Improving Passive Microwave Precipitation Estimates over Land Toward
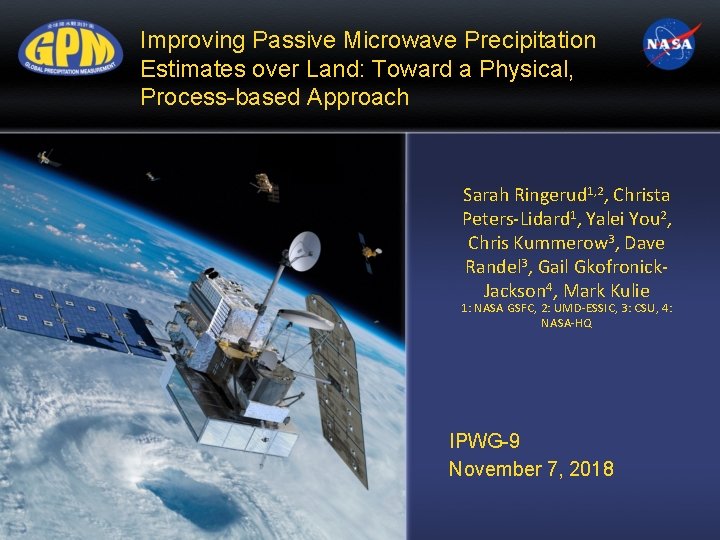
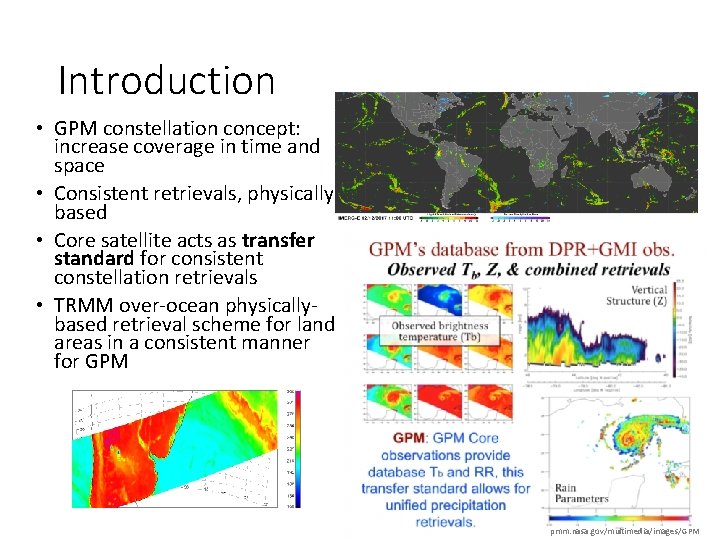
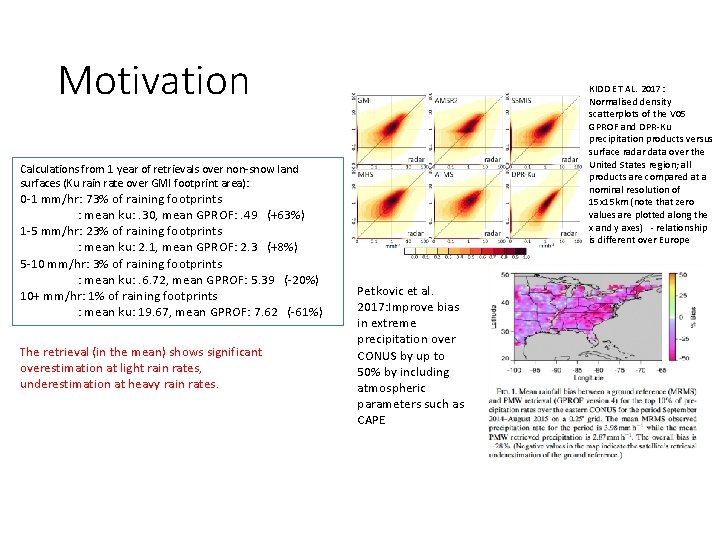
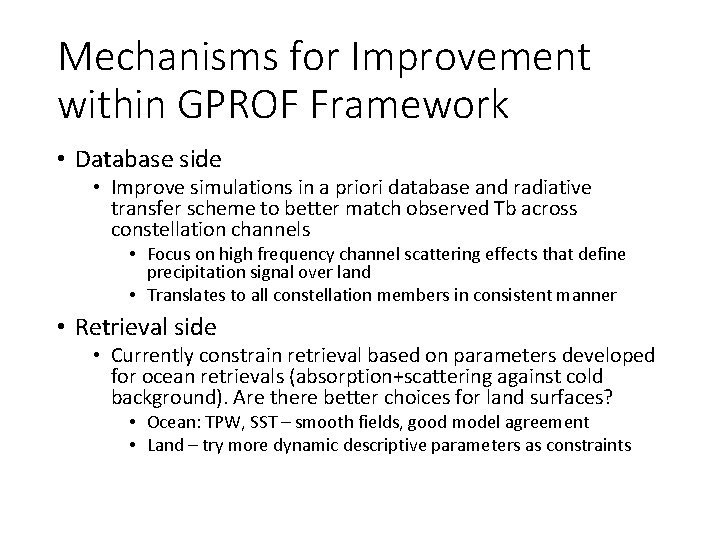
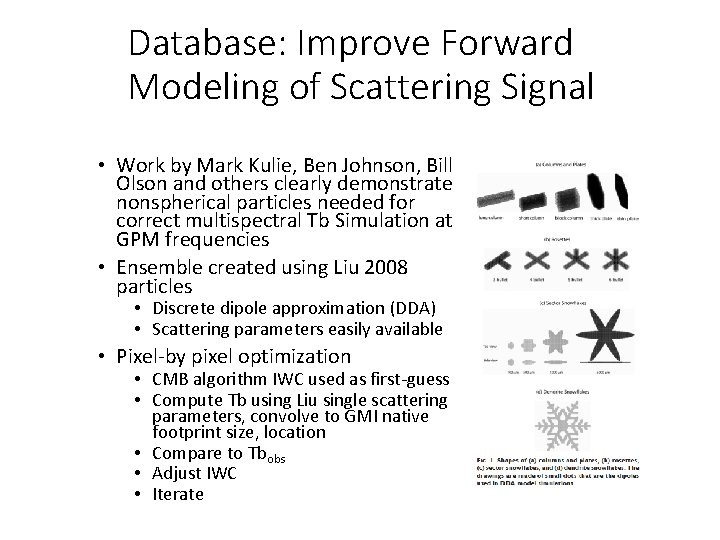
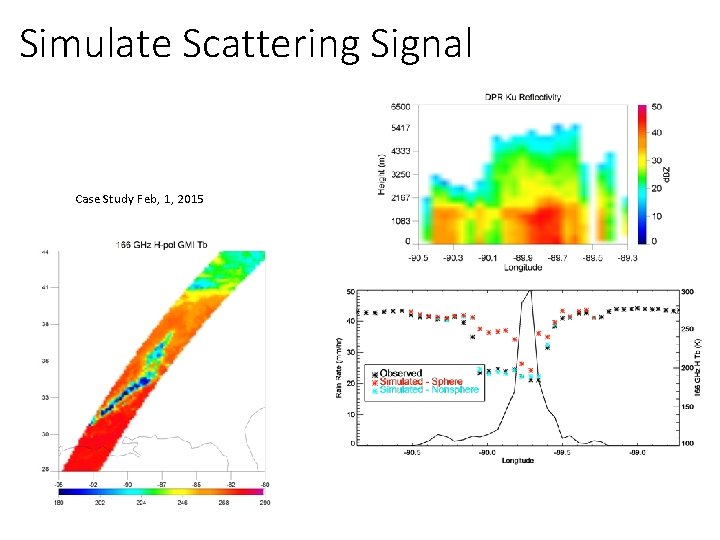
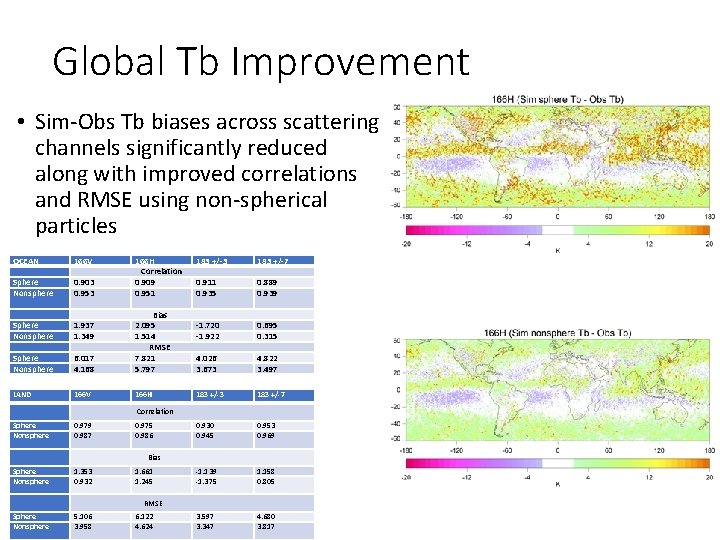
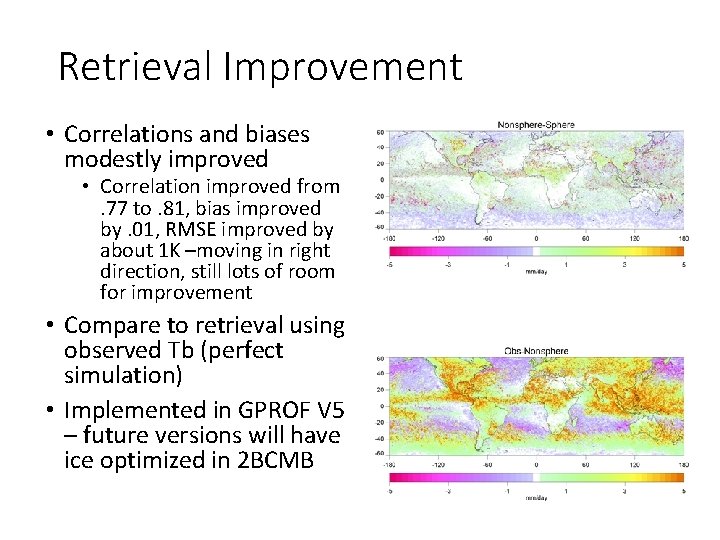
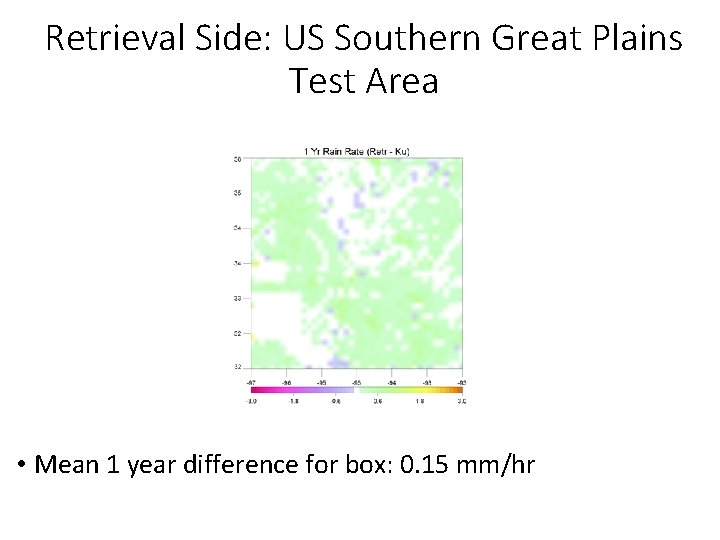
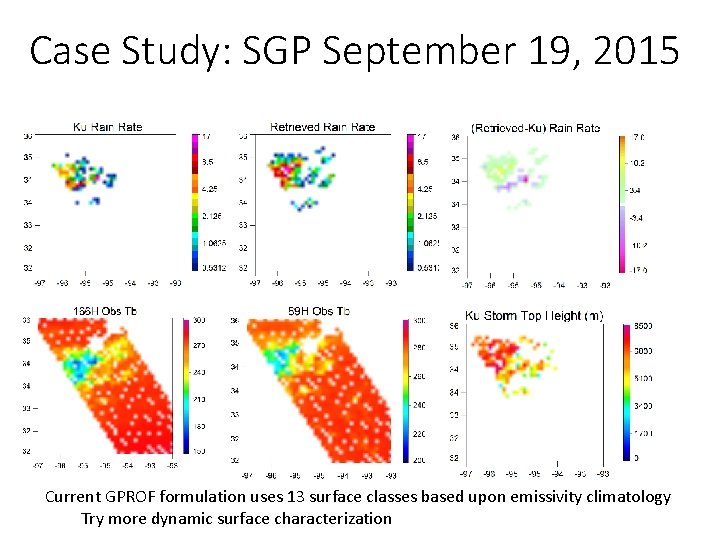
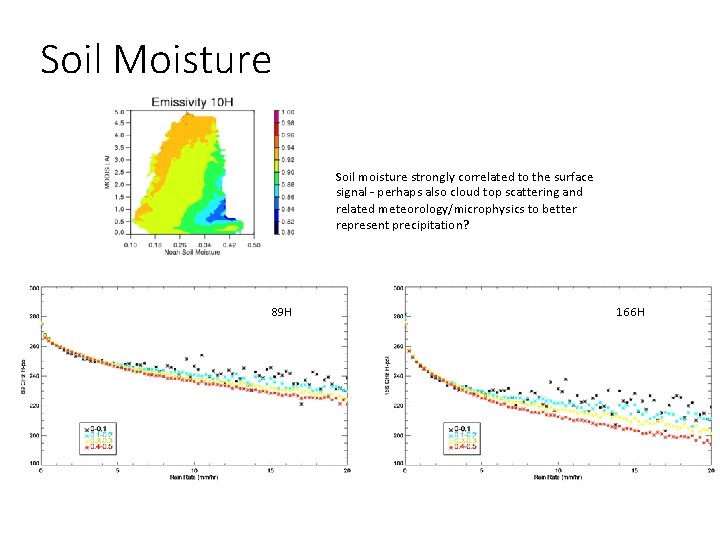
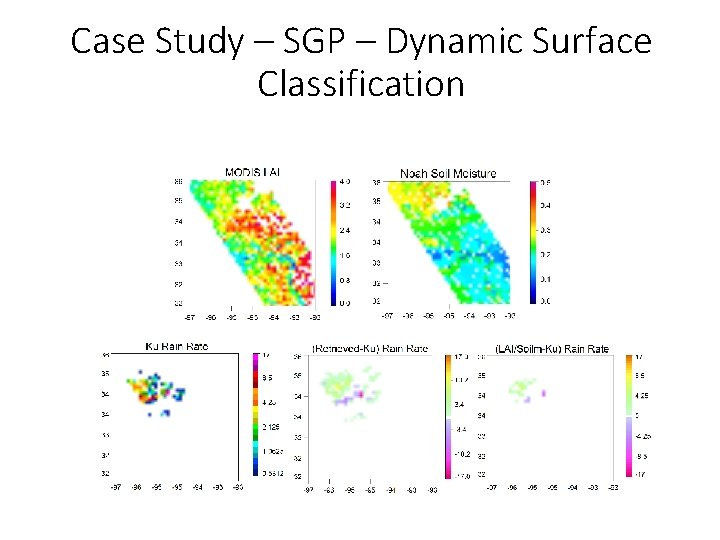
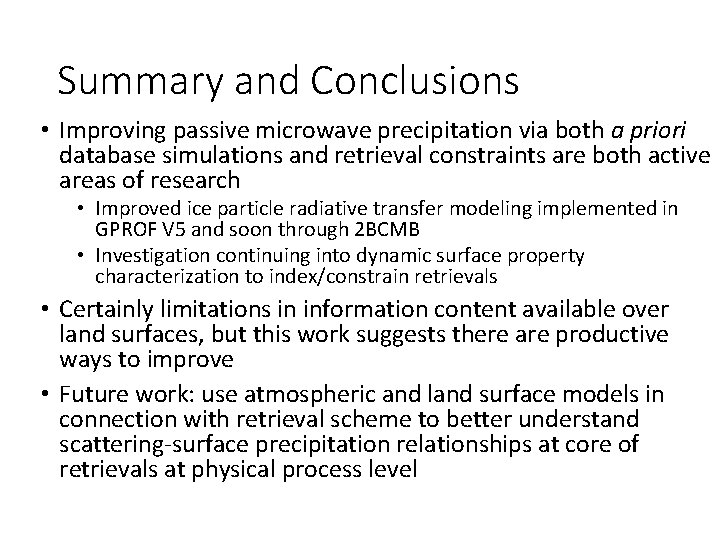
- Slides: 13
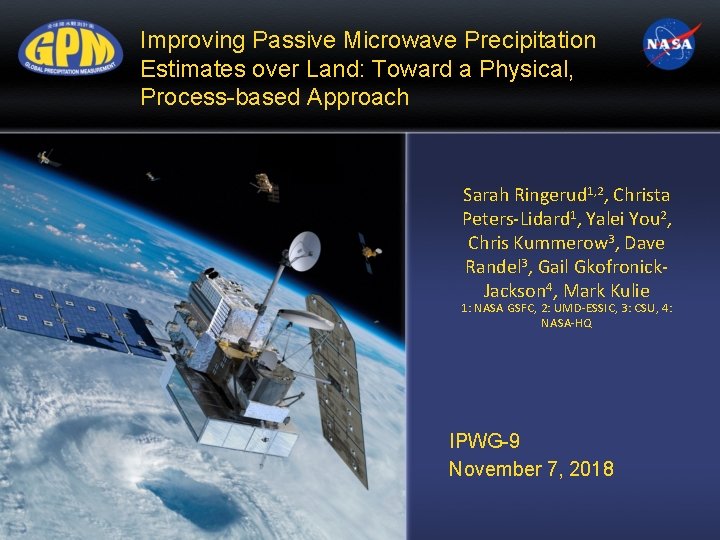
Improving Passive Microwave Precipitation Estimates over Land: Toward a Physical, Process-based Approach Sarah Ringerud 1, 2, Christa Peters-Lidard 1, Yalei You 2, Chris Kummerow 3, Dave Randel 3, Gail Gkofronick. Jackson 4, Mark Kulie 1: NASA GSFC, 2: UMD-ESSIC, 3: CSU, 4: NASA-HQ IPWG-9 November 7, 2018
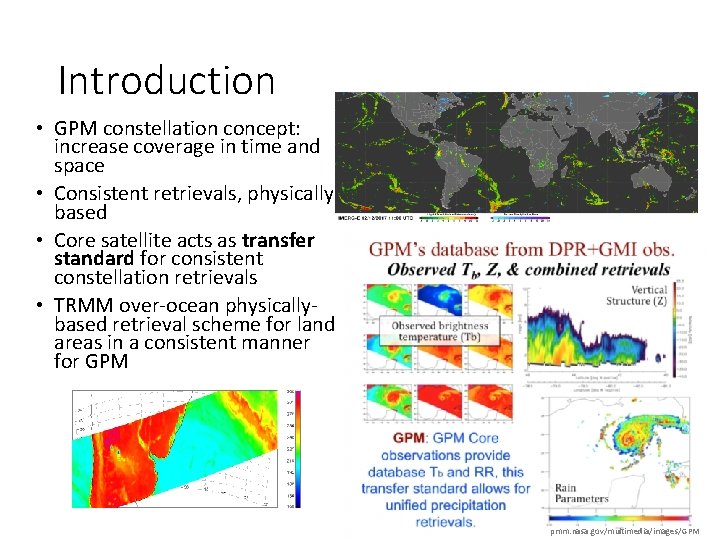
Introduction • GPM constellation concept: increase coverage in time and space • Consistent retrievals, physically based • Core satellite acts as transfer standard for consistent constellation retrievals • TRMM over-ocean physicallybased retrieval scheme for land areas in a consistent manner for GPM pmm. nasa. gov/multimedia/images/GPM
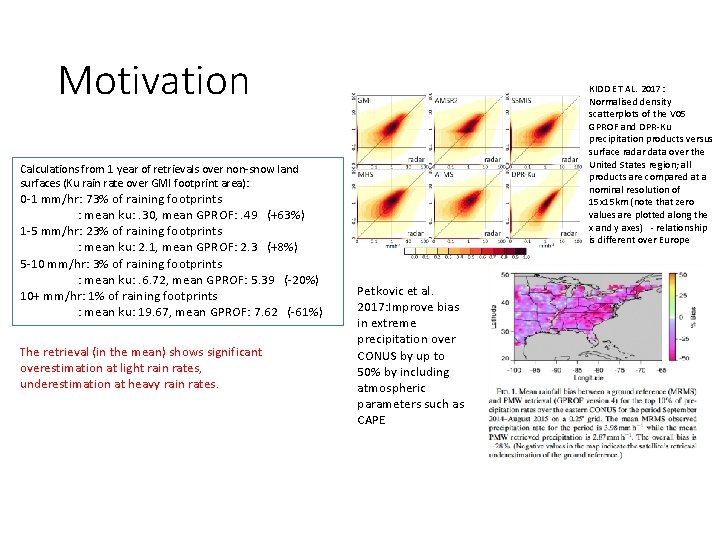
Motivation KIDD ET AL. 2017: Normalised density scatterplots of the V 05 GPROF and DPR-Ku precipitation products versus surface radar data over the United States region; all products are compared at a nominal resolution of 15 x 15 km (note that zero values are plotted along the x and y axes) - relationship is different over Europe Calculations from 1 year of retrievals over non-snow land surfaces (Ku rain rate over GMI footprint area): 0 -1 mm/hr: 73% of raining footprints : mean ku: . 30, mean GPROF: . 49 (+63%) 1 -5 mm/hr: 23% of raining footprints : mean ku: 2. 1, mean GPROF: 2. 3 (+8%) 5 -10 mm/hr: 3% of raining footprints : mean ku: . 6. 72, mean GPROF: 5. 39 (-20%) 10+ mm/hr: 1% of raining footprints : mean ku: 19. 67, mean GPROF: 7. 62 (-61%) The retrieval (in the mean) shows significant overestimation at light rain rates, underestimation at heavy rain rates. Petkovic et al. 2017: Improve bias in extreme precipitation over CONUS by up to 50% by including atmospheric parameters such as CAPE
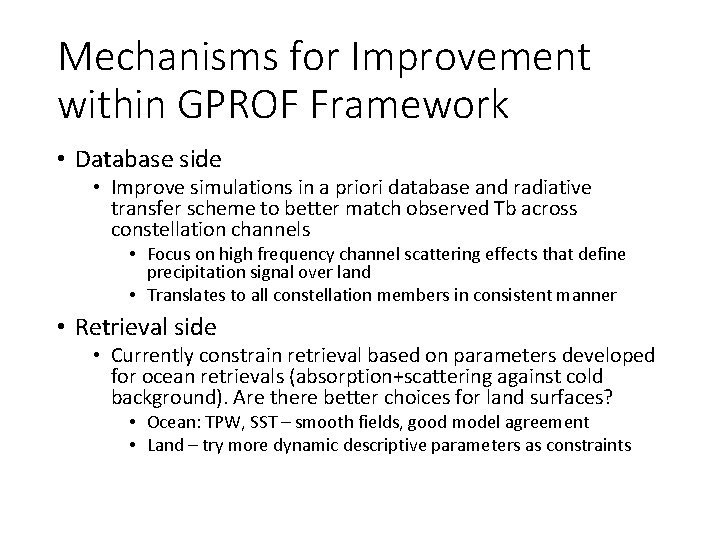
Mechanisms for Improvement within GPROF Framework • Database side • Improve simulations in a priori database and radiative transfer scheme to better match observed Tb across constellation channels • Focus on high frequency channel scattering effects that define precipitation signal over land • Translates to all constellation members in consistent manner • Retrieval side • Currently constrain retrieval based on parameters developed for ocean retrievals (absorption+scattering against cold background). Are there better choices for land surfaces? • Ocean: TPW, SST – smooth fields, good model agreement • Land – try more dynamic descriptive parameters as constraints
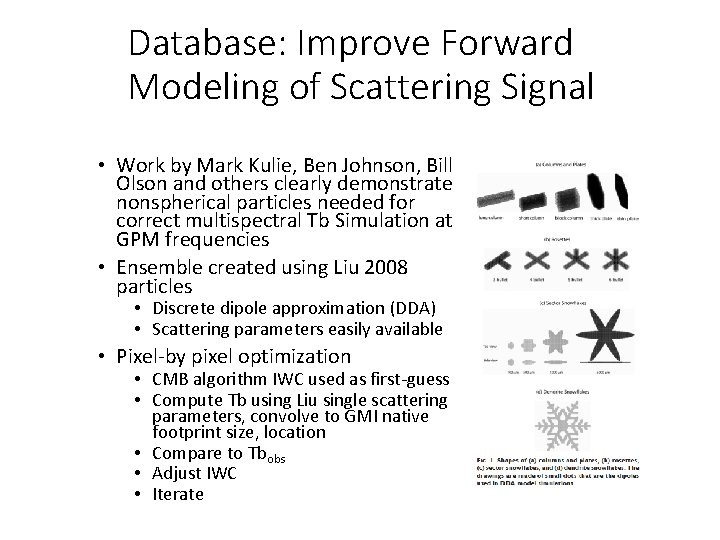
Database: Improve Forward Modeling of Scattering Signal • Work by Mark Kulie, Ben Johnson, Bill Olson and others clearly demonstrate nonspherical particles needed for correct multispectral Tb Simulation at GPM frequencies • Ensemble created using Liu 2008 particles • Discrete dipole approximation (DDA) • Scattering parameters easily available • Pixel-by pixel optimization • CMB algorithm IWC used as first-guess • Compute Tb using Liu single scattering parameters, convolve to GMI native footprint size, location • Compare to Tbobs • Adjust IWC • Iterate
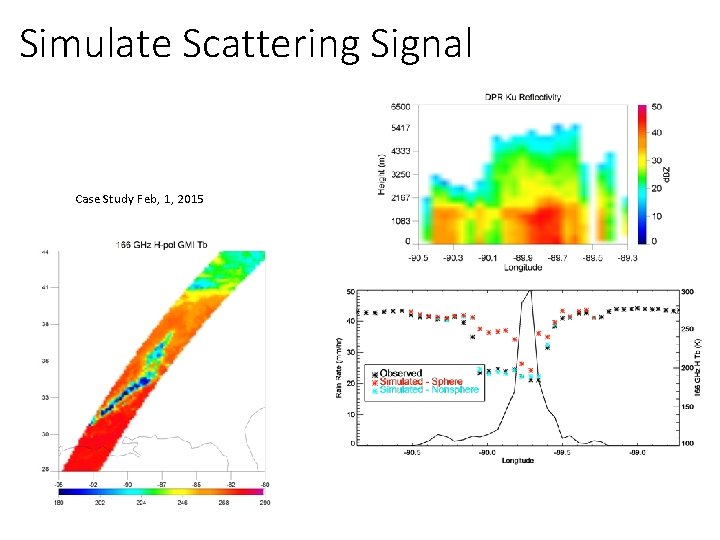
Simulate Scattering Signal Case Study Feb, 1, 2015
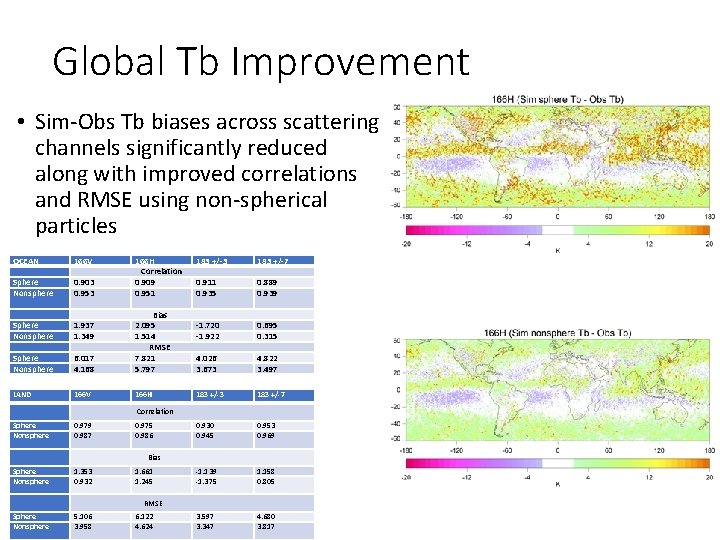
Global Tb Improvement • Sim-Obs Tb biases across scattering channels significantly reduced along with improved correlations and RMSE using non-spherical particles OCEAN 166 V 166 H Correlation 0. 909 0. 951 Sphere Nonsphere 0. 903 0. 953 Sphere Nonsphere 1. 937 1. 349 Sphere Nonsphere 6. 017 4. 168 Bias 2. 095 1. 514 RMSE 7. 821 5. 797 LAND 166 V 166 H 183 +/-3 183 +/-7 0. 911 0. 935 0. 889 0. 939 -1. 720 -1. 922 0. 695 0. 315 4. 026 3. 673 4. 822 3. 497 183 +/-3 183 +/-7 0. 930 0. 945 0. 953 0. 969 -1. 139 -1. 375 1. 158 0. 805 3. 597 3. 347 4. 680 3. 817 Correlation Sphere Nonsphere 0. 979 0. 987 0. 975 0. 986 Sphere Nonsphere 1. 353 0. 932 1. 661 1. 245 Sphere Nonsphere 5. 106 3. 958 6. 122 4. 624 Bias RMSE
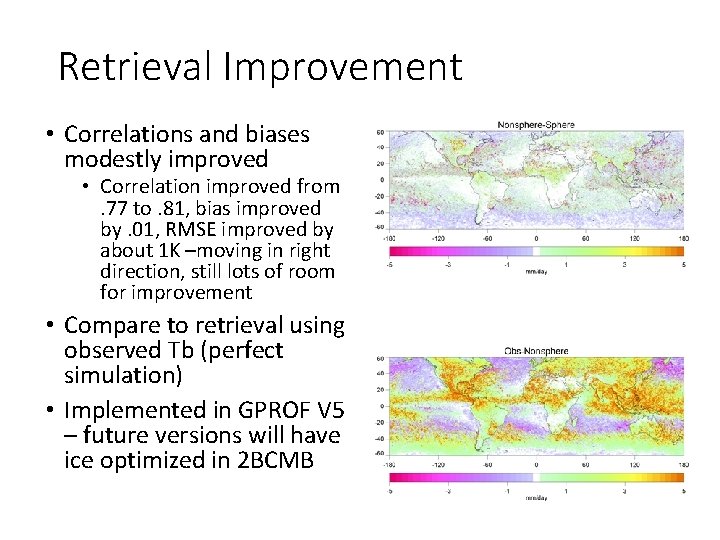
Retrieval Improvement • Correlations and biases modestly improved • Correlation improved from. 77 to. 81, bias improved by. 01, RMSE improved by about 1 K –moving in right direction, still lots of room for improvement • Compare to retrieval using observed Tb (perfect simulation) • Implemented in GPROF V 5 – future versions will have ice optimized in 2 BCMB
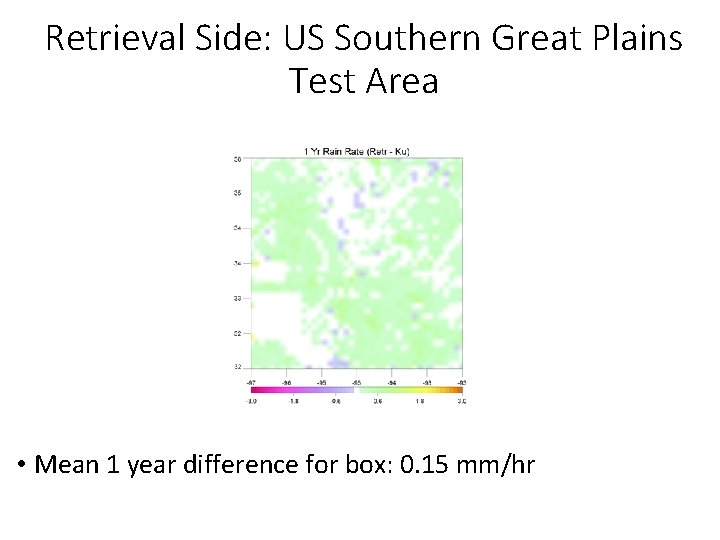
Retrieval Side: US Southern Great Plains Test Area • Mean 1 year difference for box: 0. 15 mm/hr
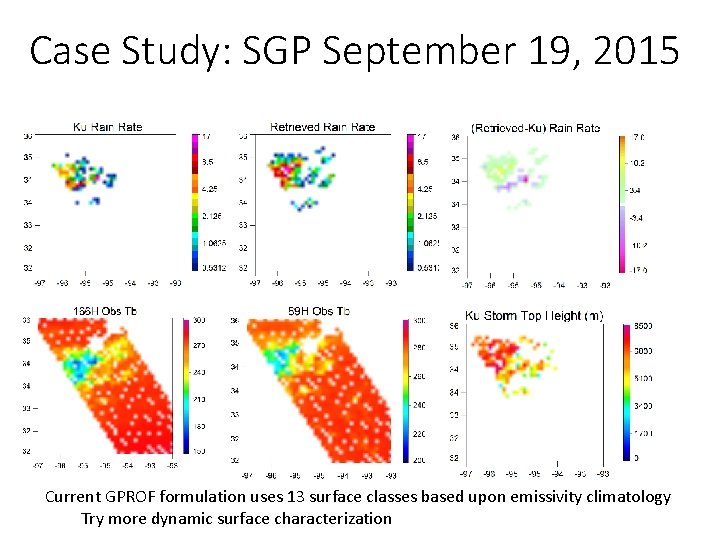
Case Study: SGP September 19, 2015 Current GPROF formulation uses 13 surface classes based upon emissivity climatology Try more dynamic surface characterization
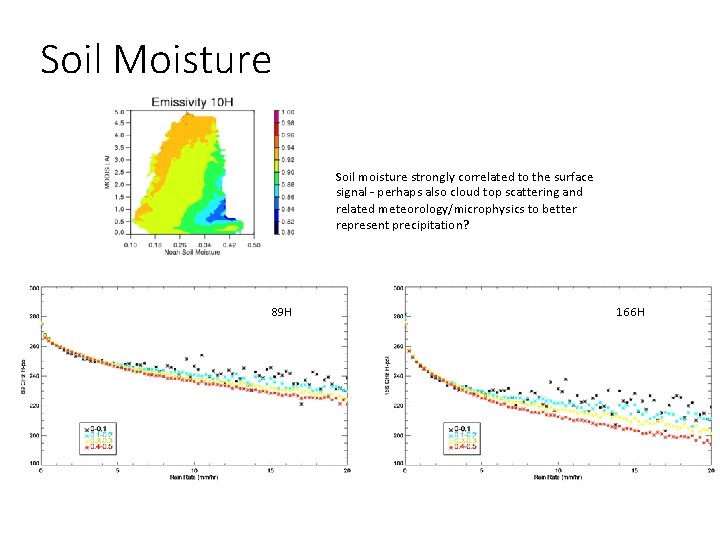
Soil Moisture Soil moisture strongly correlated to the surface signal – perhaps also cloud top scattering and related meteorology/microphysics to better represent precipitation? 89 H 166 H
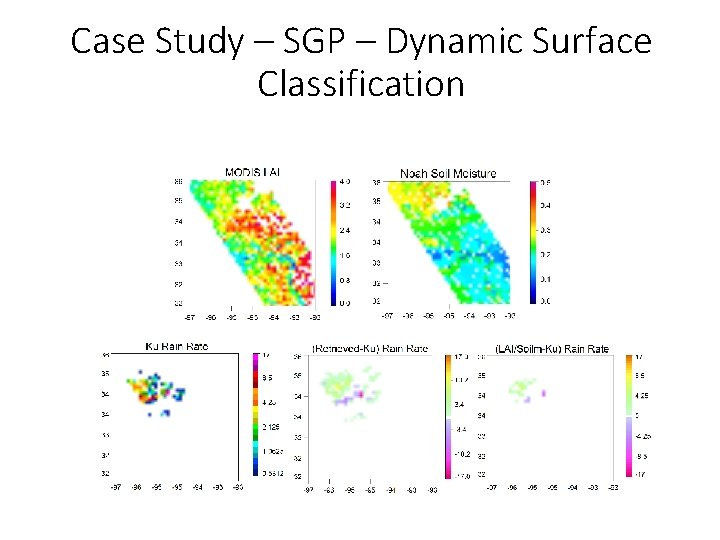
Case Study – SGP – Dynamic Surface Classification
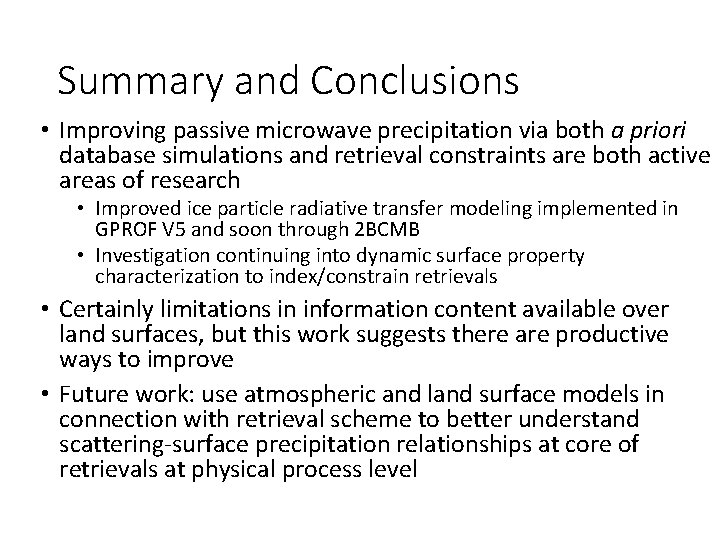
Summary and Conclusions • Improving passive microwave precipitation via both a priori database simulations and retrieval constraints are both active areas of research • Improved ice particle radiative transfer modeling implemented in GPROF V 5 and soon through 2 BCMB • Investigation continuing into dynamic surface property characterization to index/constrain retrievals • Certainly limitations in information content available over land surfaces, but this work suggests there are productive ways to improve • Future work: use atmospheric and land surface models in connection with retrieval scheme to better understand scattering-surface precipitation relationships at core of retrievals at physical process level
Co precipitation and post precipitation
Co precipitation and post precipitation
Microwave passive devices
Microwave repeater
Active and passive microwave devices
Building maintenance cost estimates
Marquis company estimates that annual manufacturing
The account analysis method estimates cost functions
1/4percentage
Job order costing
State the properties of least square estimators.
Tom murphy ucsd
Who global estimates on prevalence of hearing loss 2020
Creative industries economic estimates