Quantifying Parameter Interaction in a Generalized Sensitivity Analysis
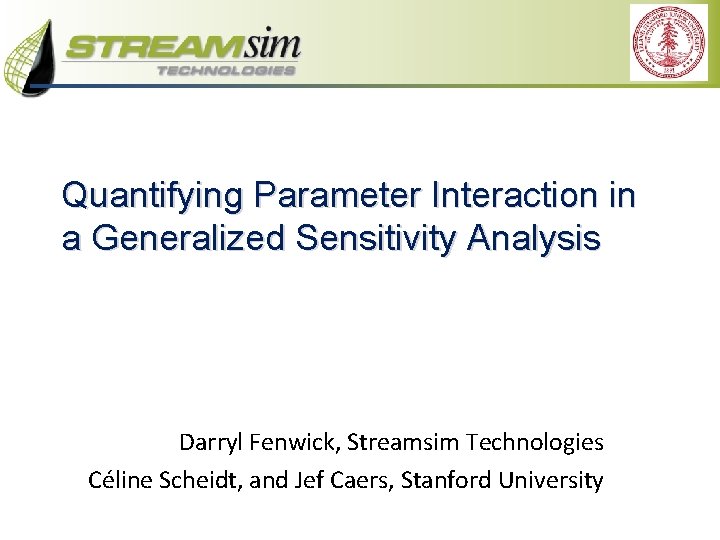
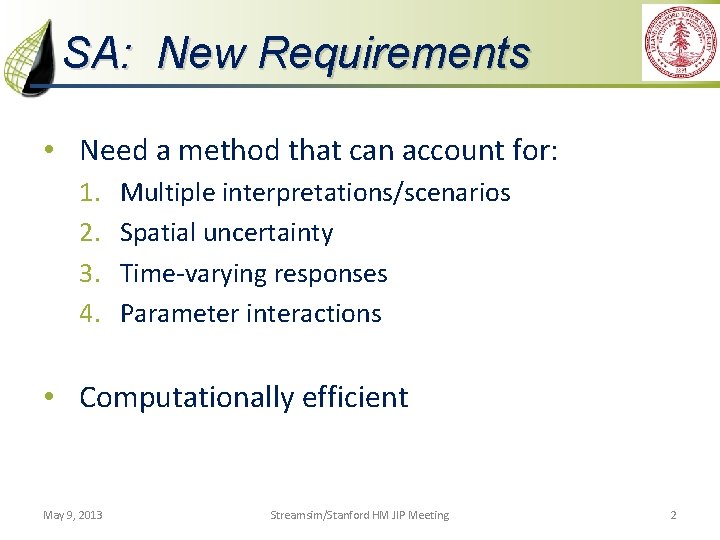
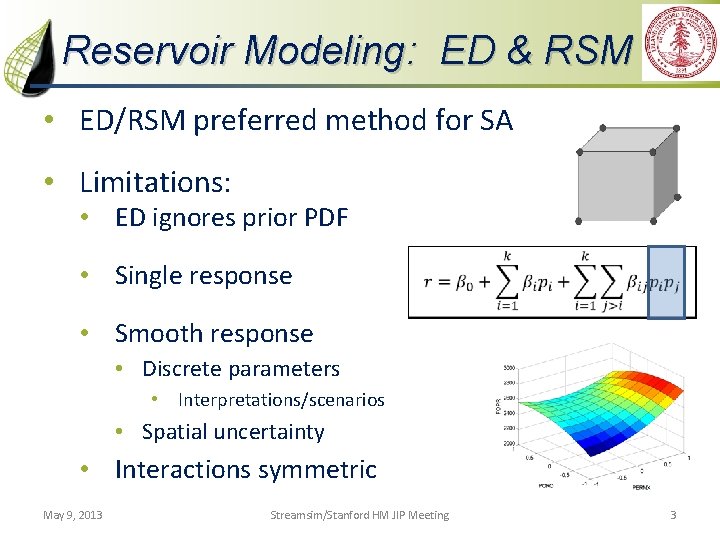
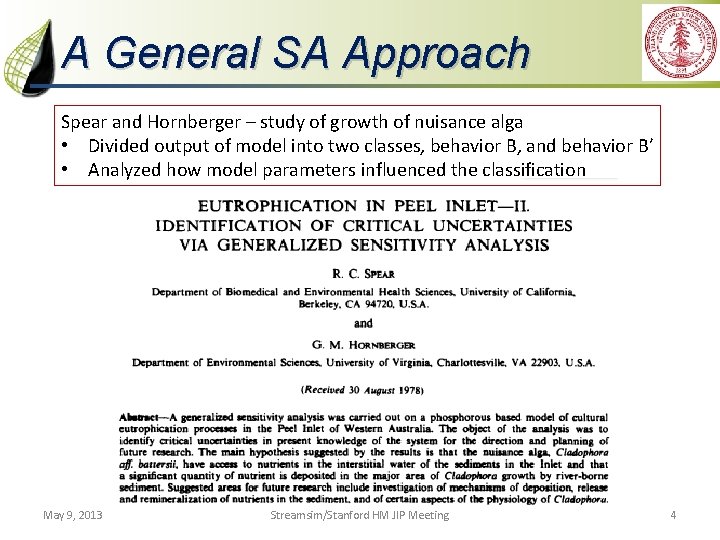
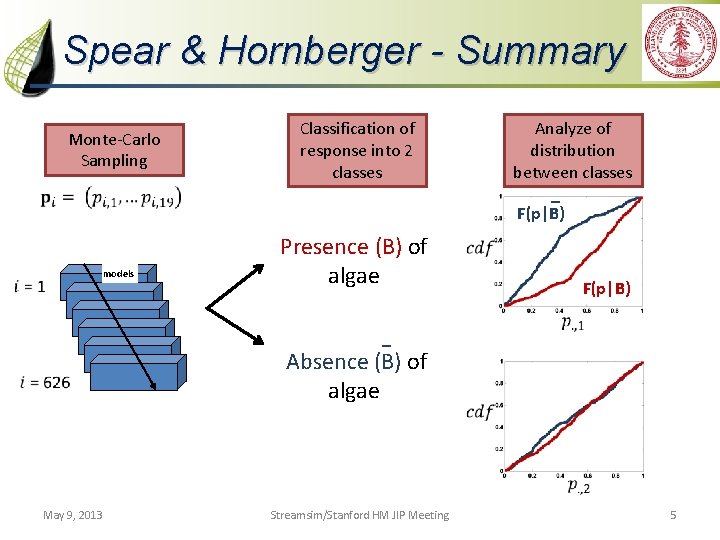
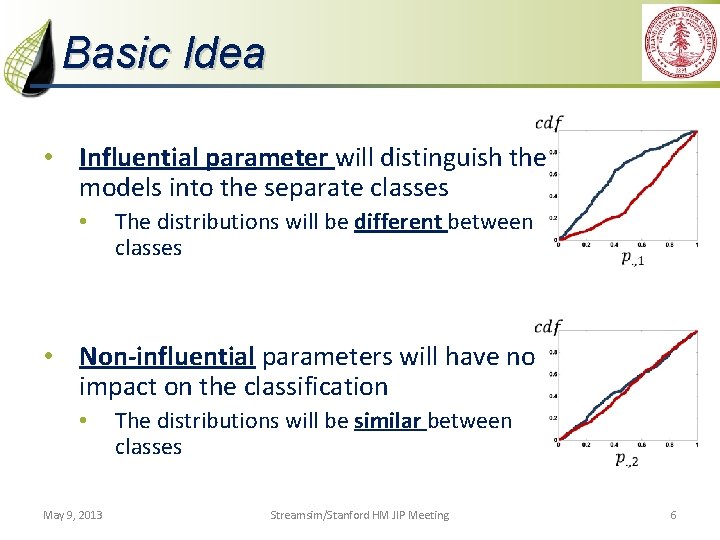
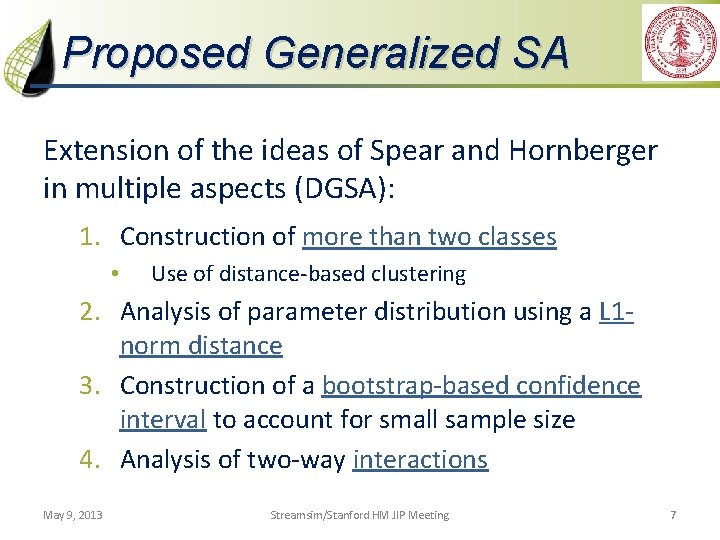
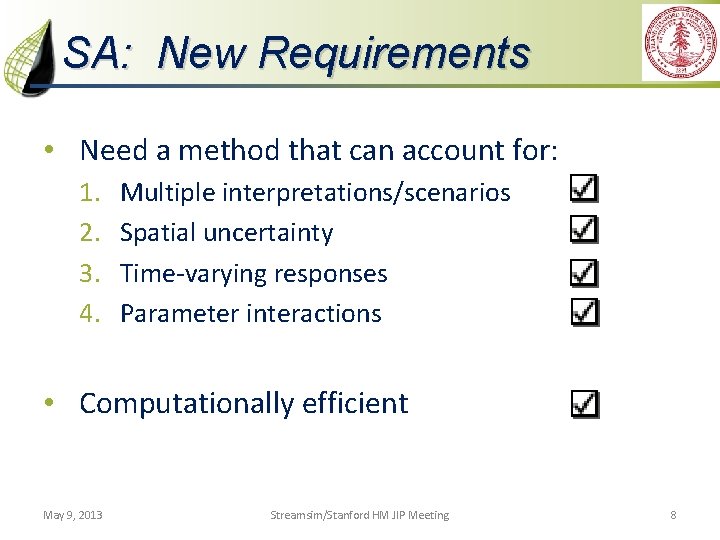
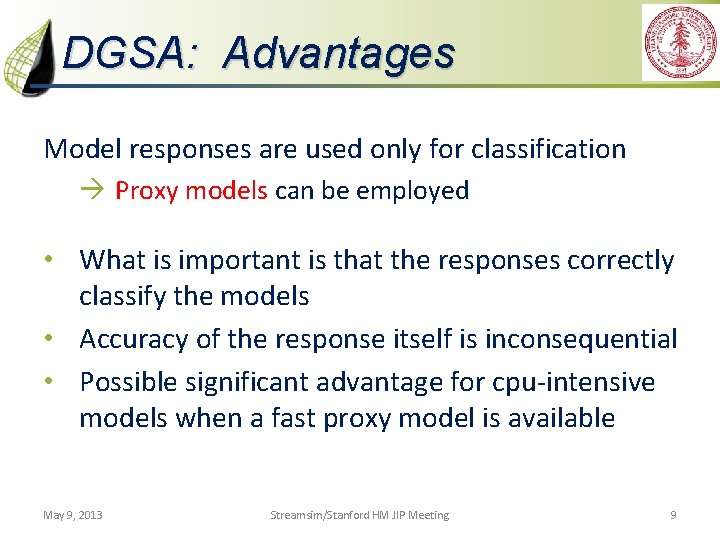
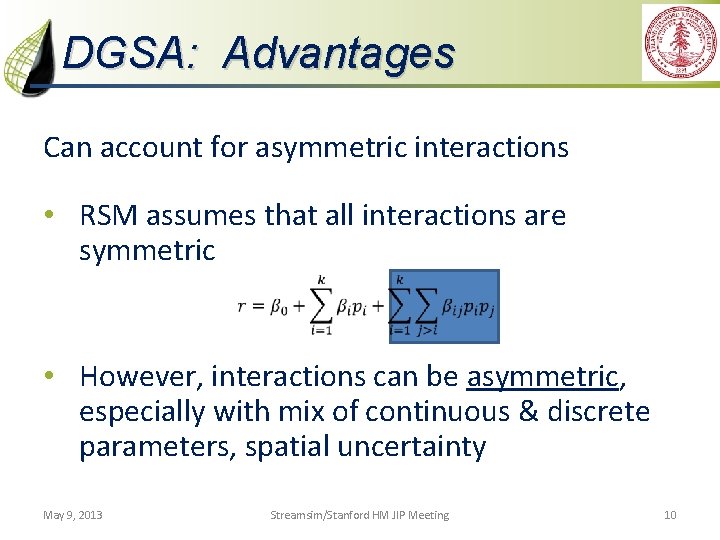
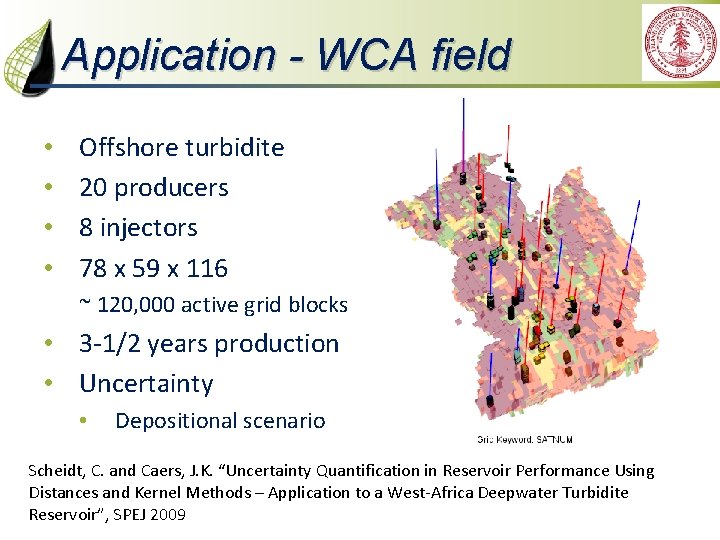
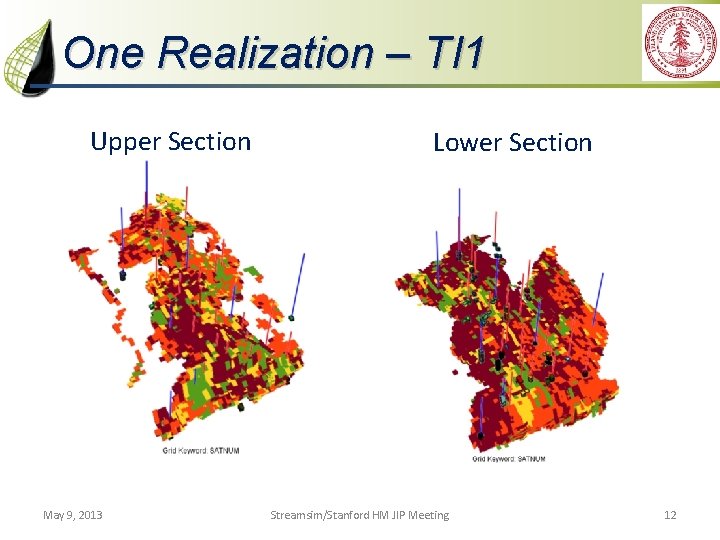
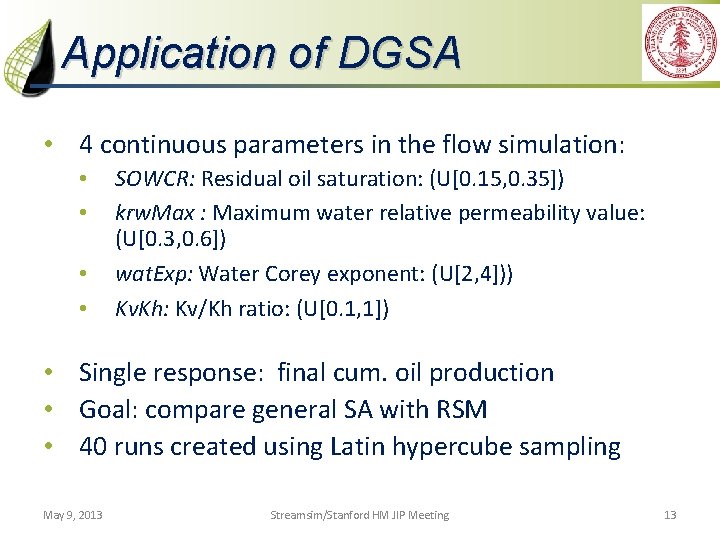
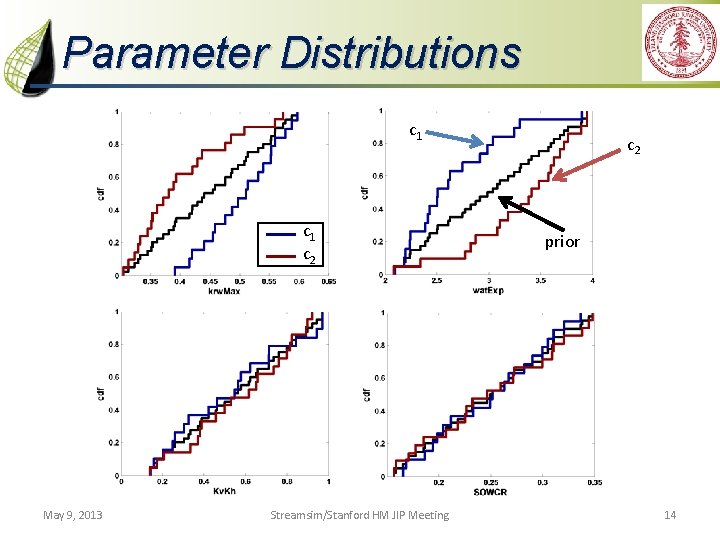
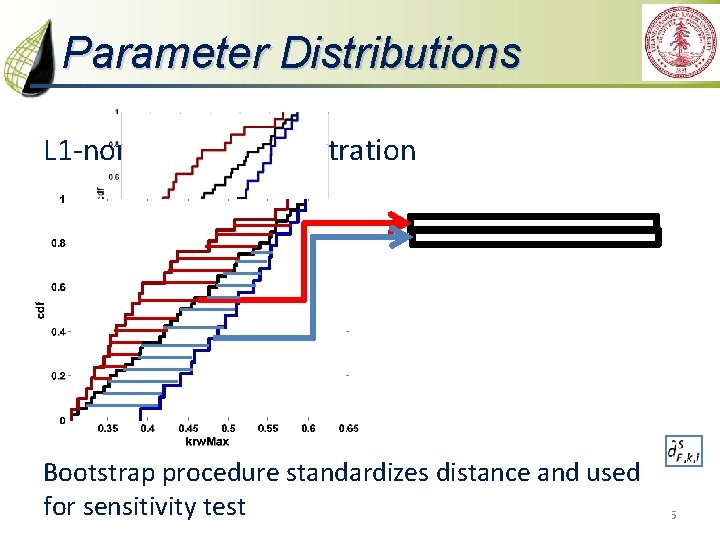
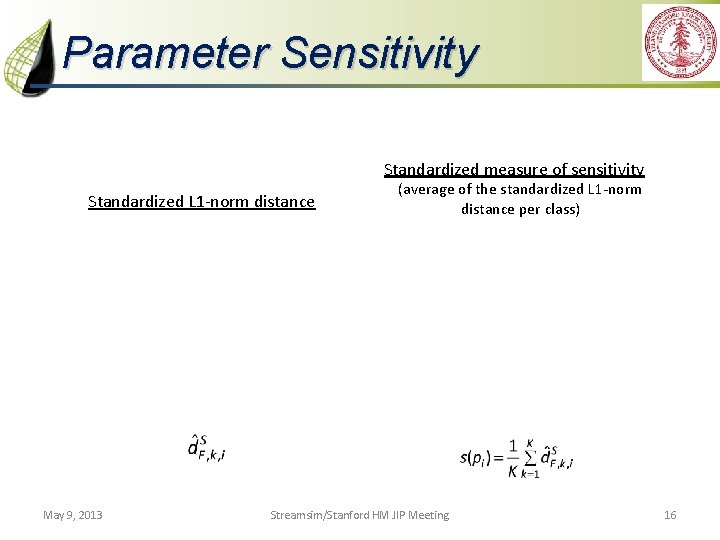
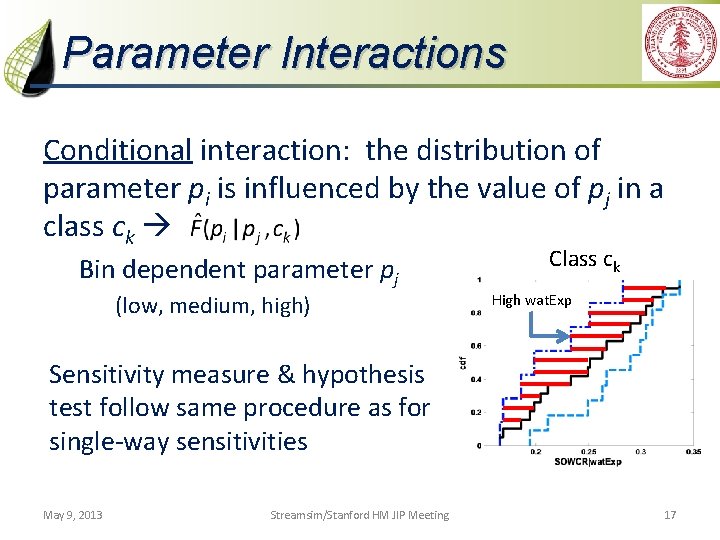
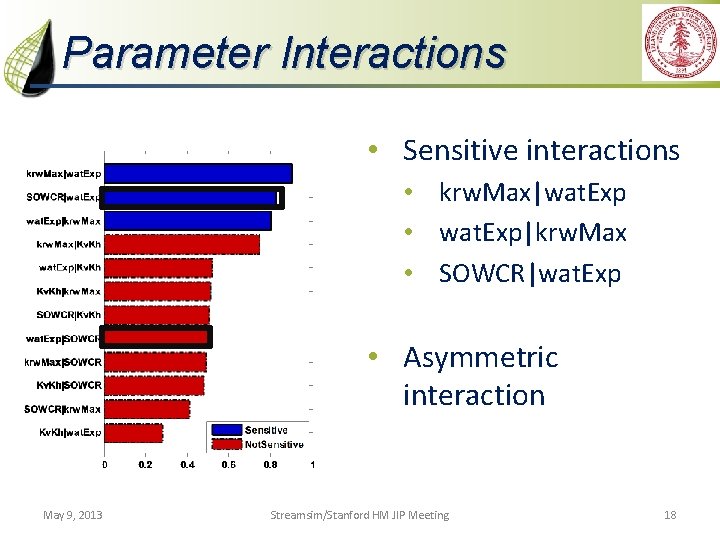
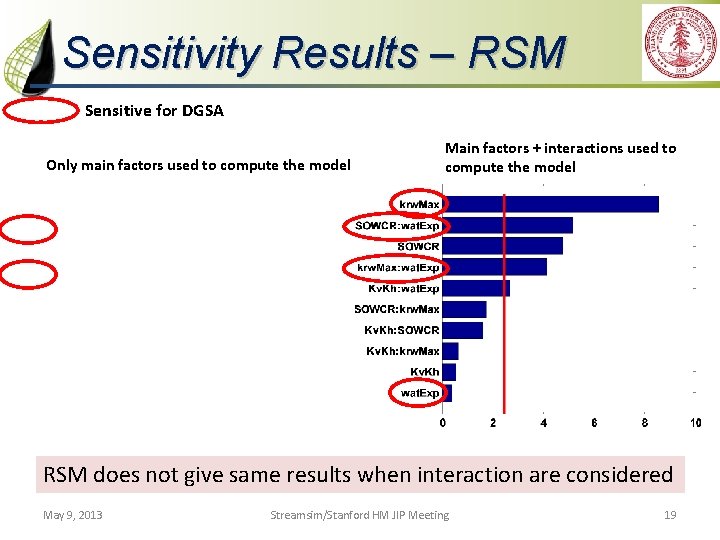
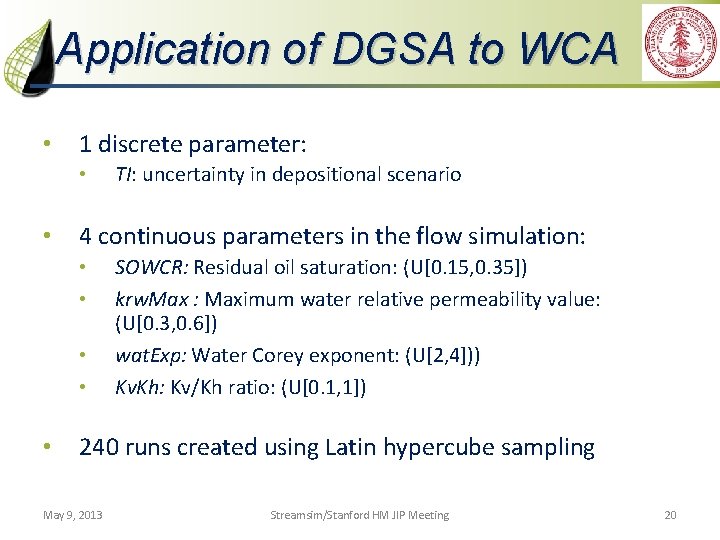
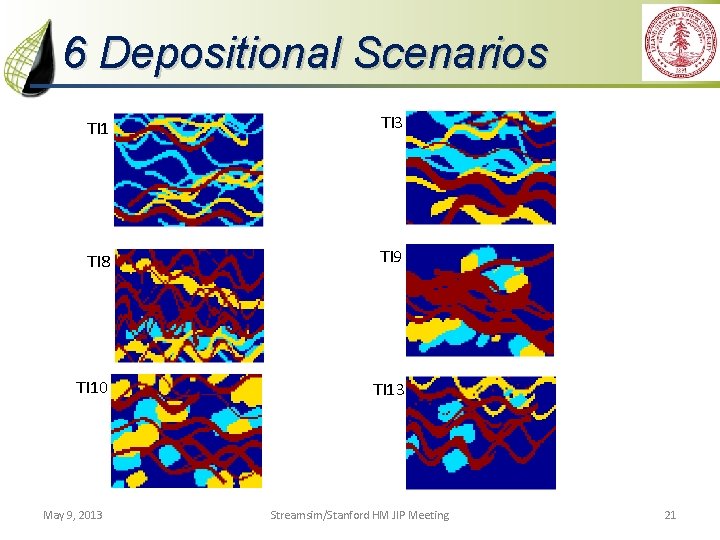
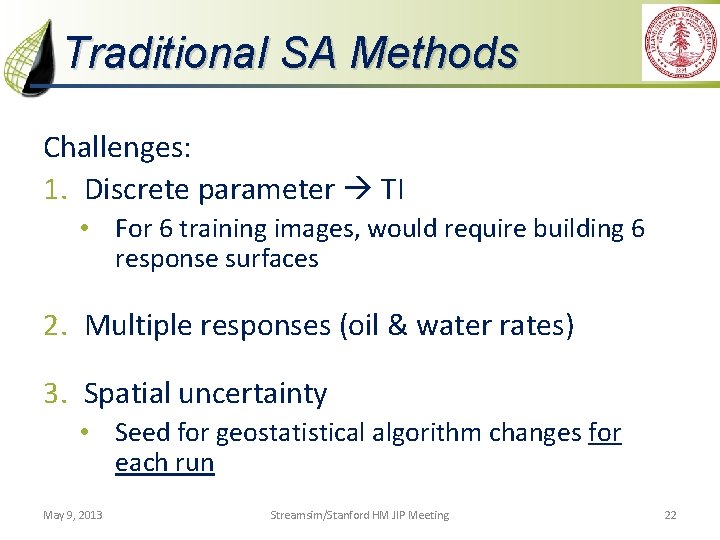
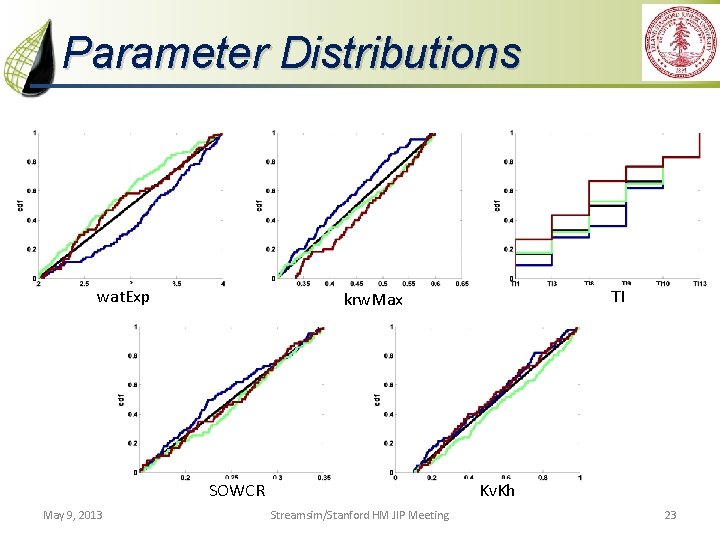
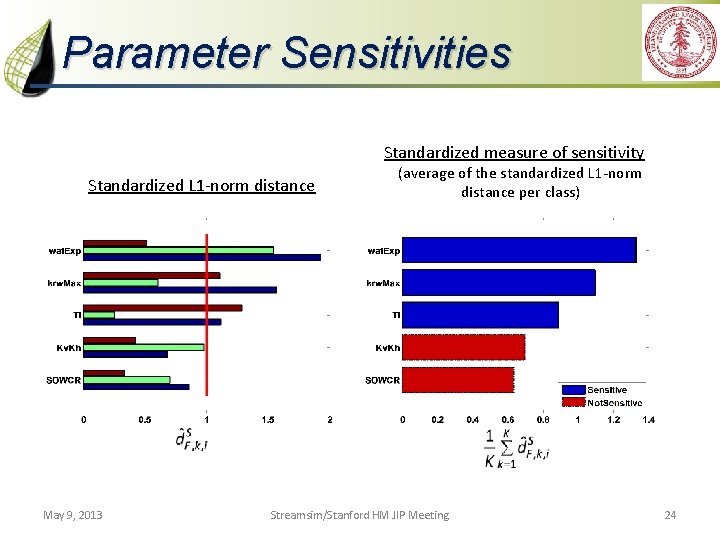
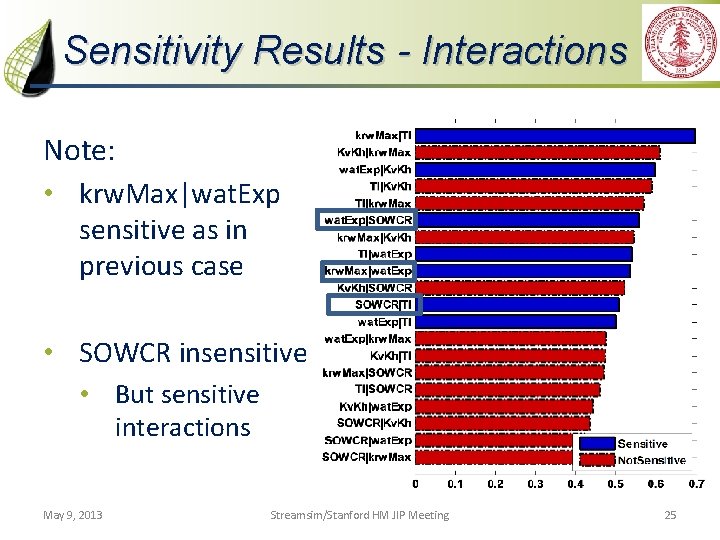
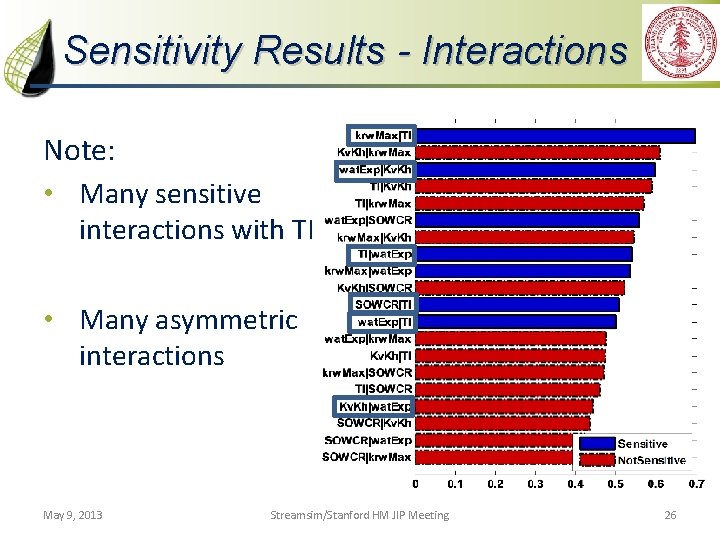
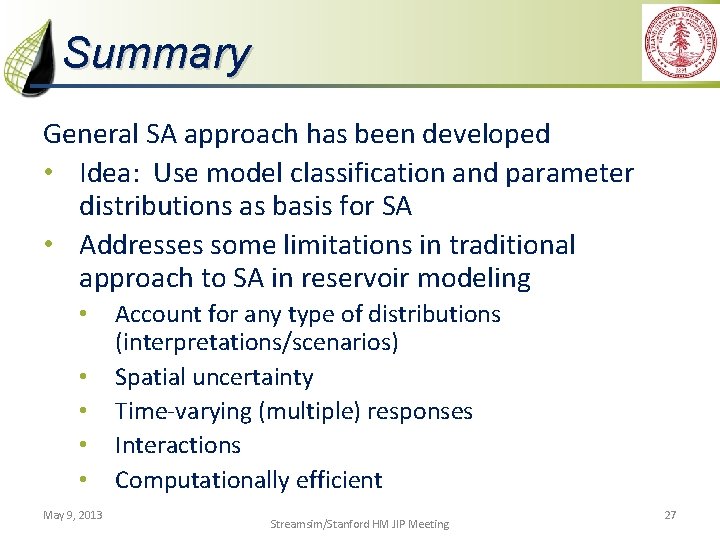
- Slides: 27
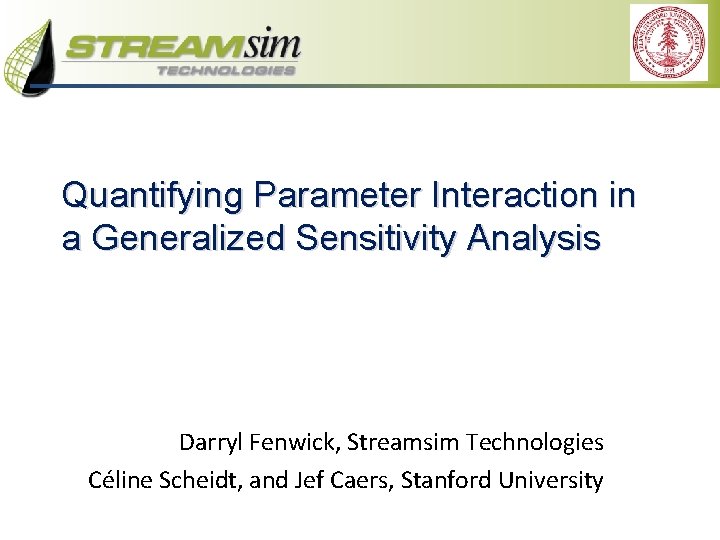
Quantifying Parameter Interaction in a Generalized Sensitivity Analysis Darryl Fenwick, Streamsim Technologies Céline Scheidt, and Jef Caers, Stanford University
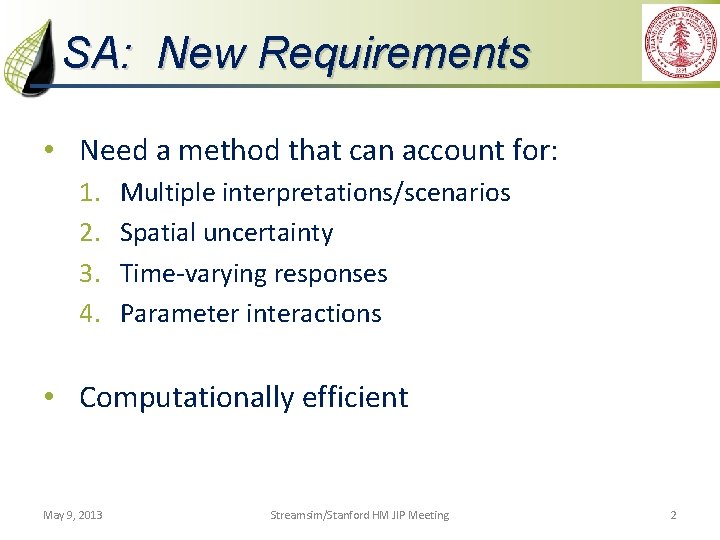
SA: New Requirements • Need a method that can account for: 1. 2. 3. 4. Multiple interpretations/scenarios Spatial uncertainty Time-varying responses Parameter interactions • Computationally efficient May 9, 2013 Streamsim/Stanford HM JIP Meeting 2
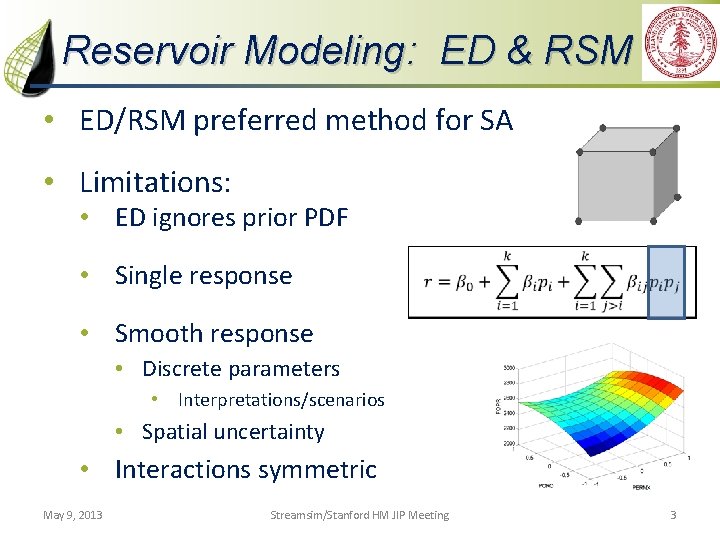
Reservoir Modeling: ED & RSM • ED/RSM preferred method for SA • Limitations: • ED ignores prior PDF • Single response • Smooth response • Discrete parameters • Interpretations/scenarios • Spatial uncertainty • Interactions symmetric May 9, 2013 Streamsim/Stanford HM JIP Meeting 3
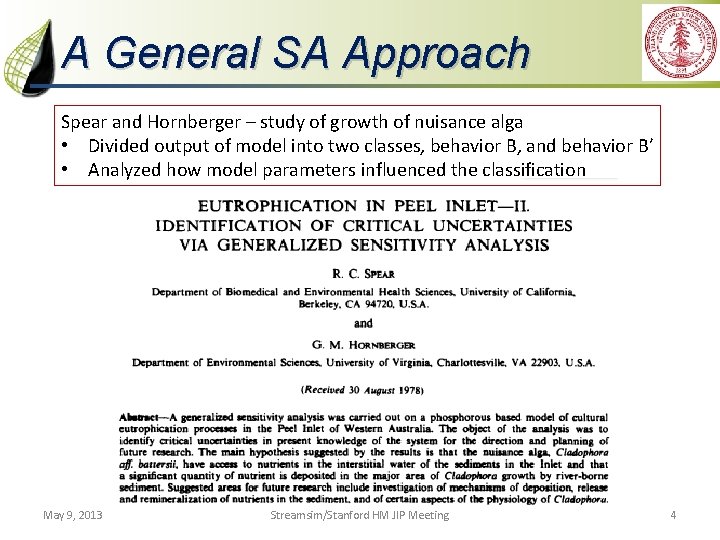
A General SA Approach Spear and Hornberger – study of growth of nuisance alga • Divided output of model into two classes, behavior B, and behavior B’ • Analyzed how model parameters influenced the classification May 9, 2013 Streamsim/Stanford HM JIP Meeting 4
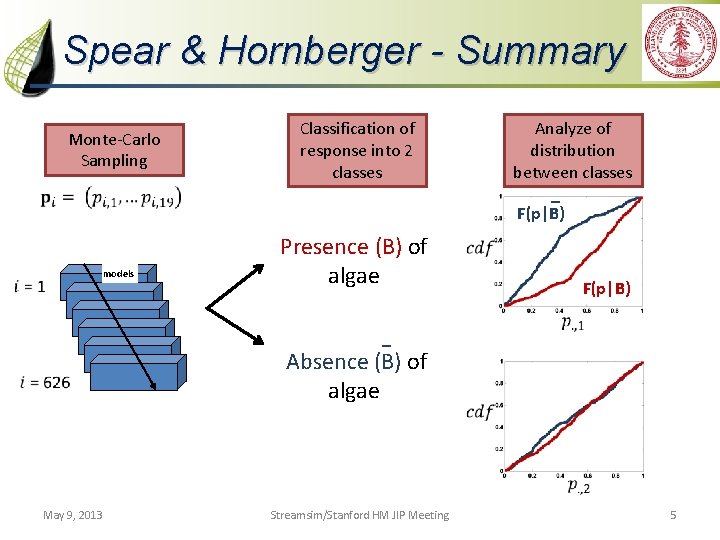
Spear & Hornberger - Summary Monte-Carlo Sampling Classification of response into 2 classes Analyze of distribution between classes _ F(p|B) models Presence (B) of algae F(p|B) _ Absence (B) of algae May 9, 2013 Streamsim/Stanford HM JIP Meeting 5
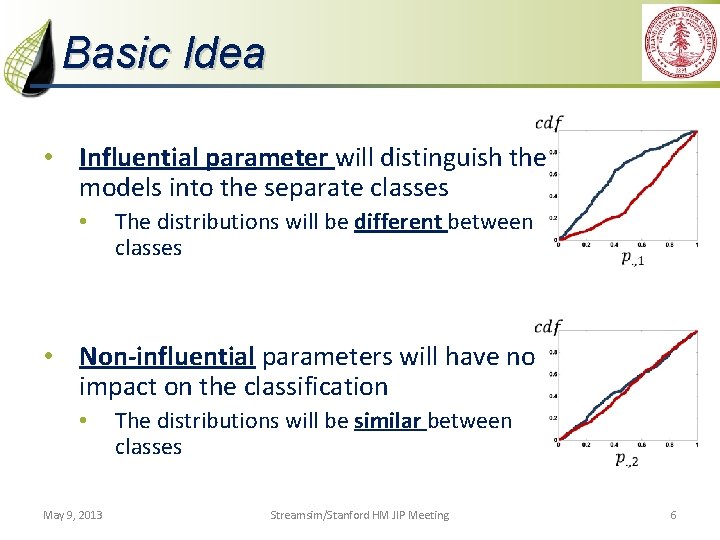
Basic Idea • Influential parameter will distinguish the models into the separate classes • The distributions will be different between classes • Non-influential parameters will have no impact on the classification • May 9, 2013 The distributions will be similar between classes Streamsim/Stanford HM JIP Meeting 6
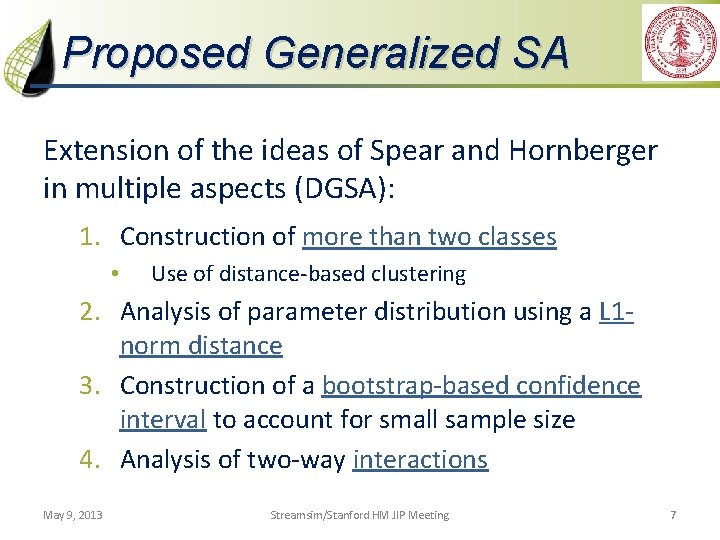
Proposed Generalized SA Extension of the ideas of Spear and Hornberger in multiple aspects (DGSA): 1. Construction of more than two classes • Use of distance-based clustering 2. Analysis of parameter distribution using a L 1 norm distance 3. Construction of a bootstrap-based confidence interval to account for small sample size 4. Analysis of two-way interactions May 9, 2013 Streamsim/Stanford HM JIP Meeting 7
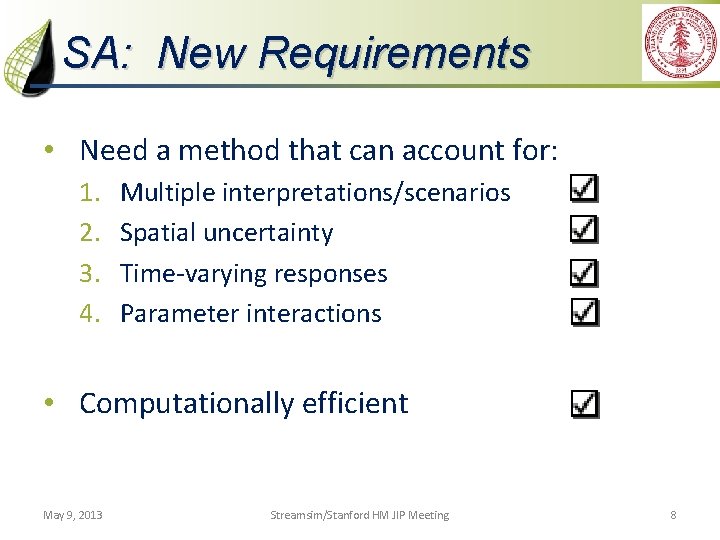
SA: New Requirements • Need a method that can account for: 1. 2. 3. 4. Multiple interpretations/scenarios Spatial uncertainty Time-varying responses Parameter interactions • Computationally efficient May 9, 2013 Streamsim/Stanford HM JIP Meeting 8
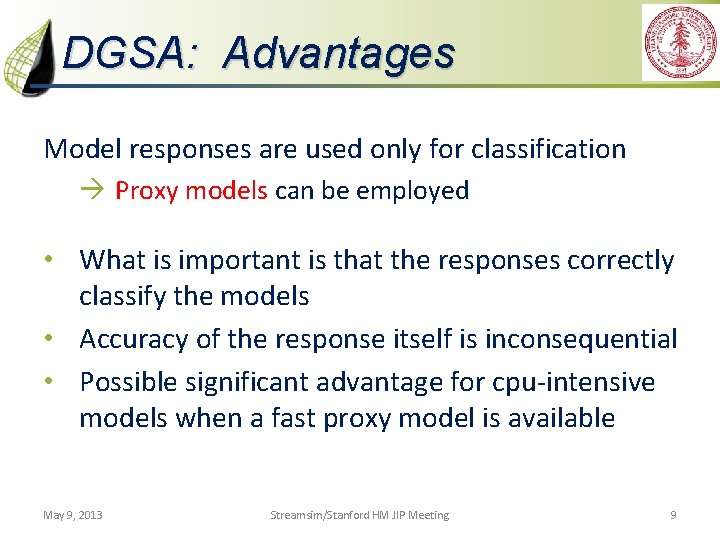
DGSA: Advantages Model responses are used only for classification Proxy models can be employed • What is important is that the responses correctly classify the models • Accuracy of the response itself is inconsequential • Possible significant advantage for cpu-intensive models when a fast proxy model is available May 9, 2013 Streamsim/Stanford HM JIP Meeting 9
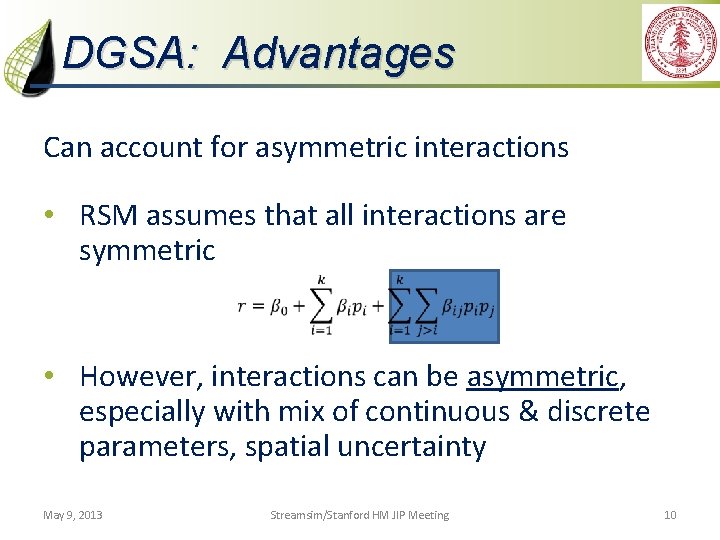
DGSA: Advantages Can account for asymmetric interactions • RSM assumes that all interactions are symmetric • However, interactions can be asymmetric, especially with mix of continuous & discrete parameters, spatial uncertainty May 9, 2013 Streamsim/Stanford HM JIP Meeting 10
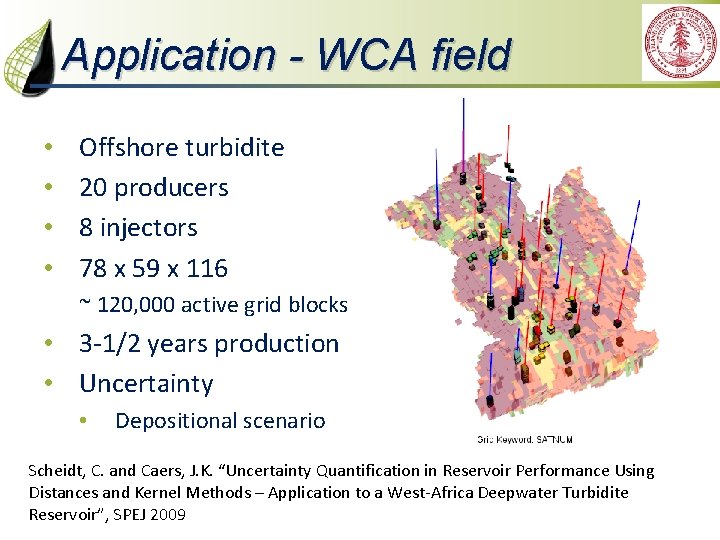
Application - WCA field • • Offshore turbidite 20 producers 8 injectors 78 x 59 x 116 ~ 120, 000 active grid blocks • 3 -1/2 years production • Uncertainty • Depositional scenario Scheidt, C. and Caers, J. K. “Uncertainty Quantification in Reservoir Performance Using Distances and Kernel Methods – Application to a West-Africa Deepwater Turbidite May 9, 2013 Streamsim/Stanford HM JIP Meeting Reservoir”, SPEJ 2009 11
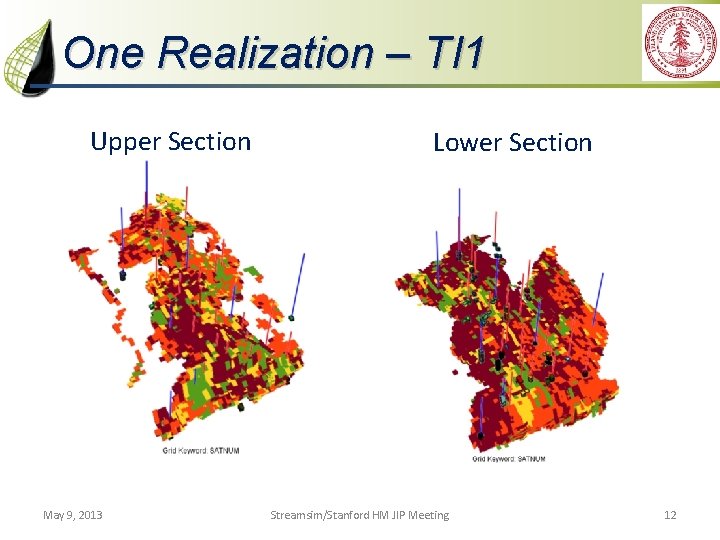
One Realization – TI 1 Upper Section May 9, 2013 Lower Section Streamsim/Stanford HM JIP Meeting 12
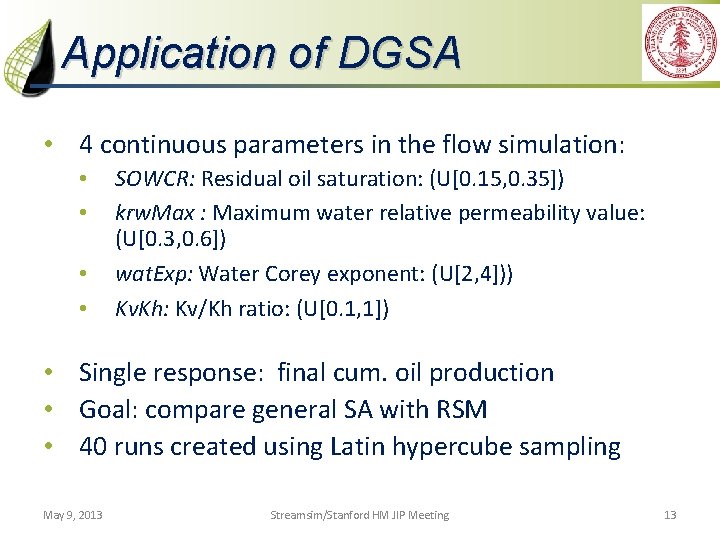
Application of DGSA • 4 continuous parameters in the flow simulation: • • SOWCR: Residual oil saturation: (U[0. 15, 0. 35]) krw. Max : Maximum water relative permeability value: (U[0. 3, 0. 6]) wat. Exp: Water Corey exponent: (U[2, 4])) Kv. Kh: Kv/Kh ratio: (U[0. 1, 1]) • Single response: final cum. oil production • Goal: compare general SA with RSM • 40 runs created using Latin hypercube sampling May 9, 2013 Streamsim/Stanford HM JIP Meeting 13
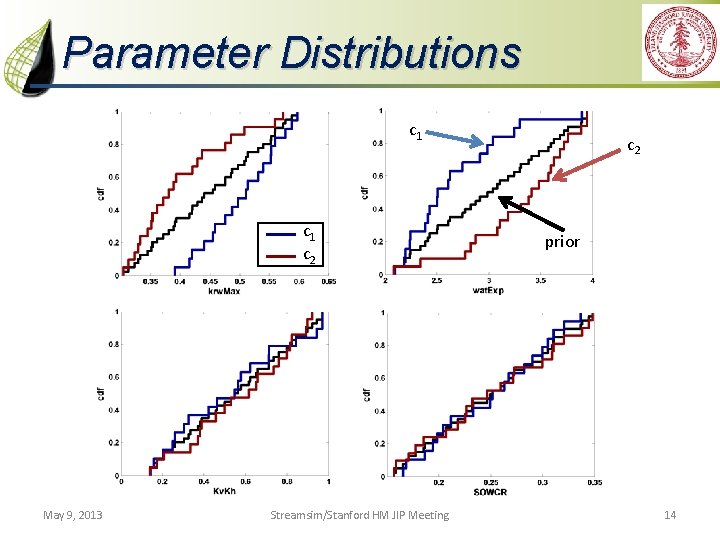
Parameter Distributions c 1 c 2 May 9, 2013 Streamsim/Stanford HM JIP Meeting c 2 prior 14
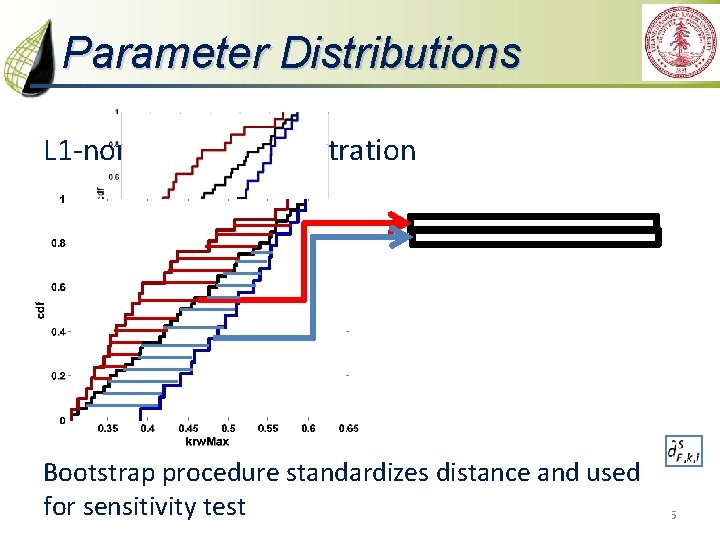
Parameter Distributions L 1 -norm distance illustration c 1 c 2 Bootstrap procedure standardizes distance and used for sensitivity test Streamsim/Stanford HM JIP Meeting May 9, 2013 15
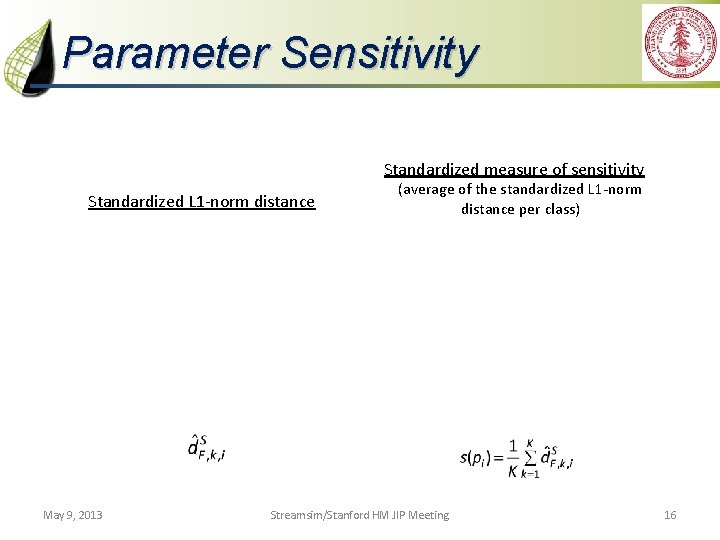
Parameter Sensitivity Standardized measure of sensitivity Standardized L 1 -norm distance May 9, 2013 (average of the standardized L 1 -norm distance per class) Streamsim/Stanford HM JIP Meeting 16
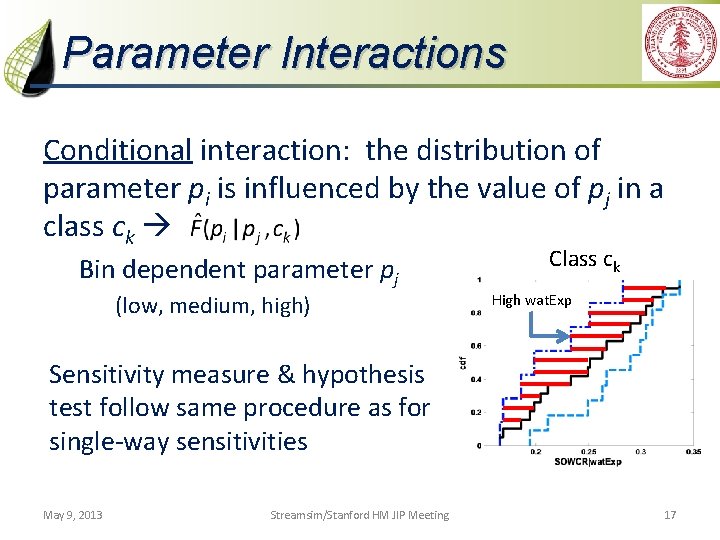
Parameter Interactions Conditional interaction: the distribution of parameter pi is influenced by the value of pj in a class ck Bin dependent parameter pj (low, medium, high) Class ck High wat. Exp Sensitivity measure & hypothesis test follow same procedure as for single-way sensitivities May 9, 2013 Streamsim/Stanford HM JIP Meeting 17
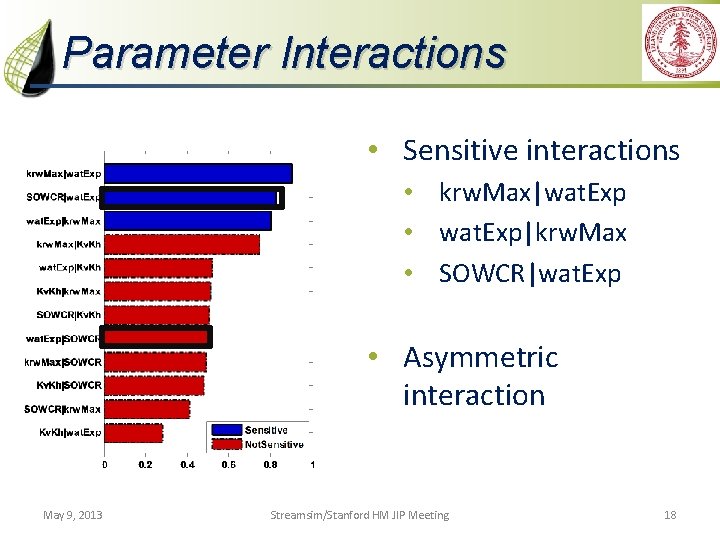
Parameter Interactions • Sensitive interactions • krw. Max|wat. Exp • wat. Exp|krw. Max • SOWCR|wat. Exp • Asymmetric interaction May 9, 2013 Streamsim/Stanford HM JIP Meeting 18
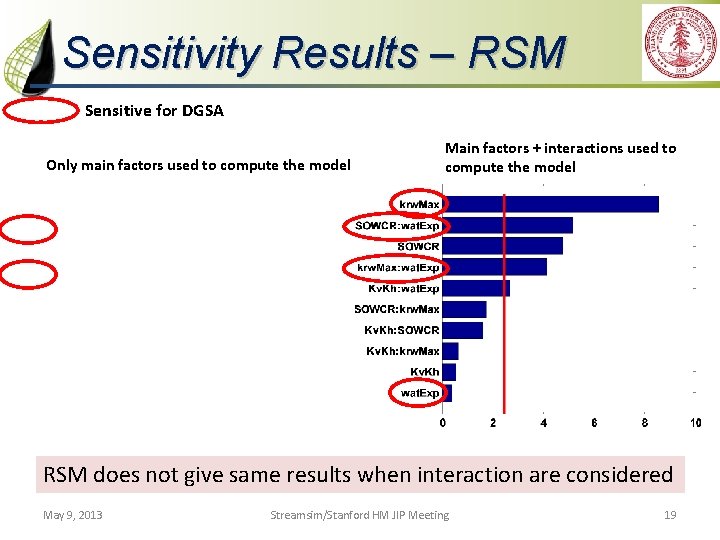
Sensitivity Results – RSM Sensitive for DGSA Only main factors used to compute the model Main factors + interactions used to compute the model RSM does not give same results when interaction are considered May 9, 2013 Streamsim/Stanford HM JIP Meeting 19
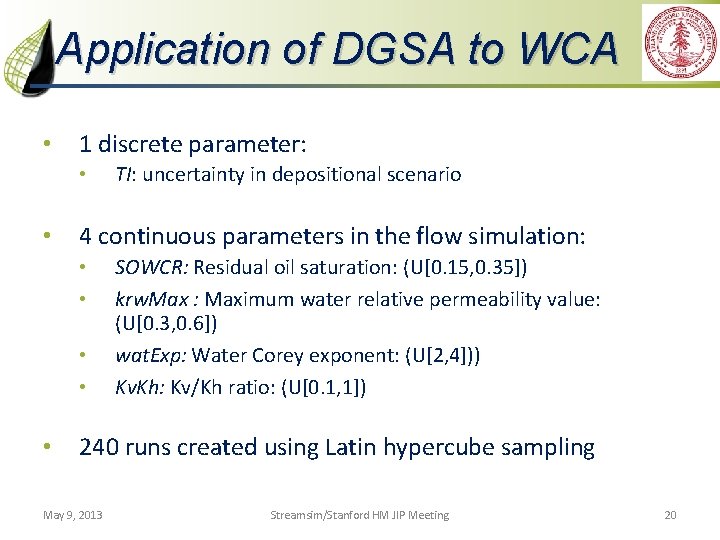
Application of DGSA to WCA • 1 discrete parameter: • • 4 continuous parameters in the flow simulation: • • • TI: uncertainty in depositional scenario SOWCR: Residual oil saturation: (U[0. 15, 0. 35]) krw. Max : Maximum water relative permeability value: (U[0. 3, 0. 6]) wat. Exp: Water Corey exponent: (U[2, 4])) Kv. Kh: Kv/Kh ratio: (U[0. 1, 1]) 240 runs created using Latin hypercube sampling May 9, 2013 Streamsim/Stanford HM JIP Meeting 20
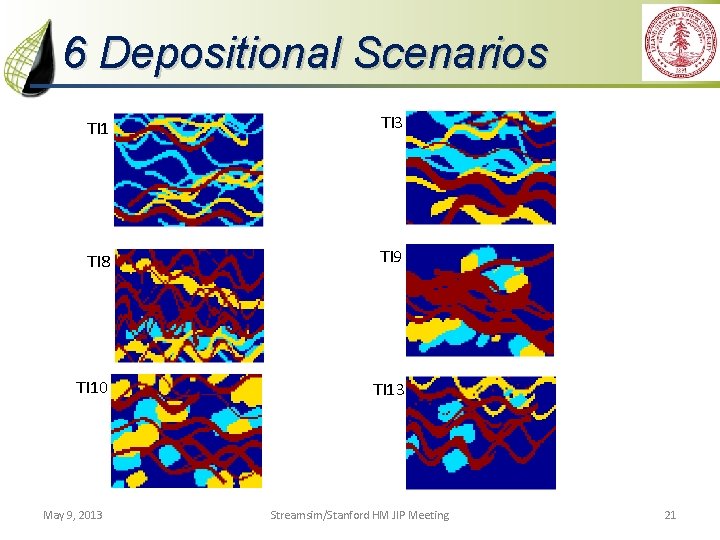
6 Depositional Scenarios TI 1 TI 3 TI 8 TI 9 TI 10 TI 13 May 9, 2013 Streamsim/Stanford HM JIP Meeting 21
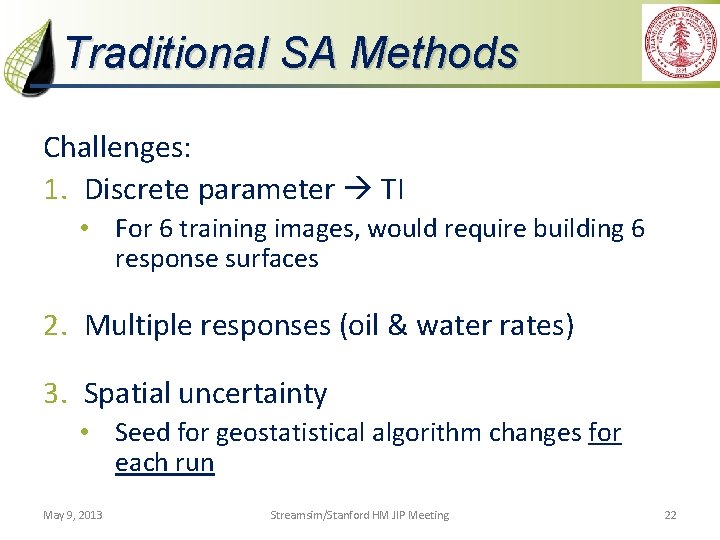
Traditional SA Methods Challenges: 1. Discrete parameter TI • For 6 training images, would require building 6 response surfaces 2. Multiple responses (oil & water rates) 3. Spatial uncertainty • Seed for geostatistical algorithm changes for each run May 9, 2013 Streamsim/Stanford HM JIP Meeting 22
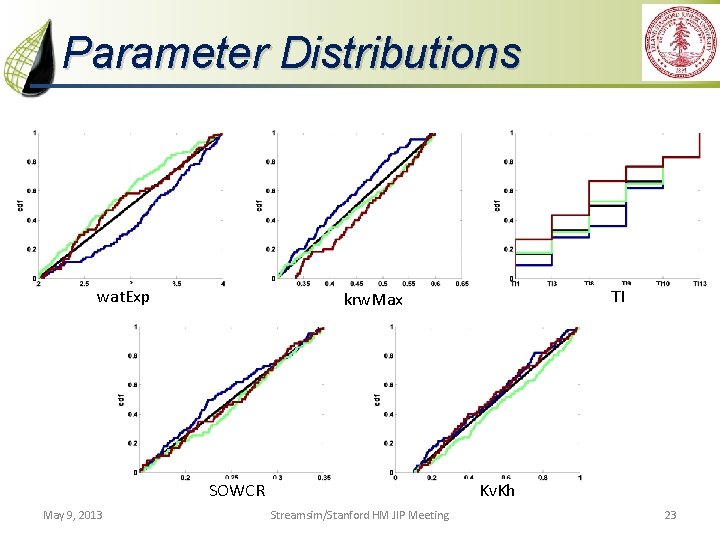
Parameter Distributions wat. Exp SOWCR May 9, 2013 TI krw. Max Kv. Kh Streamsim/Stanford HM JIP Meeting 23
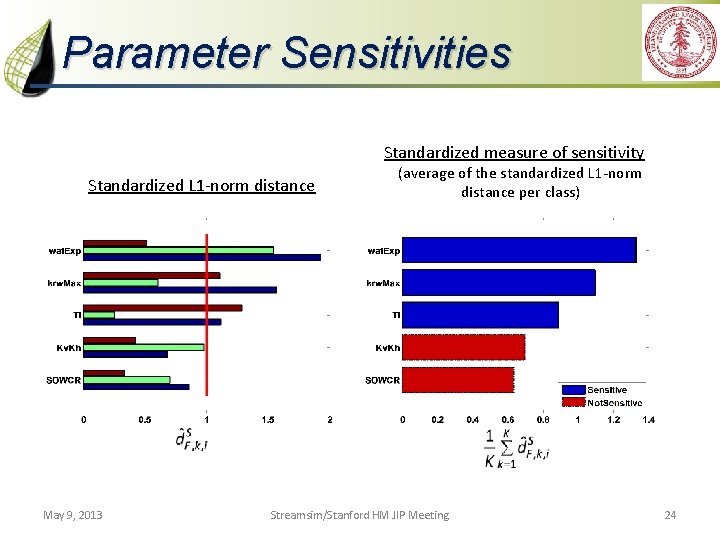
Parameter Sensitivities Standardized measure of sensitivity Standardized L 1 -norm distance May 9, 2013 (average of the standardized L 1 -norm distance per class) Streamsim/Stanford HM JIP Meeting 24
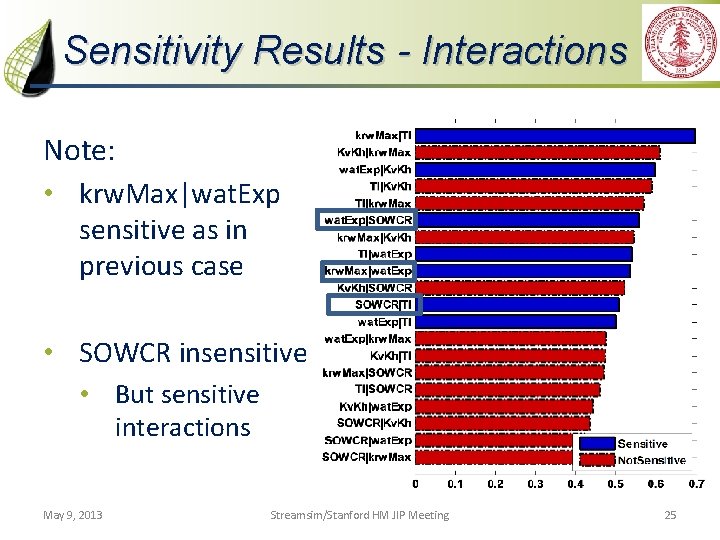
Sensitivity Results - Interactions Note: • krw. Max|wat. Exp sensitive as in previous case • SOWCR insensitive • But sensitive interactions May 9, 2013 Streamsim/Stanford HM JIP Meeting 25
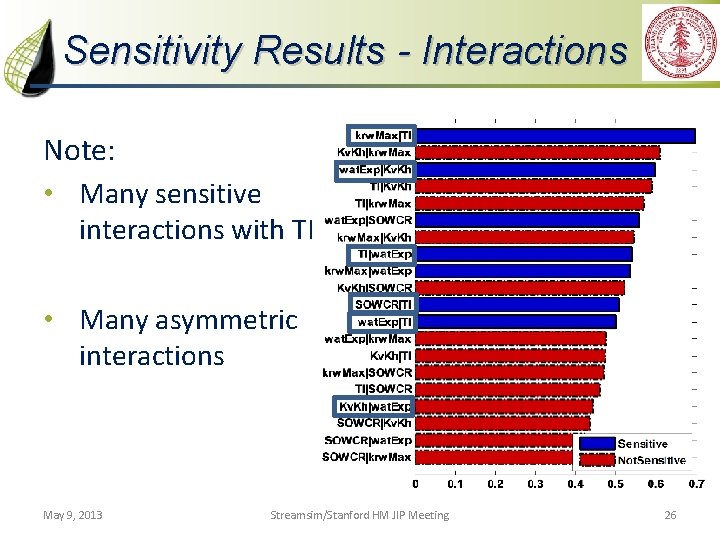
Sensitivity Results - Interactions Note: • Many sensitive interactions with TI • Many asymmetric interactions May 9, 2013 Streamsim/Stanford HM JIP Meeting 26
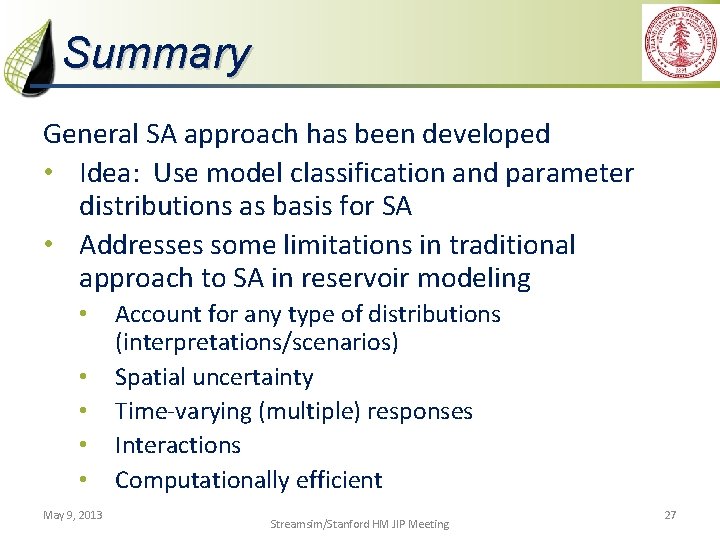
Summary General SA approach has been developed • Idea: Use model classification and parameter distributions as basis for SA • Addresses some limitations in traditional approach to SA in reservoir modeling • • • May 9, 2013 Account for any type of distributions (interpretations/scenarios) Spatial uncertainty Time-varying (multiple) responses Interactions Computationally efficient Streamsim/Stanford HM JIP Meeting 27
Everyone's an influencer quantifying influence on twitter
Quantify noun
Quantifying credit risk
George theodorakopoulos
When quantifying country risk:
Irr sensitivity analysis
Sensitivity analysis solver
Sensitivity analysis bayesian network
Sensitivity analysis simplex method
Solver shadow price
Sensitivity analysis and duality
Cap rate sensitivity analysis
Range of optimality examples
Advanced sensitivity analysis
Sensitivity analysis
Sensitivity analysis lecture notes
Interest rate risk sensitivity analysis
Profit sensitivity analysis
Psioptparam
Sensitivity analysis report
Cvp sensitivity analysis
Scenario analysis中文
Sensitivity analysis interest rate risk
Generalized principal component analysis
Generalized principal component analysis
Checklist method in entrepreneurship example
Gordon method in entrepreneurship
John dewey’s reflective thinking model