FTIR OC EC predictions in IMPROVE CSN networks
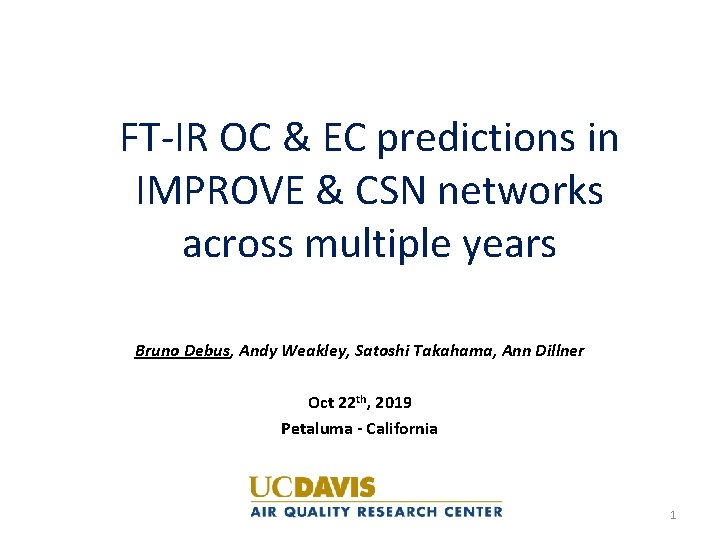
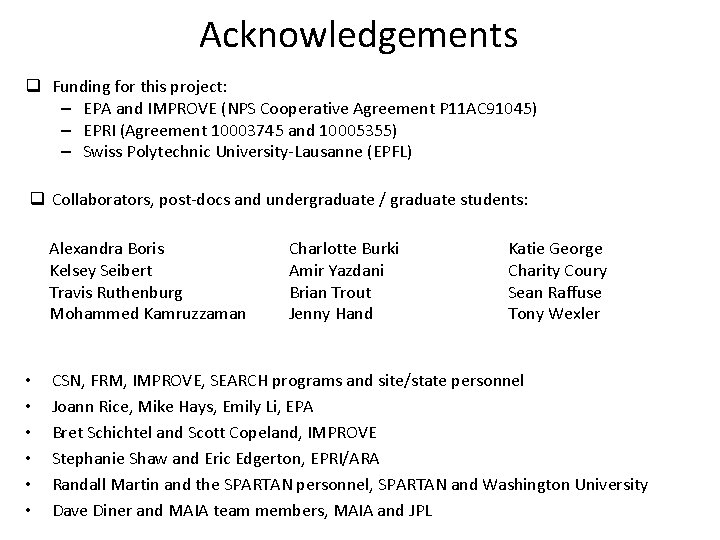
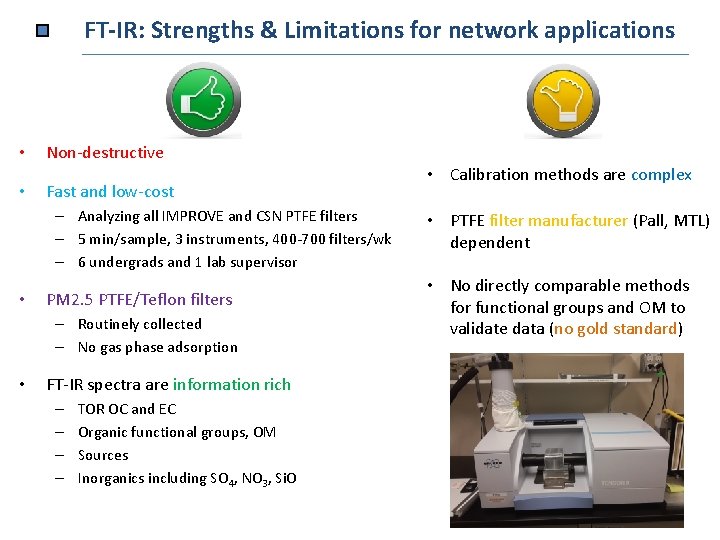
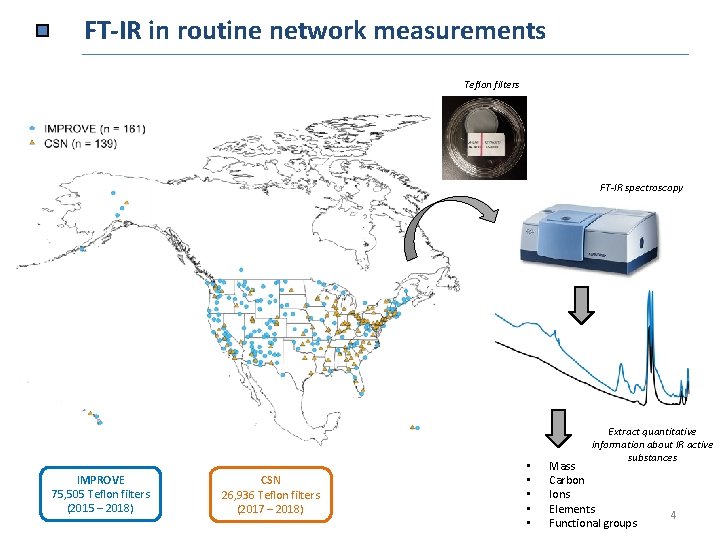
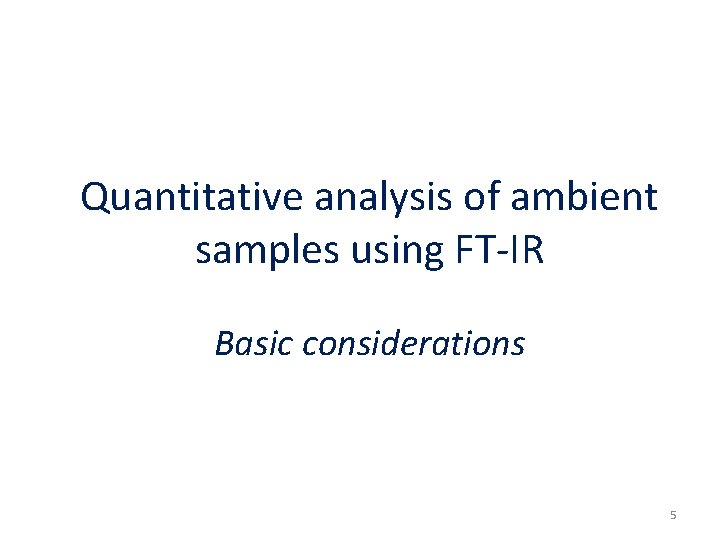
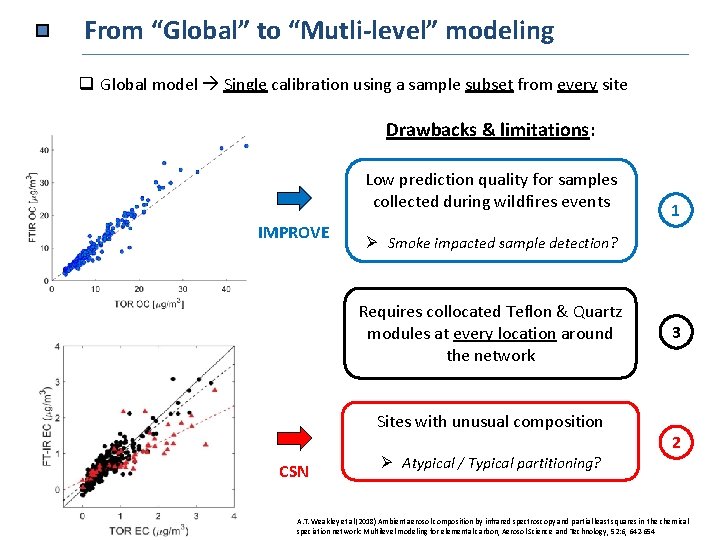
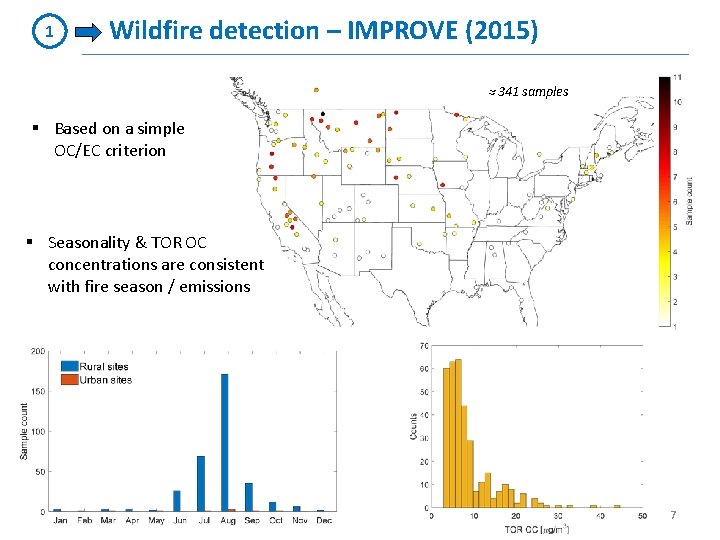
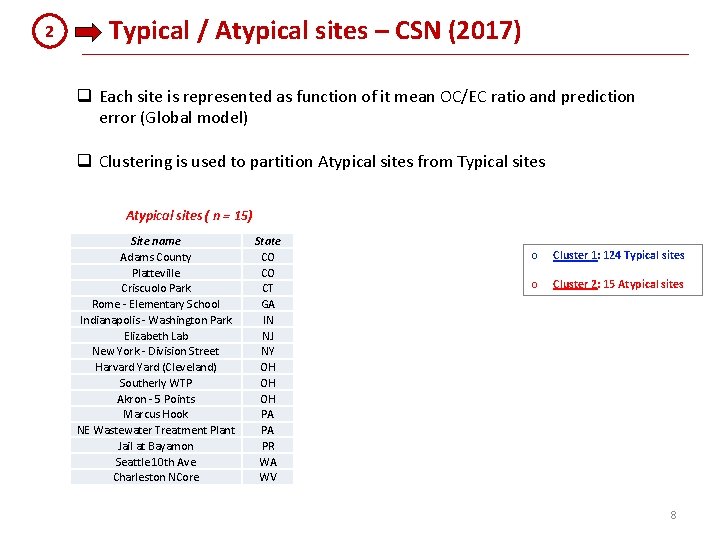
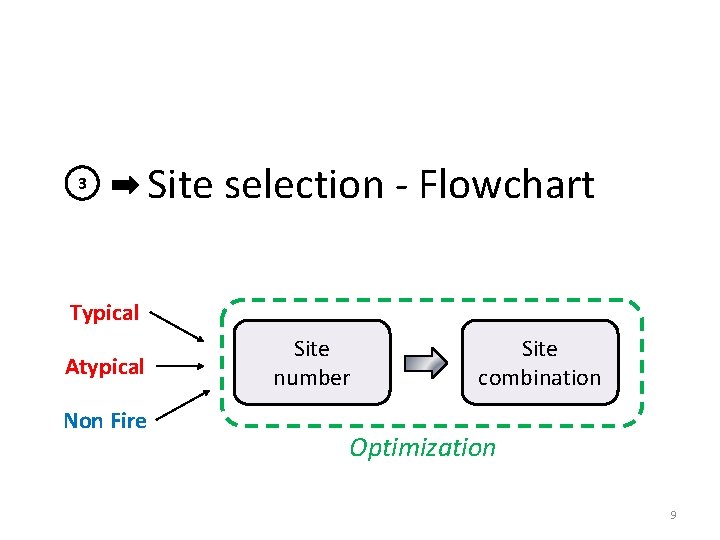
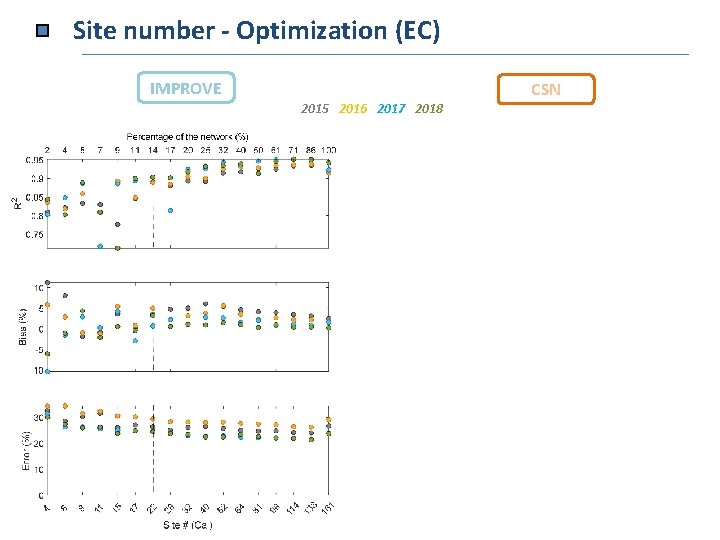
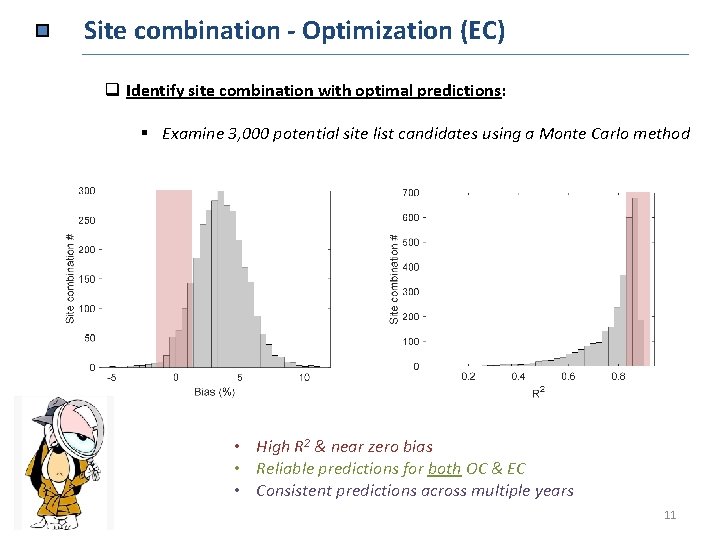
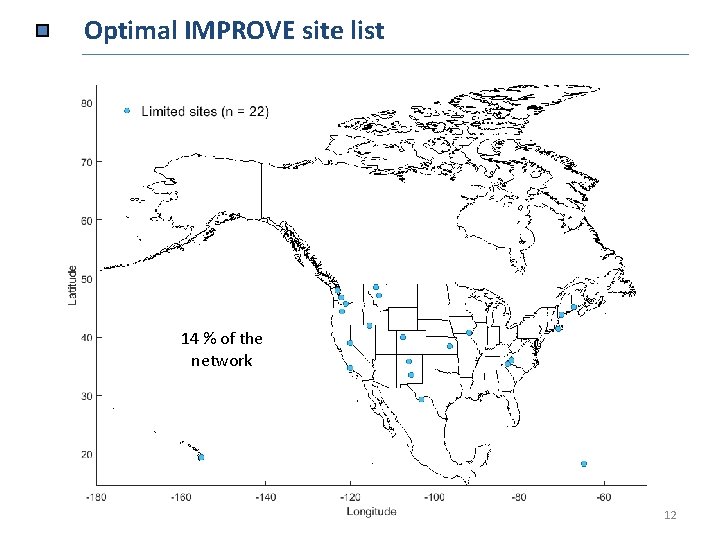
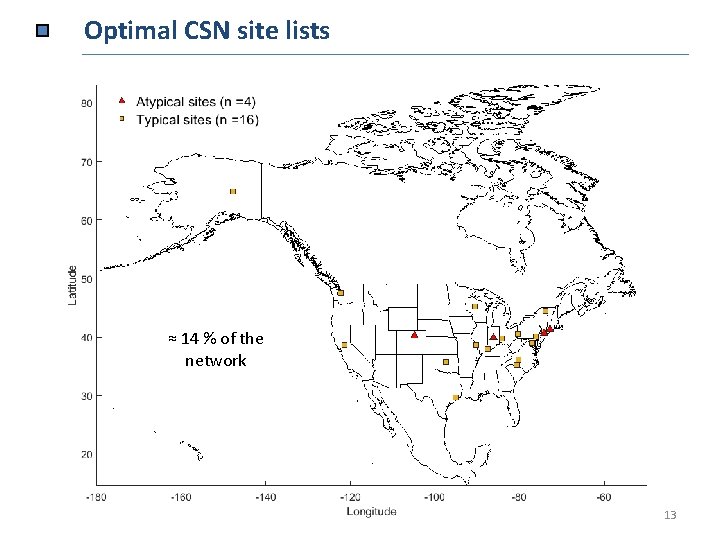
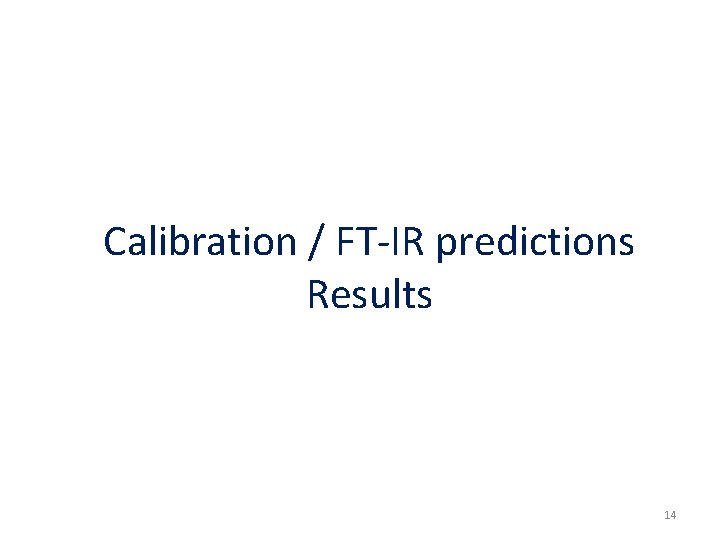
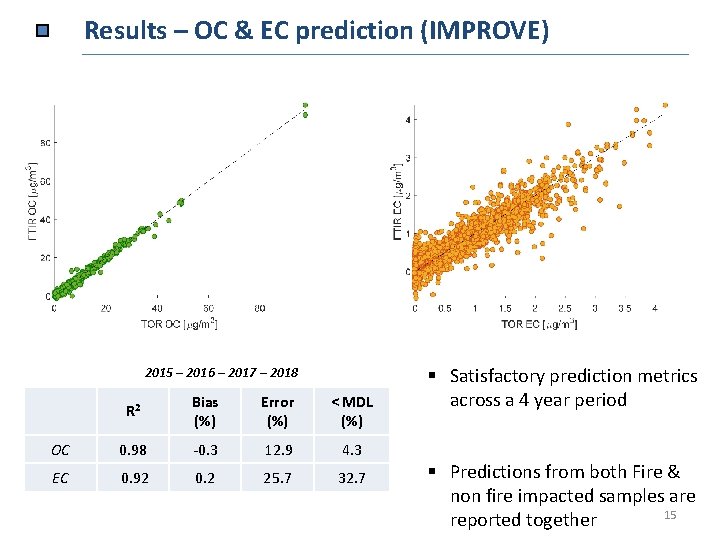
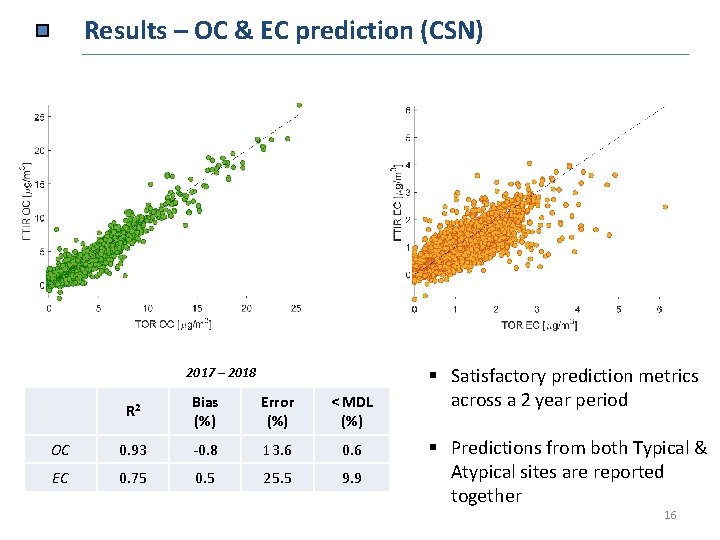
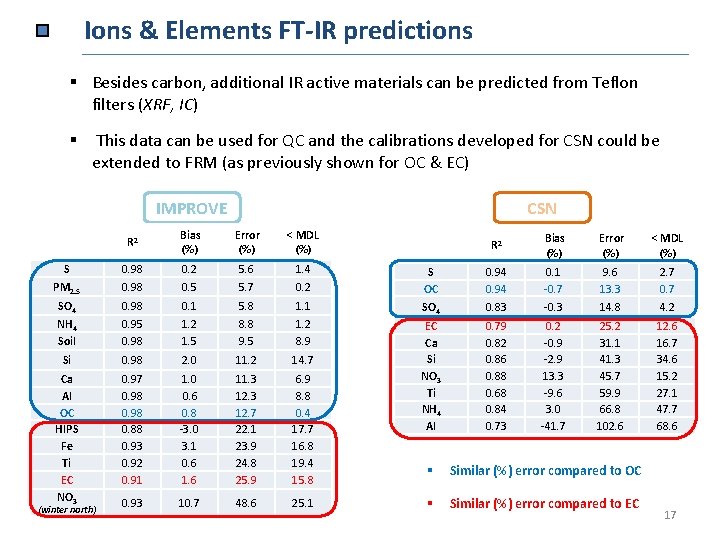
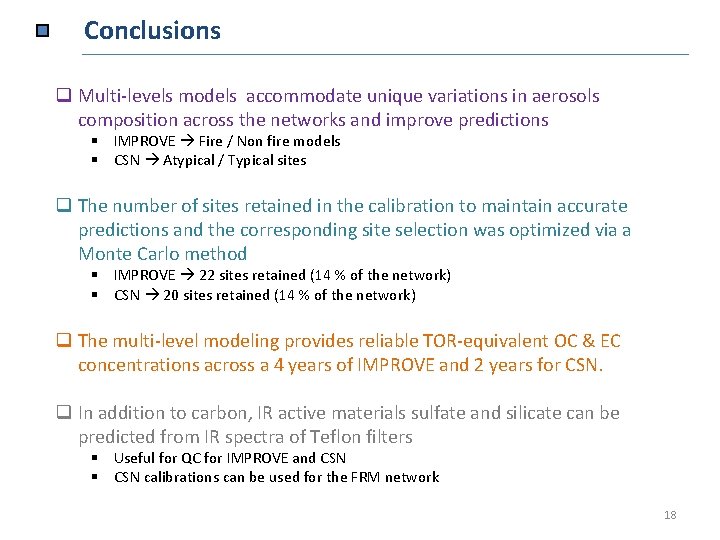
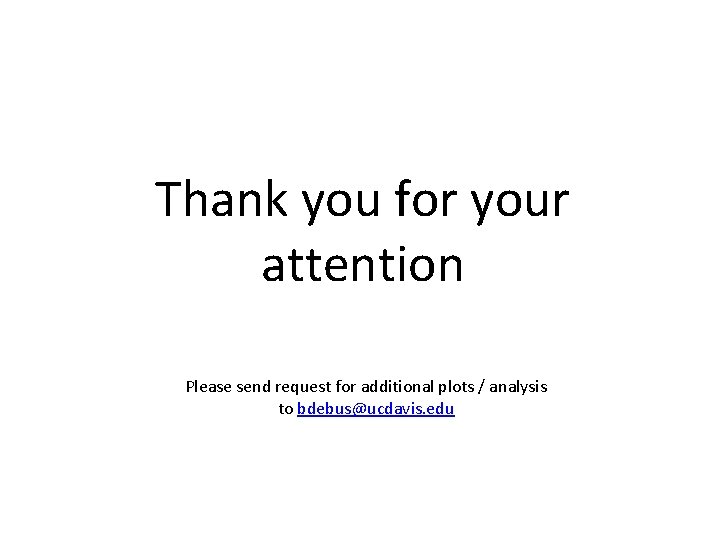
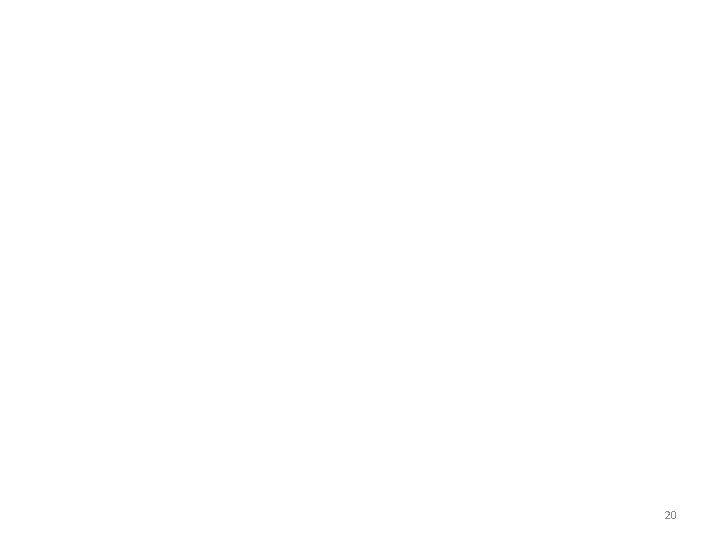
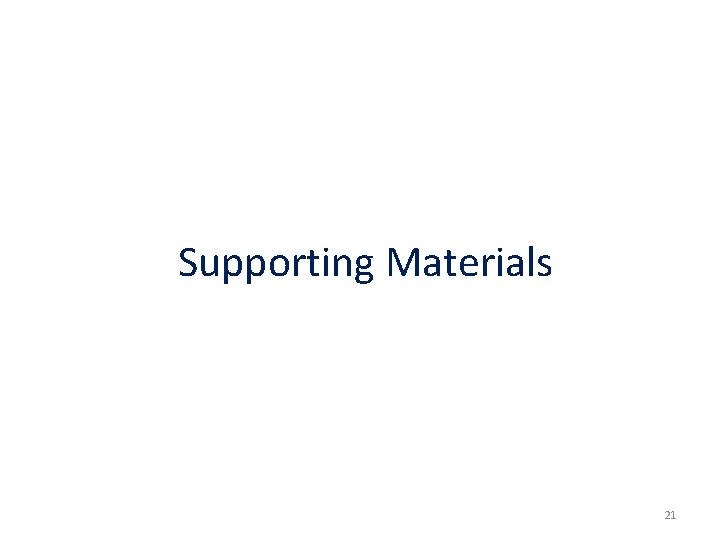
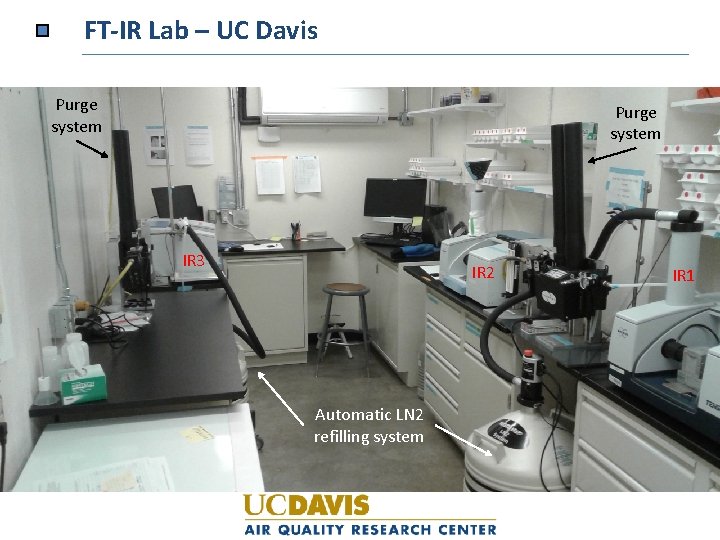
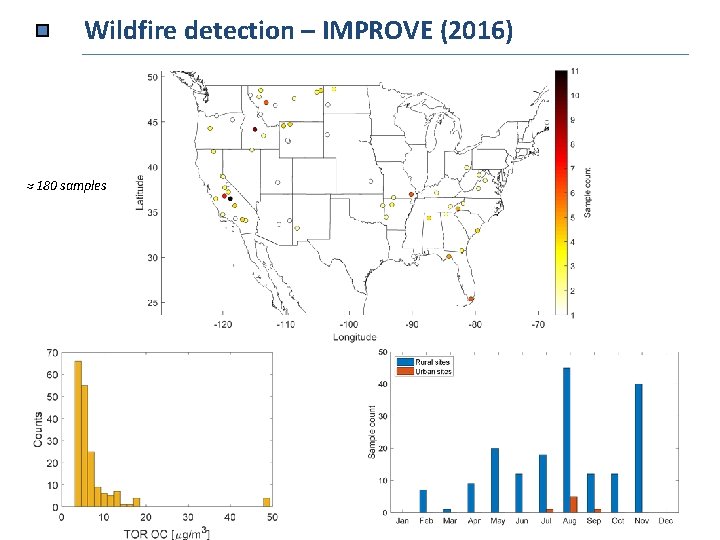
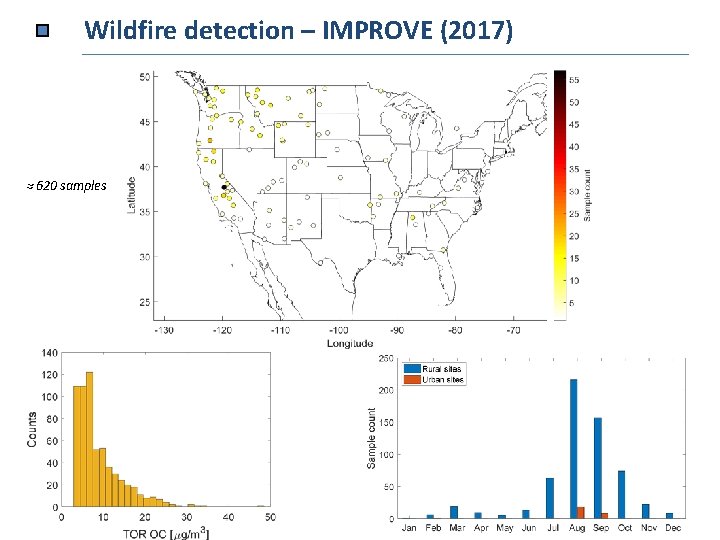
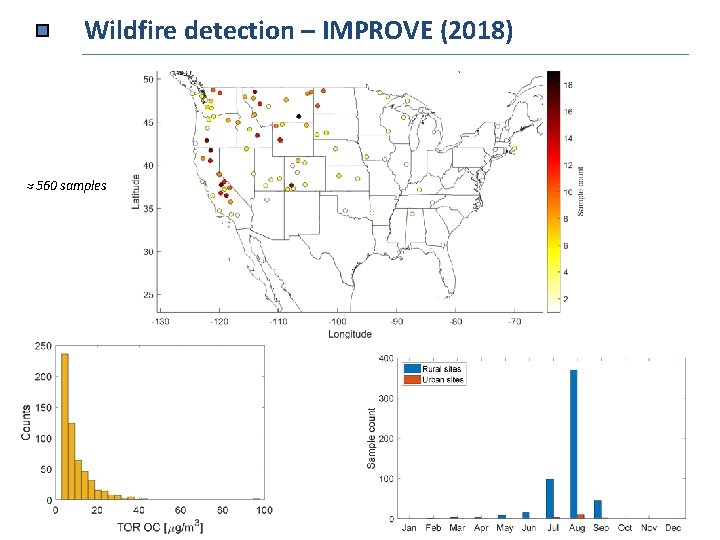
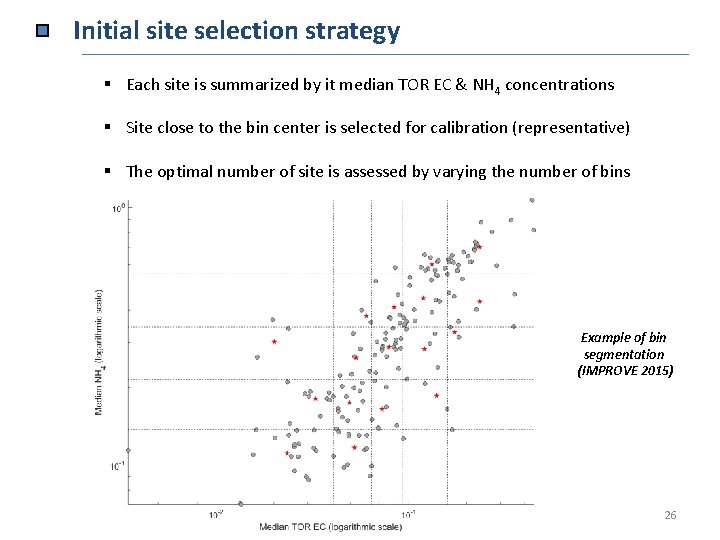
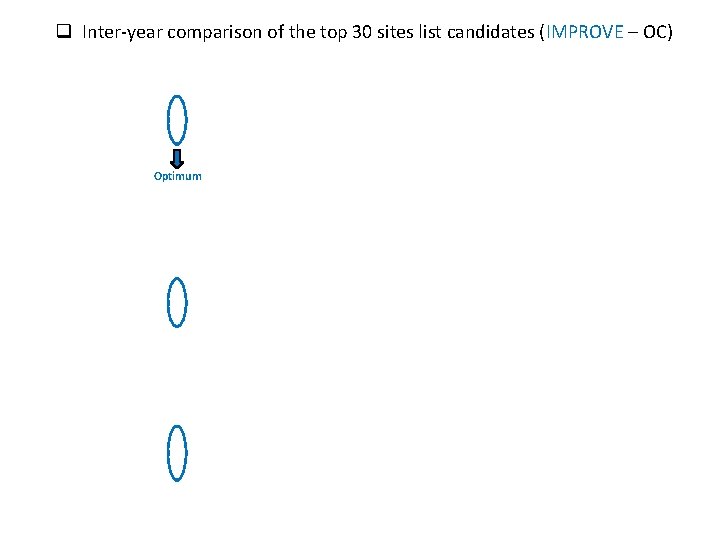
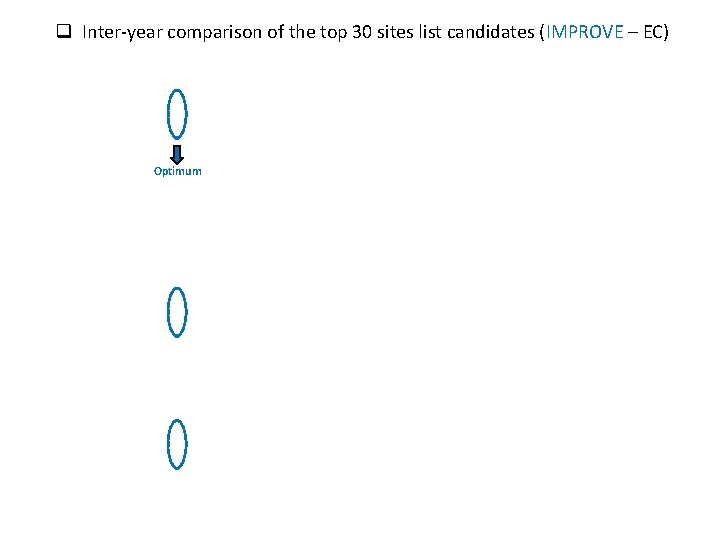
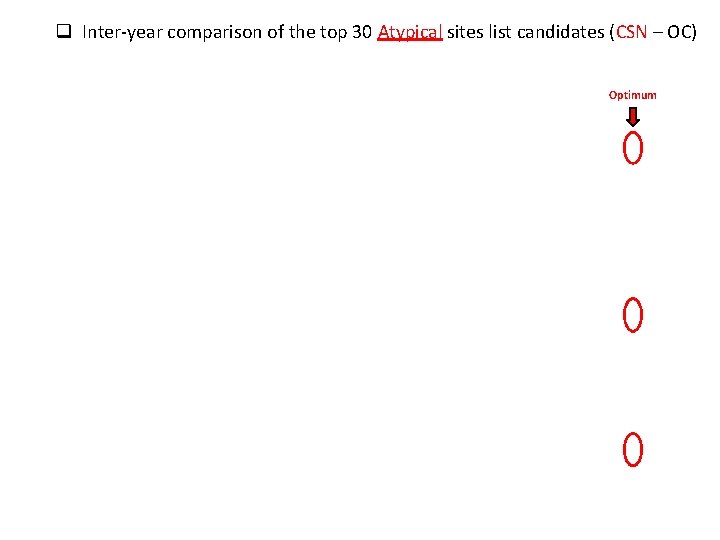
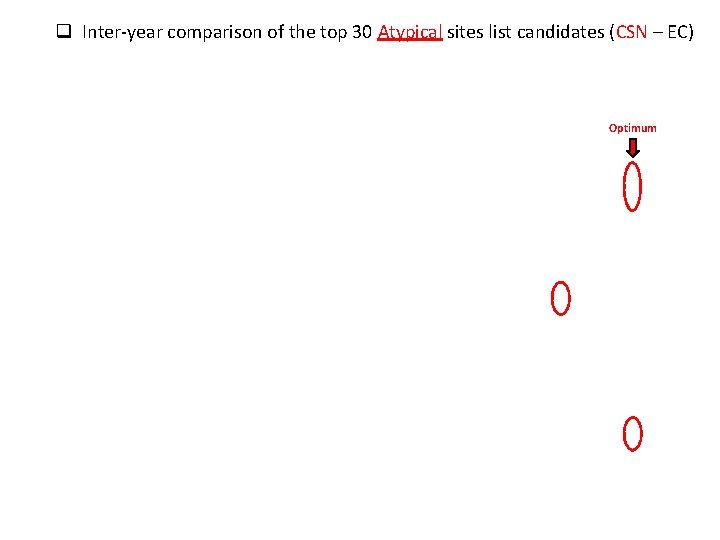
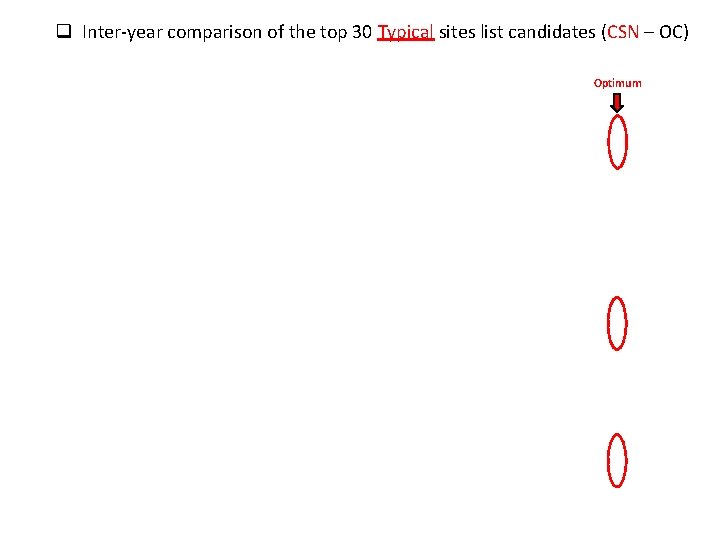
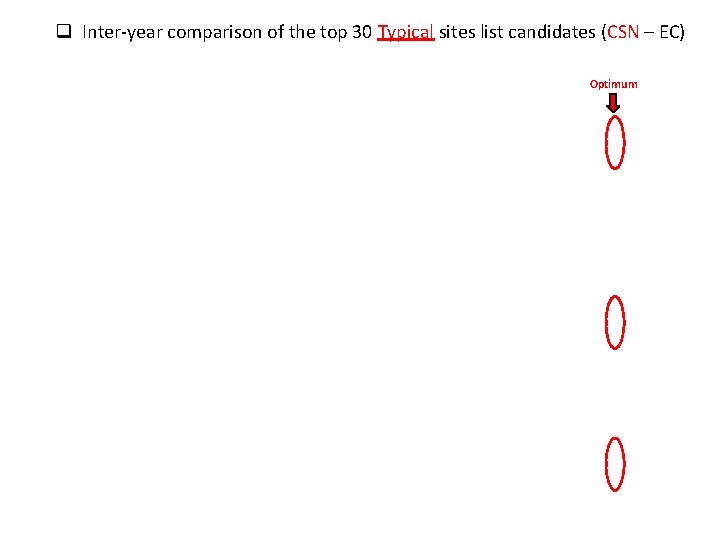
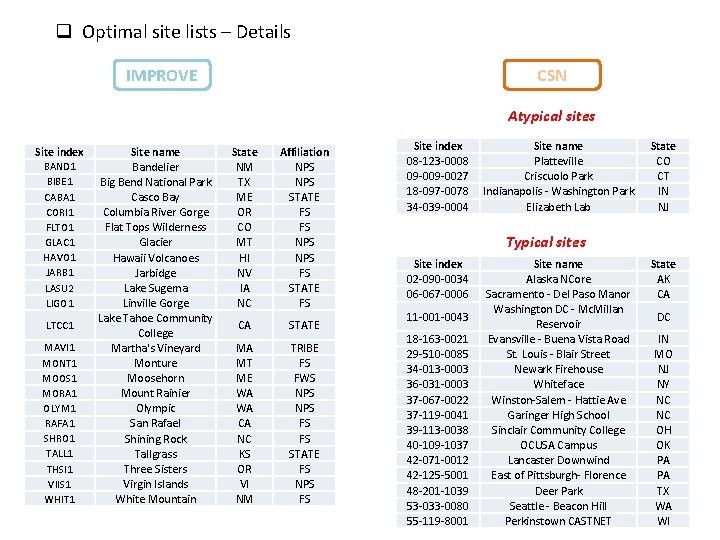
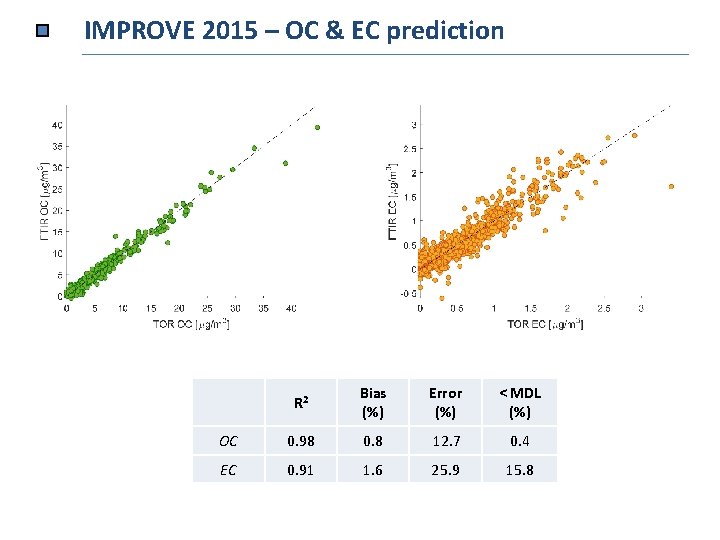
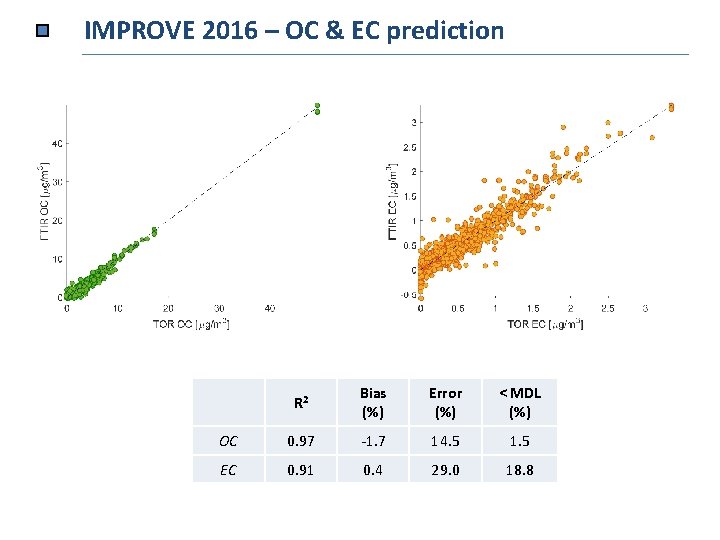
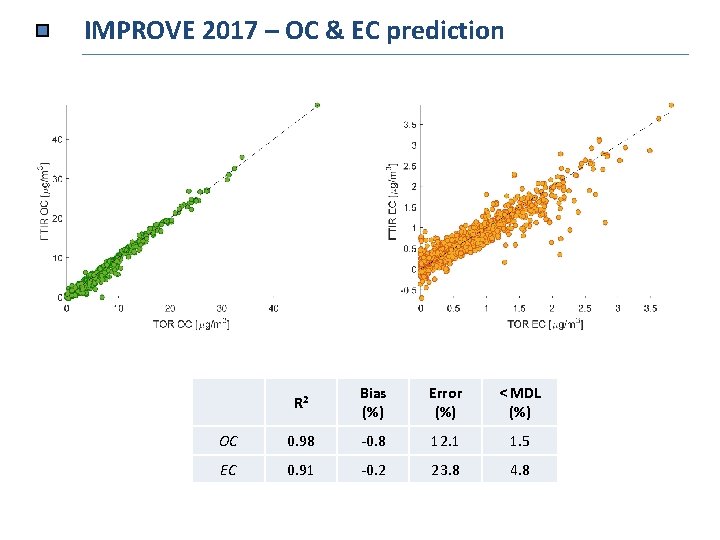
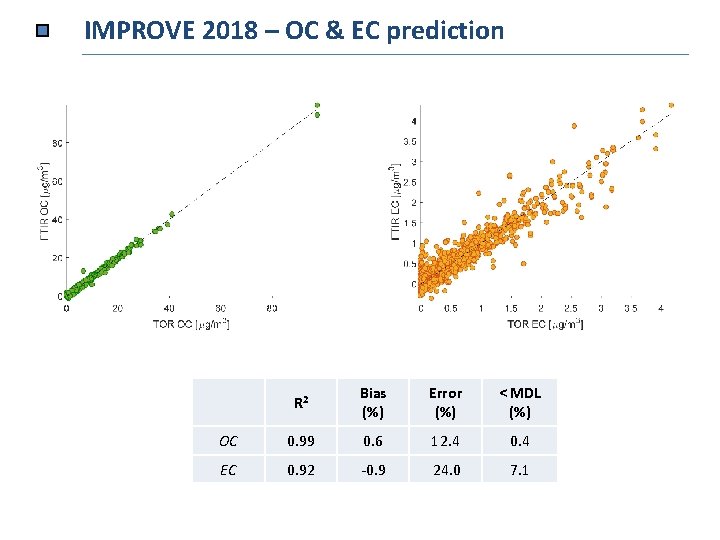
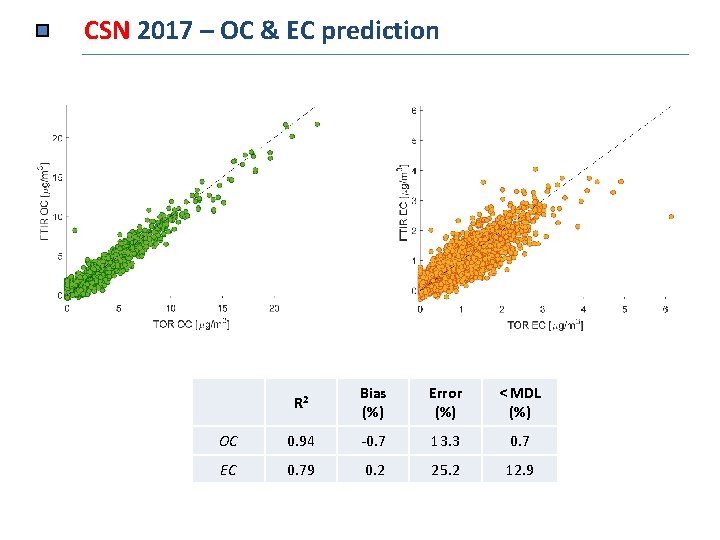
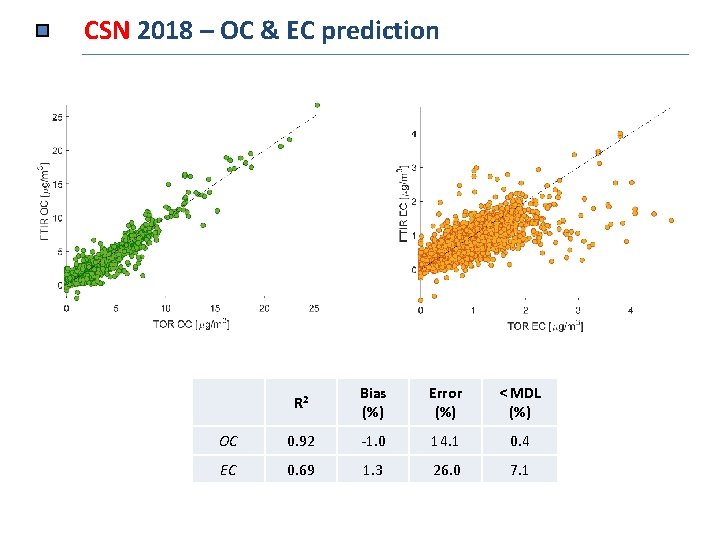
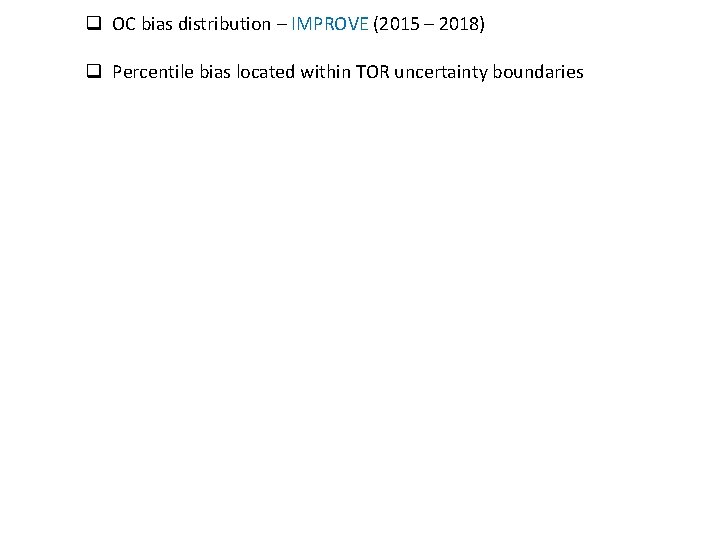
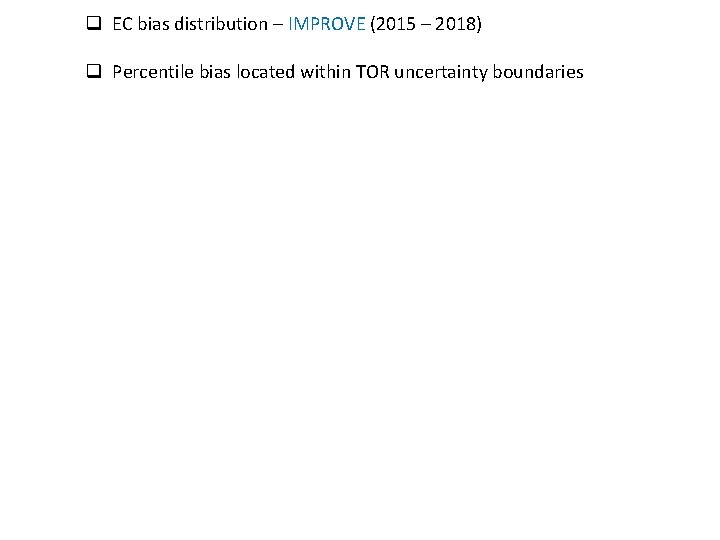
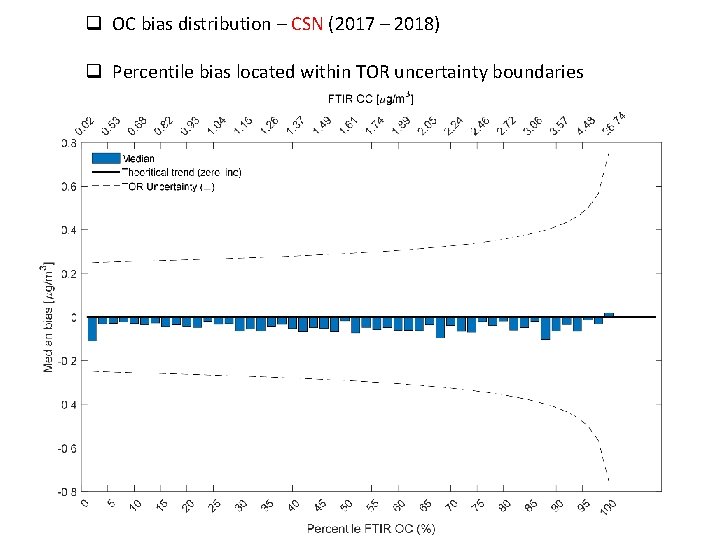
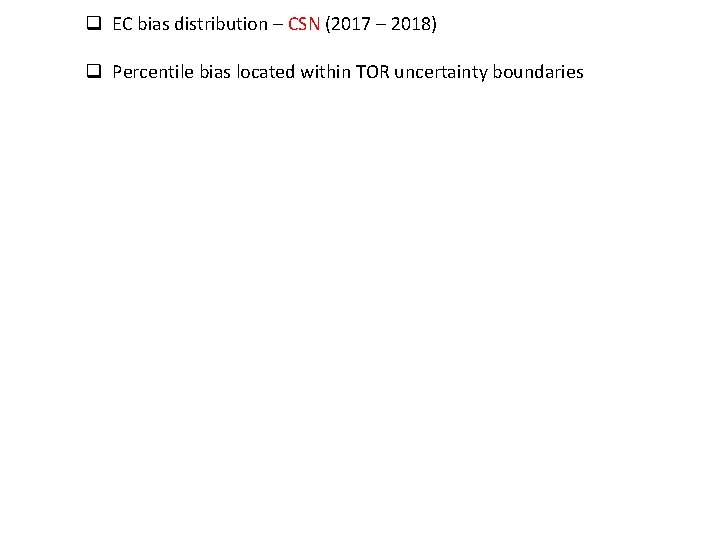
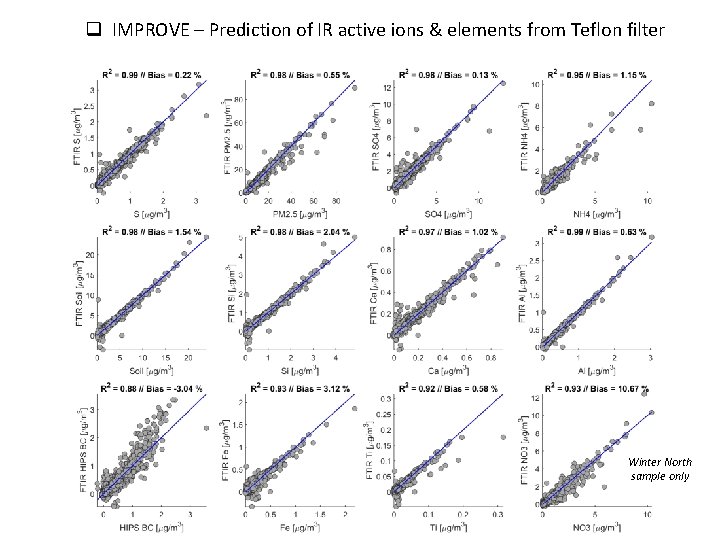
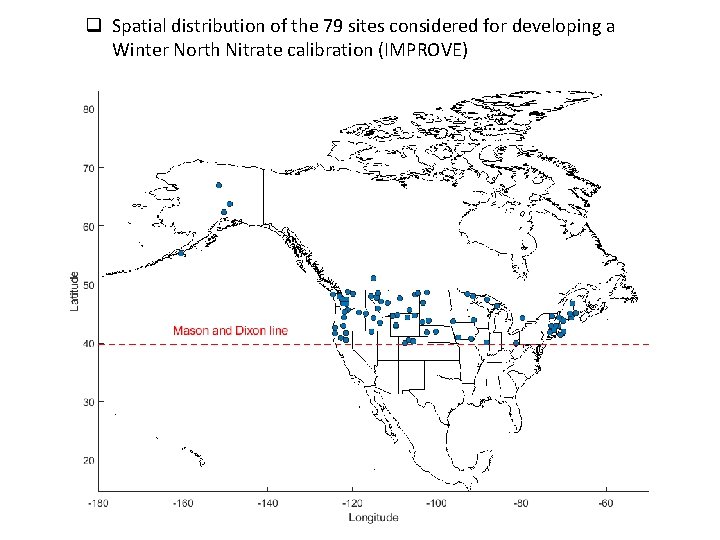
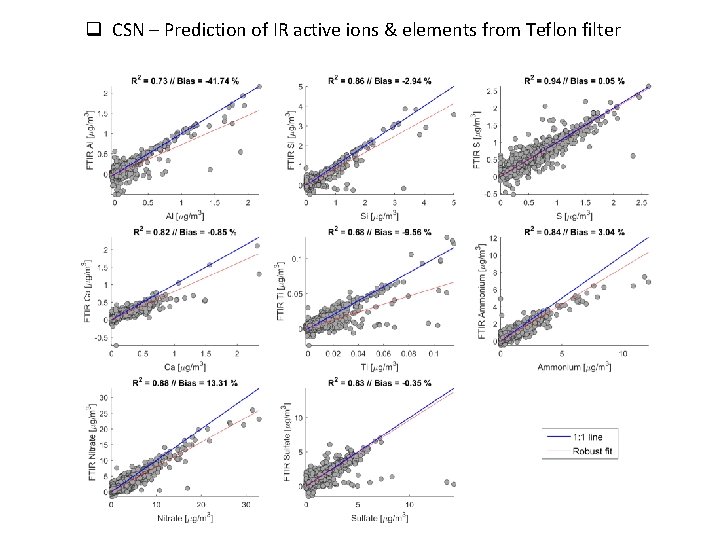
- Slides: 46
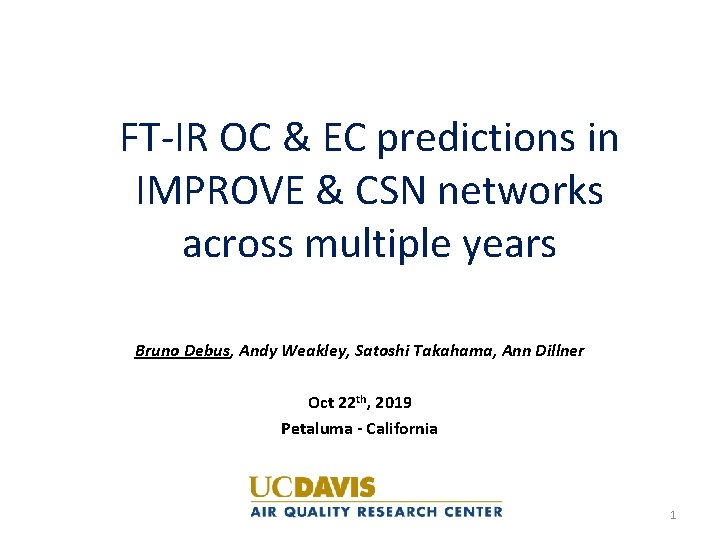
FT-IR OC & EC predictions in IMPROVE & CSN networks across multiple years Bruno Debus, Andy Weakley, Satoshi Takahama, Ann Dillner Oct 22 th, 2019 Petaluma - California 1
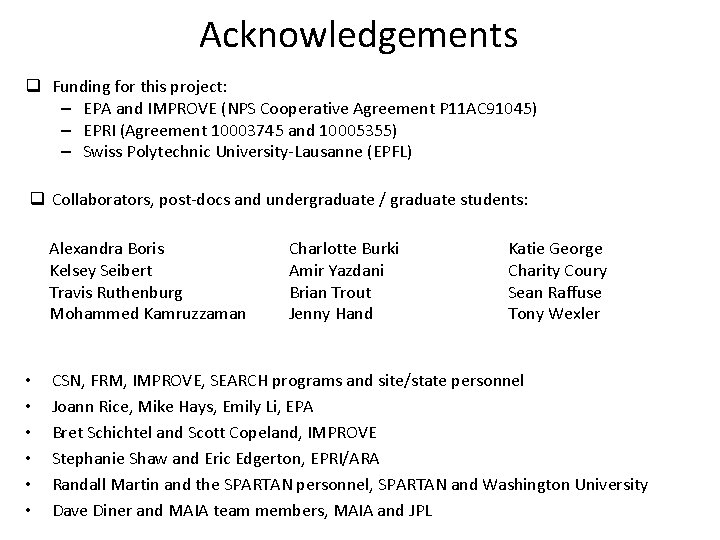
Acknowledgements q Funding for this project: – EPA and IMPROVE (NPS Cooperative Agreement P 11 AC 91045) – EPRI (Agreement 10003745 and 10005355) – Swiss Polytechnic University-Lausanne (EPFL) q Collaborators, post-docs and undergraduate / graduate students: Alexandra Boris Kelsey Seibert Travis Ruthenburg Mohammed Kamruzzaman • • • 2 Charlotte Burki Amir Yazdani Brian Trout Jenny Hand Katie George Charity Coury Sean Raffuse Tony Wexler CSN, FRM, IMPROVE, SEARCH programs and site/state personnel Joann Rice, Mike Hays, Emily Li, EPA Bret Schichtel and Scott Copeland, IMPROVE Stephanie Shaw and Eric Edgerton, EPRI/ARA Randall Martin and the SPARTAN personnel, SPARTAN and Washington University Dave Diner and MAIA team members, MAIA and JPL
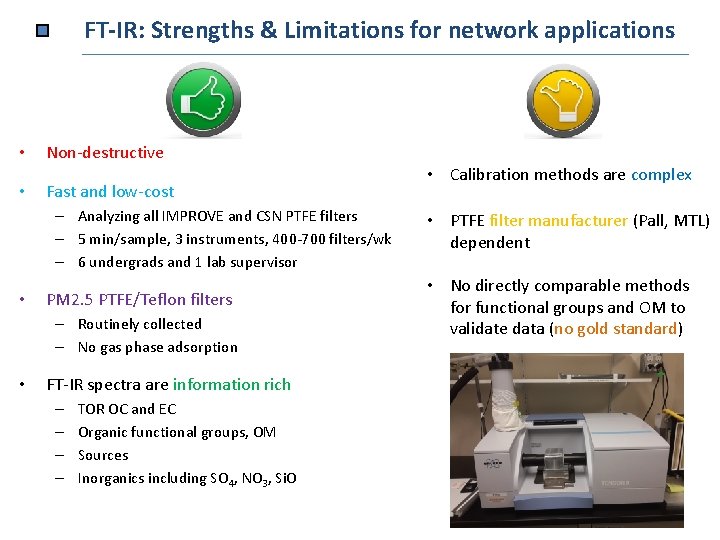
FT-IR: Strengths & Limitations for network applications • • Non-destructive Fast and low-cost – Analyzing all IMPROVE and CSN PTFE filters – 5 min/sample, 3 instruments, 400 -700 filters/wk – 6 undergrads and 1 lab supervisor • PM 2. 5 PTFE/Teflon filters – Routinely collected – No gas phase adsorption • • Calibration methods are complex • PTFE filter manufacturer (Pall, MTL) dependent • No directly comparable methods for functional groups and OM to validate data (no gold standard) FT-IR spectra are information rich – – TOR OC and EC Organic functional groups, OM Sources Inorganics including SO 4, NO 3, Si. O 3
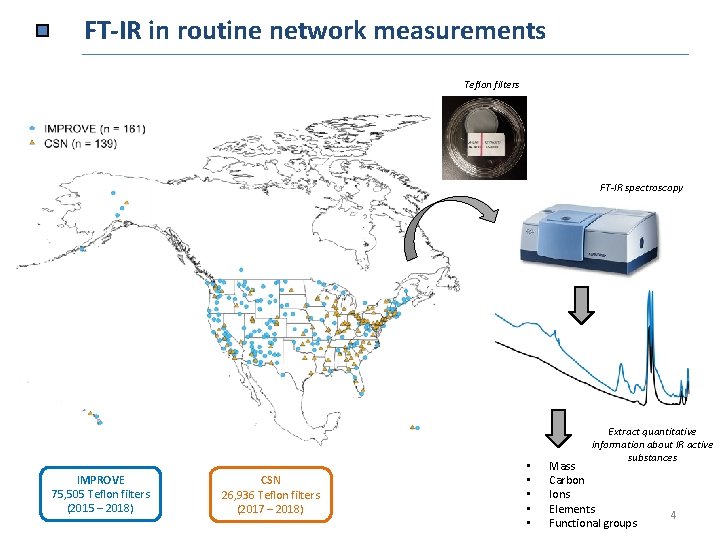
FT-IR in routine network measurements Teflon filters FT-IR spectroscopy IMPROVE 75, 505 Teflon filters (2015 – 2018) CSN 26, 936 Teflon filters (2017 – 2018) • • • Extract quantitative information about IR active substances Mass Carbon Ions Elements Functional groups 4
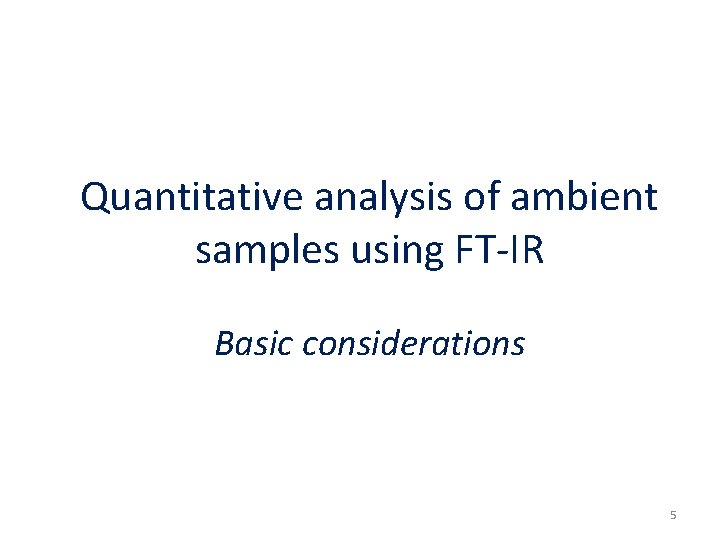
Quantitative analysis of ambient samples using FT-IR Basic considerations 5
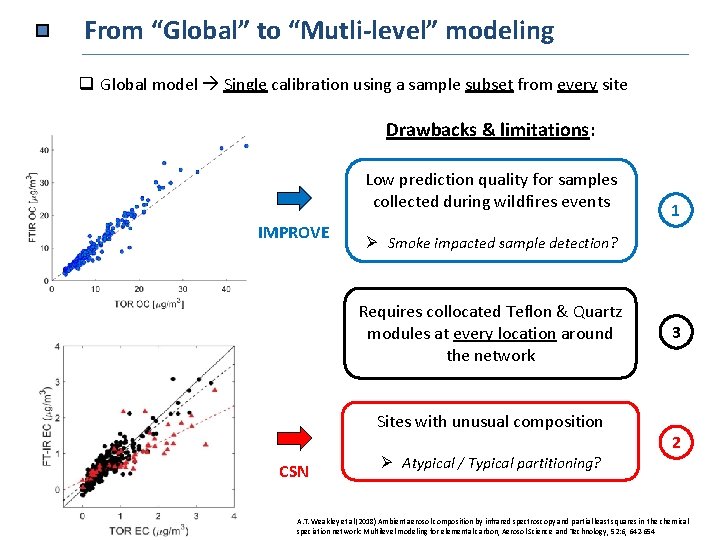
From “Global” to “Mutli-level” modeling q Global model Single calibration using a sample subset from every site Drawbacks & limitations: Low prediction quality for samples collected during wildfires events IMPROVE Ø Smoke impacted sample detection? Requires collocated Teflon & Quartz modules at every location around the network Sites with unusual composition CSN 1 Ø Atypical / Typical partitioning? 3 2 A. T. Weakley et al(2018) Ambient aerosol composition by infrared spectroscopy and partial least squares in the chemical speciation network: Multilevel modeling for elemental carbon, Aerosol Science and Technology, 52: 6, 642 -654
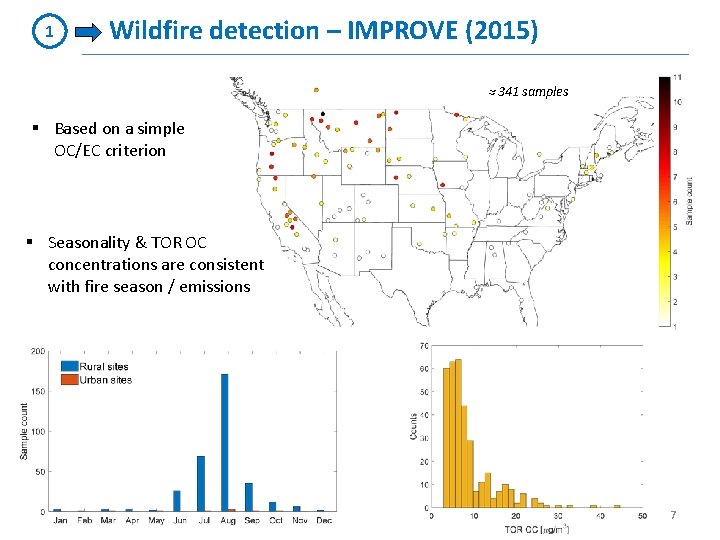
1 Wildfire detection – IMPROVE (2015) ≈ 341 samples § Based on a simple OC/EC criterion § Seasonality & TOR OC concentrations are consistent with fire season / emissions 7
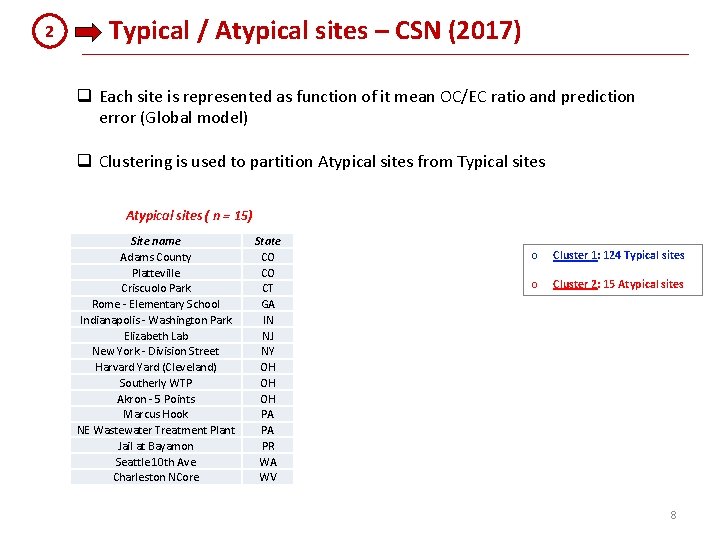
2 Typical / Atypical sites – CSN (2017) q Each site is represented as function of it mean OC/EC ratio and prediction error (Global model) q Clustering is used to partition Atypical sites from Typical sites Atypical sites ( n = 15) Site name Adams County Platteville Criscuolo Park Rome - Elementary School Indianapolis - Washington Park Elizabeth Lab New York - Division Street Harvard Yard (Cleveland) Southerly WTP Akron - 5 Points Marcus Hook NE Wastewater Treatment Plant Jail at Bayamon Seattle 10 th Ave Charleston NCore State CO CO CT GA IN NJ NY OH OH OH PA PA PR WA WV o Cluster 1: 124 Typical sites o Cluster 2: 15 Atypical sites 8
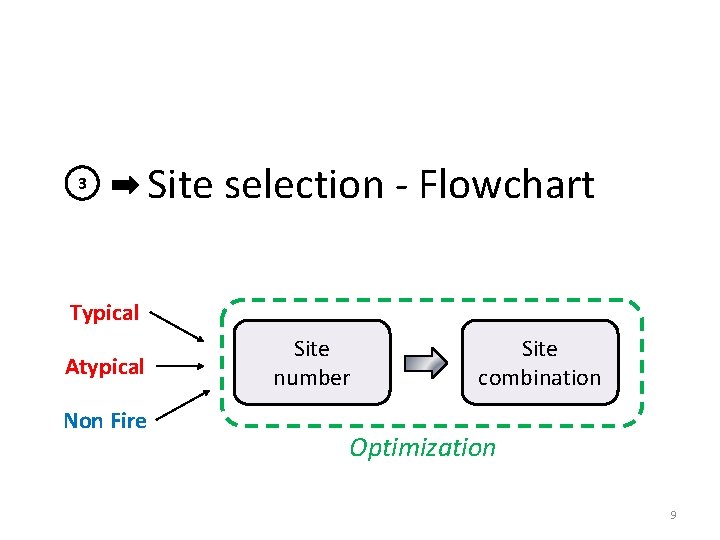
3 Site selection - Flowchart Typical Atypical Non Fire Site number Site combination Optimization 9
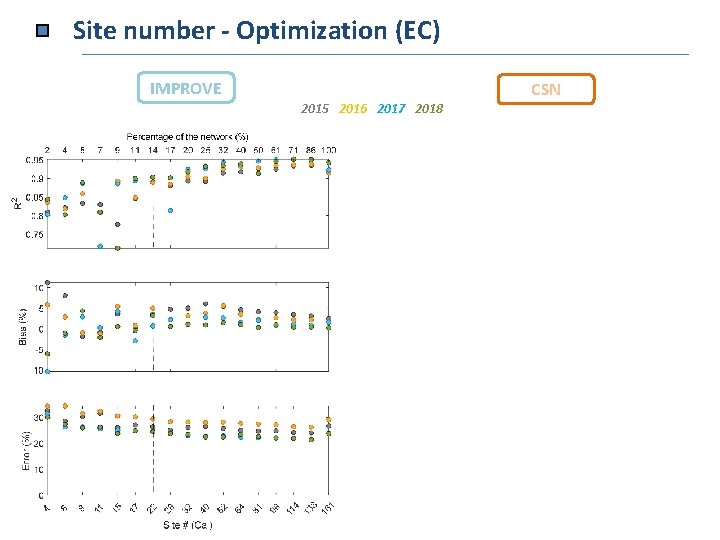
Site number - Optimization (EC) IMPROVE 2015 2016 2017 2018 CSN
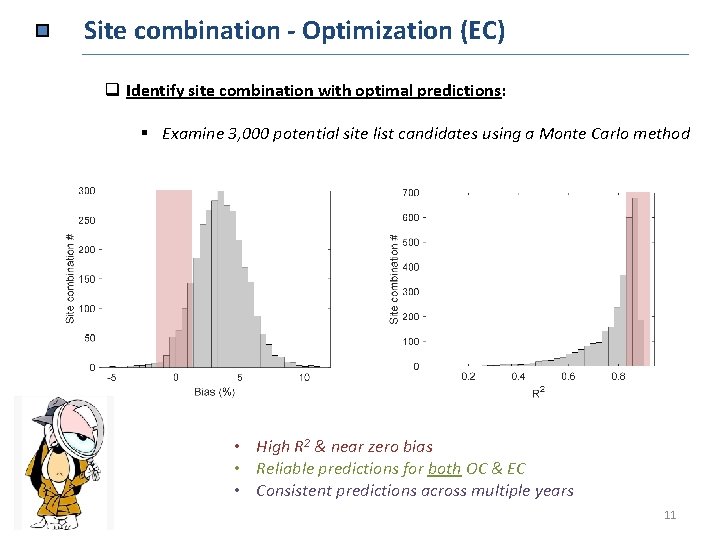
Site combination - Optimization (EC) q Identify site combination with optimal predictions: § Examine 3, 000 potential site list candidates using a Monte Carlo method • High R 2 & near zero bias • Reliable predictions for both OC & EC • Consistent predictions across multiple years 11
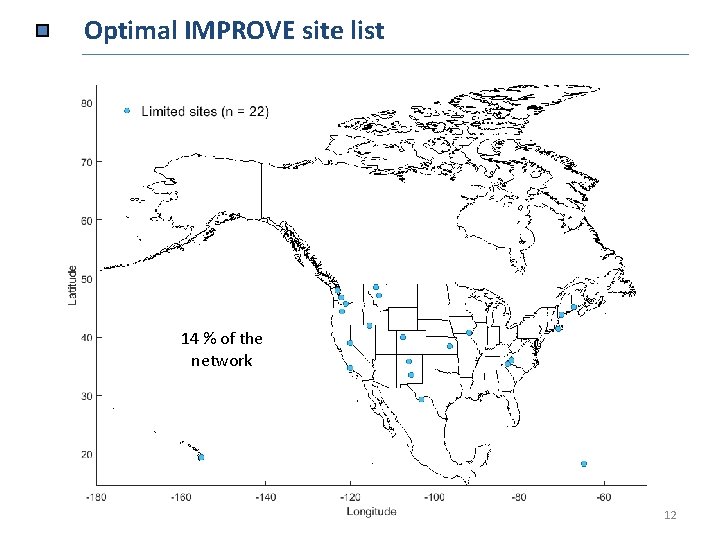
Optimal IMPROVE site list 14 % of the network 12
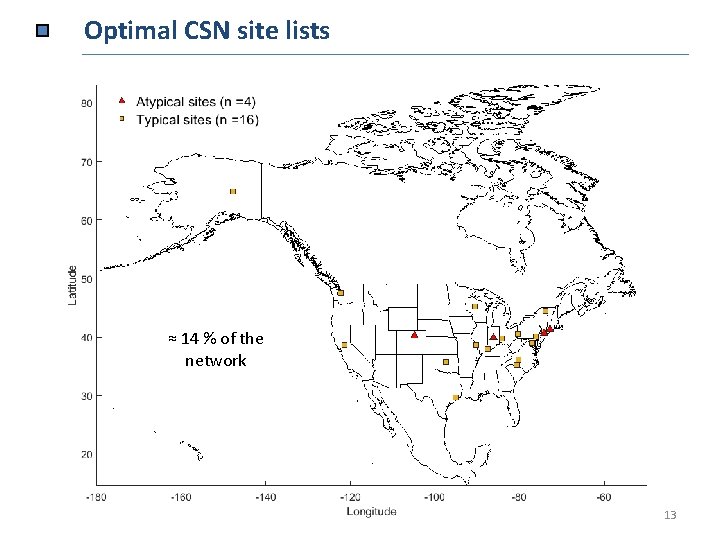
Optimal CSN site lists ≈ 14 % of the network 13
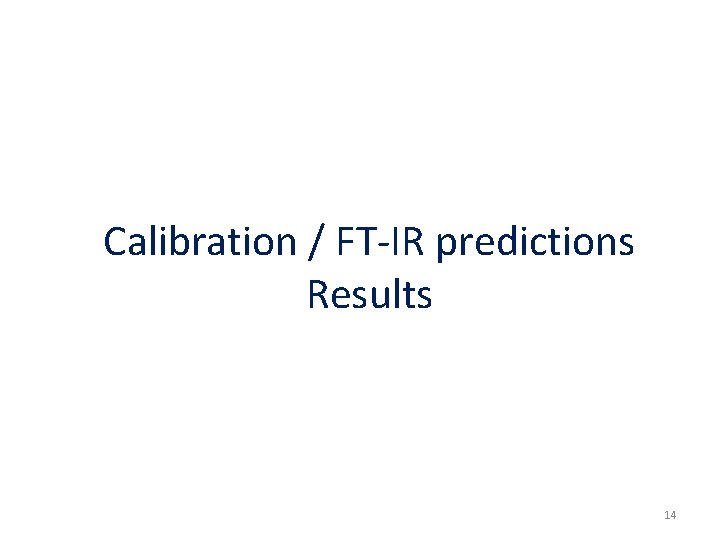
Calibration / FT-IR predictions Results 14
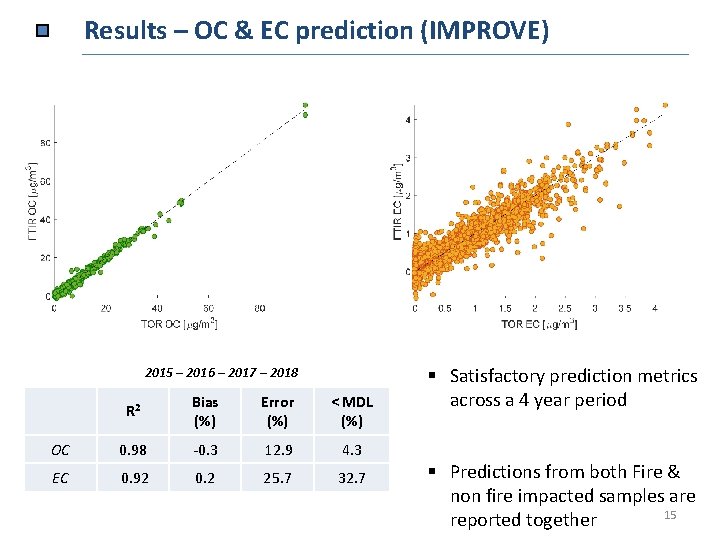
Results – OC & EC prediction (IMPROVE) 2015 – 2016 – 2017 – 2018 R 2 Bias (%) Error (%) < MDL (%) OC 0. 98 -0. 3 12. 9 4. 3 EC 0. 92 0. 2 25. 7 32. 7 § Satisfactory prediction metrics across a 4 year period § Predictions from both Fire & non fire impacted samples are 15 reported together
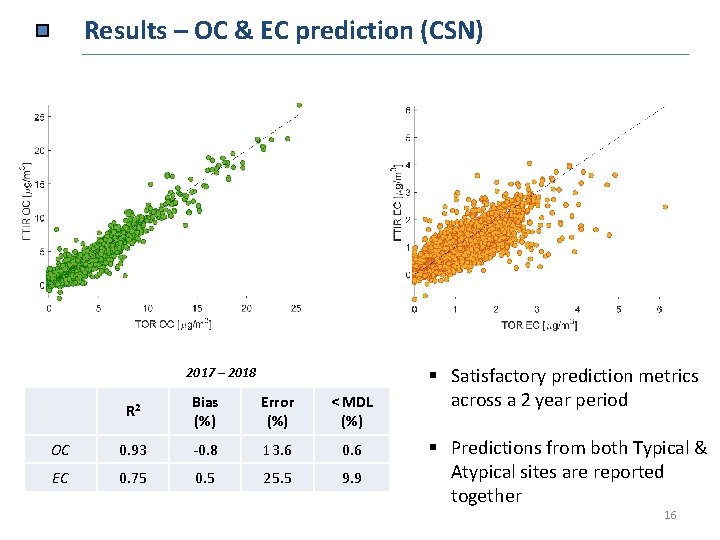
Results – OC & EC prediction (CSN) 2017 – 2018 R 2 Bias (%) Error (%) < MDL (%) OC 0. 93 -0. 8 13. 6 0. 6 EC 0. 75 0. 5 25. 5 9. 9 § Satisfactory prediction metrics across a 2 year period § Predictions from both Typical & Atypical sites are reported together 16
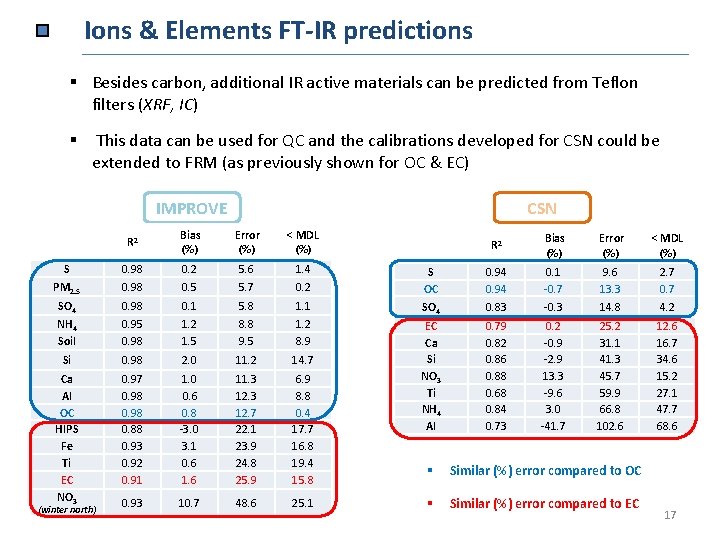
Ions & Elements FT-IR predictions § Besides carbon, additional IR active materials can be predicted from Teflon filters (XRF, IC) § This data can be used for QC and the calibrations developed for CSN could be extended to FRM (as previously shown for OC & EC) IMPROVE CSN R 2 Bias (%) Error (%) < MDL (%) S PM 2. 5 0. 98 0. 2 0. 5 5. 6 5. 7 1. 4 0. 2 SO 4 0. 98 0. 1 5. 8 NH 4 Soil 0. 95 0. 98 1. 2 1. 5 Si 0. 98 Ca Al OC HIPS Fe Ti EC NO 3 (winter north) R 2 Bias (%) Error (%) < MDL (%) S OC 0. 94 0. 1 -0. 7 9. 6 13. 3 2. 7 0. 7 1. 1 SO 4 0. 83 -0. 3 14. 8 4. 2 8. 8 9. 5 1. 2 8. 9 2. 0 11. 2 14. 7 0. 98 0. 88 0. 93 0. 92 0. 91 1. 0 0. 6 0. 8 -3. 0 3. 1 0. 6 11. 3 12. 7 22. 1 23. 9 24. 8 25. 9 6. 9 8. 8 0. 4 17. 7 16. 8 19. 4 15. 8 EC Ca Si NO 3 Ti NH 4 Al 0. 79 0. 82 0. 86 0. 88 0. 68 0. 84 0. 73 0. 2 -0. 9 -2. 9 13. 3 -9. 6 3. 0 -41. 7 25. 2 31. 1 41. 3 45. 7 59. 9 66. 8 102. 6 16. 7 34. 6 15. 2 27. 1 47. 7 68. 6 0. 93 10. 7 48. 6 25. 1 § Similar (%) error compared to OC § Similar (%) error compared to EC 17
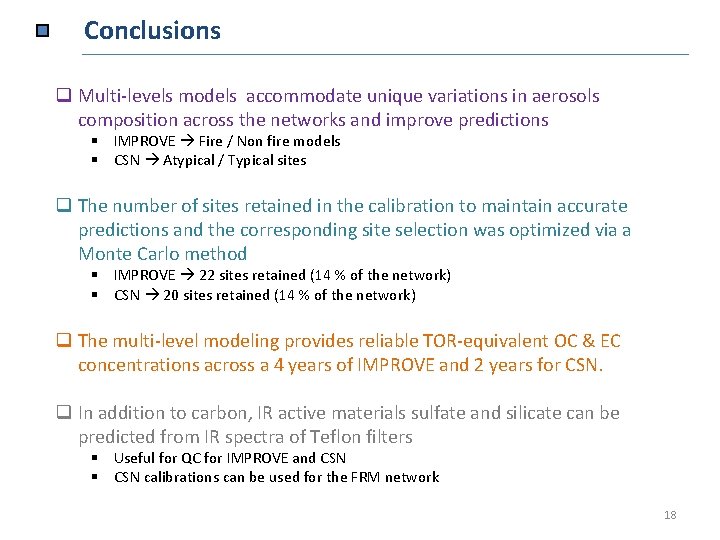
Conclusions q Multi-levels models accommodate unique variations in aerosols composition across the networks and improve predictions § IMPROVE Fire / Non fire models § CSN Atypical / Typical sites q The number of sites retained in the calibration to maintain accurate predictions and the corresponding site selection was optimized via a Monte Carlo method § IMPROVE 22 sites retained (14 % of the network) § CSN 20 sites retained (14 % of the network) q The multi-level modeling provides reliable TOR-equivalent OC & EC concentrations across a 4 years of IMPROVE and 2 years for CSN. q In addition to carbon, IR active materials sulfate and silicate can be predicted from IR spectra of Teflon filters § Useful for QC for IMPROVE and CSN § CSN calibrations can be used for the FRM network 18
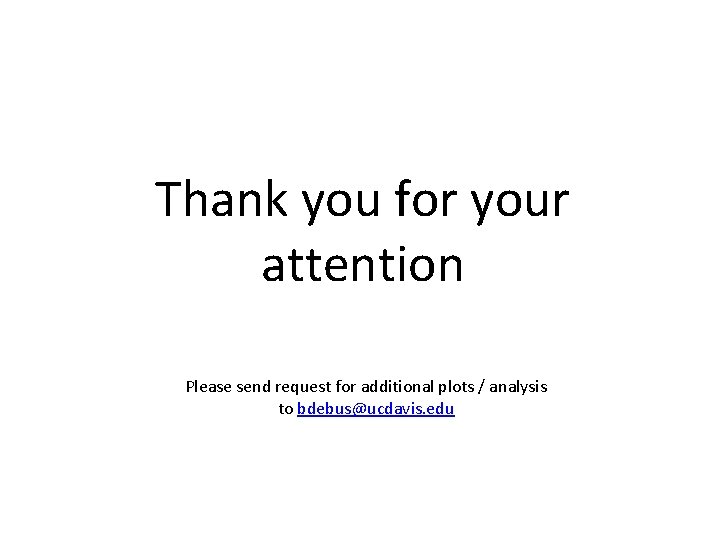
Thank you for your attention Please send request for additional plots / analysis to bdebus@ucdavis. edu
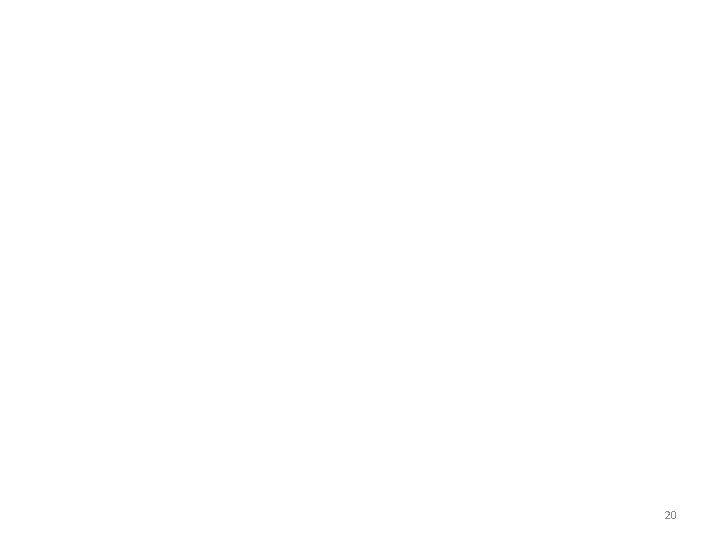
20
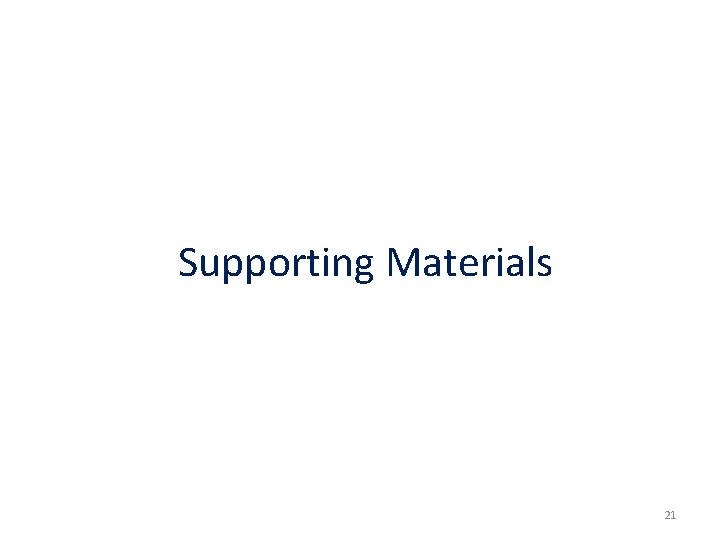
Supporting Materials 21
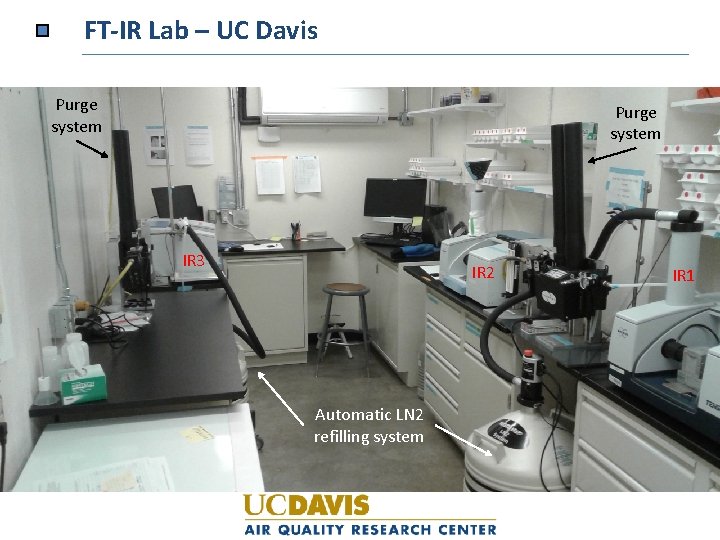
FT-IR Lab – UC Davis Purge system IR 3 IR 2 Automatic LN 2 refilling system IR 1
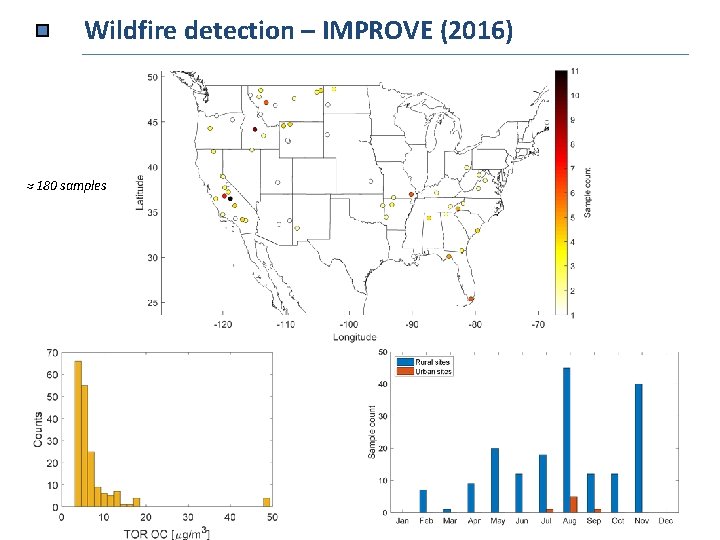
Wildfire detection – IMPROVE (2016) ≈ 180 samples
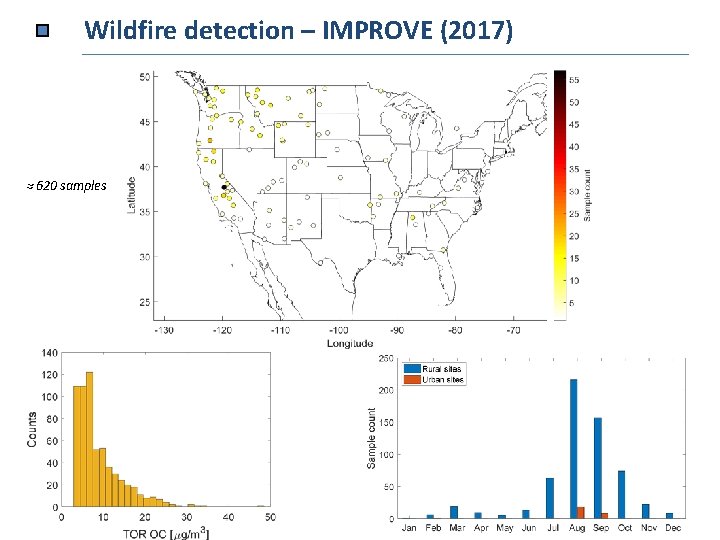
Wildfire detection – IMPROVE (2017) ≈ 620 samples
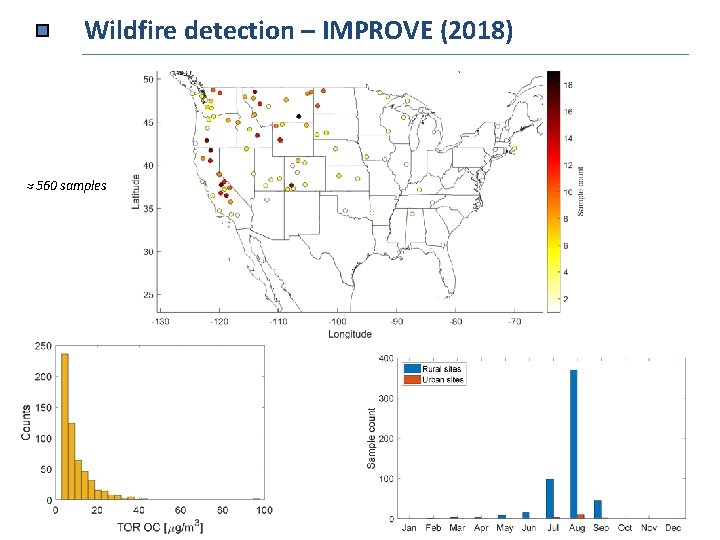
Wildfire detection – IMPROVE (2018) ≈ 560 samples
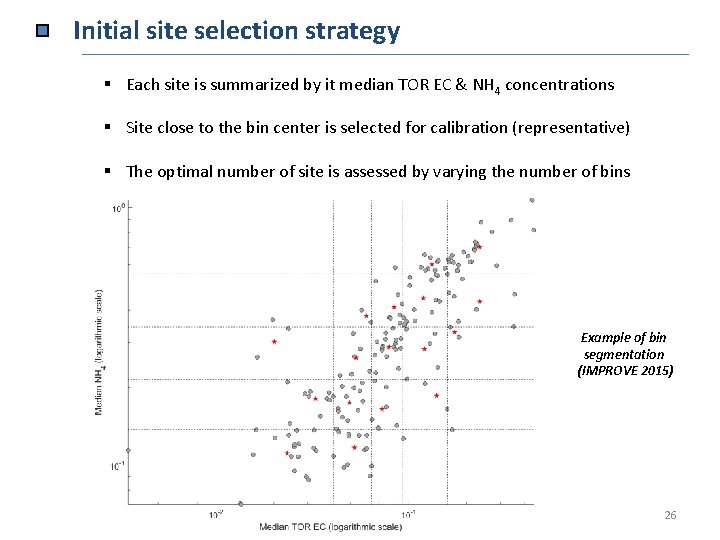
Initial site selection strategy § Each site is summarized by it median TOR EC & NH 4 concentrations § Site close to the bin center is selected for calibration (representative) § The optimal number of site is assessed by varying the number of bins Example of bin segmentation (IMPROVE 2015) 26
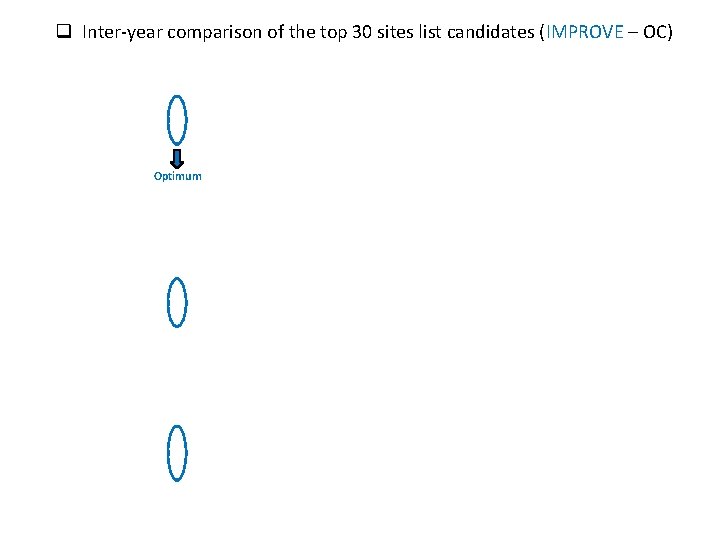
q Inter-year comparison of the top 30 sites list candidates (IMPROVE – OC) Optimum
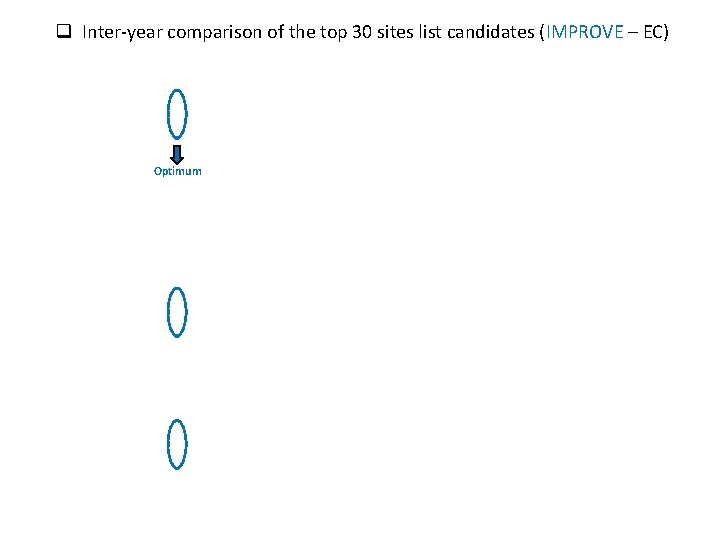
q Inter-year comparison of the top 30 sites list candidates (IMPROVE – EC) Optimum
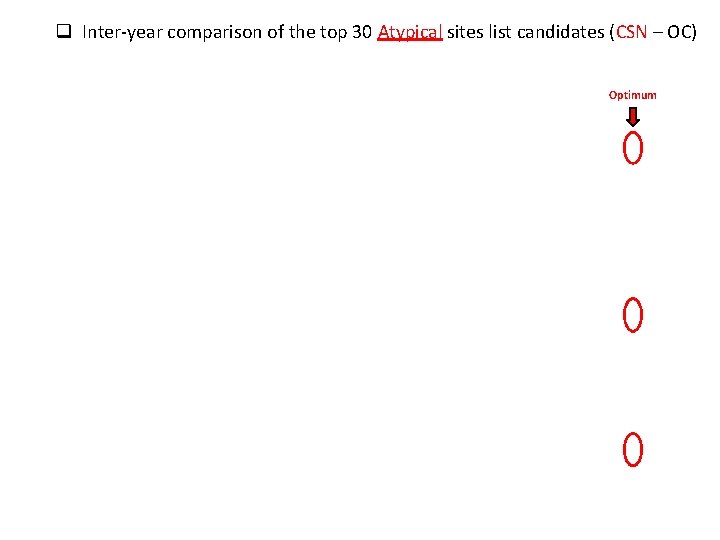
q Inter-year comparison of the top 30 Atypical sites list candidates (CSN – OC) Optimum
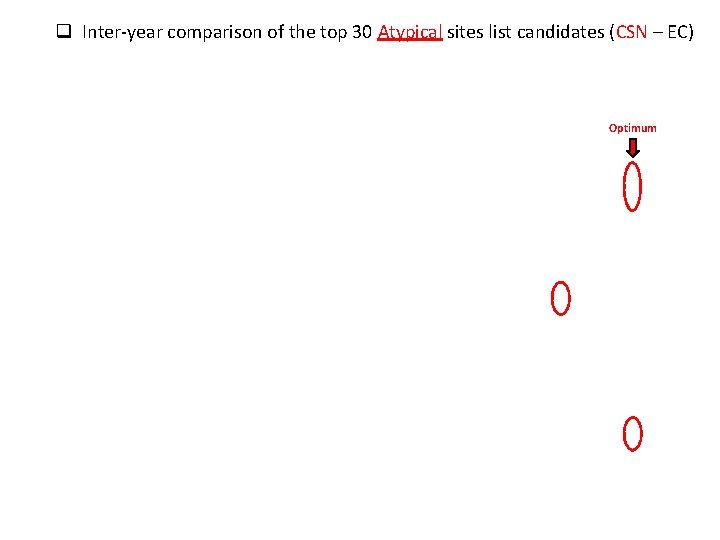
q Inter-year comparison of the top 30 Atypical sites list candidates (CSN – EC) Optimum
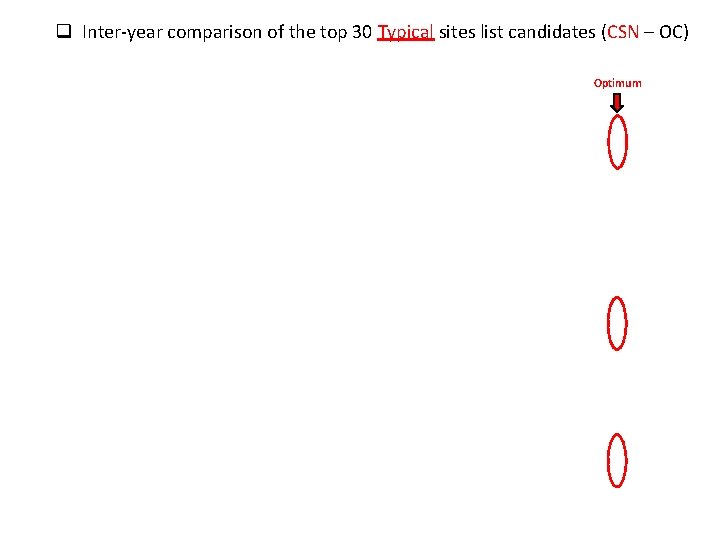
q Inter-year comparison of the top 30 Typical sites list candidates (CSN – OC) Optimum
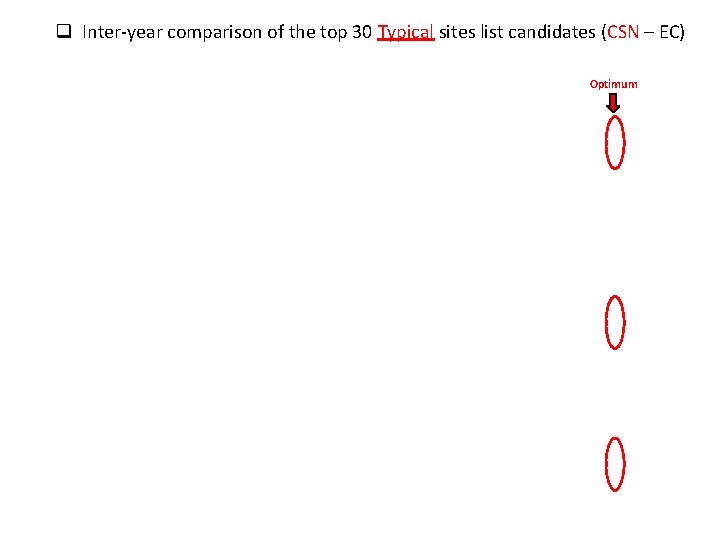
q Inter-year comparison of the top 30 Typical sites list candidates (CSN – EC) Optimum
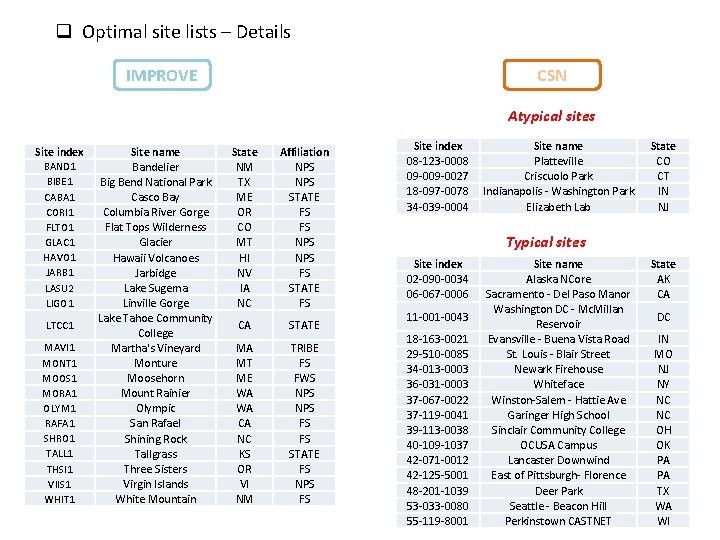
q Optimal site lists – Details IMPROVE CSN Atypical sites Site index BAND 1 BIBE 1 CABA 1 CORI 1 FLTO 1 GLAC 1 HAVO 1 JARB 1 LASU 2 LIGO 1 LTCC 1 MAVI 1 MONT 1 MOOS 1 MORA 1 OLYM 1 RAFA 1 SHRO 1 TALL 1 THSI 1 VIIS 1 WHIT 1 Site name Bandelier Big Bend National Park Casco Bay Columbia River Gorge Flat Tops Wilderness Glacier Hawaii Volcanoes Jarbidge Lake Sugema Linville Gorge Lake Tahoe Community College Martha's Vineyard Monture Moosehorn Mount Rainier Olympic San Rafael Shining Rock Tallgrass Three Sisters Virgin Islands White Mountain State NM TX ME OR CO MT HI NV IA NC Affiliation NPS STATE FS FS NPS FS STATE FS CA STATE MA MT ME WA WA CA NC KS OR VI NM TRIBE FS FWS NPS FS FS STATE FS NPS FS Site index 08 -123 -0008 09 -0027 18 -097 -0078 34 -039 -0004 Site name Platteville Criscuolo Park Indianapolis - Washington Park Elizabeth Lab State CO CT IN NJ Typical sites Site index 02 -090 -0034 06 -067 -0006 11 -0043 18 -163 -0021 29 -510 -0085 34 -013 -0003 36 -031 -0003 37 -067 -0022 37 -119 -0041 39 -113 -0038 40 -109 -1037 42 -071 -0012 42 -125 -5001 48 -201 -1039 53 -033 -0080 55 -119 -8001 Site name Alaska NCore Sacramento - Del Paso Manor Washington DC - Mc. Millan Reservoir Evansville - Buena Vista Road St. Louis - Blair Street Newark Firehouse Whiteface Winston-Salem - Hattie Ave Garinger High School Sinclair Community College OCUSA Campus Lancaster Downwind East of Pittsburgh- Florence Deer Park Seattle - Beacon Hill Perkinstown CASTNET State AK CA DC IN MO NJ NY NC NC OH OK PA PA TX WA WI
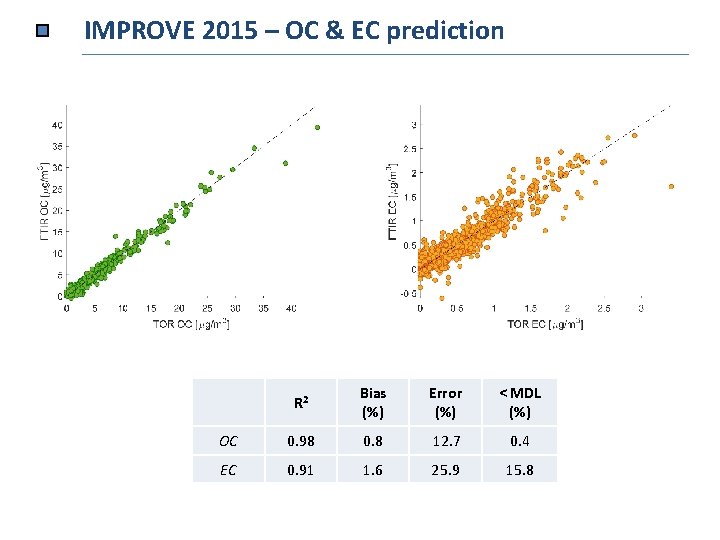
IMPROVE 2015 – OC & EC prediction R 2 Bias (%) Error (%) < MDL (%) OC 0. 98 0. 8 12. 7 0. 4 EC 0. 91 1. 6 25. 9 15. 8
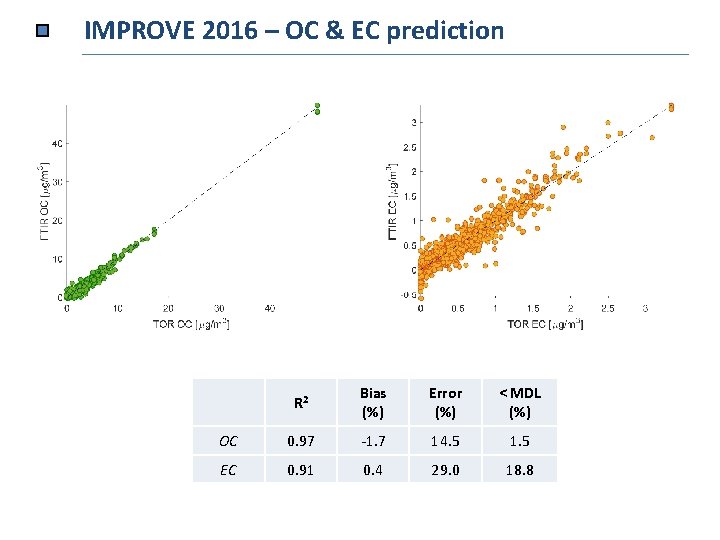
IMPROVE 2016 – OC & EC prediction R 2 Bias (%) Error (%) < MDL (%) OC 0. 97 -1. 7 14. 5 1. 5 EC 0. 91 0. 4 29. 0 18. 8
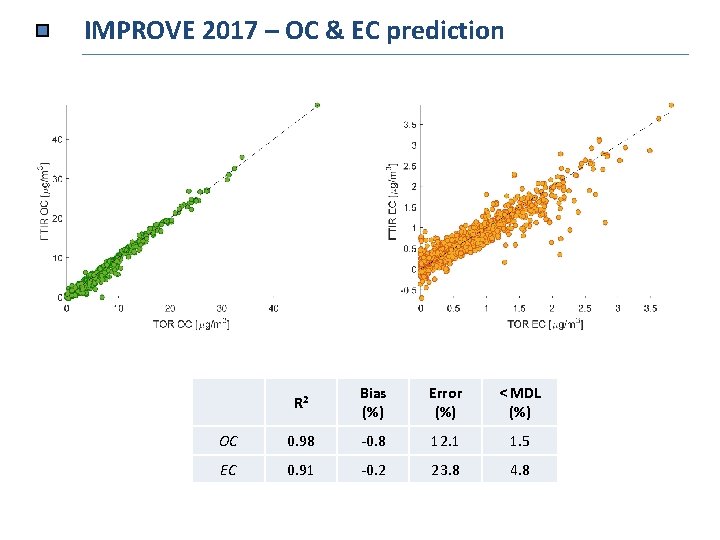
IMPROVE 2017 – OC & EC prediction R 2 Bias (%) Error (%) < MDL (%) OC 0. 98 -0. 8 12. 1 1. 5 EC 0. 91 -0. 2 23. 8 4. 8
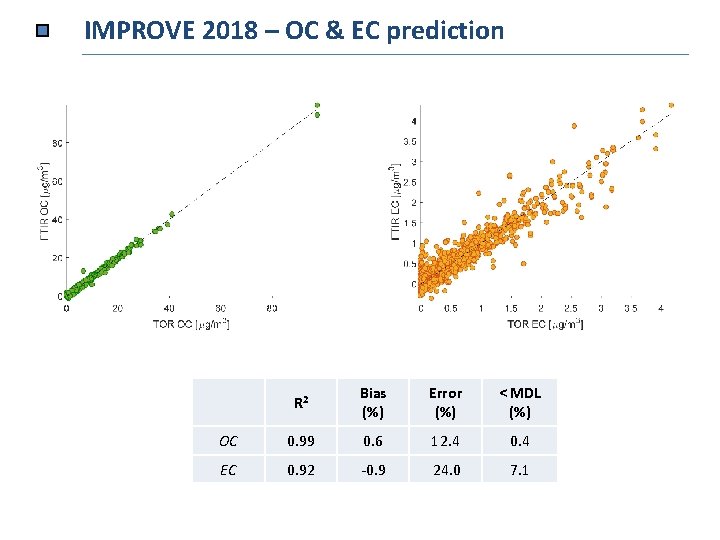
IMPROVE 2018 – OC & EC prediction R 2 Bias (%) Error (%) < MDL (%) OC 0. 99 0. 6 12. 4 0. 4 EC 0. 92 -0. 9 24. 0 7. 1
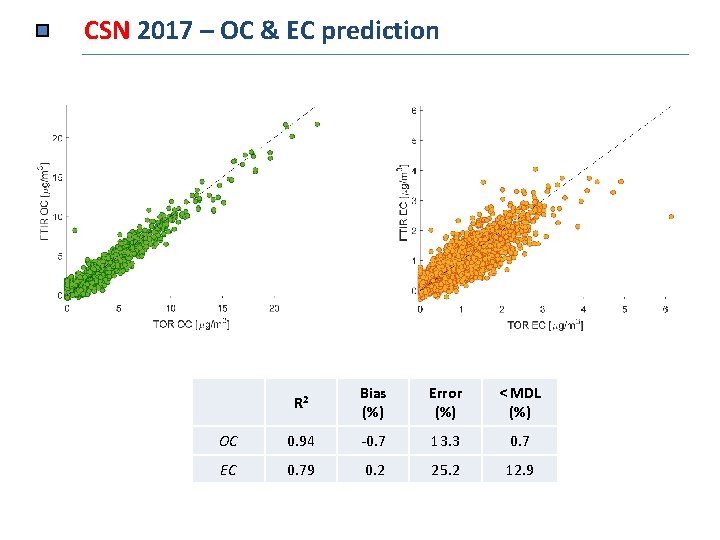
CSN 2017 – OC & EC prediction R 2 Bias (%) Error (%) < MDL (%) OC 0. 94 -0. 7 13. 3 0. 7 EC 0. 79 0. 2 25. 2 12. 9
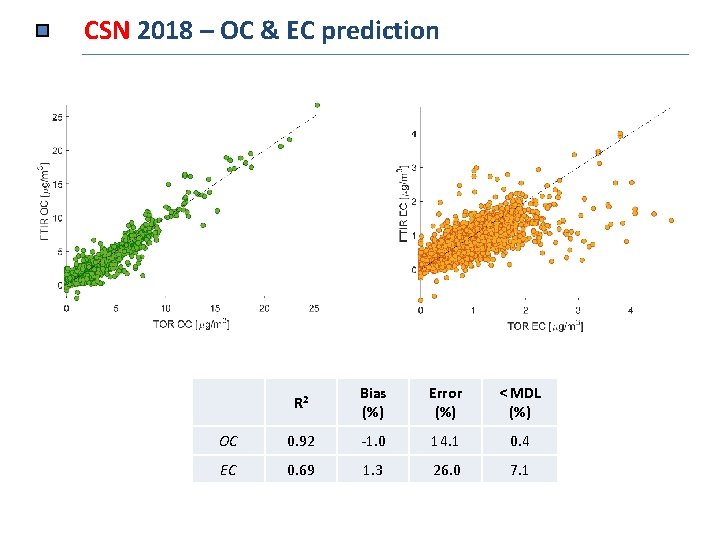
CSN 2018 – OC & EC prediction R 2 Bias (%) Error (%) < MDL (%) OC 0. 92 -1. 0 14. 1 0. 4 EC 0. 69 1. 3 26. 0 7. 1
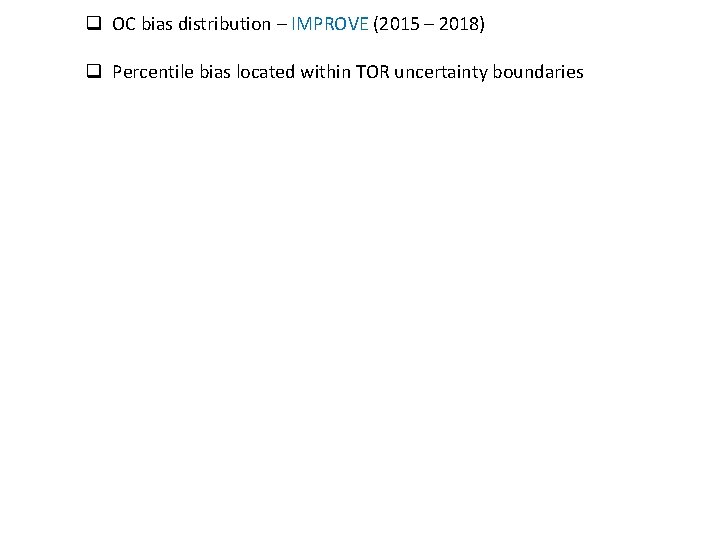
q OC bias distribution – IMPROVE (2015 – 2018) q Percentile bias located within TOR uncertainty boundaries
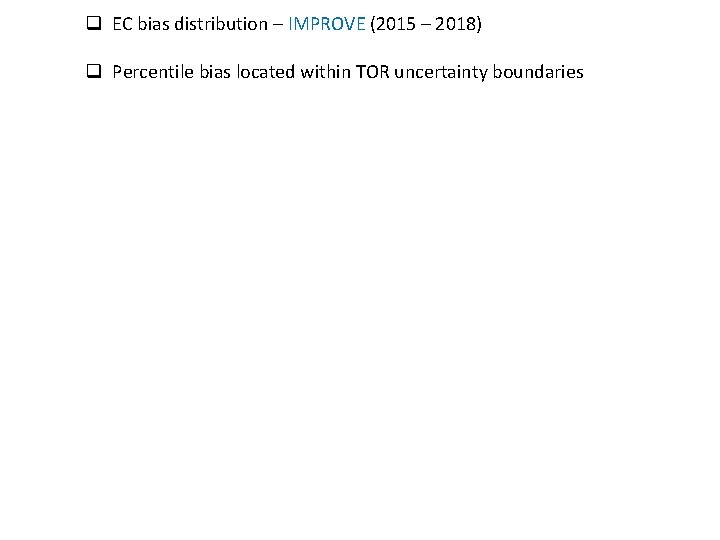
q EC bias distribution – IMPROVE (2015 – 2018) q Percentile bias located within TOR uncertainty boundaries
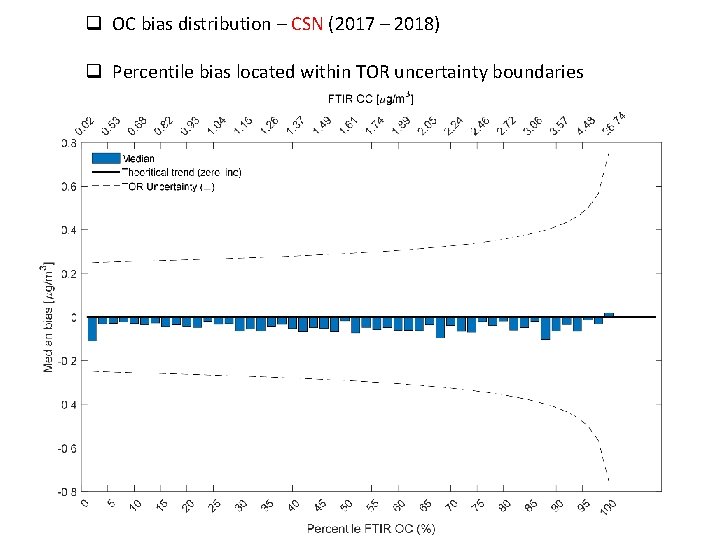
q OC bias distribution – CSN (2017 – 2018) q Percentile bias located within TOR uncertainty boundaries
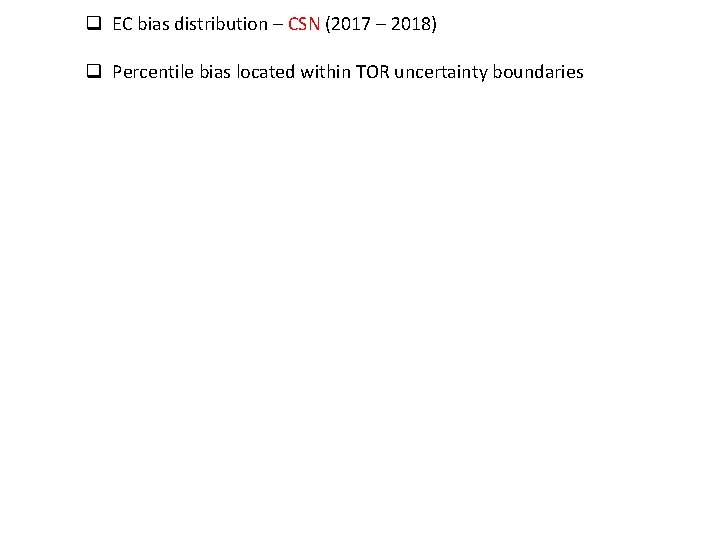
q EC bias distribution – CSN (2017 – 2018) q Percentile bias located within TOR uncertainty boundaries
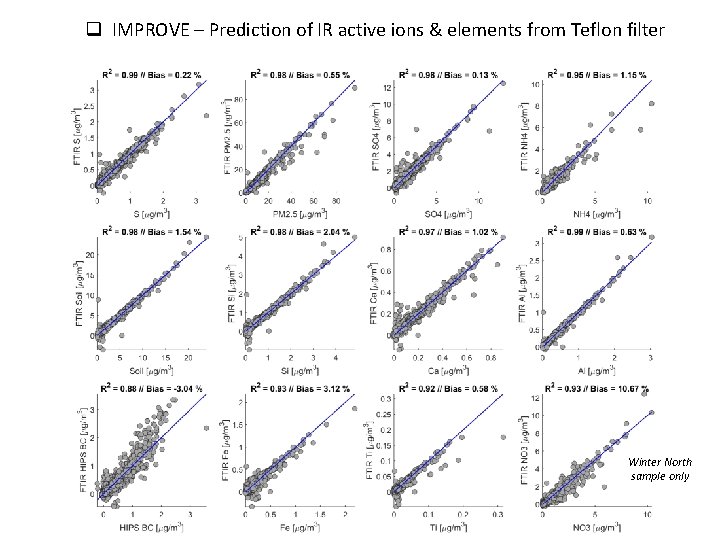
q IMPROVE – Prediction of IR active ions & elements from Teflon filter Winter North sample only
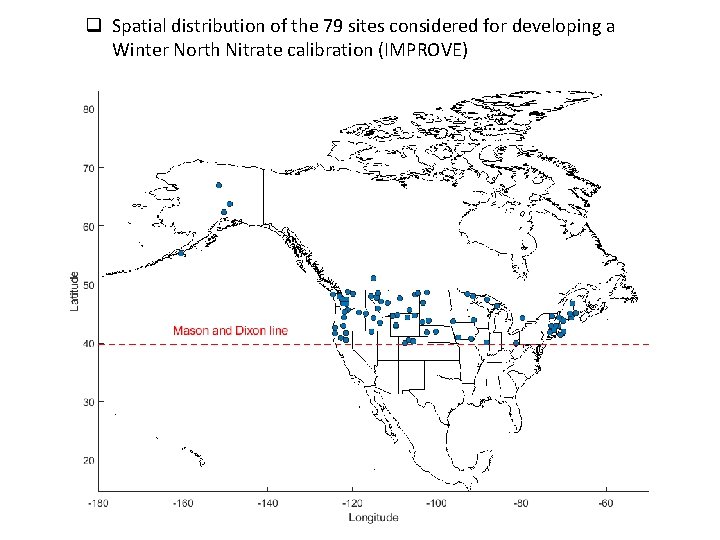
q Spatial distribution of the 79 sites considered for developing a Winter North Nitrate calibration (IMPROVE)
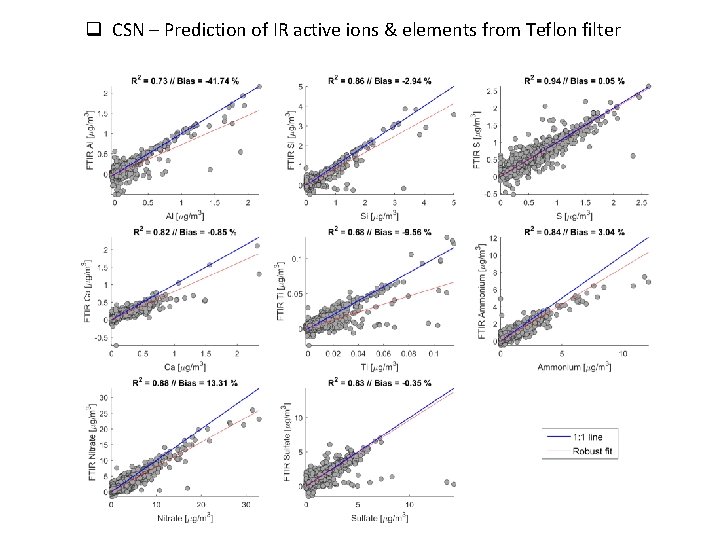
q CSN – Prediction of IR active ions & elements from Teflon filter
Ftir touch screen
Jacquinot advantage
Ftir advantages and disadvantages
Ftir principle and theory
Ftir spectrometer
Difference between ir and raman spectroscopy
Ftir instrument principle
Ftir
Ftir spectra
Ftir troubleshooting
Ftir
Aromatic compound ir spectrum
Difference between virtual circuit and datagram subnet
Backbone networks in computer networks
Swedish csn
Site:slidetodoc.com
Bodový svar značení
John romn
čsn 341010
Hold blast surface passivator
När får man csn
čsn 730540-2
čsn 73 4108 hygienická zařízení a šatny
čsn en 61936-1
čsn 01 4915
čsn 02 1036
Quai de willebroeck
Linceo csn
What does pending buyer action mean in coupa
Součinitel prostupu tepla dveře
čsn 01 6910
Cca csn review
čsn 73 6102
Csn academic probation
Csn ladok
It's your turn to make predictions
Why is making predictions important in reading
Good readers making predictions by
Barry smith predictions
Blackberry stock us
Simple shape poems
Assumptions generalizations predictions vs. conclusion
Can you make predictions about tech devices
Will may might for predictions
Limitations of punnett squares in large populations
Take a look at the following pictures and answer
How is experimental probability used to make predictions