PSLC Data Shop Introduction http pslcdatashop org Slides
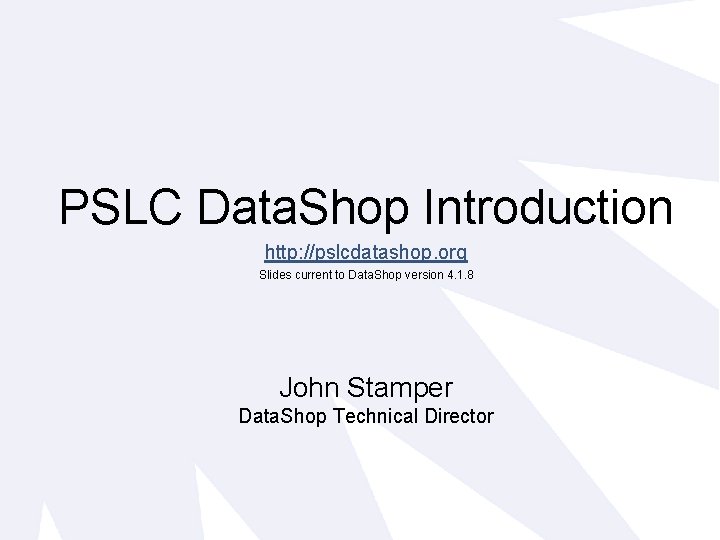
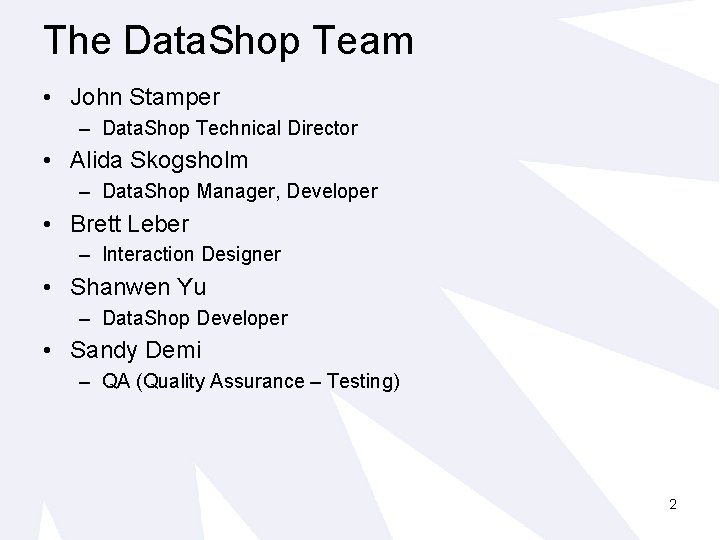
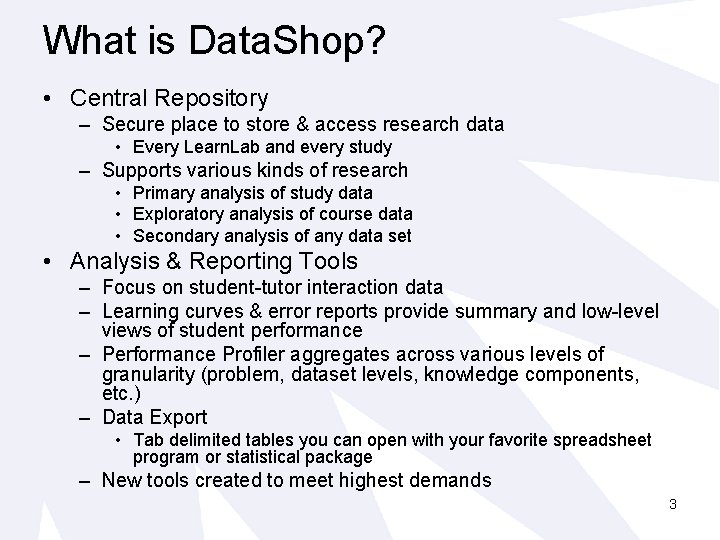
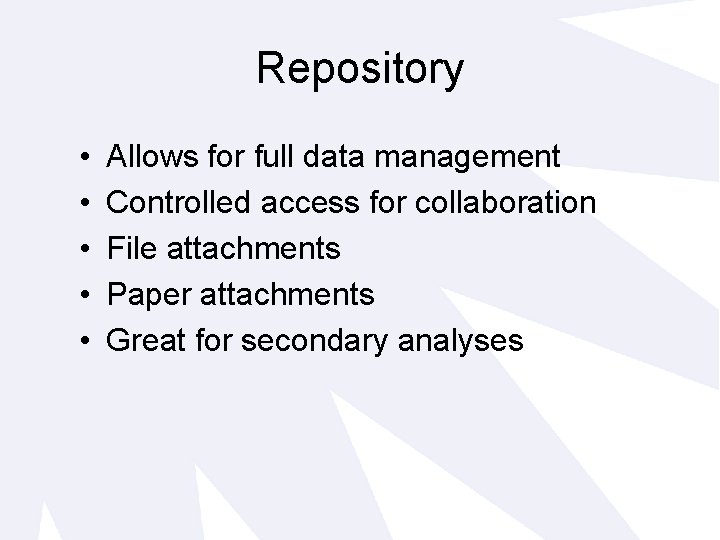
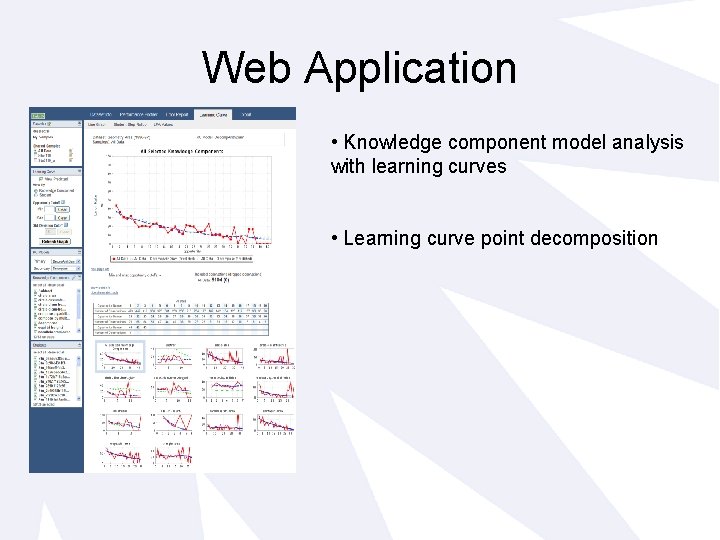
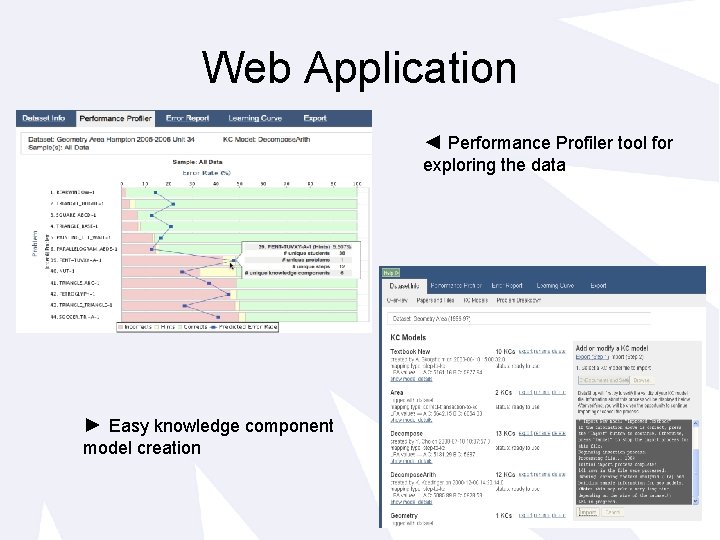
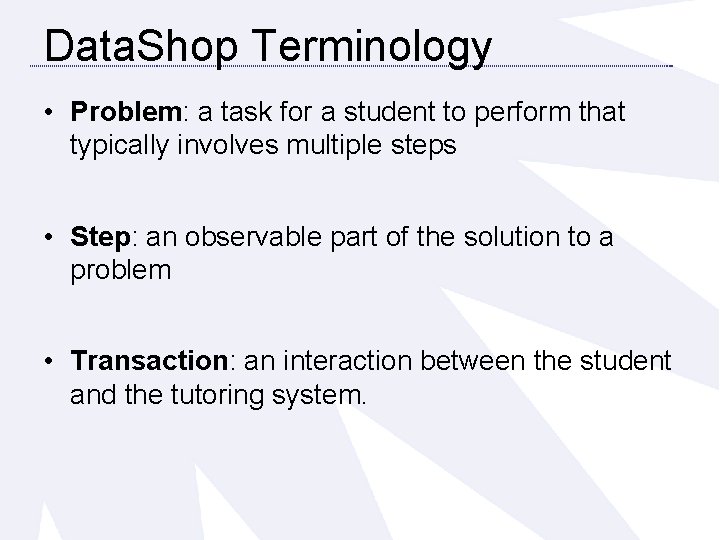
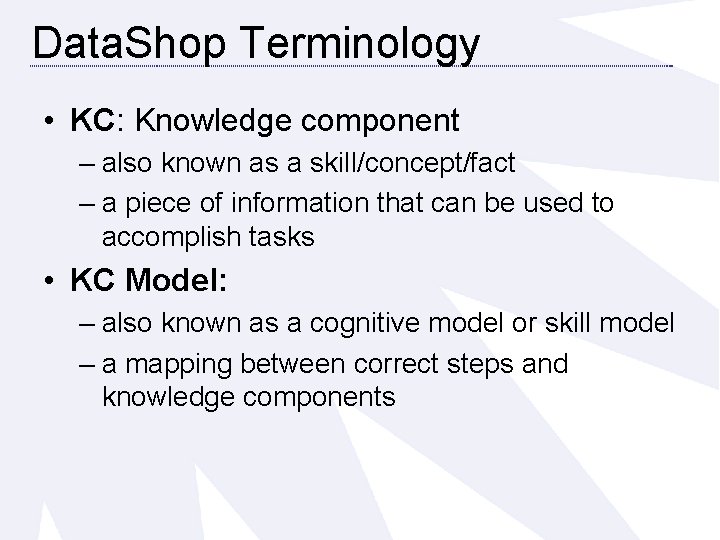
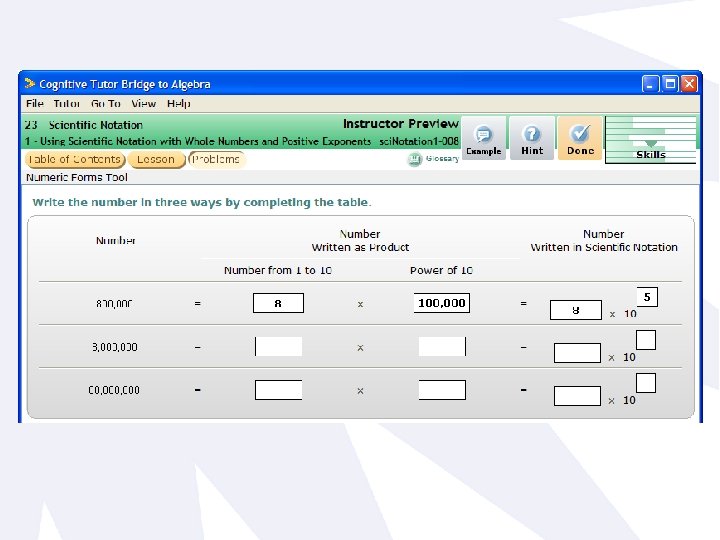
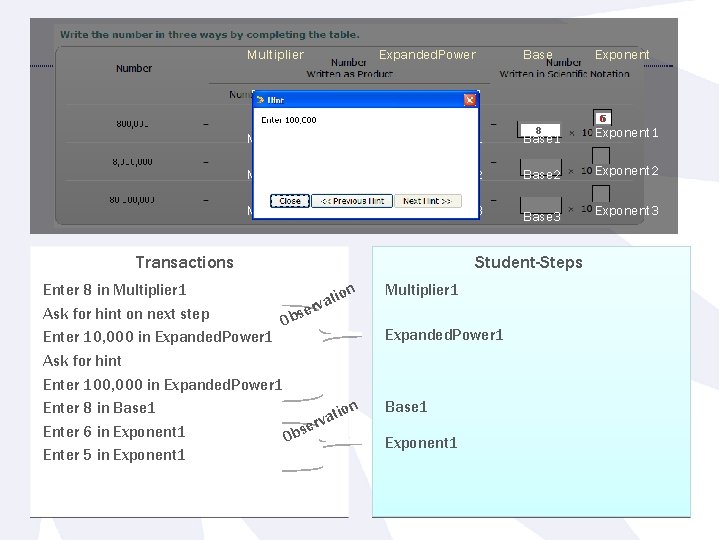
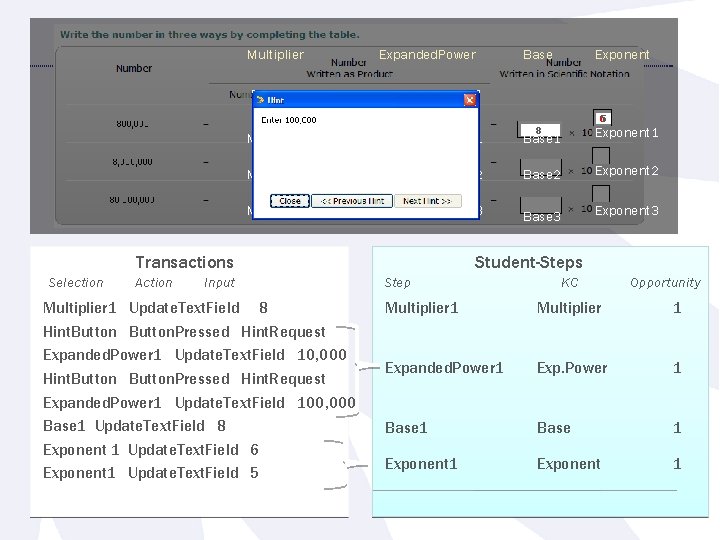
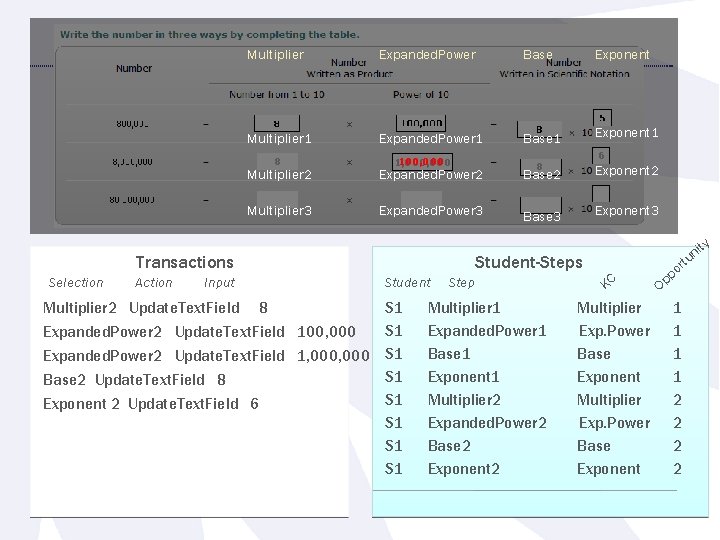
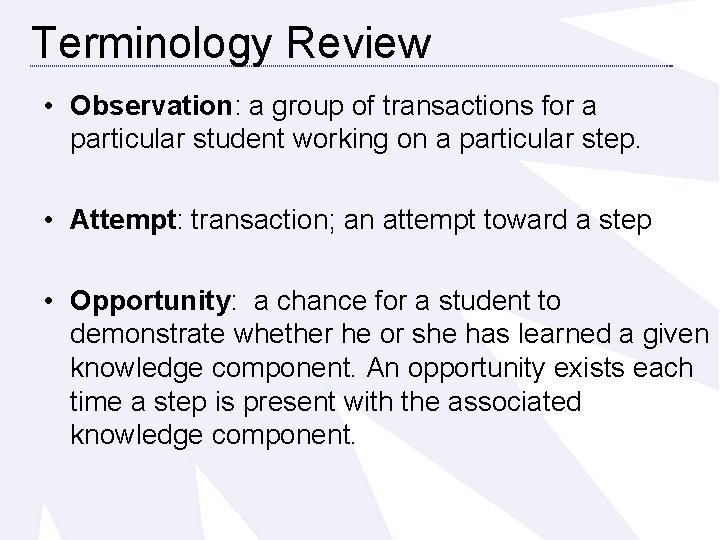
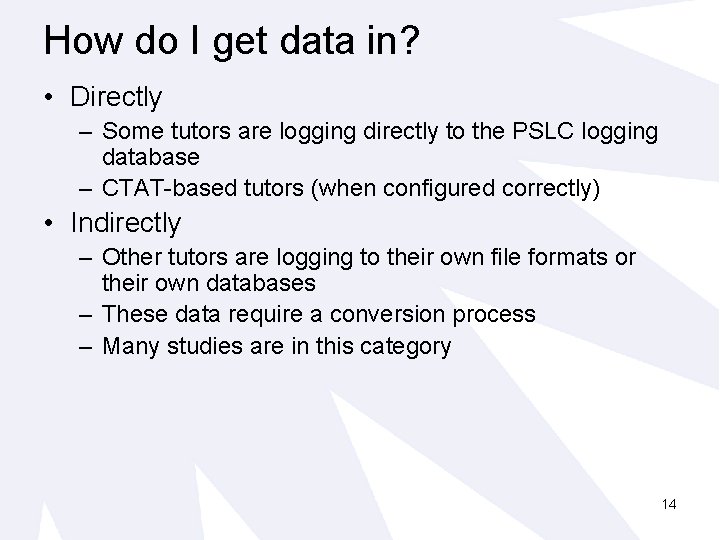
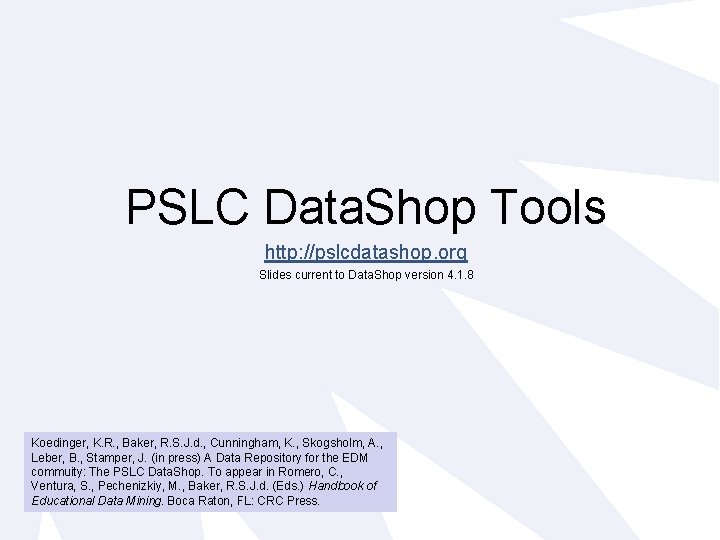
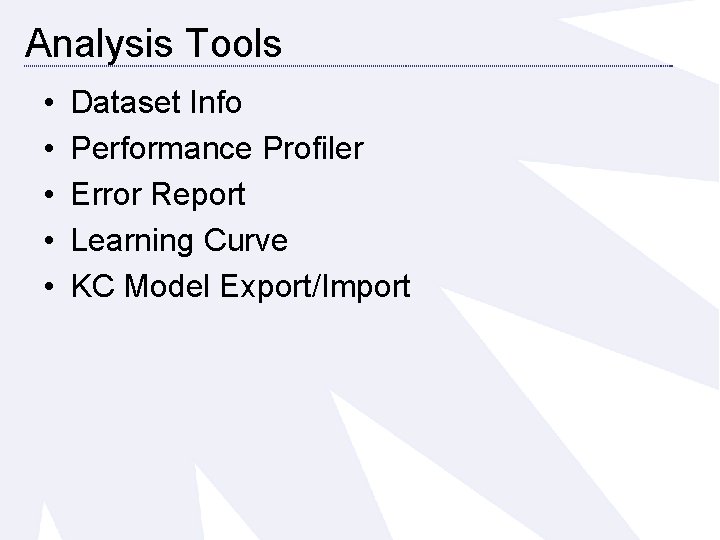
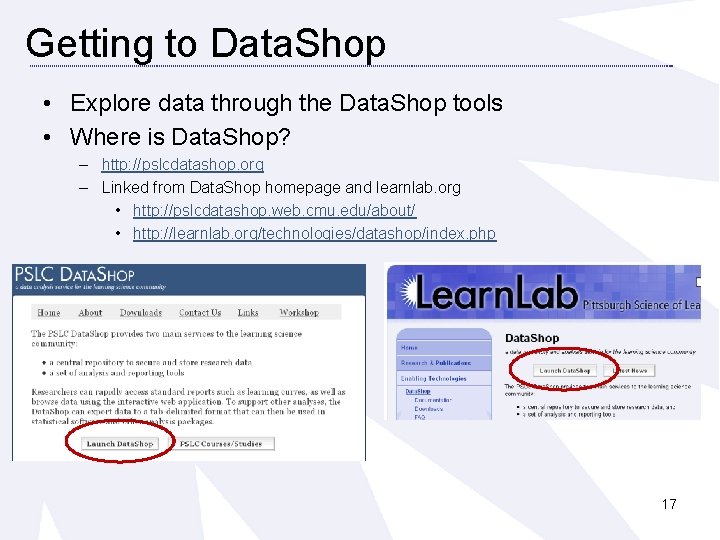
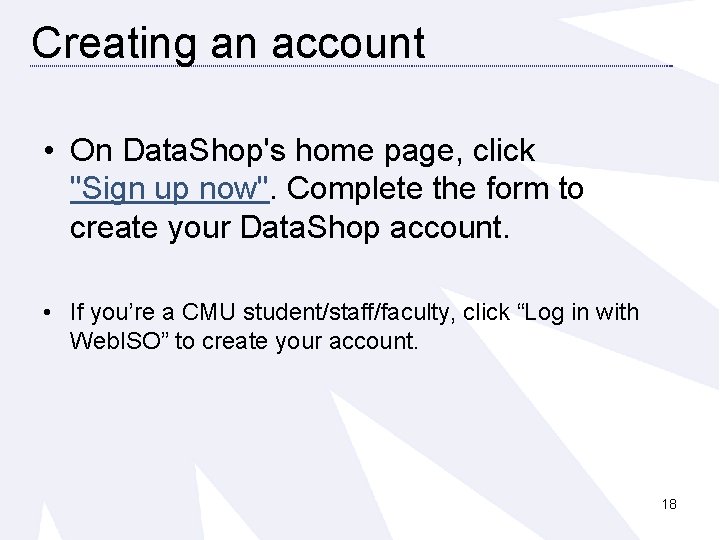
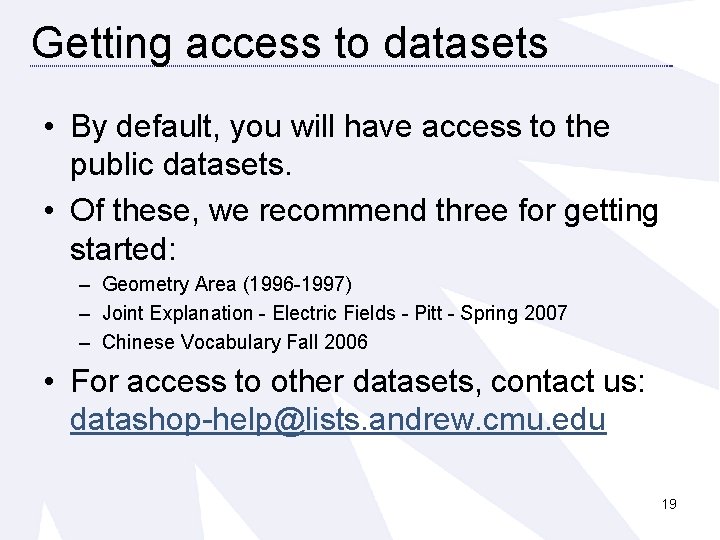
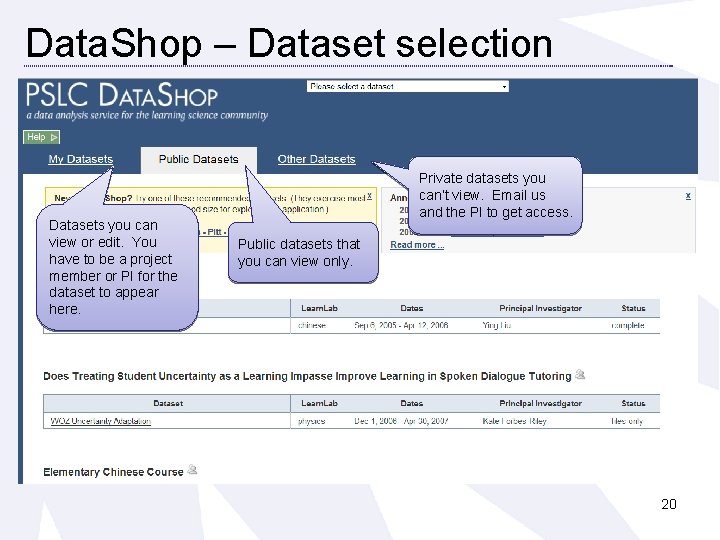
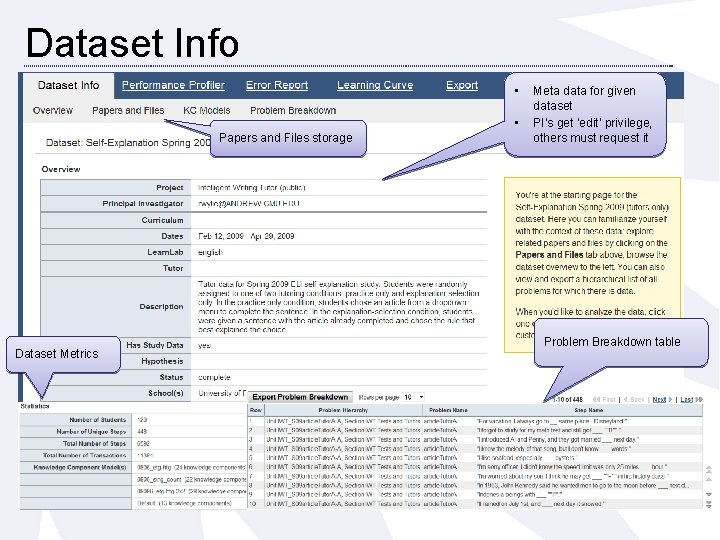
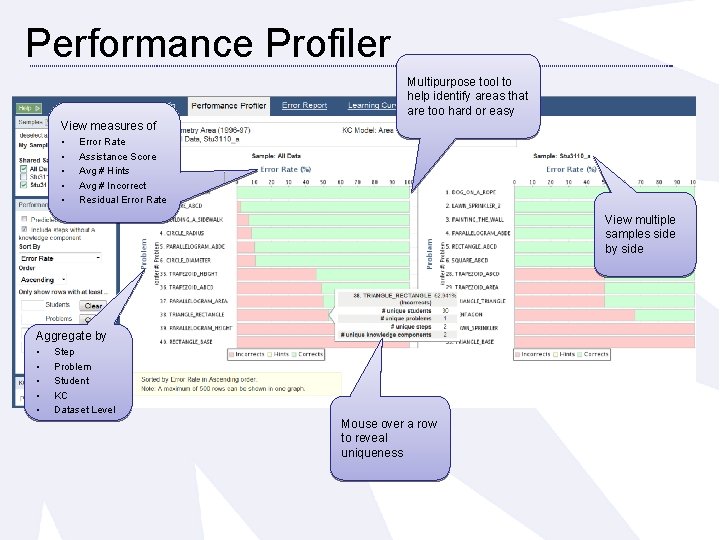
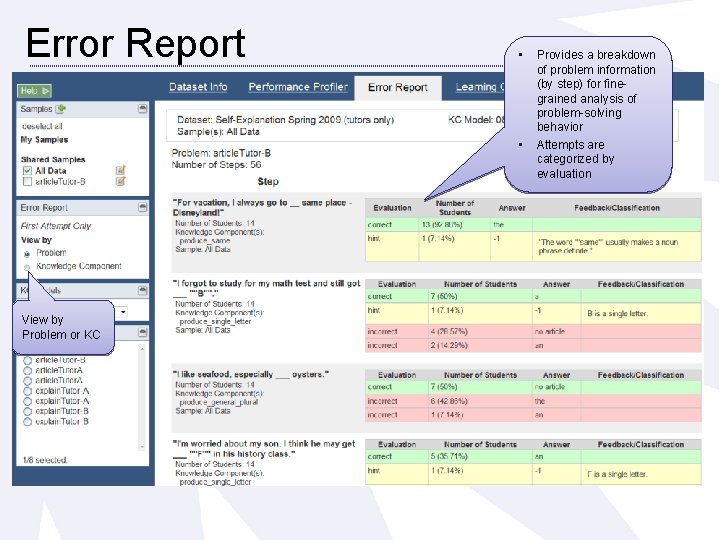
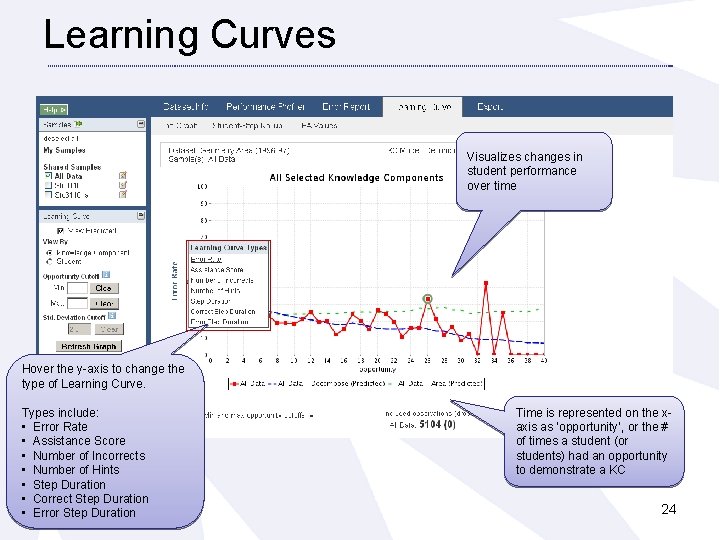
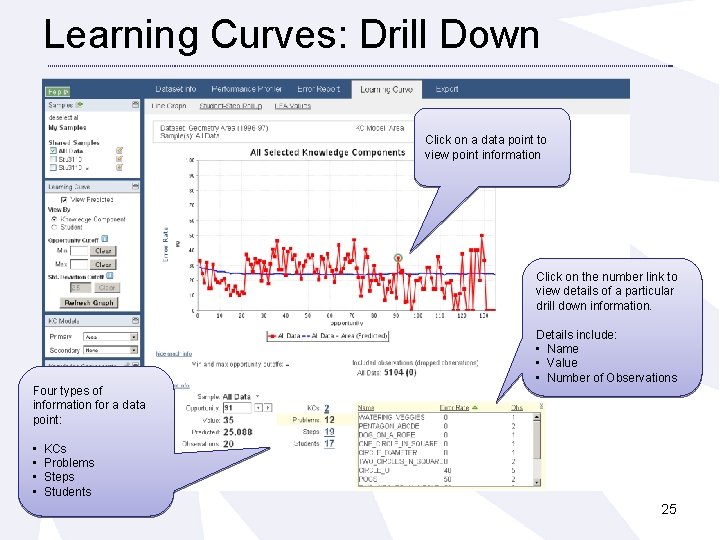
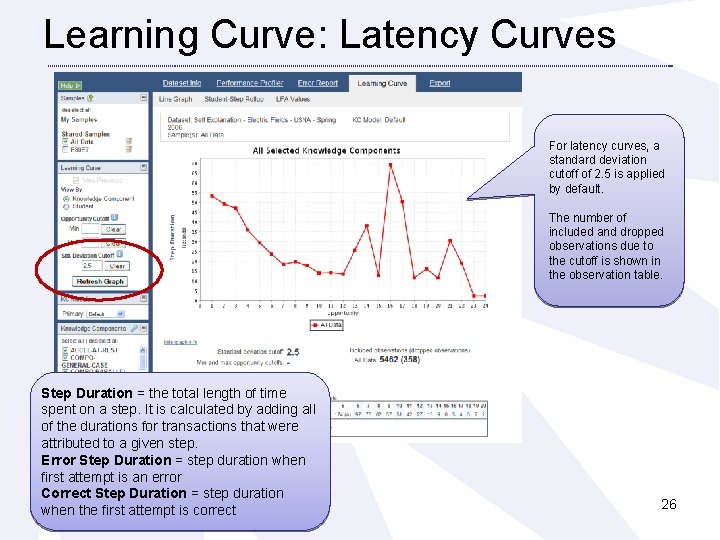
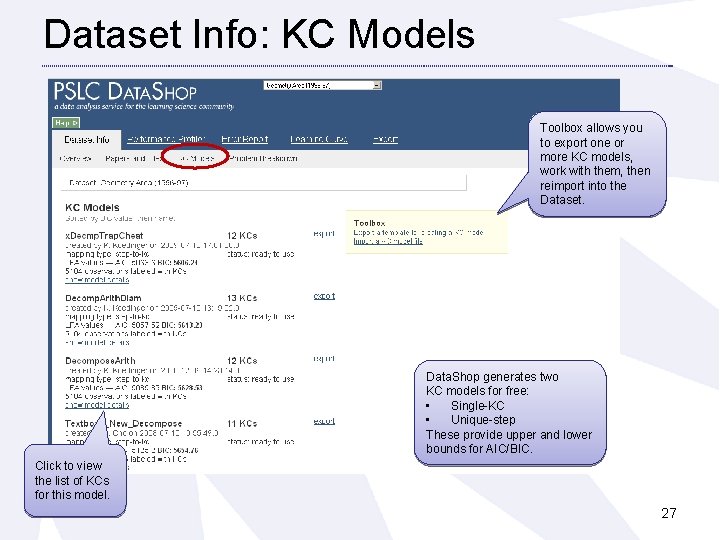
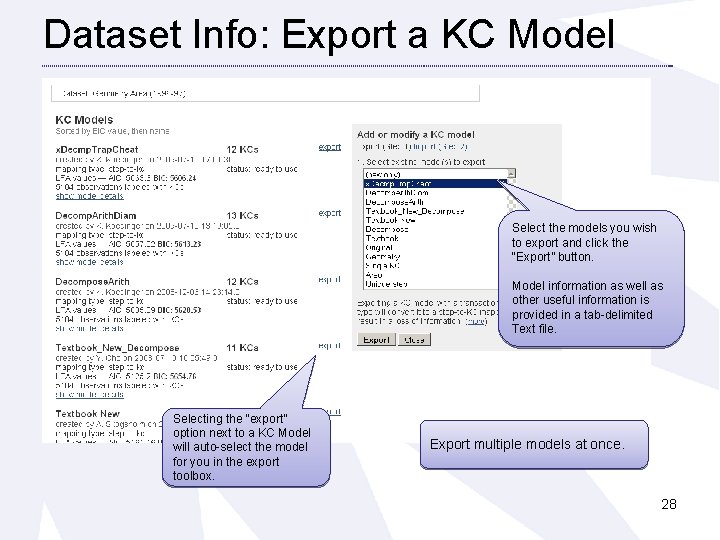
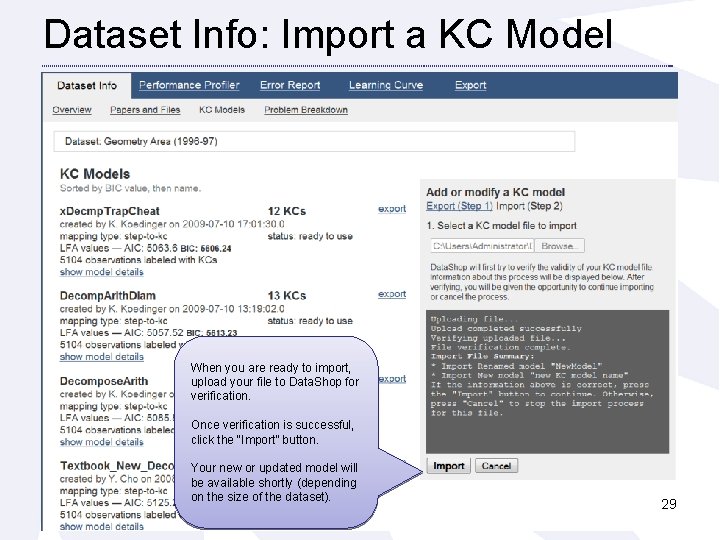
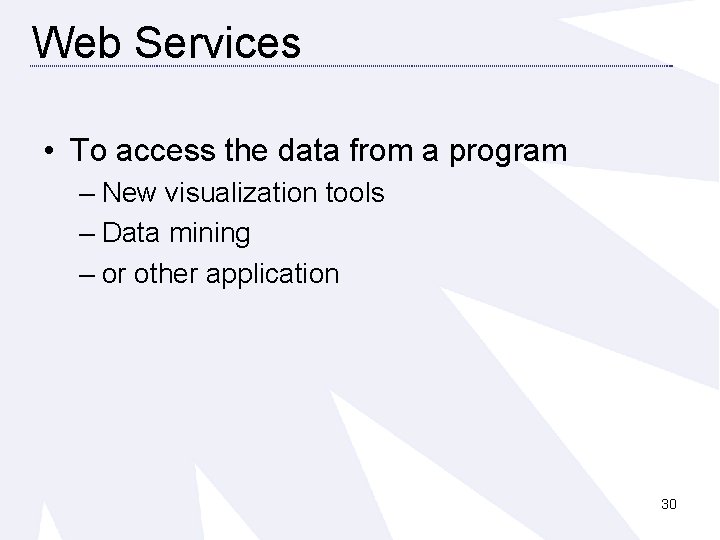
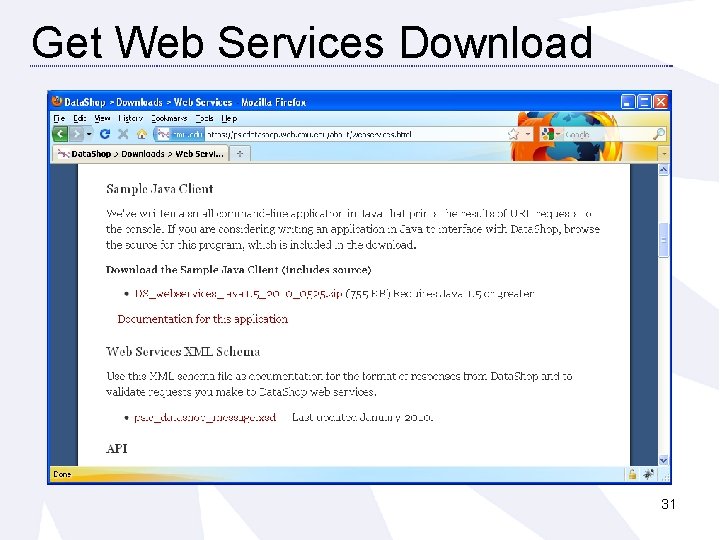
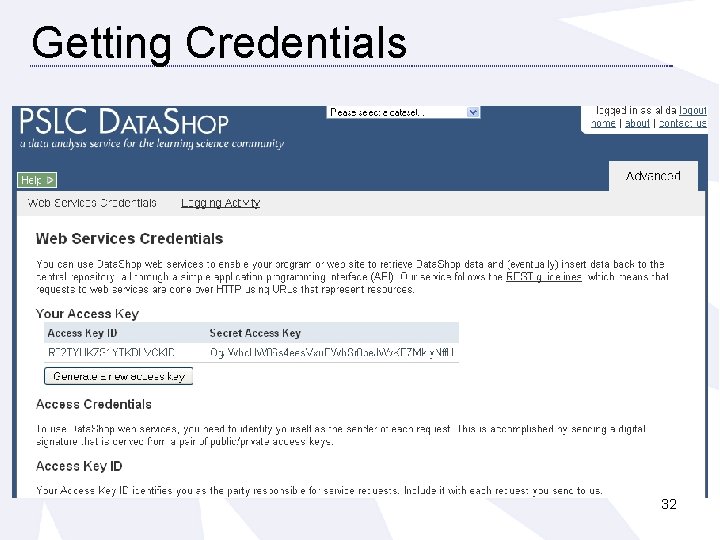
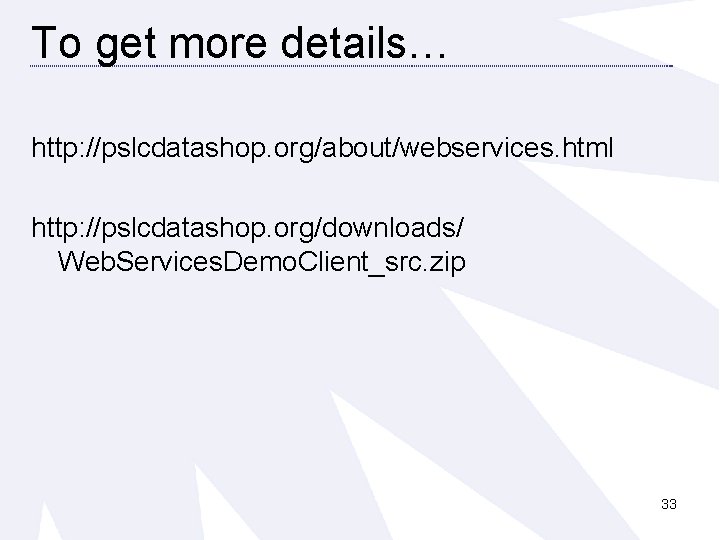
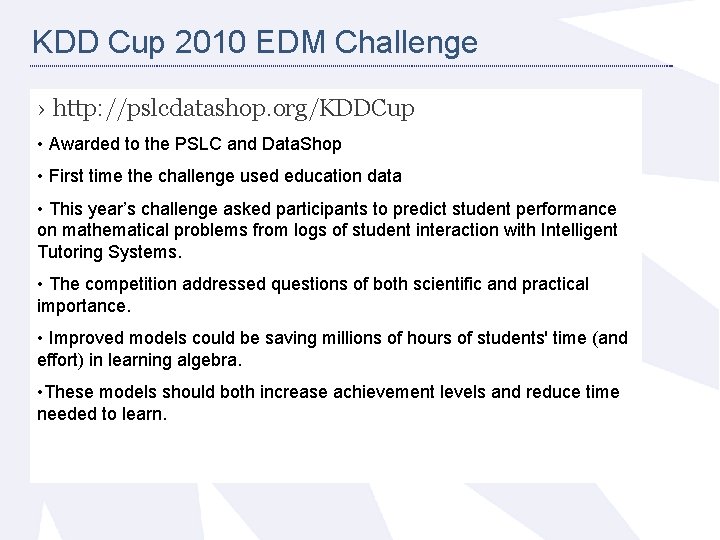
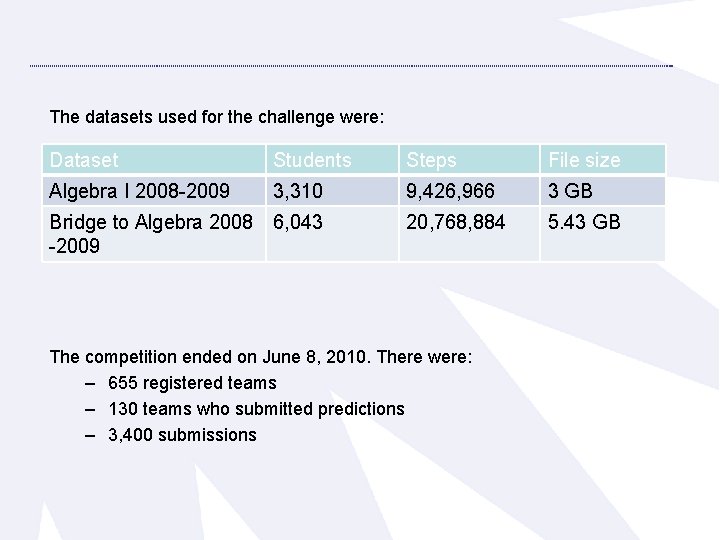
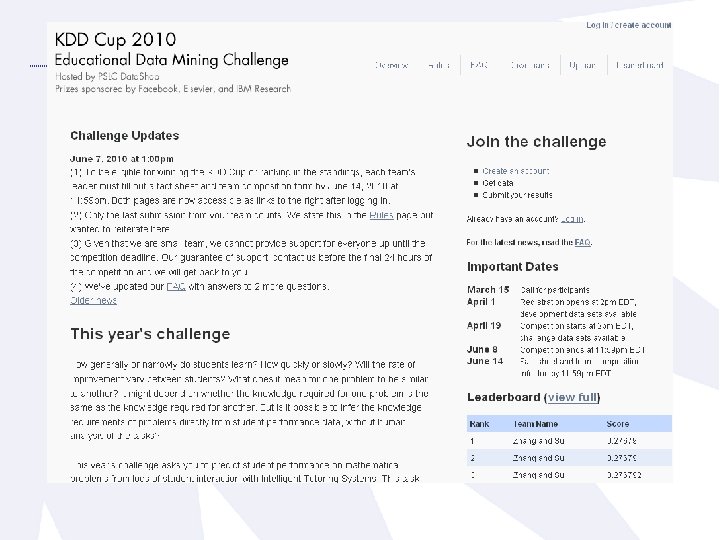
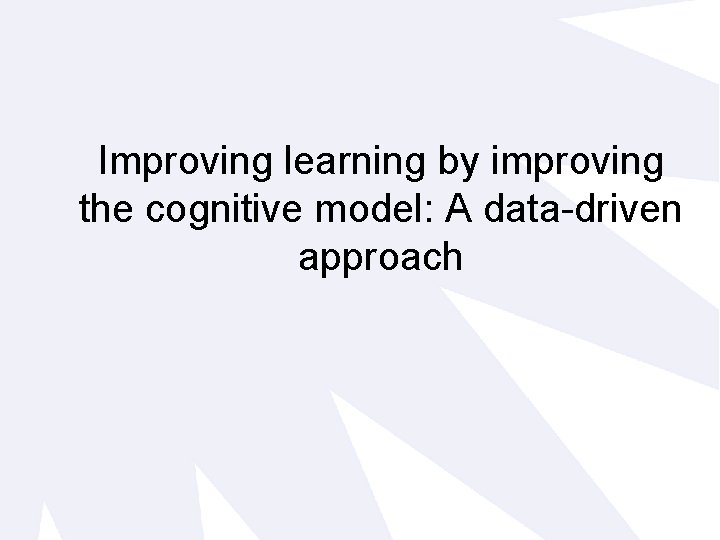
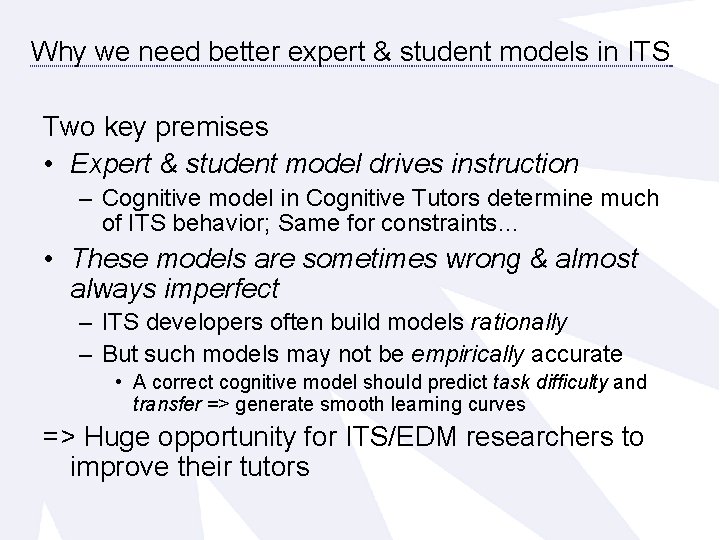
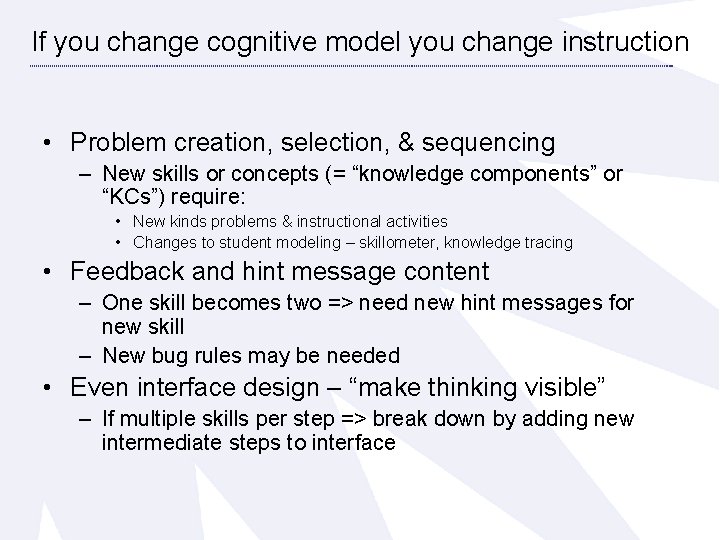
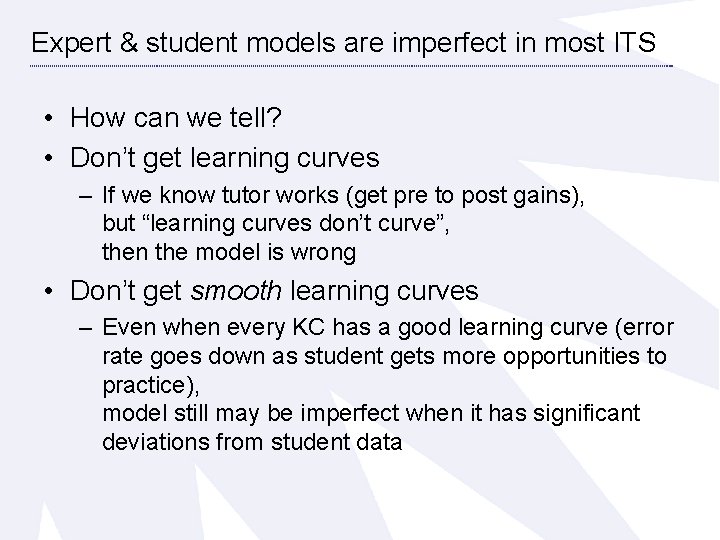
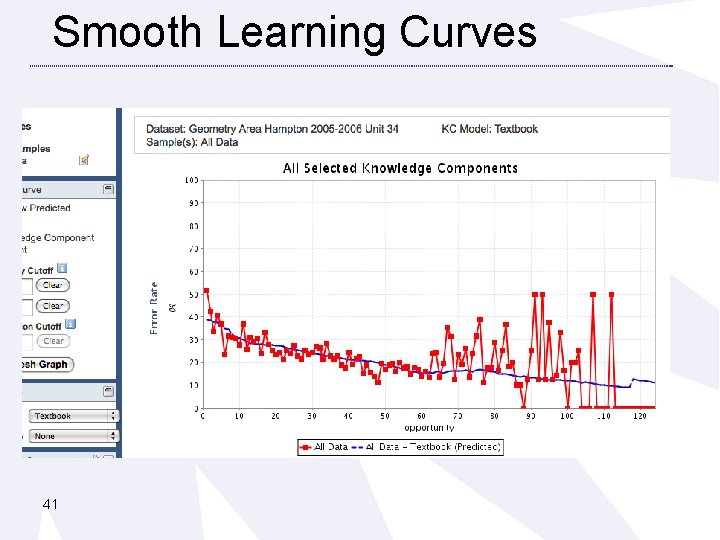
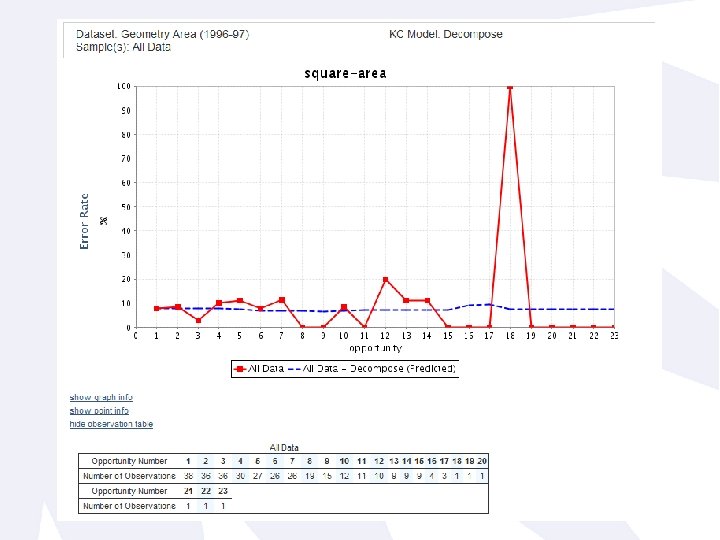
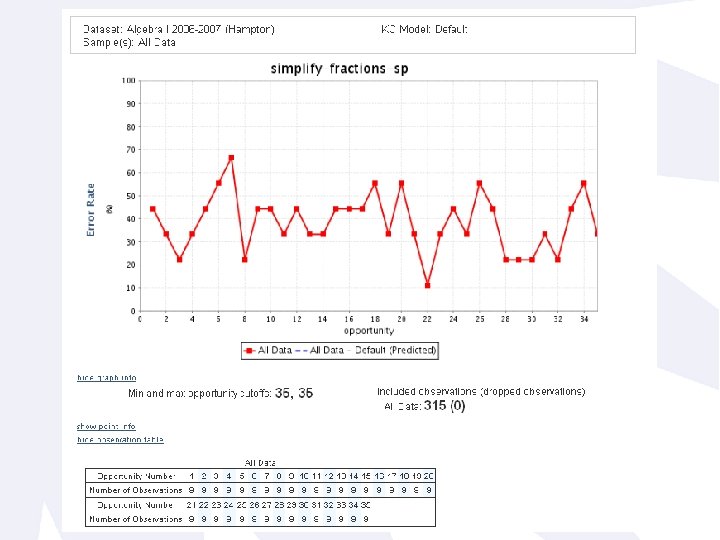
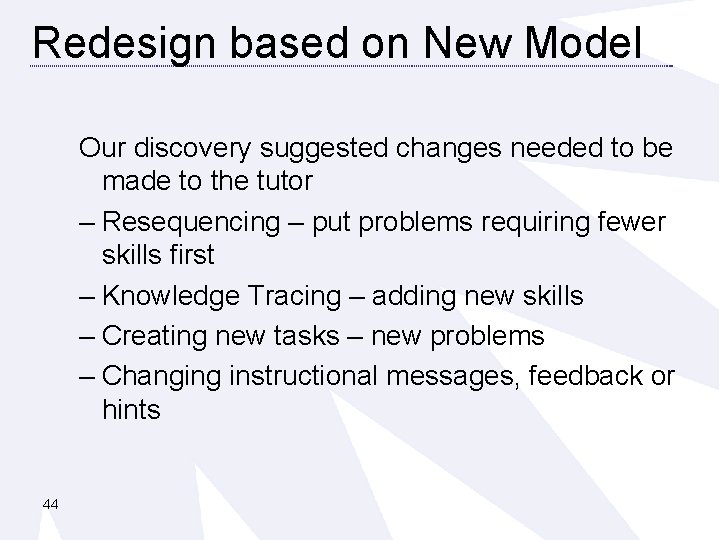
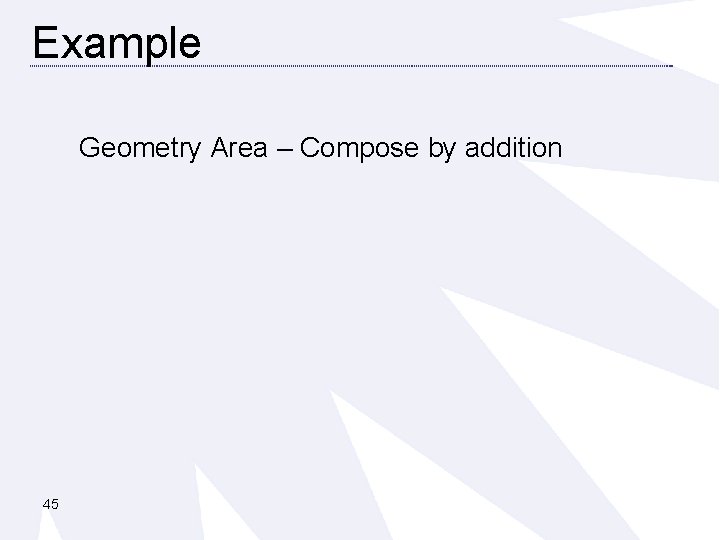
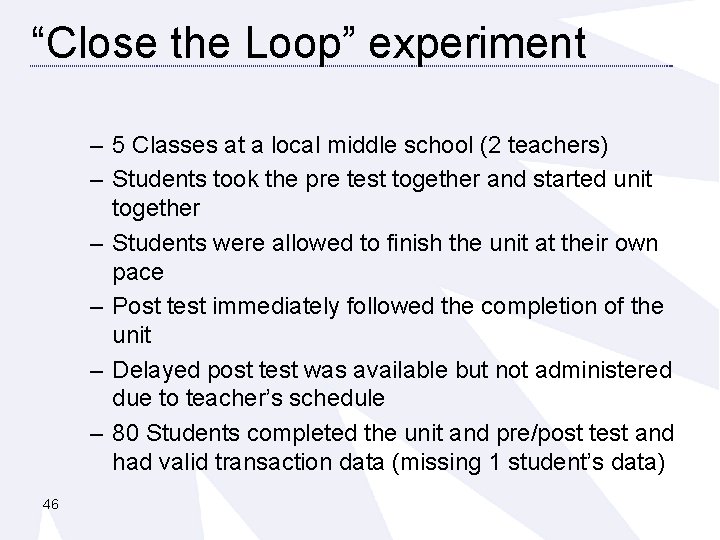
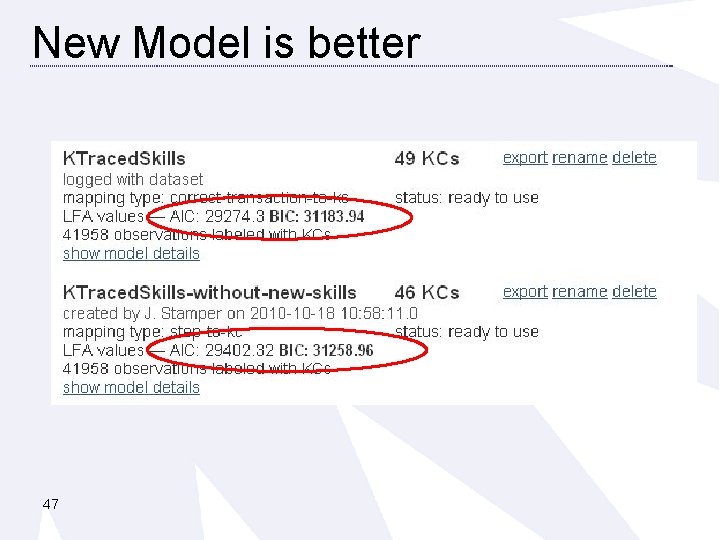
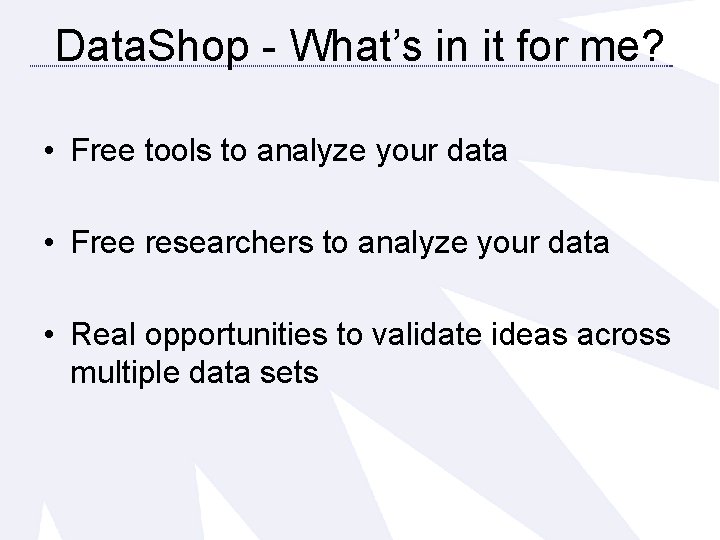
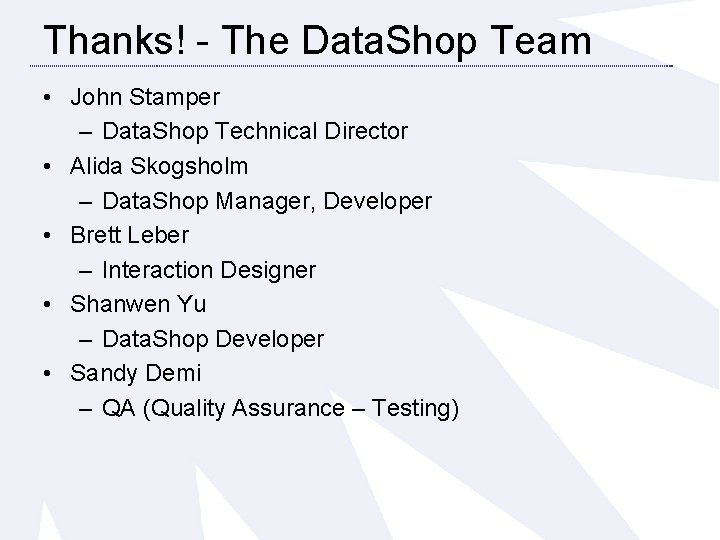
- Slides: 49
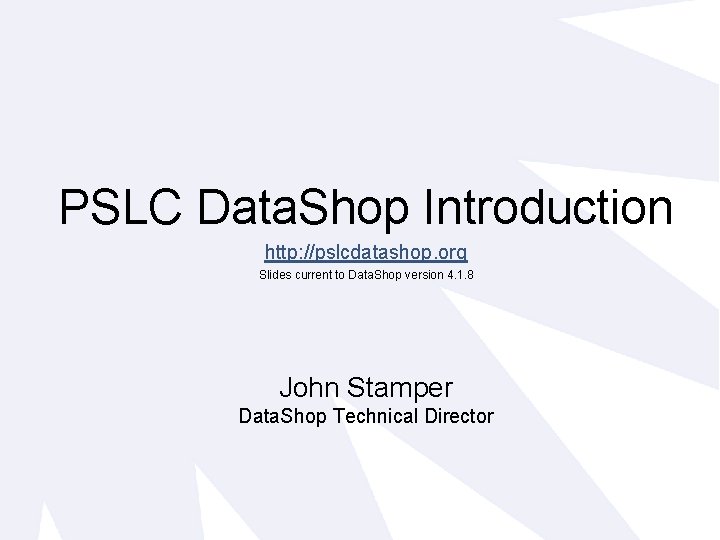
PSLC Data. Shop Introduction http: //pslcdatashop. org Slides current to Data. Shop version 4. 1. 8 John Stamper Data. Shop Technical Director
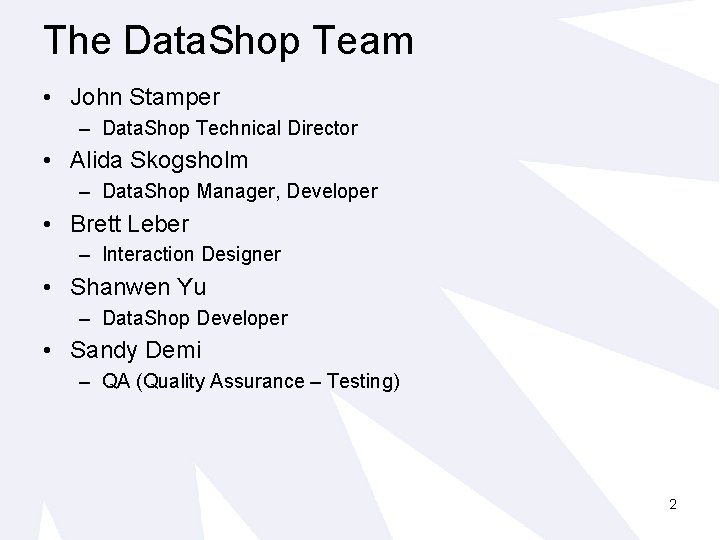
The Data. Shop Team • John Stamper – Data. Shop Technical Director • Alida Skogsholm – Data. Shop Manager, Developer • Brett Leber – Interaction Designer • Shanwen Yu – Data. Shop Developer • Sandy Demi – QA (Quality Assurance – Testing) 2
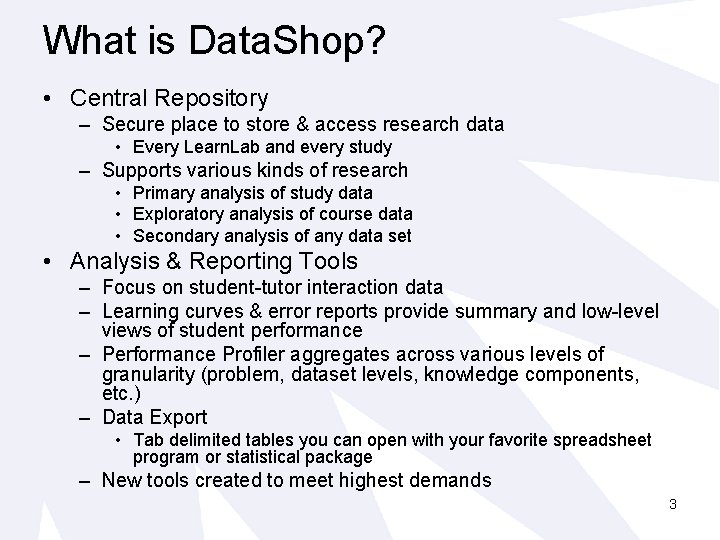
What is Data. Shop? • Central Repository – Secure place to store & access research data • Every Learn. Lab and every study – Supports various kinds of research • Primary analysis of study data • Exploratory analysis of course data • Secondary analysis of any data set • Analysis & Reporting Tools – Focus on student-tutor interaction data – Learning curves & error reports provide summary and low-level views of student performance – Performance Profiler aggregates across various levels of granularity (problem, dataset levels, knowledge components, etc. ) – Data Export • Tab delimited tables you can open with your favorite spreadsheet program or statistical package – New tools created to meet highest demands 3
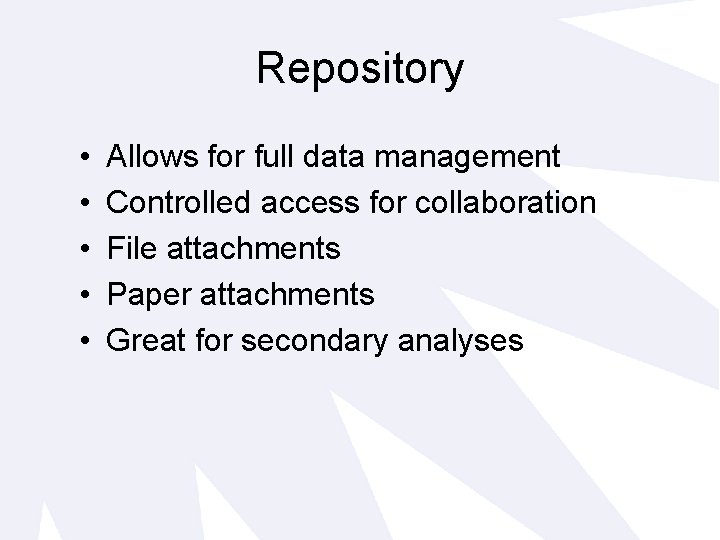
Repository • • • Allows for full data management Controlled access for collaboration File attachments Paper attachments Great for secondary analyses
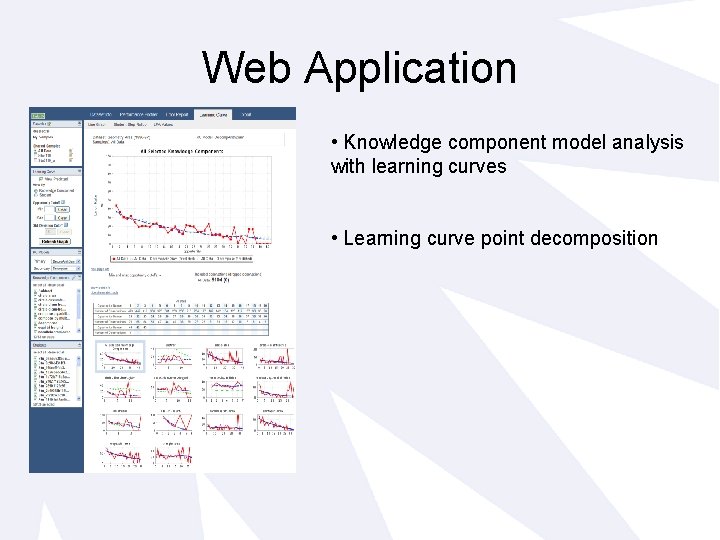
Web Application • Knowledge component model analysis with learning curves • Learning curve point decomposition
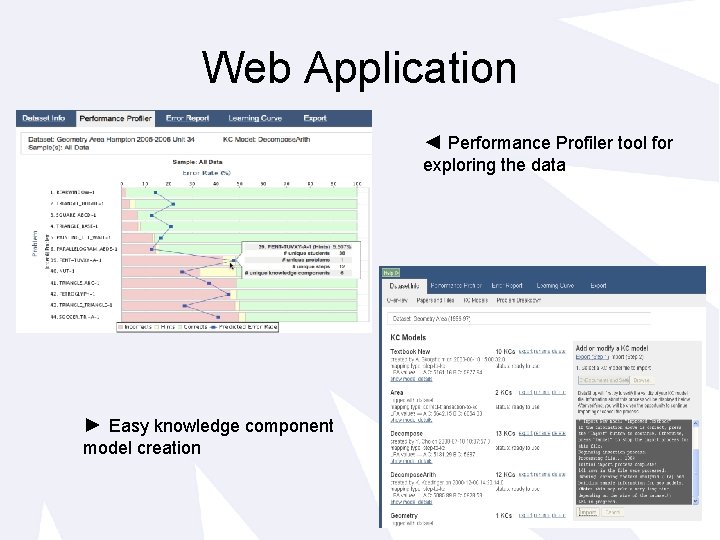
Web Application ◄ Performance Profiler tool for exploring the data ► Easy knowledge component model creation
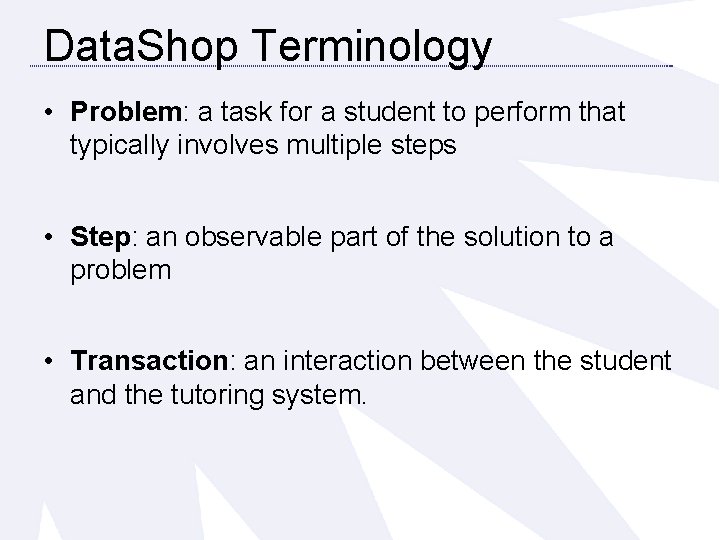
Data. Shop Terminology • Problem: a task for a student to perform that typically involves multiple steps • Step: an observable part of the solution to a problem • Transaction: an interaction between the student and the tutoring system.
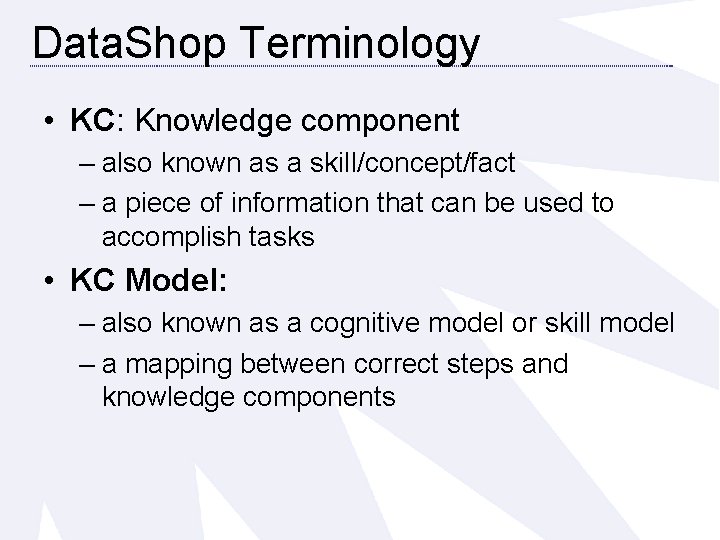
Data. Shop Terminology • KC: Knowledge component – also known as a skill/concept/fact – a piece of information that can be used to accomplish tasks • KC Model: – also known as a cognitive model or skill model – a mapping between correct steps and knowledge components
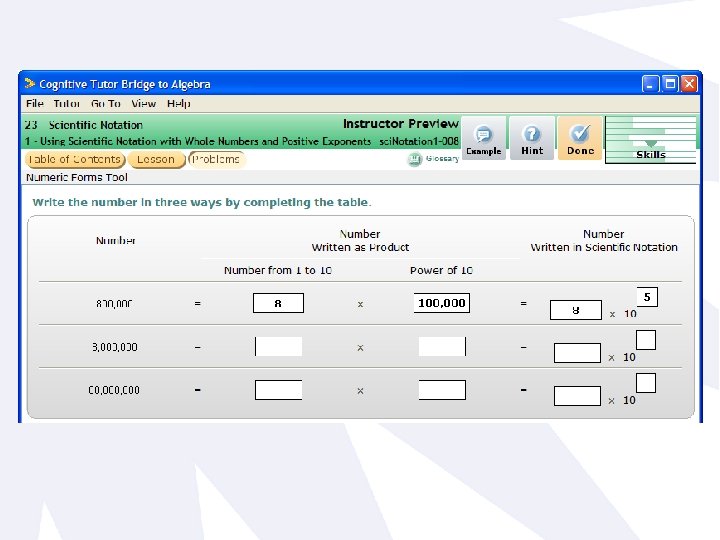
Base 1 6 Base 2 Base 3 Expanded. Power 1 100, 000 Expanded. Power 2 Expanded. Power 3 Exponent 1 8 Exponent 2 Exponent 3 General. Help. Goal. N ode Multiplier 1 6 Multiplier 2 Multiplier 3
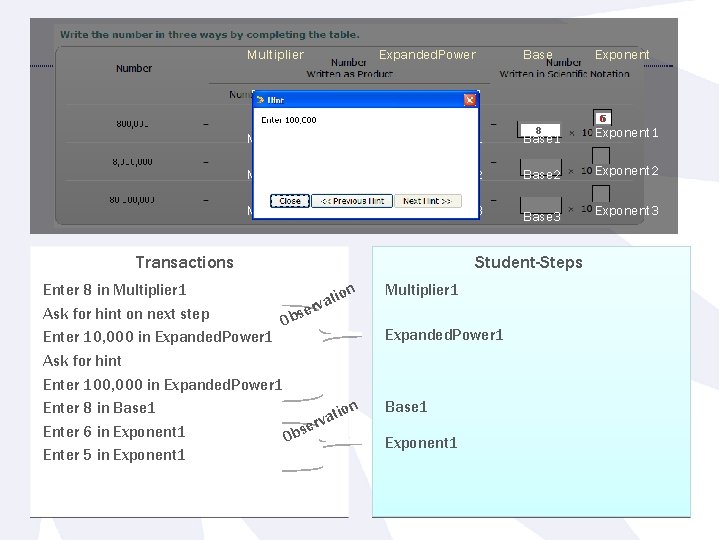
Multiplier 8 Expanded. Power 100, 000 10, 000 Base 8 Exponent 6 5 Multiplier 1 Expanded. Power 1 Base 1 Exponent 1 Multiplier 2 Expanded. Power 2 Base 2 Exponent 2 Multiplier 3 Expanded. Power 3 Base 3 Exponent 3 Transactions Enter 8 in Multiplier 1 ion t a v Ask for hint on next step ser b O Enter 10, 000 in Expanded. Power 1 Ask for hint Enter 100, 000 in Expanded. Power 1 Enter 8 in Base 1 ion t a v ser Enter 6 in Exponent 1 b O Enter 5 in Exponent 1 Student-Steps Multiplier 1 Expanded. Power 1 Base 1 Exponent 1
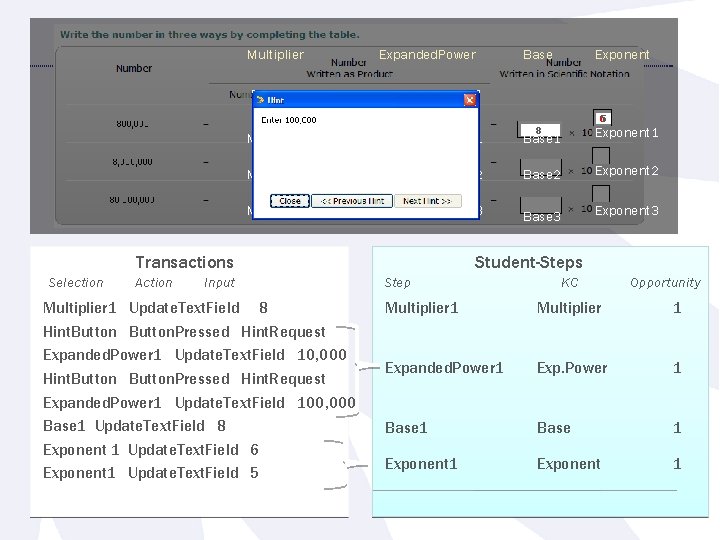
Multiplier 8 Expanded. Power 100, 000 10, 000 Action Exponent 6 5 8 Multiplier 1 Expanded. Power 1 Base 1 Exponent 1 Multiplier 2 Expanded. Power 2 Base 2 Exponent 2 Multiplier 3 Expanded. Power 3 Base 3 Exponent 3 Transactions Selection Base Input Multiplier 1 Update. Text. Field 8 Hint. Button. Pressed Hint. Request Expanded. Power 1 Update. Text. Field 10, 000 Hint. Button. Pressed Hint. Request Expanded. Power 1 Update. Text. Field 100, 000 Base 1 Update. Text. Field 8 Exponent 1 Update. Text. Field 6 Exponent 1 Update. Text. Field 5 Student-Steps Step KC Opportunity Multiplier 1 Expanded. Power 1 Exp. Power 1 Base 1 Exponent 1
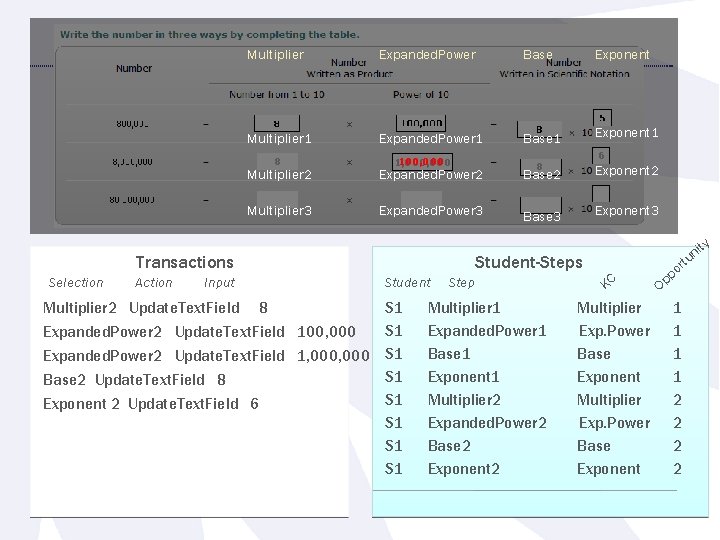
Multiplier Expanded. Power Base Exponent Multiplier 1 Expanded. Power 1 Base 1 Exponent 1 8 100, 000 1, 000 Multiplier 2 Expanded. Power 2 Base 2 Exponent 2 Multiplier 3 Expanded. Power 3 Base 3 Exponent 3 Transactions Selection Action 6 8 Input Multiplier 2 Update. Text. Field 8 Expanded. Power 2 Update. Text. Field 100, 000 Expanded. Power 2 Update. Text. Field 1, 000 Base 2 Update. Text. Field 8 Exponent 2 Update. Text. Field 6 Student-Steps Student S 1 S 1 Step Multiplier 1 Expanded. Power 1 Base 1 Exponent 1 Multiplier 2 Expanded. Power 2 Base 2 Exponent 2 ty KC Multiplier Exp. Power Base Exponent ni tr u o p Op 1 1 2 2
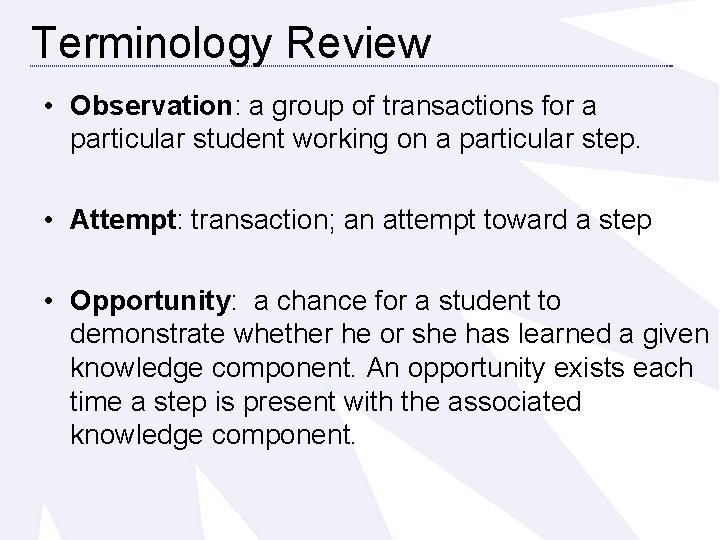
Terminology Review • Observation: a group of transactions for a particular student working on a particular step. • Attempt: transaction; an attempt toward a step • Opportunity: a chance for a student to demonstrate whether he or she has learned a given knowledge component. An opportunity exists each time a step is present with the associated knowledge component.
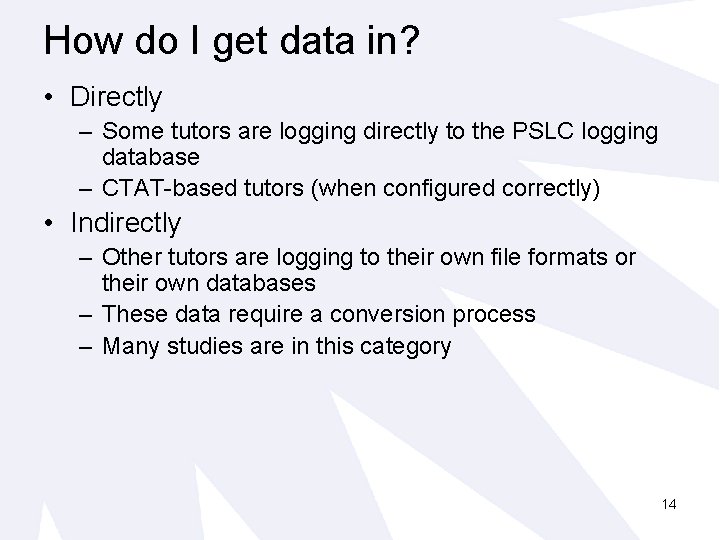
How do I get data in? • Directly – Some tutors are logging directly to the PSLC logging database – CTAT-based tutors (when configured correctly) • Indirectly – Other tutors are logging to their own file formats or their own databases – These data require a conversion process – Many studies are in this category 14
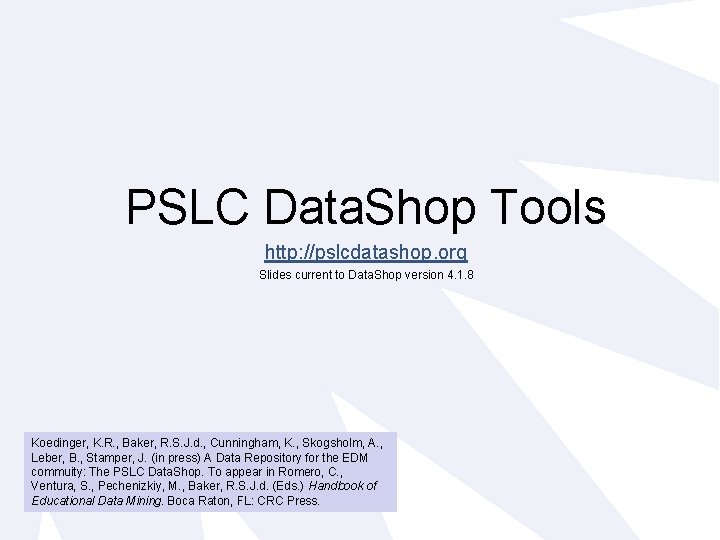
PSLC Data. Shop Tools http: //pslcdatashop. org Slides current to Data. Shop version 4. 1. 8 Koedinger, K. R. , Baker, R. S. J. d. , Cunningham, K. , Skogsholm, A. , Leber, B. , Stamper, J. (in press) A Data Repository for the EDM commuity: The PSLC Data. Shop. To appear in Romero, C. , Ventura, S. , Pechenizkiy, M. , Baker, R. S. J. d. (Eds. ) Handbook of Educational Data Mining. Boca Raton, FL: CRC Press.
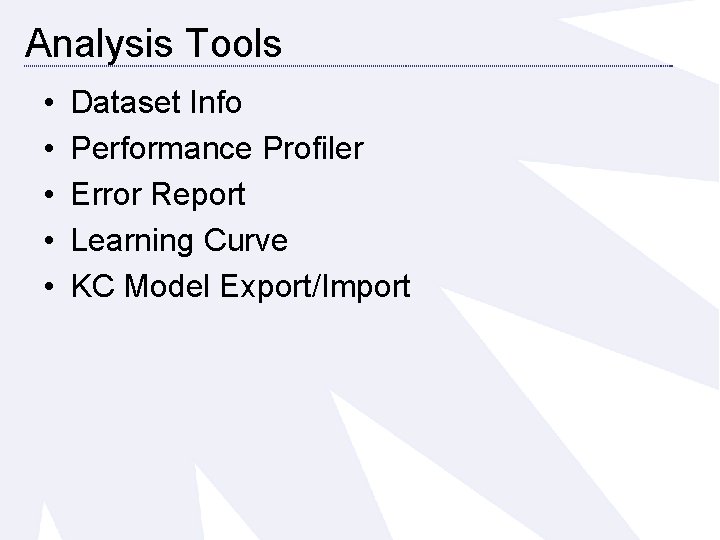
Analysis Tools • • • Dataset Info Performance Profiler Error Report Learning Curve KC Model Export/Import
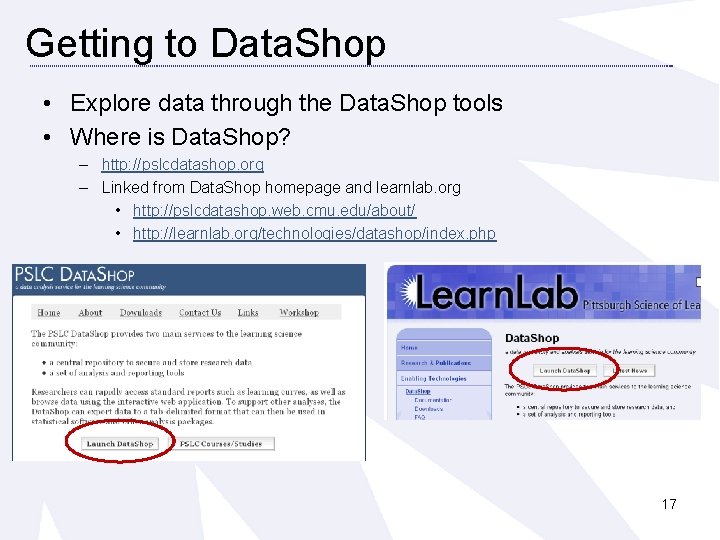
Getting to Data. Shop • Explore data through the Data. Shop tools • Where is Data. Shop? – http: //pslcdatashop. org – Linked from Data. Shop homepage and learnlab. org • http: //pslcdatashop. web. cmu. edu/about/ • http: //learnlab. org/technologies/datashop/index. php 17
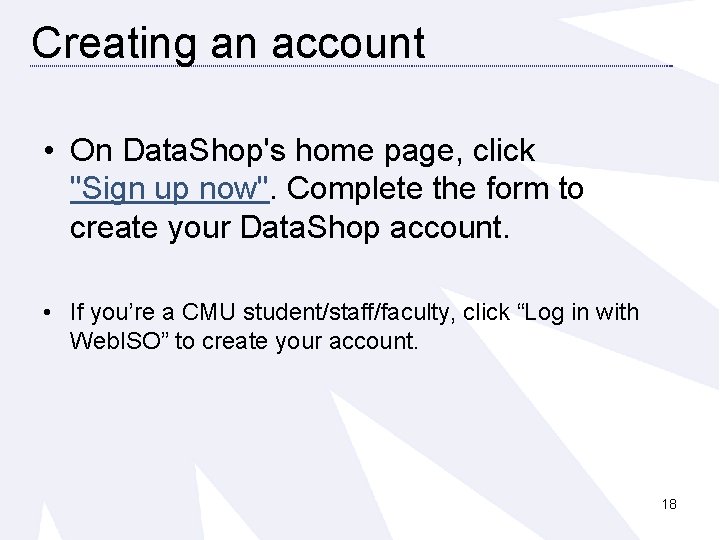
Creating an account • On Data. Shop's home page, click "Sign up now". Complete the form to create your Data. Shop account. • If you’re a CMU student/staff/faculty, click “Log in with Web. ISO” to create your account. 18
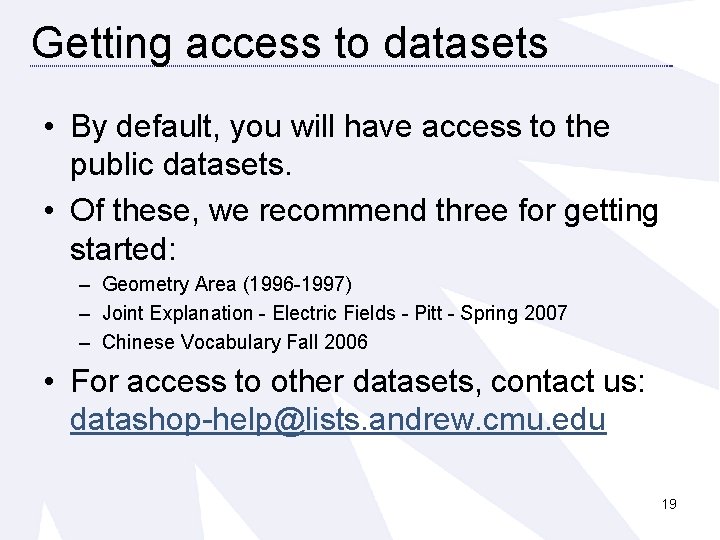
Getting access to datasets • By default, you will have access to the public datasets. • Of these, we recommend three for getting started: – Geometry Area (1996 -1997) – Joint Explanation - Electric Fields - Pitt - Spring 2007 – Chinese Vocabulary Fall 2006 • For access to other datasets, contact us: datashop-help@lists. andrew. cmu. edu 19
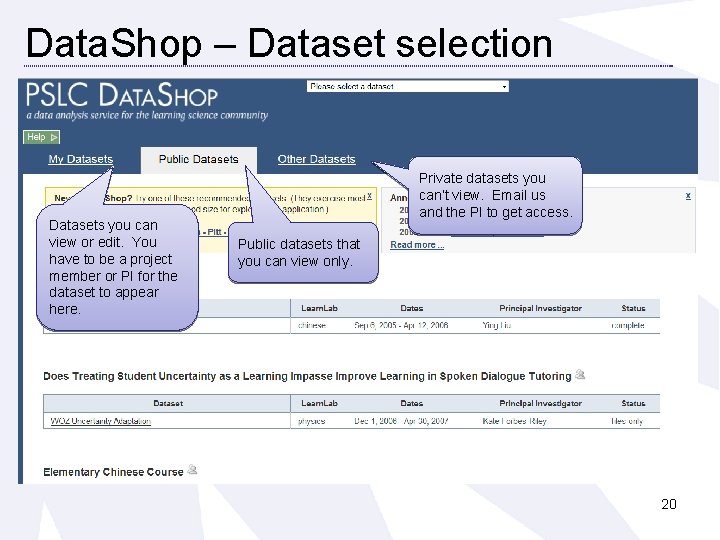
Data. Shop – Dataset selection Datasets you can view or edit. You have to be a project member or PI for the dataset to appear here. Private datasets you can’t view. Email us and the PI to get access. Public datasets that you can view only. 20
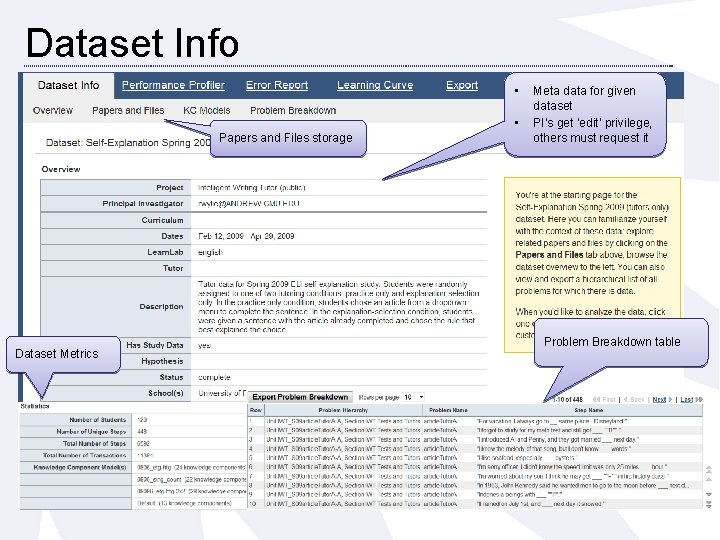
Dataset Info • • Papers and Files storage Dataset Metrics Meta data for given dataset PI’s get ‘edit’ privilege, others must request it Problem Breakdown table 21
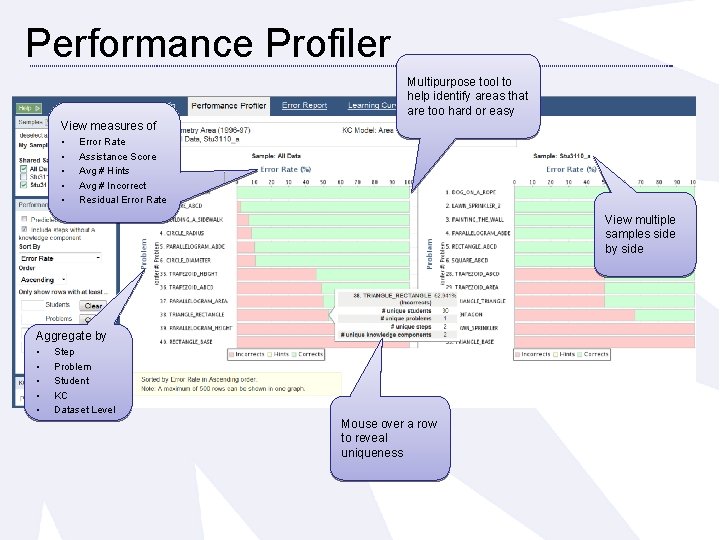
Performance Profiler Multipurpose tool to help identify areas that are too hard or easy View measures of • • • Error Rate Assistance Score Avg # Hints Avg # Incorrect Residual Error Rate View multiple samples side by side Aggregate by • • • Step Problem Student KC Dataset Level Mouse over a row to reveal uniqueness
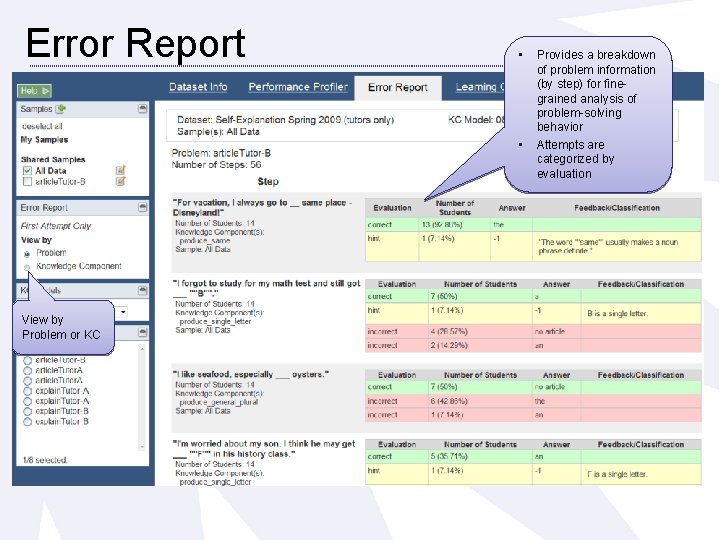
Error Report • • View by Problem or KC Provides a breakdown of problem information (by step) for finegrained analysis of problem-solving behavior Attempts are categorized by evaluation
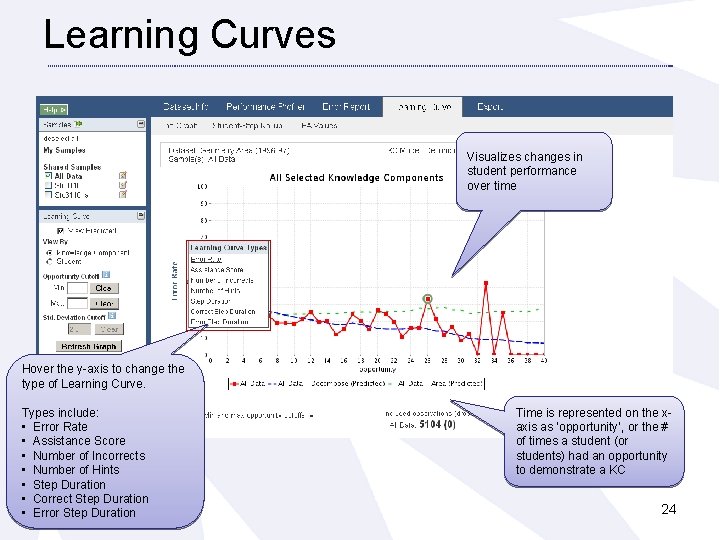
Learning Curves Visualizes changes in student performance over time Hover the y-axis to change the type of Learning Curve. Types include: • Error Rate • Assistance Score • Number of Incorrects • Number of Hints • Step Duration • Correct Step Duration • Error Step Duration Time is represented on the xaxis as ‘opportunity’, or the # of times a student (or students) had an opportunity to demonstrate a KC 24
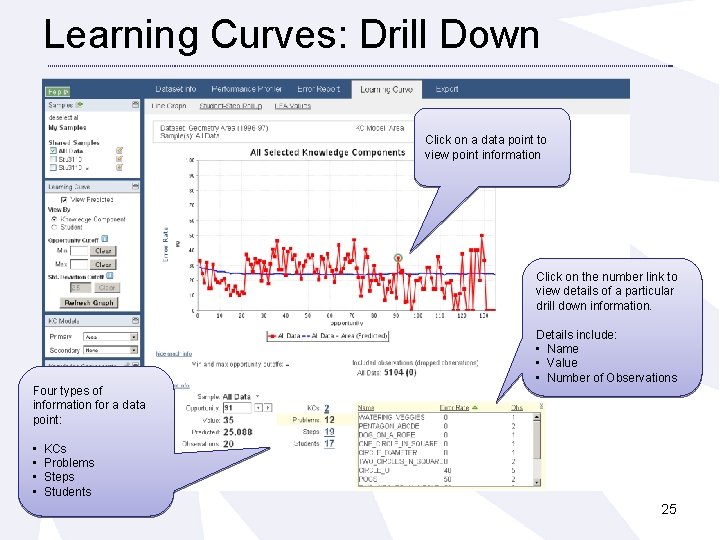
Learning Curves: Drill Down Click on a data point to view point information Click on the number link to view details of a particular drill down information. Four types of information for a data point: • • Details include: • Name • Value • Number of Observations KCs Problems Steps Students 25
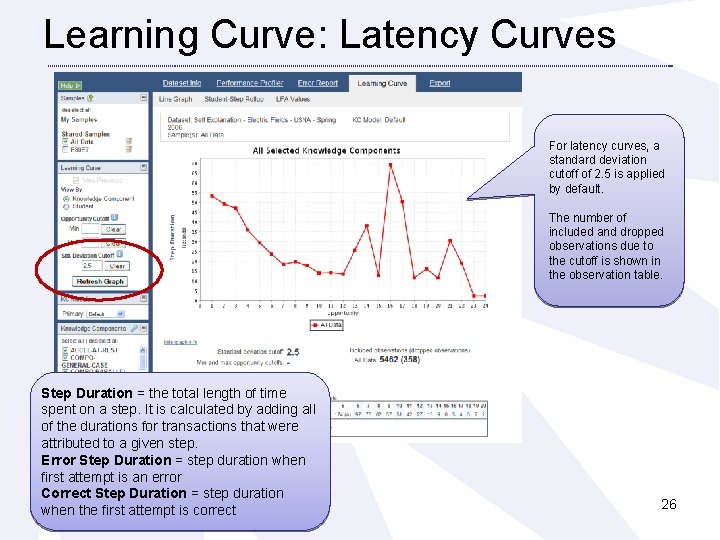
Learning Curve: Latency Curves For latency curves, a standard deviation cutoff of 2. 5 is applied by default. The number of included and dropped observations due to the cutoff is shown in the observation table. Step Duration = the total length of time spent on a step. It is calculated by adding all of the durations for transactions that were attributed to a given step. Error Step Duration = step duration when first attempt is an error Correct Step Duration = step duration when the first attempt is correct 26
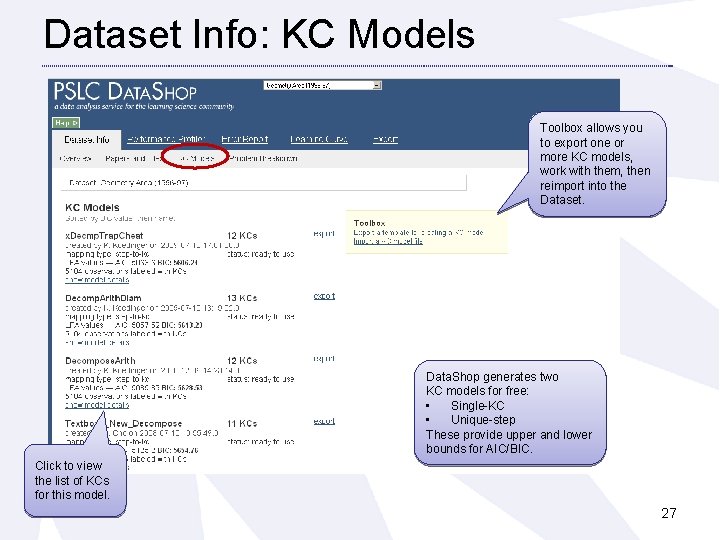
Dataset Info: KC Models Toolbox allows you to export one or more KC models, work with them, then reimport into the Dataset. Handy information displayed for each KC Model: • Name • # of KCs in the model • Created By Data. Shop generates two • Mapping Type • AIC & BIC Values KC models for free: • Single-KC • Unique-step These provide upper and lower bounds for AIC/BIC. Click to view the list of KCs for this model. 27
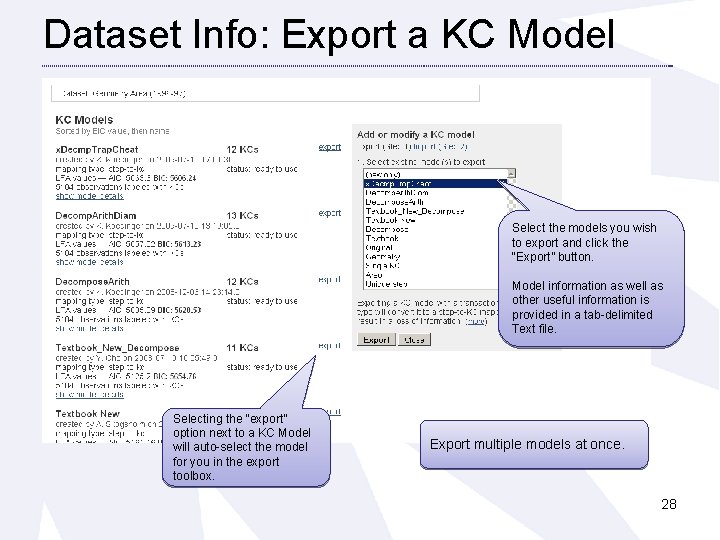
Dataset Info: Export a KC Model Select the models you wish to export and click the “Export” button. Model information as well as other useful information is provided in a tab-delimited Text file. Selecting the “export” option next to a KC Model will auto-select the model for you in the export toolbox. Export multiple models at once. 28
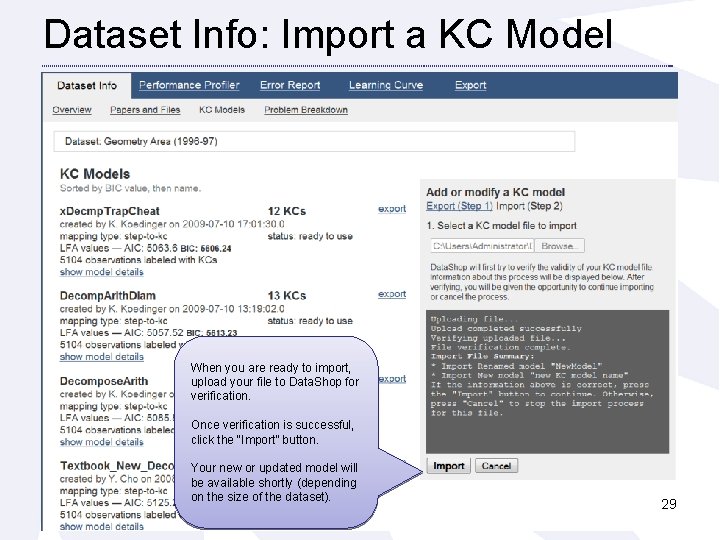
Dataset Info: Import a KC Model When you are ready to import, upload your file to Data. Shop for verification. Once verification is successful, click the “Import” button. Your new or updated model will be available shortly (depending on the size of the dataset). 29
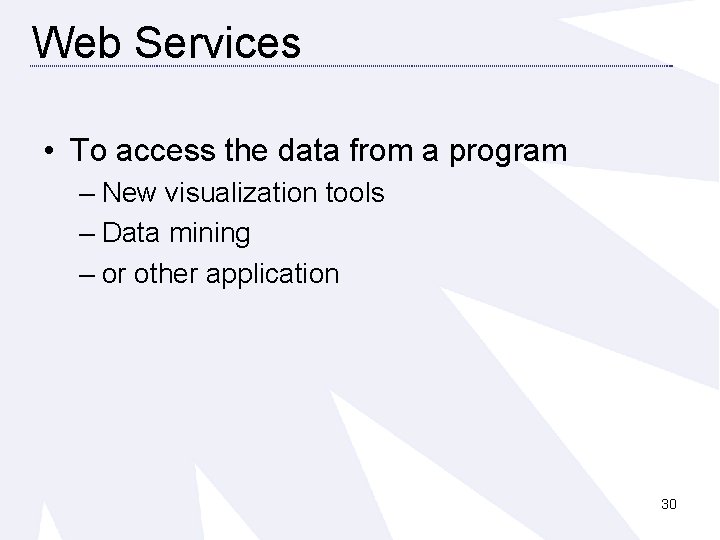
Web Services • To access the data from a program – New visualization tools – Data mining – or other application 30
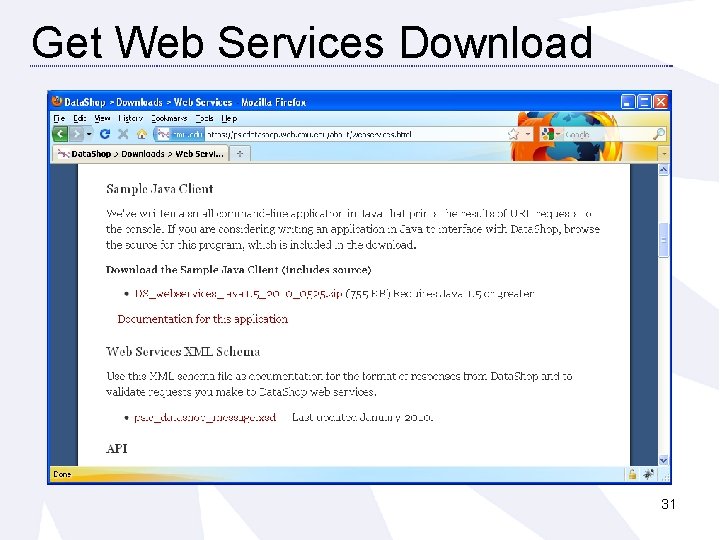
Get Web Services Download 31
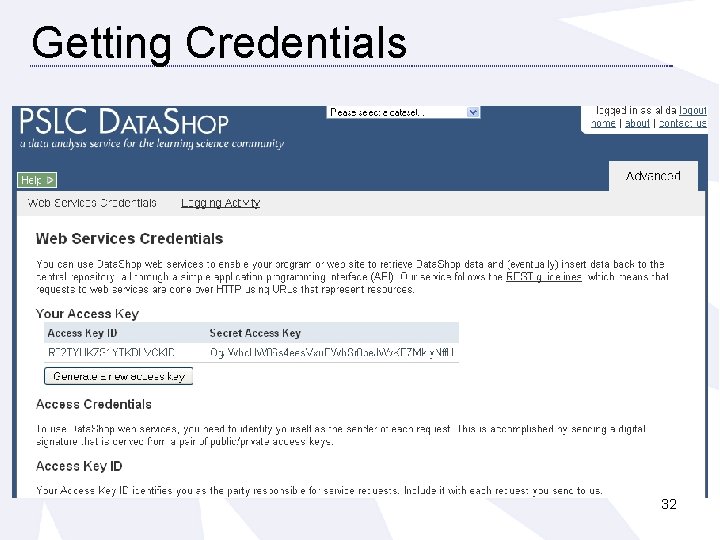
Getting Credentials 32
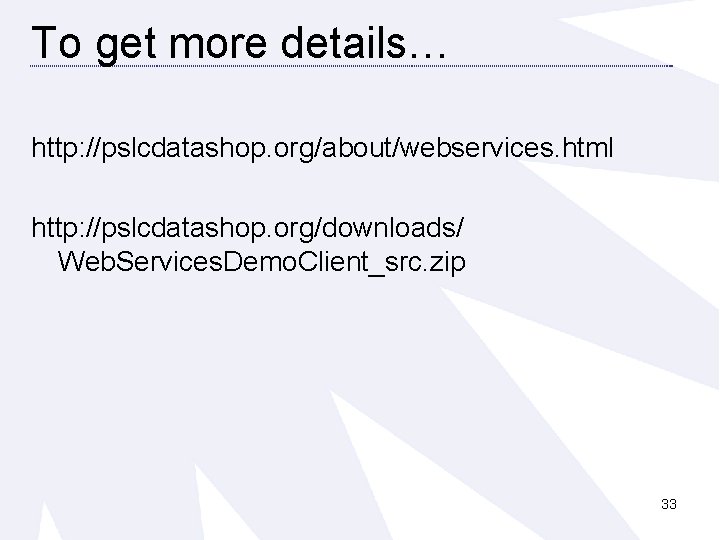
To get more details… http: //pslcdatashop. org/about/webservices. html http: //pslcdatashop. org/downloads/ Web. Services. Demo. Client_src. zip 33
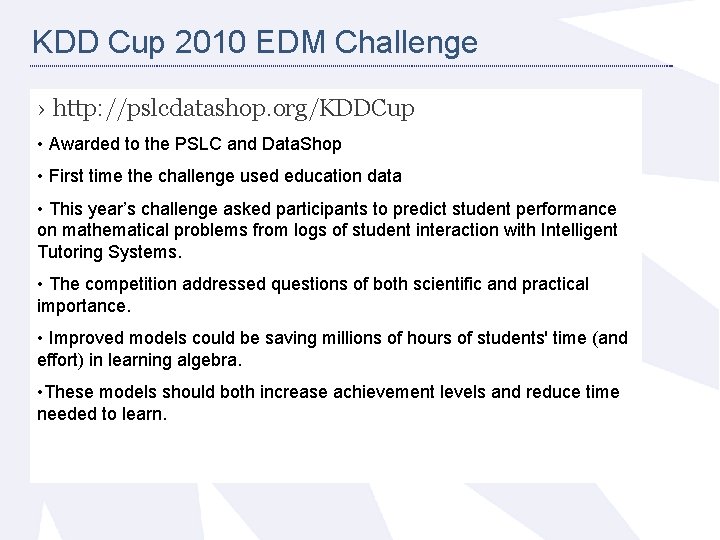
KDD Cup 2010 EDM Challenge › http: //pslcdatashop. org/KDDCup • Awarded to the PSLC and Data. Shop • First time the challenge used education data • This year’s challenge asked participants to predict student performance on mathematical problems from logs of student interaction with Intelligent Tutoring Systems. • The competition addressed questions of both scientific and practical importance. • Improved models could be saving millions of hours of students' time (and effort) in learning algebra. • These models should both increase achievement levels and reduce time needed to learn.
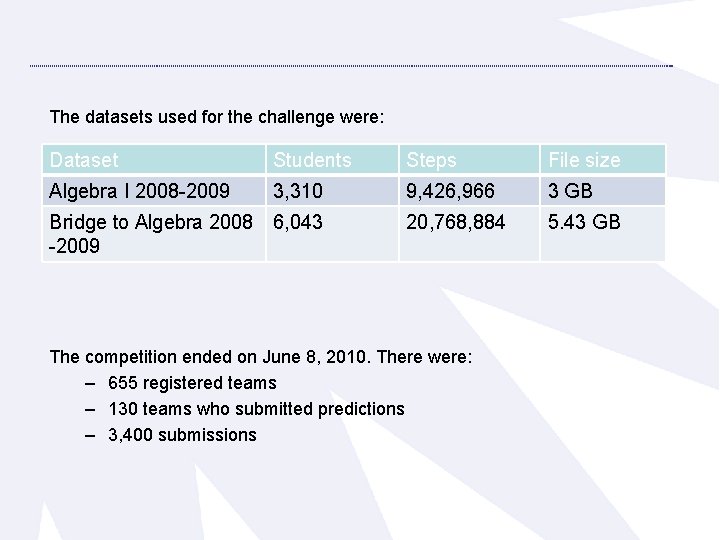
The datasets used for the challenge were: Dataset Students Steps File size Algebra I 2008 -2009 3, 310 9, 426, 966 3 GB 20, 768, 884 5. 43 GB Bridge to Algebra 2008 6, 043 -2009 The competition ended on June 8, 2010. There were: – 655 registered teams – 130 teams who submitted predictions – 3, 400 submissions
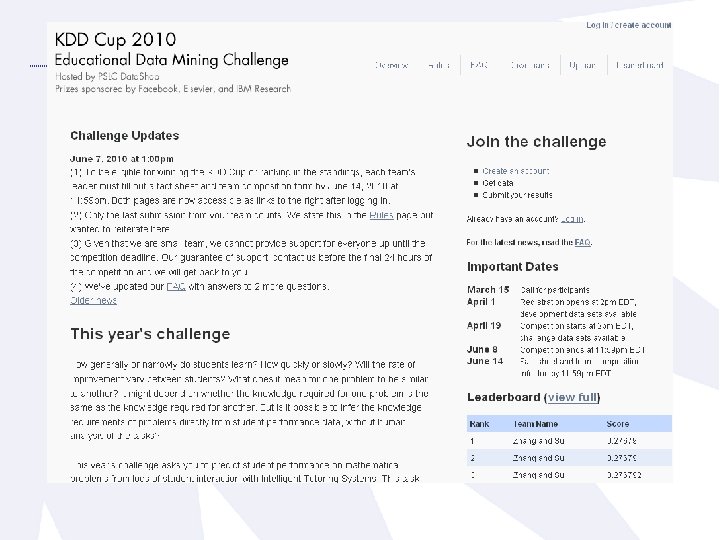
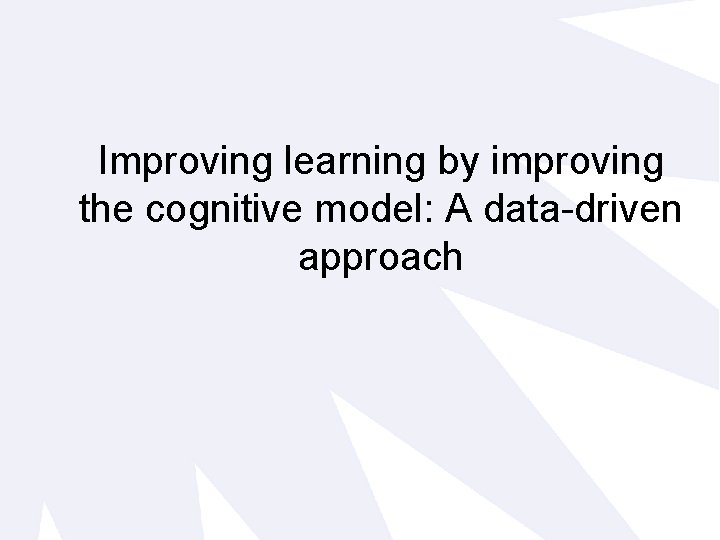
Improving learning by improving the cognitive model: A data-driven approach
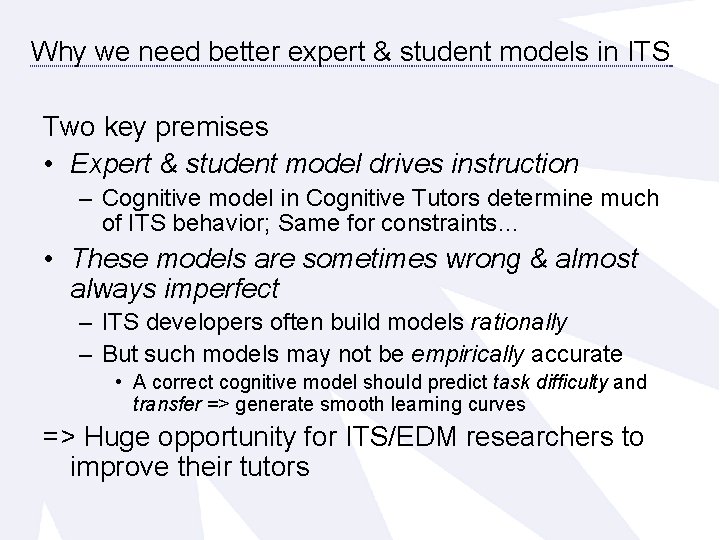
Why we need better expert & student models in ITS Two key premises • Expert & student model drives instruction – Cognitive model in Cognitive Tutors determine much of ITS behavior; Same for constraints… • These models are sometimes wrong & almost always imperfect – ITS developers often build models rationally – But such models may not be empirically accurate • A correct cognitive model should predict task difficulty and transfer => generate smooth learning curves => Huge opportunity for ITS/EDM researchers to improve their tutors
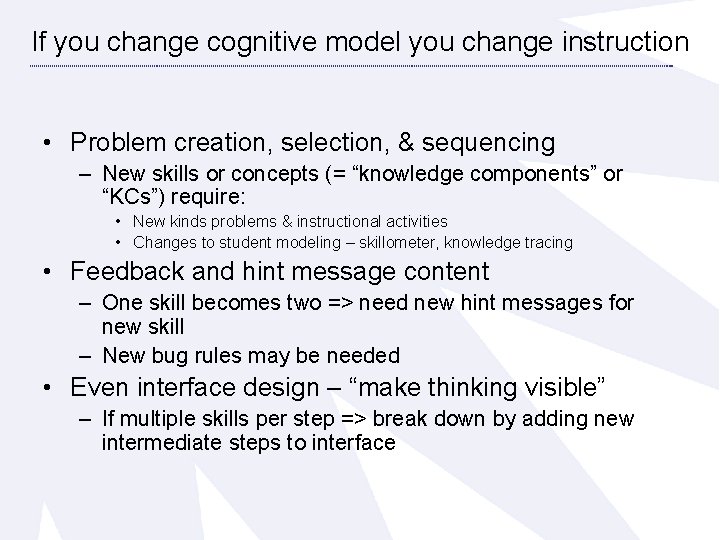
If you change cognitive model you change instruction • Problem creation, selection, & sequencing – New skills or concepts (= “knowledge components” or “KCs”) require: • New kinds problems & instructional activities • Changes to student modeling – skillometer, knowledge tracing • Feedback and hint message content – One skill becomes two => need new hint messages for new skill – New bug rules may be needed • Even interface design – “make thinking visible” – If multiple skills per step => break down by adding new intermediate steps to interface
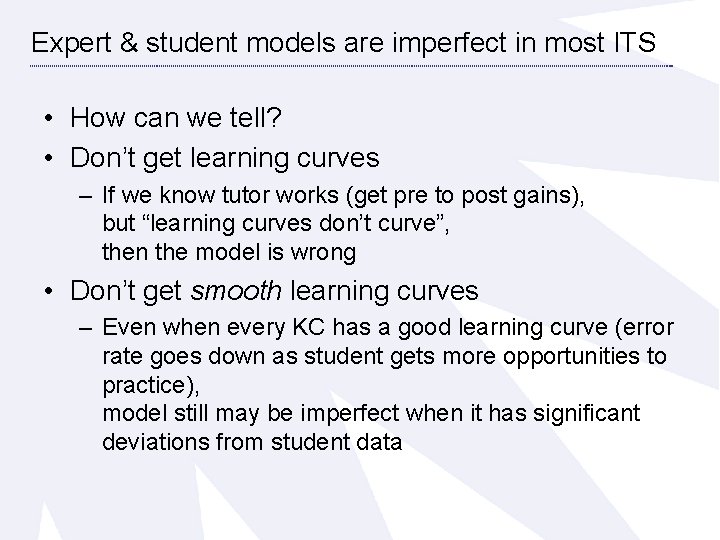
Expert & student models are imperfect in most ITS • How can we tell? • Don’t get learning curves – If we know tutor works (get pre to post gains), but “learning curves don’t curve”, then the model is wrong • Don’t get smooth learning curves – Even when every KC has a good learning curve (error rate goes down as student gets more opportunities to practice), model still may be imperfect when it has significant deviations from student data
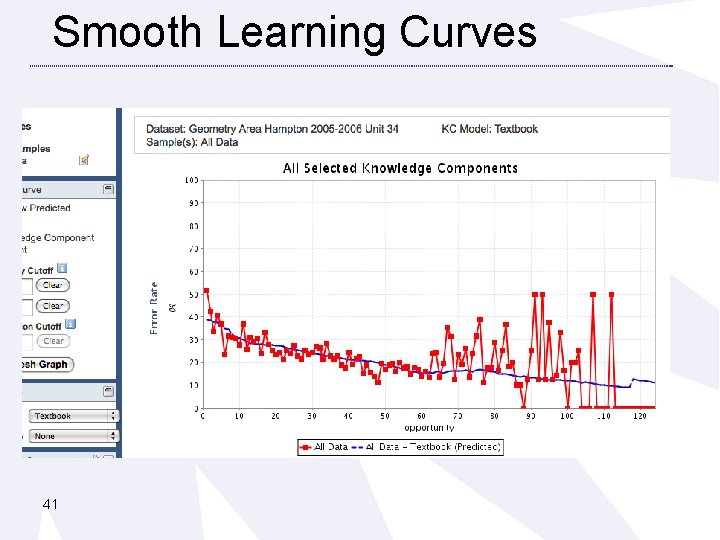
Smooth Learning Curves 41
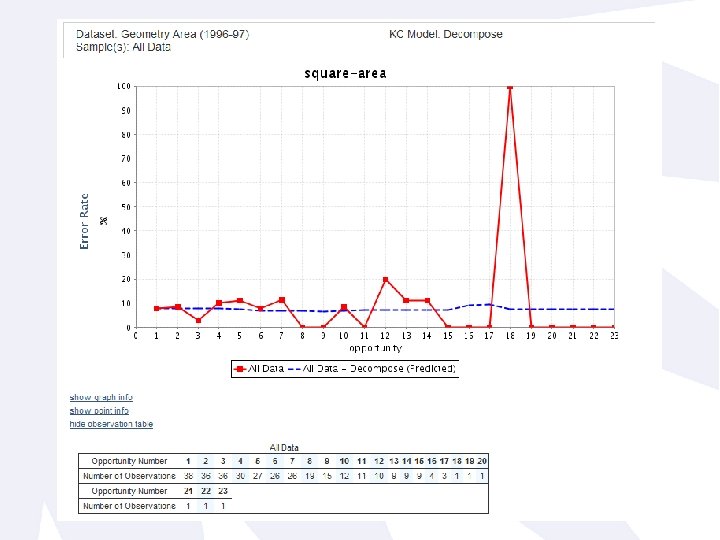
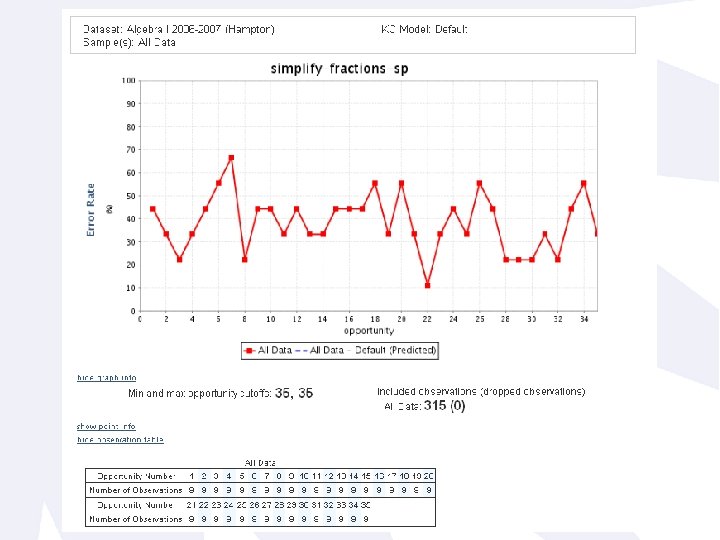
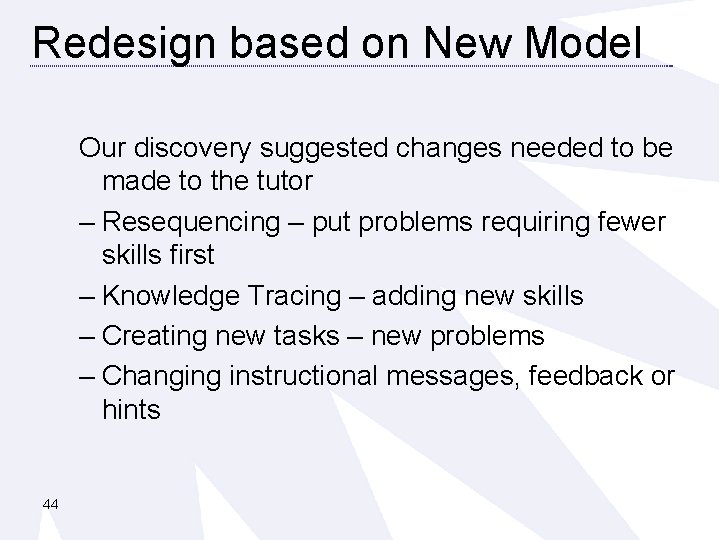
Redesign based on New Model Our discovery suggested changes needed to be made to the tutor – Resequencing – put problems requiring fewer skills first – Knowledge Tracing – adding new skills – Creating new tasks – new problems – Changing instructional messages, feedback or hints 44
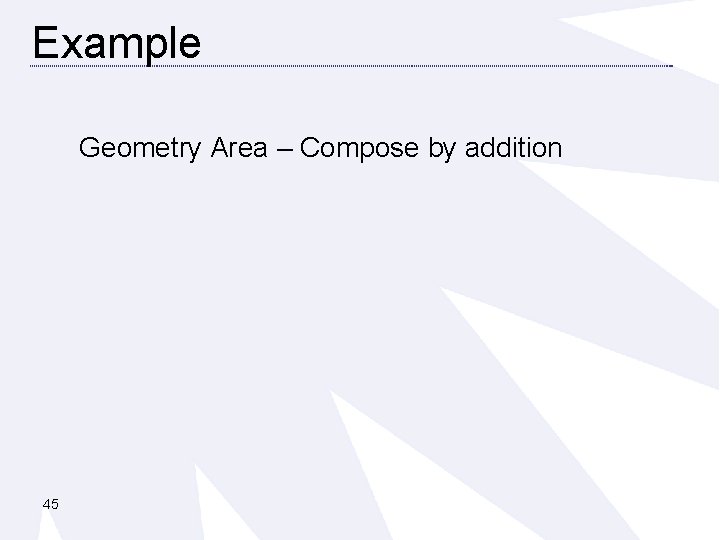
Example Geometry Area – Compose by addition 45
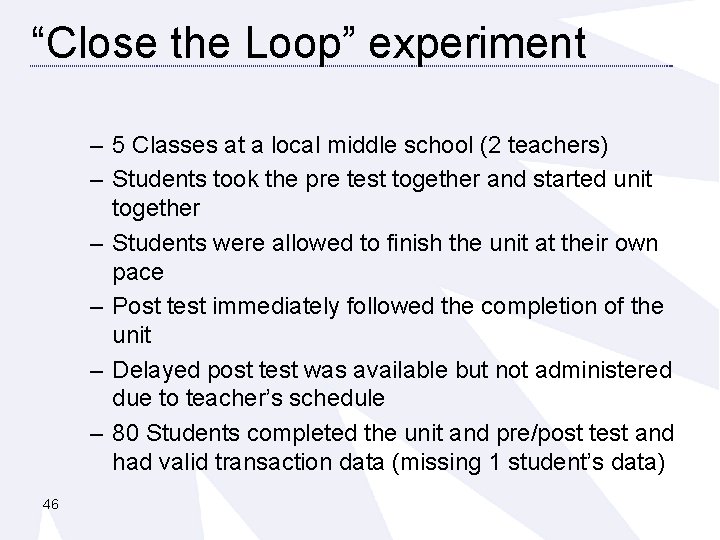
“Close the Loop” experiment – 5 Classes at a local middle school (2 teachers) – Students took the pre test together and started unit together – Students were allowed to finish the unit at their own pace – Post test immediately followed the completion of the unit – Delayed post test was available but not administered due to teacher’s schedule – 80 Students completed the unit and pre/post test and had valid transaction data (missing 1 student’s data) 46
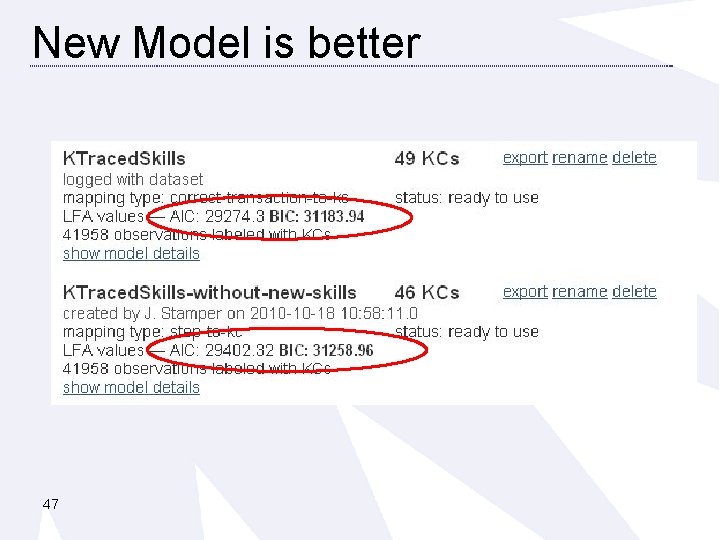
New Model is better 47
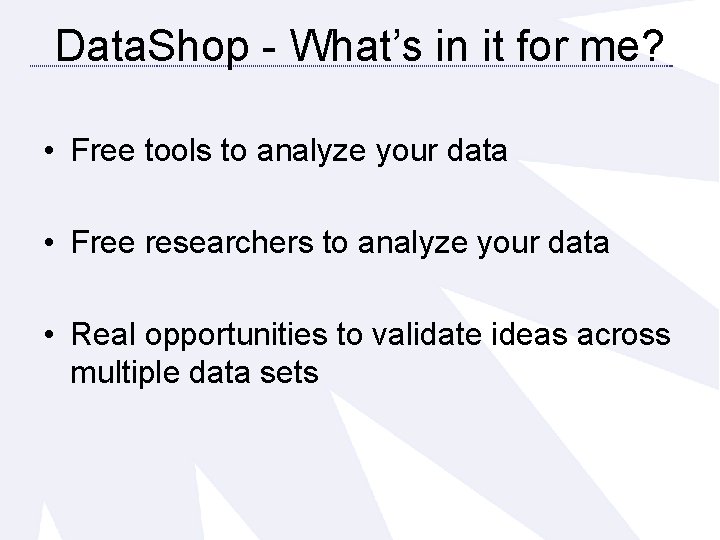
Data. Shop - What’s in it for me? • Free tools to analyze your data • Free researchers to analyze your data • Real opportunities to validate ideas across multiple data sets
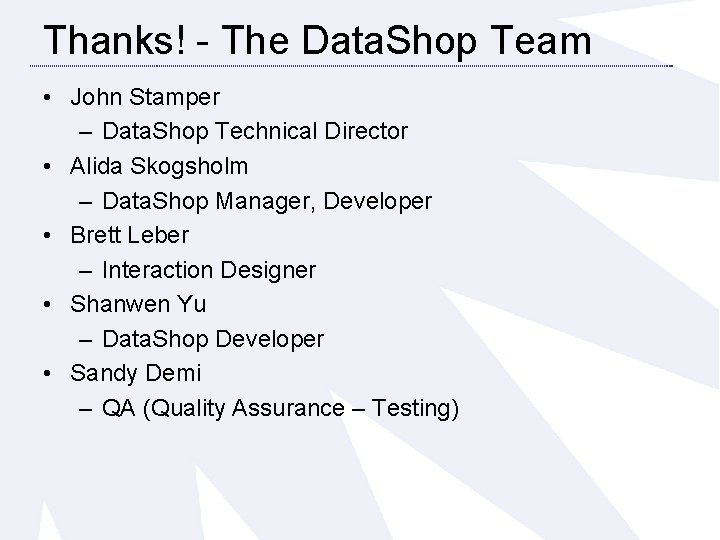
Thanks! - The Data. Shop Team • John Stamper – Data. Shop Technical Director • Alida Skogsholm – Data. Shop Manager, Developer • Brett Leber – Interaction Designer • Shanwen Yu – Data. Shop Developer • Sandy Demi – QA (Quality Assurance – Testing)
Pslc datashop
Job shop process layout
A small child slides down the four frictionless slides
A crane lowers a girder into place
Http://hdr.undp.org/en/data
Http //mbs.meb.gov.tr/ http //www.alantercihleri.com
Siat ung sistem informasi akademik
Machine learning lecture
Introduction to machine learning slides
Introduction to algorithms 강의
Introduction to machine learning slides
Http://www.blueplanetbiomes.org/world_biomes.htm
Http//oh.portal.airast.org
Http://criterion.ets.org
Icas score levels
Http://www.pbs.org/ktca/liberty/
Http://mbgnet.mobot.org/
Official smarter balanced summative assessment
Http //pisa.ets.org/school questionnaire
Www.python.org /download
Http://www.finaid.org/calculators/loanpayments.phtml
Education planner learning styles
Www.python.com
Http //education.jlab.org/qa/pen number.html
Http://hdr.undp.org/en/countries
Http://fsassessments.org/
Http://library.thinkquest.org
Http://www.blueplanetbiomes.org/world_biomes.htm
A long walk to water summary
Interesting facts about venus
Http://www.nineplanets.org
Http://www.nineplanets.org
Http://nineplanets.org/
Quiero ser bombera juntos seconde
Http://insidechnsf.chnsf.org/
Http://www.piday.org/million/
Http//student portal logisys.org
Www.dop.gov.mm http //myanmar.unfpa.org/census
Https://playground.tensorflow.org/
Http://www.stopdisastersgame.org/
Https://kidshealth.org/en/kids/digestive-system.html
Http//www.python.org
What are the different types of tundra biomes
World wide science
Temperate forest plants adaptations
Thymeleaf layout
Eva.depp.taocloud
Http //www.python.org/
Http://www.springframework.org/schema/p
Python org