Land surface temperature LST and its applications Darren
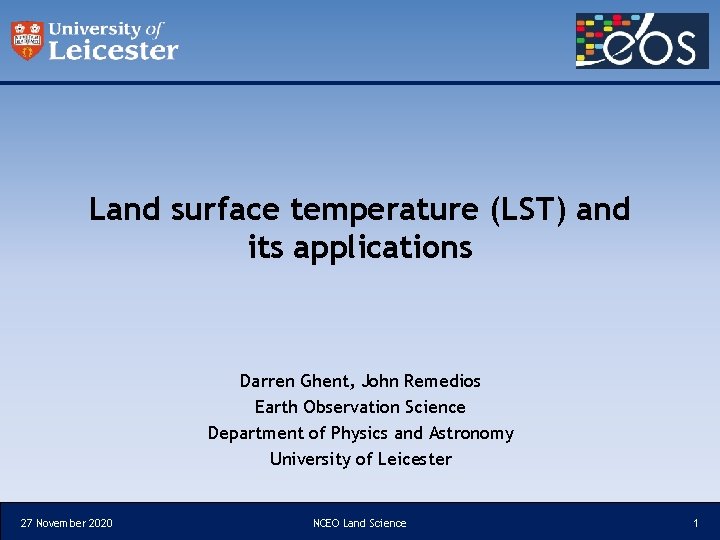
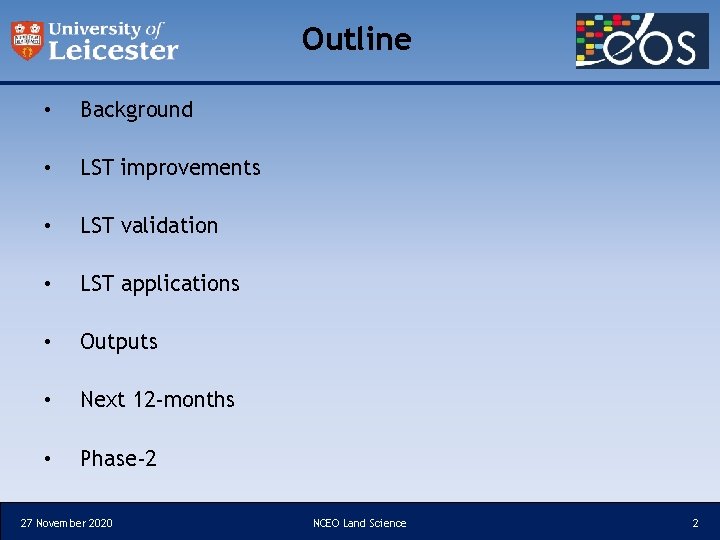
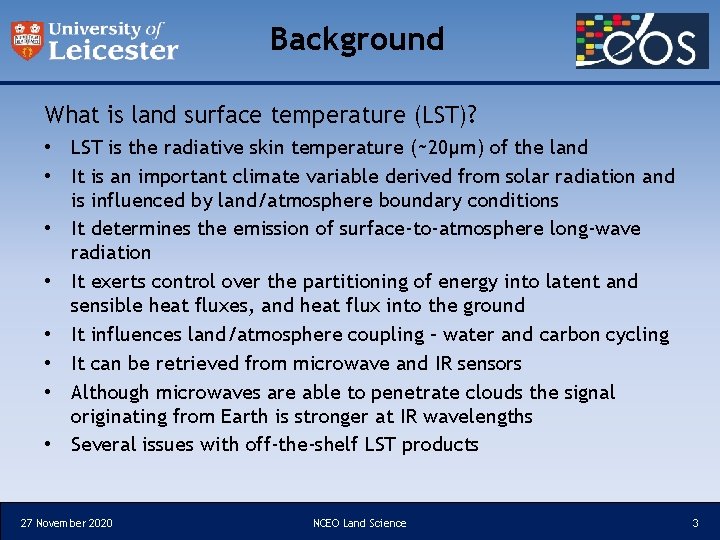
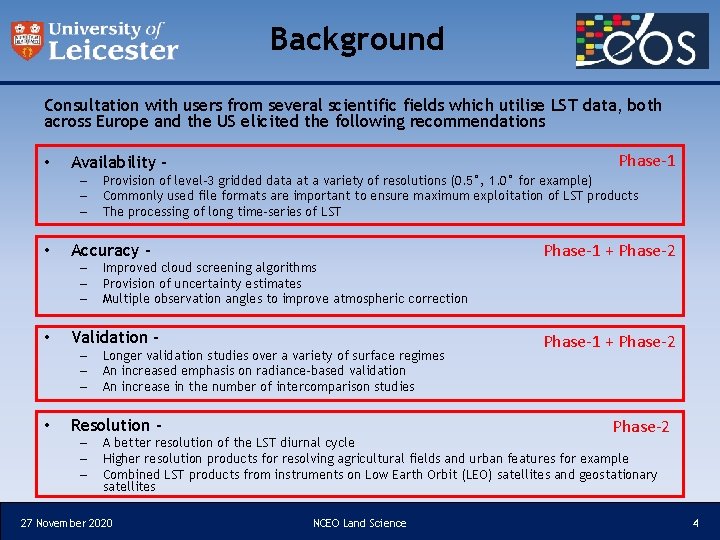
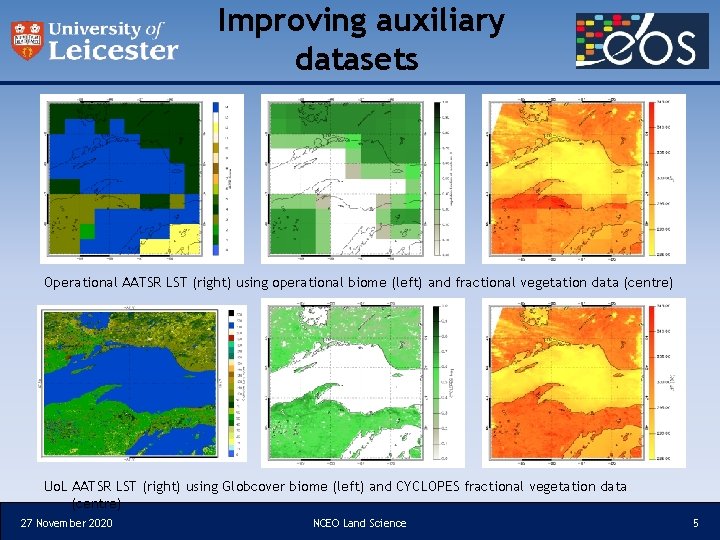
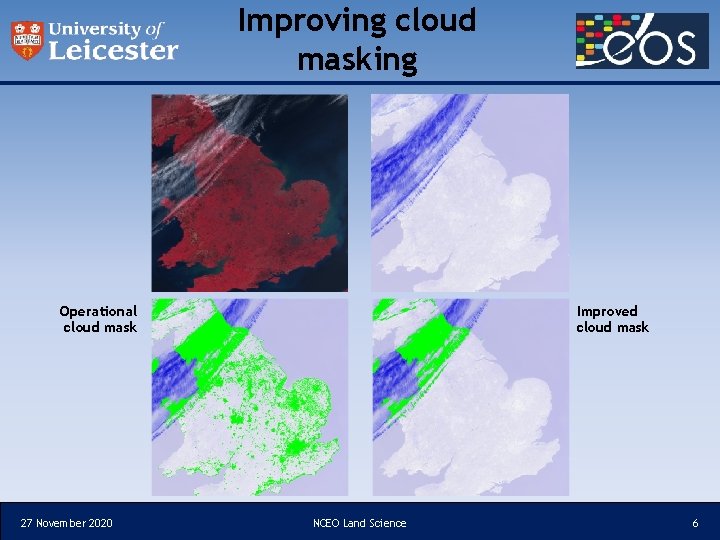
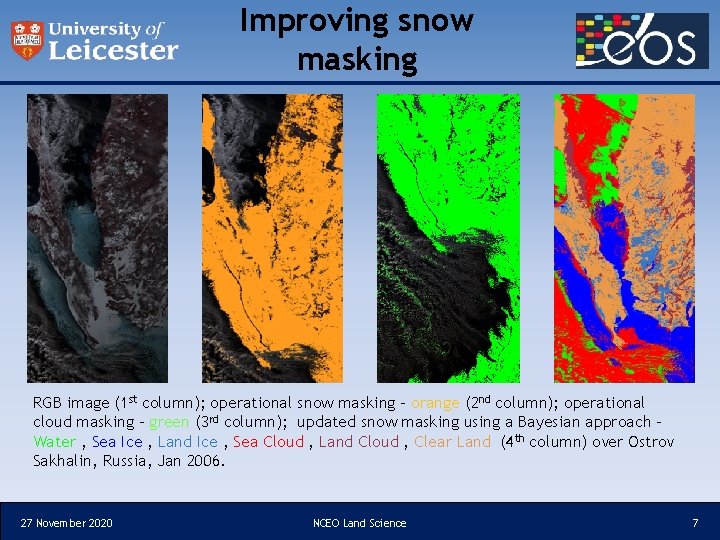
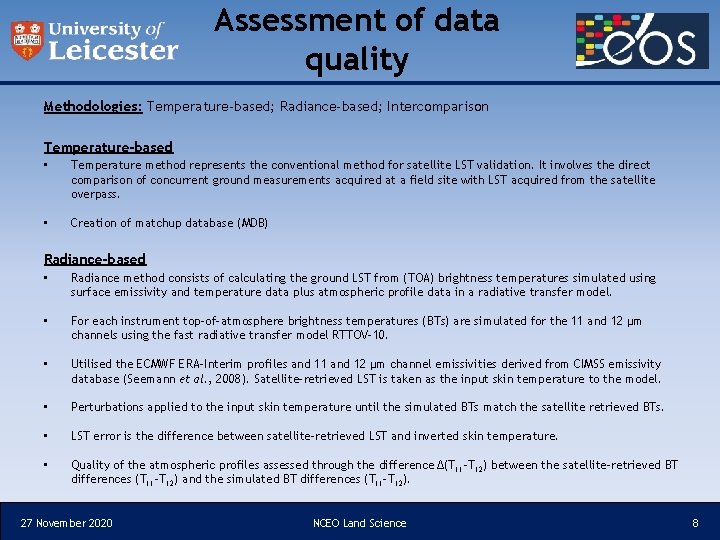
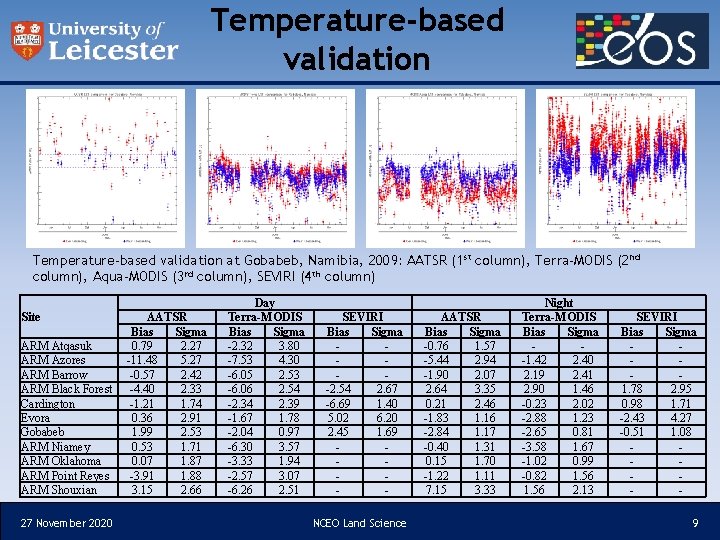
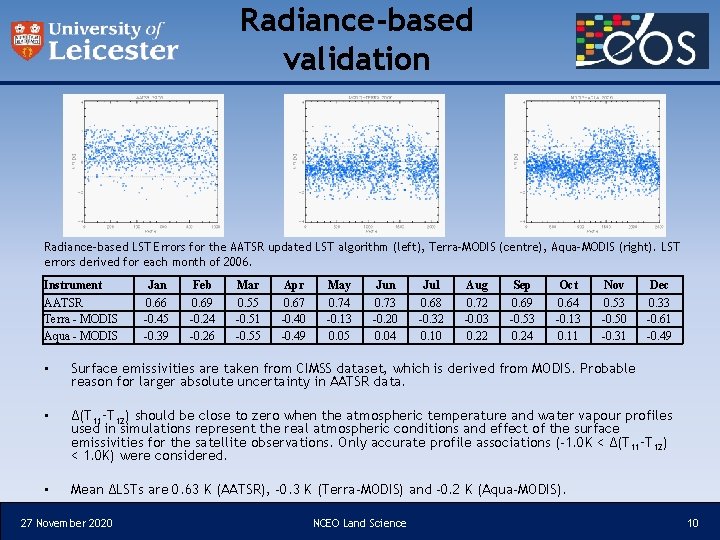
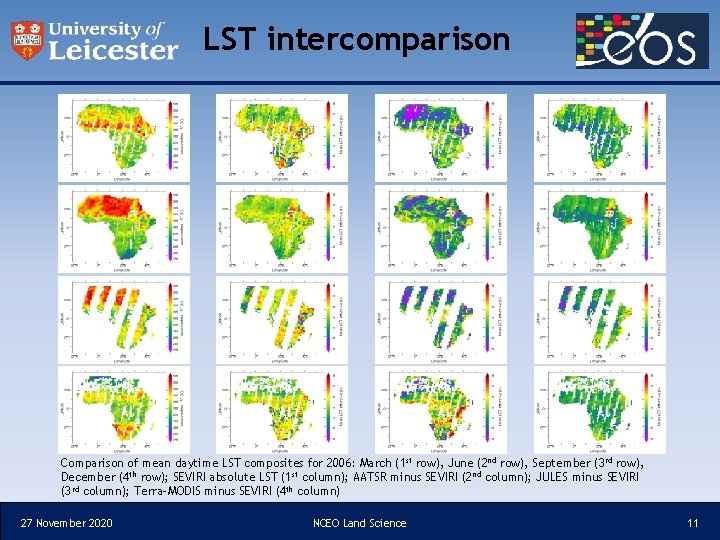
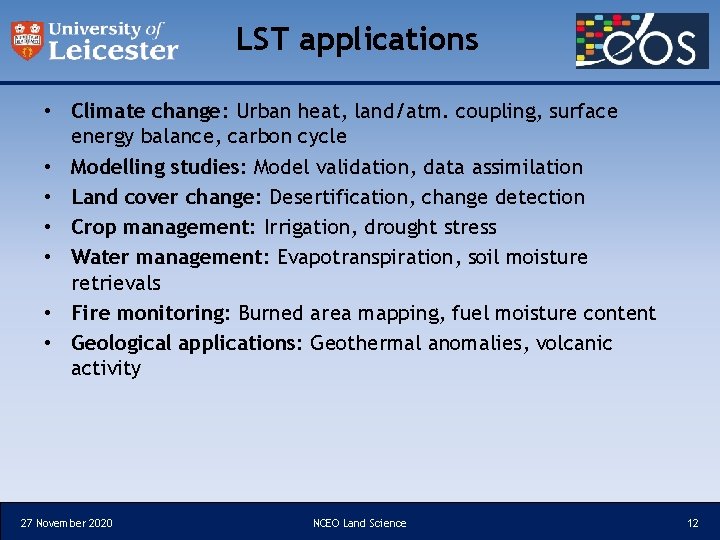
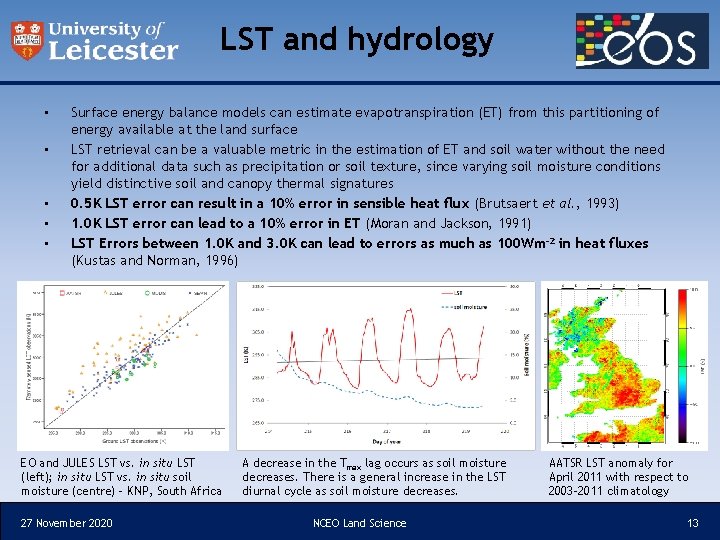
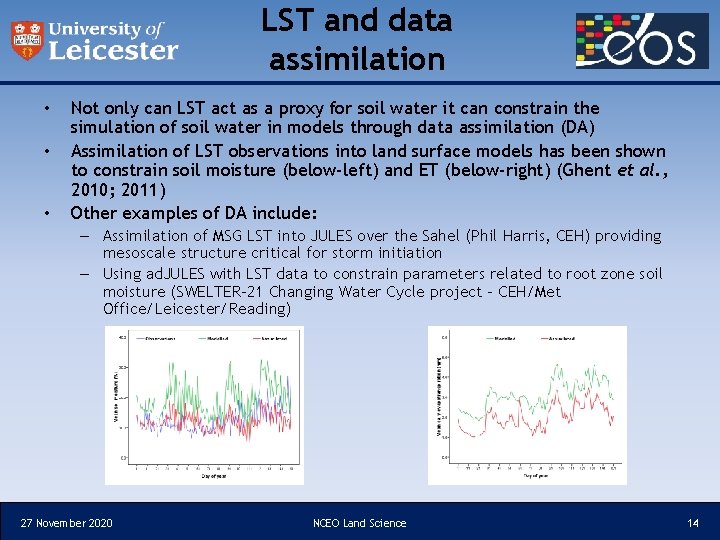
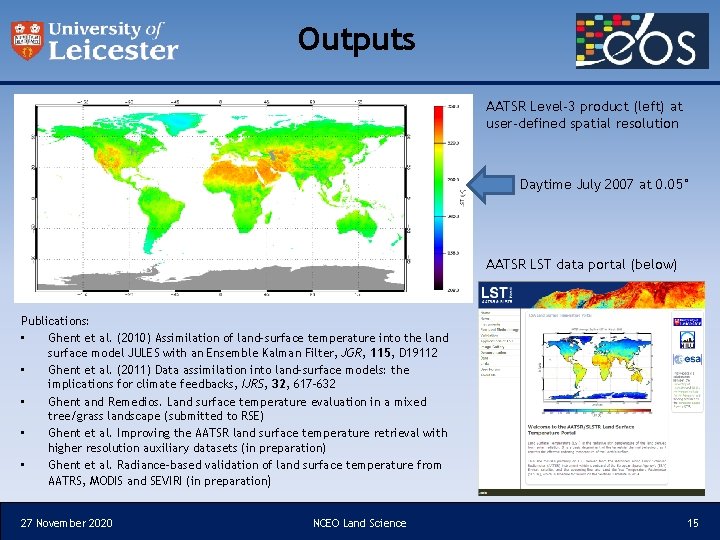
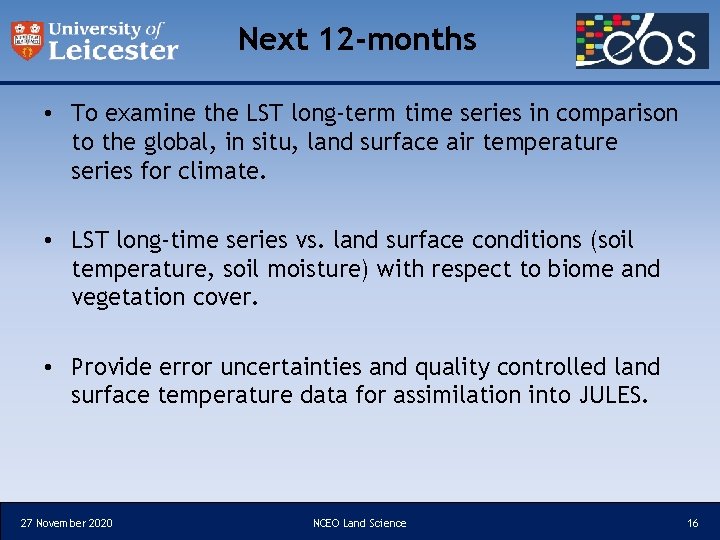
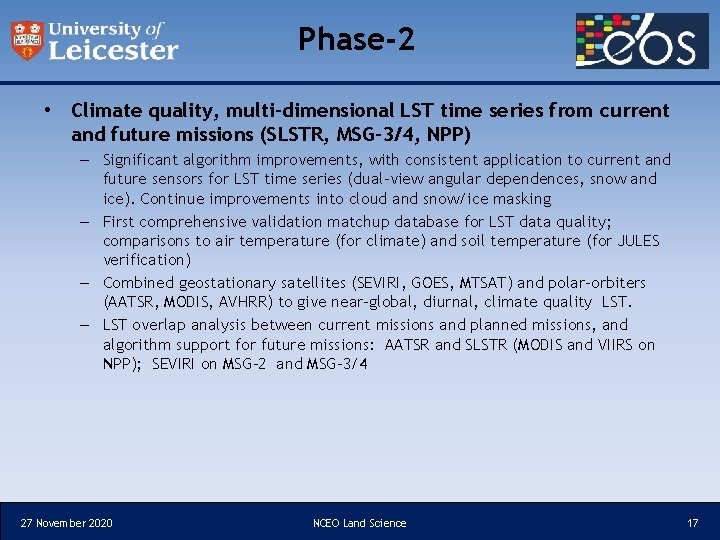
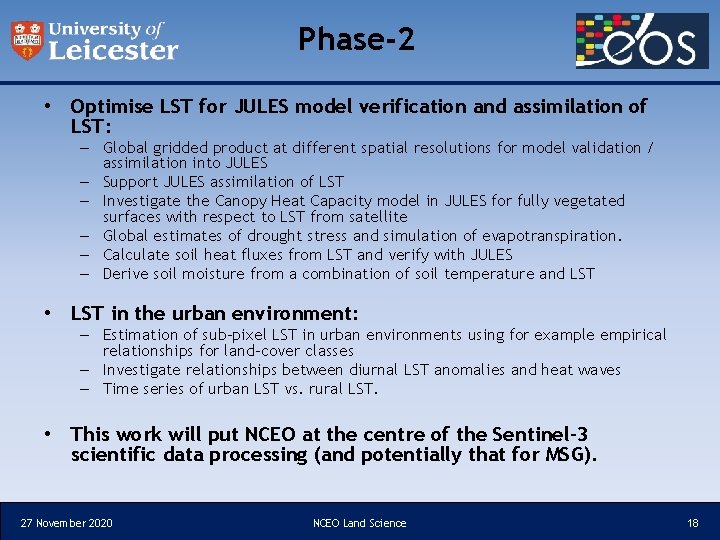
- Slides: 18
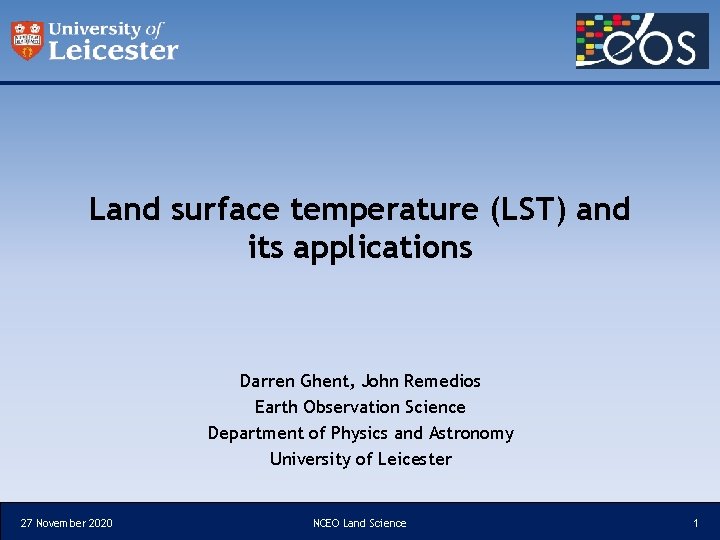
Land surface temperature (LST) and its applications Darren Ghent, John Remedios Earth Observation Science Department of Physics and Astronomy University of Leicester 27 November 2020 NCEO Land Science 1
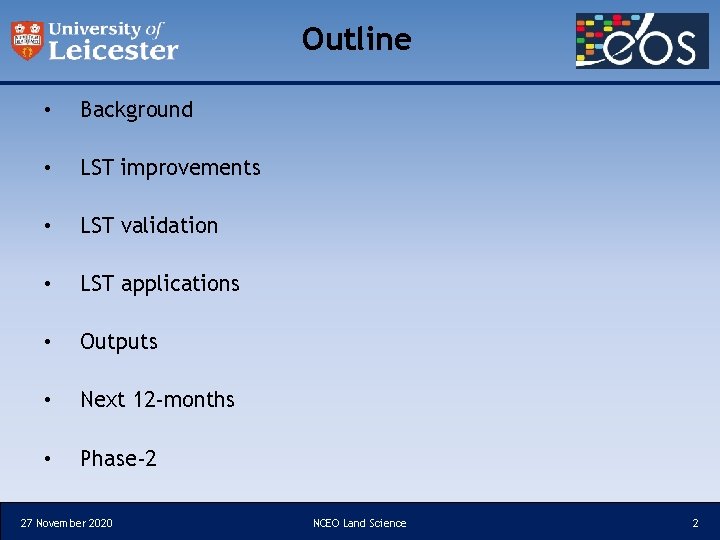
Outline • Background • LST improvements • LST validation • LST applications • Outputs • Next 12 -months • Phase-2 27 November 2020 NCEO Land Science 2
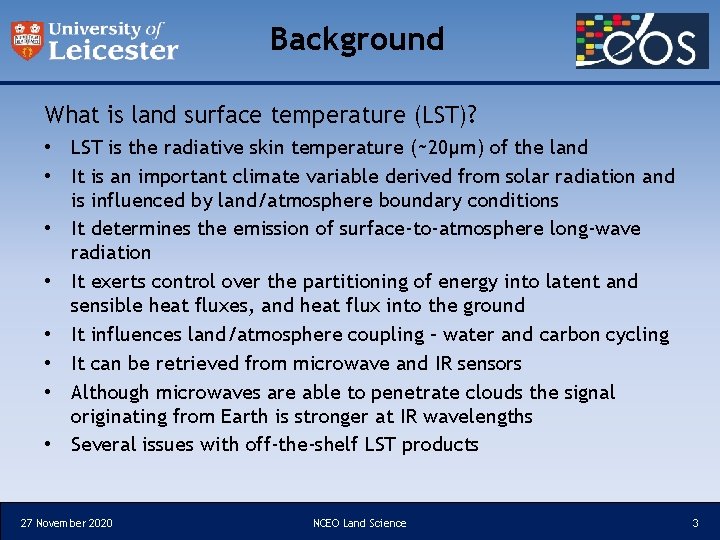
Background What is land surface temperature (LST)? • LST is the radiative skin temperature (~20μm) of the land • It is an important climate variable derived from solar radiation and is influenced by land/atmosphere boundary conditions • It determines the emission of surface-to-atmosphere long-wave radiation • It exerts control over the partitioning of energy into latent and sensible heat fluxes, and heat flux into the ground • It influences land/atmosphere coupling – water and carbon cycling • It can be retrieved from microwave and IR sensors • Although microwaves are able to penetrate clouds the signal originating from Earth is stronger at IR wavelengths • Several issues with off-the-shelf LST products 27 November 2020 NCEO Land Science 3
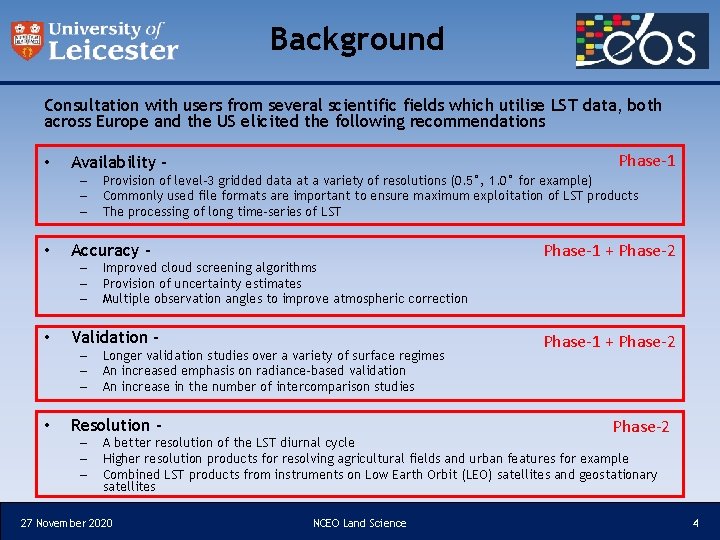
Background Consultation with users from several scientific fields which utilise LST data, both across Europe and the US elicited the following recommendations • – – – • Improved cloud screening algorithms Provision of uncertainty estimates Multiple observation angles to improve atmospheric correction Validation – – • Provision of level-3 gridded data at a variety of resolutions (0. 5°, 1. 0° for example) Commonly used file formats are important to ensure maximum exploitation of LST products The processing of long time-series of LST Accuracy – – • Phase-1 Availability – Longer validation studies over a variety of surface regimes An increased emphasis on radiance-based validation An increase in the number of intercomparison studies Resolution – – Phase-1 + Phase-2 A better resolution of the LST diurnal cycle Higher resolution products for resolving agricultural fields and urban features for example Combined LST products from instruments on Low Earth Orbit (LEO) satellites and geostationary satellites 27 November 2020 NCEO Land Science 4
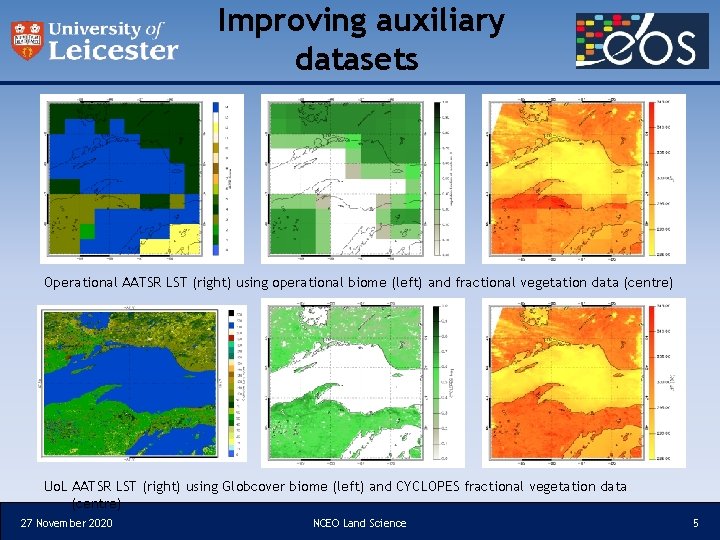
Improving auxiliary datasets Operational AATSR LST (right) using operational biome (left) and fractional vegetation data (centre) Uo. L AATSR LST (right) using Globcover biome (left) and CYCLOPES fractional vegetation data (centre) 27 November 2020 NCEO Land Science 5
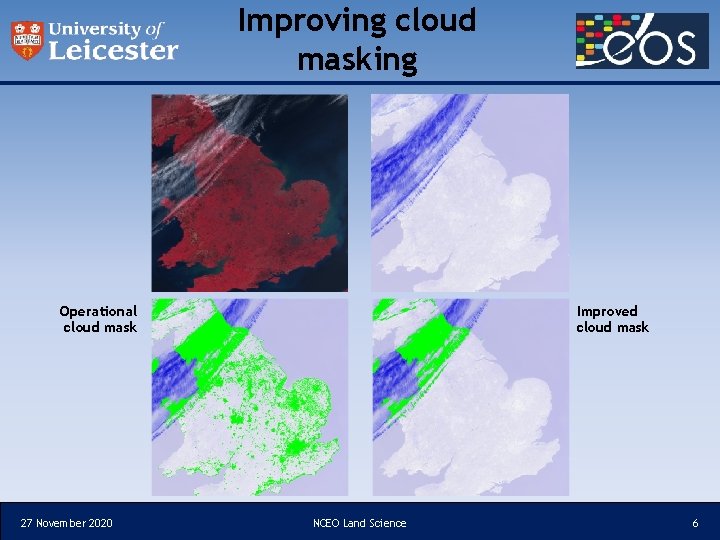
Improving cloud masking Operational cloud mask 27 November 2020 Improved cloud mask NCEO Land Science 6
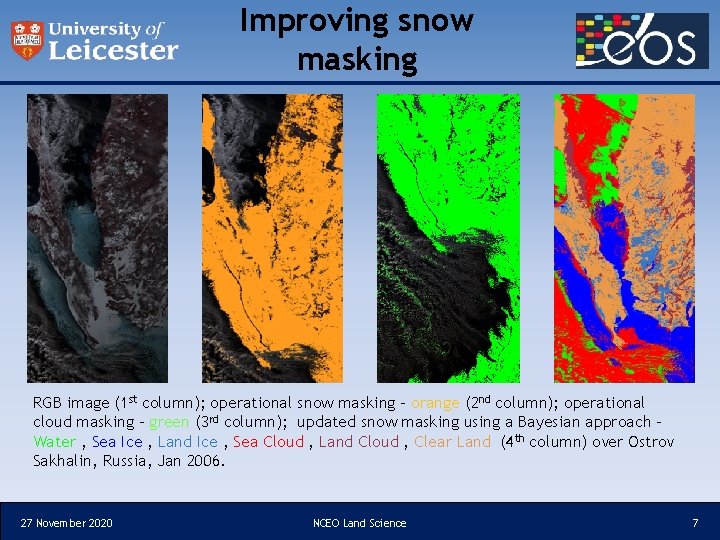
Improving snow masking RGB image (1 st column); operational snow masking – orange (2 nd column); operational cloud masking – green (3 rd column); updated snow masking using a Bayesian approach Water , Sea Ice , Land Ice , Sea Cloud , Land Cloud , Clear Land (4 th column) over Ostrov Sakhalin, Russia, Jan 2006. 27 November 2020 NCEO Land Science 7
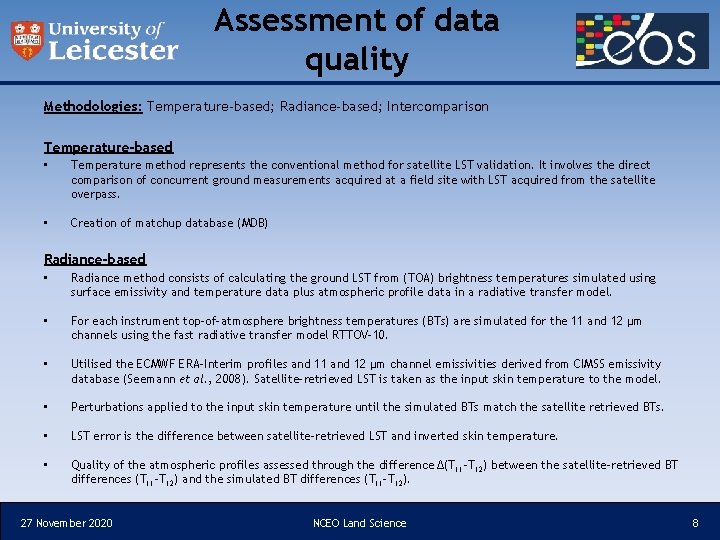
Assessment of data quality Methodologies: Temperature-based; Radiance-based; Intercomparison Temperature-based • Temperature method represents the conventional method for satellite LST validation. It involves the direct comparison of concurrent ground measurements acquired at a field site with LST acquired from the satellite overpass. • Creation of matchup database (MDB) Radiance-based • Radiance method consists of calculating the ground LST from (TOA) brightness temperatures simulated using surface emissivity and temperature data plus atmospheric profile data in a radiative transfer model. • For each instrument top-of-atmosphere brightness temperatures (BTs) are simulated for the 11 and 12 μm channels using the fast radiative transfer model RTTOV-10. • Utilised the ECMWF ERA-Interim profiles and 11 and 12 μm channel emissivities derived from CIMSS emissivity database (Seemann et al. , 2008). Satellite-retrieved LST is taken as the input skin temperature to the model. • Perturbations applied to the input skin temperature until the simulated BTs match the satellite retrieved BTs. • LST error is the difference between satellite-retrieved LST and inverted skin temperature. • Quality of the atmospheric profiles assessed through the difference Δ(T 11–T 12) between the satellite-retrieved BT differences (T 11–T 12) and the simulated BT differences (T 11–T 12). 27 November 2020 NCEO Land Science 8
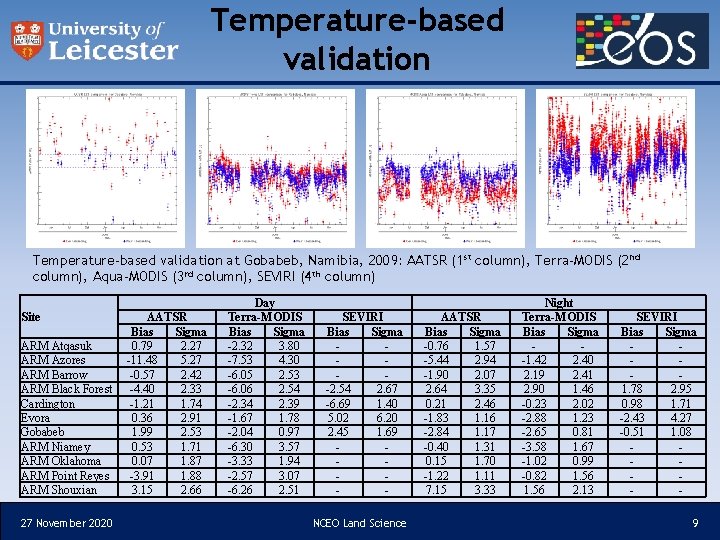
Temperature-based validation at Gobabeb, Namibia, 2009: AATSR (1 st column), Terra-MODIS (2 nd column), Aqua-MODIS (3 rd column), SEVIRI (4 th column) Site ARM Atqasuk ARM Azores ARM Barrow ARM Black Forest Cardington Evora Gobabeb ARM Niamey ARM Oklahoma ARM Point Reyes ARM Shouxian 27 November 2020 AATSR Bias Sigma 0. 79 2. 27 -11. 48 5. 27 -0. 57 2. 42 -4. 40 2. 33 -1. 21 1. 74 0. 36 2. 91 1. 99 2. 53 0. 53 1. 71 0. 07 1. 87 -3. 91 1. 88 3. 15 2. 66 Day Terra-MODIS Bias Sigma -2. 32 3. 80 -7. 53 4. 30 -6. 05 2. 53 -6. 06 2. 54 -2. 34 2. 39 -1. 67 1. 78 -2. 04 0. 97 -6. 30 3. 57 -3. 33 1. 94 -2. 57 3. 07 -6. 26 2. 51 SEVIRI Bias Sigma -2. 54 2. 67 -6. 69 1. 40 5. 02 6. 20 2. 45 1. 69 NCEO Land Science AATSR Bias Sigma -0. 76 1. 57 -5. 44 2. 94 -1. 90 2. 07 2. 64 3. 35 0. 21 2. 46 -1. 83 1. 16 -2. 84 1. 17 -0. 40 1. 31 0. 15 1. 70 -1. 22 1. 11 7. 15 3. 33 Night Terra-MODIS Bias Sigma -1. 42 2. 40 2. 19 2. 41 2. 90 1. 46 -0. 23 2. 02 -2. 88 1. 23 -2. 65 0. 81 -3. 58 1. 67 -1. 02 0. 99 -0. 82 1. 56 2. 13 SEVIRI Bias Sigma 1. 78 2. 95 0. 98 1. 71 -2. 43 4. 27 -0. 51 1. 08 9
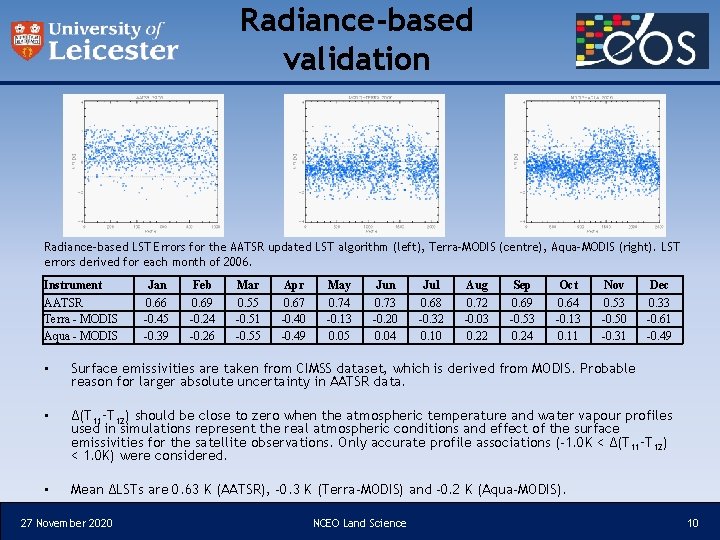
Radiance-based validation Radiance-based LST Errors for the AATSR updated LST algorithm (left), Terra-MODIS (centre), Aqua-MODIS (right). LST errors derived for each month of 2006. Instrument AATSR Terra - MODIS Aqua - MODIS Jan 0. 66 -0. 45 -0. 39 Feb 0. 69 -0. 24 -0. 26 Mar 0. 55 -0. 51 -0. 55 Apr 0. 67 -0. 40 -0. 49 May 0. 74 -0. 13 0. 05 Jun 0. 73 -0. 20 0. 04 Jul 0. 68 -0. 32 0. 10 Aug 0. 72 -0. 03 0. 22 Sep 0. 69 -0. 53 0. 24 Oct 0. 64 -0. 13 0. 11 Nov 0. 53 -0. 50 -0. 31 Dec 0. 33 -0. 61 -0. 49 • Surface emissivities are taken from CIMSS dataset, which is derived from MODIS. Probable reason for larger absolute uncertainty in AATSR data. • Δ(T 11–T 12) should be close to zero when the atmospheric temperature and water vapour profiles used in simulations represent the real atmospheric conditions and effect of the surface emissivities for the satellite observations. Only accurate profile associations (-1. 0 K < Δ(T 11–T 12) < 1. 0 K) were considered. • Mean ΔLSTs are 0. 63 K (AATSR), -0. 3 K (Terra-MODIS) and -0. 2 K (Aqua-MODIS). 27 November 2020 NCEO Land Science 10
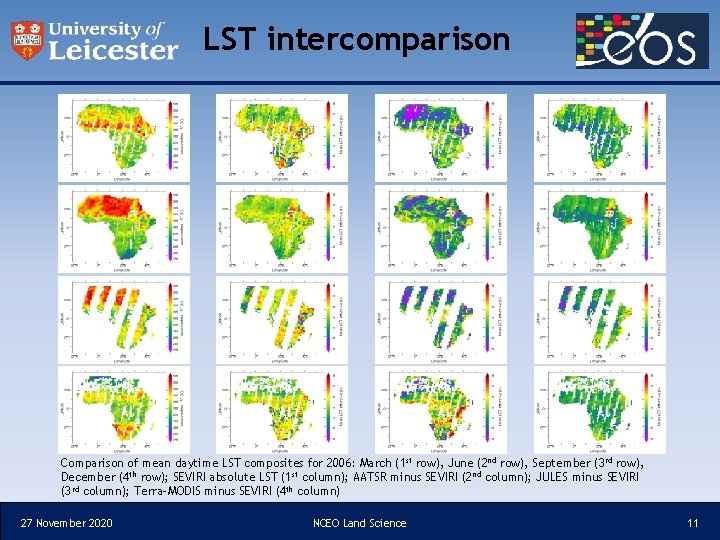
LST intercomparison Comparison of mean daytime LST composites for 2006: March (1 st row), June (2 nd row), September (3 rd row), December (4 th row); SEVIRI absolute LST (1 st column); AATSR minus SEVIRI (2 nd column); JULES minus SEVIRI (3 rd column); Terra-MODIS minus SEVIRI (4 th column) 27 November 2020 NCEO Land Science 11
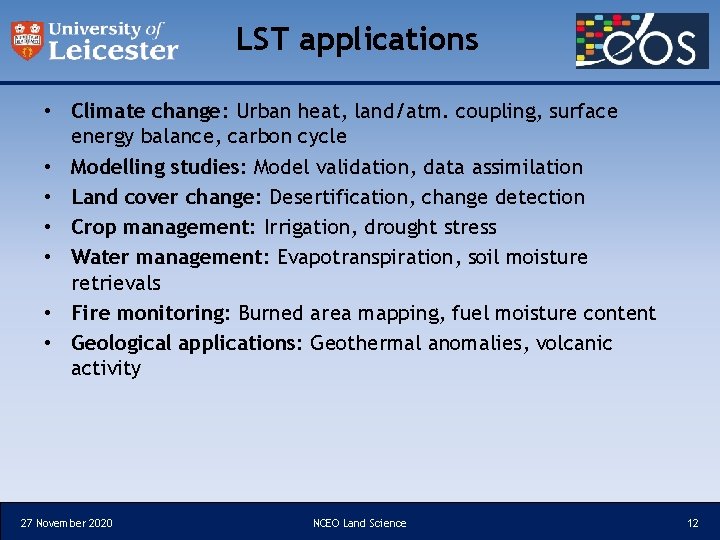
LST applications • Climate change: Urban heat, land/atm. coupling, surface energy balance, carbon cycle • Modelling studies: Model validation, data assimilation • Land cover change: Desertification, change detection • Crop management: Irrigation, drought stress • Water management: Evapotranspiration, soil moisture retrievals • Fire monitoring: Burned area mapping, fuel moisture content • Geological applications: Geothermal anomalies, volcanic activity 27 November 2020 NCEO Land Science 12
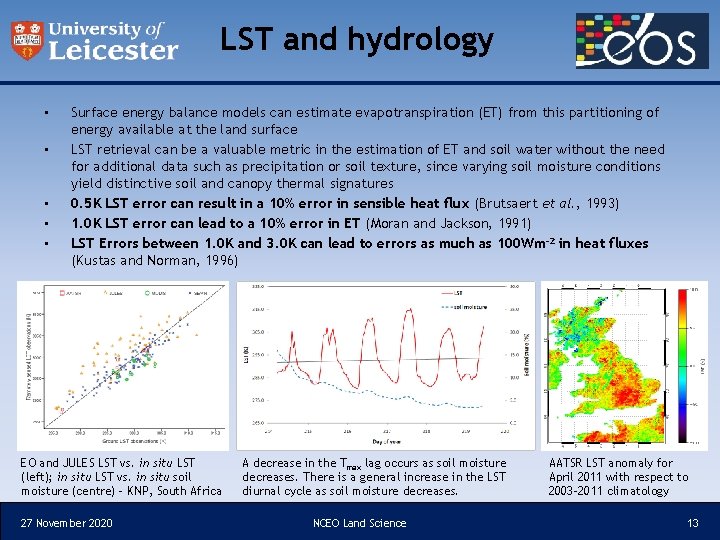
LST and hydrology • • • Surface energy balance models can estimate evapotranspiration (ET) from this partitioning of energy available at the land surface LST retrieval can be a valuable metric in the estimation of ET and soil water without the need for additional data such as precipitation or soil texture, since varying soil moisture conditions yield distinctive soil and canopy thermal signatures 0. 5 K LST error can result in a 10% error in sensible heat flux (Brutsaert et al. , 1993) 1. 0 K LST error can lead to a 10% error in ET (Moran and Jackson, 1991) LST Errors between 1. 0 K and 3. 0 K can lead to errors as much as 100 Wm -2 in heat fluxes (Kustas and Norman, 1996) EO and JULES LST vs. in situ LST (left); in situ LST vs. in situ soil moisture (centre) - KNP, South Africa 27 November 2020 A decrease in the Tmax lag occurs as soil moisture decreases. There is a general increase in the LST diurnal cycle as soil moisture decreases. NCEO Land Science AATSR LST anomaly for April 2011 with respect to 2003 -2011 climatology 13
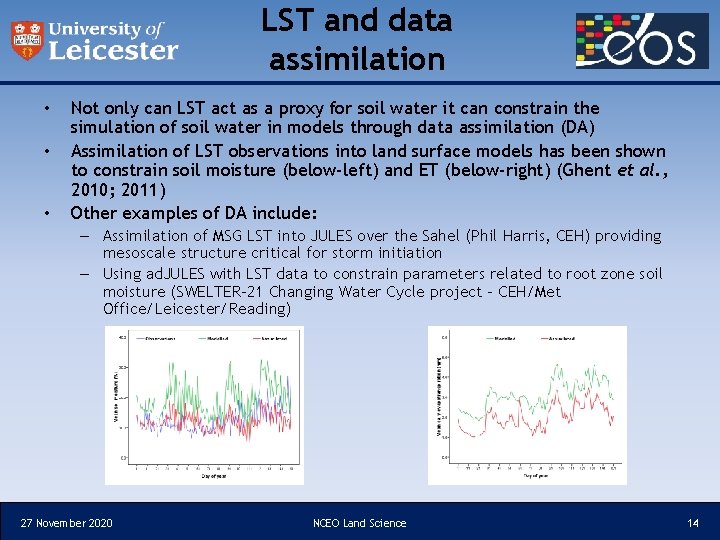
LST and data assimilation • • • Not only can LST act as a proxy for soil water it can constrain the simulation of soil water in models through data assimilation (DA) Assimilation of LST observations into land surface models has been shown to constrain soil moisture (below-left) and ET (below-right) (Ghent et al. , 2010; 2011) Other examples of DA include: – Assimilation of MSG LST into JULES over the Sahel (Phil Harris, CEH) providing mesoscale structure critical for storm initiation – Using ad. JULES with LST data to constrain parameters related to root zone soil moisture (SWELTER-21 Changing Water Cycle project - CEH/Met Office/Leicester/Reading) 27 November 2020 NCEO Land Science 14
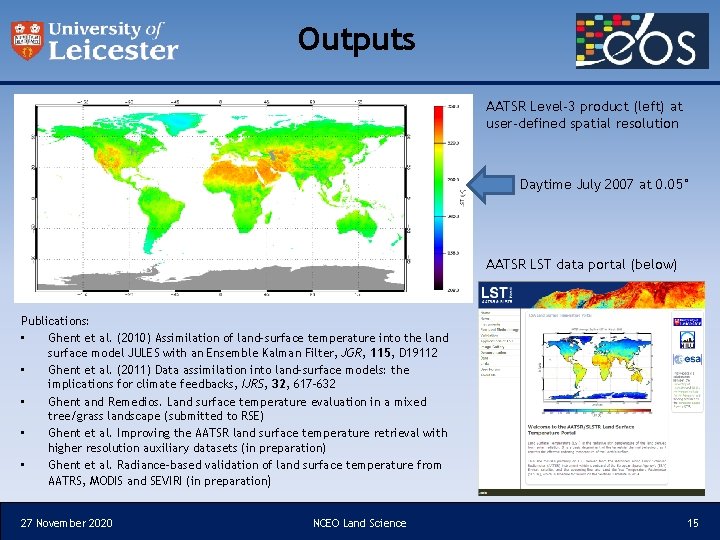
Outputs AATSR Level-3 product (left) at user-defined spatial resolution Daytime July 2007 at 0. 05° AATSR LST data portal (below) Publications: • Ghent et al. (2010) Assimilation of land-surface temperature into the land surface model JULES with an Ensemble Kalman Filter, JGR, 115, D 19112 • Ghent et al. (2011) Data assimilation into land-surface models: the implications for climate feedbacks, IJRS, 32, 617 -632 • Ghent and Remedios. Land surface temperature evaluation in a mixed tree/grass landscape (submitted to RSE) • Ghent et al. Improving the AATSR land surface temperature retrieval with higher resolution auxiliary datasets (in preparation) • Ghent et al. Radiance-based validation of land surface temperature from AATRS, MODIS and SEVIRI (in preparation) 27 November 2020 NCEO Land Science 15
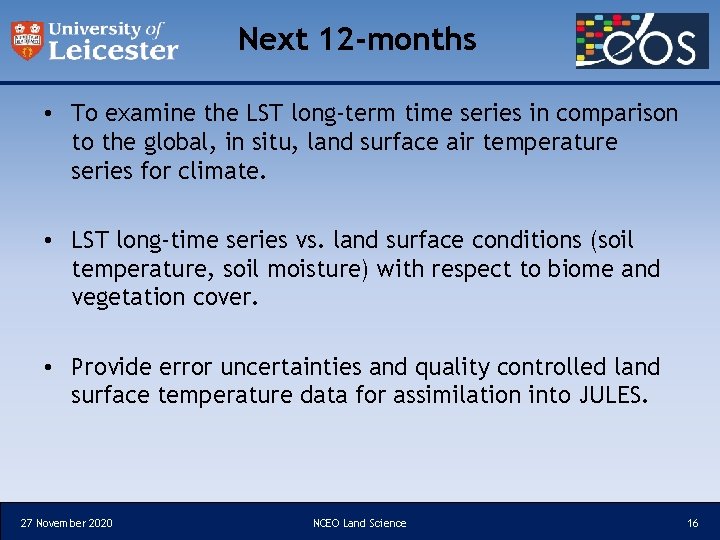
Next 12 -months • To examine the LST long-term time series in comparison to the global, in situ, land surface air temperature series for climate. • LST long-time series vs. land surface conditions (soil temperature, soil moisture) with respect to biome and vegetation cover. • Provide error uncertainties and quality controlled land surface temperature data for assimilation into JULES. 27 November 2020 NCEO Land Science 16
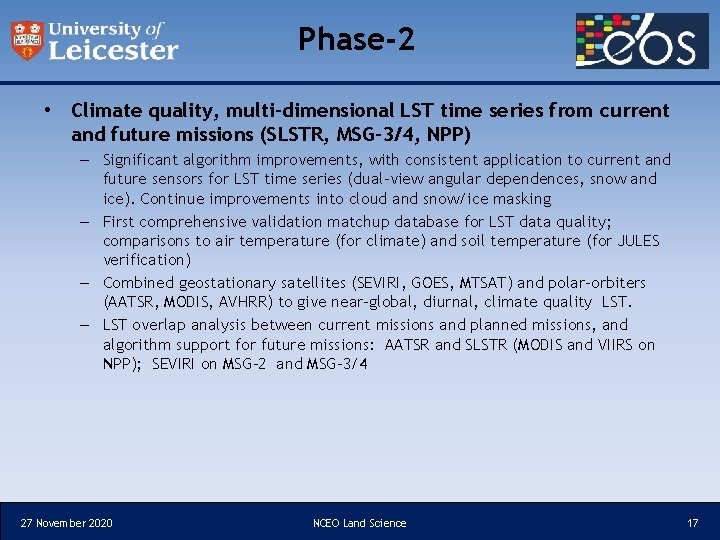
Phase-2 • Climate quality, multi-dimensional LST time series from current and future missions (SLSTR, MSG-3/4, NPP) – Significant algorithm improvements, with consistent application to current and future sensors for LST time series (dual-view angular dependences, snow and ice). Continue improvements into cloud and snow/ice masking – First comprehensive validation matchup database for LST data quality; comparisons to air temperature (for climate) and soil temperature (for JULES verification) – Combined geostationary satellites (SEVIRI, GOES, MTSAT) and polar-orbiters (AATSR, MODIS, AVHRR) to give near-global, diurnal, climate quality LST. – LST overlap analysis between current missions and planned missions, and algorithm support for future missions: AATSR and SLSTR (MODIS and VIIRS on NPP); SEVIRI on MSG-2 and MSG-3/4 27 November 2020 NCEO Land Science 17
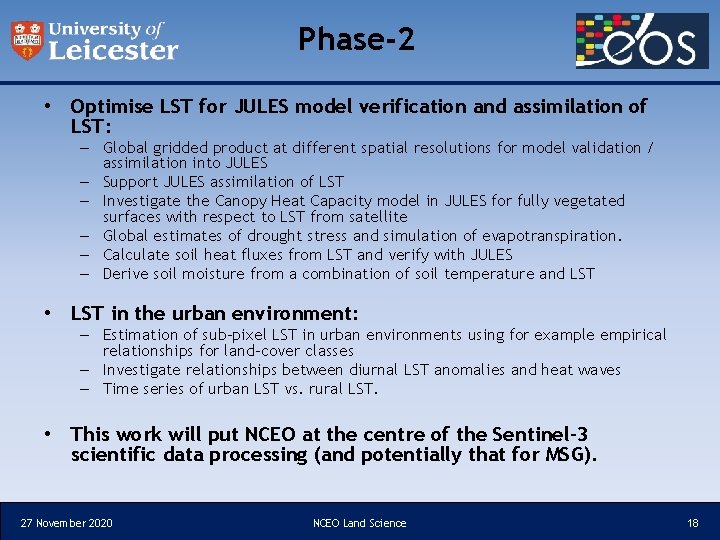
Phase-2 • Optimise LST for JULES model verification and assimilation of LST: – Global gridded product at different spatial resolutions for model validation / assimilation into JULES – Support JULES assimilation of LST – Investigate the Canopy Heat Capacity model in JULES for fully vegetated surfaces with respect to LST from satellite – Global estimates of drought stress and simulation of evapotranspiration. – Calculate soil heat fluxes from LST and verify with JULES – Derive soil moisture from a combination of soil temperature and LST • LST in the urban environment: – Estimation of sub-pixel LST in urban environments using for example empirical relationships for land-cover classes – Investigate relationships between diurnal LST anomalies and heat waves – Time series of urban LST vs. rural LST. • This work will put NCEO at the centre of the Sentinel-3 scientific data processing (and potentially that for MSG). 27 November 2020 NCEO Land Science 18
Total float= * 1 point lst-est lst-eft est-lft eft-lst
Est eft lst lft
Den lektiologiske grundmodel
Lst camera
Classificazione di parigi polipi
Lmu lst
Float activity
Ar-lst
Difference between curie temperature and neel temperature
Difference between curie temperature and neel temperature
Ferrimagnetism
What is a cro
The fourier transform and its applications
Daniel spielman spectral graph theory
A brief introduction to spectral graph theory
Diagonalizable matrix
Dimensional analysis and its applications
Fast random walk with restart and its applications
Z effective of chlorine