Is this quarter fair Is this quarter fair
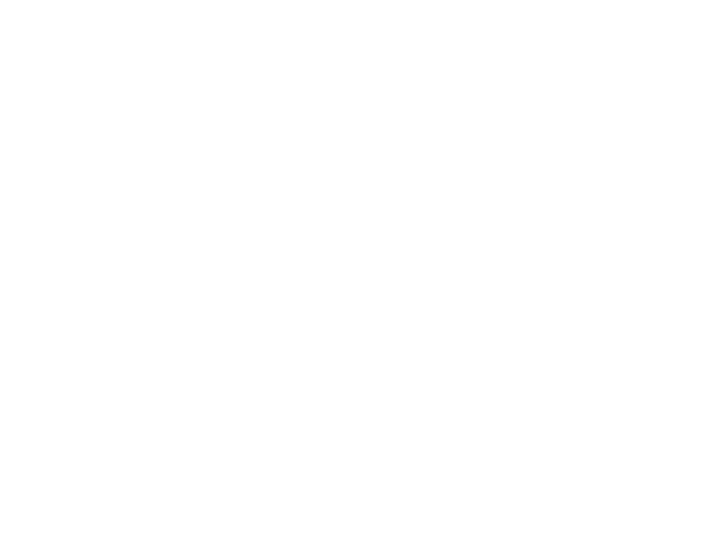
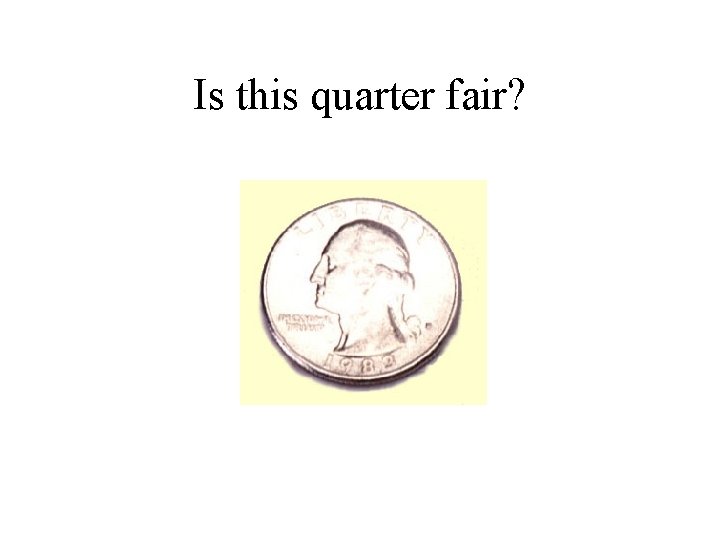
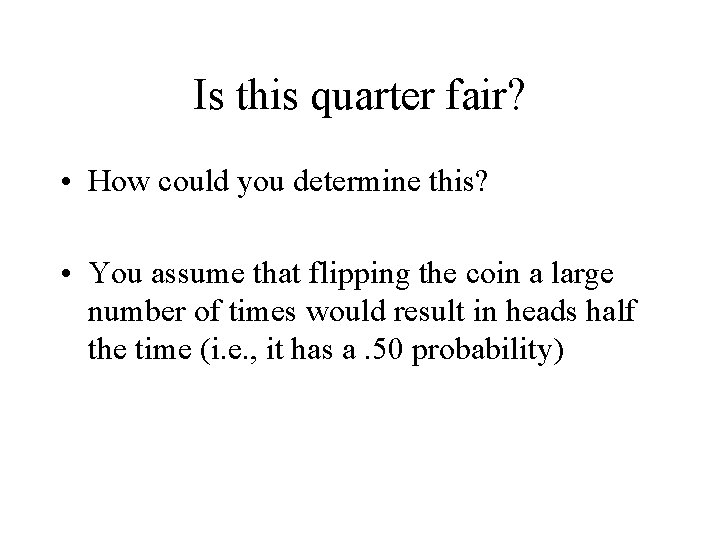
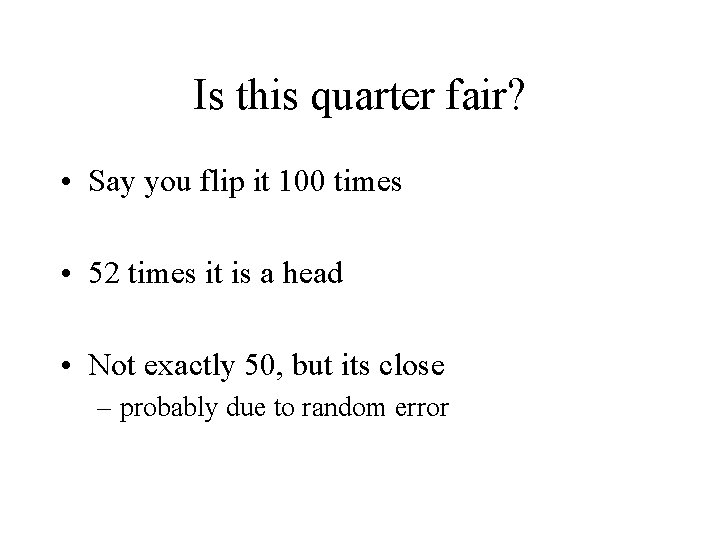
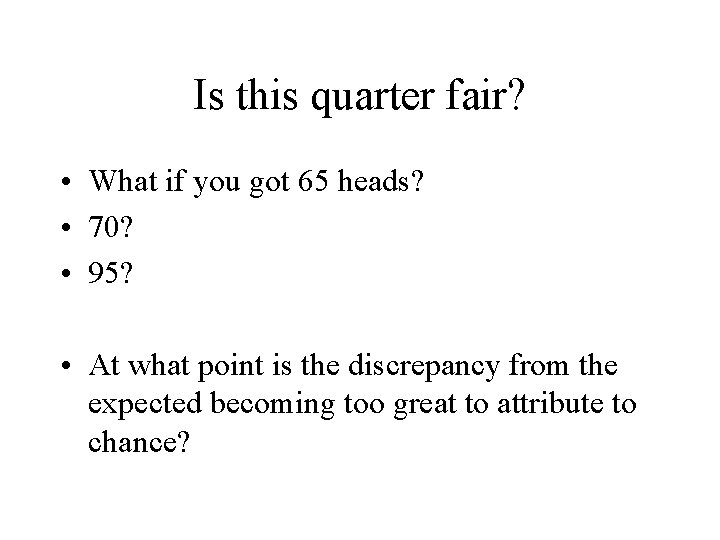
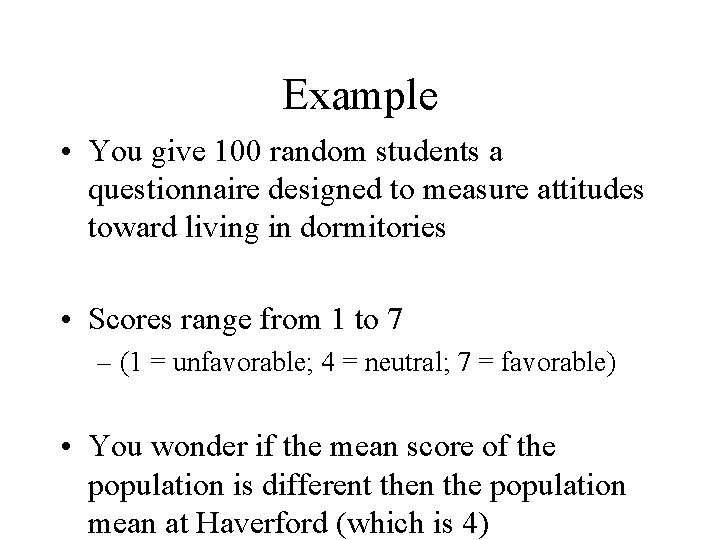
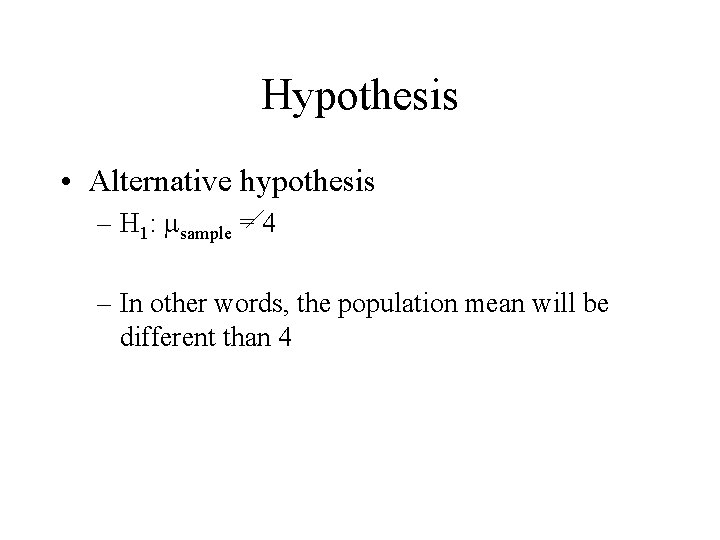
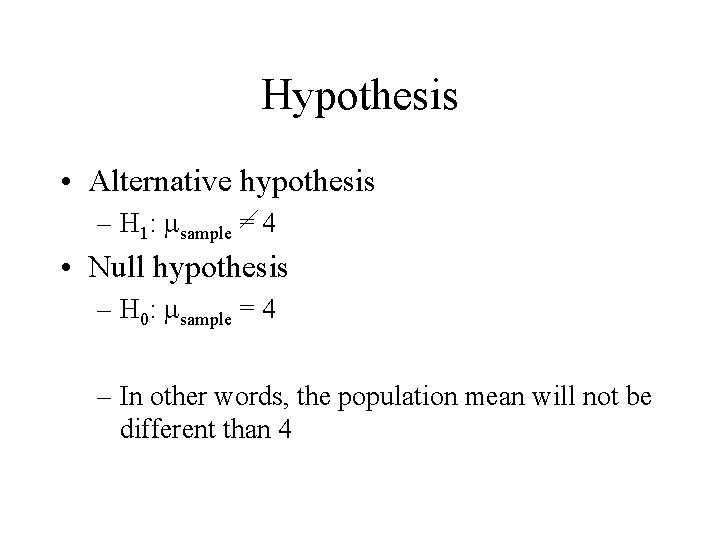
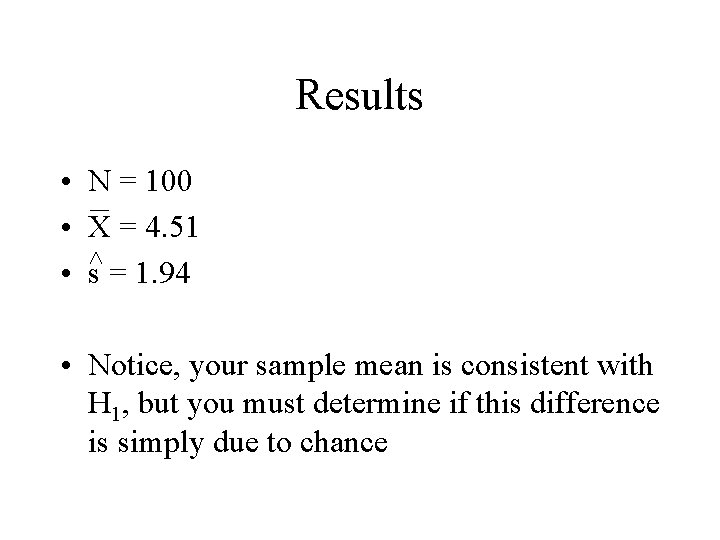
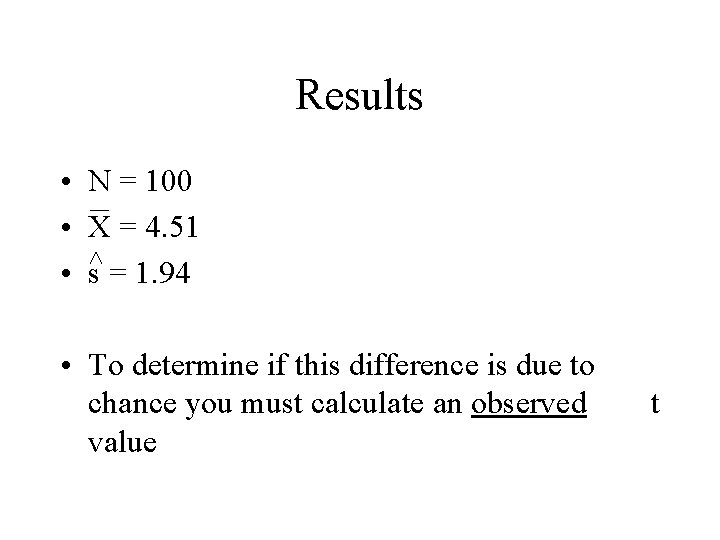
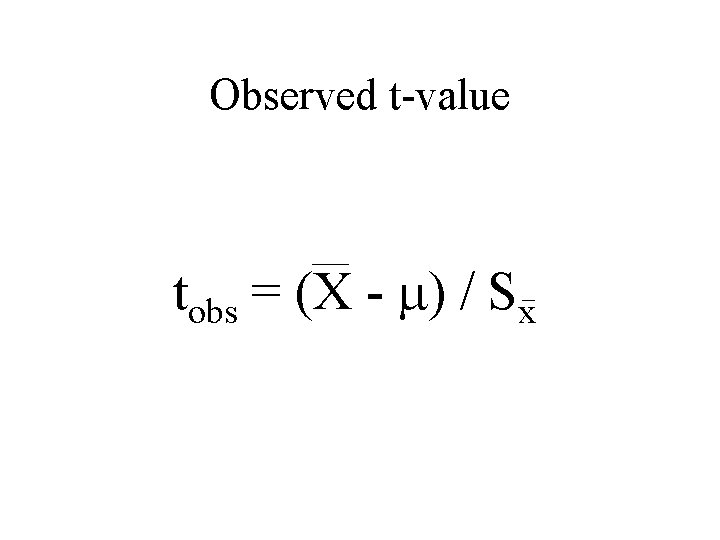
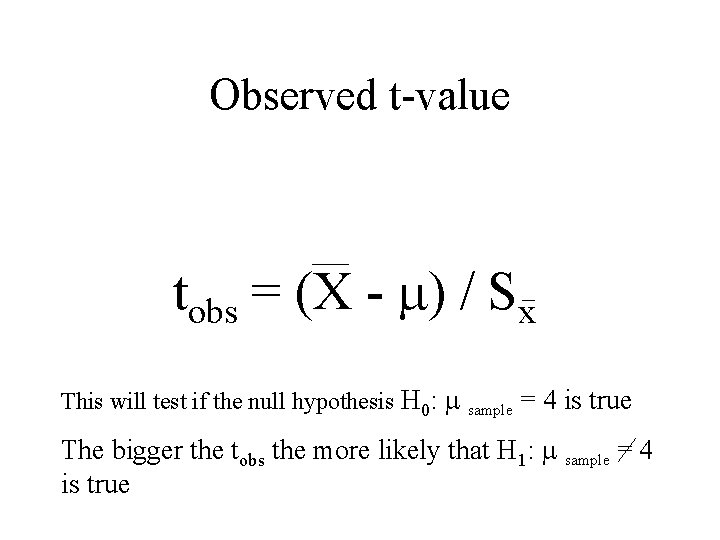
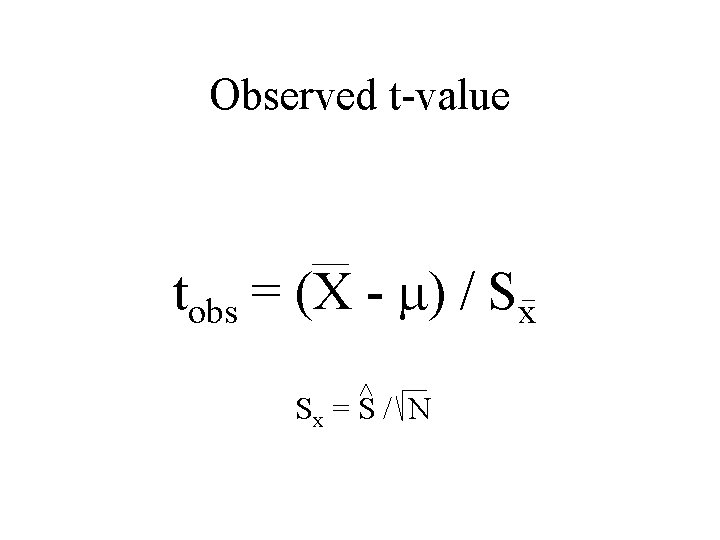
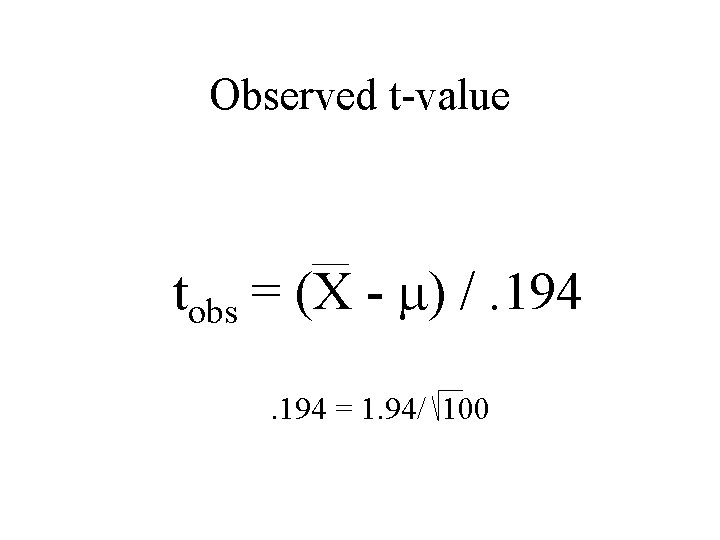
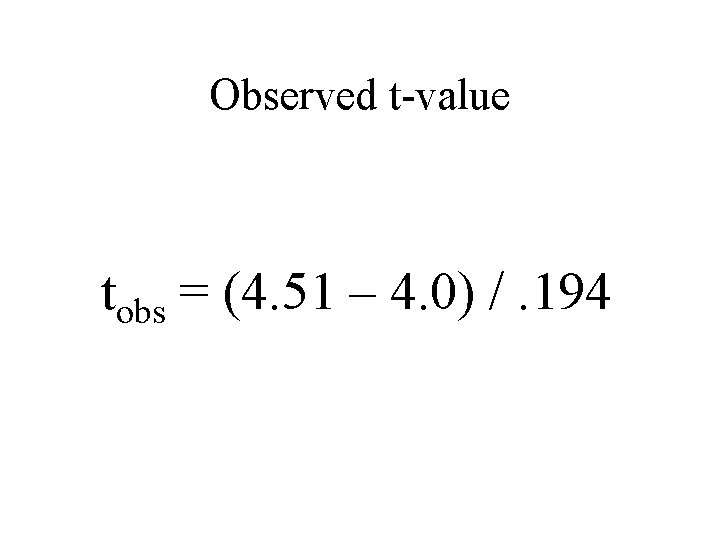
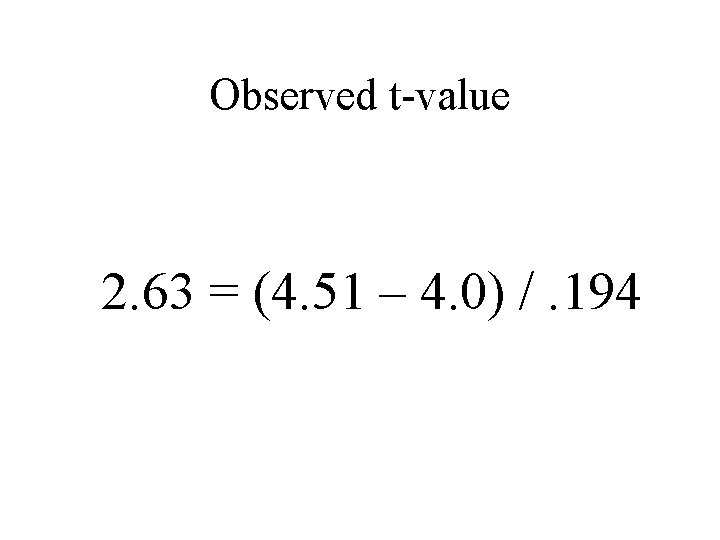
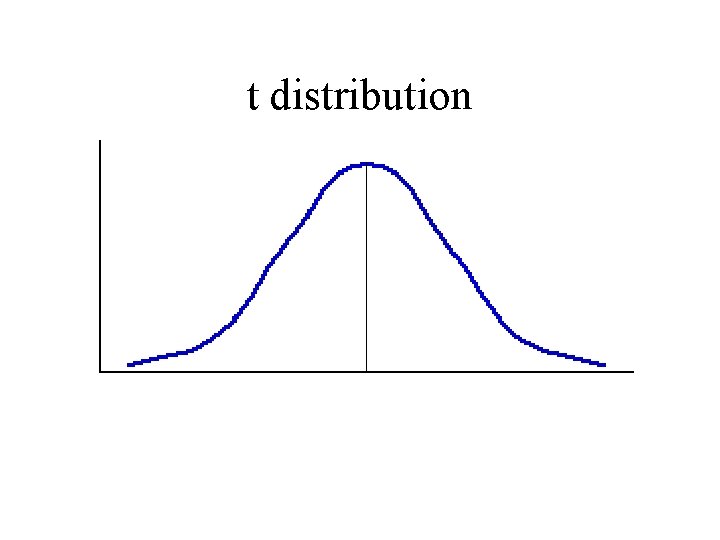
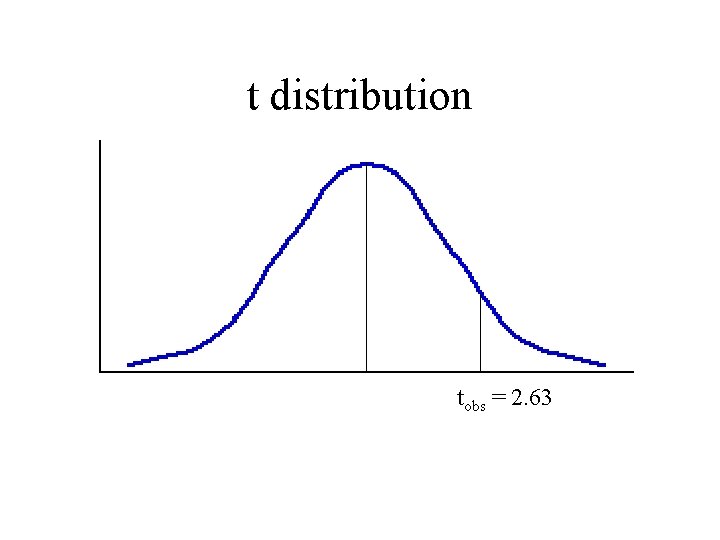
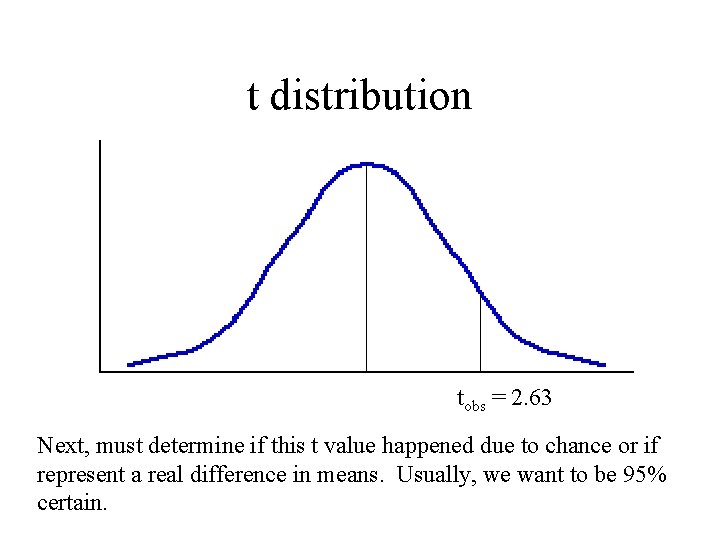
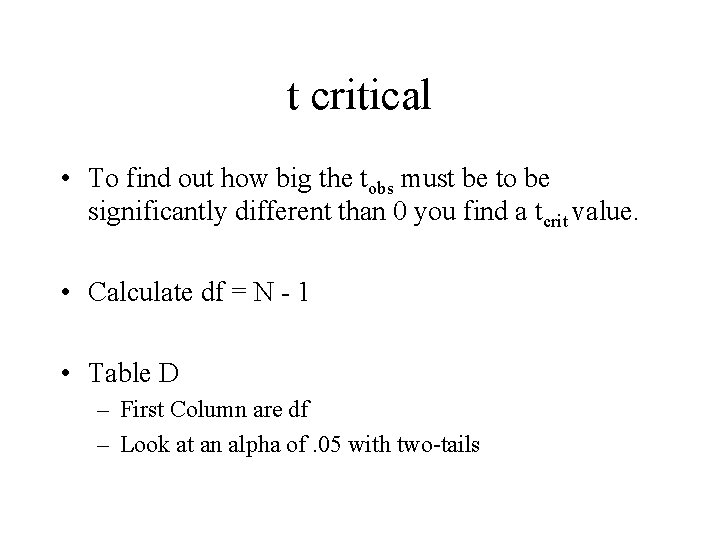
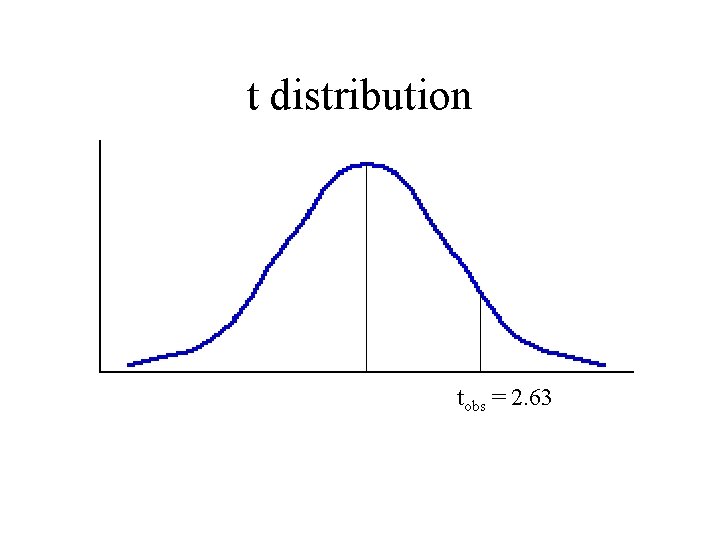
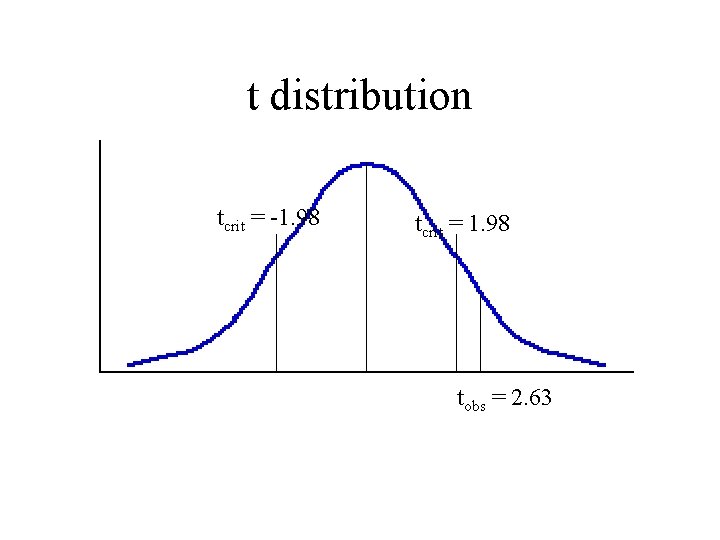
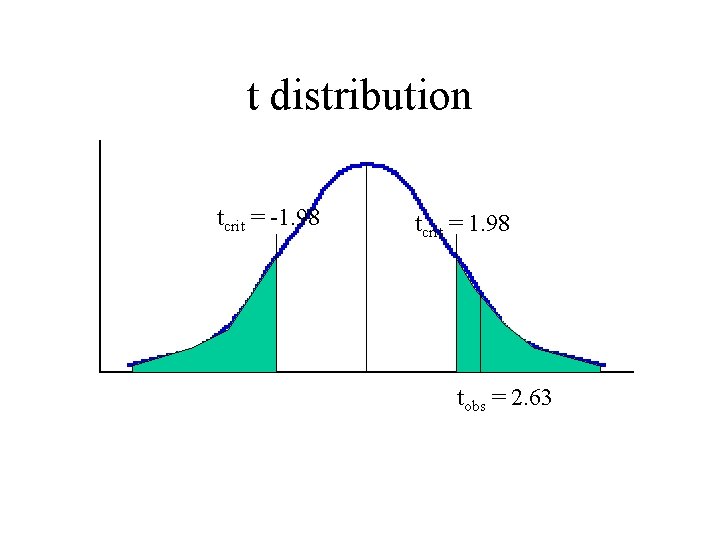
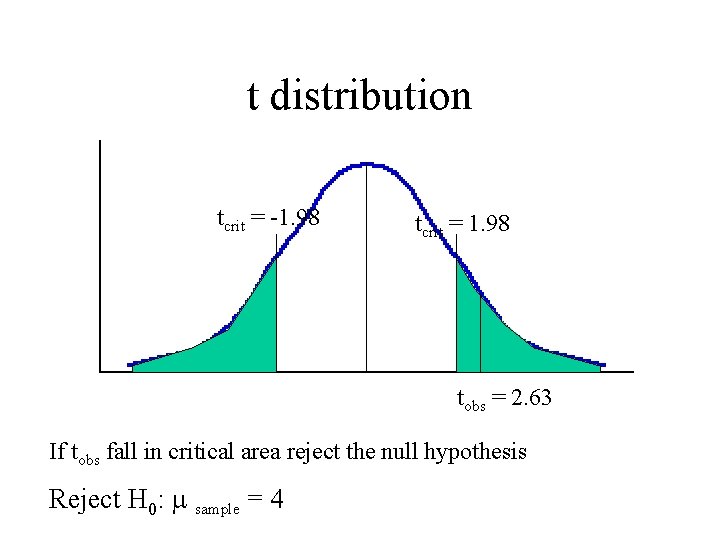
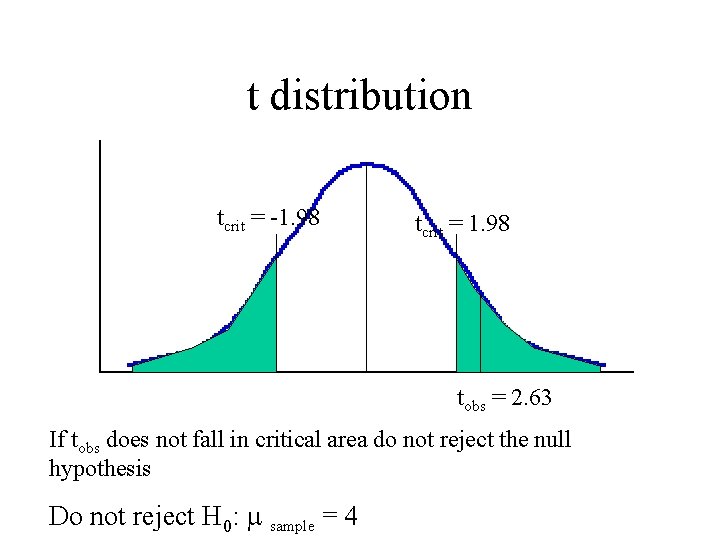
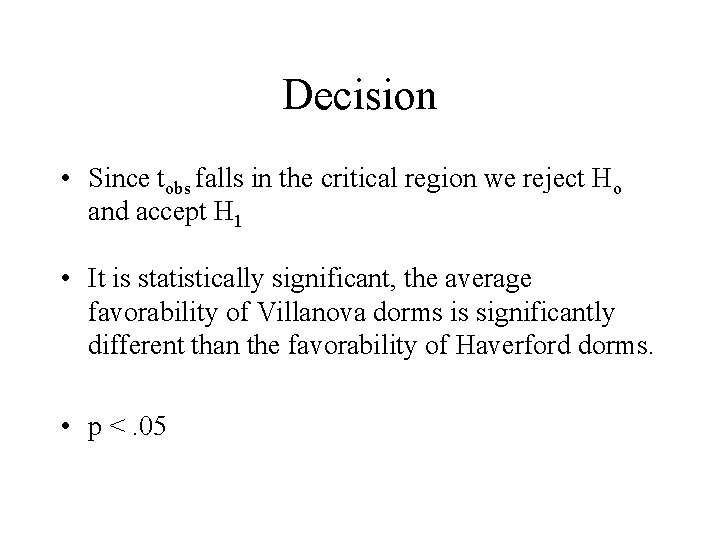
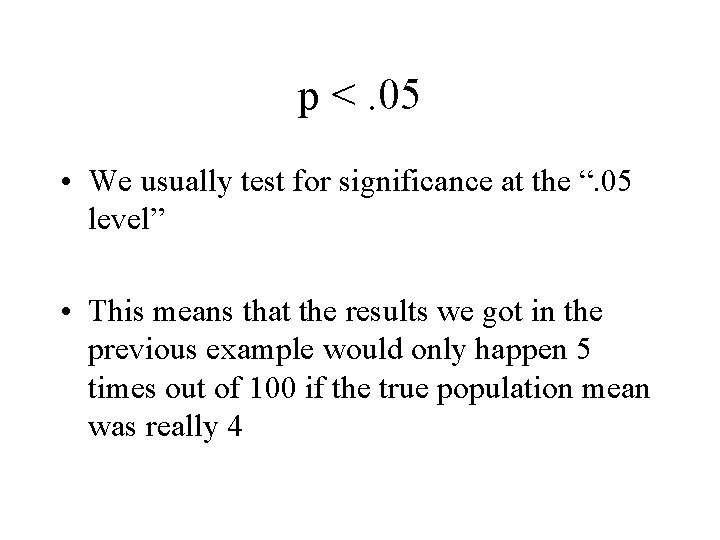
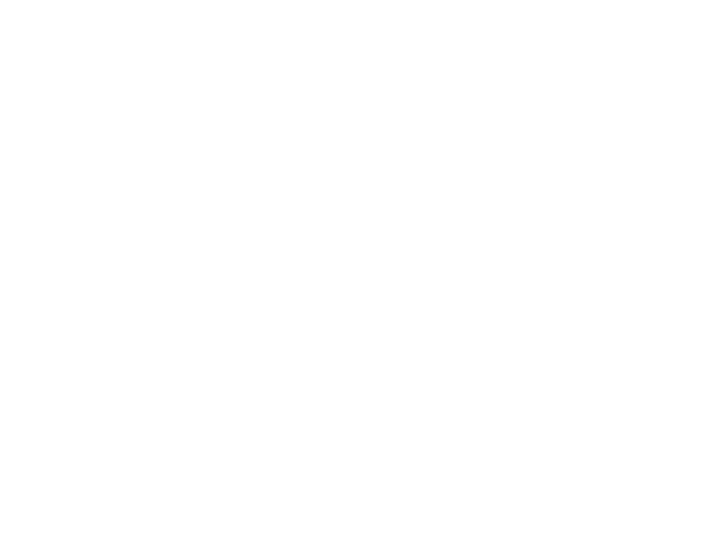
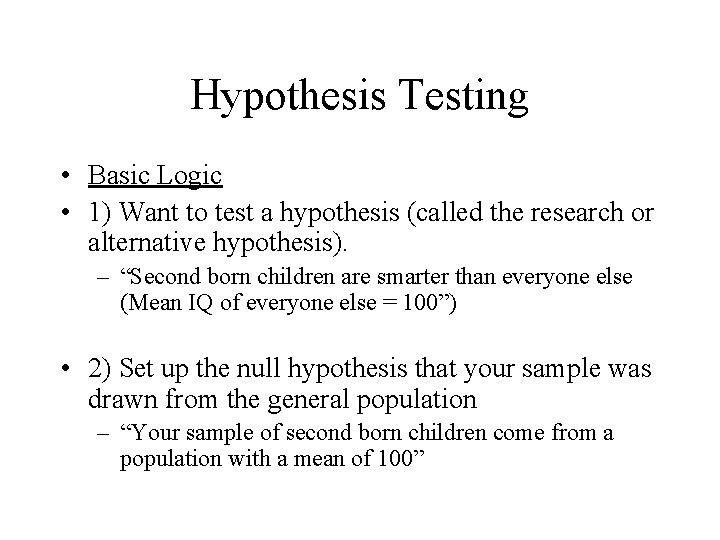
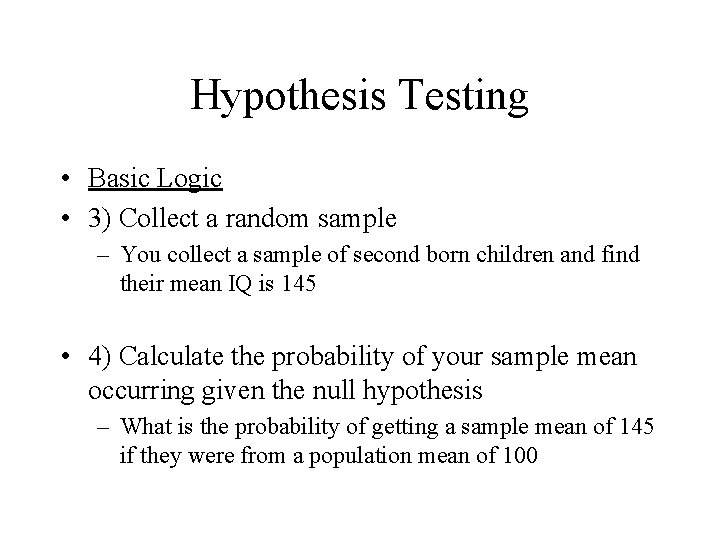
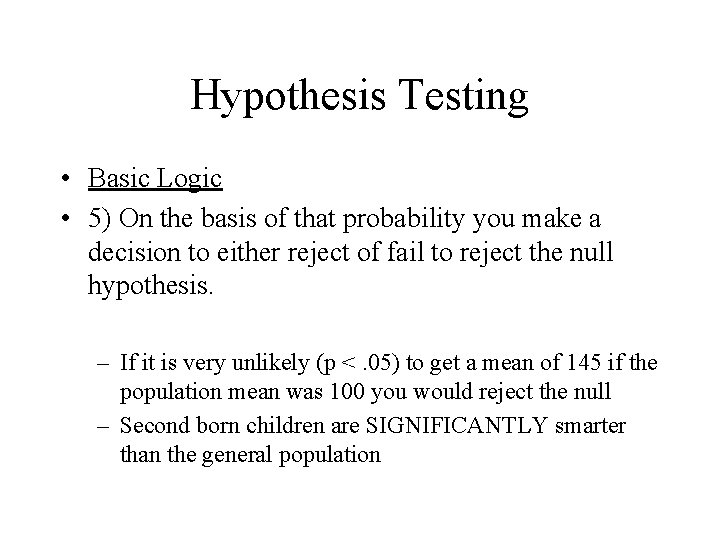
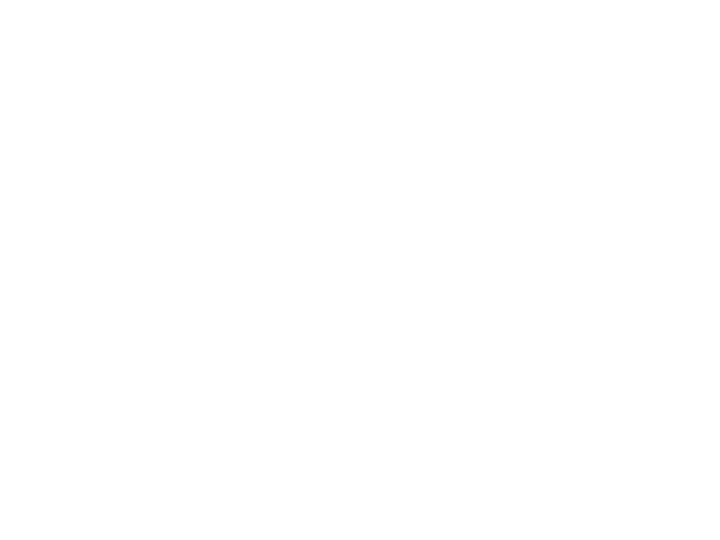
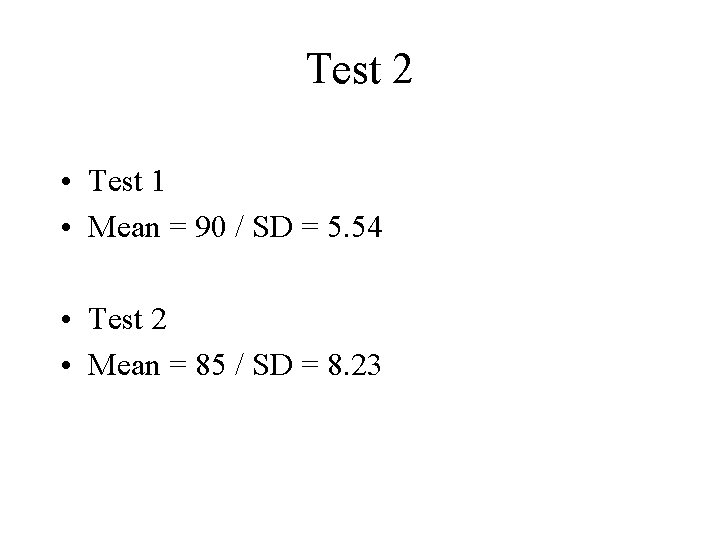
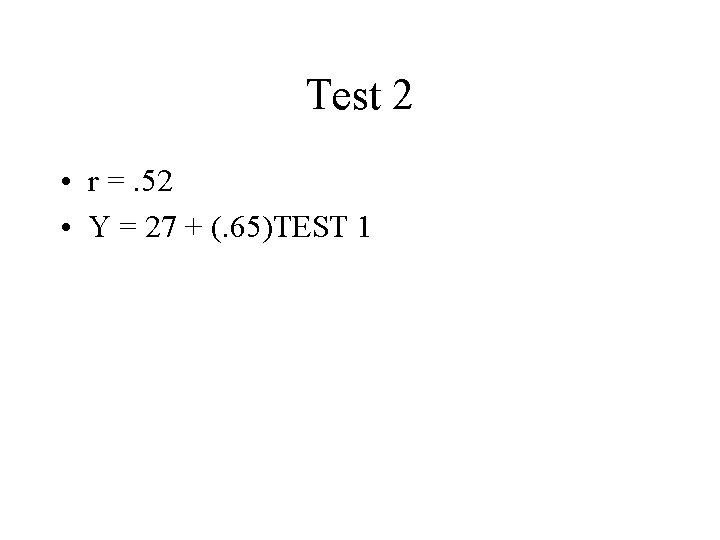
- Slides: 34
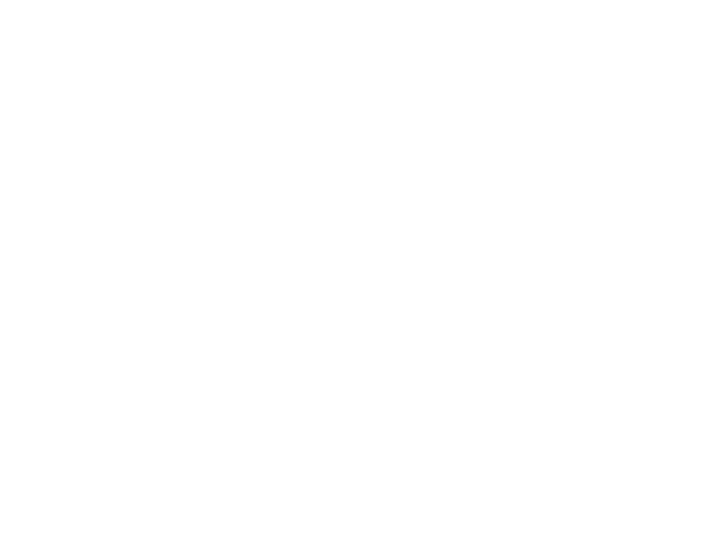
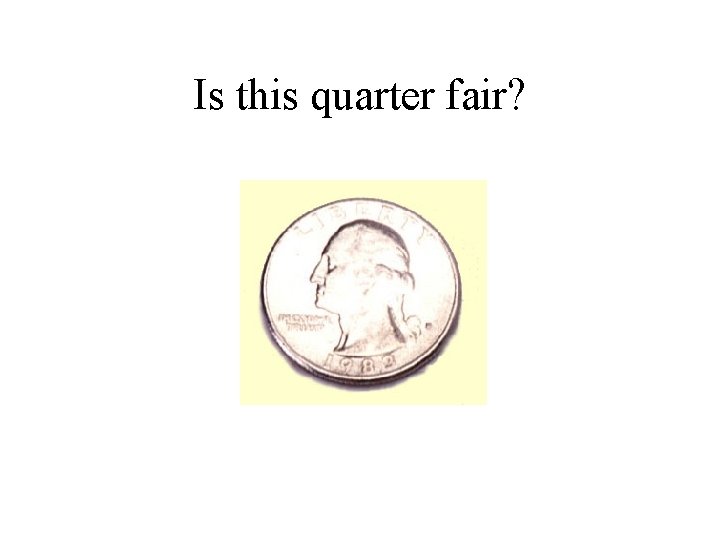
Is this quarter fair?
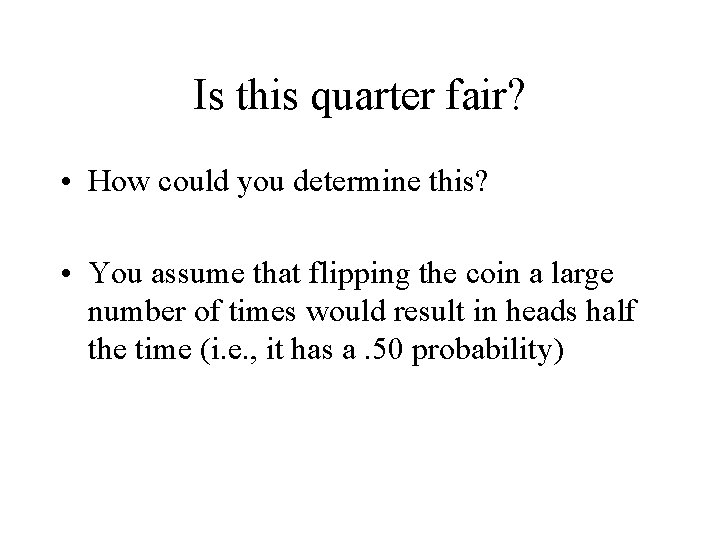
Is this quarter fair? • How could you determine this? • You assume that flipping the coin a large number of times would result in heads half the time (i. e. , it has a. 50 probability)
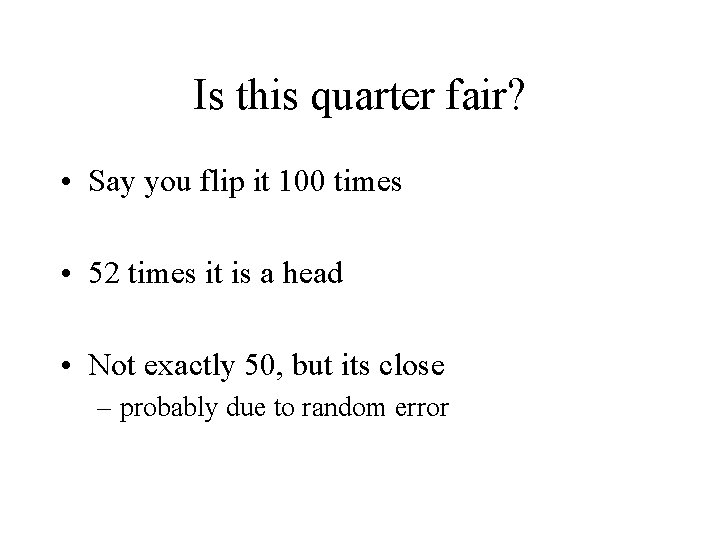
Is this quarter fair? • Say you flip it 100 times • 52 times it is a head • Not exactly 50, but its close – probably due to random error
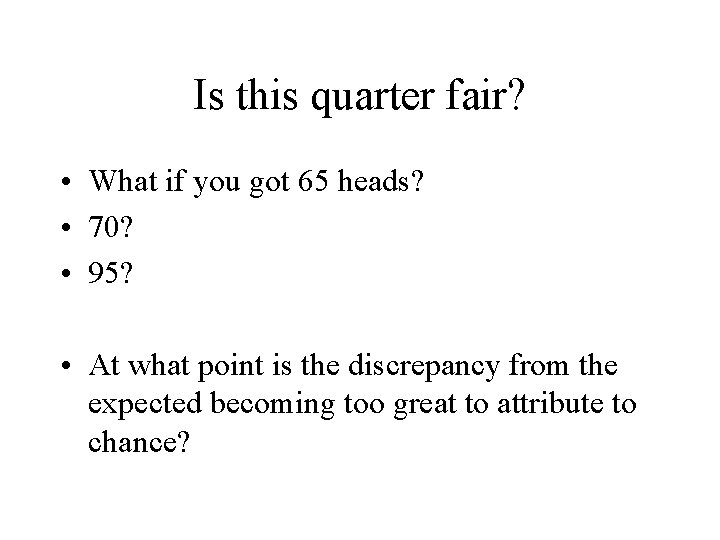
Is this quarter fair? • What if you got 65 heads? • 70? • 95? • At what point is the discrepancy from the expected becoming too great to attribute to chance?
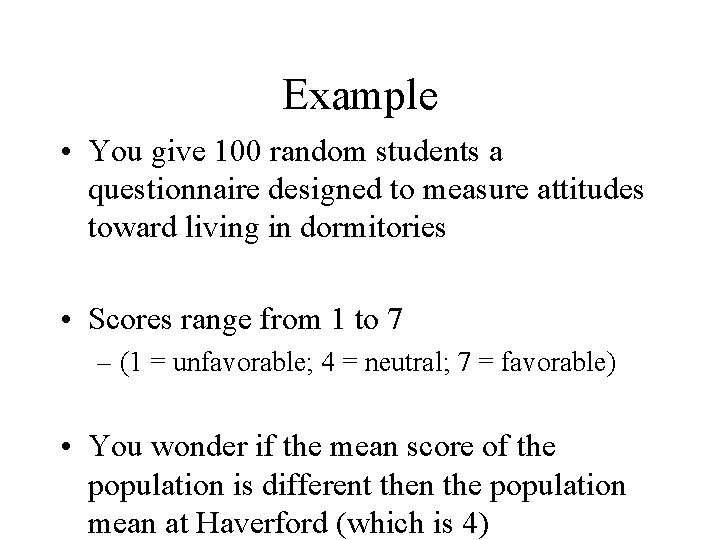
Example • You give 100 random students a questionnaire designed to measure attitudes toward living in dormitories • Scores range from 1 to 7 – (1 = unfavorable; 4 = neutral; 7 = favorable) • You wonder if the mean score of the population is different then the population mean at Haverford (which is 4)
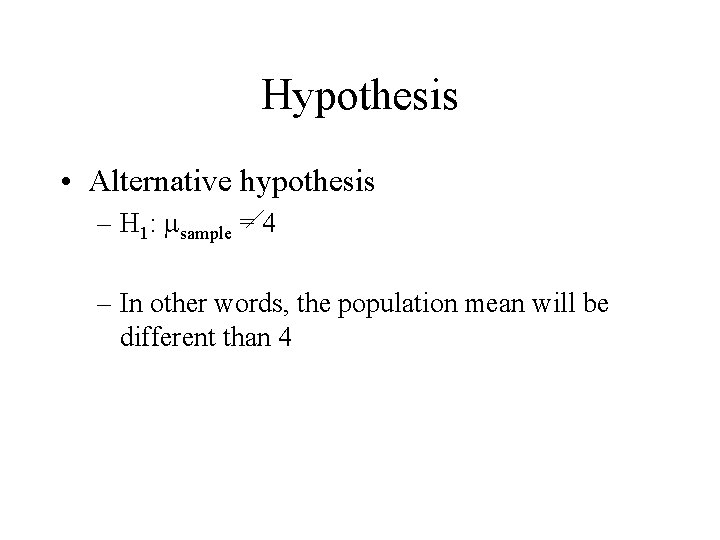
Hypothesis • Alternative hypothesis – H 1: sample = 4 – In other words, the population mean will be different than 4
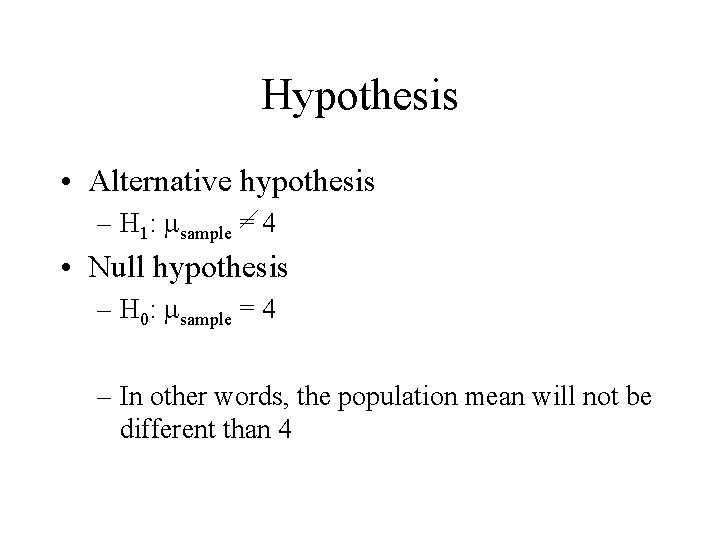
Hypothesis • Alternative hypothesis – H 1: sample = 4 • Null hypothesis – H 0: sample = 4 – In other words, the population mean will not be different than 4
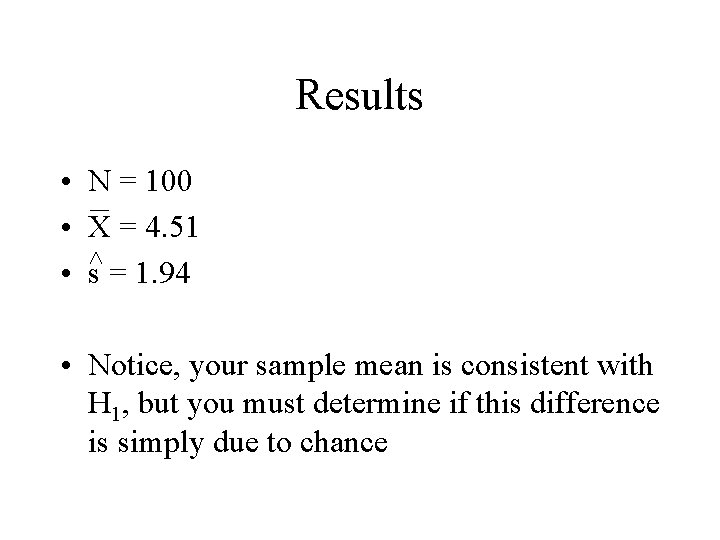
Results • N = 100 • X = 4. 51 • s = 1. 94 • Notice, your sample mean is consistent with H 1, but you must determine if this difference is simply due to chance
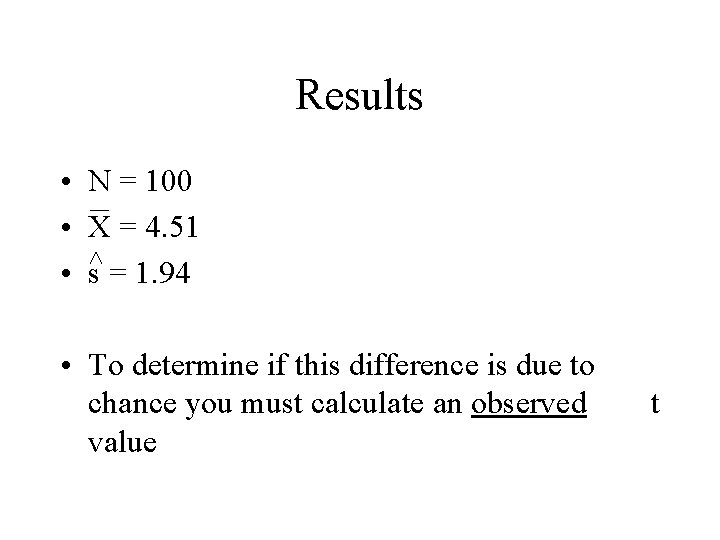
Results • N = 100 • X = 4. 51 • s = 1. 94 • To determine if this difference is due to chance you must calculate an observed value t
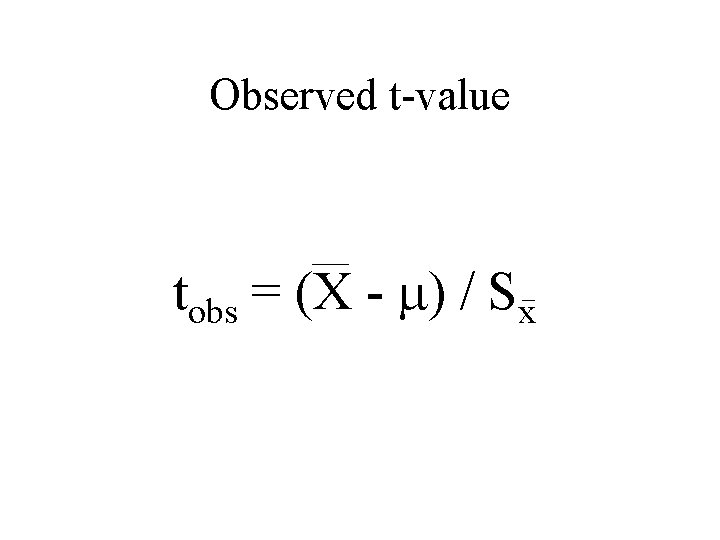
Observed t-value tobs = (X - ) / Sx
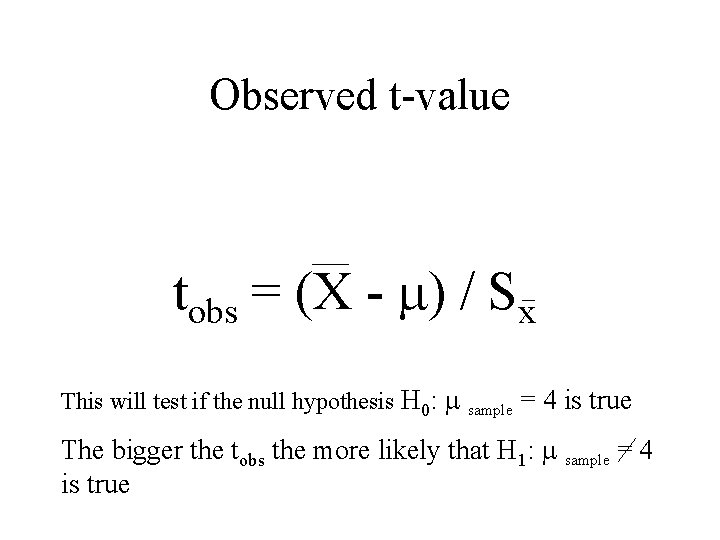
Observed t-value tobs = (X - ) / Sx This will test if the null hypothesis H 0: sample = 4 is true The bigger the tobs the more likely that H 1: sample = 4 is true
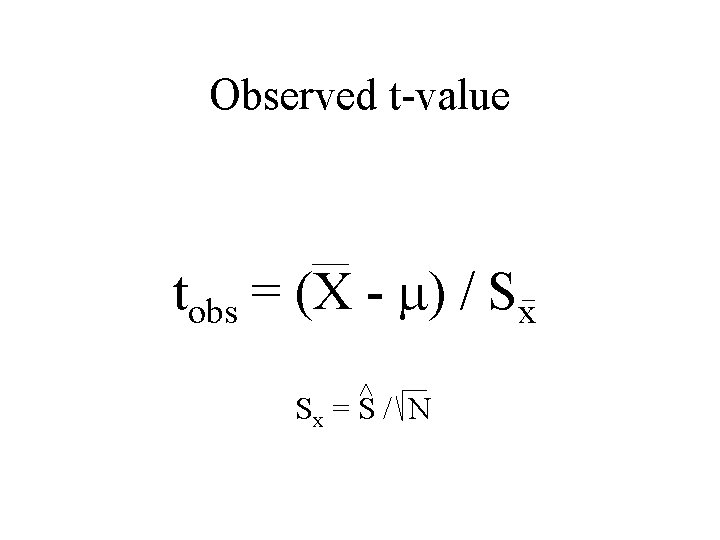
Observed t-value tobs = (X - ) / Sx Sx = S / N
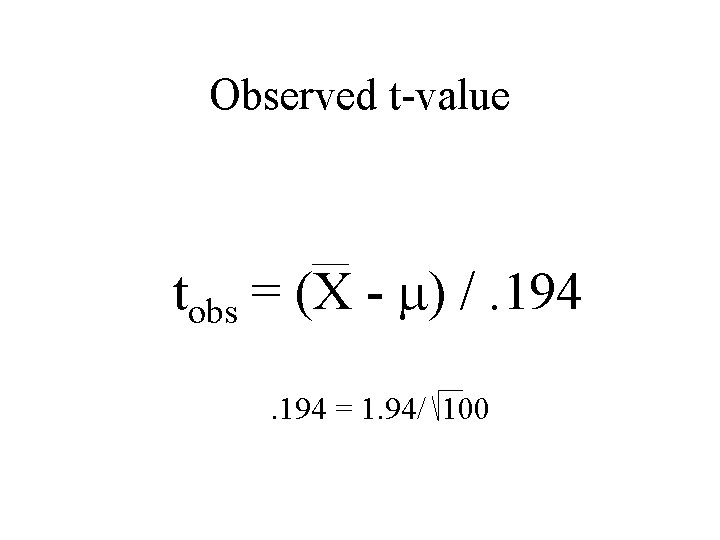
Observed t-value tobs = (X - ) /. 194 = 1. 94/ 100
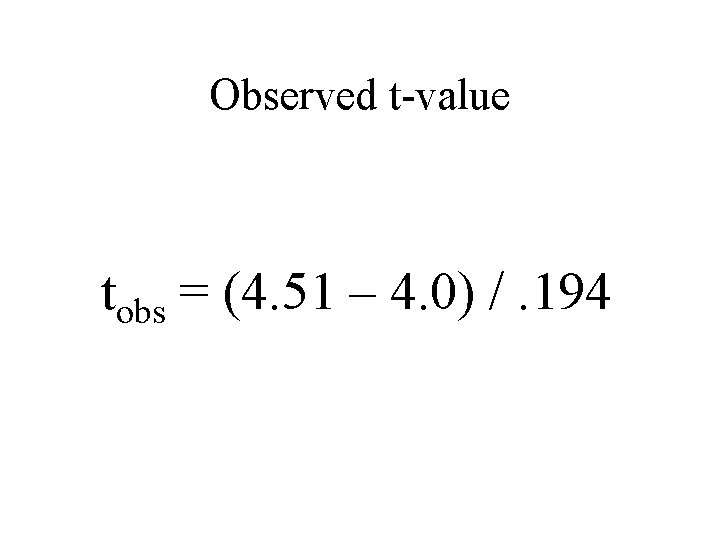
Observed t-value tobs = (4. 51 – 4. 0) /. 194
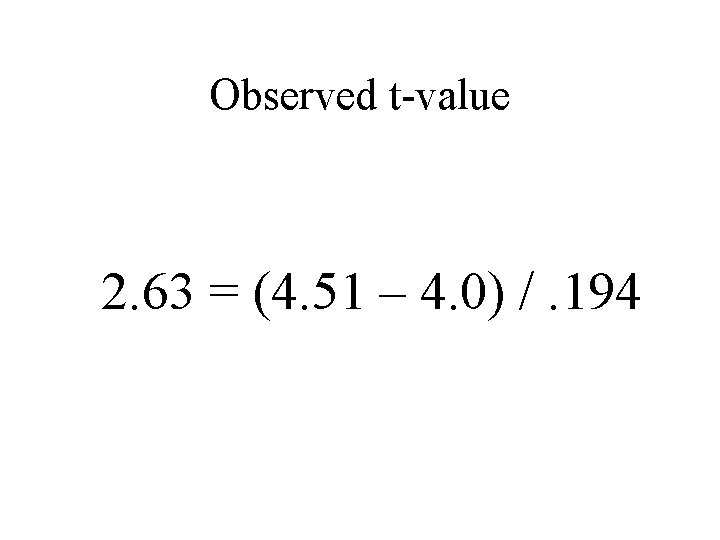
Observed t-value 2. 63 = (4. 51 – 4. 0) /. 194
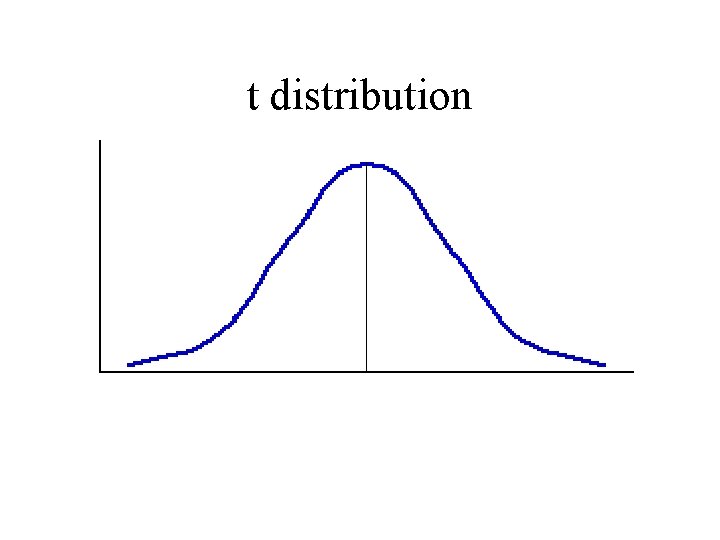
t distribution
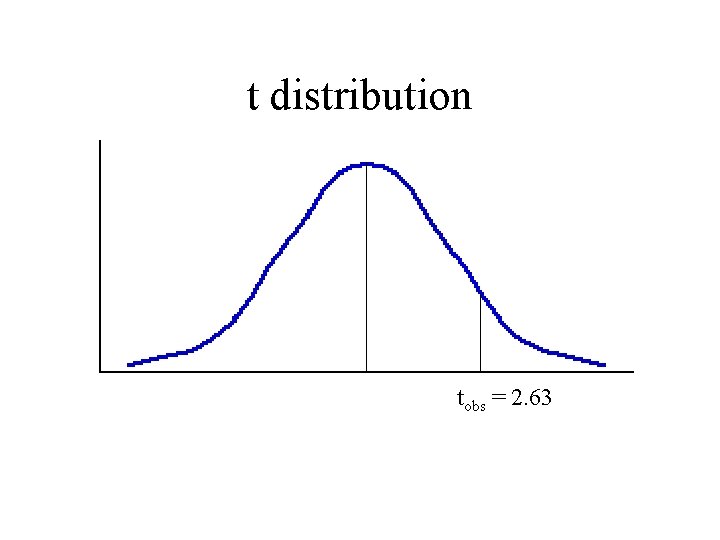
t distribution tobs = 2. 63
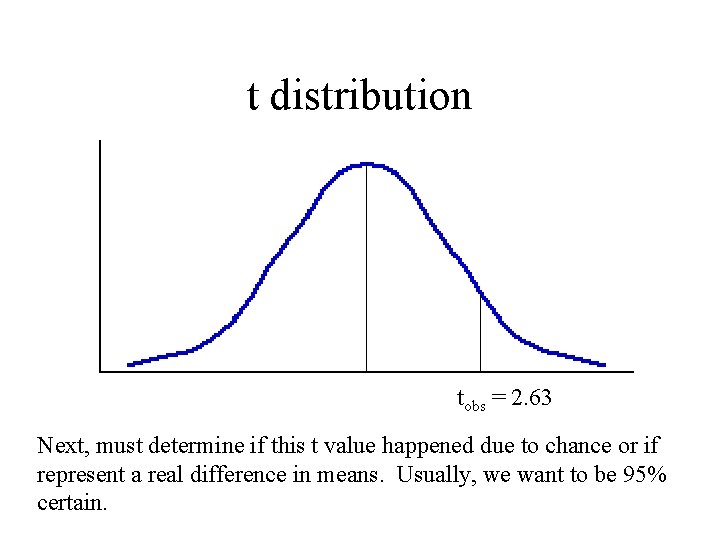
t distribution tobs = 2. 63 Next, must determine if this t value happened due to chance or if represent a real difference in means. Usually, we want to be 95% certain.
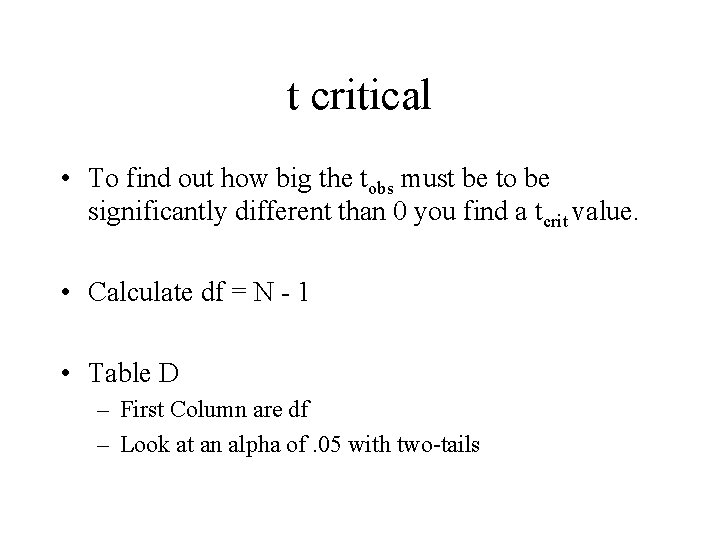
t critical • To find out how big the tobs must be to be significantly different than 0 you find a tcrit value. • Calculate df = N - 1 • Table D – First Column are df – Look at an alpha of. 05 with two-tails
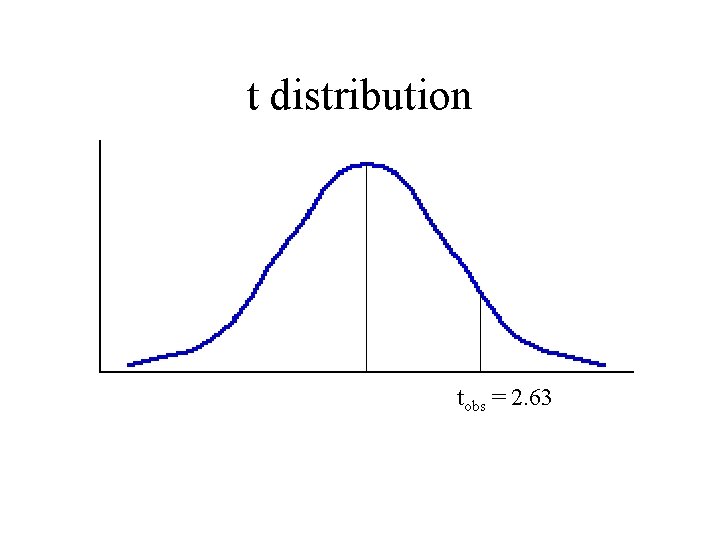
t distribution tobs = 2. 63
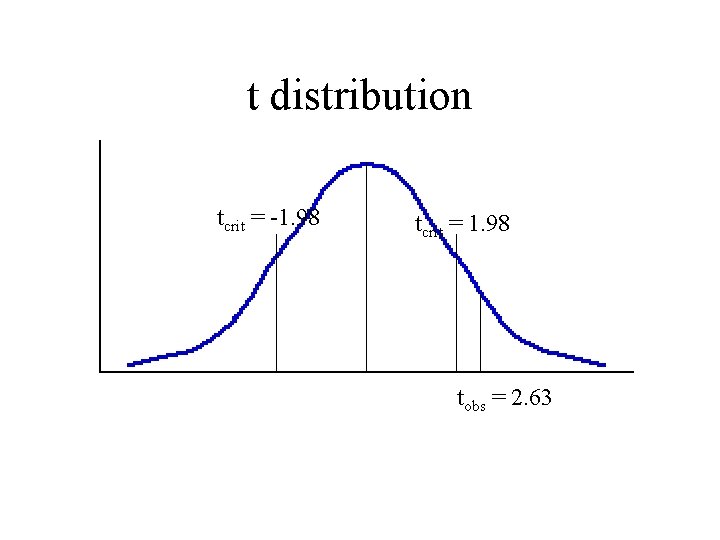
t distribution tcrit = -1. 98 tcrit = 1. 98 tobs = 2. 63
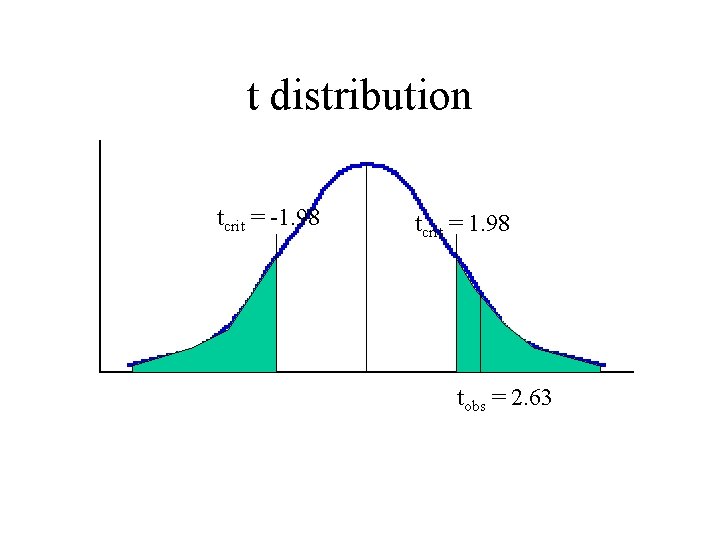
t distribution tcrit = -1. 98 tcrit = 1. 98 tobs = 2. 63
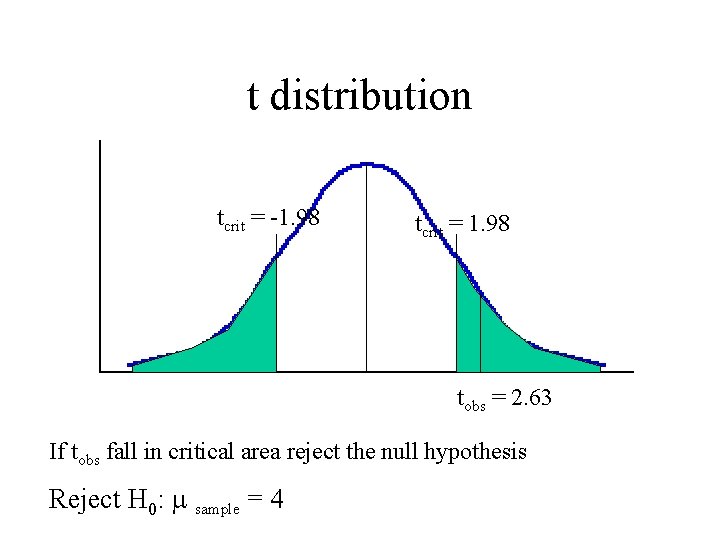
t distribution tcrit = -1. 98 tcrit = 1. 98 tobs = 2. 63 If tobs fall in critical area reject the null hypothesis Reject H 0: sample = 4
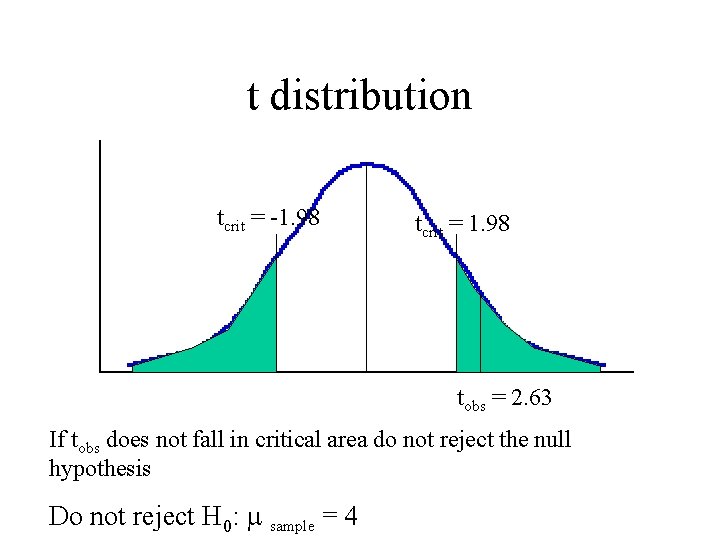
t distribution tcrit = -1. 98 tcrit = 1. 98 tobs = 2. 63 If tobs does not fall in critical area do not reject the null hypothesis Do not reject H 0: sample = 4
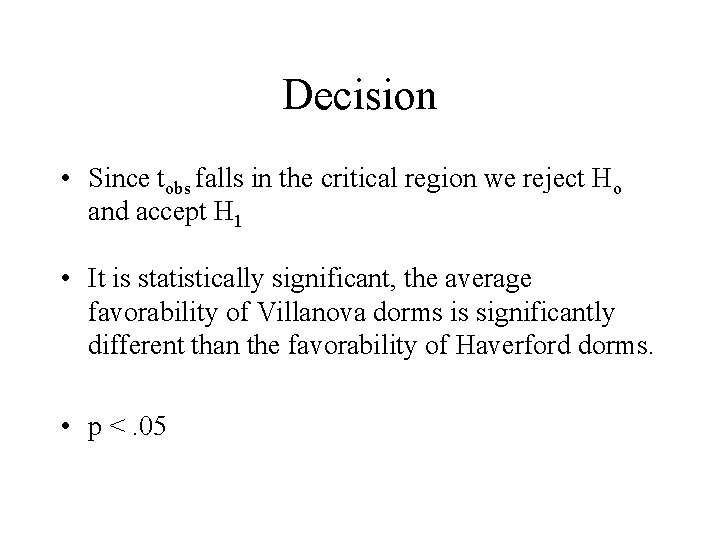
Decision • Since tobs falls in the critical region we reject Ho and accept H 1 • It is statistically significant, the average favorability of Villanova dorms is significantly different than the favorability of Haverford dorms. • p <. 05
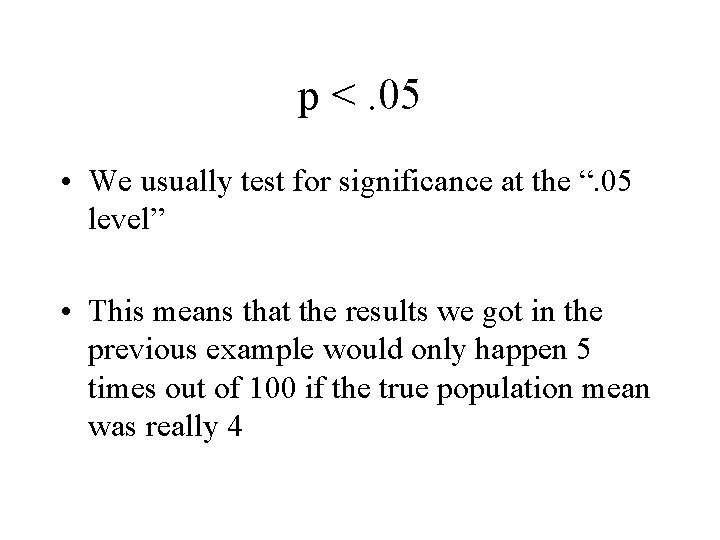
p <. 05 • We usually test for significance at the “. 05 level” • This means that the results we got in the previous example would only happen 5 times out of 100 if the true population mean was really 4
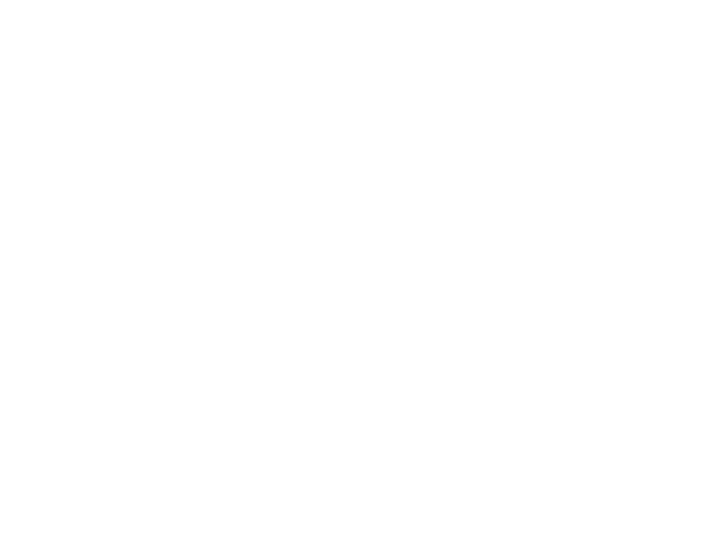
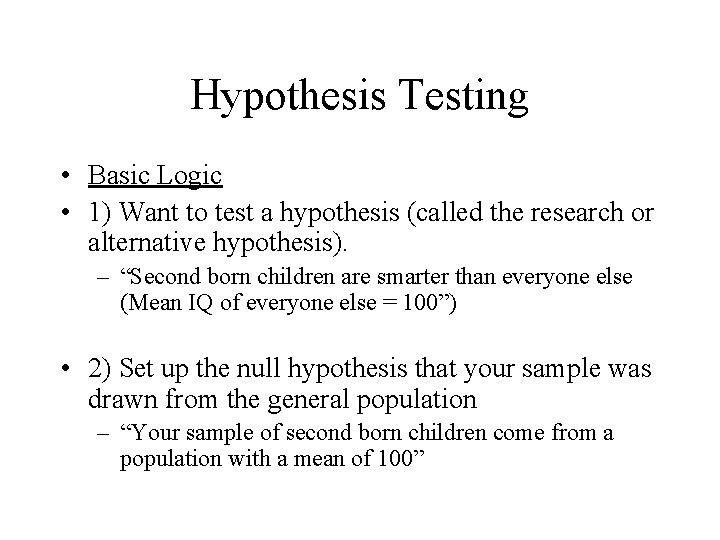
Hypothesis Testing • Basic Logic • 1) Want to test a hypothesis (called the research or alternative hypothesis). – “Second born children are smarter than everyone else (Mean IQ of everyone else = 100”) • 2) Set up the null hypothesis that your sample was drawn from the general population – “Your sample of second born children come from a population with a mean of 100”
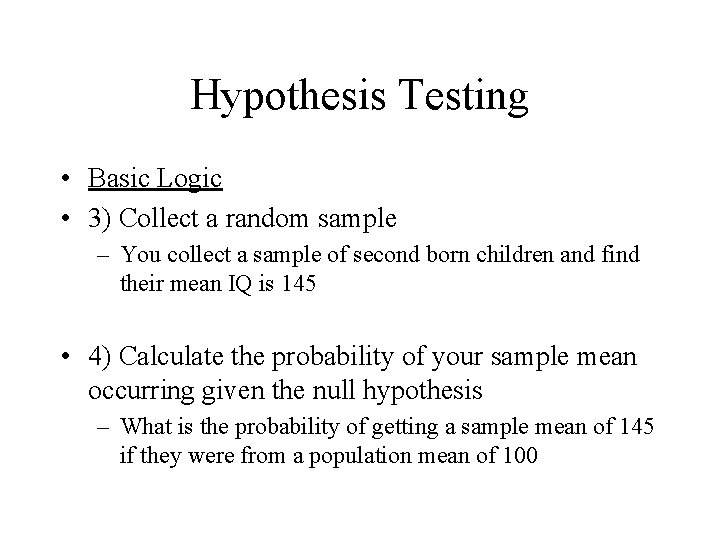
Hypothesis Testing • Basic Logic • 3) Collect a random sample – You collect a sample of second born children and find their mean IQ is 145 • 4) Calculate the probability of your sample mean occurring given the null hypothesis – What is the probability of getting a sample mean of 145 if they were from a population mean of 100
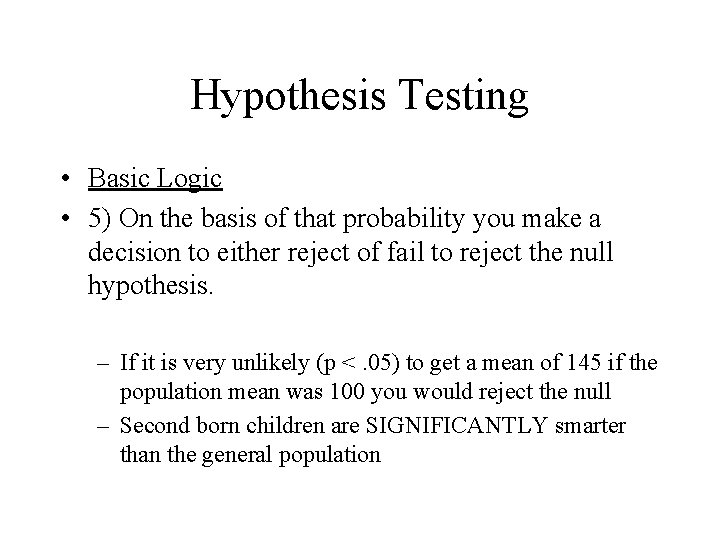
Hypothesis Testing • Basic Logic • 5) On the basis of that probability you make a decision to either reject of fail to reject the null hypothesis. – If it is very unlikely (p <. 05) to get a mean of 145 if the population mean was 100 you would reject the null – Second born children are SIGNIFICANTLY smarter than the general population
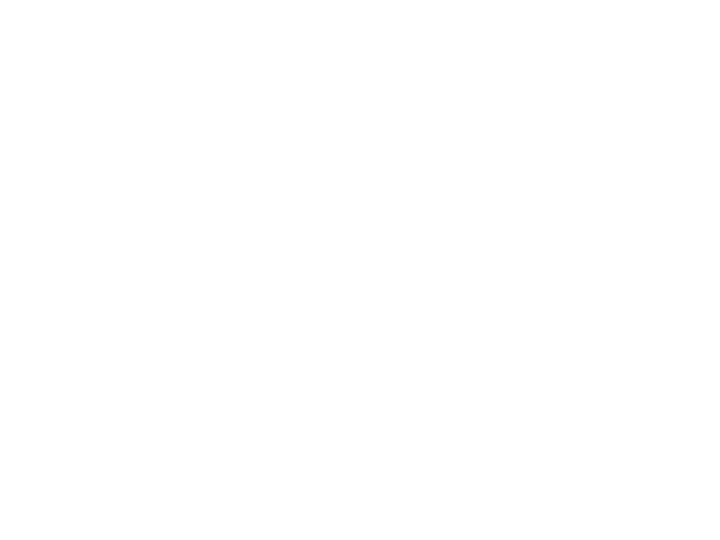
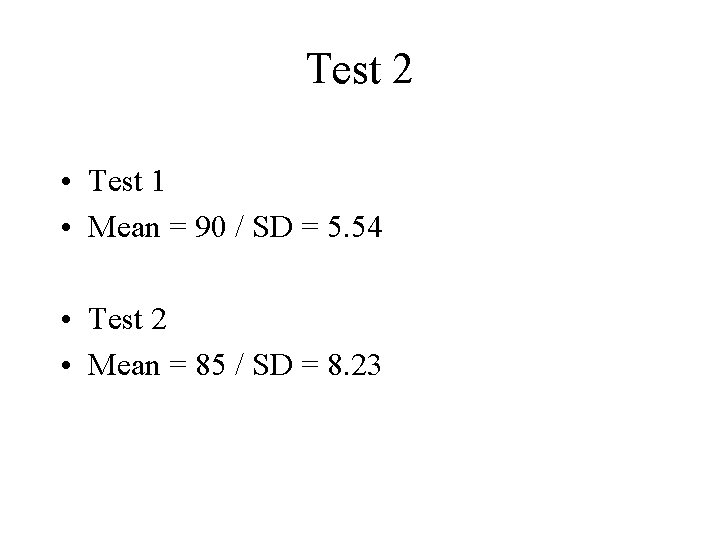
Test 2 • Test 1 • Mean = 90 / SD = 5. 54 • Test 2 • Mean = 85 / SD = 8. 23
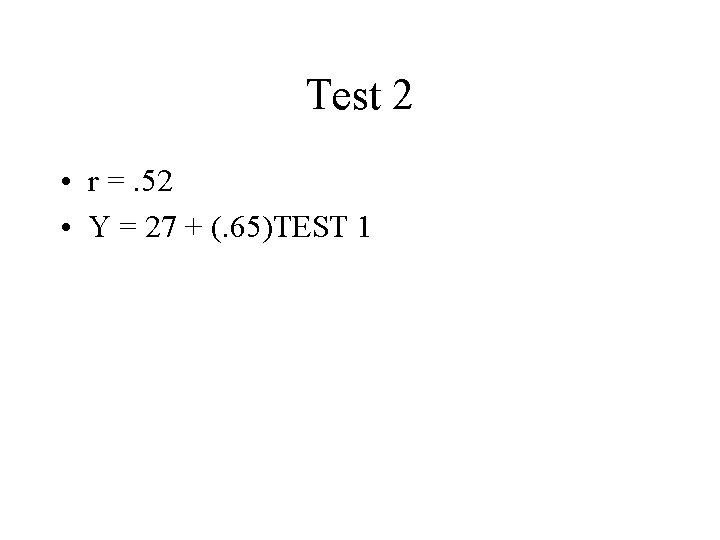
Test 2 • r =. 52 • Y = 27 + (. 65)TEST 1
Difference between quarter past and quarter to
Quarter past
Significato fair play
Literary devices in macbeth examples
Examples of fair is foul and foul is fair in macbeth
Foul is fair macbeth
Shakespeare 14 line poem
Syntax literary device
Foul is fair and fair is foul literary device
Fourier series equation
Reading and writing quarter 3
Welcome to quarter 2
Volume of a prism
Marketplace simulation game
Perimeter formula for quarter circle
It's quarter past one
Proximal half crown
Arrowhead clasp
Clock quarter past
Put your right hand in
Negative quarter power scaling
Whats a quarter past 12
Chapter 3 geometry test
Welcome to quarter 3
First quarter moon gif
The groove angle of the pulley for rope drive is usually
English 5 quarter 4 week 1
The head of the virgin in three-quarter view facing right
Principles of marketing module 9
It's a quarter to two.
The moon is our nearest neighbour in space
A crane lowers a girder into place
English 9 quarter 2 week 3
Jones matrix for half wave plate
Quarter