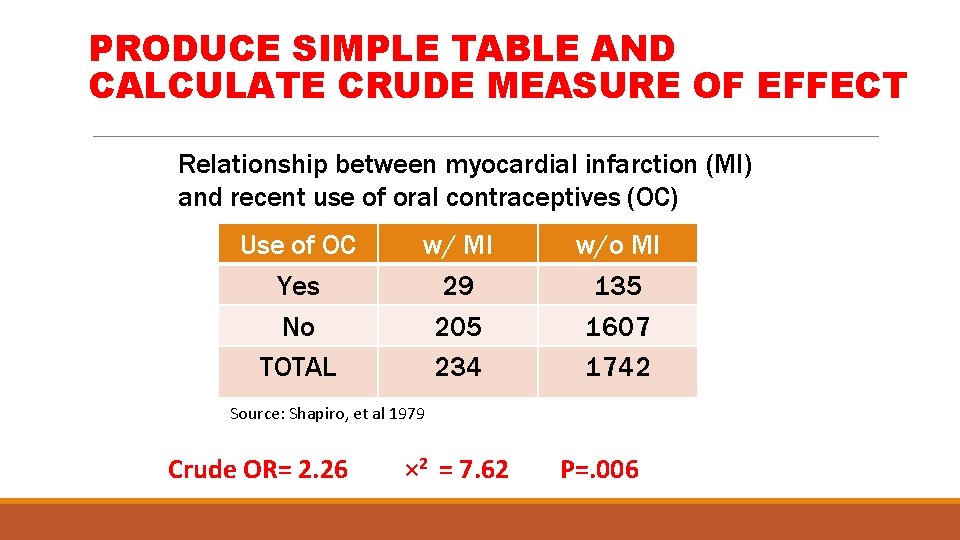
PRODUCE SIMPLE TABLE AND CALCULATE CRUDE MEASURE OF EFFECT Relationship between myocardial infarction (MI) and recent use of oral contraceptives (OC) Use of OC Yes No TOTAL w/ MI 29 205 234 w/o MI 135 1607 1742 Source: Shapiro, et al 1979 Crude OR= 2. 26 × 2 = 7. 62 P=. 006
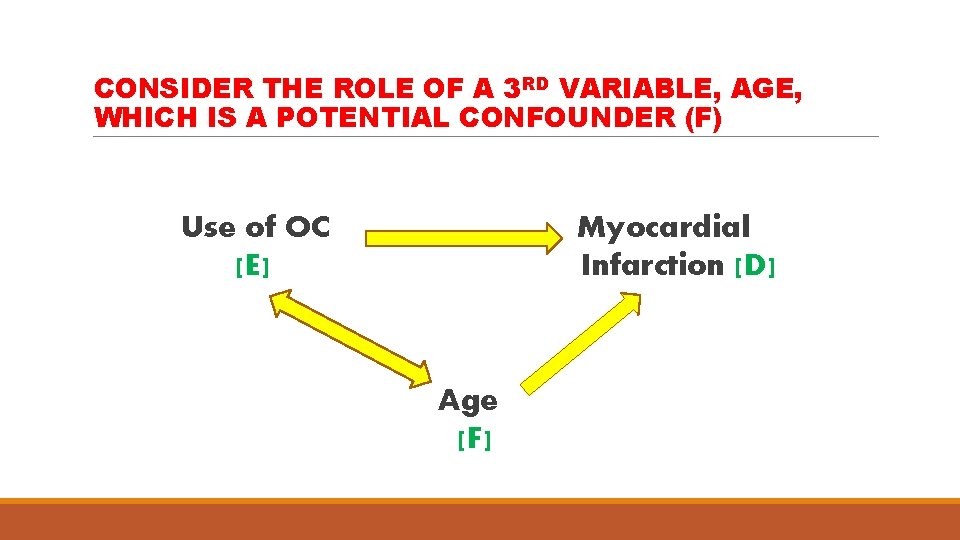
CONSIDER THE ROLE OF A 3 RD VARIABLE, AGE, WHICH IS A POTENTIAL CONFOUNDER (F) Use of OC [E] Myocardial Infarction [D] Age [F]
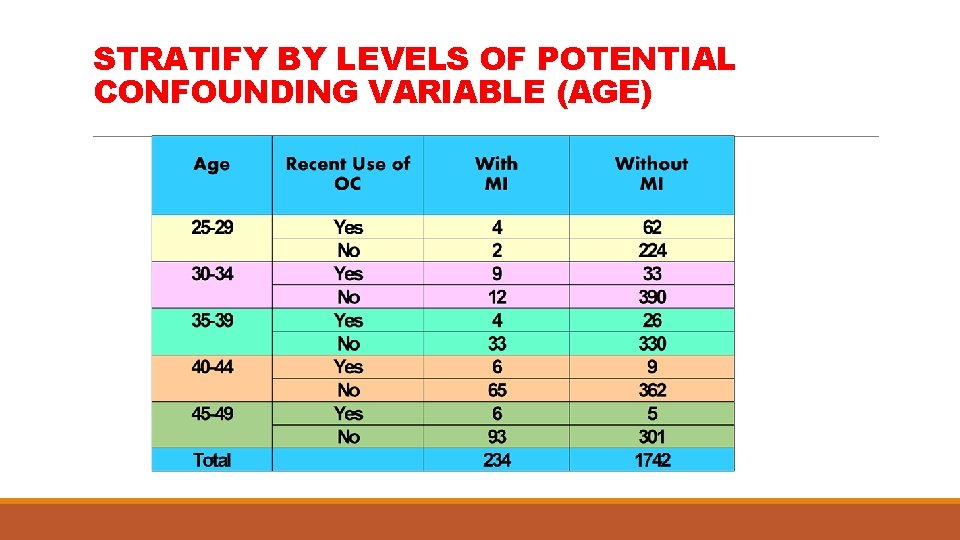
STRATIFY BY LEVELS OF POTENTIAL CONFOUNDING VARIABLE (AGE)
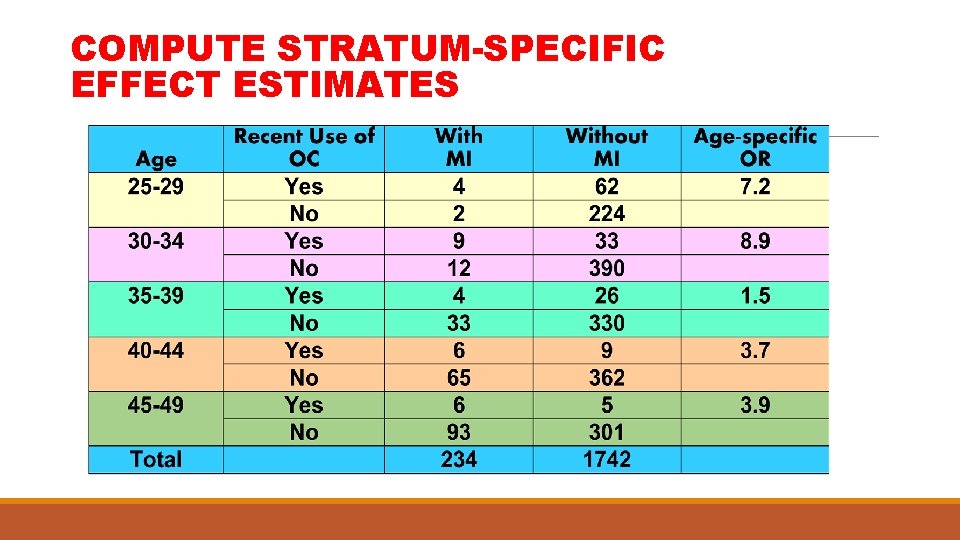
COMPUTE STRATUM-SPECIFIC EFFECT ESTIMATES
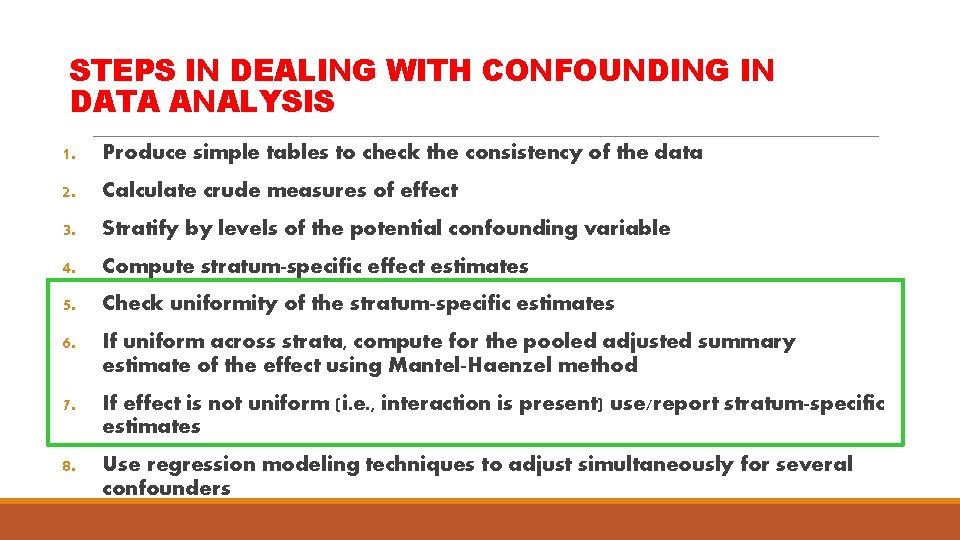
STEPS IN DEALING WITH CONFOUNDING IN DATA ANALYSIS 1. Produce simple tables to check the consistency of the data 2. Calculate crude measures of effect 3. Stratify by levels of the potential confounding variable 4. Compute stratum-specific effect estimates 5. Check uniformity of the stratum-specific estimates 6. If uniform across strata, compute for the pooled adjusted summary estimate of the effect using Mantel-Haenzel method 7. If effect is not uniform (i. e. , interaction is present) use/report stratum-specific estimates 8. Use regression modeling techniques to adjust simultaneously for several confounders
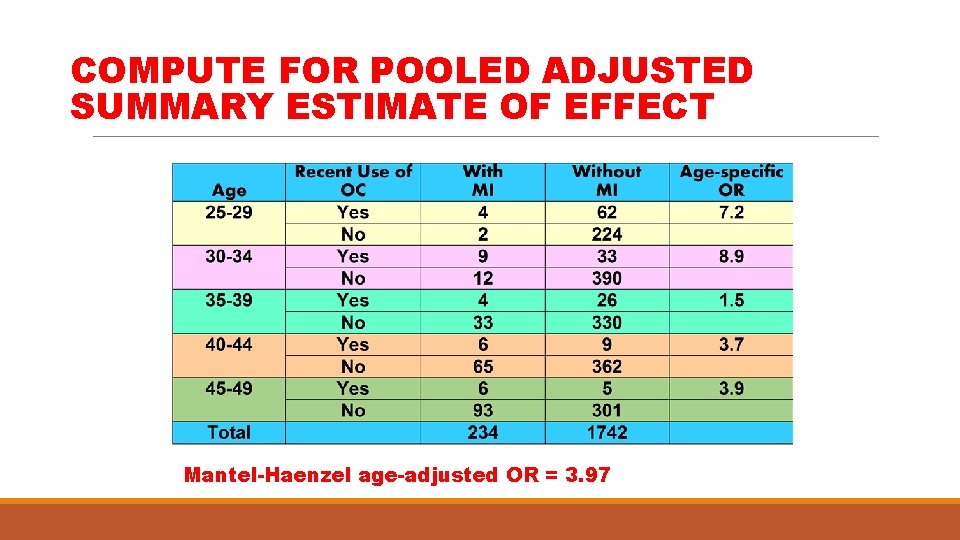
COMPUTE FOR POOLED ADJUSTED SUMMARY ESTIMATE OF EFFECT Mantel-Haenzel age-adjusted OR = 3. 97
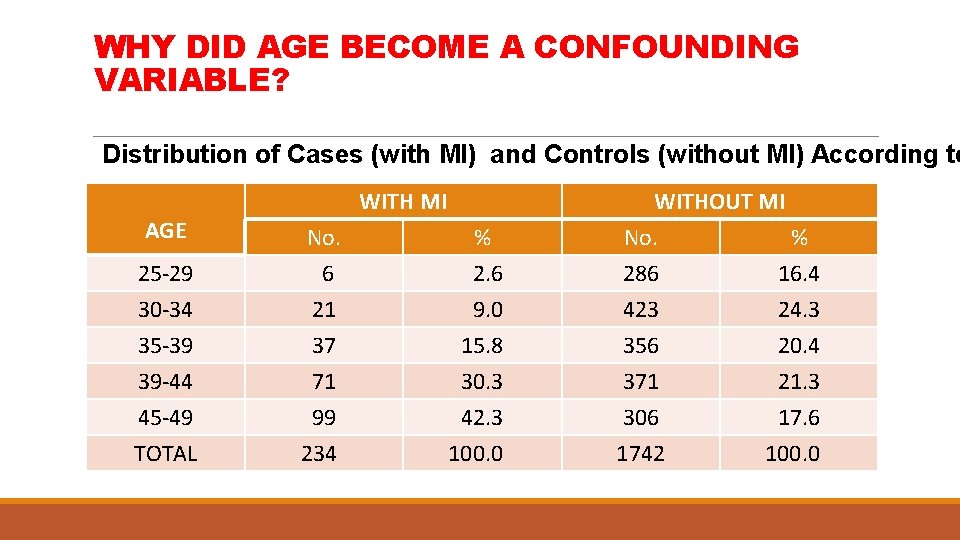
WHY DID AGE BECOME A CONFOUNDING VARIABLE? Distribution of Cases (with MI) and Controls (without MI) According to AGE WITH MI 25 -29 30 -34 No. 6 21 % 2. 6 9. 0 35 -39 39 -44 45 -49 TOTAL 37 71 99 234 15. 8 30. 3 42. 3 100. 0 WITHOUT MI No. % 286 16. 4 423 24. 3 356 371 306 1742 20. 4 21. 3 17. 6 100. 0
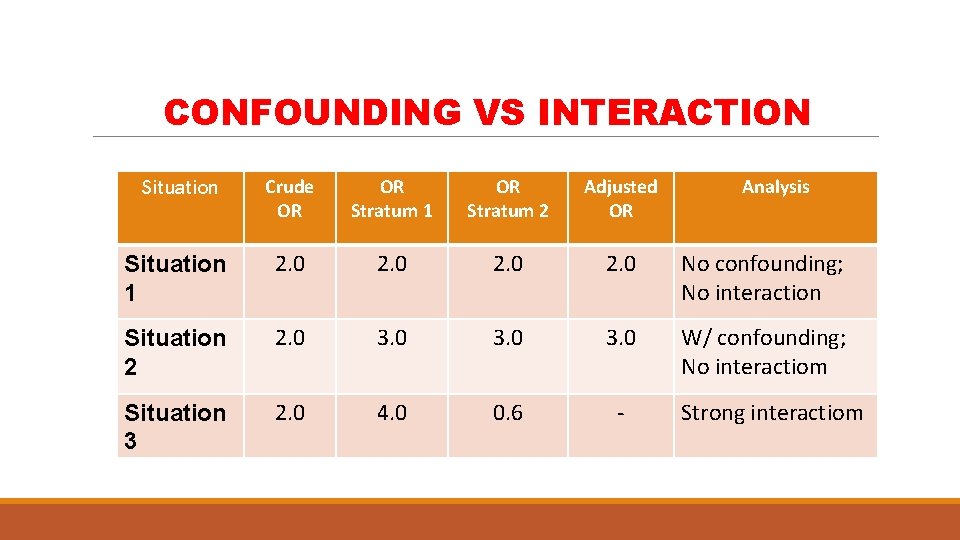
CONFOUNDING VS INTERACTION Situation Crude OR OR Stratum 1 OR Stratum 2 Adjusted OR Analysis Situation 1 2. 0 No confounding; No interaction Situation 2 2. 0 3. 0 W/ confounding; No interactiom Situation 3 2. 0 4. 0 0. 6 - Strong interactiom
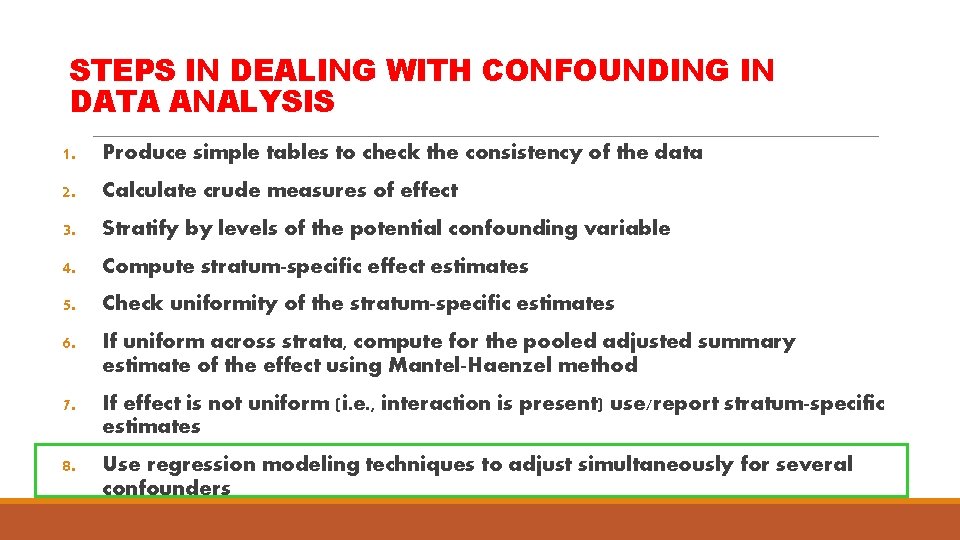
STEPS IN DEALING WITH CONFOUNDING IN DATA ANALYSIS 1. Produce simple tables to check the consistency of the data 2. Calculate crude measures of effect 3. Stratify by levels of the potential confounding variable 4. Compute stratum-specific effect estimates 5. Check uniformity of the stratum-specific estimates 6. If uniform across strata, compute for the pooled adjusted summary estimate of the effect using Mantel-Haenzel method 7. If effect is not uniform (i. e. , interaction is present) use/report stratum-specific estimates 8. Use regression modeling techniques to adjust simultaneously for several confounders
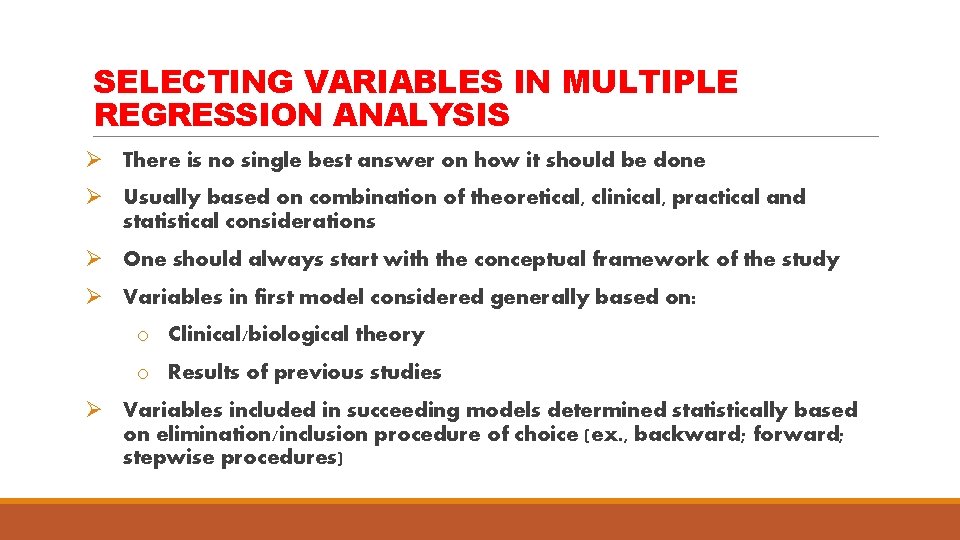
SELECTING VARIABLES IN MULTIPLE REGRESSION ANALYSIS Ø There is no single best answer on how it should be done Ø Usually based on combination of theoretical, clinical, practical and statistical considerations Ø One should always start with the conceptual framework of the study Ø Variables in first model considered generally based on: o Clinical/biological theory o Results of previous studies Ø Variables included in succeeding models determined statistically based on elimination/inclusion procedure of choice (ex. , backward; forward; stepwise procedures)
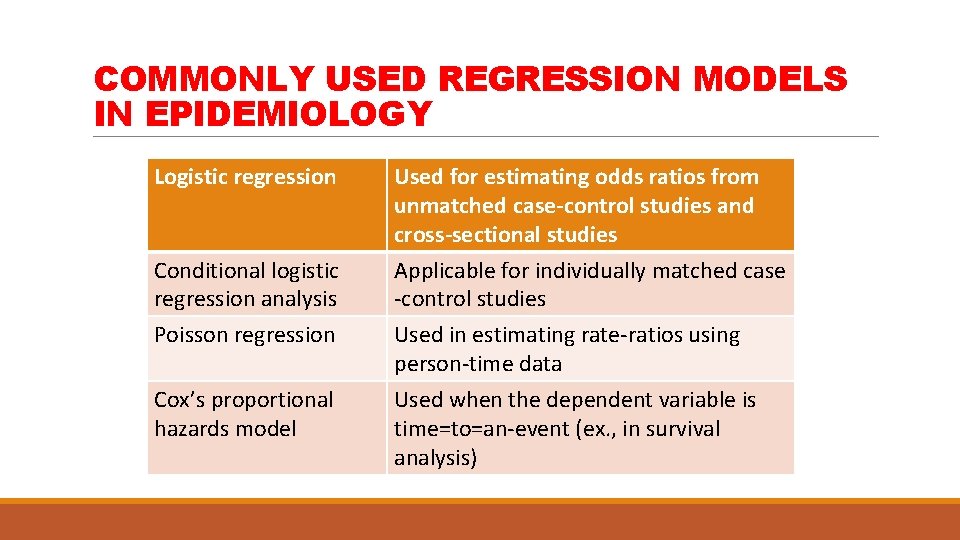
COMMONLY USED REGRESSION MODELS IN EPIDEMIOLOGY Logistic regression Conditional logistic regression analysis Poisson regression Cox’s proportional hazards model Used for estimating odds ratios from unmatched case-control studies and cross-sectional studies Applicable for individually matched case -control studies Used in estimating rate-ratios using person-time data Used when the dependent variable is time=to=an-event (ex. , in survival analysis)
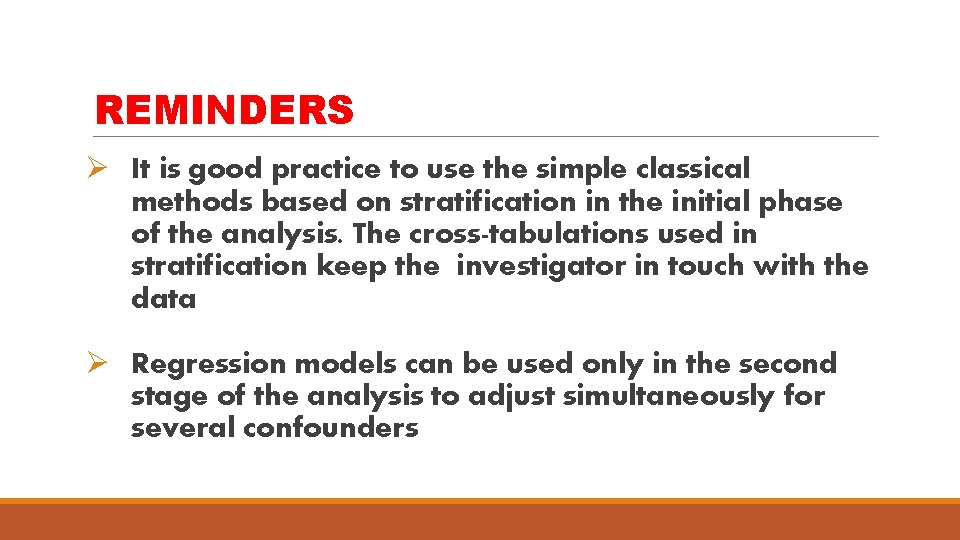
REMINDERS Ø It is good practice to use the simple classical methods based on stratification in the initial phase of the analysis. The cross-tabulations used in stratification keep the investigator in touch with the data Ø Regression models can be used only in the second stage of the analysis to adjust simultaneously for several confounders
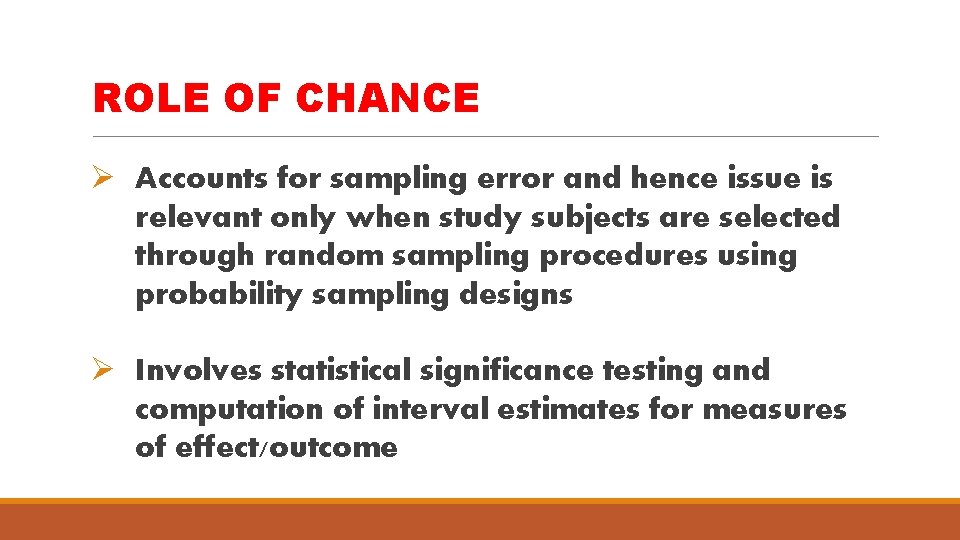
ROLE OF CHANCE Ø Accounts for sampling error and hence issue is relevant only when study subjects are selected through random sampling procedures using probability sampling designs Ø Involves statistical significance testing and computation of interval estimates for measures of effect/outcome
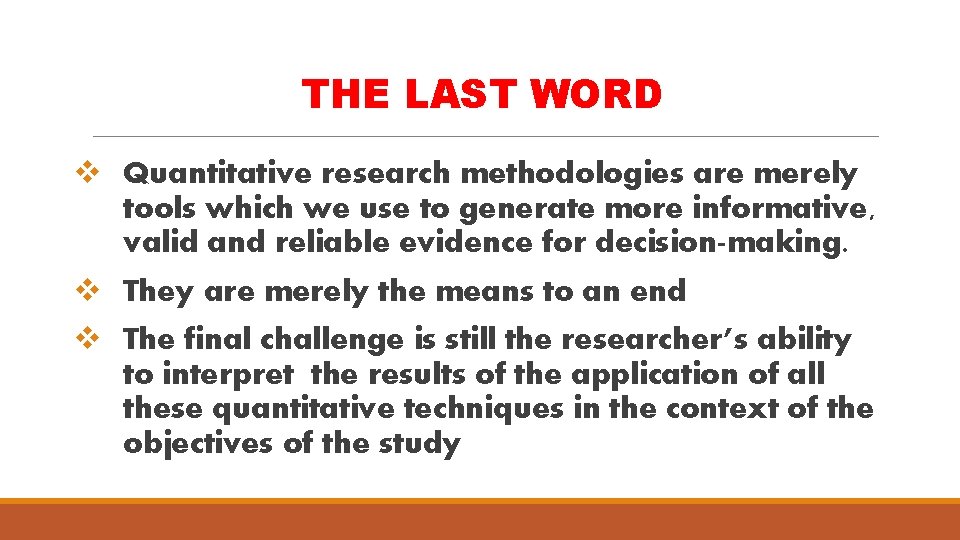
THE LAST WORD v Quantitative research methodologies are merely tools which we use to generate more informative, valid and reliable evidence for decision-making. v They are merely the means to an end v The final challenge is still the researcher’s ability to interpret the results of the application of all these quantitative techniques in the context of the objectives of the study
Up sampler and down sampler are
Up sampler and down sampler are
Ophelia mendoza
Types of empirical research
System design methodologies
Vlsi basics
Wikipedia agile project management
Define the relationship chapter 16
People oriented methodologies
What is domain
What is indigenous research
Business performance management methodologies
Methodologies for cross-domain data fusion: an overview
Census at school random sampler
Personal dust sampler
Surface exploration
Types of water sampler
Iread sampler
Siliceous ooze
Alat elektronika analog
Ny nextera question sampler
Glsl sampler
Clamshell sampler definition
Gibbs sampler
Pelican grain sampler
Thermal precipitator air sampler
Spt energy calibration
Skc impinger
Chromatic sampler
Nys question sampler
Ophelia astronomia
Ophelia's flowers worksheet
Foils of hamlet
Ophelia tells her father in scene i that hamlet is behaving
Summary of act 2 of hamlet
Summary of hamlet act 1 scene 3
Ophelia gregory crewdson
Shakespearean prince of denmark
Hamlet characters
Ophelia monologue flowers
Ophelia's daughter
Arthur hughes ophelia 1852
Who is ophelia's brother in hamlet
Prince hamlet
Holistic quantitative or qualitative
Data gathering for quantitative research
Sampling methods in qualitative and quantitative research
Example of appendices in research paper