Optimal SSFP PulseSequence Design for Tissue Density Estimation
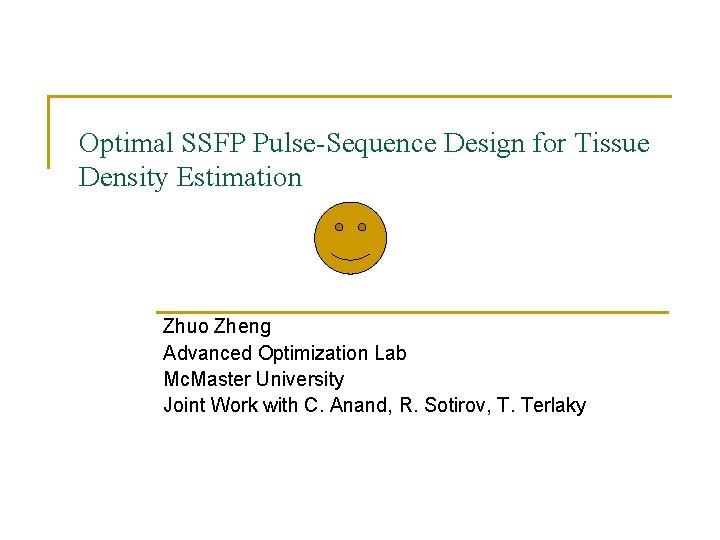
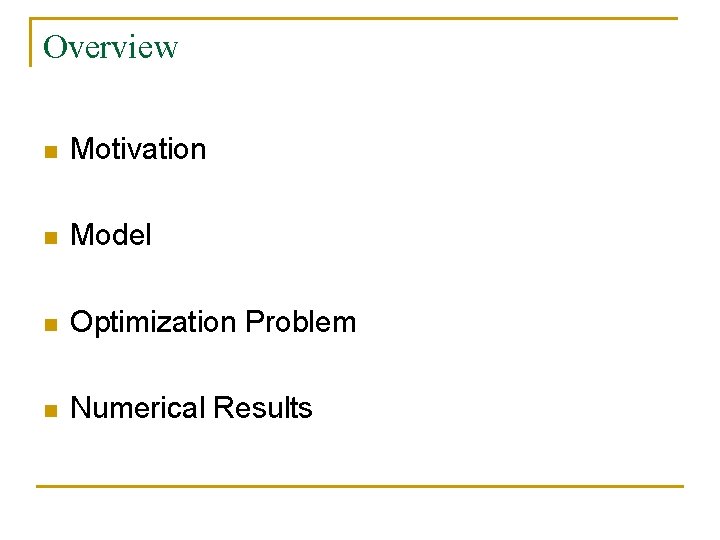
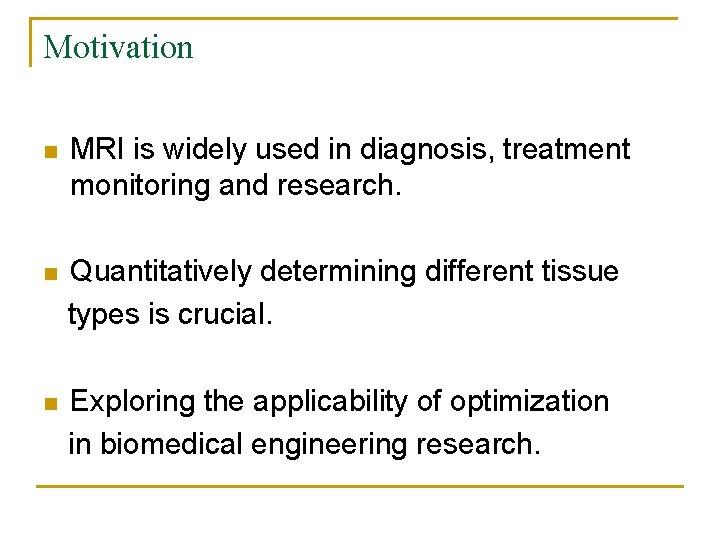
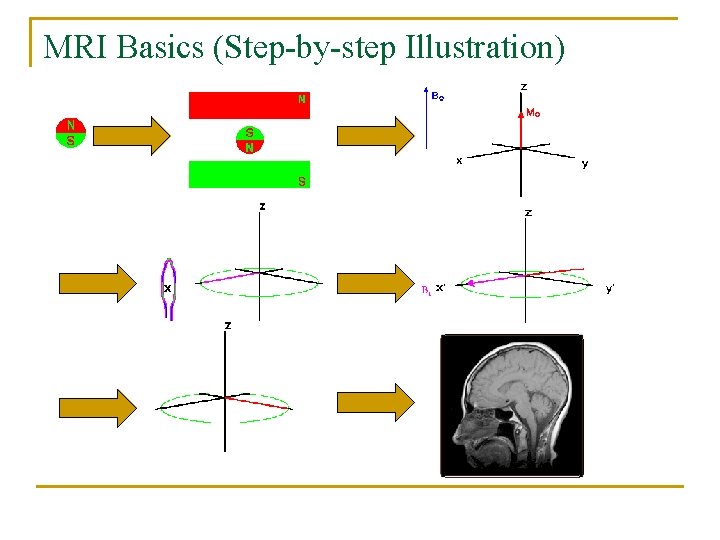
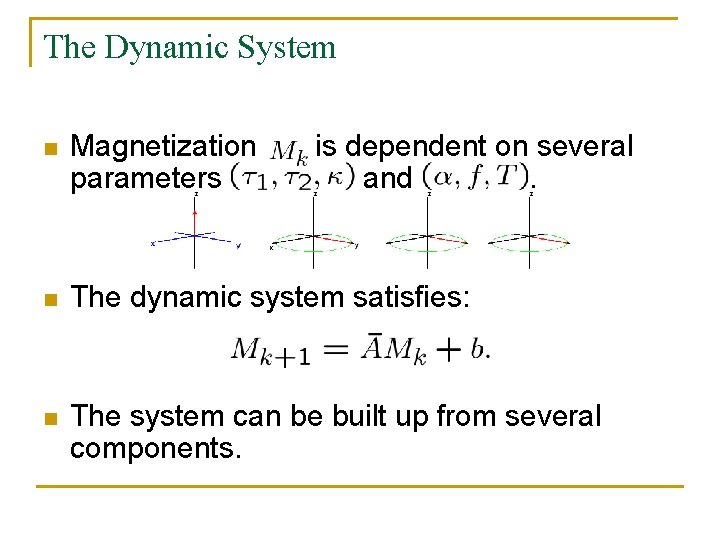
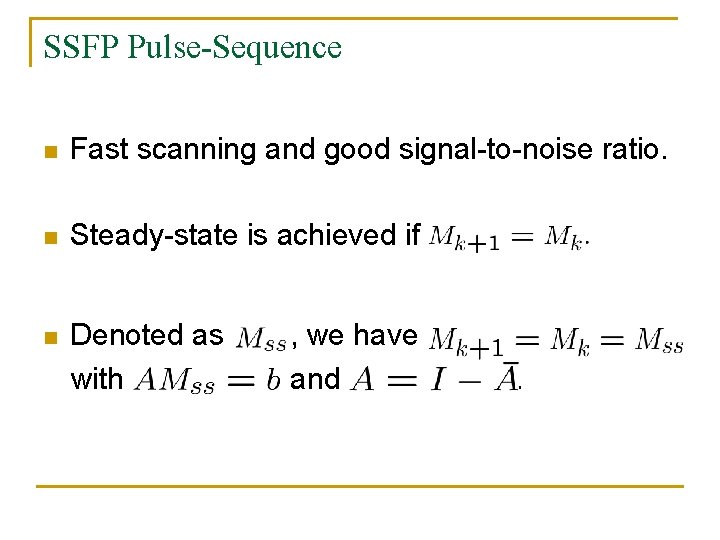
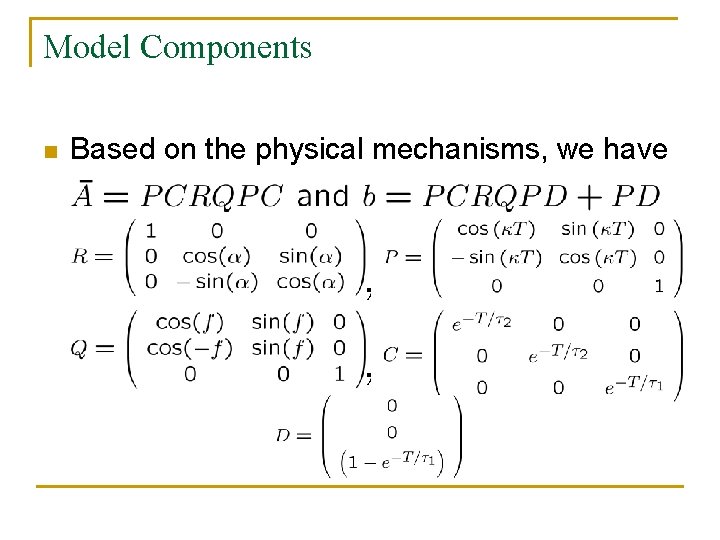
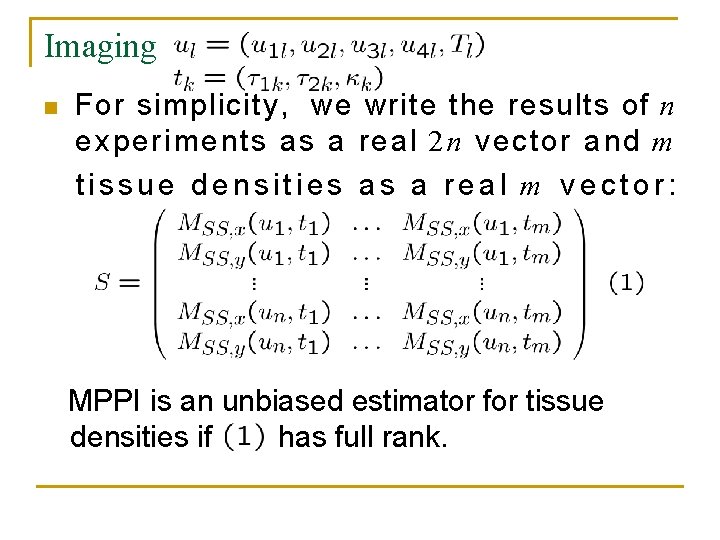
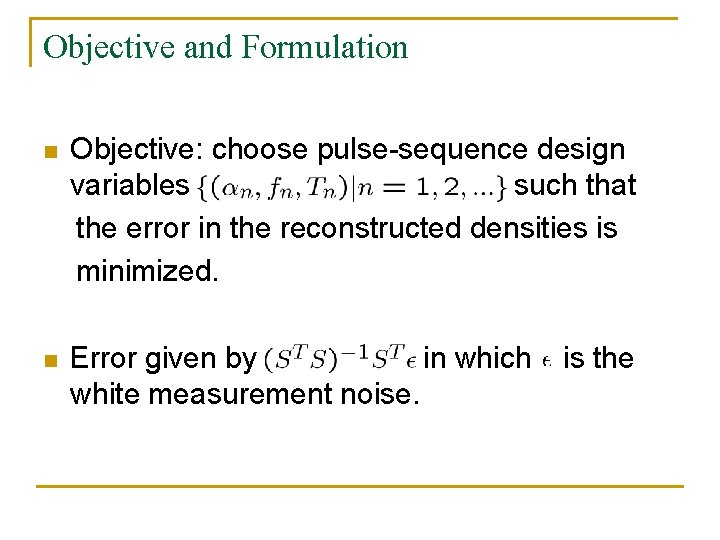
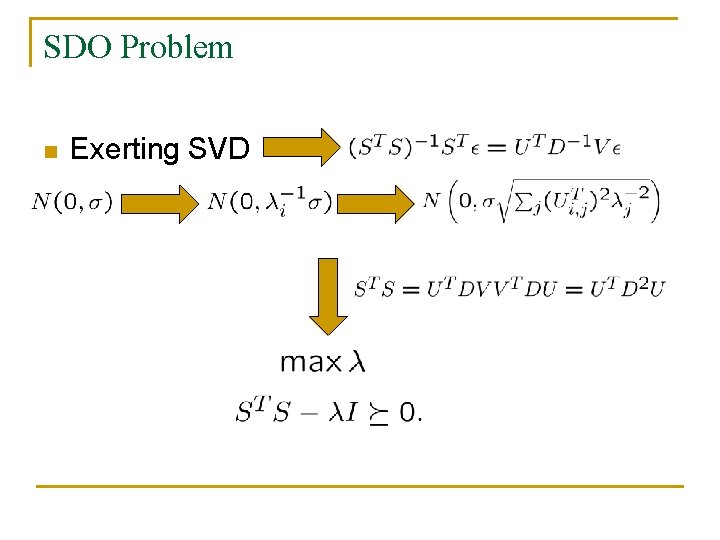
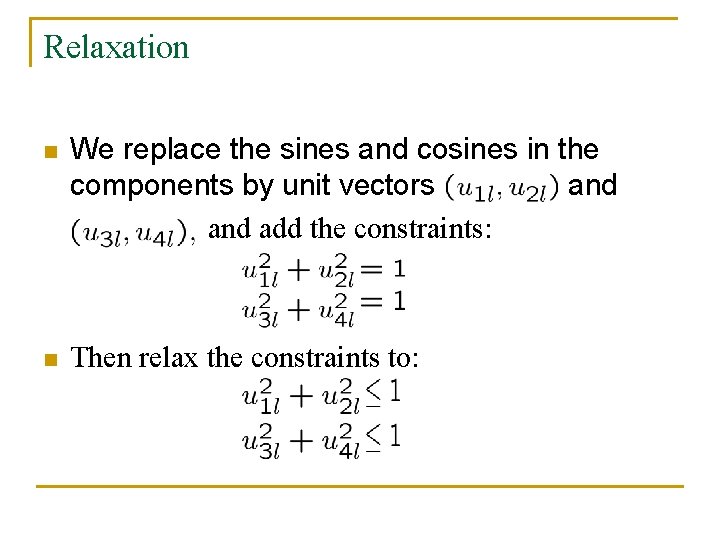
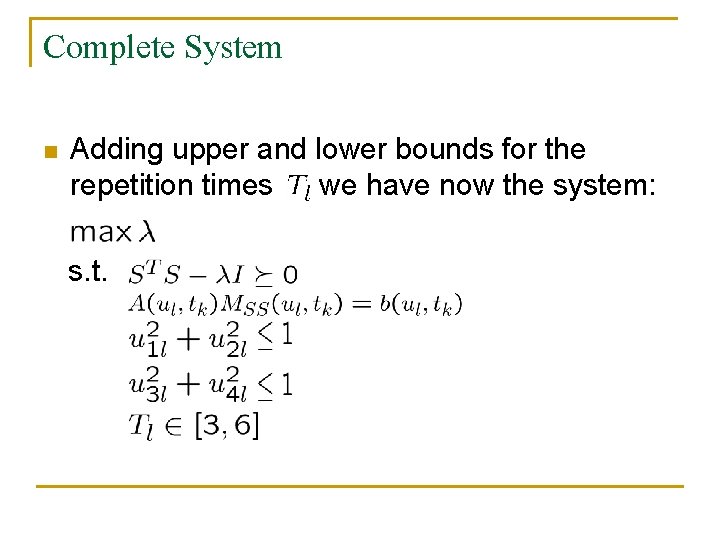
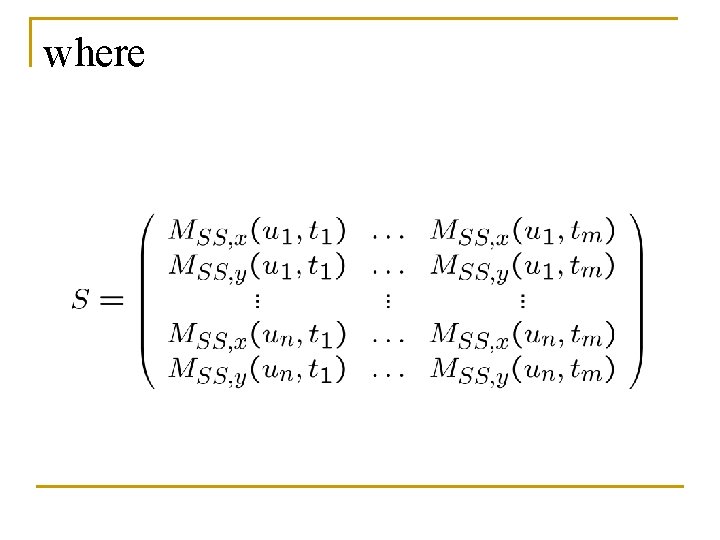
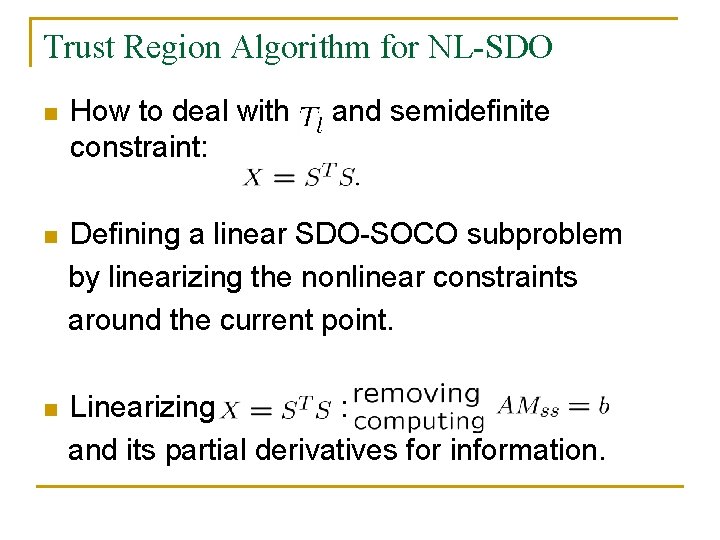
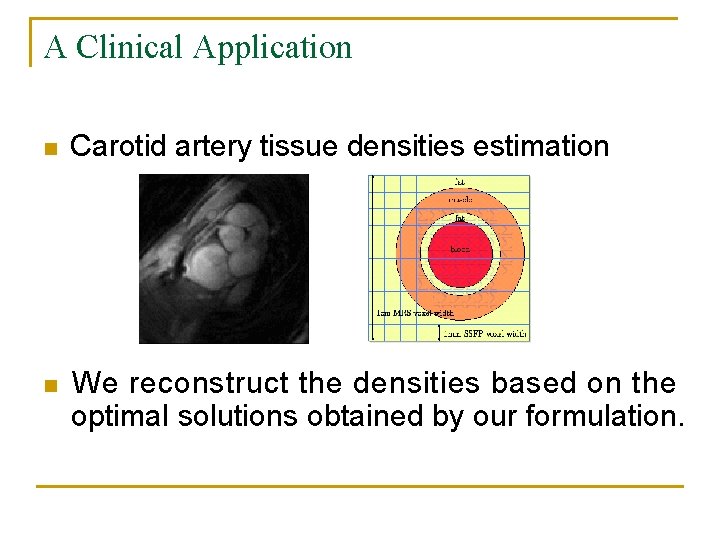
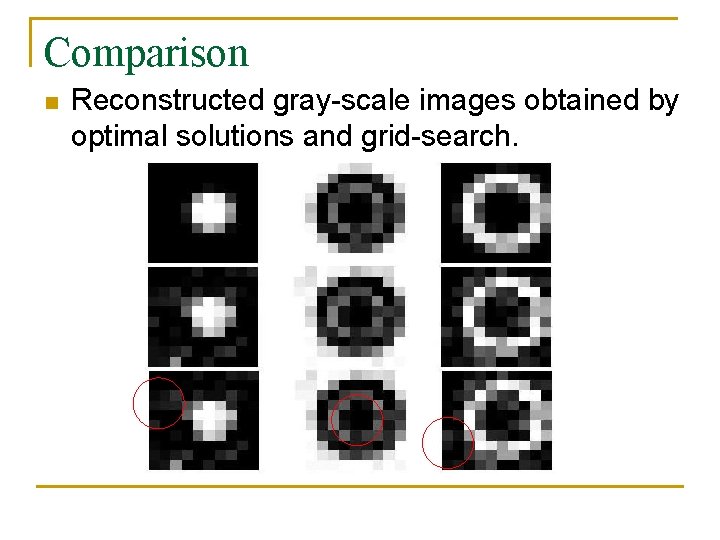
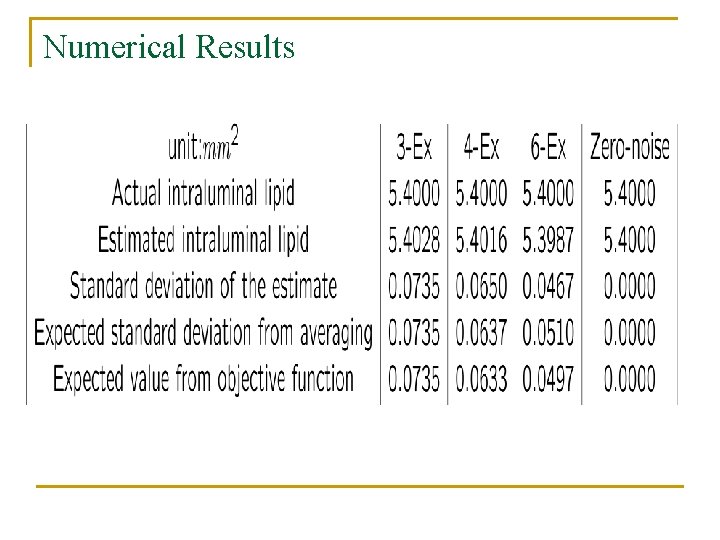
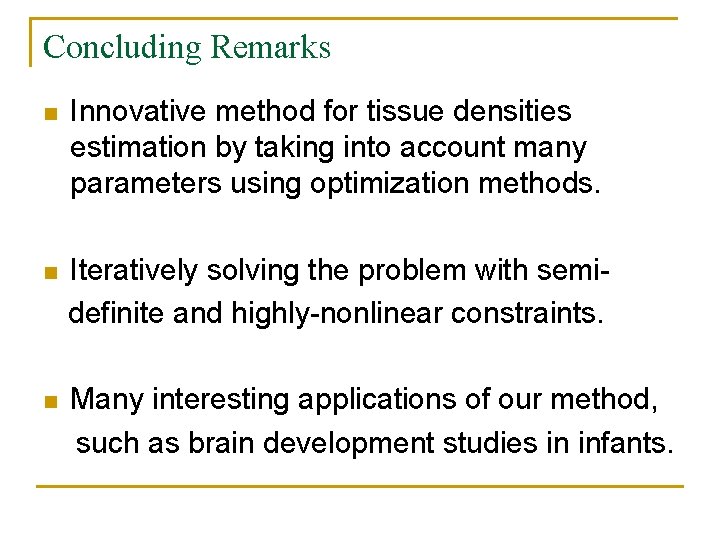
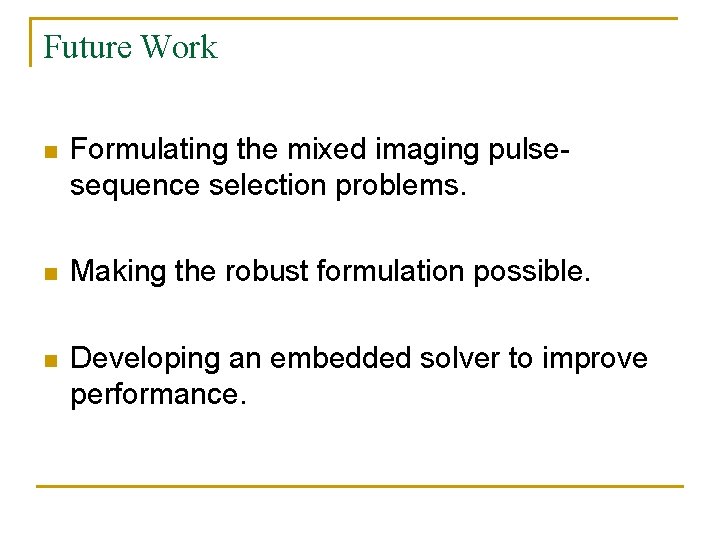
- Slides: 19
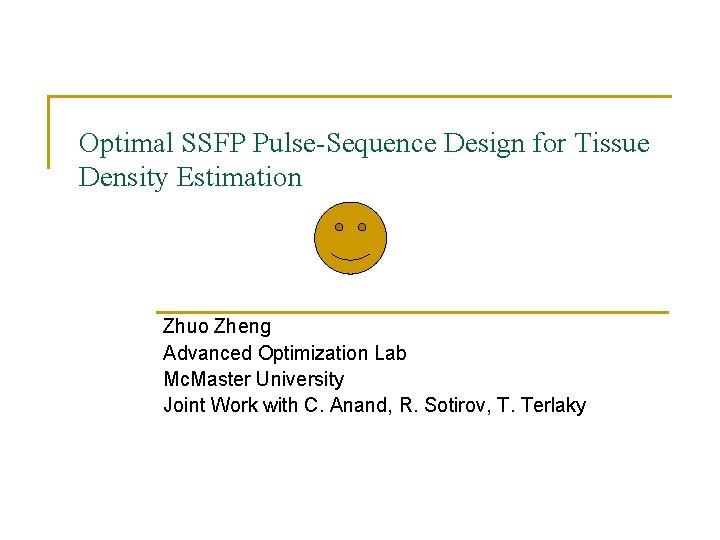
Optimal SSFP Pulse-Sequence Design for Tissue Density Estimation Zhuo Zheng Advanced Optimization Lab Mc. Master University Joint Work with C. Anand, R. Sotirov, T. Terlaky
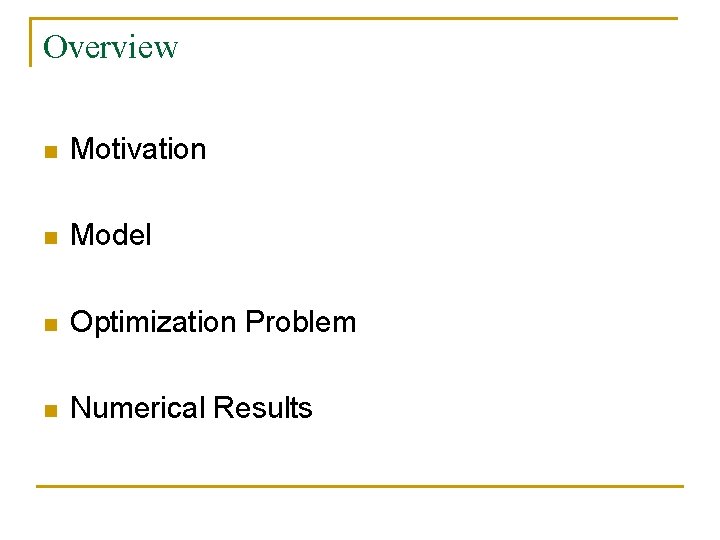
Overview n Motivation n Model n Optimization Problem n Numerical Results
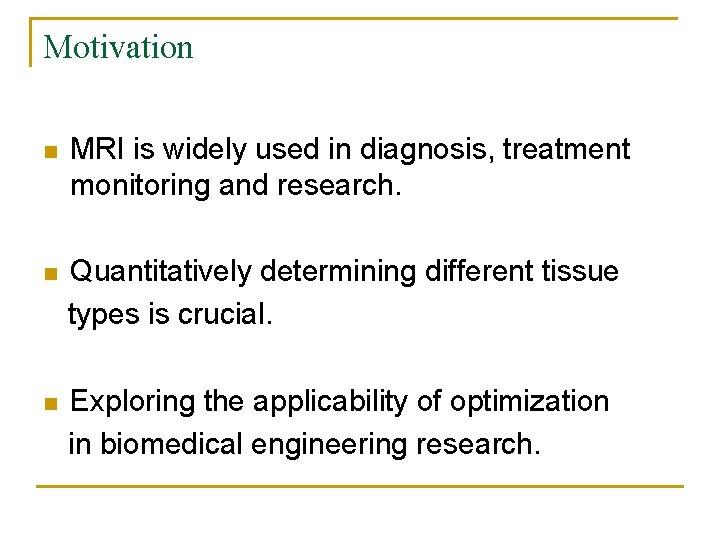
Motivation n MRI is widely used in diagnosis, treatment monitoring and research. n Quantitatively determining different tissue types is crucial. n Exploring the applicability of optimization in biomedical engineering research.
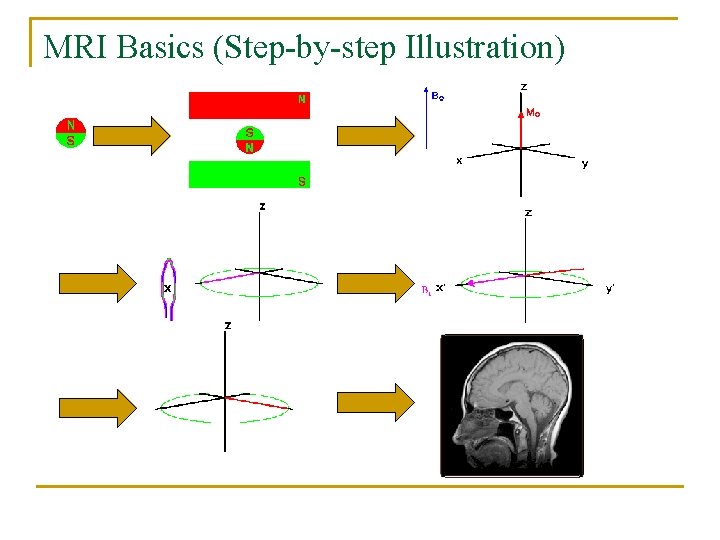
MRI Basics (Step-by-step Illustration)
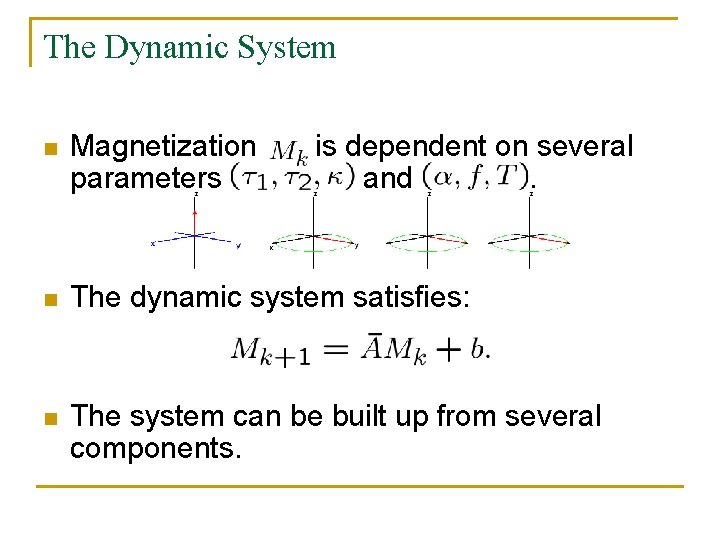
The Dynamic System n Magnetization parameters is dependent on several and. n The dynamic system satisfies: n The system can be built up from several components.
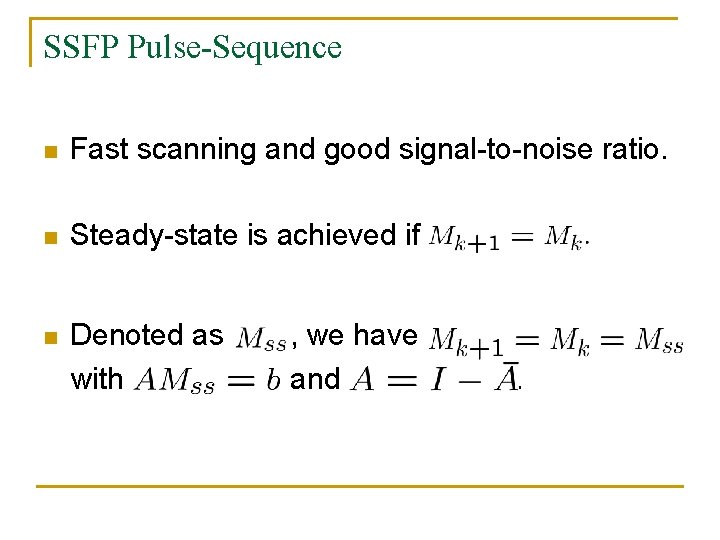
SSFP Pulse-Sequence n Fast scanning and good signal-to-noise ratio. n Steady-state is achieved if n Denoted as with , we have and .
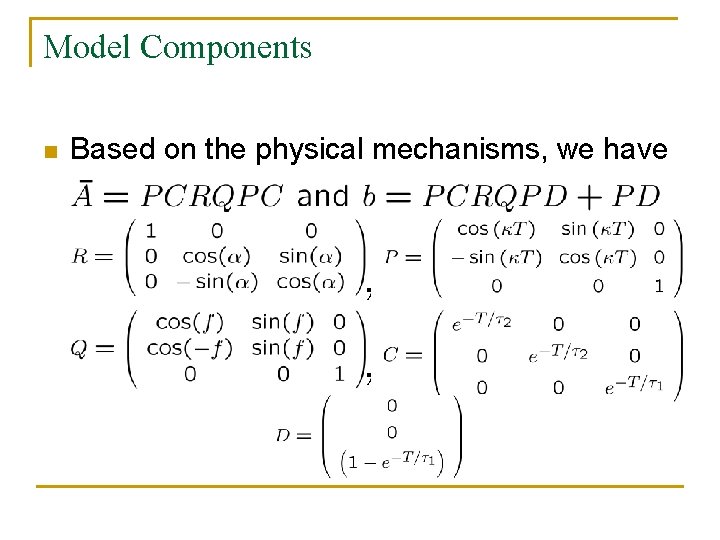
Model Components n Based on the physical mechanisms, we have
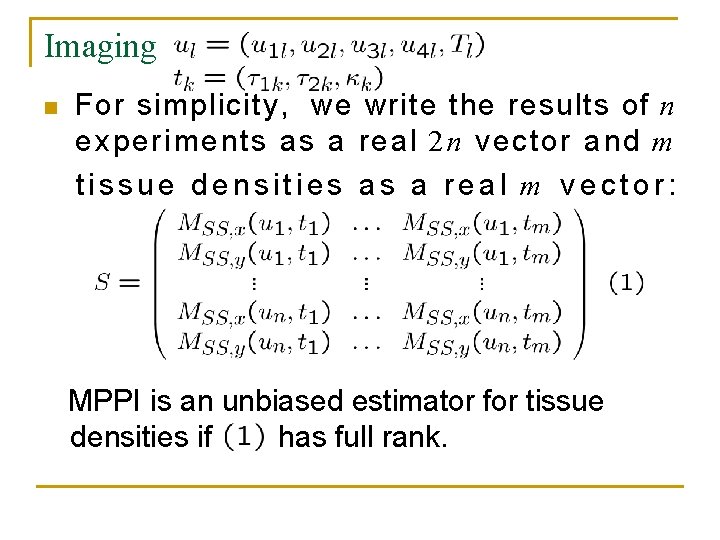
Imaging n For simplicity, we write the results of n experiments as a real 2 n vector and m tissue densities as a real m vector: MPPI is an unbiased estimator for tissue densities if has full rank.
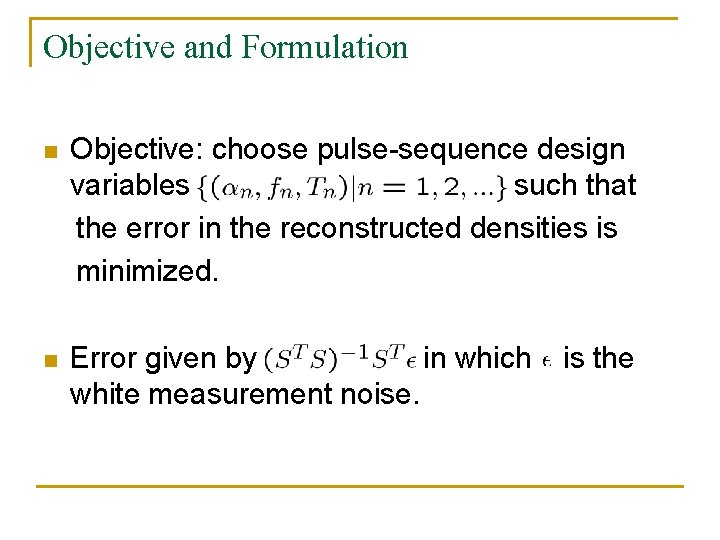
Objective and Formulation n Objective: choose pulse-sequence design variables such that the error in the reconstructed densities is minimized. n Error given by in which white measurement noise. is the
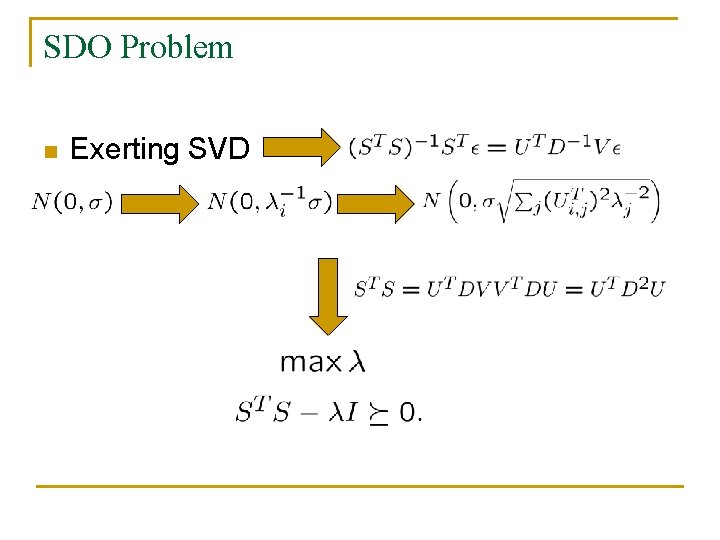
SDO Problem n Exerting SVD
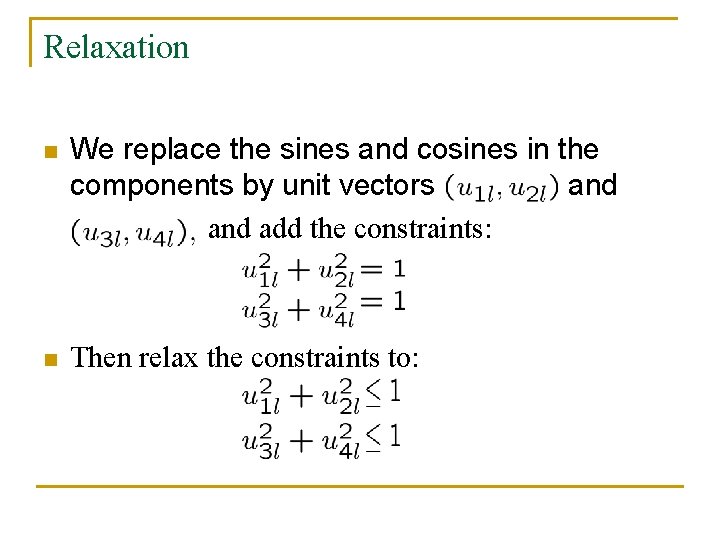
Relaxation n We replace the sines and cosines in the components by unit vectors and add the constraints: n Then relax the constraints to:
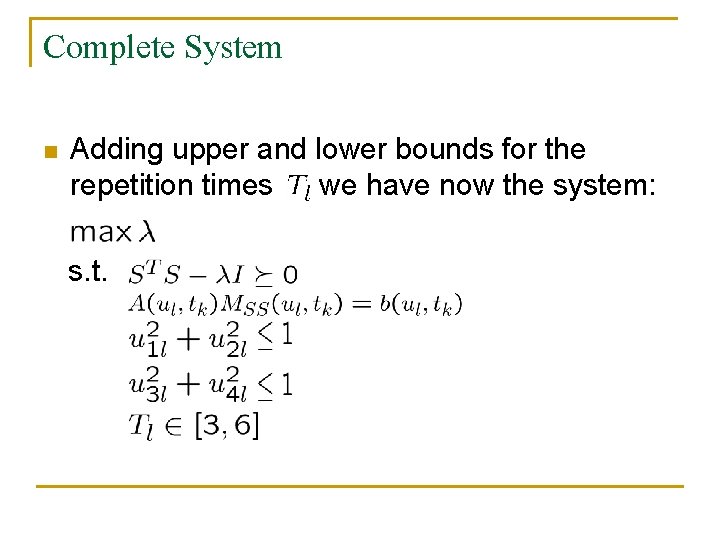
Complete System n Adding upper and lower bounds for the repetition times we have now the system: s. t.
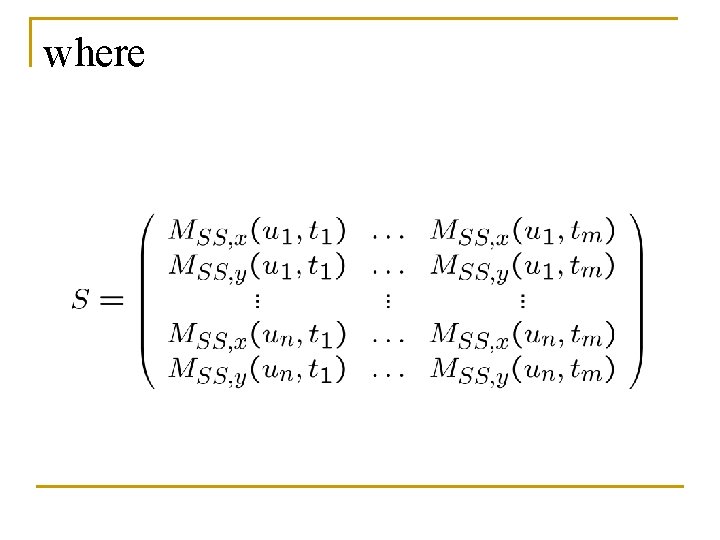
where
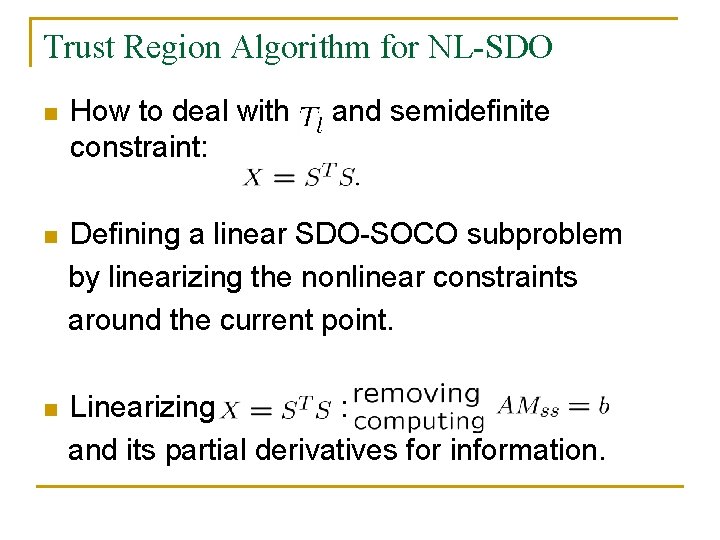
Trust Region Algorithm for NL-SDO n How to deal with constraint: and semidefinite n Defining a linear SDO-SOCO subproblem by linearizing the nonlinear constraints around the current point. n Linearizing : and its partial derivatives for information.
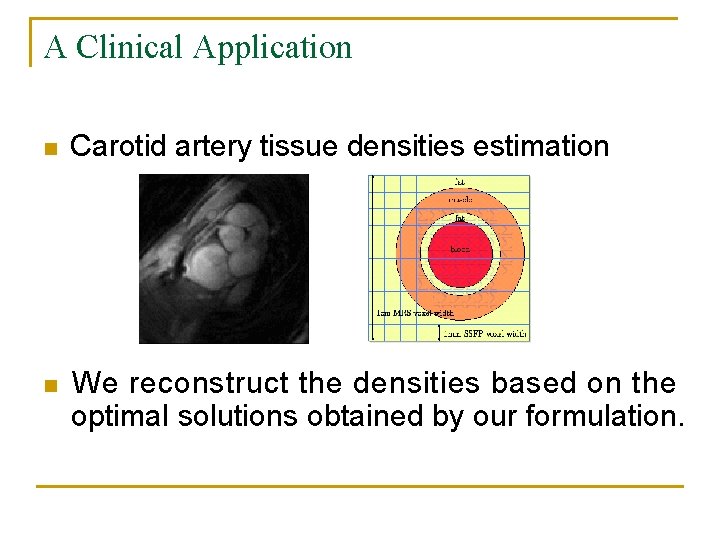
A Clinical Application n Carotid artery tissue densities estimation n We reconstruct the densities based on the optimal solutions obtained by our formulation.
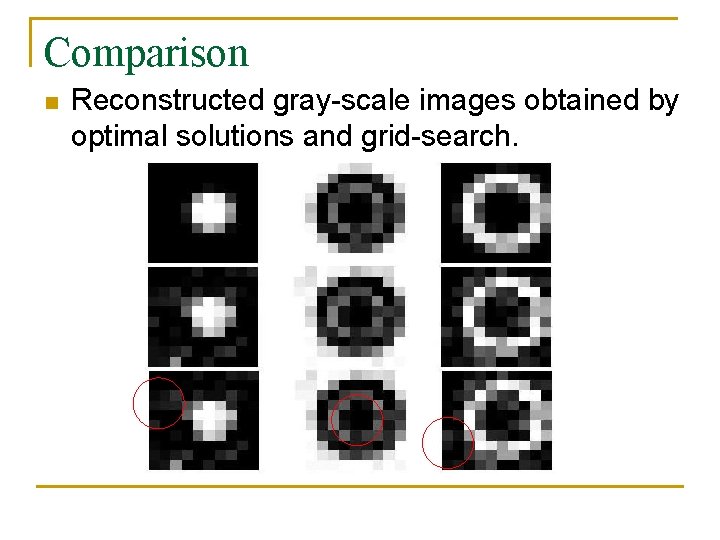
Comparison n Reconstructed gray-scale images obtained by optimal solutions and grid-search.
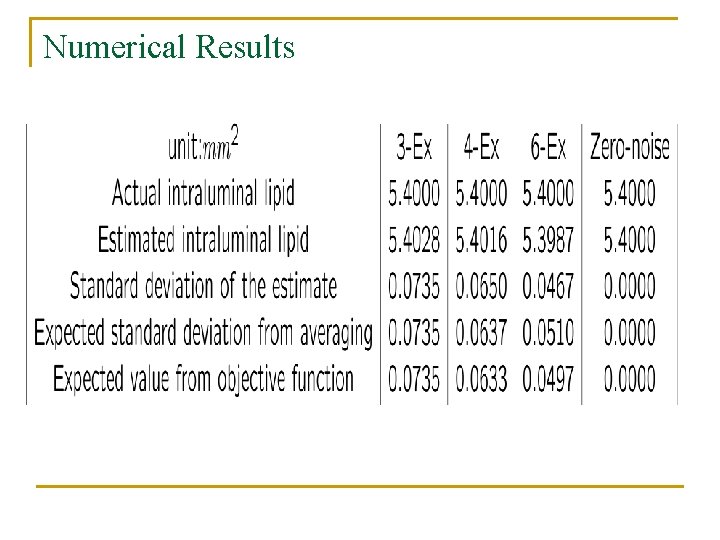
Numerical Results
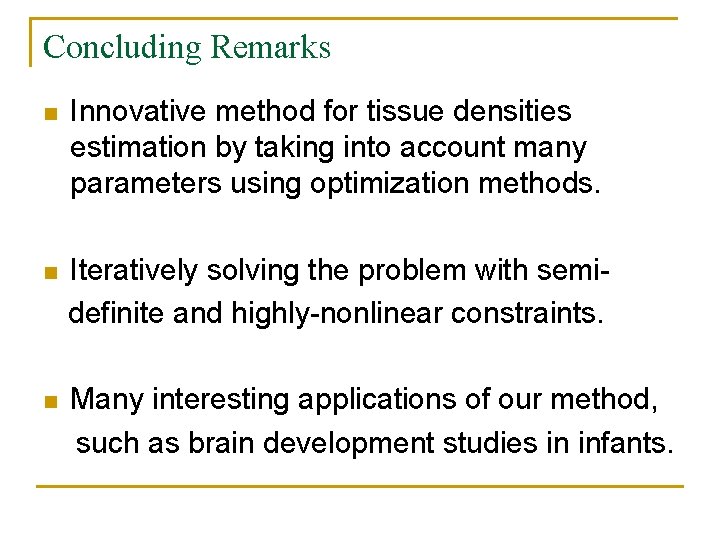
Concluding Remarks n Innovative method for tissue densities estimation by taking into account many parameters using optimization methods. n Iteratively solving the problem with semidefinite and highly-nonlinear constraints. n Many interesting applications of our method, such as brain development studies in infants.
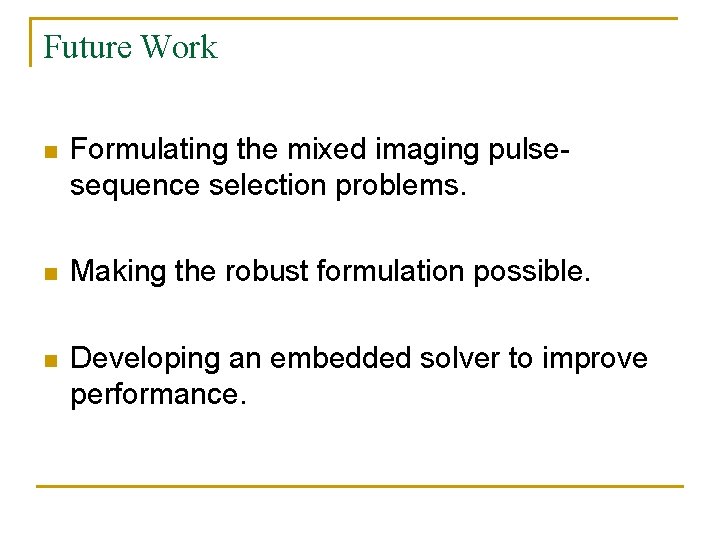
Future Work n Formulating the mixed imaging pulsesequence selection problems. n Making the robust formulation possible. n Developing an embedded solver to improve performance.
Density estimation trees
Formula for specific gravity
Planar density bcc
Physiological density
Linear density of atoms
Nda full dac
What does arithmetic density tell us
Tissue density
Perforation plates
Fspos vägledning för kontinuitetshantering
Novell typiska drag
Nationell inriktning för artificiell intelligens
Returpilarna
Shingelfrisyren
En lathund för arbete med kontinuitetshantering
Underlag för särskild löneskatt på pensionskostnader
Tidbok
Sura för anatom
Densitet vatten
Datorkunskap för nybörjare