Lecture 5 Model Evaluation Elements of Model evaluation
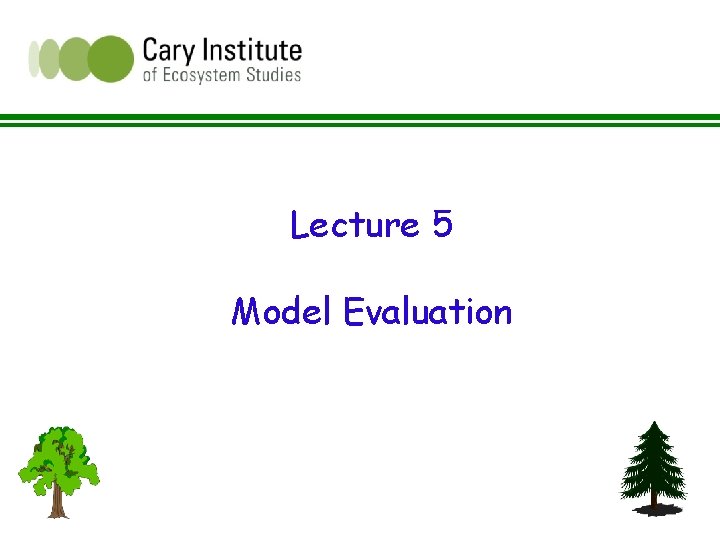
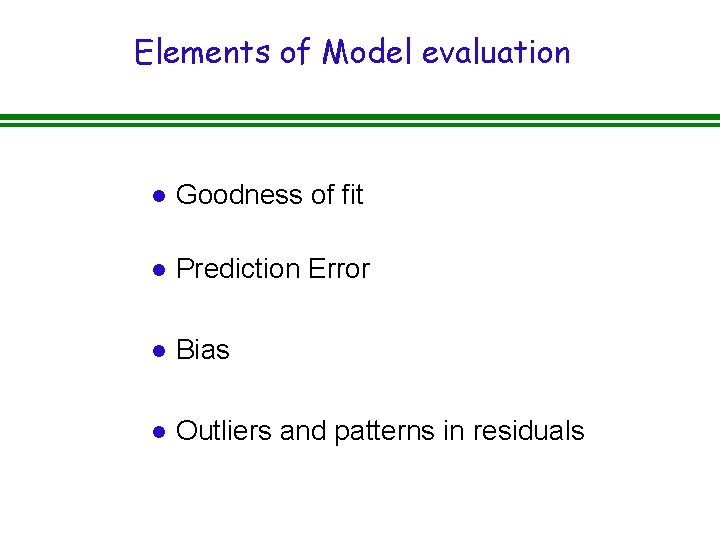
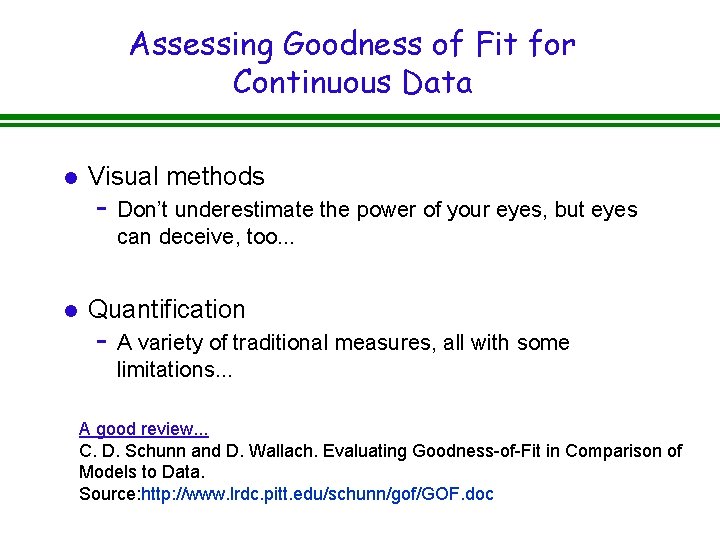
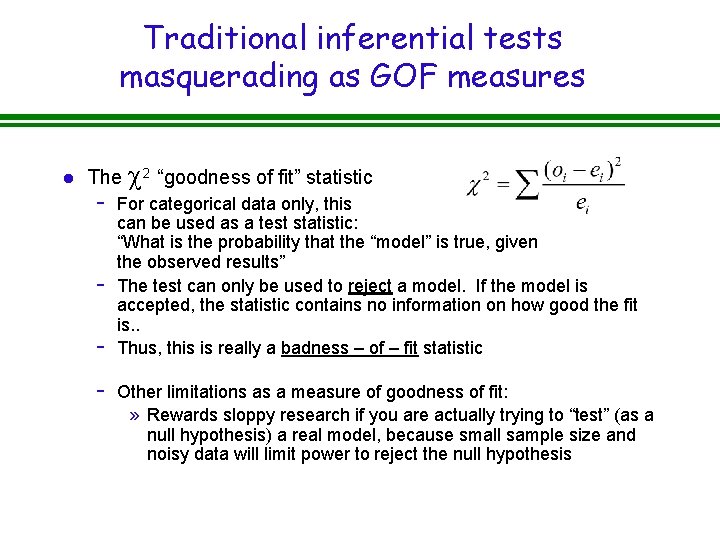
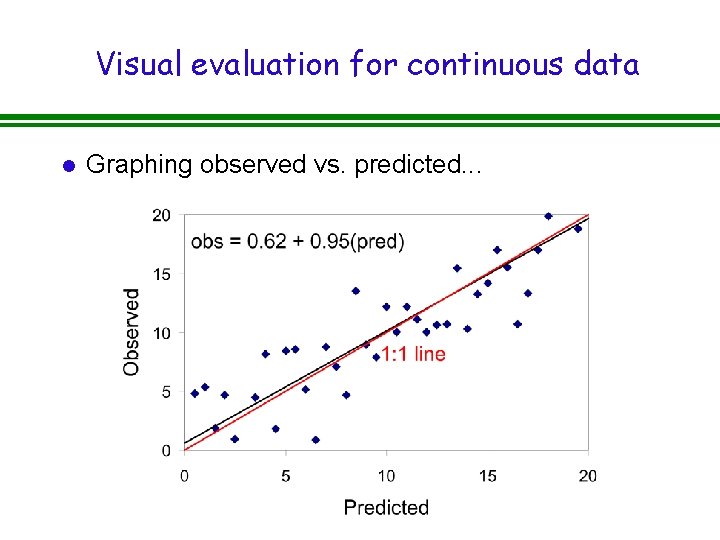
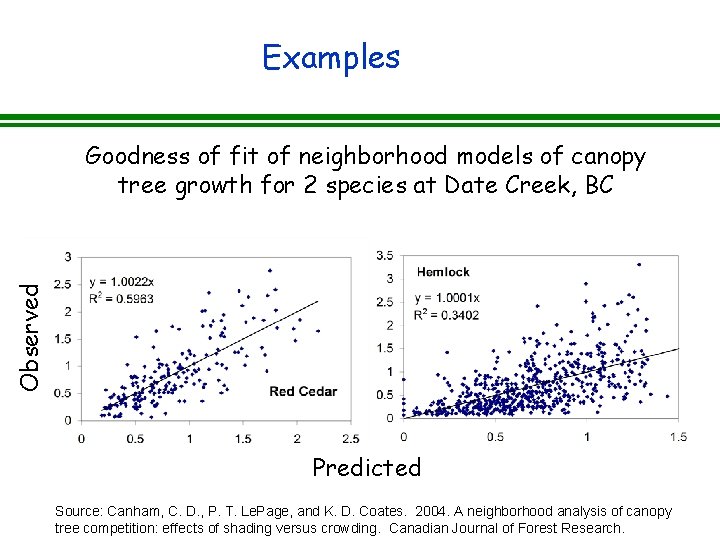
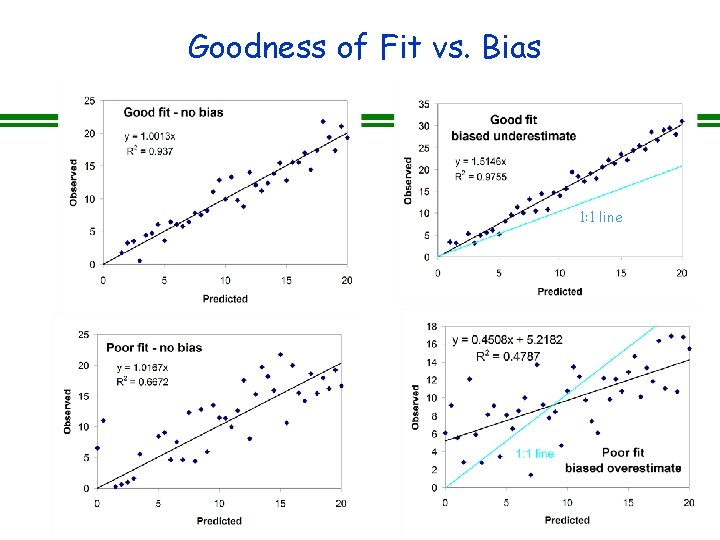
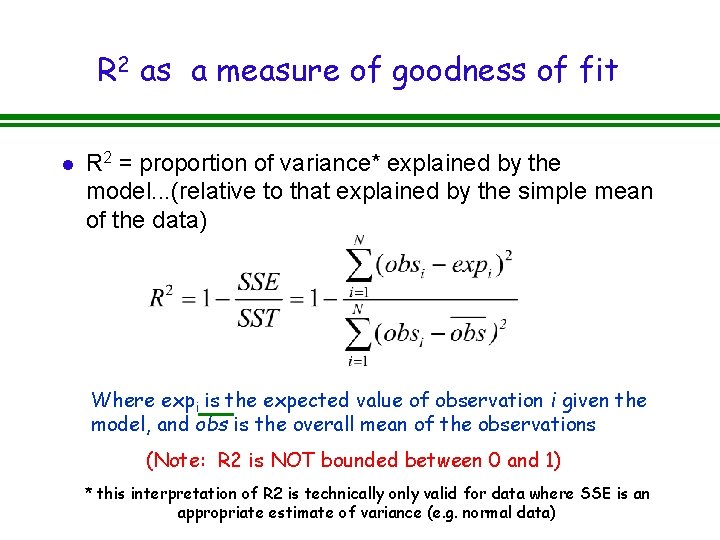
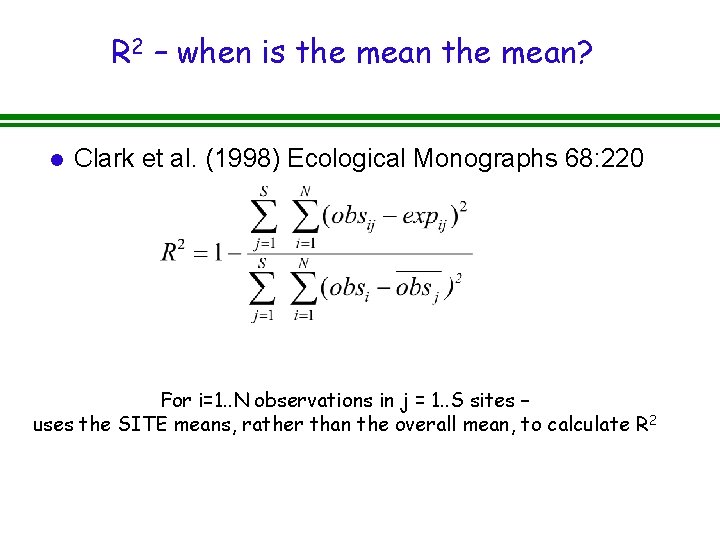
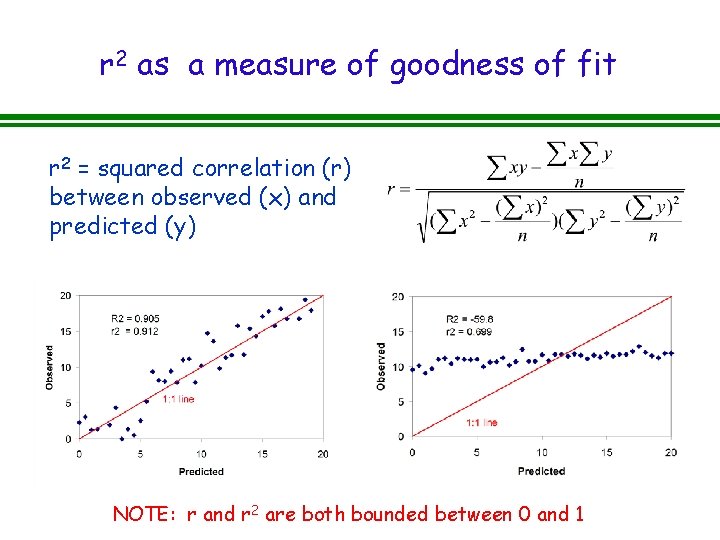
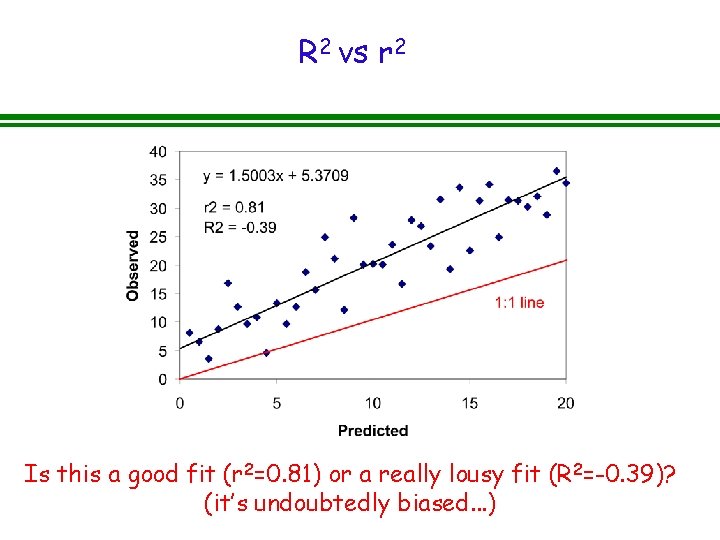
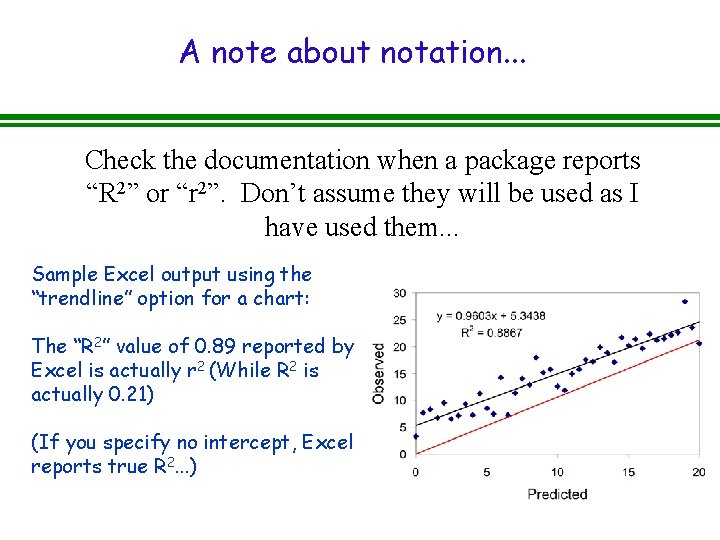
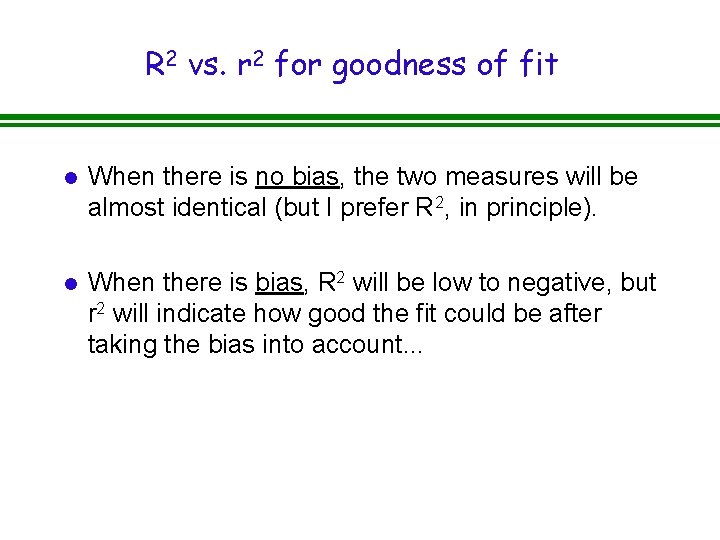
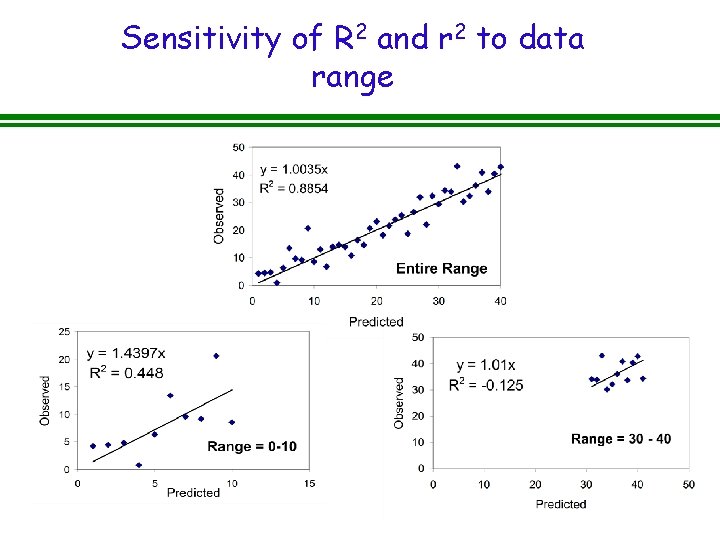
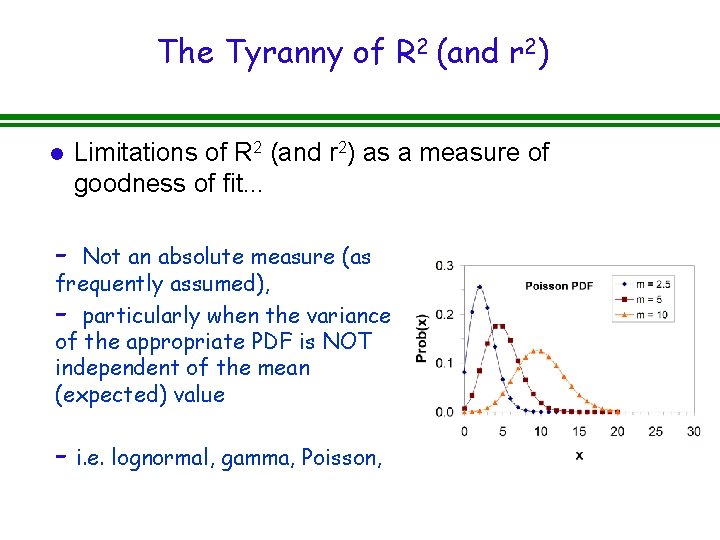
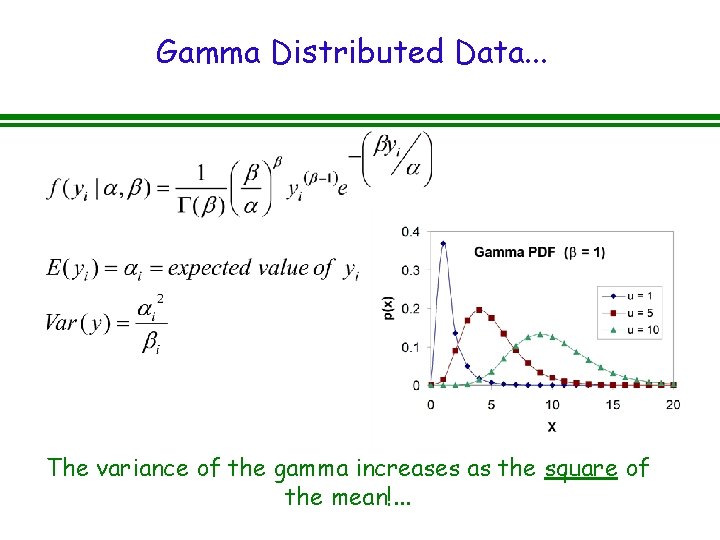
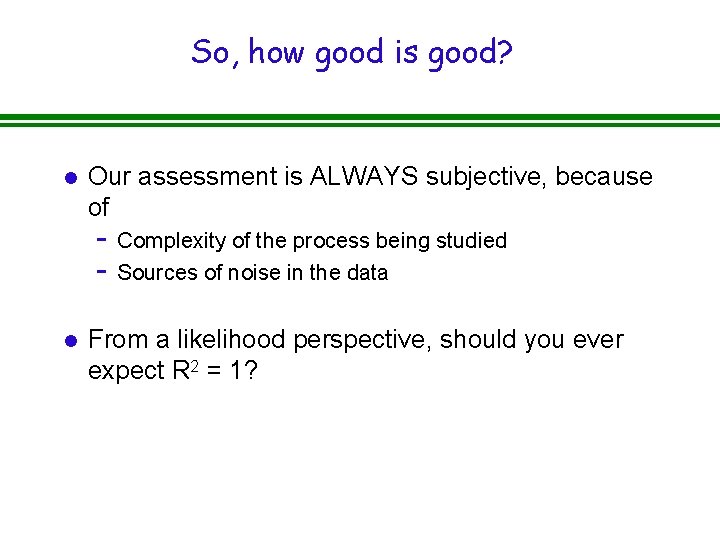
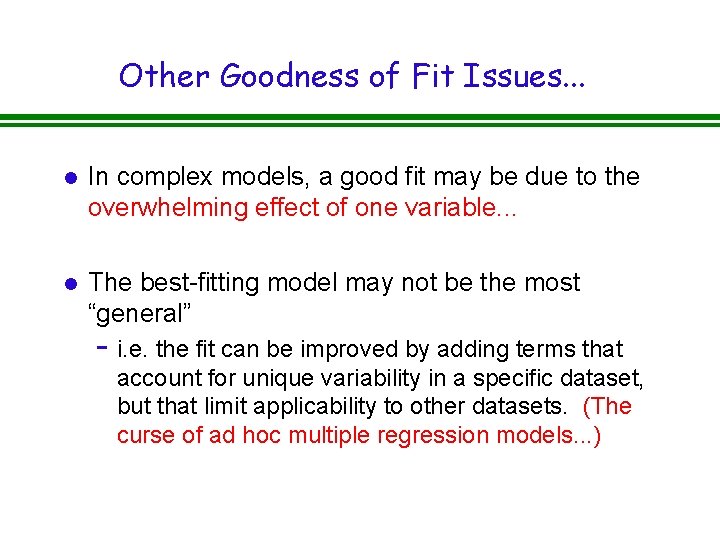
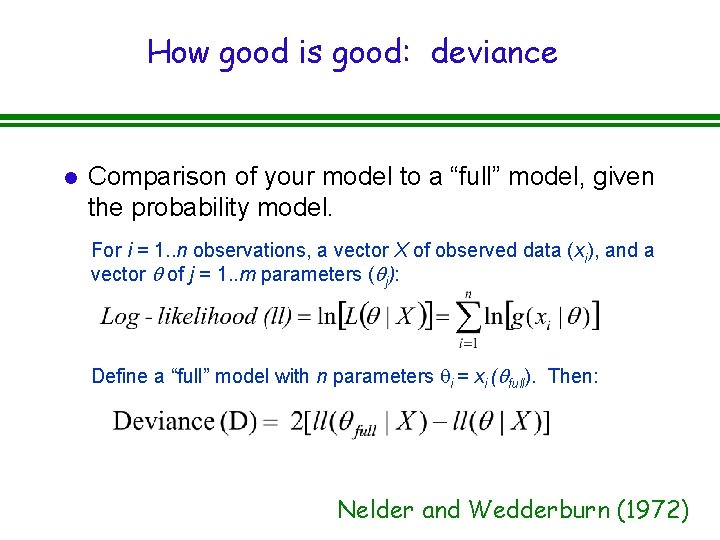
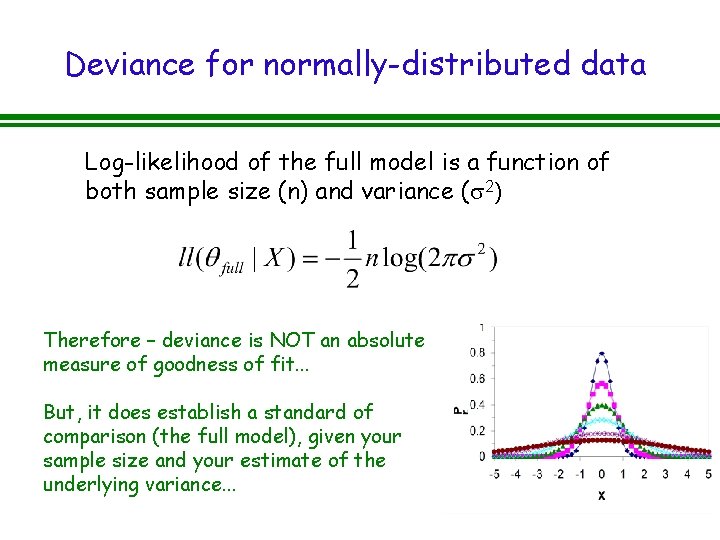
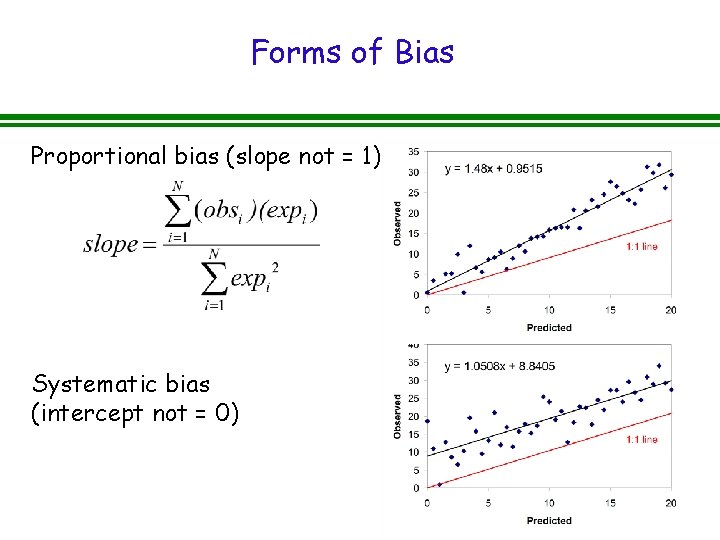
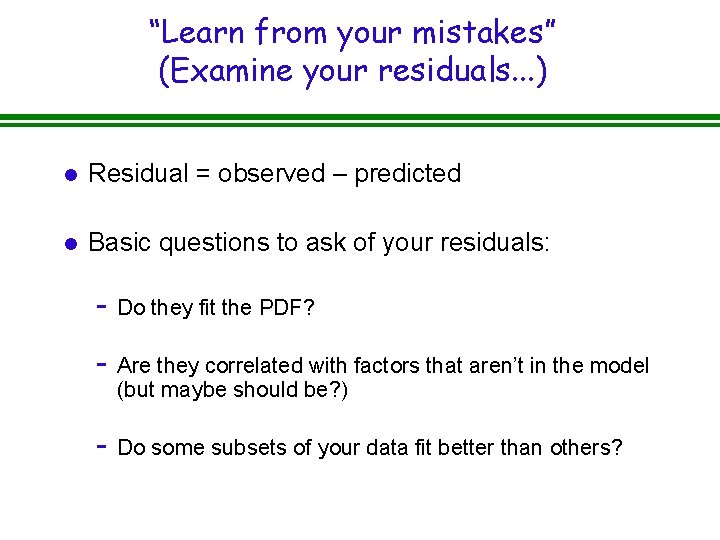
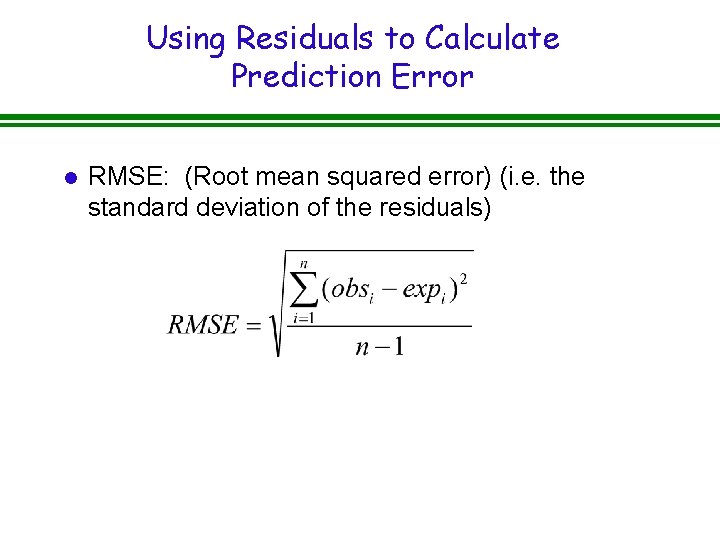
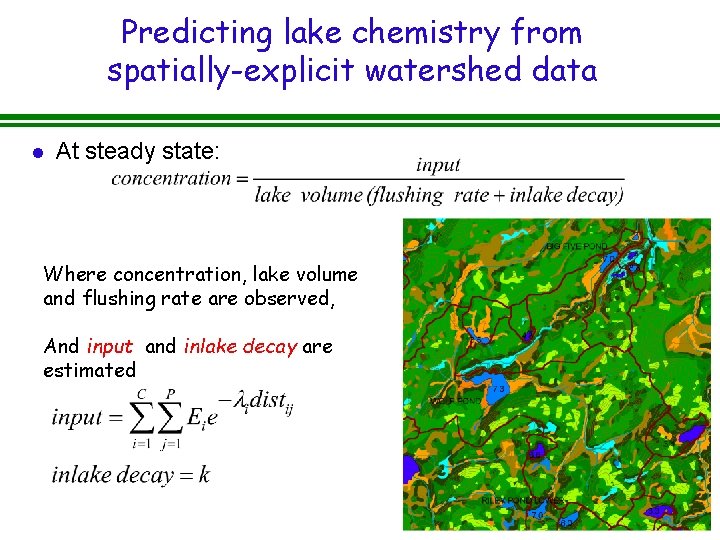
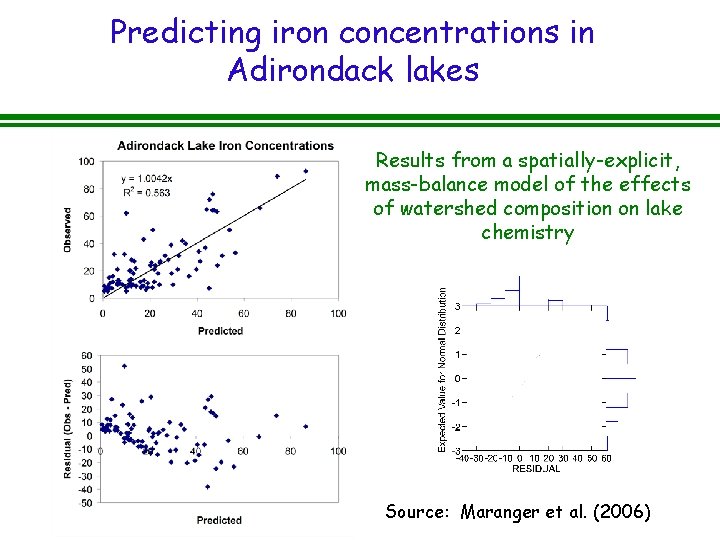
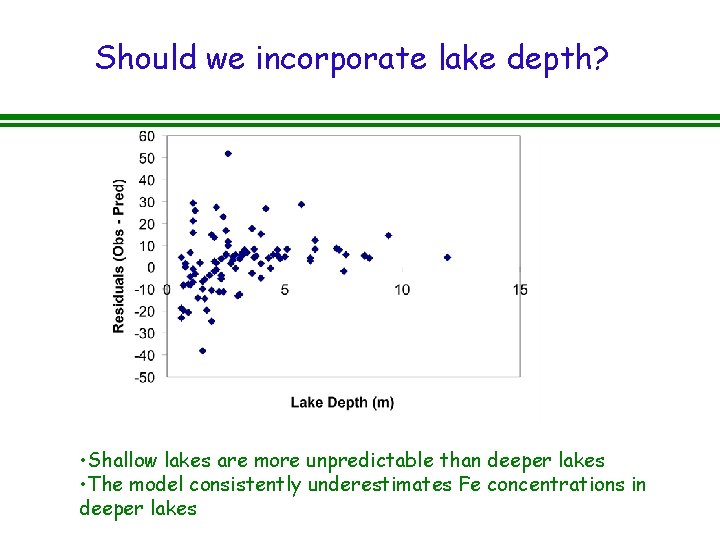
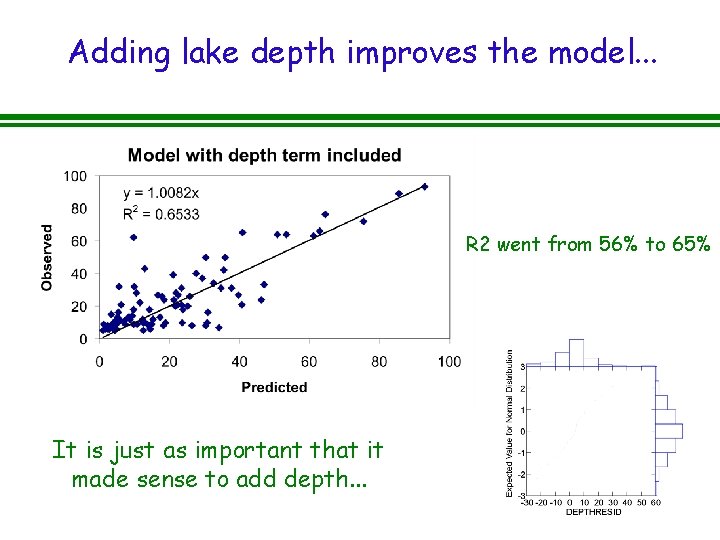
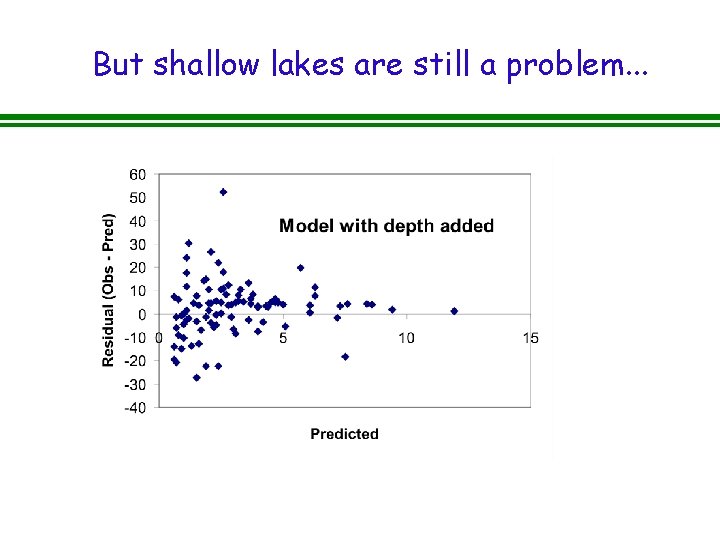
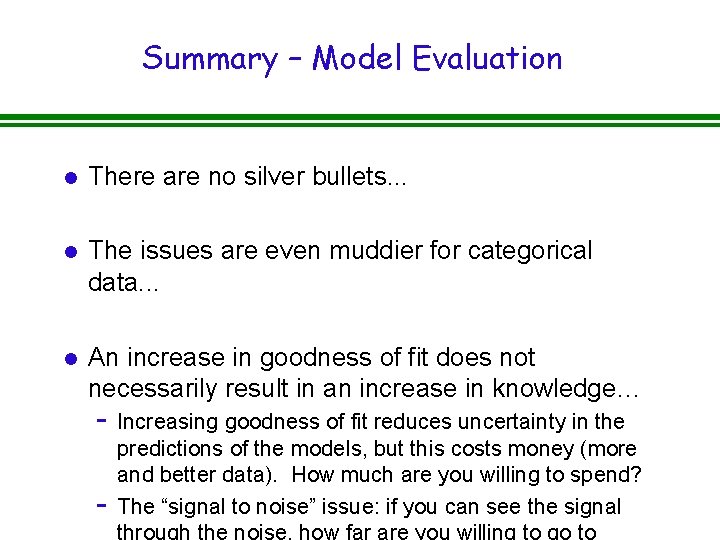
- Slides: 29
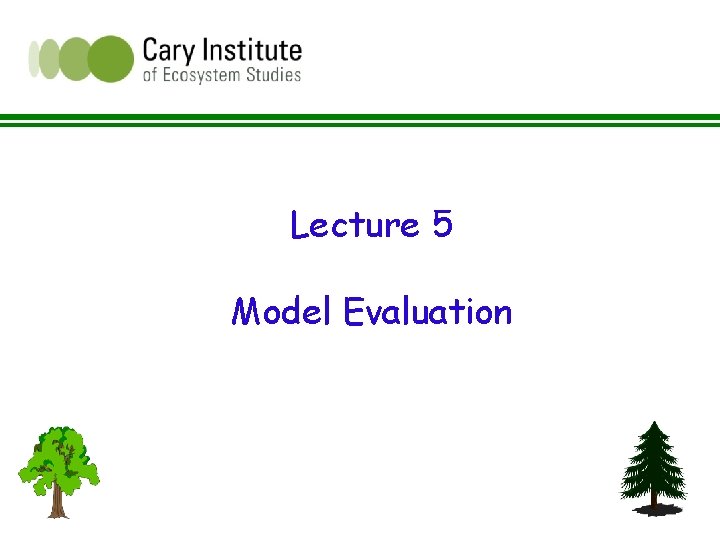
Lecture 5 Model Evaluation
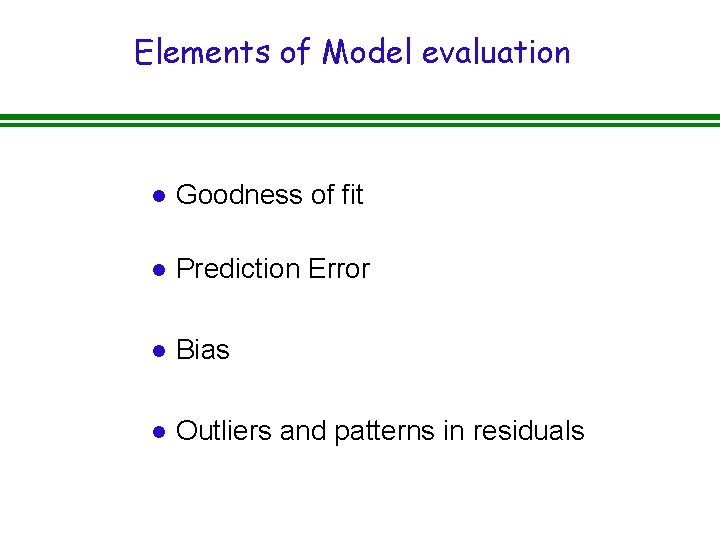
Elements of Model evaluation l Goodness of fit l Prediction Error l Bias l Outliers and patterns in residuals
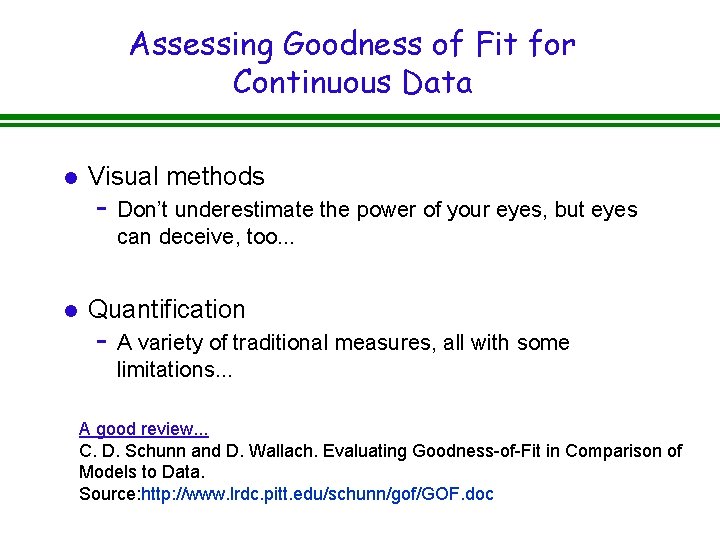
Assessing Goodness of Fit for Continuous Data l Visual methods - Don’t underestimate the power of your eyes, but eyes can deceive, too. . . l Quantification - A variety of traditional measures, all with some limitations. . . A good review. . . C. D. Schunn and D. Wallach. Evaluating Goodness-of-Fit in Comparison of Models to Data. Source: http: //www. lrdc. pitt. edu/schunn/gof/GOF. doc
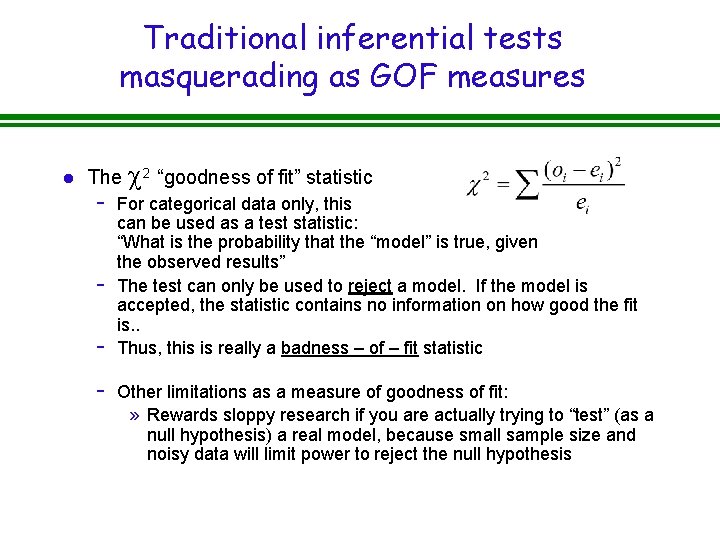
Traditional inferential tests masquerading as GOF measures l The c 2 “goodness of fit” statistic - For categorical data only, this can be used as a test statistic: “What is the probability that the “model” is true, given the observed results” The test can only be used to reject a model. If the model is accepted, the statistic contains no information on how good the fit is. . Thus, this is really a badness – of – fit statistic Other limitations as a measure of goodness of fit: » Rewards sloppy research if you are actually trying to “test” (as a null hypothesis) a real model, because small sample size and noisy data will limit power to reject the null hypothesis
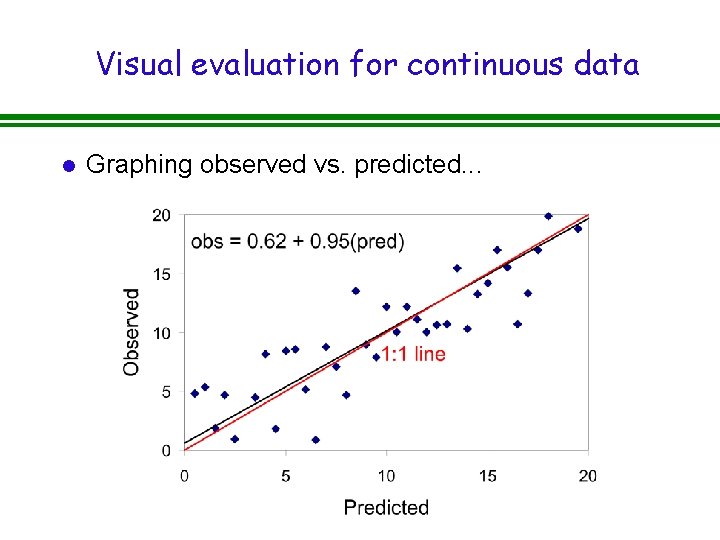
Visual evaluation for continuous data l Graphing observed vs. predicted. . .
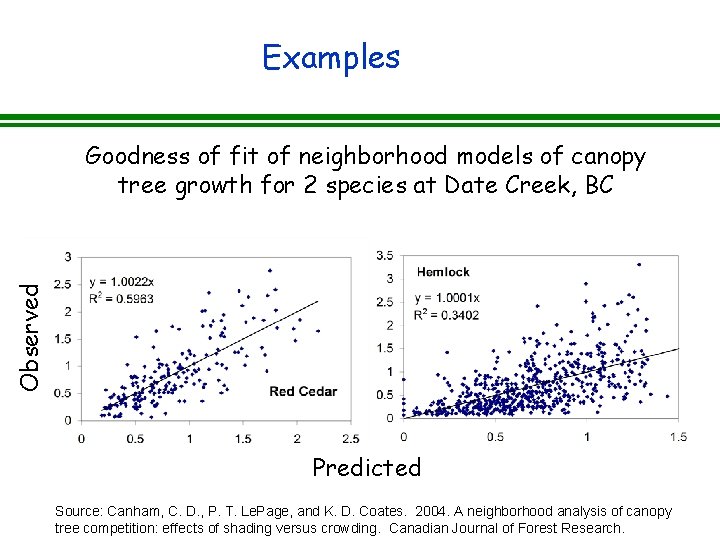
Examples Observed Goodness of fit of neighborhood models of canopy tree growth for 2 species at Date Creek, BC Predicted Source: Canham, C. D. , P. T. Le. Page, and K. D. Coates. 2004. A neighborhood analysis of canopy tree competition: effects of shading versus crowding. Canadian Journal of Forest Research.
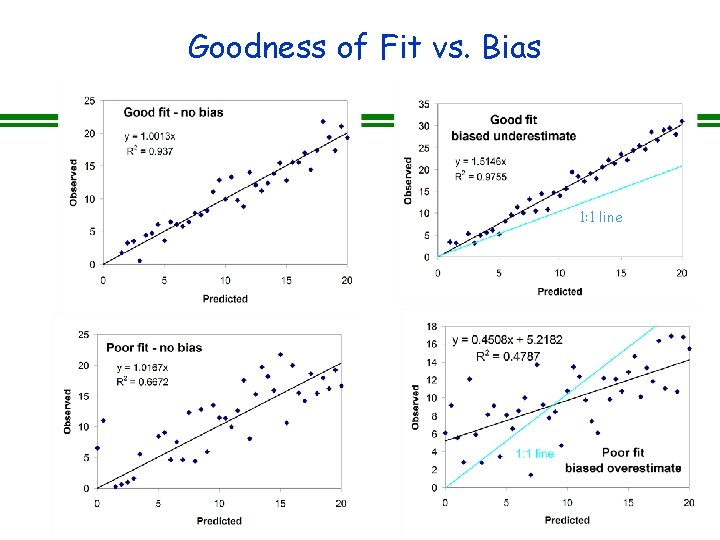
Goodness of Fit vs. Bias 1: 1 line
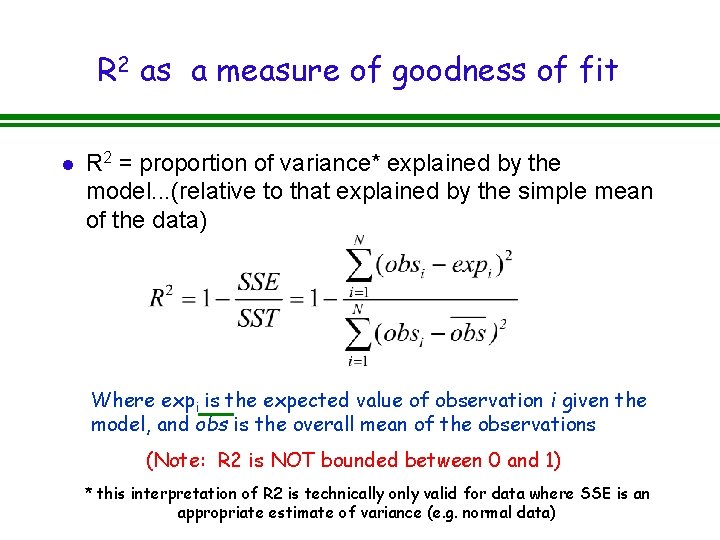
R 2 as a measure of goodness of fit l R 2 = proportion of variance* explained by the model. . . (relative to that explained by the simple mean of the data) Where expi is the expected value of observation i given the model, and obs is the overall mean of the observations (Note: R 2 is NOT bounded between 0 and 1) * this interpretation of R 2 is technically only valid for data where SSE is an appropriate estimate of variance (e. g. normal data)
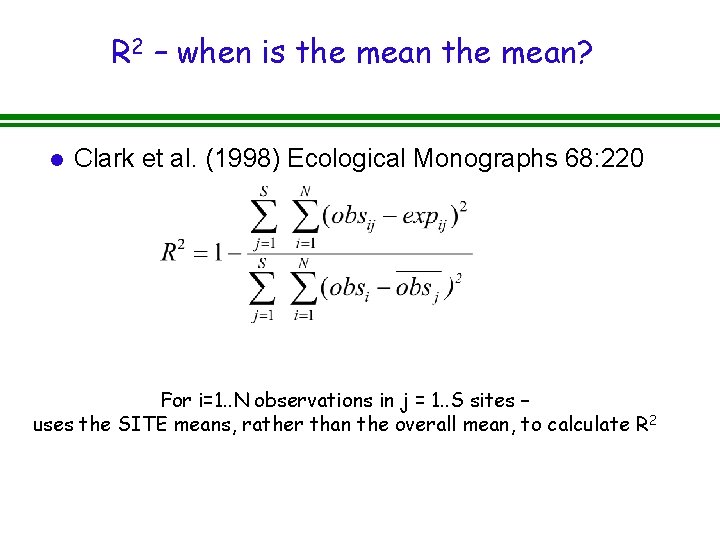
R 2 – when is the mean? l Clark et al. (1998) Ecological Monographs 68: 220 For i=1. . N observations in j = 1. . S sites – uses the SITE means, rather than the overall mean, to calculate R 2
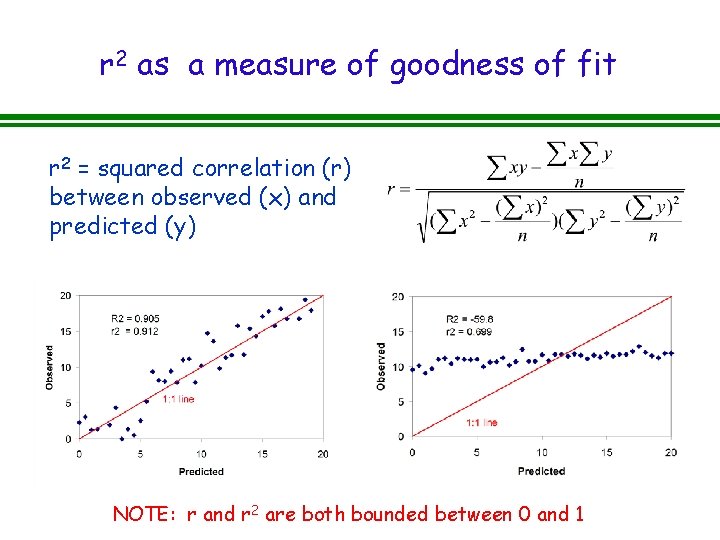
r 2 as a measure of goodness of fit r 2 = squared correlation (r) between observed (x) and predicted (y) NOTE: r and r 2 are both bounded between 0 and 1
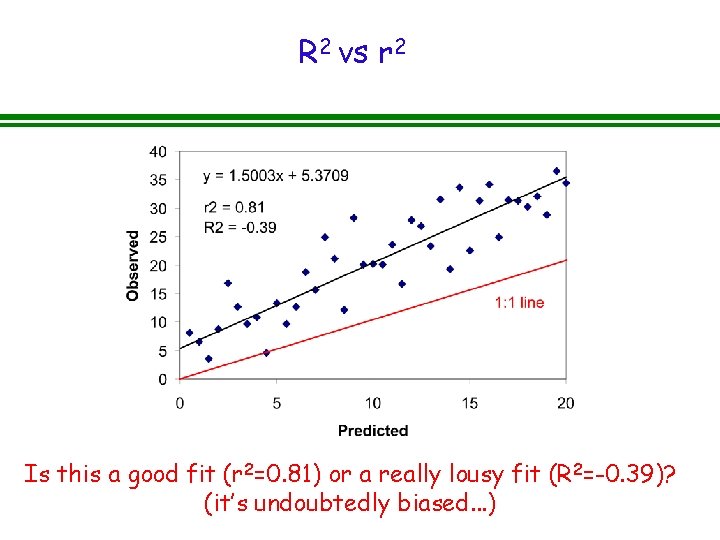
R 2 vs r 2 Is this a good fit (r 2=0. 81) or a really lousy fit (R 2=-0. 39)? (it’s undoubtedly biased. . . )
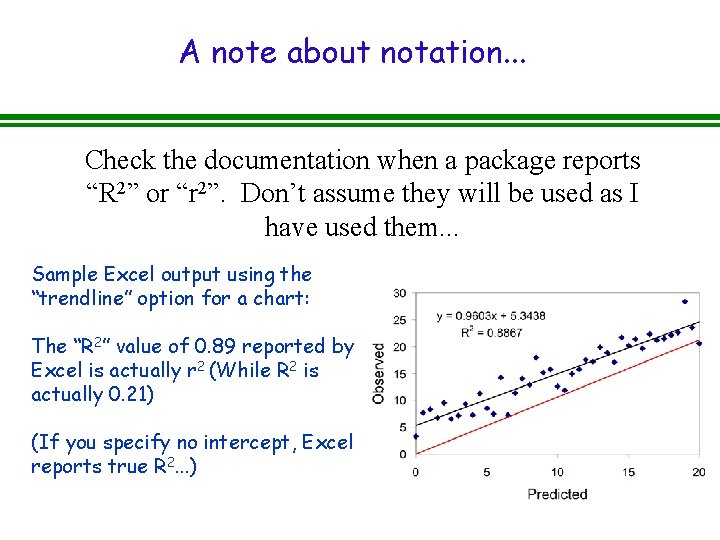
A note about notation. . . Check the documentation when a package reports “R 2” or “r 2”. Don’t assume they will be used as I have used them. . . Sample Excel output using the “trendline” option for a chart: The “R 2” value of 0. 89 reported by Excel is actually r 2 (While R 2 is actually 0. 21) (If you specify no intercept, Excel reports true R 2. . . )
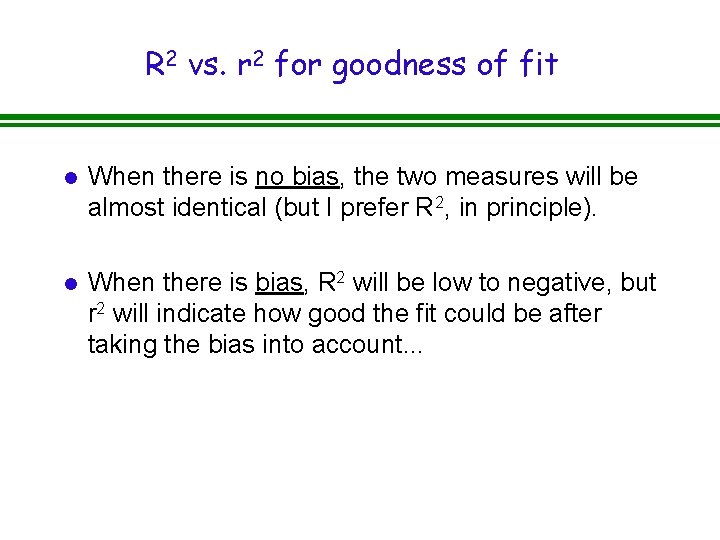
R 2 vs. r 2 for goodness of fit l When there is no bias, the two measures will be almost identical (but I prefer R 2, in principle). l When there is bias, R 2 will be low to negative, but r 2 will indicate how good the fit could be after taking the bias into account. . .
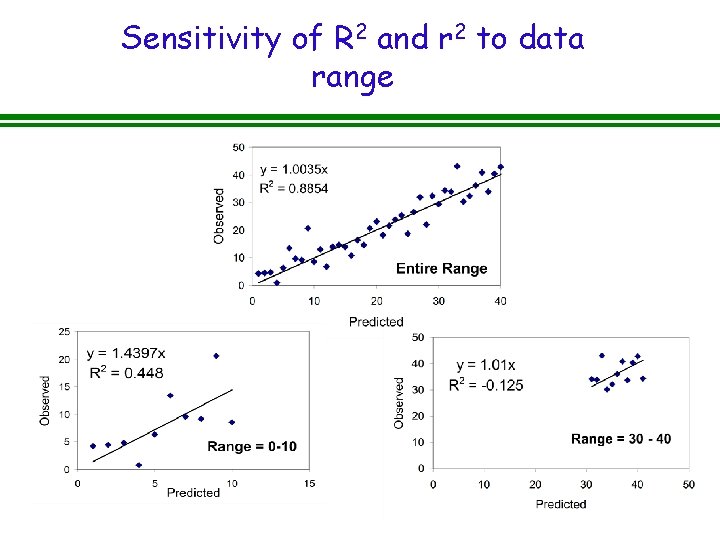
Sensitivity of R 2 and r 2 to data range
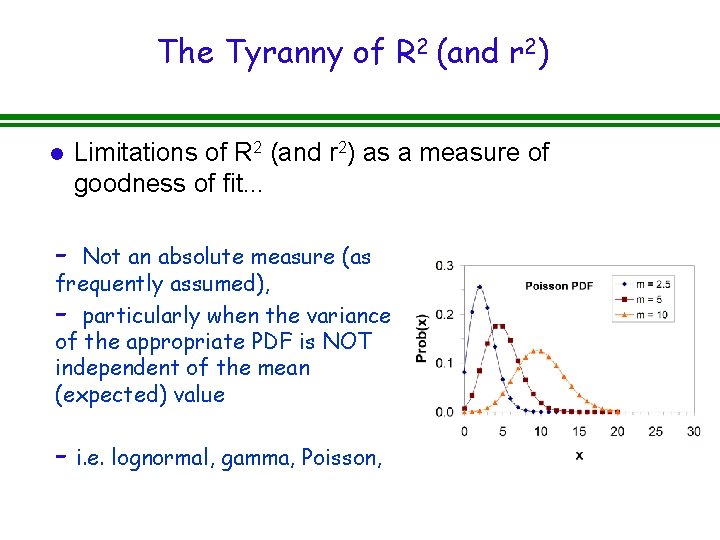
The Tyranny of R 2 (and r 2) l Limitations of R 2 (and r 2) as a measure of goodness of fit. . . - Not an absolute measure (as frequently assumed), - particularly when the variance of the appropriate PDF is NOT independent of the mean (expected) value - i. e. lognormal, gamma, Poisson,
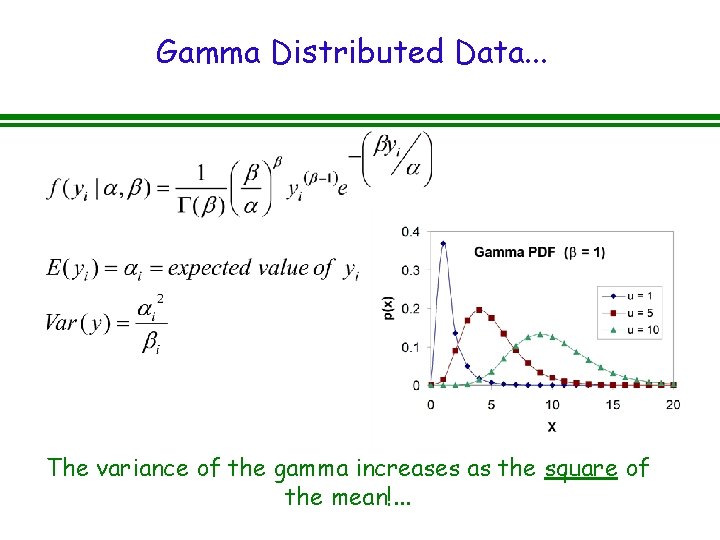
Gamma Distributed Data. . . The variance of the gamma increases as the square of the mean!. . .
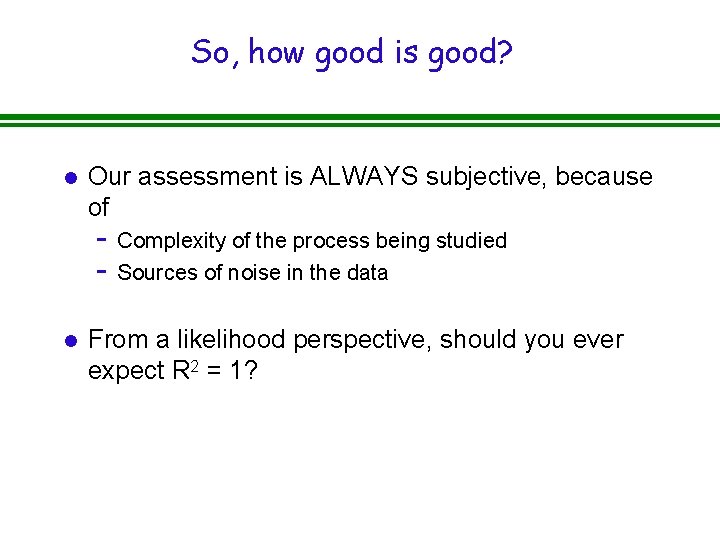
So, how good is good? l Our assessment is ALWAYS subjective, because of - Complexity of the process being studied - Sources of noise in the data l From a likelihood perspective, should you ever expect R 2 = 1?
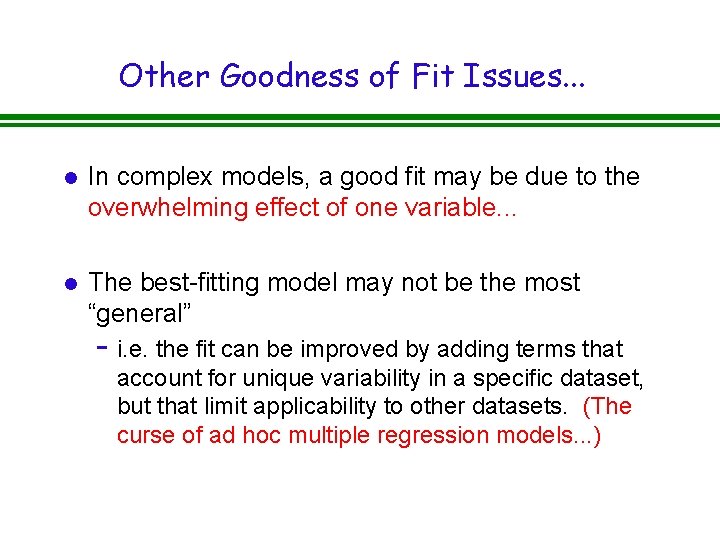
Other Goodness of Fit Issues. . . l In complex models, a good fit may be due to the overwhelming effect of one variable. . . l The best-fitting model may not be the most “general” - i. e. the fit can be improved by adding terms that account for unique variability in a specific dataset, but that limit applicability to other datasets. (The curse of ad hoc multiple regression models. . . )
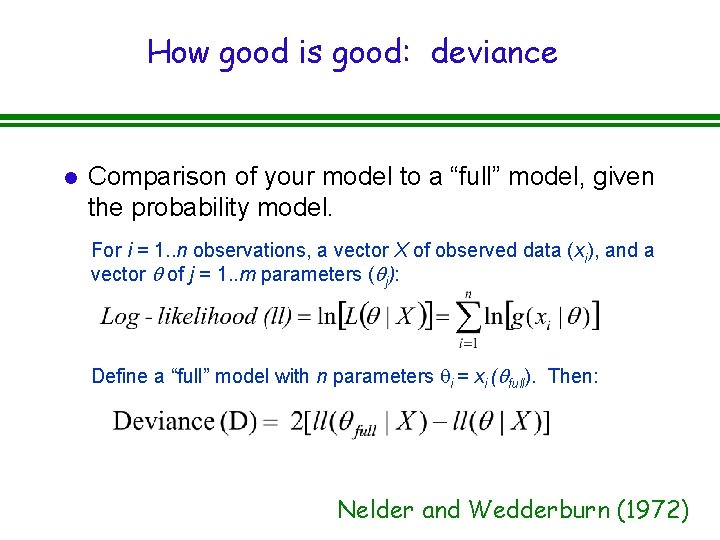
How good is good: deviance l Comparison of your model to a “full” model, given the probability model. For i = 1. . n observations, a vector X of observed data (xi), and a vector q of j = 1. . m parameters (qj): Define a “full” model with n parameters qi = xi (qfull). Then: Nelder and Wedderburn (1972)
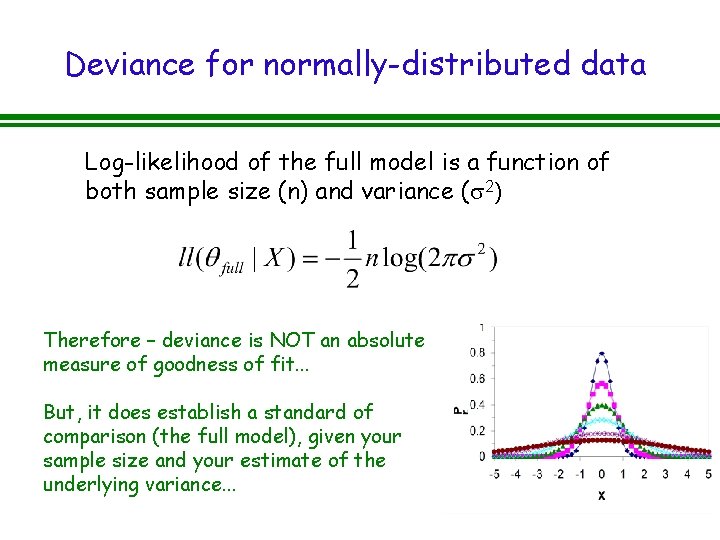
Deviance for normally-distributed data Log-likelihood of the full model is a function of both sample size (n) and variance (s 2) Therefore – deviance is NOT an absolute measure of goodness of fit. . . But, it does establish a standard of comparison (the full model), given your sample size and your estimate of the underlying variance. . .
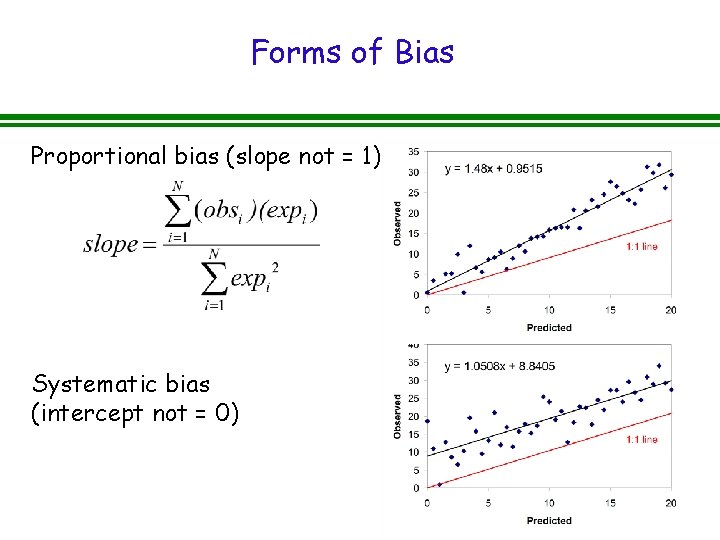
Forms of Bias Proportional bias (slope not = 1) Systematic bias (intercept not = 0)
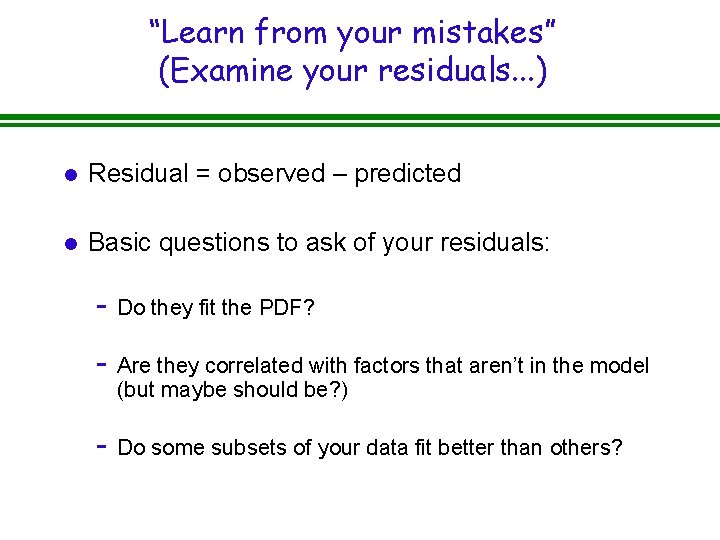
“Learn from your mistakes” (Examine your residuals. . . ) l Residual = observed – predicted l Basic questions to ask of your residuals: - Do they fit the PDF? - Are they correlated with factors that aren’t in the model (but maybe should be? ) - Do some subsets of your data fit better than others?
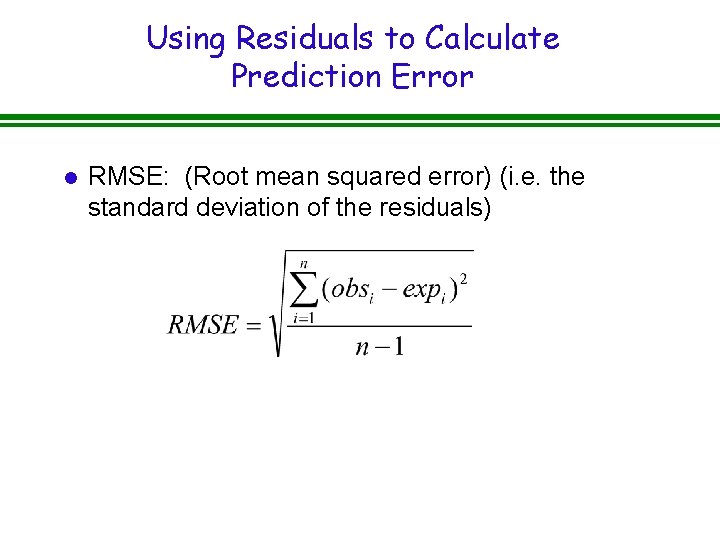
Using Residuals to Calculate Prediction Error l RMSE: (Root mean squared error) (i. e. the standard deviation of the residuals)
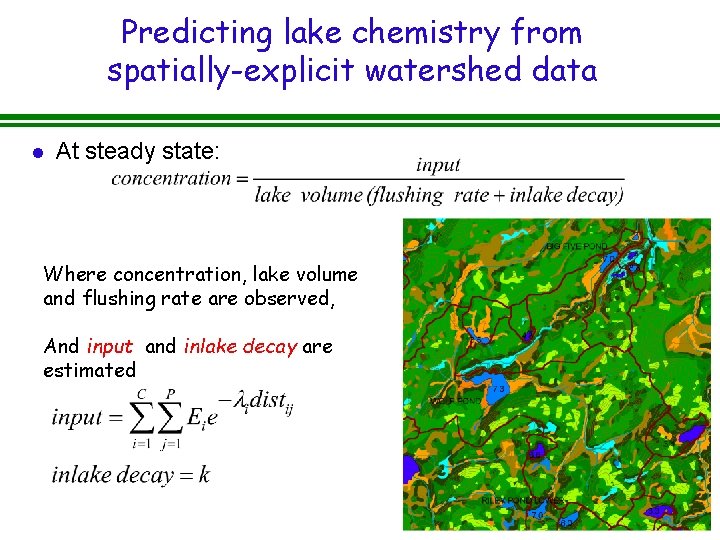
Predicting lake chemistry from spatially-explicit watershed data l At steady state: Where concentration, lake volume and flushing rate are observed, And input and inlake decay are estimated
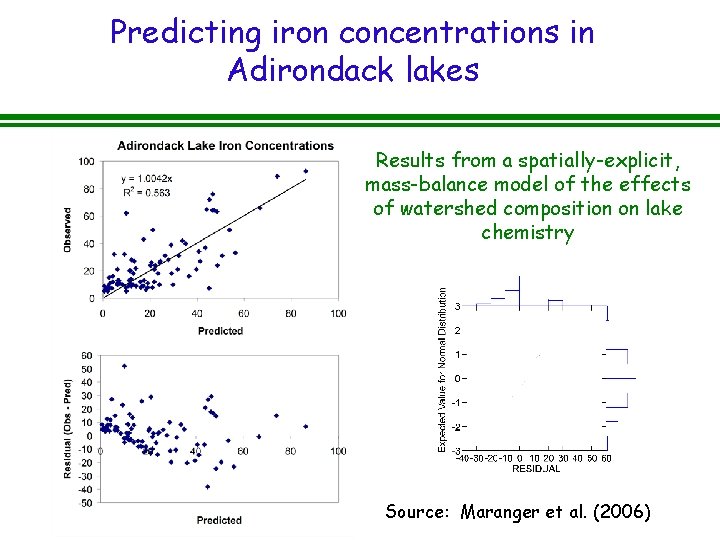
Predicting iron concentrations in Adirondack lakes Results from a spatially-explicit, mass-balance model of the effects of watershed composition on lake chemistry Source: Maranger et al. (2006)
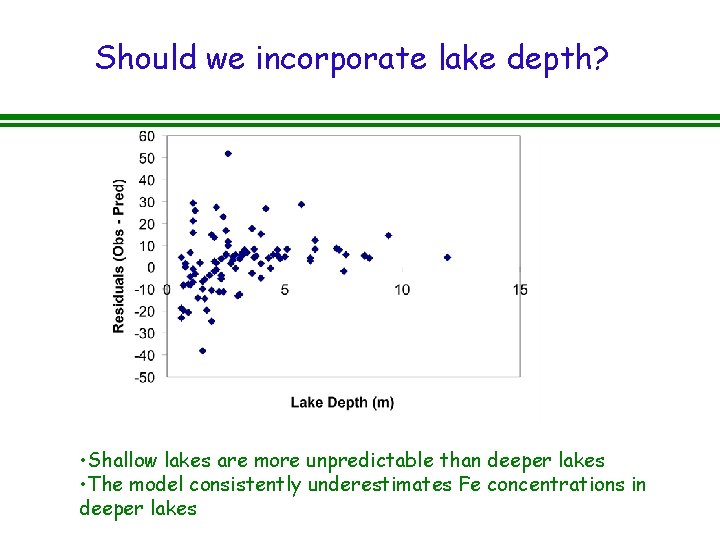
Should we incorporate lake depth? • Shallow lakes are more unpredictable than deeper lakes • The model consistently underestimates Fe concentrations in deeper lakes
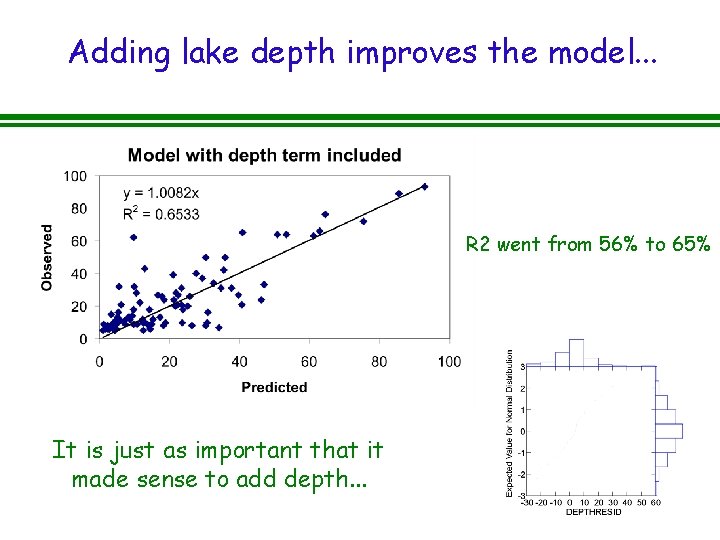
Adding lake depth improves the model. . . R 2 went from 56% to 65% It is just as important that it made sense to add depth. . .
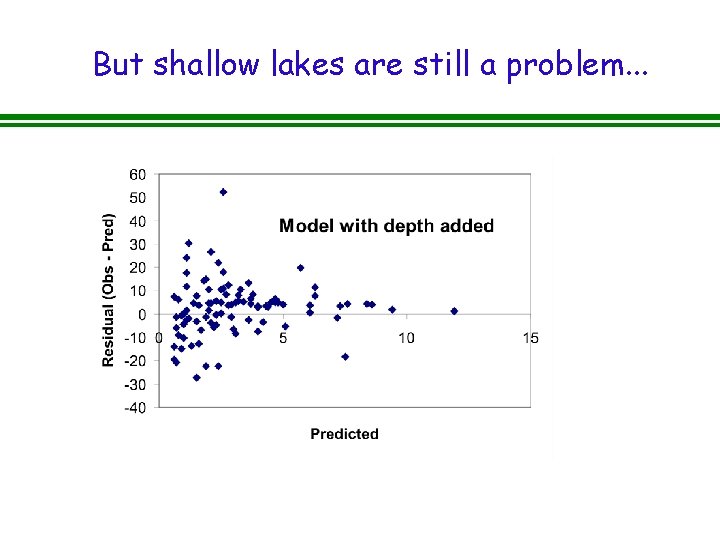
But shallow lakes are still a problem. . .
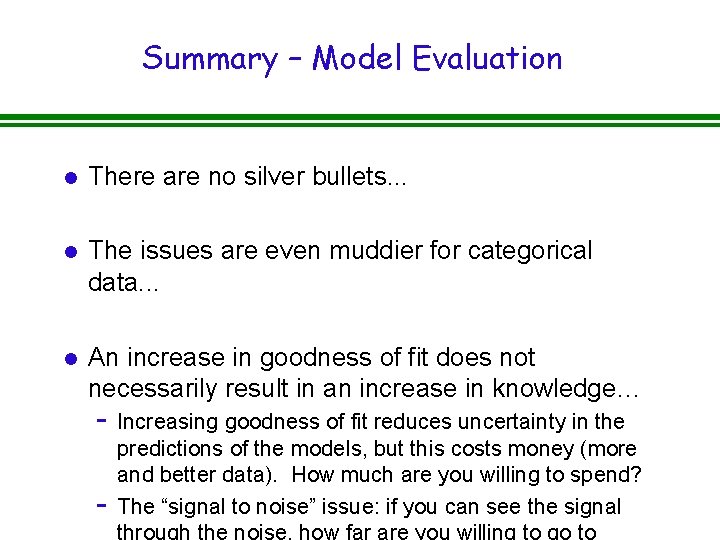
Summary – Model Evaluation l There are no silver bullets. . . l The issues are even muddier for categorical data. . . l An increase in goodness of fit does not necessarily result in an increase in knowledge… - Increasing goodness of fit reduces uncertainty in the - predictions of the models, but this costs money (more and better data). How much are you willing to spend? The “signal to noise” issue: if you can see the signal
01:640:244 lecture notes - lecture 15: plat, idah, farad
Elements of evaluation process
Osi model lecture
Literary technical and performance elements of drama
Elements and sub elements
Molecular element
Http //elements.wlonk.com/elements table.htm
Elements in a folktale
What are the literary elements of a folktale
Marzano elements
Rusbult's investment theory
Marzano teacher evaluation model 2018
Difference between formative and summative assessment
Define curriculum evaluation
Two process model of phobias evaluation
Cipp evaluation model
Stronge teacher evaluation
Flashlight triad model of evaluation
Multi store model of memory
Cipp evaluation model
Brinkerhoff's six-stage model of evaluation
Model evaluation and selection
Regression model evaluation
Stronge leader effectiveness performance evaluation model
Met model
Model evaluation metrics
Model evaluation
Marzano teacher evaluation model
Performance evaluation model
Project procurement management lecture notes