GoodnessofFit Tests with Censored Data Edsel A Pena
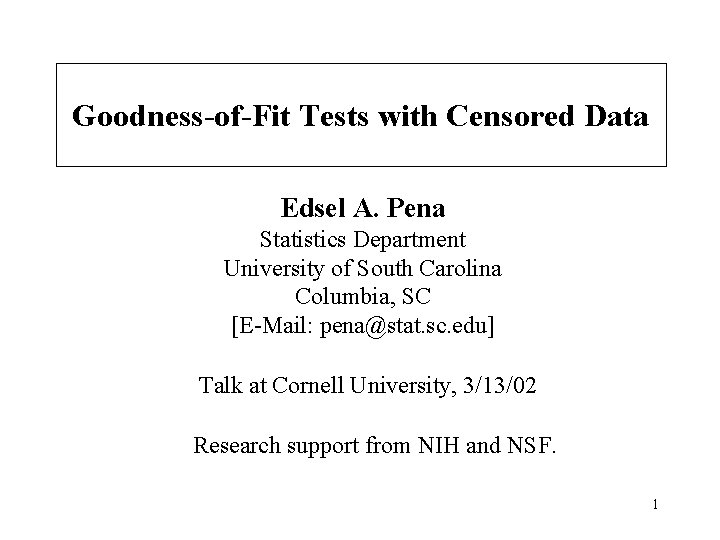
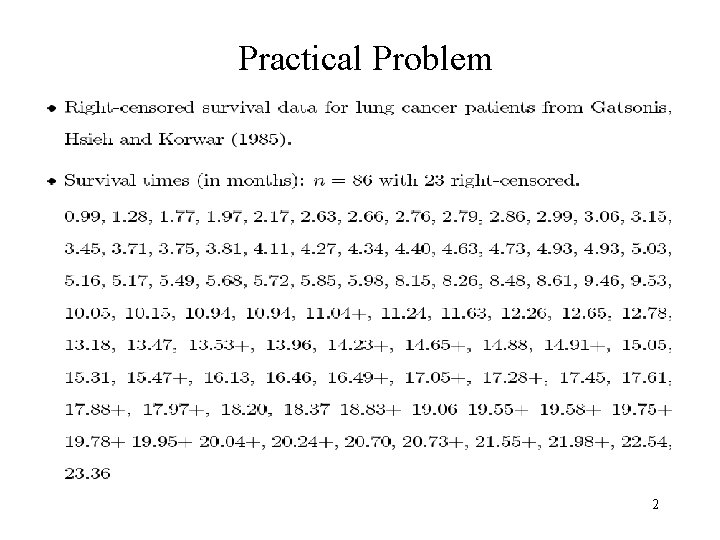
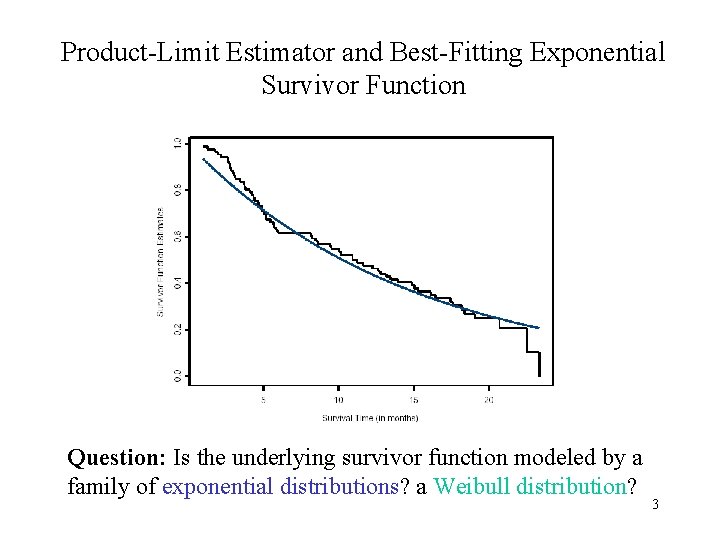
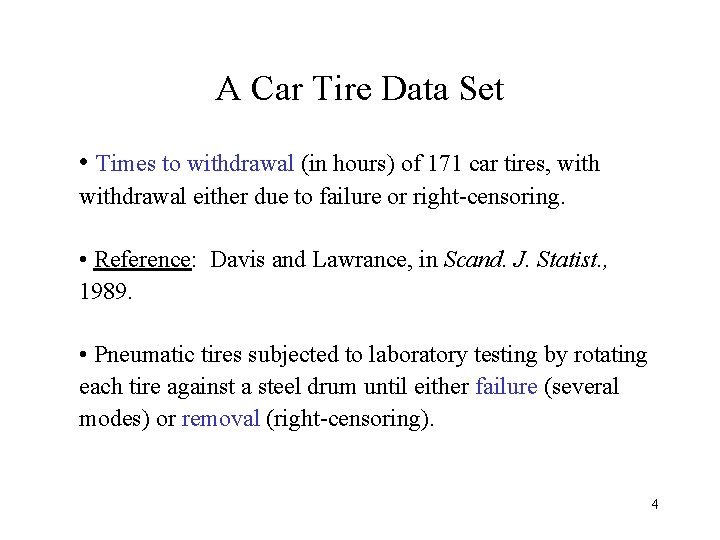
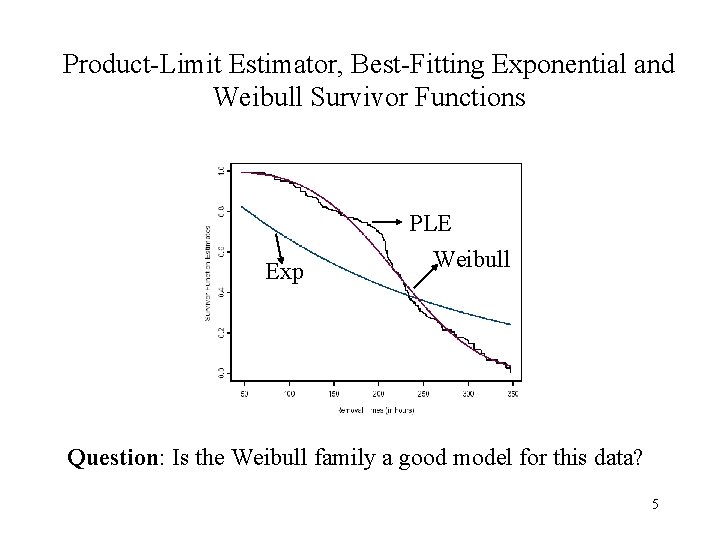
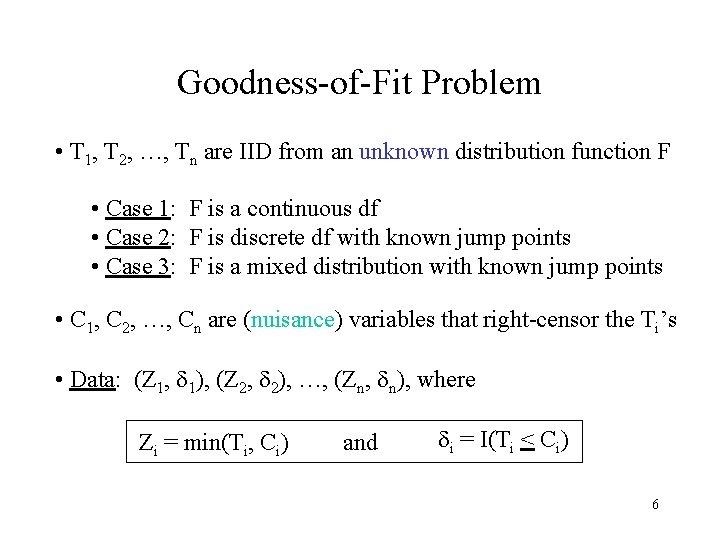
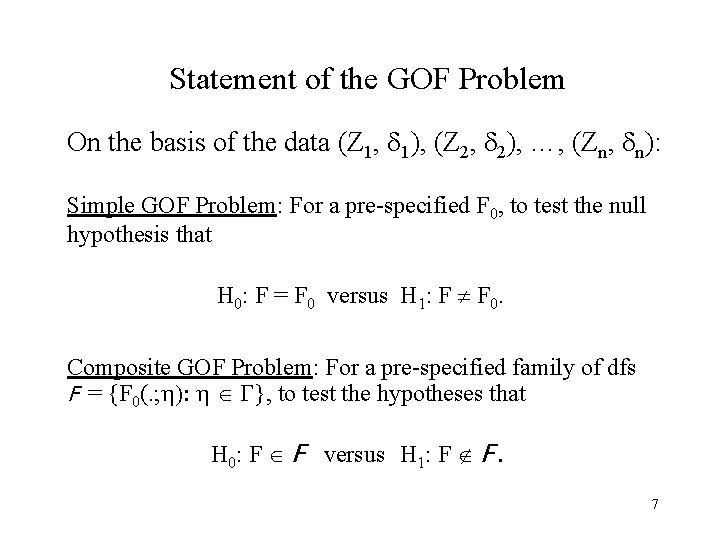
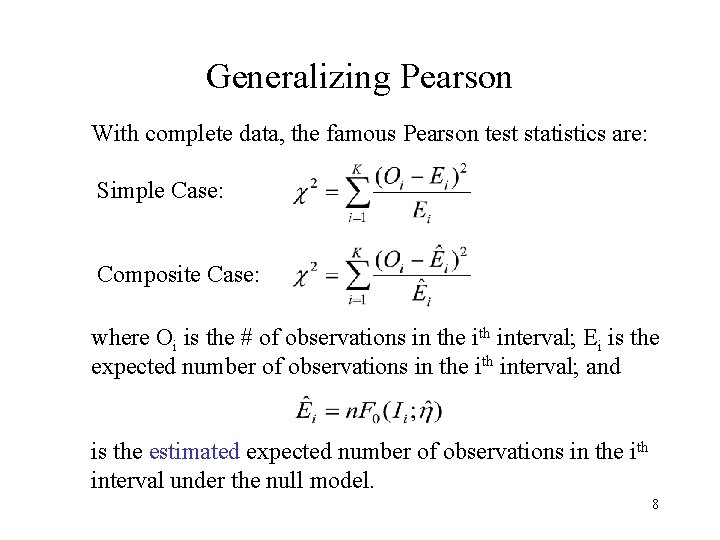
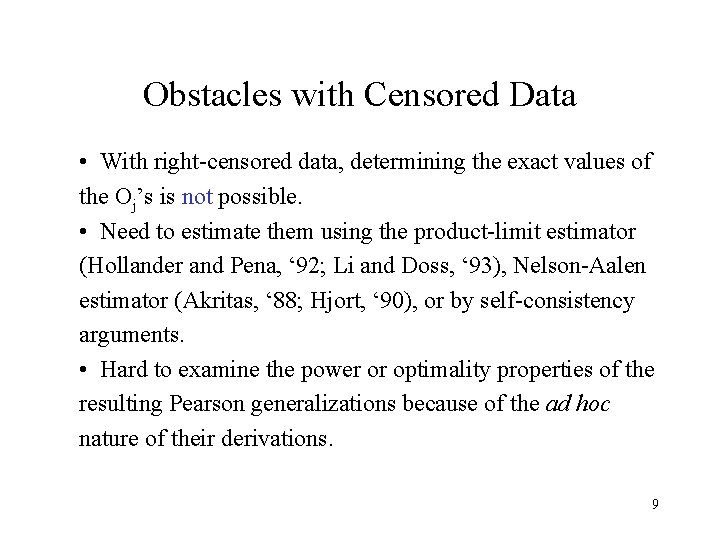
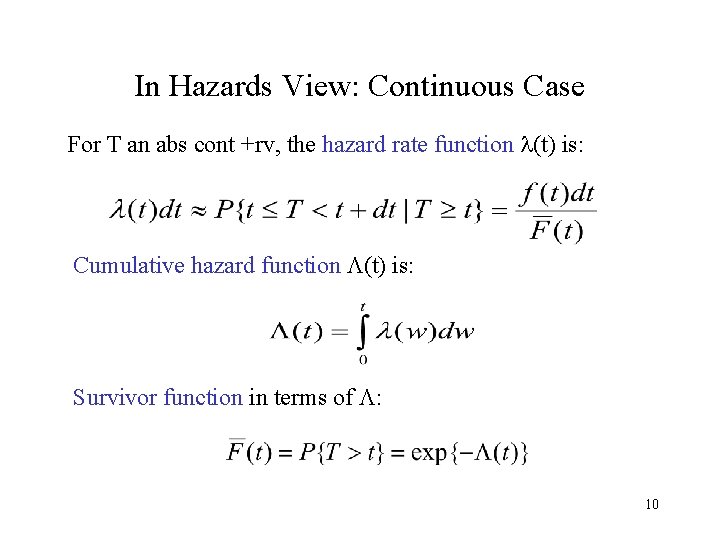
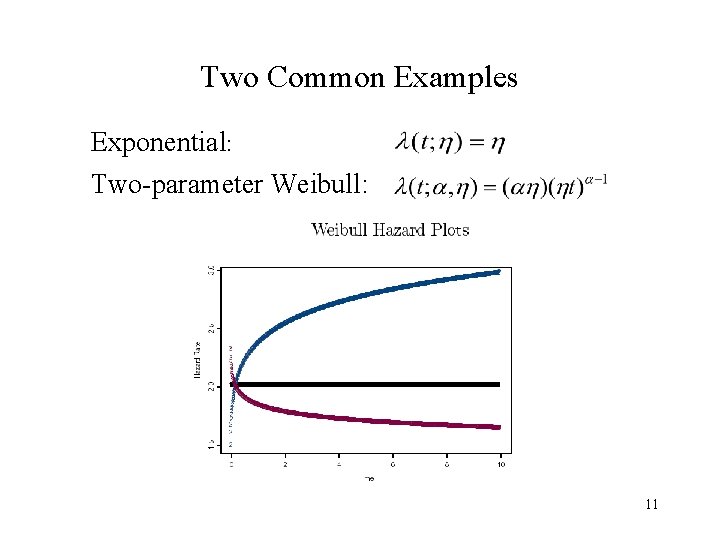
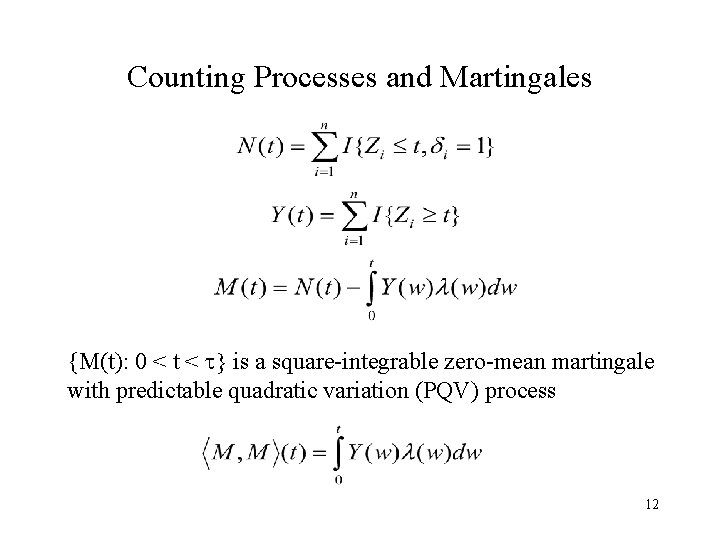
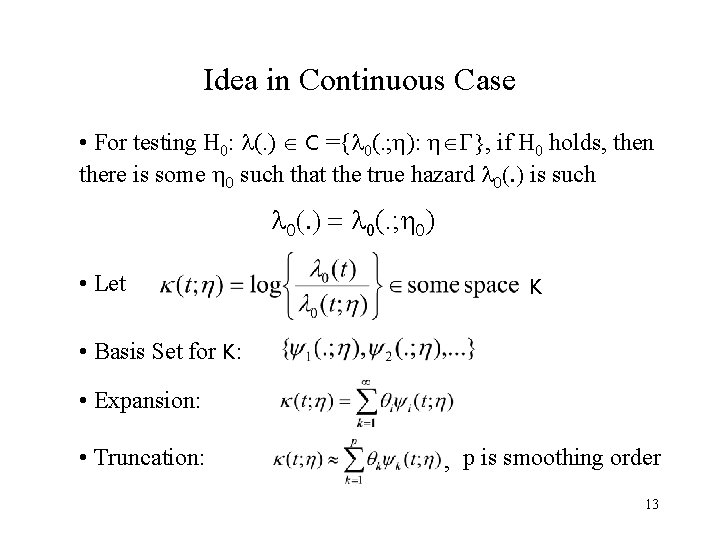
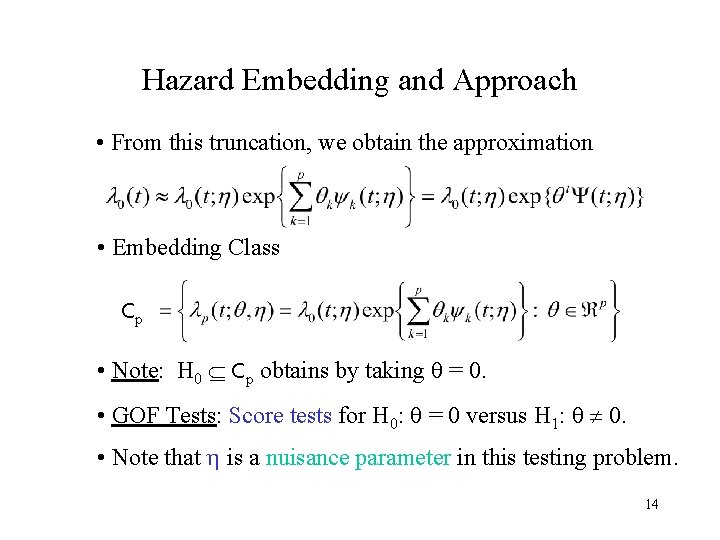
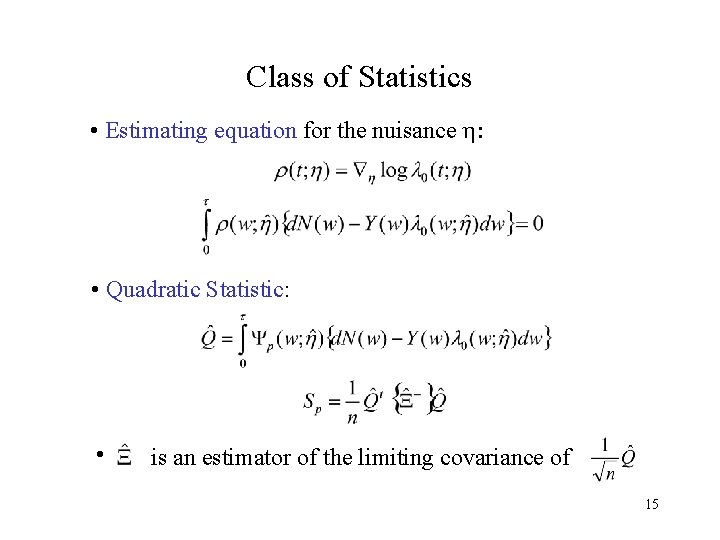
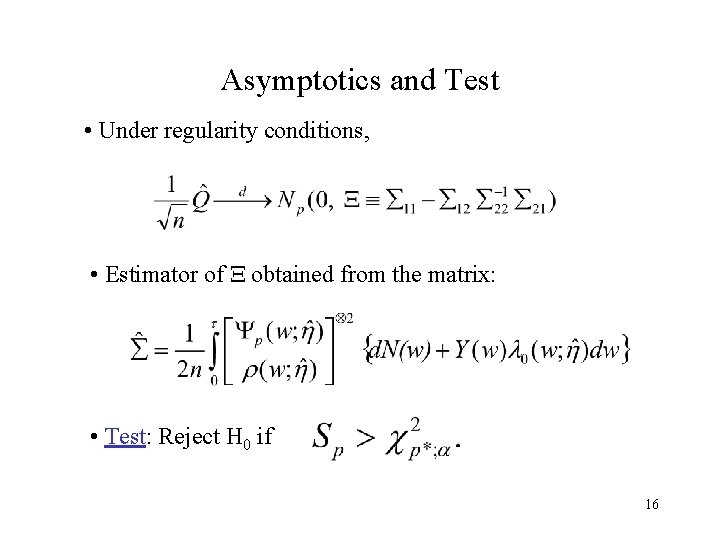
![A Choice of Generalizing Pearson • Partition [0, t] into 0 = a 1 A Choice of Generalizing Pearson • Partition [0, t] into 0 = a 1](https://slidetodoc.com/presentation_image_h2/5c62d5c1a25d34596a20c58b128b58d7/image-17.jpg)
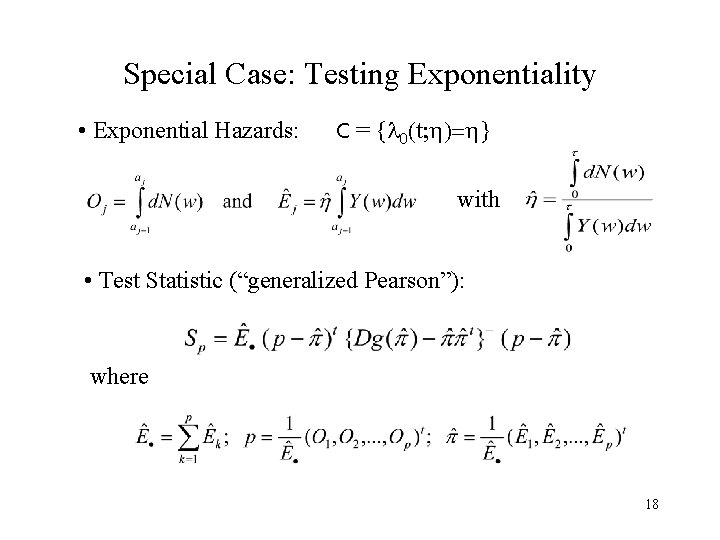
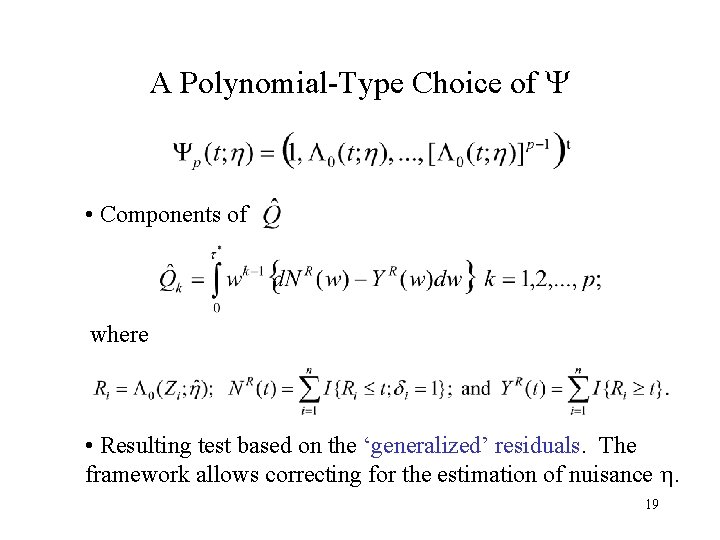
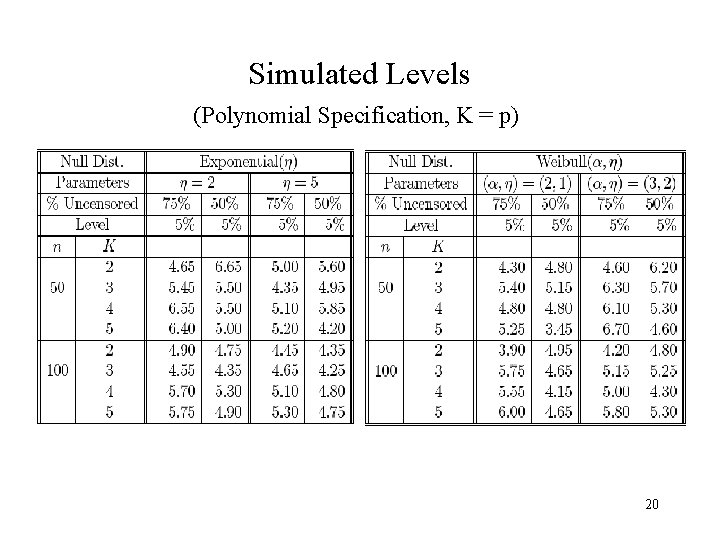
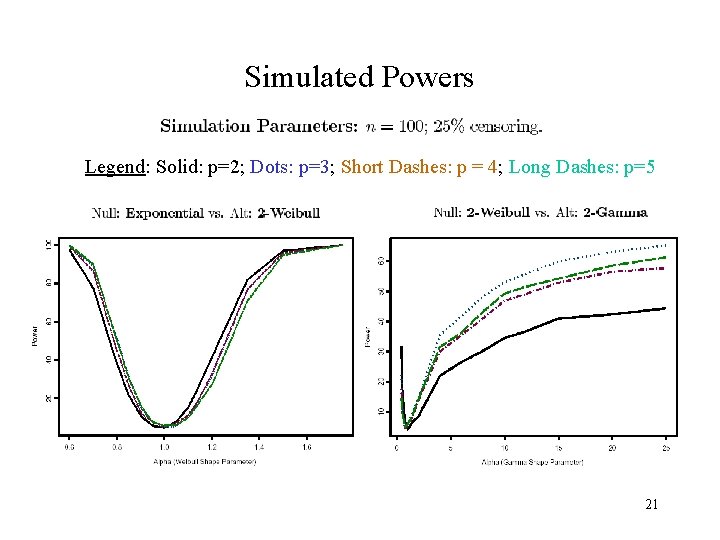
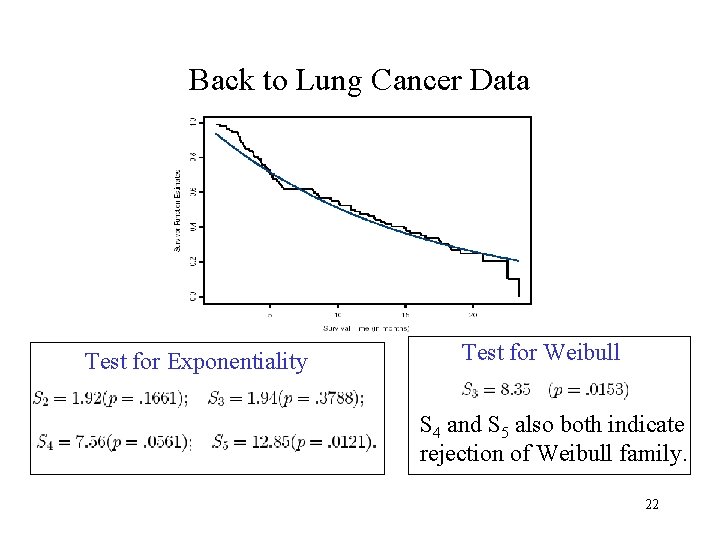
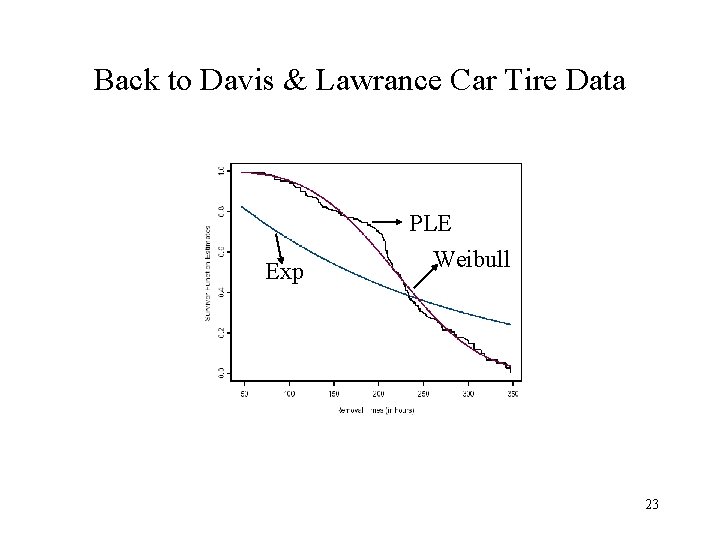
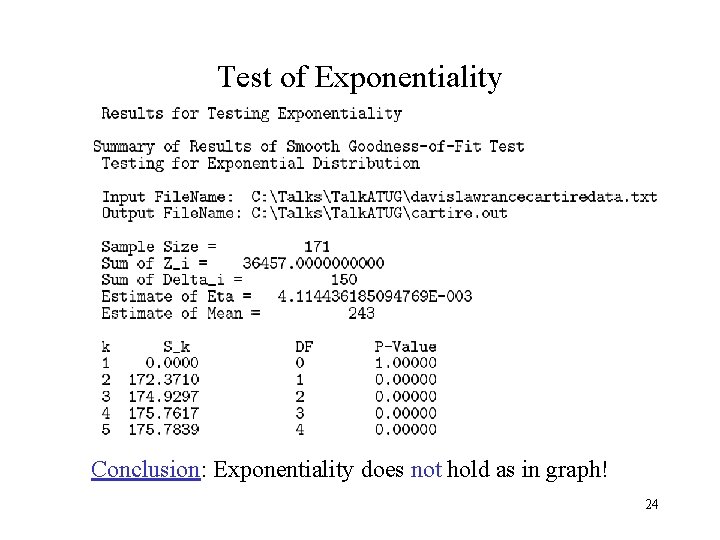
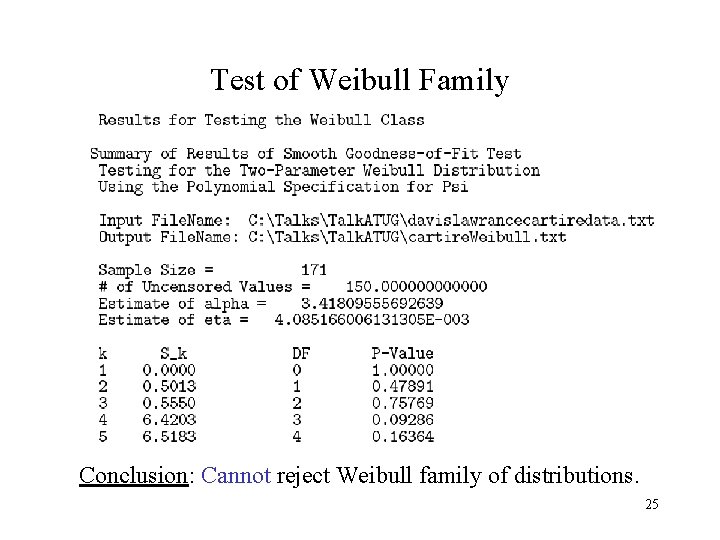
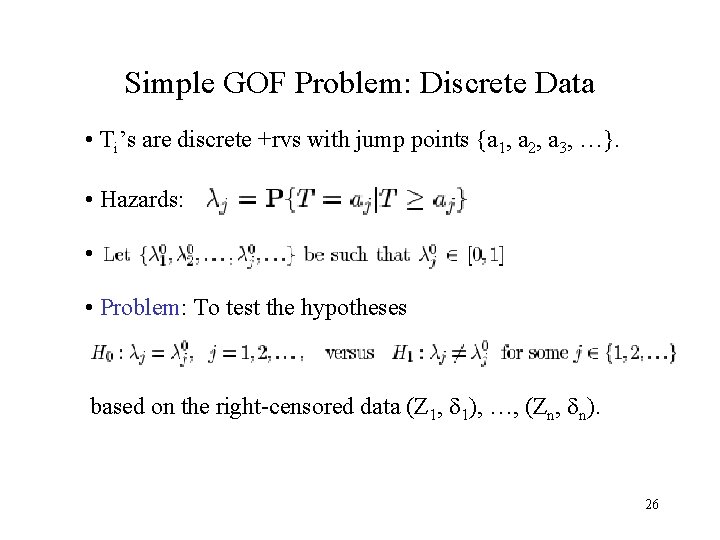
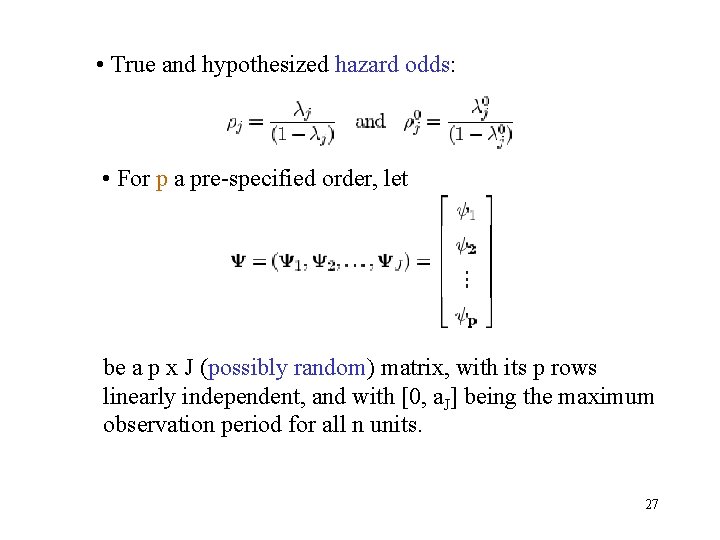
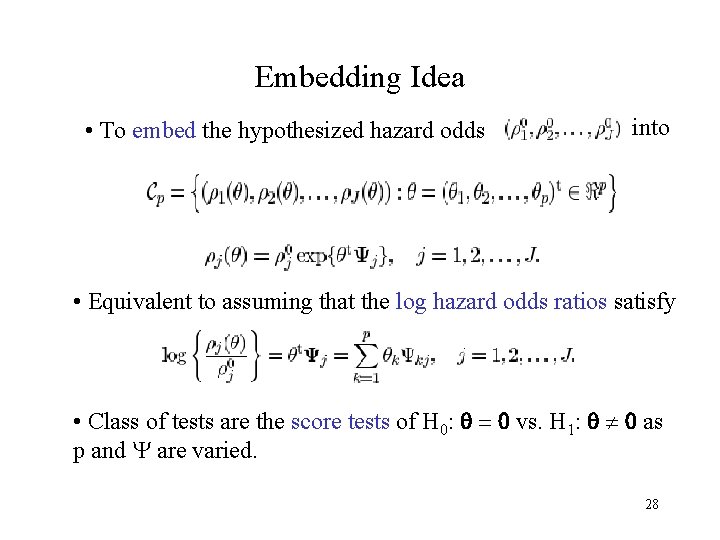
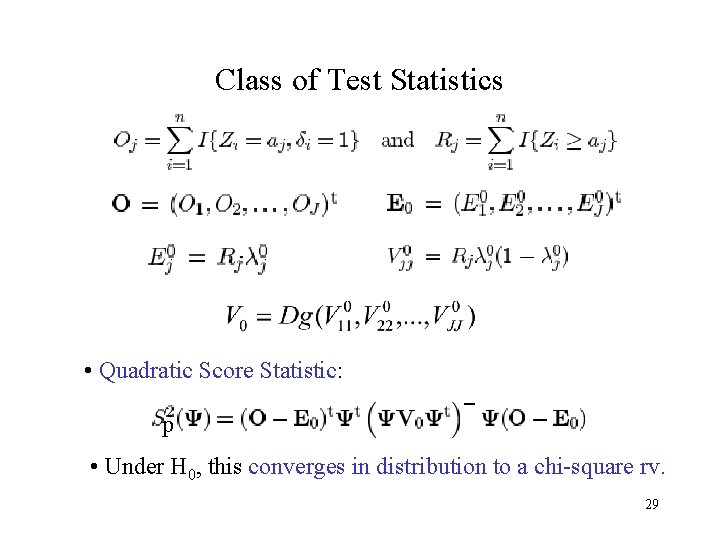
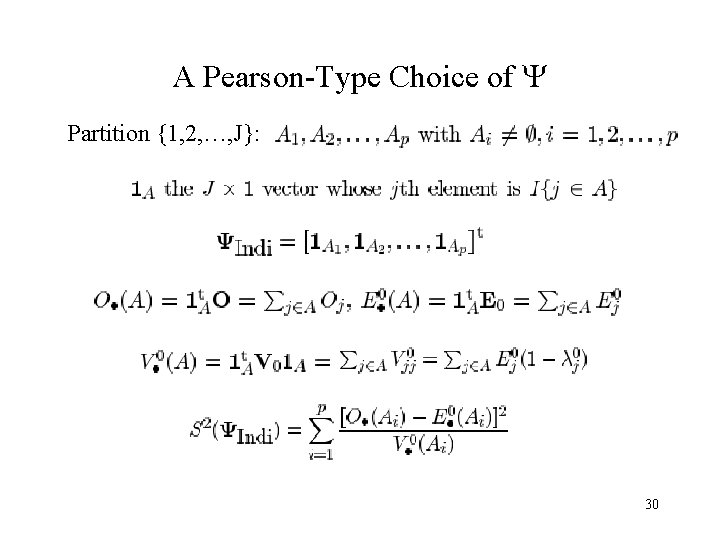
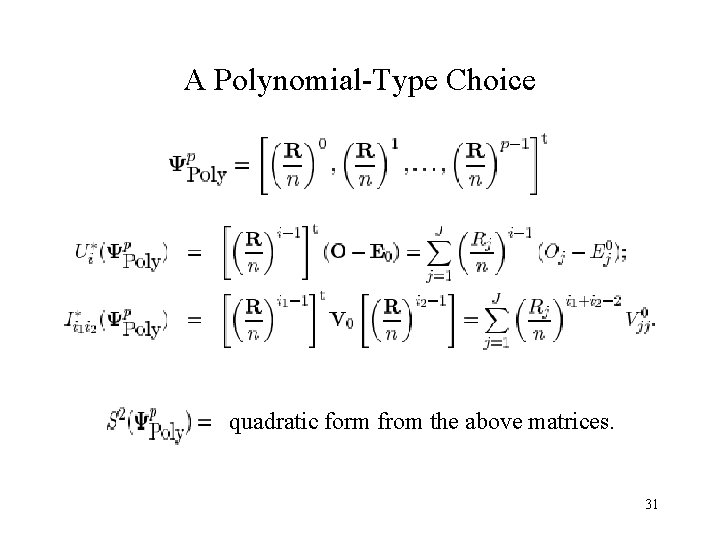
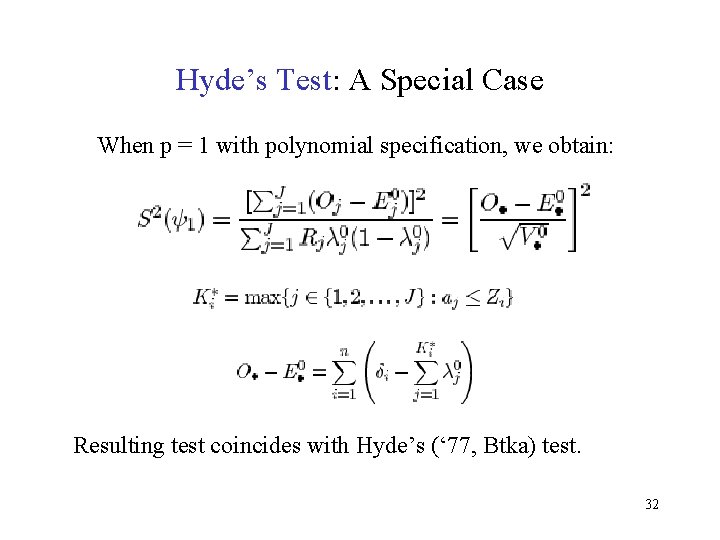
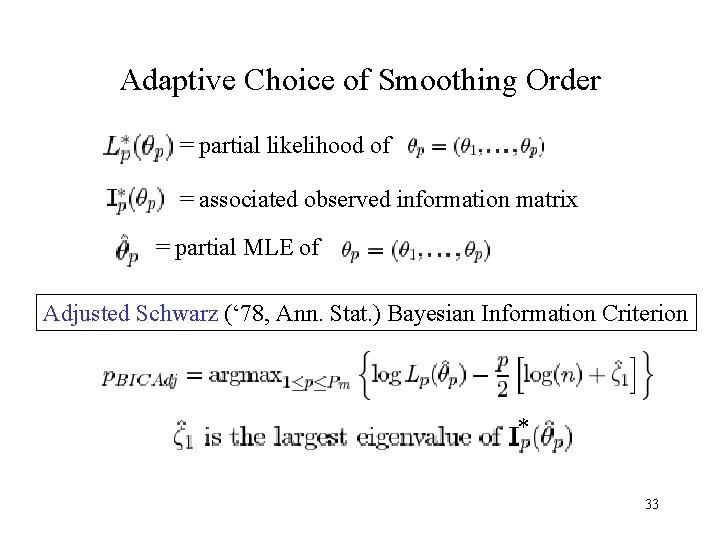
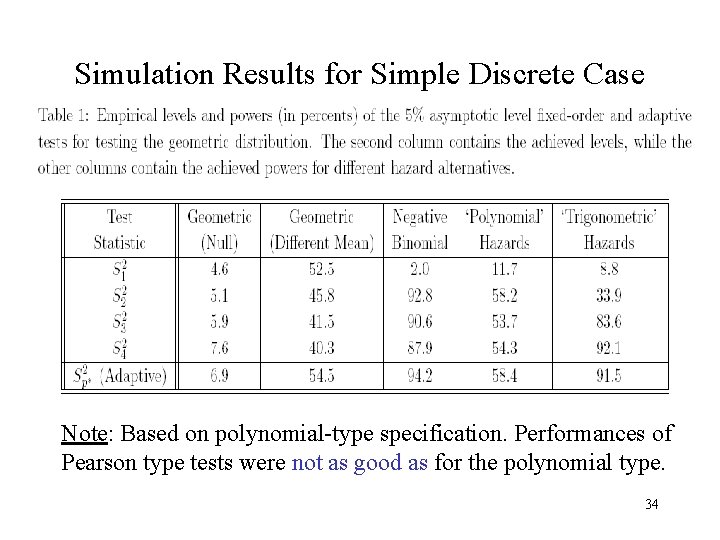
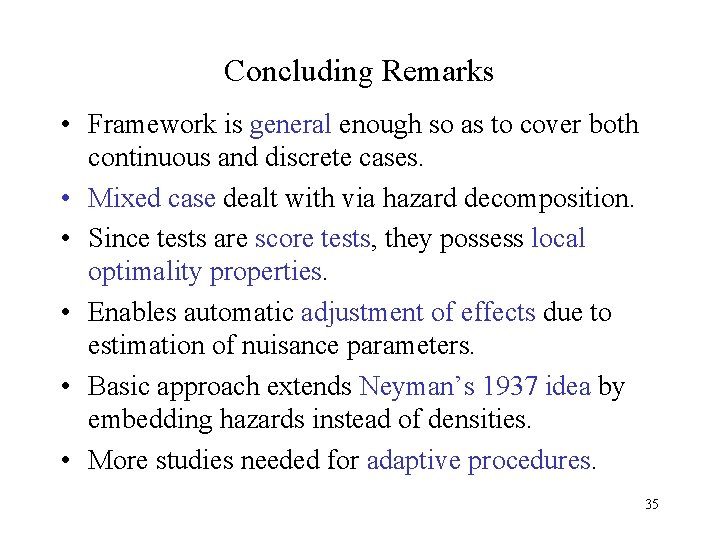
- Slides: 35
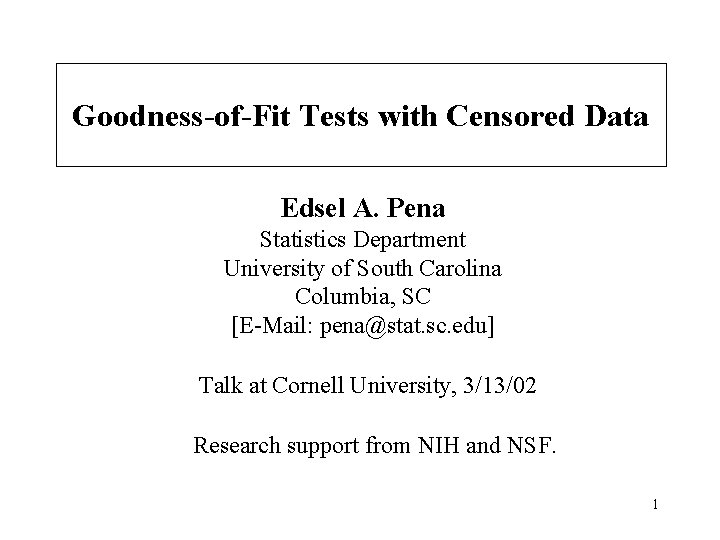
Goodness-of-Fit Tests with Censored Data Edsel A. Pena Statistics Department University of South Carolina Columbia, SC [E-Mail: pena@stat. sc. edu] Talk at Cornell University, 3/13/02 Research support from NIH and NSF. 1
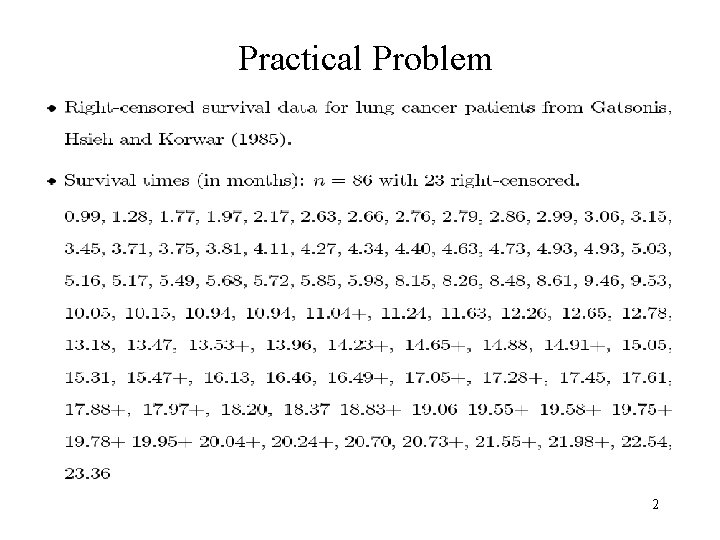
Practical Problem 2
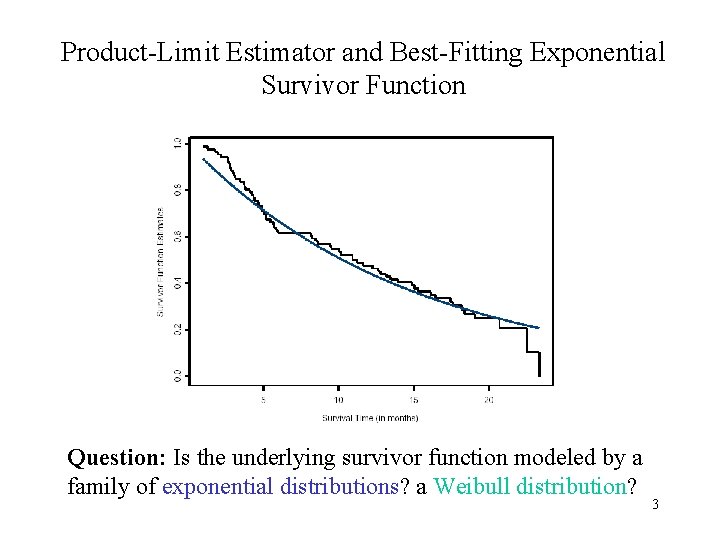
Product-Limit Estimator and Best-Fitting Exponential Survivor Function Question: Is the underlying survivor function modeled by a family of exponential distributions? a Weibull distribution? 3
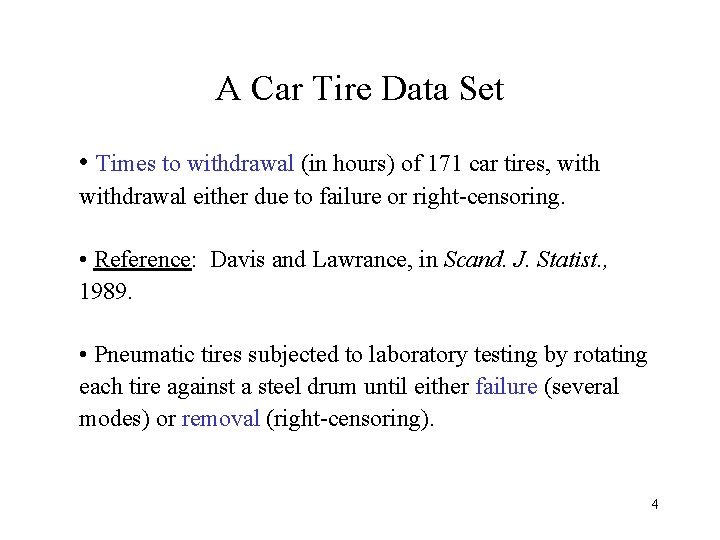
A Car Tire Data Set • Times to withdrawal (in hours) of 171 car tires, withdrawal either due to failure or right-censoring. • Reference: Davis and Lawrance, in Scand. J. Statist. , 1989. • Pneumatic tires subjected to laboratory testing by rotating each tire against a steel drum until either failure (several modes) or removal (right-censoring). 4
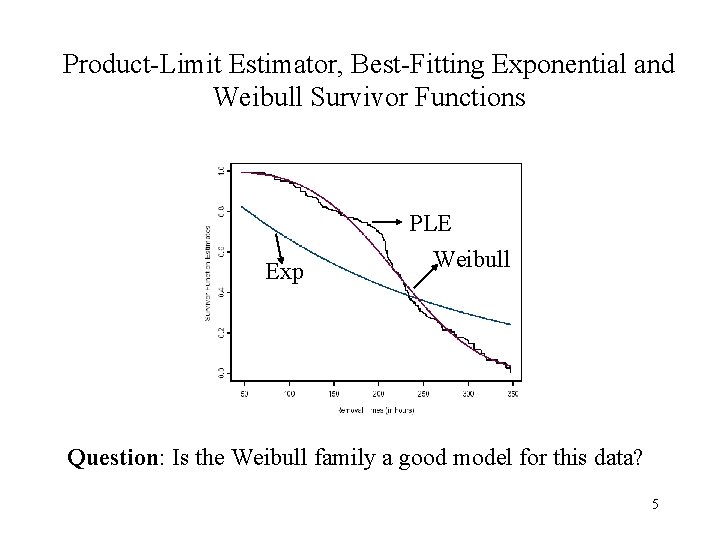
Product-Limit Estimator, Best-Fitting Exponential and Weibull Survivor Functions PLE Exp Weibull Question: Is the Weibull family a good model for this data? 5
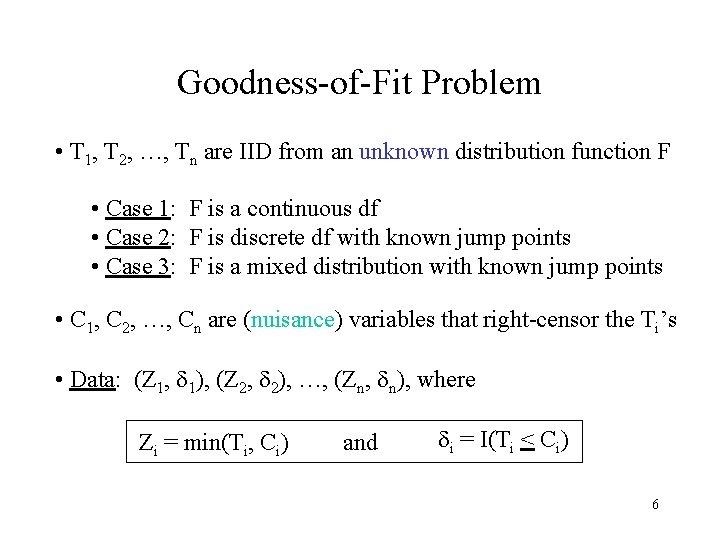
Goodness-of-Fit Problem • T 1, T 2, …, Tn are IID from an unknown distribution function F • Case 1: F is a continuous df • Case 2: F is discrete df with known jump points • Case 3: F is a mixed distribution with known jump points • C 1, C 2, …, Cn are (nuisance) variables that right-censor the Ti’s • Data: (Z 1, d 1), (Z 2, d 2), …, (Zn, dn), where Zi = min(Ti, Ci) and di = I(Ti < Ci) 6
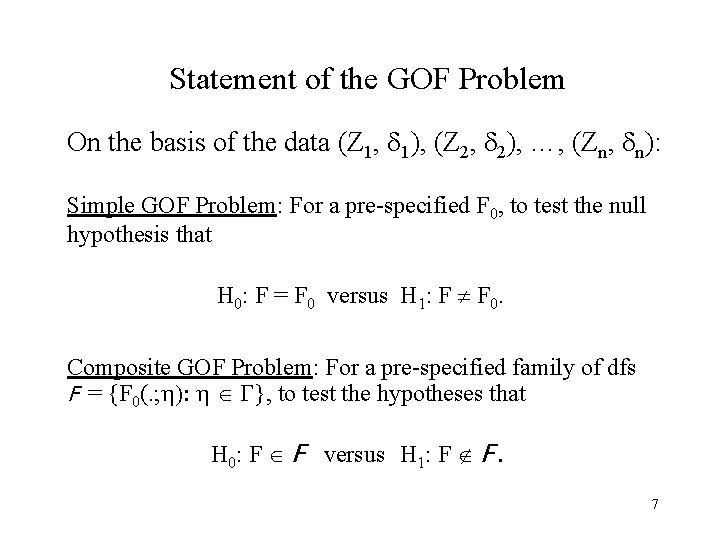
Statement of the GOF Problem On the basis of the data (Z 1, d 1), (Z 2, d 2), …, (Zn, dn): Simple GOF Problem: For a pre-specified F 0, to test the null hypothesis that H 0: F = F 0 versus H 1: F F 0. Composite GOF Problem: For a pre-specified family of dfs F = {F 0(. ; h): h G}, to test the hypotheses that H 0: F F versus H 1: F F. 7
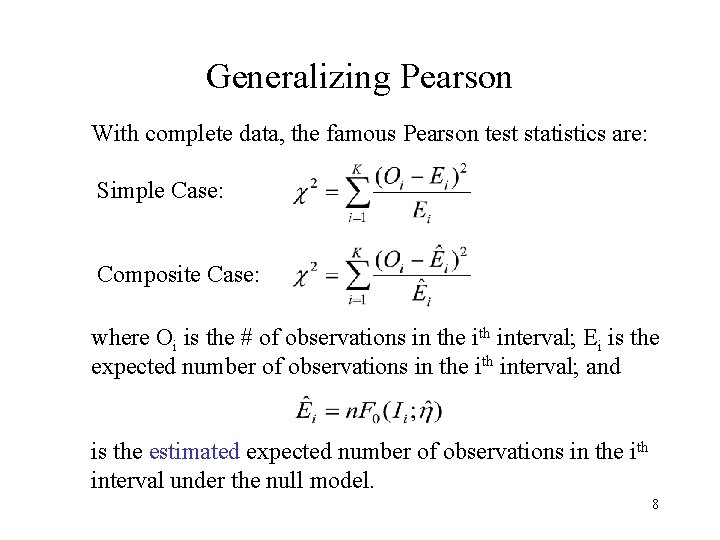
Generalizing Pearson With complete data, the famous Pearson test statistics are: Simple Case: Composite Case: where Oi is the # of observations in the ith interval; Ei is the expected number of observations in the ith interval; and is the estimated expected number of observations in the ith interval under the null model. 8
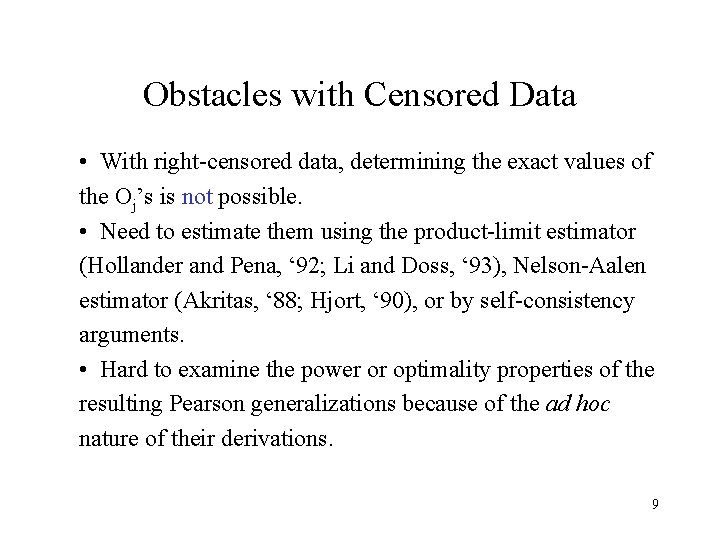
Obstacles with Censored Data • With right-censored data, determining the exact values of the Oj’s is not possible. • Need to estimate them using the product-limit estimator (Hollander and Pena, ‘ 92; Li and Doss, ‘ 93), Nelson-Aalen estimator (Akritas, ‘ 88; Hjort, ‘ 90), or by self-consistency arguments. • Hard to examine the power or optimality properties of the resulting Pearson generalizations because of the ad hoc nature of their derivations. 9
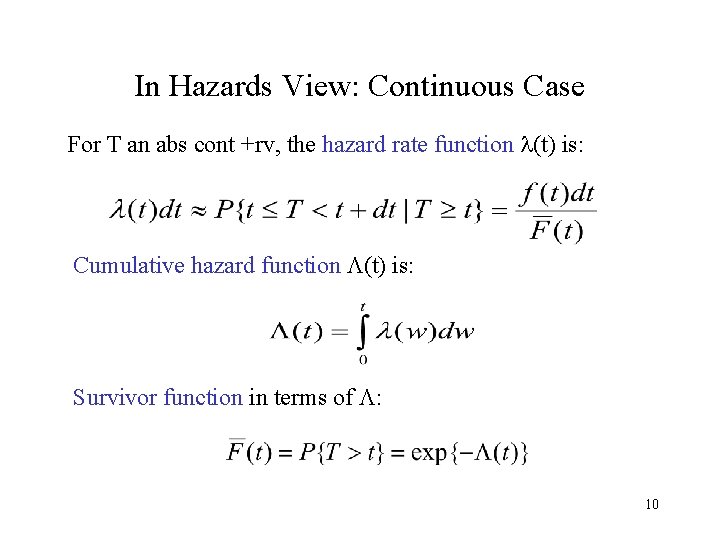
In Hazards View: Continuous Case For T an abs cont +rv, the hazard rate function (t) is: Cumulative hazard function (t) is: Survivor function in terms of : 10
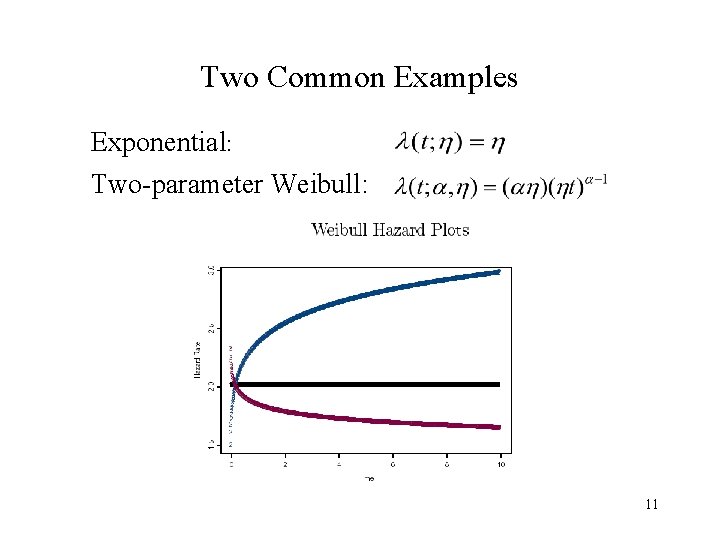
Two Common Examples Exponential: Two-parameter Weibull: 11
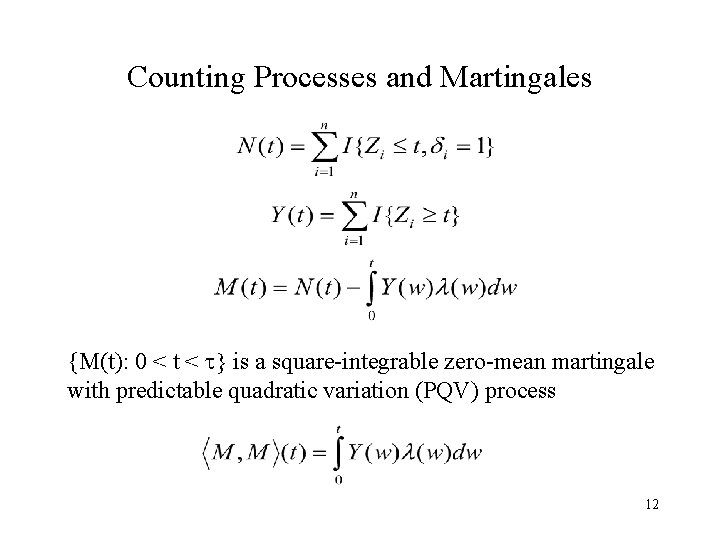
Counting Processes and Martingales {M(t): 0 < t} is a square-integrable zero-mean martingale with predictable quadratic variation (PQV) process 12
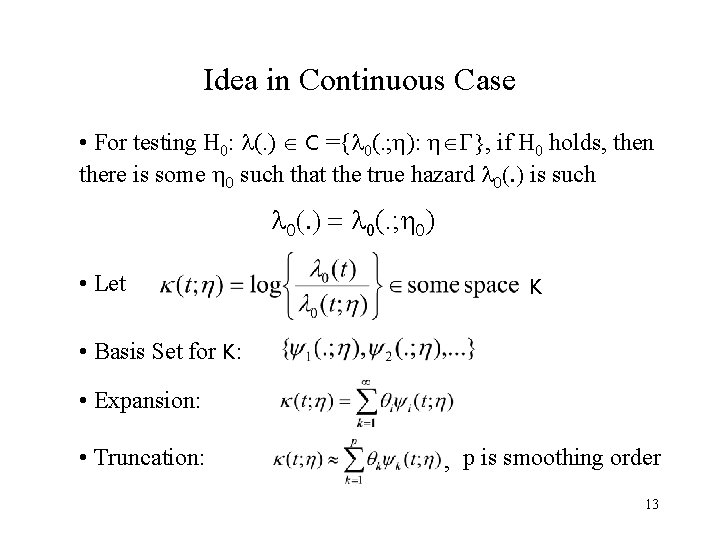
Idea in Continuous Case • For testing H 0: (. ) C ={ 0(. ; h): h G}, if H 0 holds, then there is some h 0 such that the true hazard 0(. ) is such 0(. ) = 0(. ; h 0) • Let K • Basis Set for K: • Expansion: • Truncation: , p is smoothing order 13
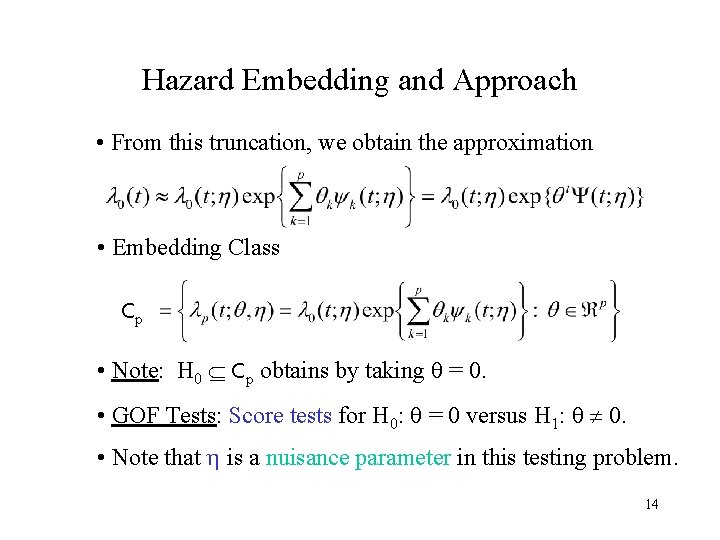
Hazard Embedding and Approach • From this truncation, we obtain the approximation • Embedding Class Cp • Note: H 0 Cp obtains by taking = 0. • GOF Tests: Score tests for H 0: = 0 versus H 1: 0. • Note that h is a nuisance parameter in this testing problem. 14
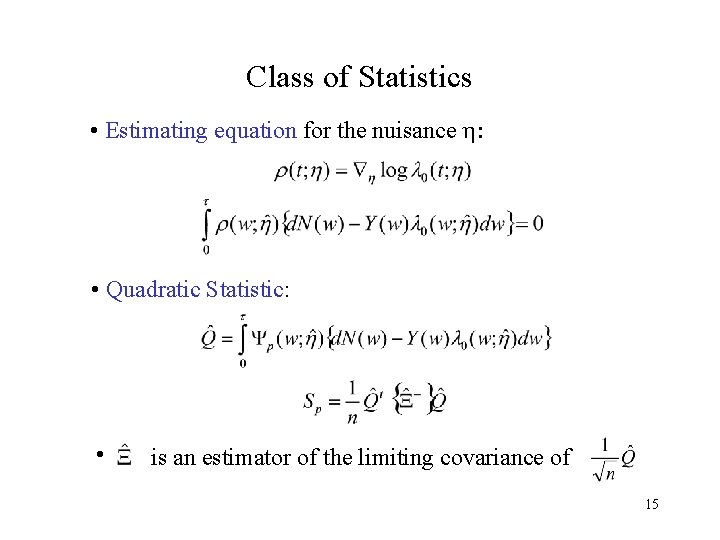
Class of Statistics • Estimating equation for the nuisance h: • Quadratic Statistic: • is an estimator of the limiting covariance of 15
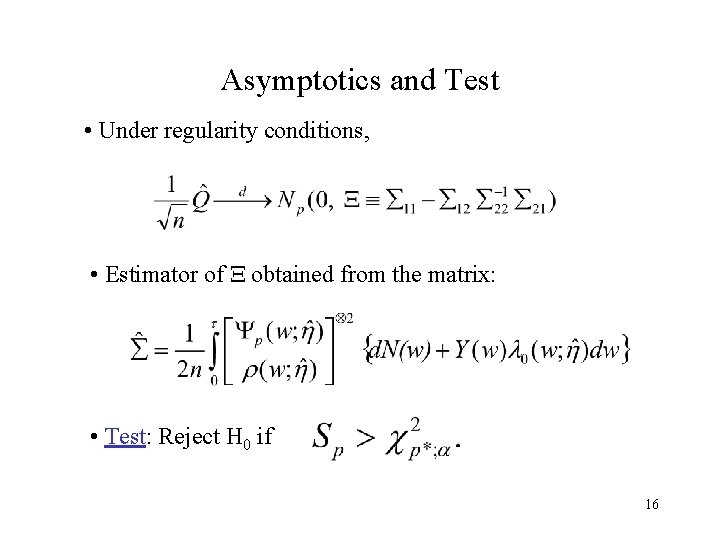
Asymptotics and Test • Under regularity conditions, • Estimator of X obtained from the matrix: • Test: Reject H 0 if 16
![A Choice of Generalizing Pearson Partition 0 t into 0 a 1 A Choice of Generalizing Pearson • Partition [0, t] into 0 = a 1](https://slidetodoc.com/presentation_image_h2/5c62d5c1a25d34596a20c58b128b58d7/image-17.jpg)
A Choice of Generalizing Pearson • Partition [0, t] into 0 = a 1 < a 2 < … < ap = t, and let • Then • are dynamic expected frequencies 17
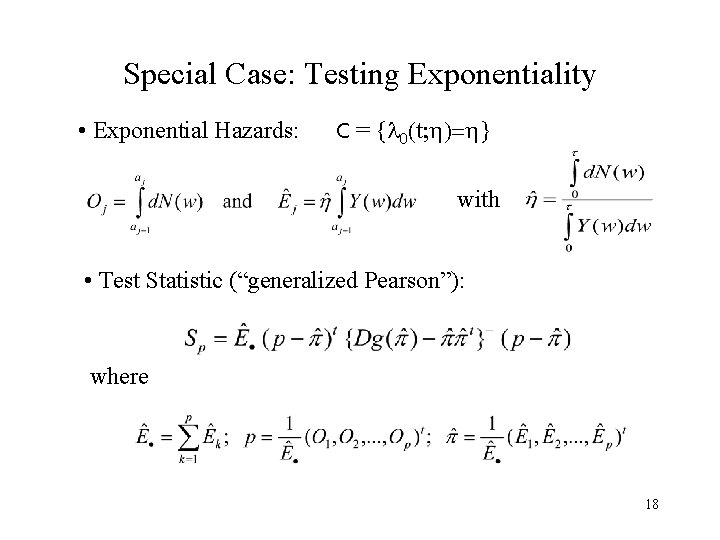
Special Case: Testing Exponentiality • Exponential Hazards: C = { 0(t; h)=h} with • Test Statistic (“generalized Pearson”): where 18
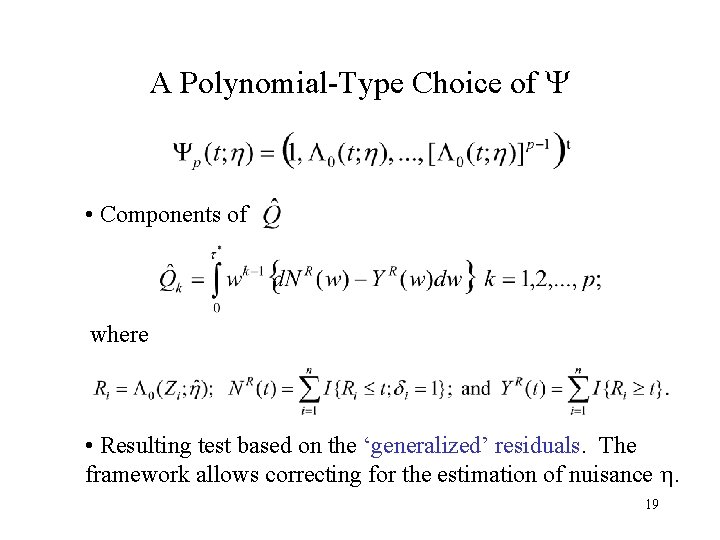
A Polynomial-Type Choice of • Components of where • Resulting test based on the ‘generalized’ residuals. The framework allows correcting for the estimation of nuisance h. 19
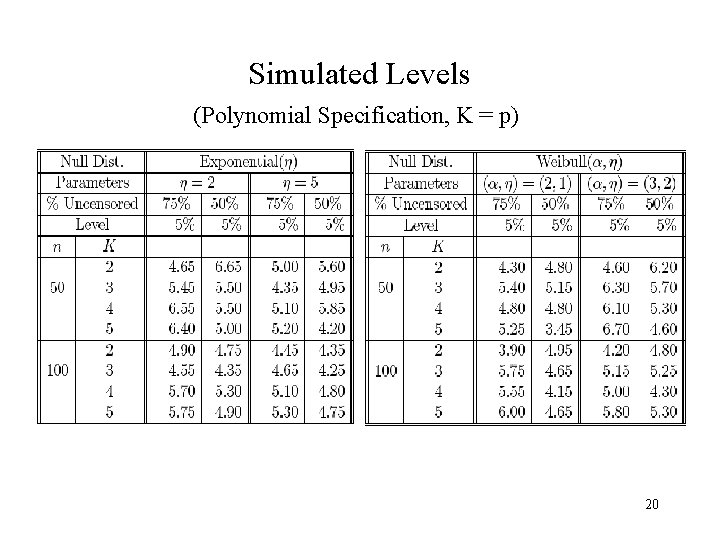
Simulated Levels (Polynomial Specification, K = p) 20
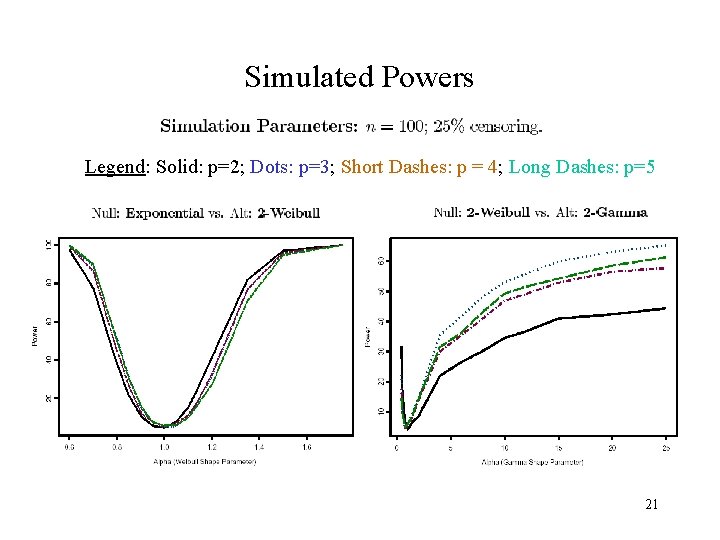
Simulated Powers Legend: Solid: p=2; Dots: p=3; Short Dashes: p = 4; Long Dashes: p=5 21
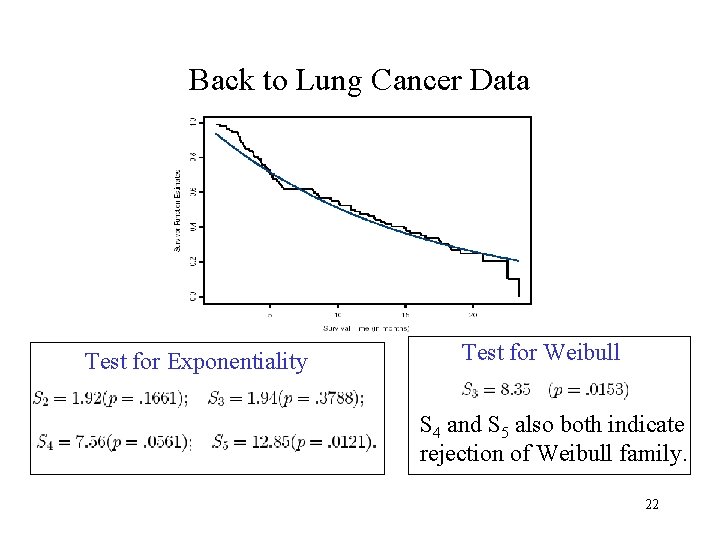
Back to Lung Cancer Data Test for Exponentiality Test for Weibull S 4 and S 5 also both indicate rejection of Weibull family. 22
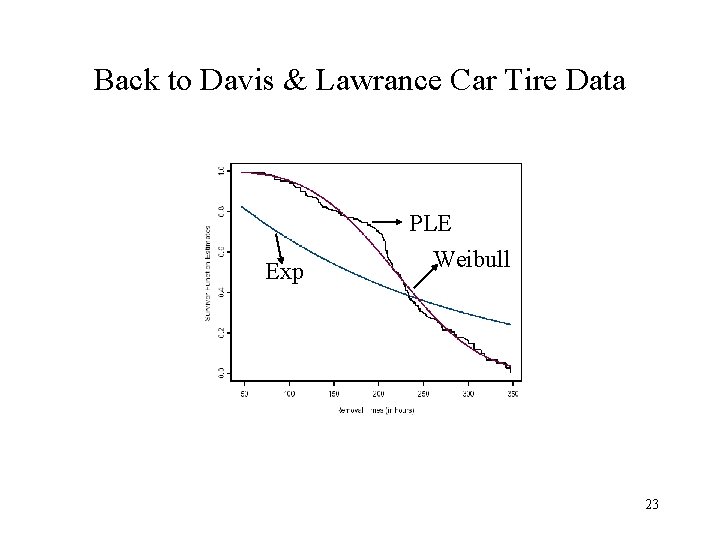
Back to Davis & Lawrance Car Tire Data PLE Exp Weibull 23
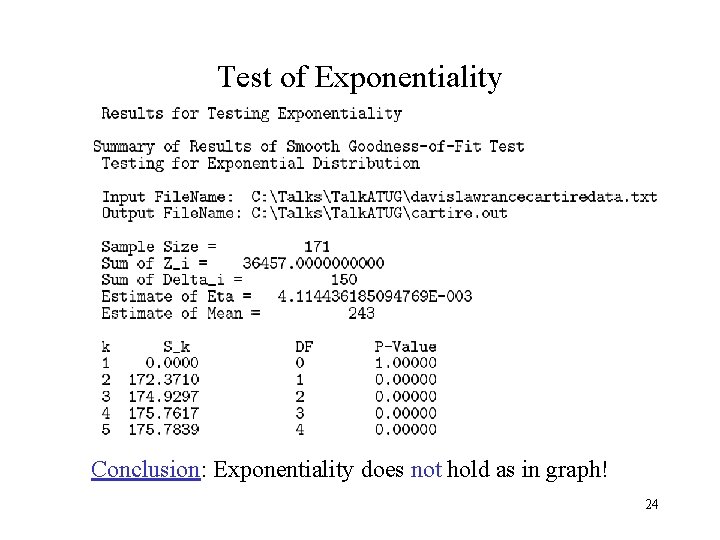
Test of Exponentiality Conclusion: Exponentiality does not hold as in graph! 24
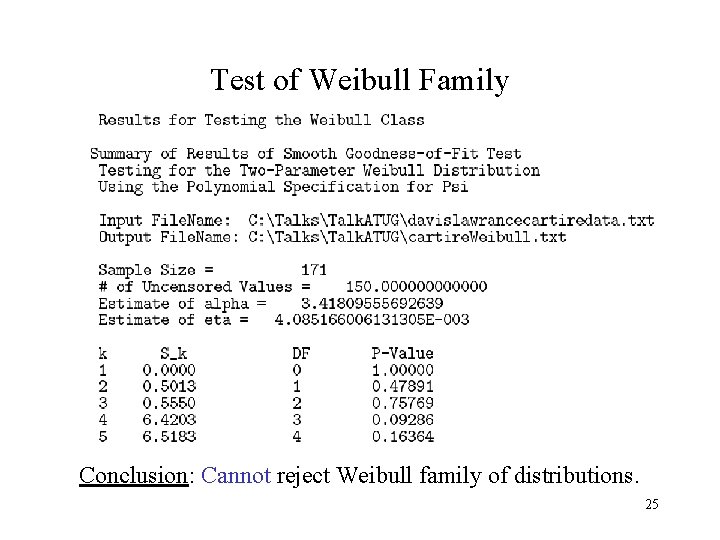
Test of Weibull Family Conclusion: Cannot reject Weibull family of distributions. 25
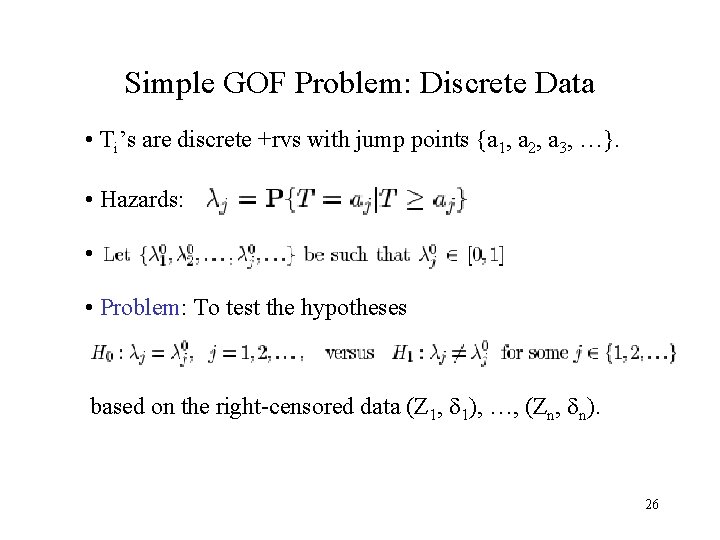
Simple GOF Problem: Discrete Data • Ti’s are discrete +rvs with jump points {a 1, a 2, a 3, …}. • Hazards: • • Problem: To test the hypotheses based on the right-censored data (Z 1, d 1), …, (Zn, dn). 26
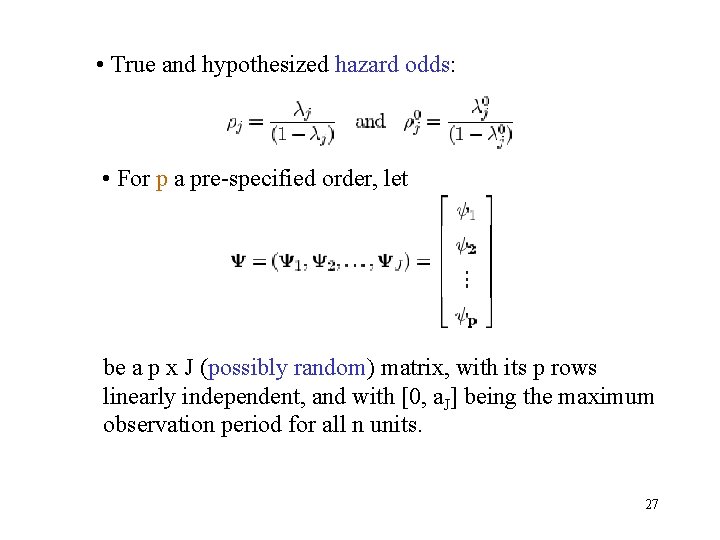
• True and hypothesized hazard odds: • For p a pre-specified order, let be a p x J (possibly random) matrix, with its p rows linearly independent, and with [0, a. J] being the maximum observation period for all n units. 27
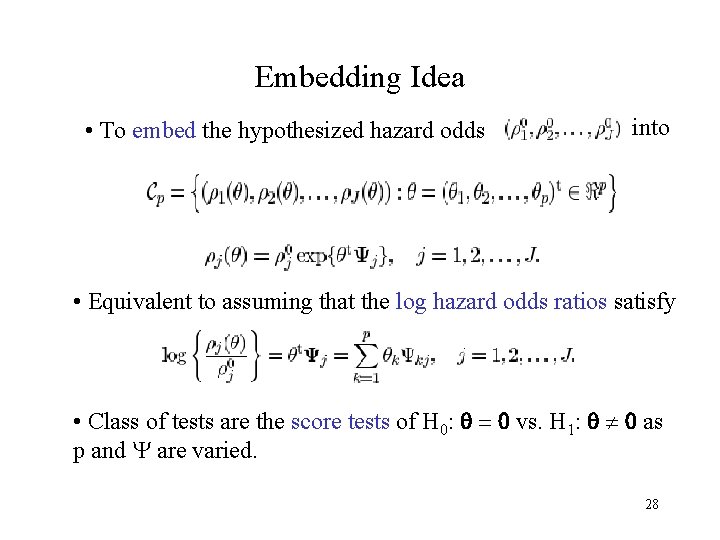
Embedding Idea • To embed the hypothesized hazard odds into • Equivalent to assuming that the log hazard odds ratios satisfy • Class of tests are the score tests of H 0: q = 0 vs. H 1: q 0 as p and are varied. 28
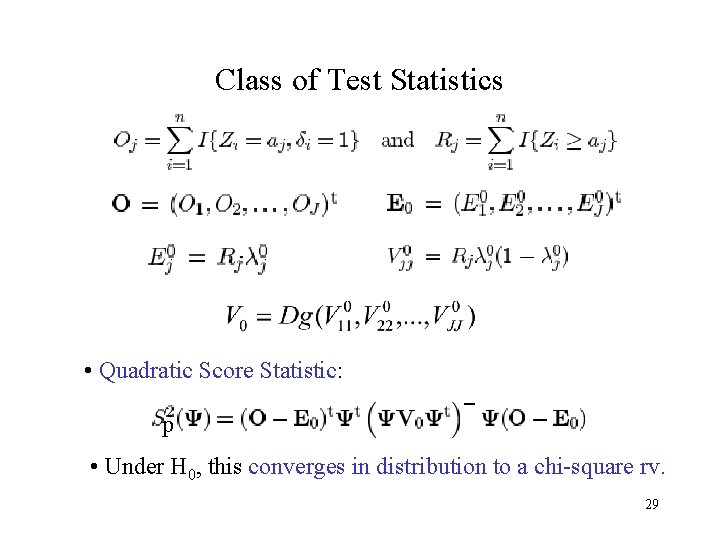
Class of Test Statistics • Quadratic Score Statistic: p • Under H 0, this converges in distribution to a chi-square rv. 29
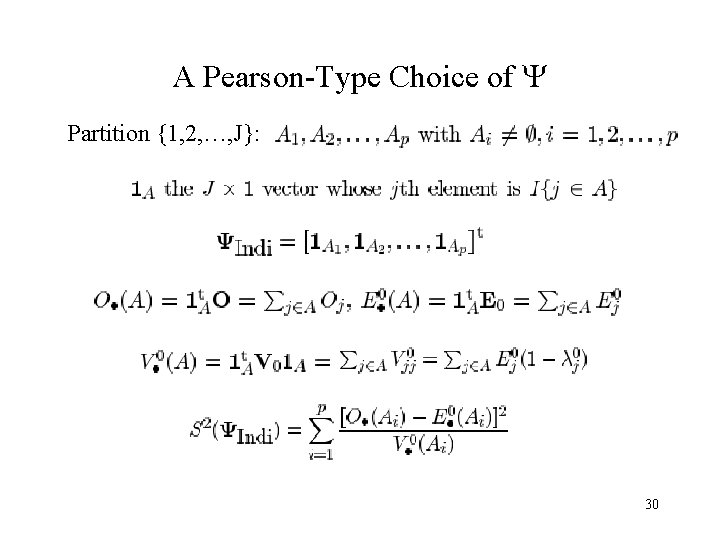
A Pearson-Type Choice of Partition {1, 2, …, J}: 30
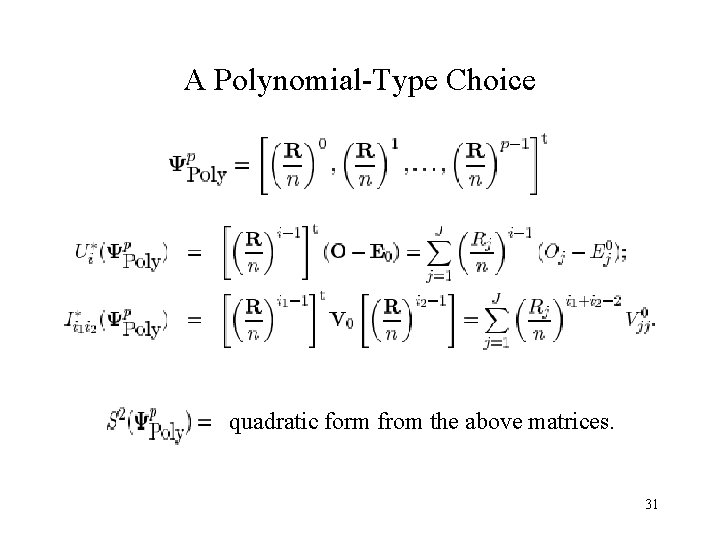
A Polynomial-Type Choice quadratic form from the above matrices. 31
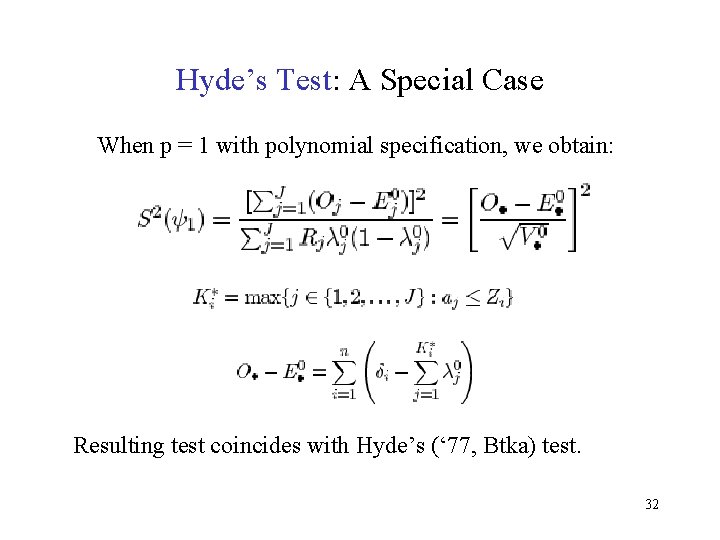
Hyde’s Test: A Special Case When p = 1 with polynomial specification, we obtain: Resulting test coincides with Hyde’s (‘ 77, Btka) test. 32
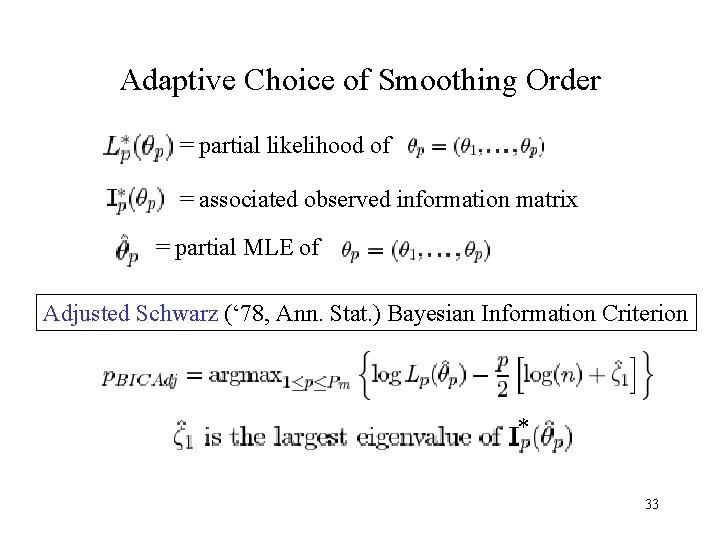
Adaptive Choice of Smoothing Order = partial likelihood of = associated observed information matrix = partial MLE of Adjusted Schwarz (‘ 78, Ann. Stat. ) Bayesian Information Criterion * 33
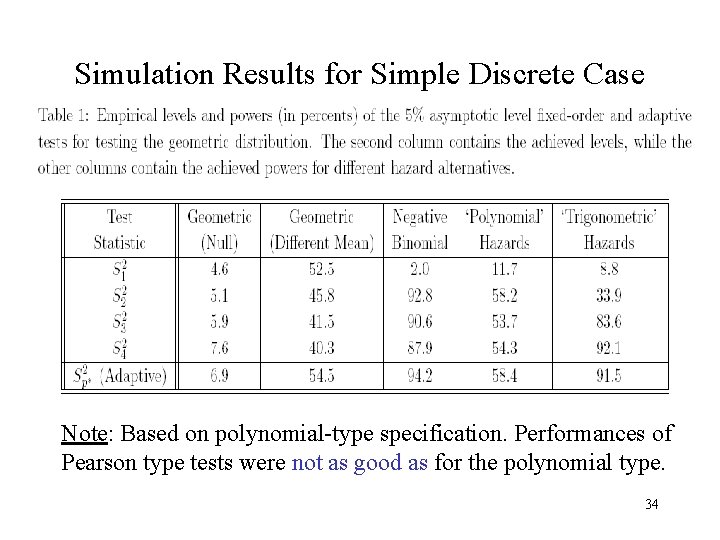
Simulation Results for Simple Discrete Case Note: Based on polynomial-type specification. Performances of Pearson type tests were not as good as for the polynomial type. 34
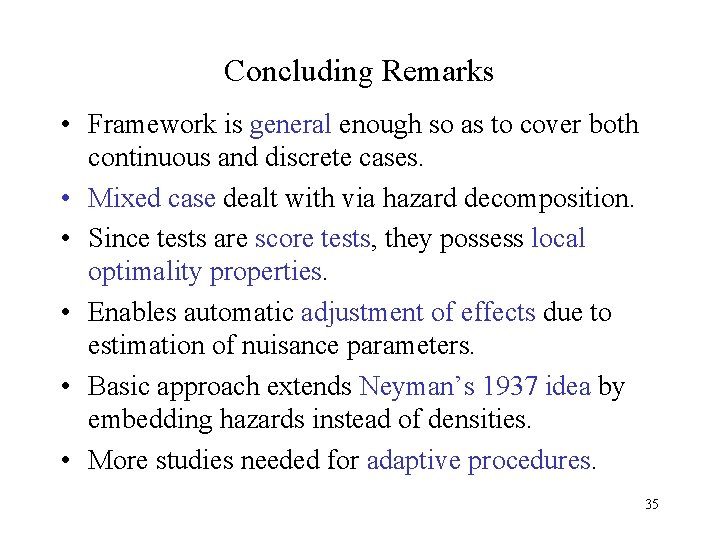
Concluding Remarks • Framework is general enough so as to cover both continuous and discrete cases. • Mixed case dealt with via hazard decomposition. • Since tests are score tests, they possess local optimality properties. • Enables automatic adjustment of effects due to estimation of nuisance parameters. • Basic approach extends Neyman’s 1937 idea by embedding hazards instead of densities. • More studies needed for adaptive procedures. 35
Edsel pena
Perdigo
Pepe piña pica piña pica piña pepe peña
Ace different tests iq tests still
Statistical tests for ordinal data
Biochemical data, medical tests, and procedures (bd)
Contoh soal peta pekerja dan mesin
Le llaman guerrero
Struttura inferno
Canto 26 inferno testo
Alessandra pena
Literatura mexicana
A ogni giorno basta la sua pena
Dr alberto pena
Teorie assolute e relative della pena
Analisi testo argomentativo esempi
Pena in the time of the butterflies
What is dissociative identity disorder
Vale la pena leer
Ivan antonio peña rocha
Ser feliz es no tener miedo de los propios sentimientos
Pena muscle stimulator
Que es el valor de la responsabilidad
Queda livre
Legge di contrappasso
Irene peña grau
O que é atenuante
Conservas peña cambados
Year 4 mental maths test
Turbidity apes
Romberga tests
Dirty mind test questions
Number sense uil practice
Uil mathematics test
Mechanical reasoning exam
Shrek ordinary world