ECGS 017 Fisheries management Talk nr 3 Risk
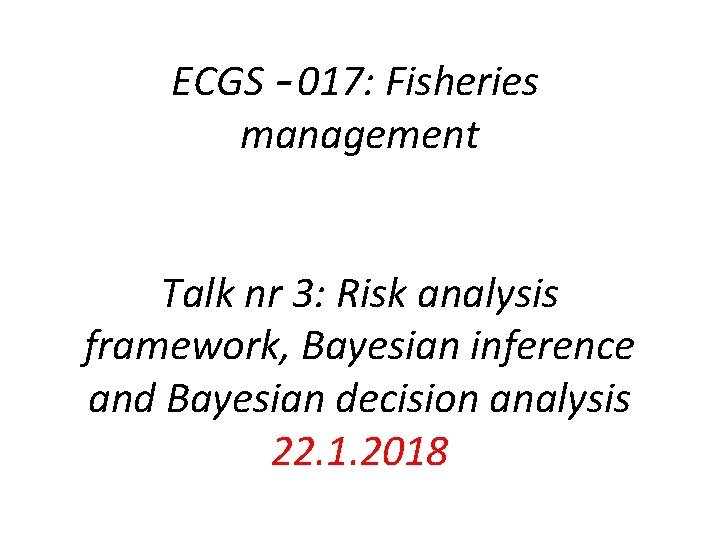
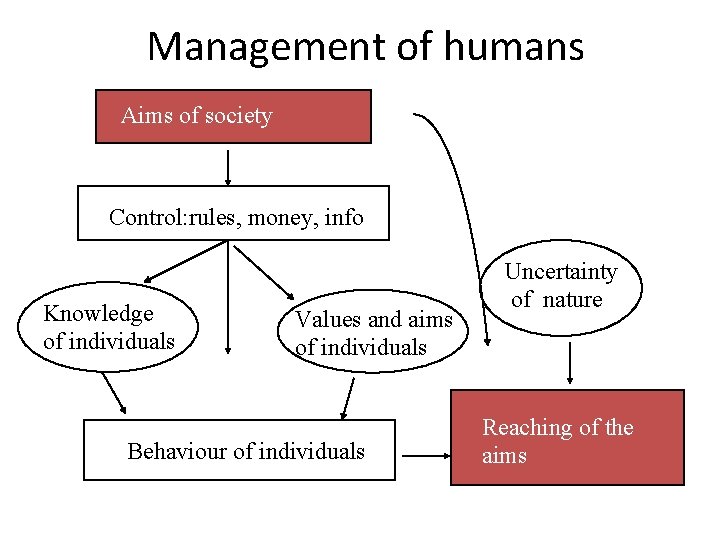
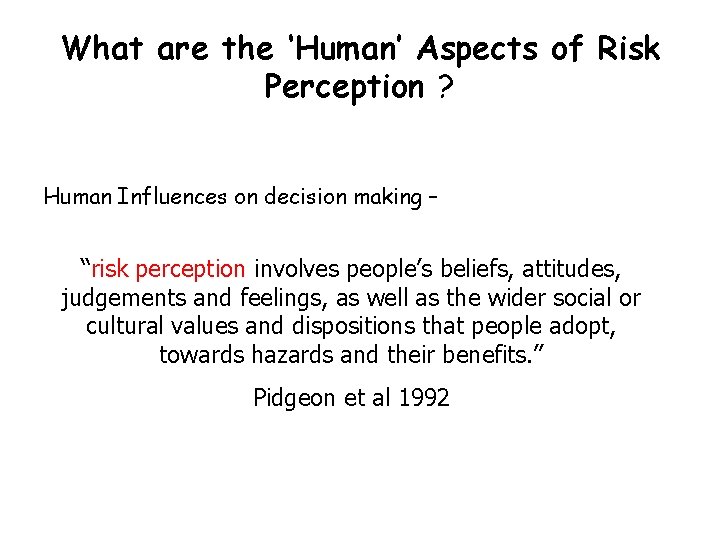
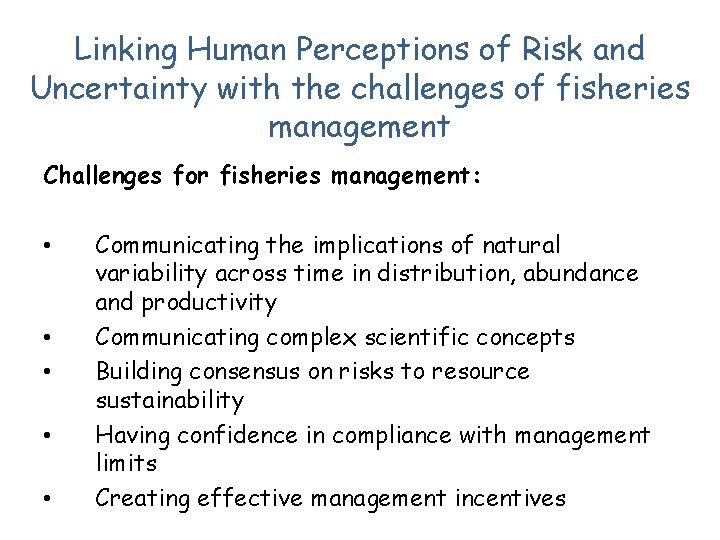
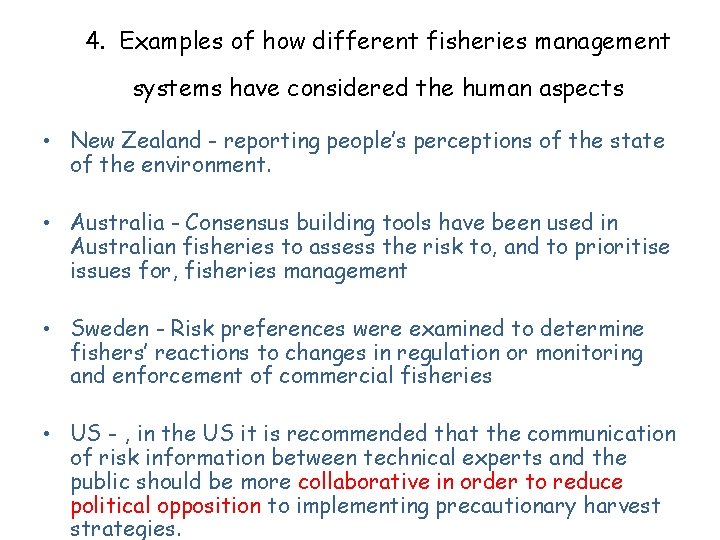
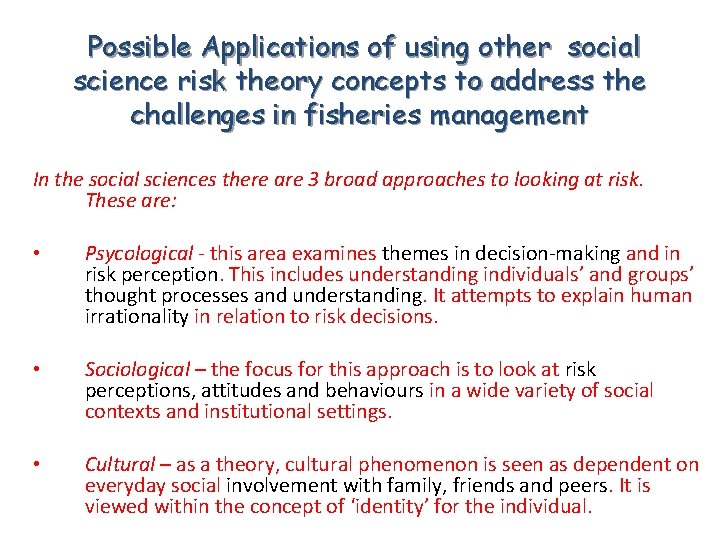
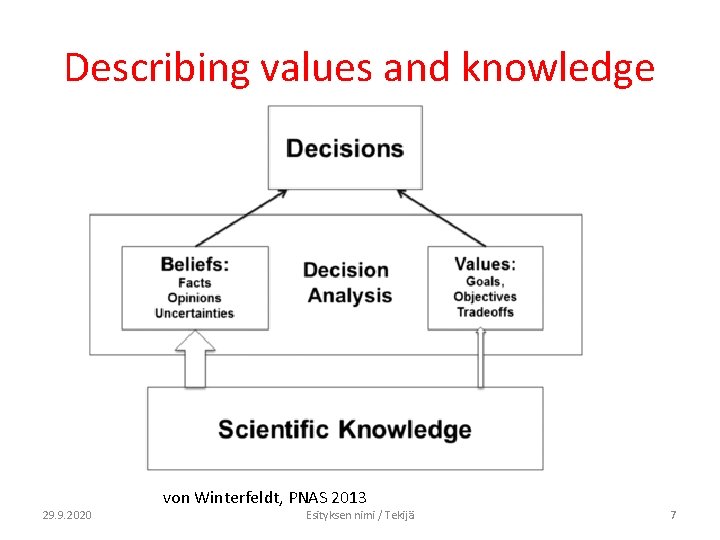
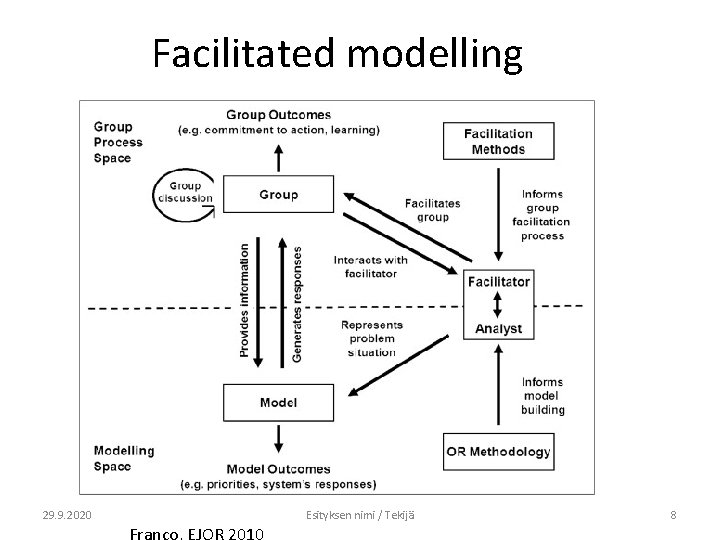
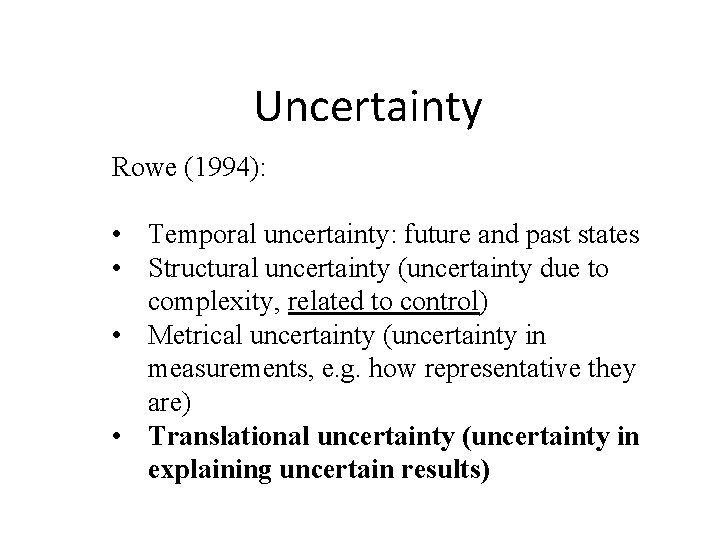
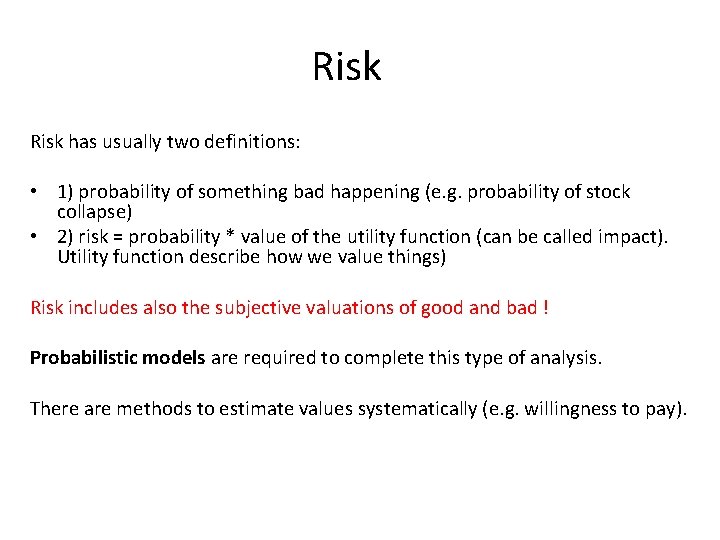
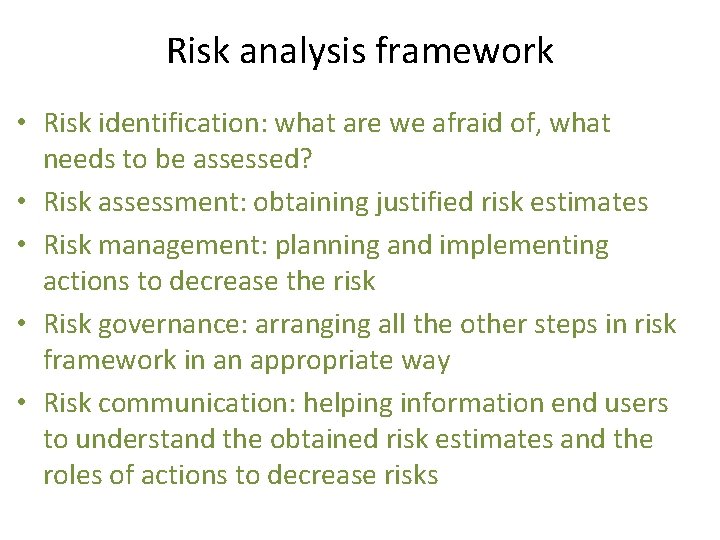
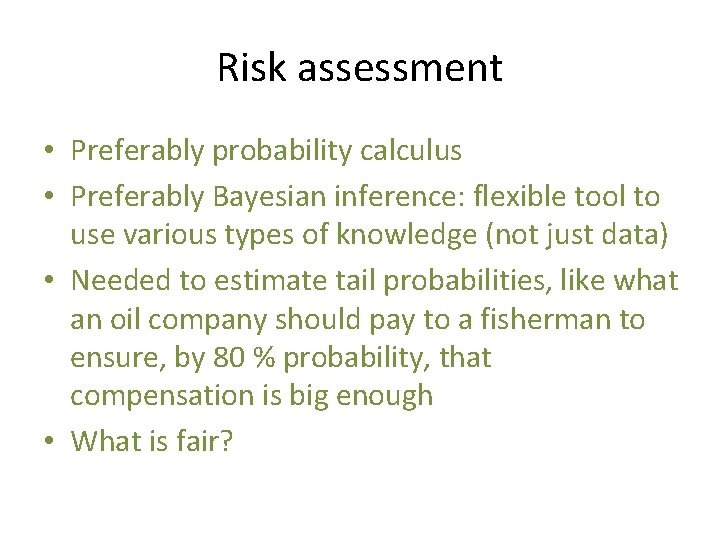
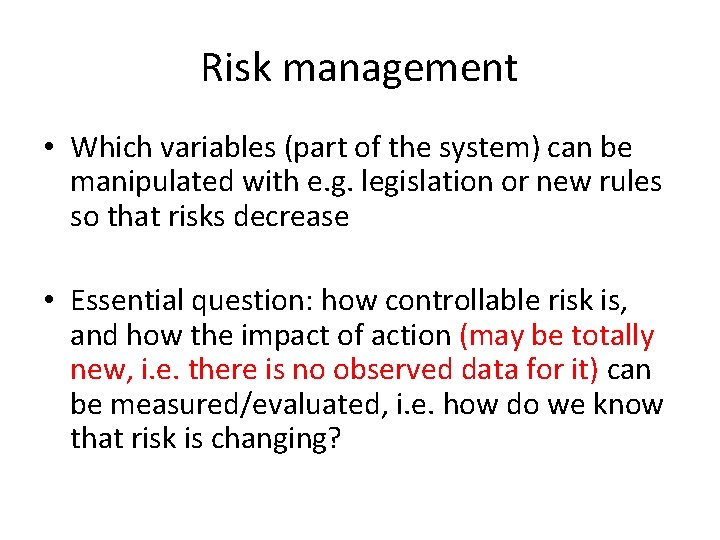
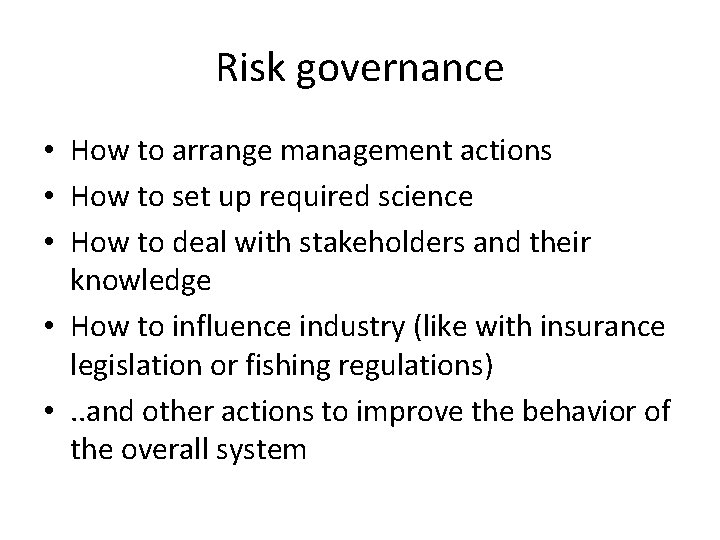
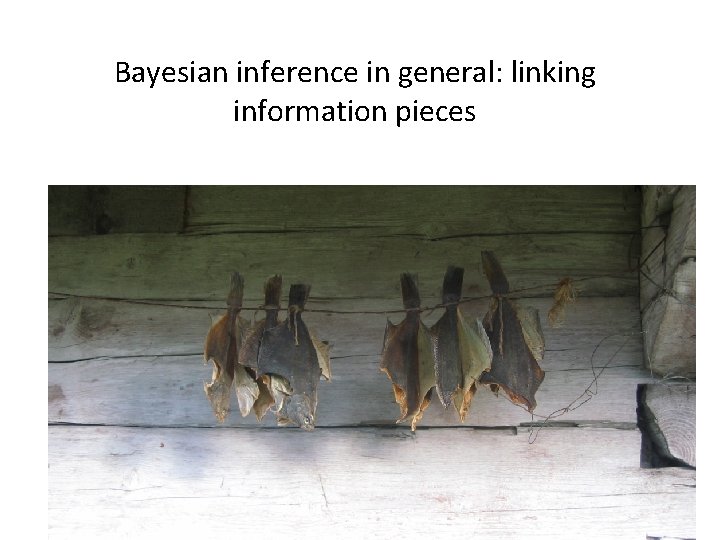
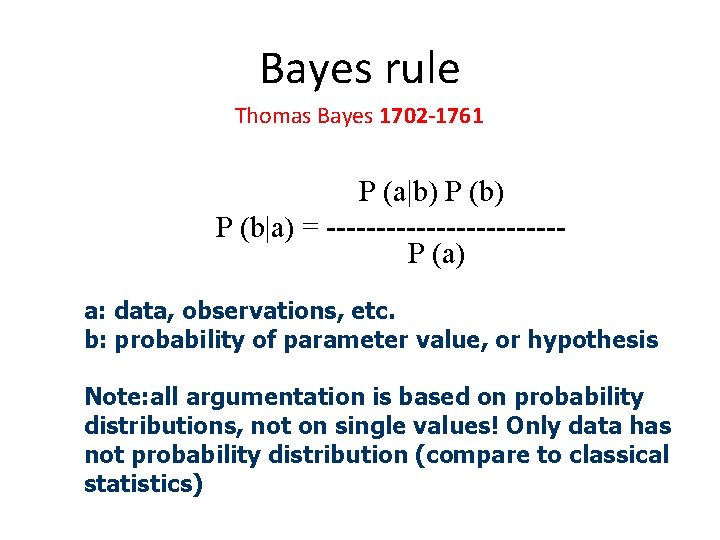
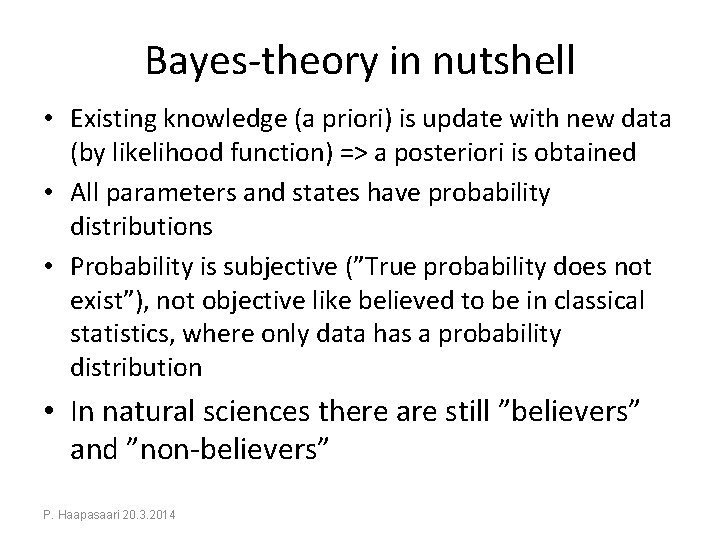
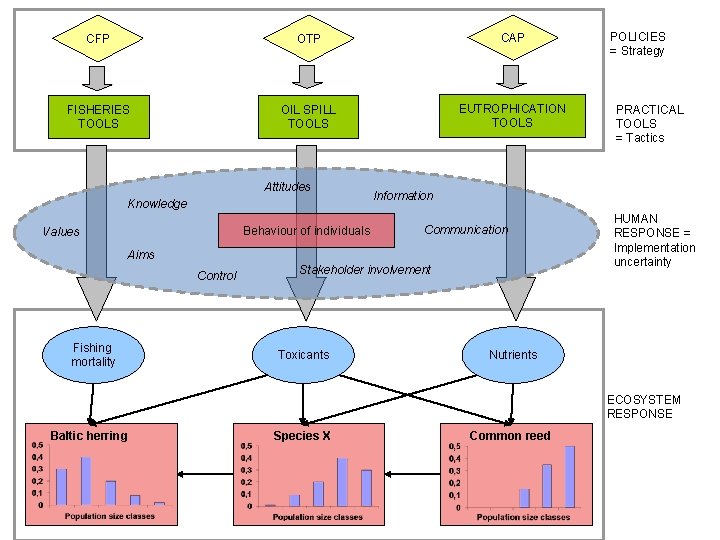
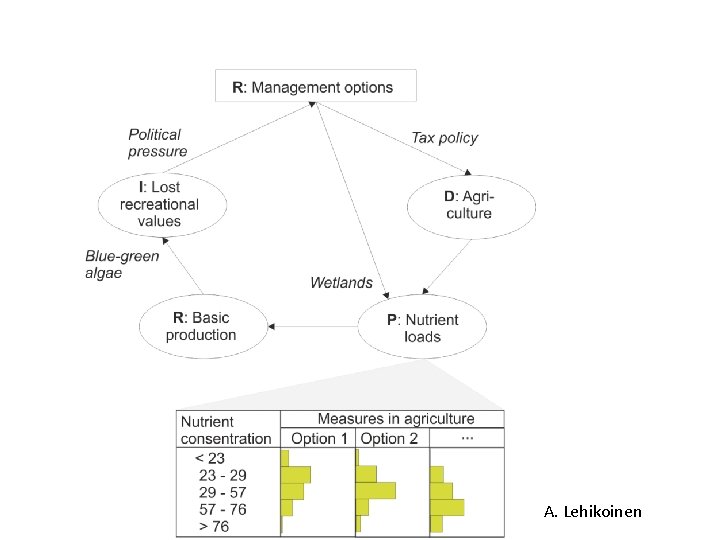
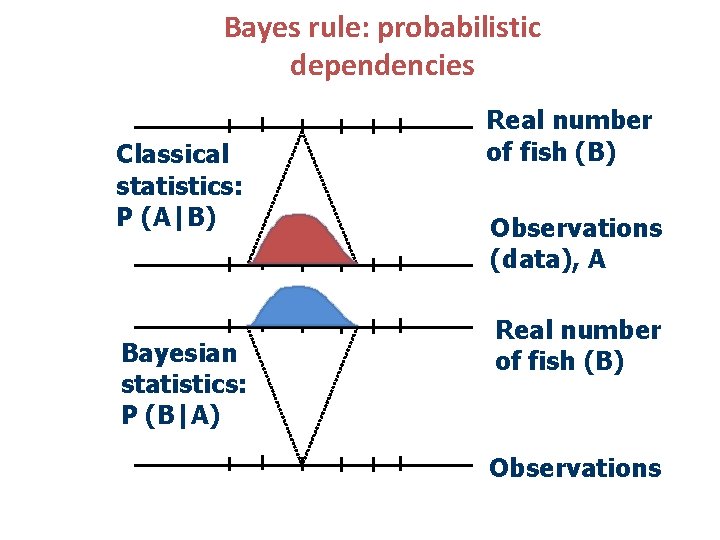
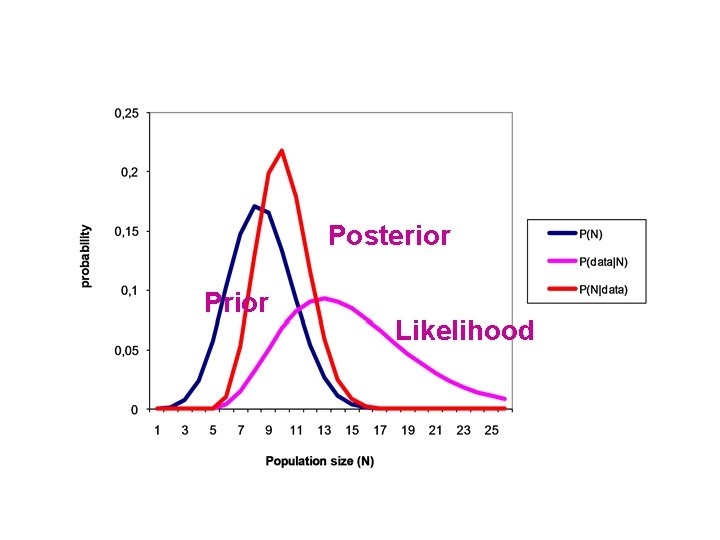
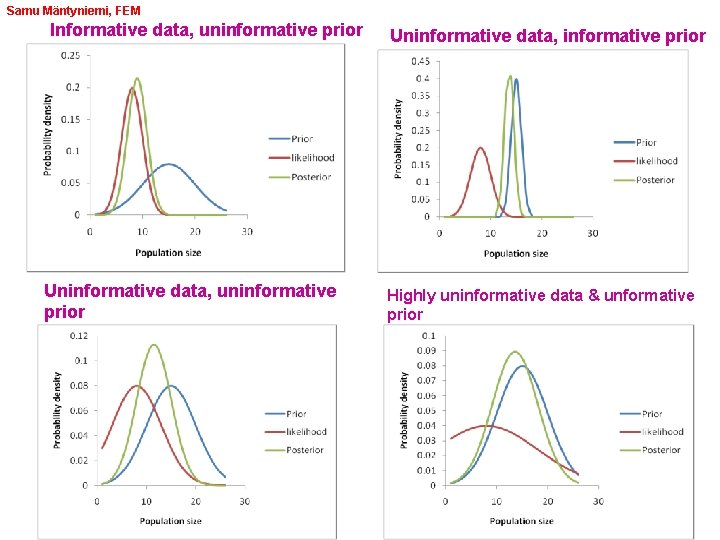
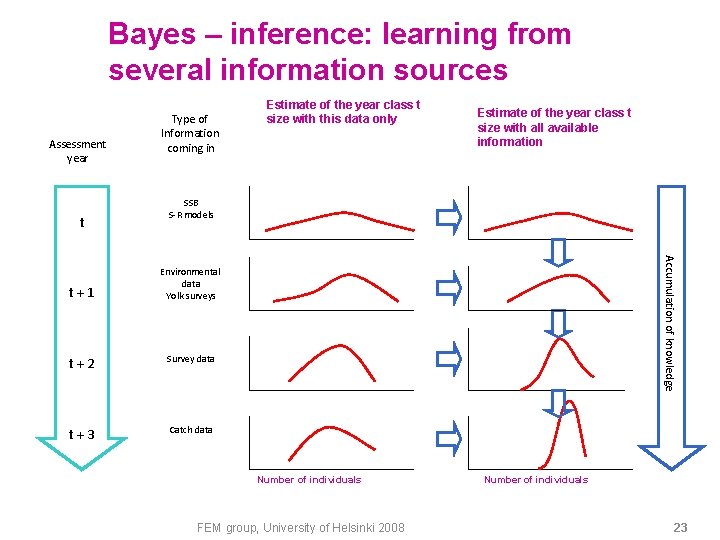
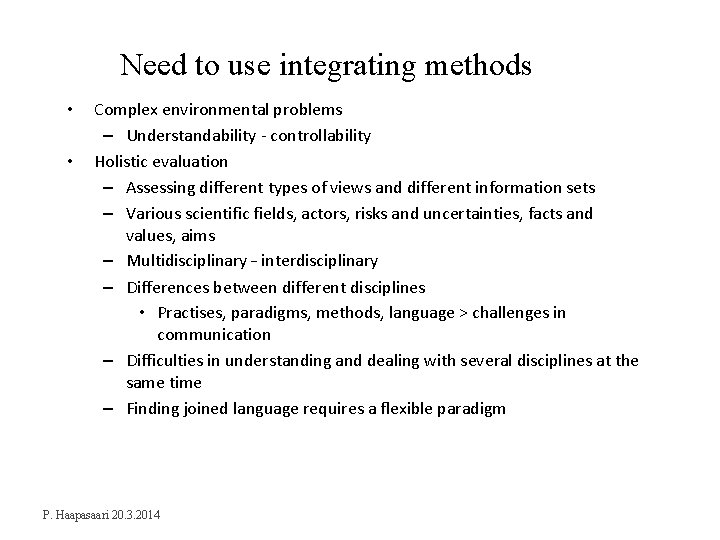
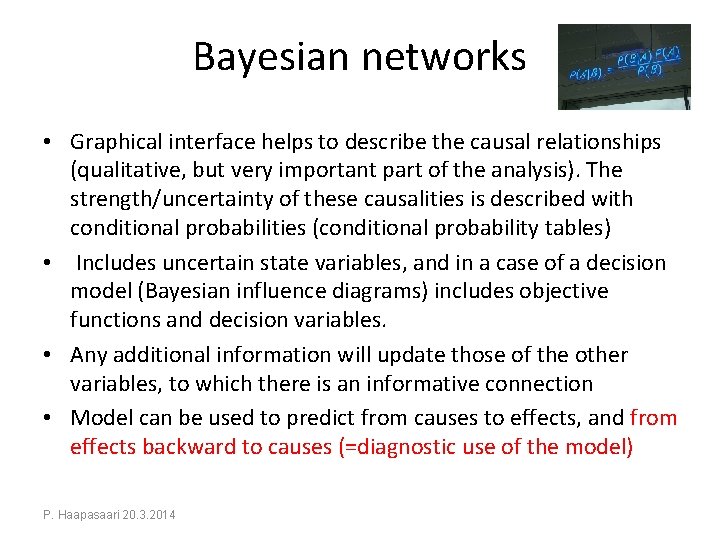
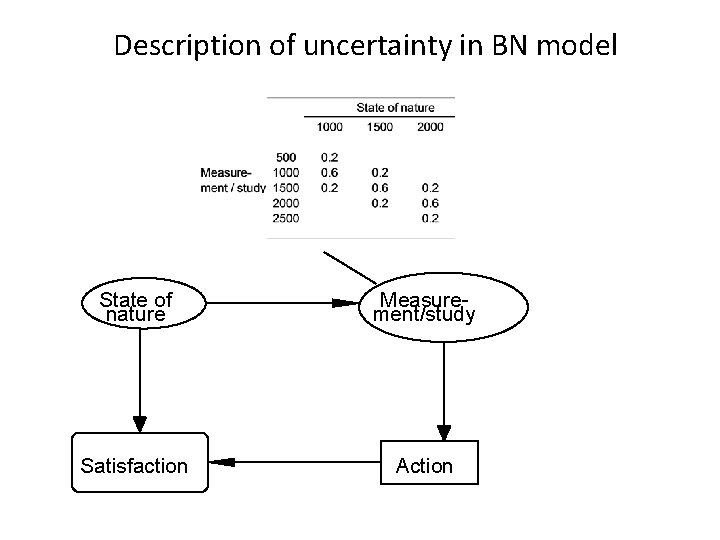
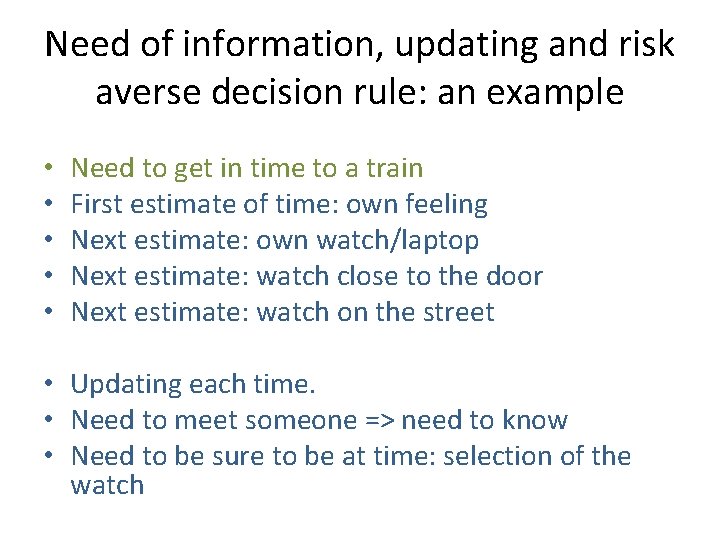
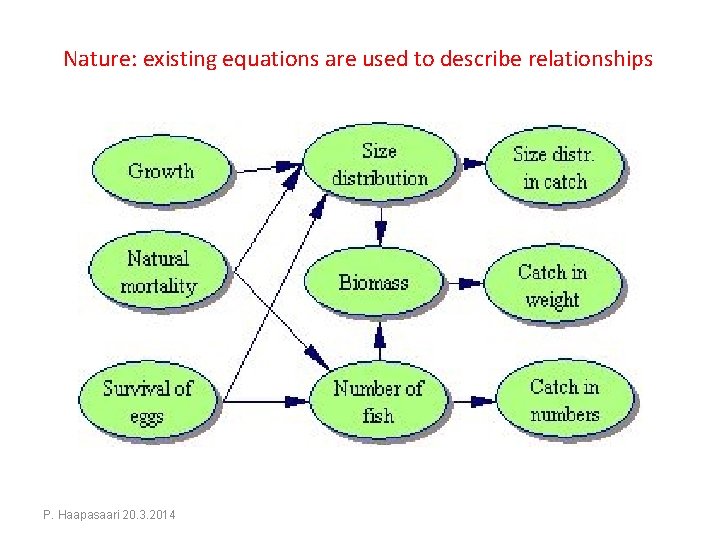
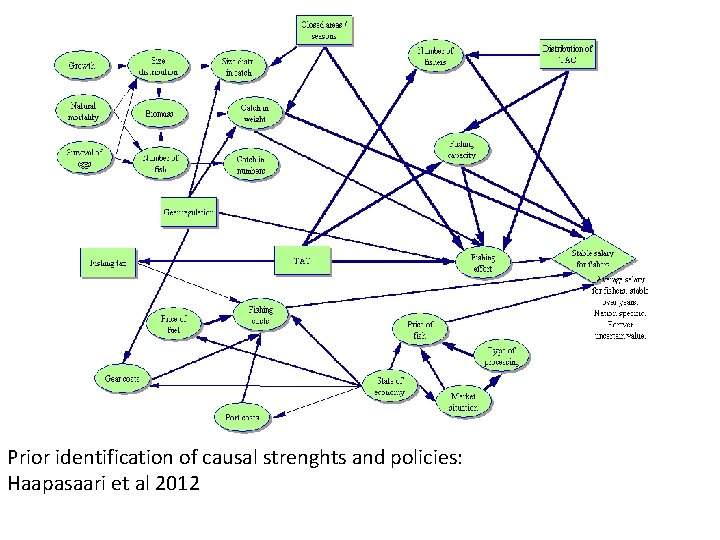
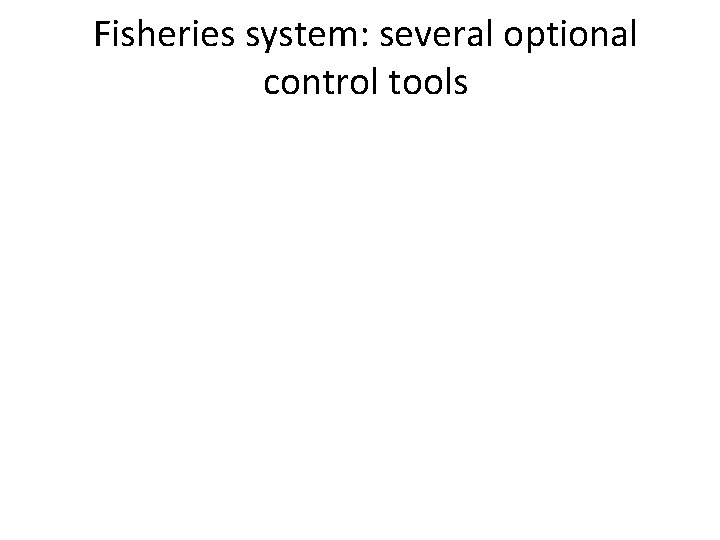
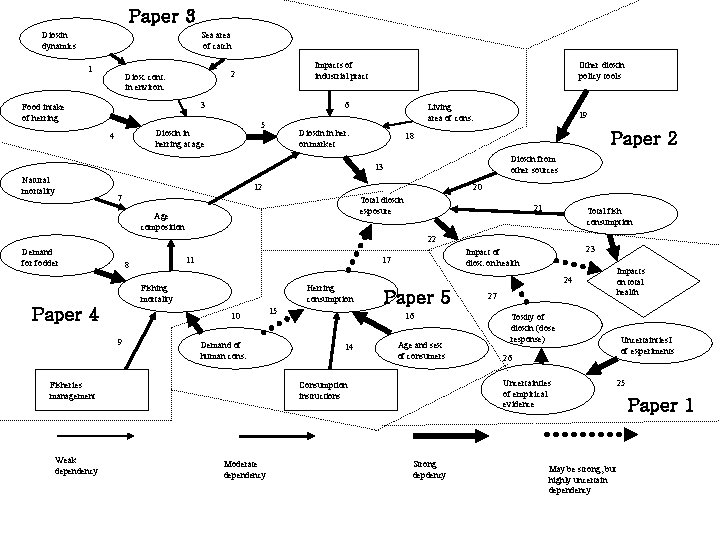
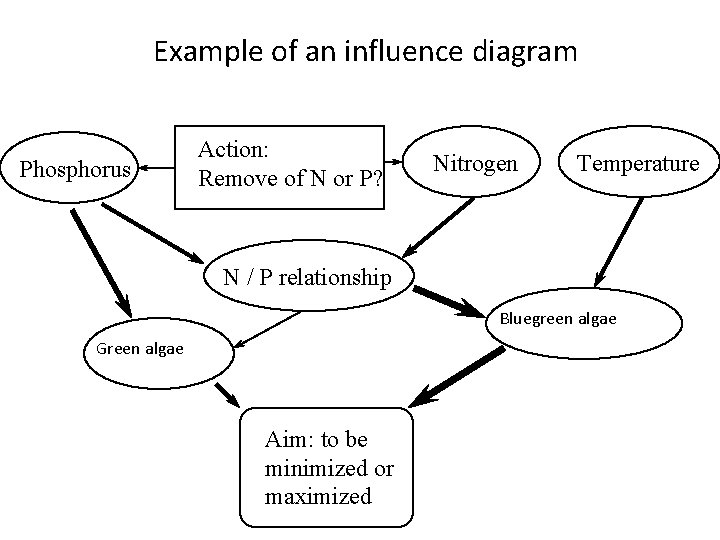
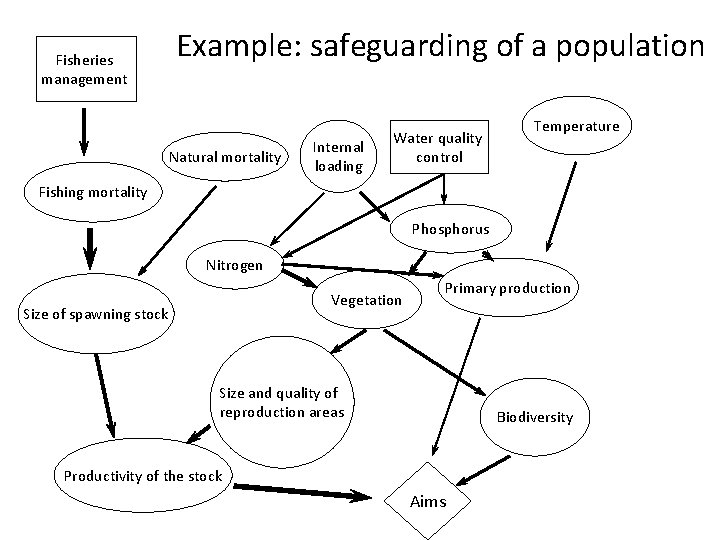
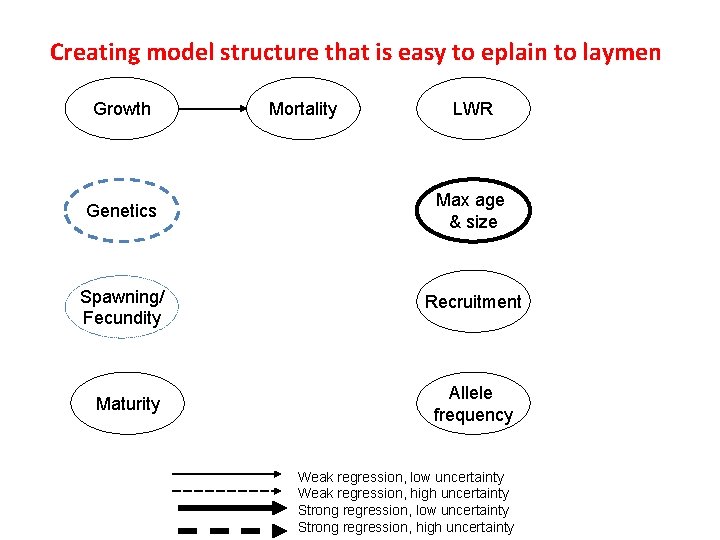
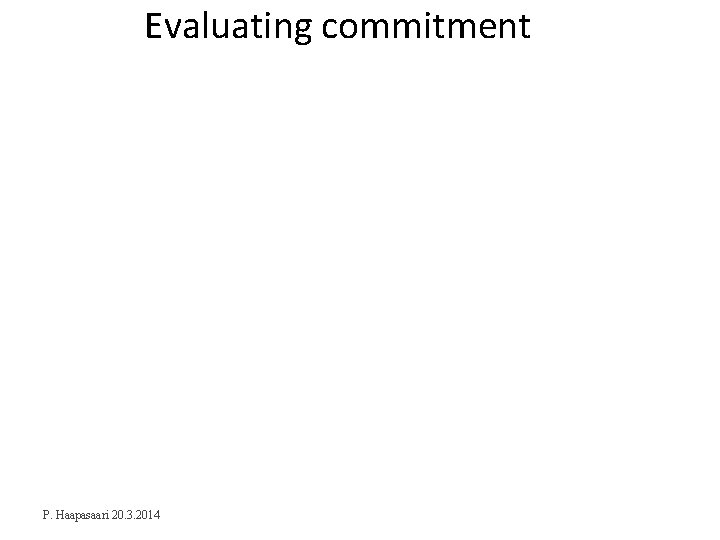
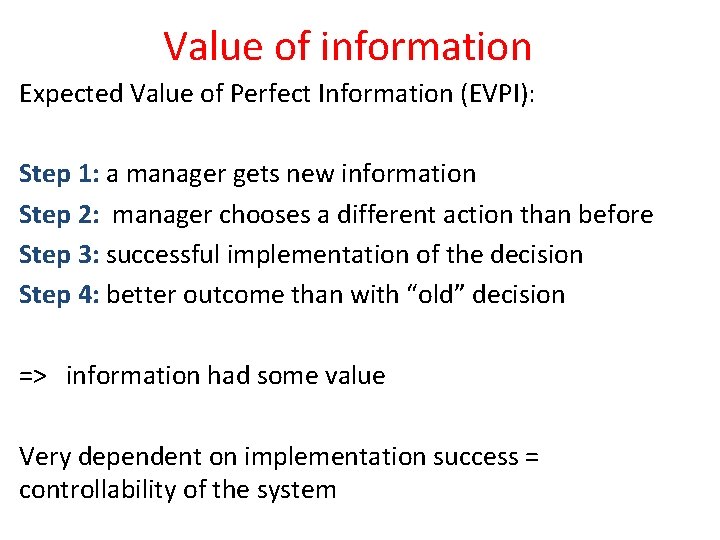
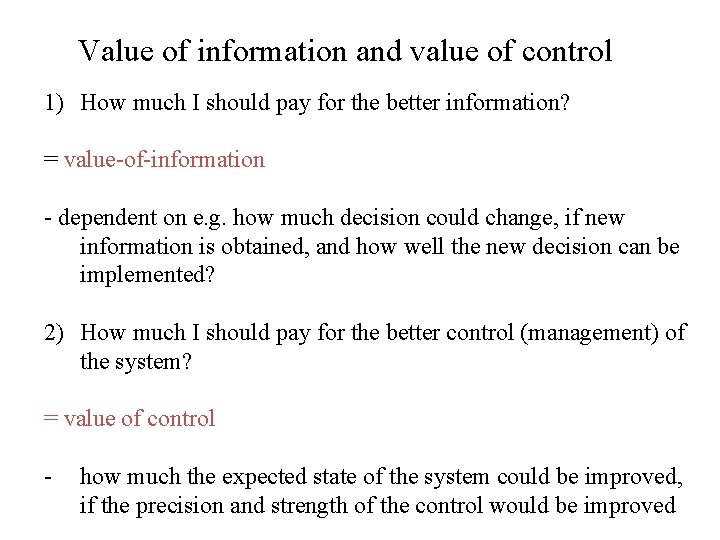
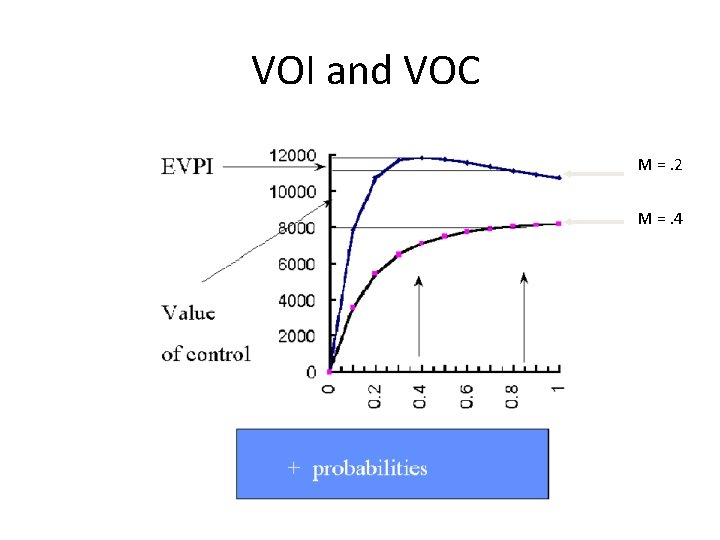
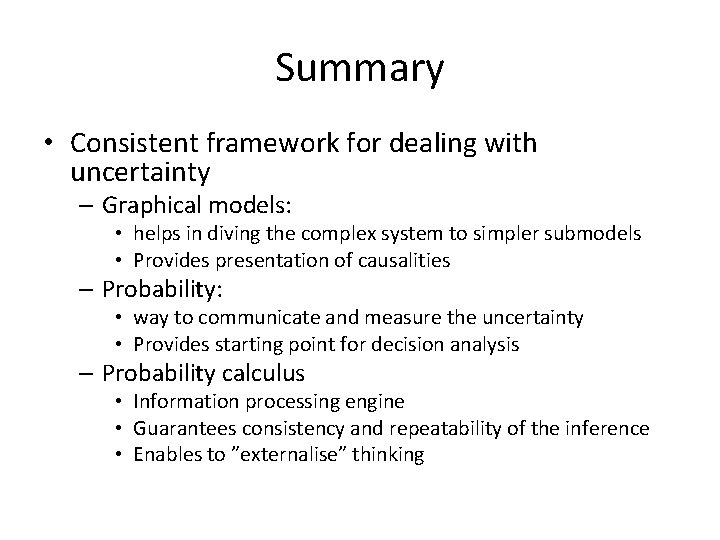
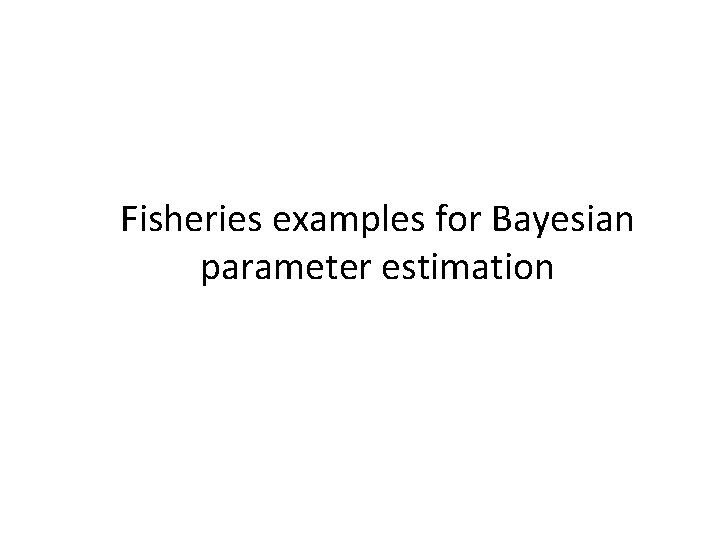
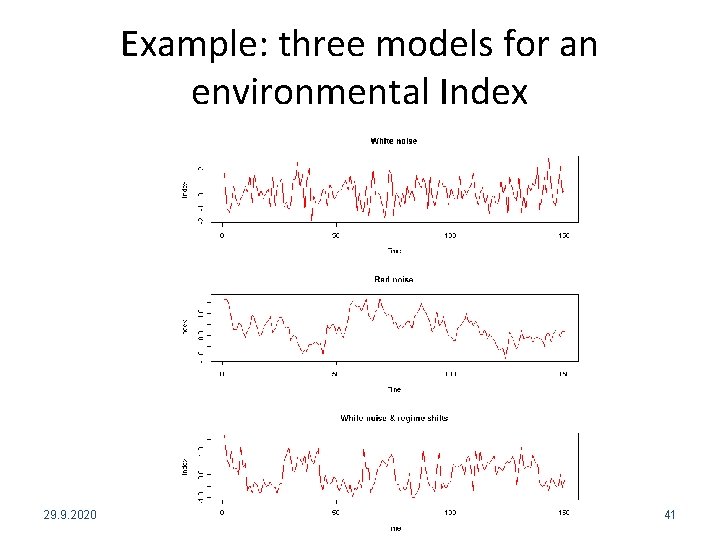
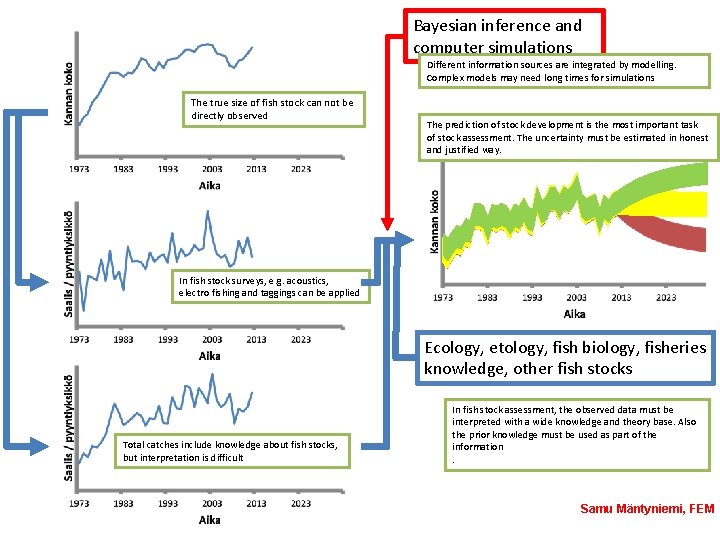
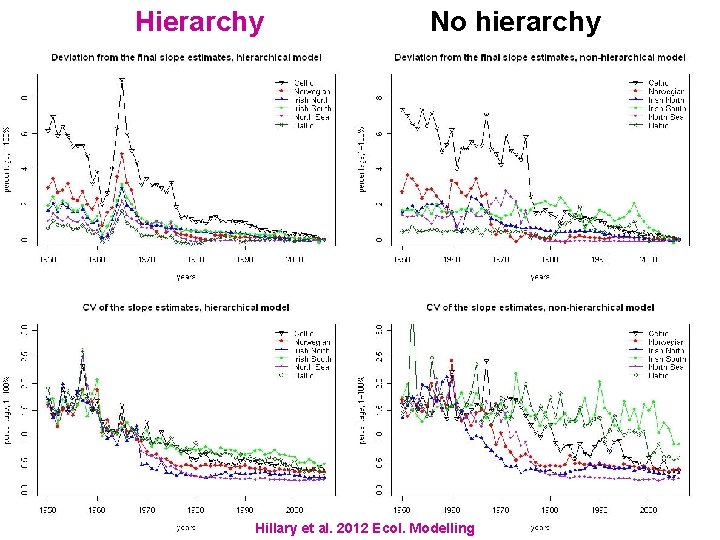
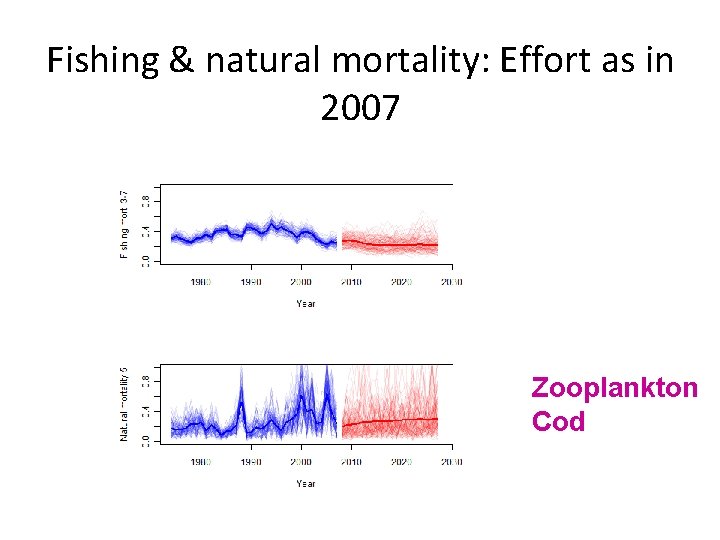
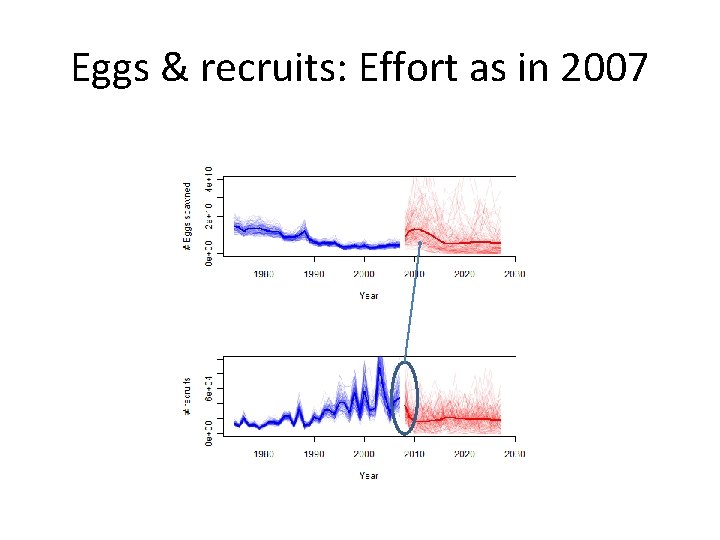
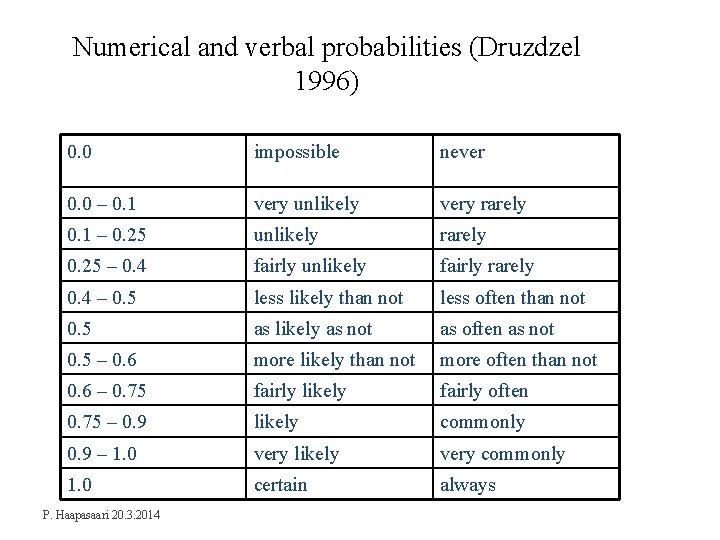
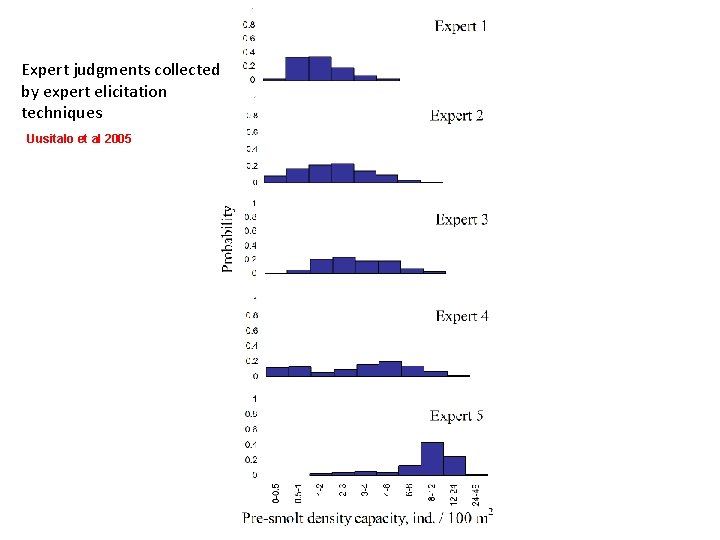
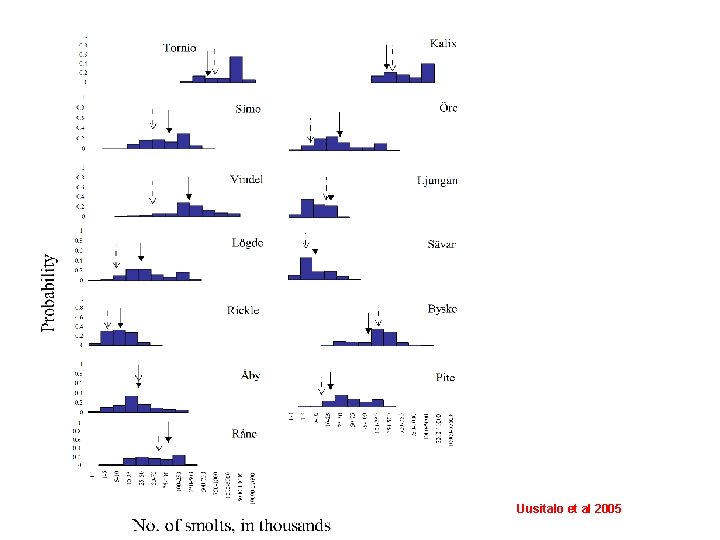
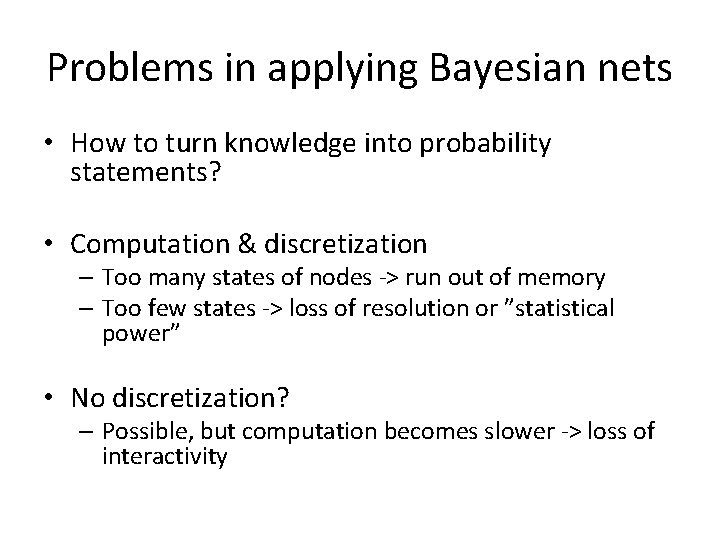
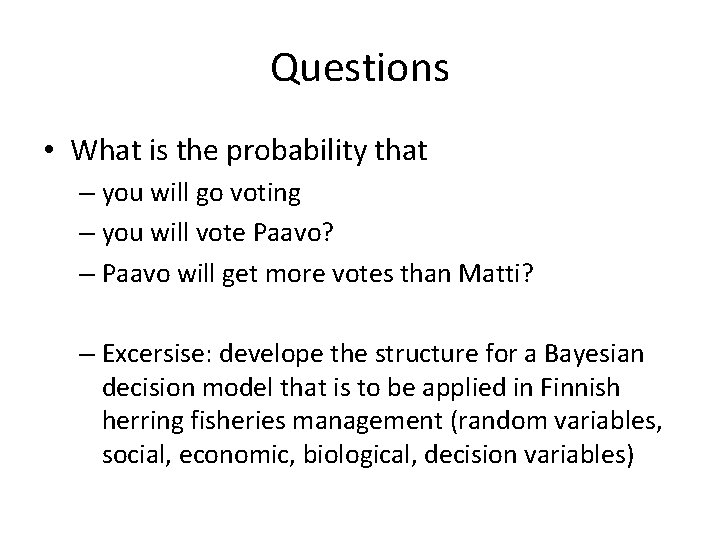
- Slides: 50
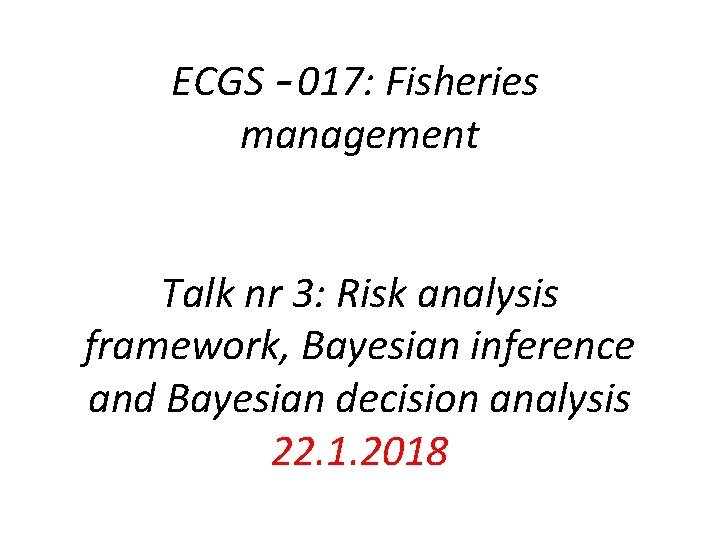
ECGS – 017: Fisheries management Talk nr 3: Risk analysis framework, Bayesian inference and Bayesian decision analysis 22. 1. 2018
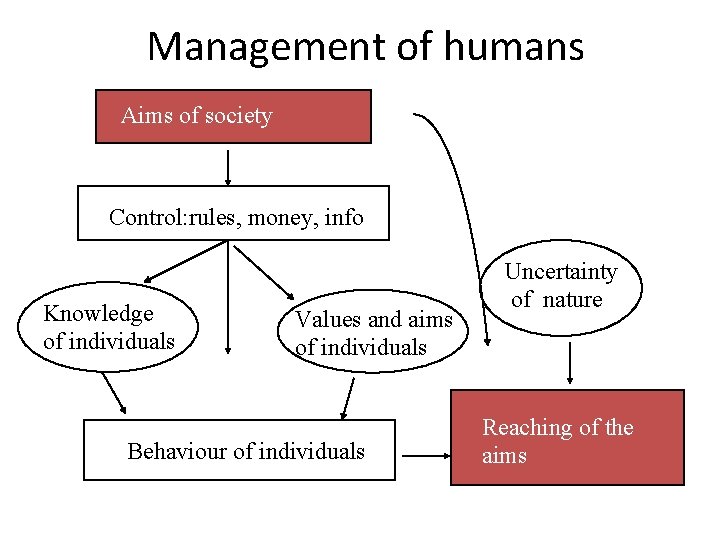
Management of humans Aims of society Control: rules, money, info Knowledge of individuals Values and aims of individuals Behaviour of individuals Uncertainty of nature Reaching of the aims
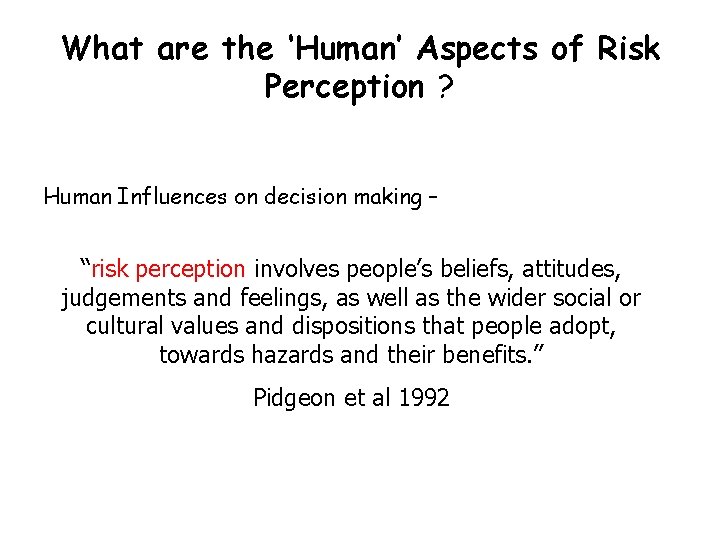
What are the ‘Human’ Aspects of Risk Perception ? Human Influences on decision making – “risk perception involves people’s beliefs, attitudes, judgements and feelings, as well as the wider social or cultural values and dispositions that people adopt, towards hazards and their benefits. ” Pidgeon et al 1992
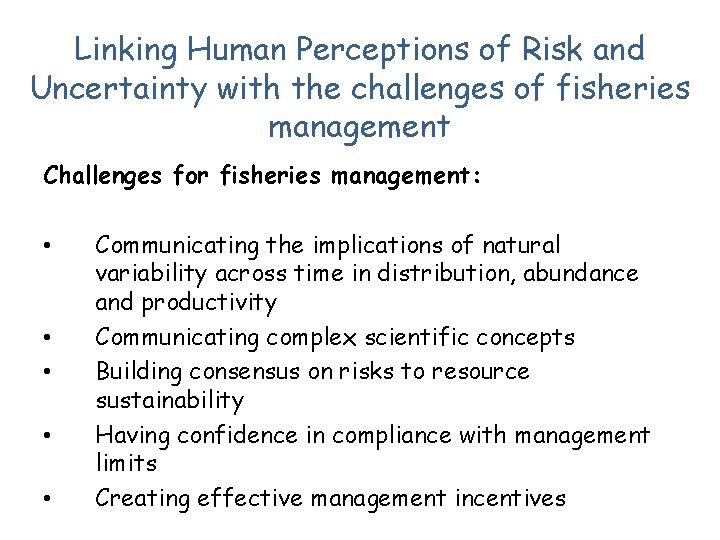
Linking Human Perceptions of Risk and Uncertainty with the challenges of fisheries management Challenges for fisheries management: • • • Communicating the implications of natural variability across time in distribution, abundance and productivity Communicating complex scientific concepts Building consensus on risks to resource sustainability Having confidence in compliance with management limits Creating effective management incentives
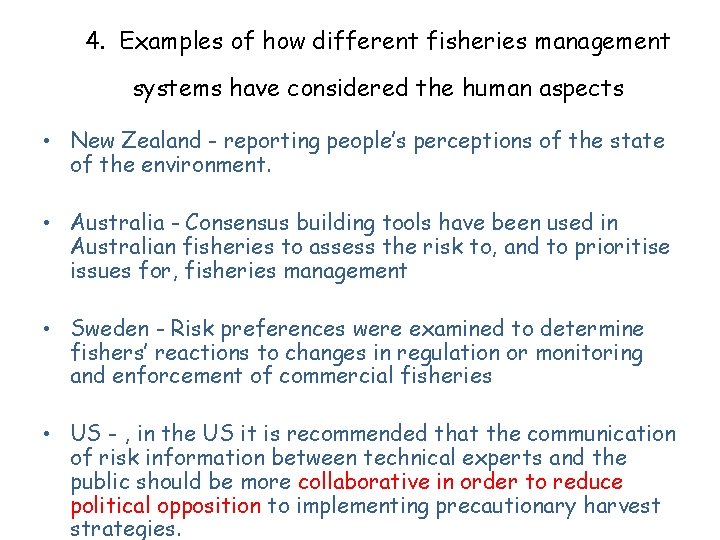
4. Examples of how different fisheries management systems have considered the human aspects • New Zealand - reporting people’s perceptions of the state of the environment. • Australia - Consensus building tools have been used in Australian fisheries to assess the risk to, and to prioritise issues for, fisheries management • Sweden - Risk preferences were examined to determine fishers’ reactions to changes in regulation or monitoring and enforcement of commercial fisheries • US - , in the US it is recommended that the communication of risk information between technical experts and the public should be more collaborative in order to reduce political opposition to implementing precautionary harvest strategies.
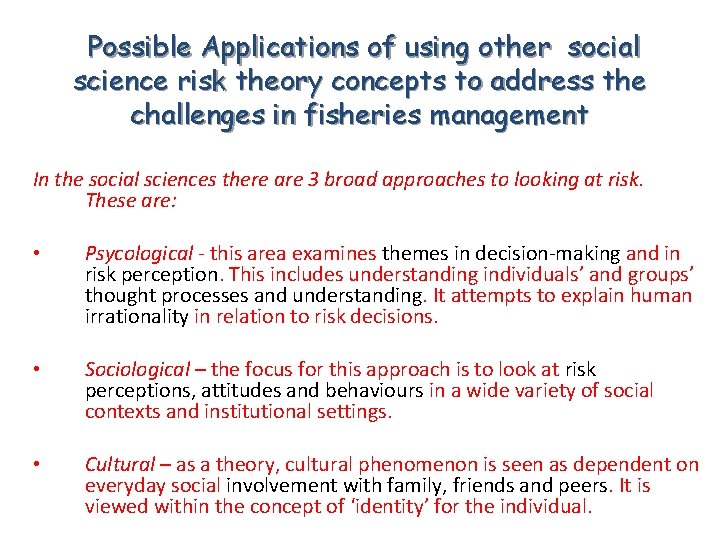
Possible Applications of using other social science risk theory concepts to address the challenges in fisheries management In the social sciences there are 3 broad approaches to looking at risk. These are: • Psycological - this area examines themes in decision-making and in risk perception. This includes understanding individuals’ and groups’ thought processes and understanding. It attempts to explain human irrationality in relation to risk decisions. • Sociological – the focus for this approach is to look at risk perceptions, attitudes and behaviours in a wide variety of social contexts and institutional settings. • Cultural – as a theory, cultural phenomenon is seen as dependent on everyday social involvement with family, friends and peers. It is viewed within the concept of ‘identity’ for the individual.
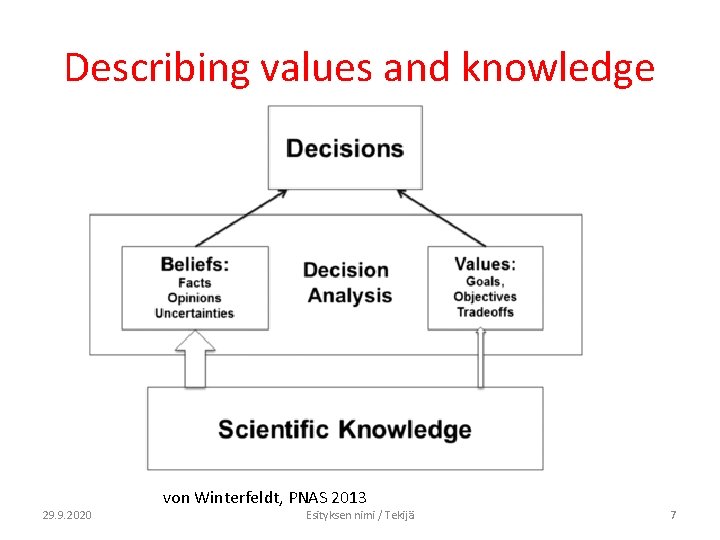
Describing values and knowledge 29. 9. 2020 von Winterfeldt, PNAS 2013 Esityksen nimi / Tekijä 7
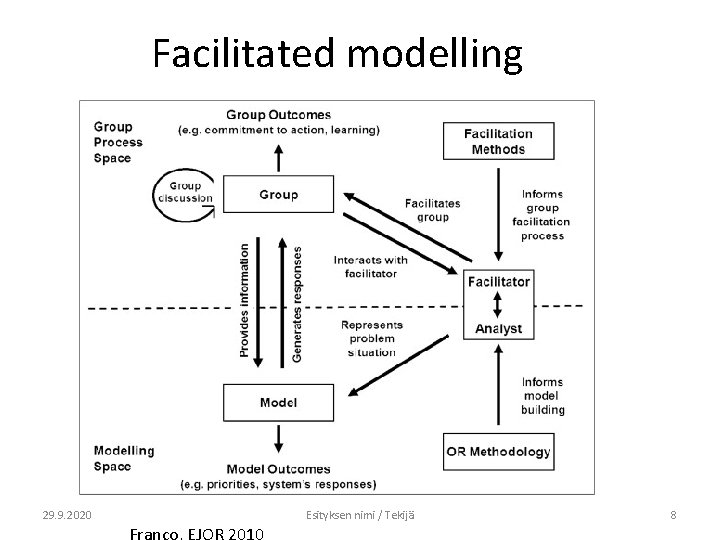
Facilitated modelling 29. 9. 2020 Esityksen nimi / Tekijä Franco, EJOR 2010 8
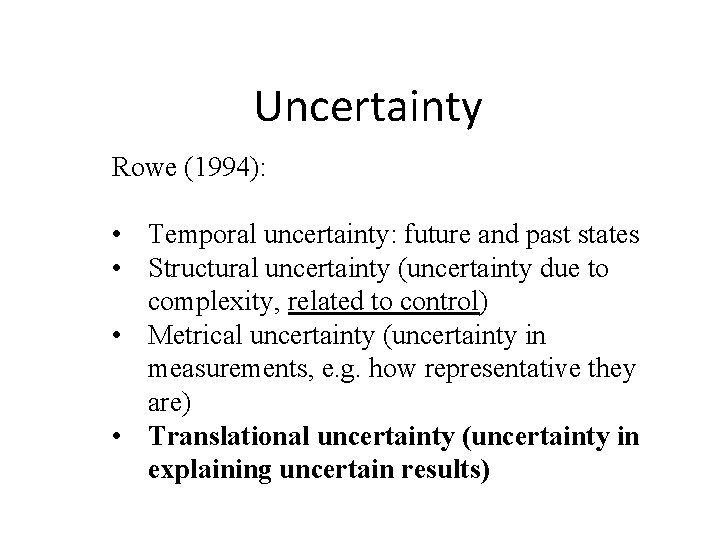
Uncertainty Rowe (1994): • Temporal uncertainty: future and past states • Structural uncertainty (uncertainty due to complexity, related to control) • Metrical uncertainty (uncertainty in measurements, e. g. how representative they are) • Translational uncertainty (uncertainty in explaining uncertain results)
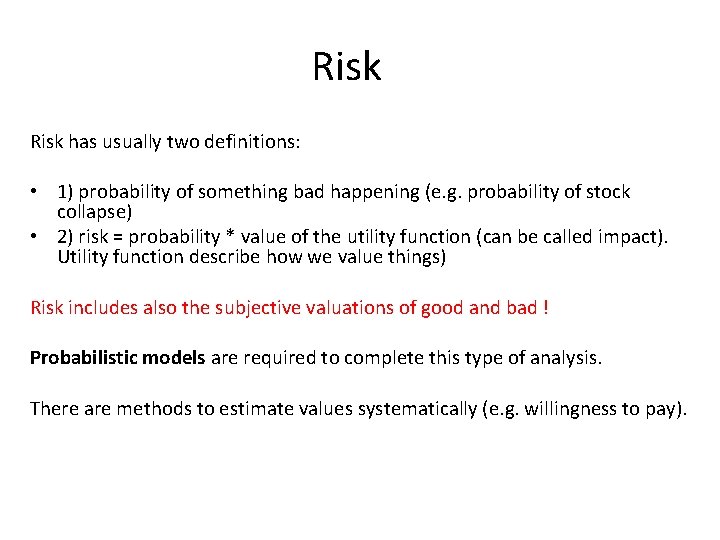
Risk has usually two definitions: • 1) probability of something bad happening (e. g. probability of stock collapse) • 2) risk = probability * value of the utility function (can be called impact). Utility function describe how we value things) Risk includes also the subjective valuations of good and bad ! Probabilistic models are required to complete this type of analysis. There are methods to estimate values systematically (e. g. willingness to pay).
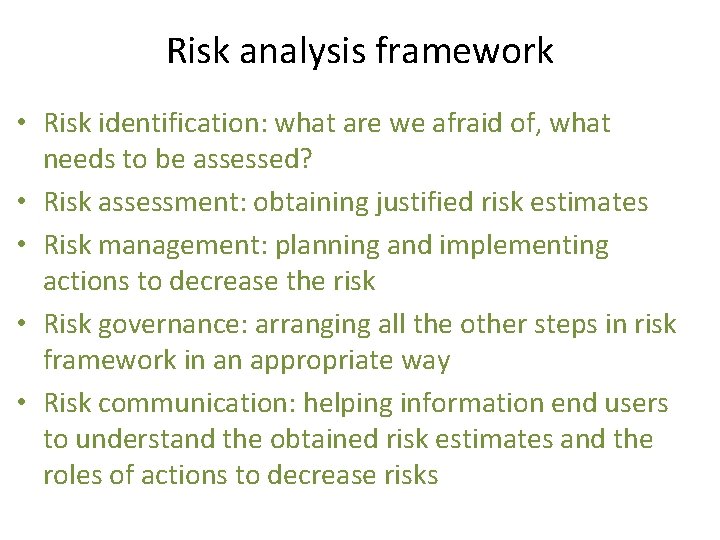
Risk analysis framework • Risk identification: what are we afraid of, what needs to be assessed? • Risk assessment: obtaining justified risk estimates • Risk management: planning and implementing actions to decrease the risk • Risk governance: arranging all the other steps in risk framework in an appropriate way • Risk communication: helping information end users to understand the obtained risk estimates and the roles of actions to decrease risks
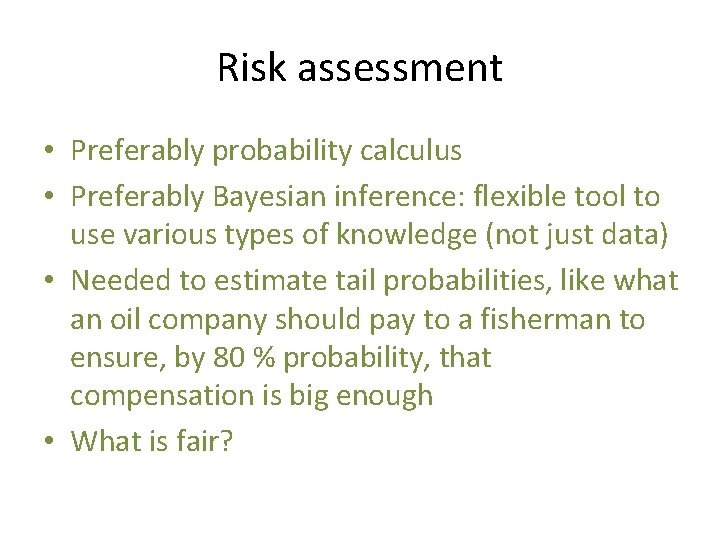
Risk assessment • Preferably probability calculus • Preferably Bayesian inference: flexible tool to use various types of knowledge (not just data) • Needed to estimate tail probabilities, like what an oil company should pay to a fisherman to ensure, by 80 % probability, that compensation is big enough • What is fair?
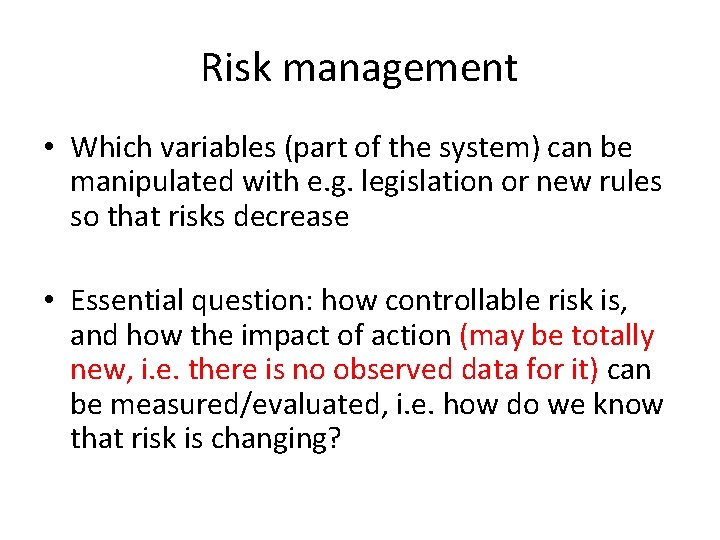
Risk management • Which variables (part of the system) can be manipulated with e. g. legislation or new rules so that risks decrease • Essential question: how controllable risk is, and how the impact of action (may be totally new, i. e. there is no observed data for it) can be measured/evaluated, i. e. how do we know that risk is changing?
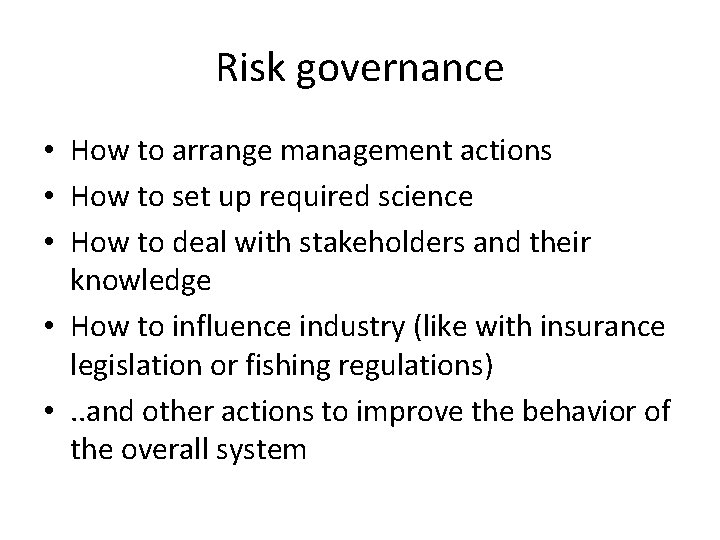
Risk governance • How to arrange management actions • How to set up required science • How to deal with stakeholders and their knowledge • How to influence industry (like with insurance legislation or fishing regulations) • . . and other actions to improve the behavior of the overall system
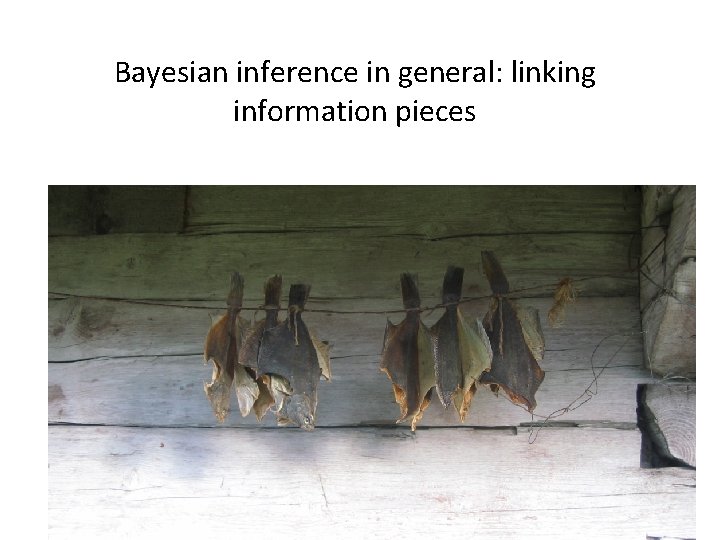
Bayesian inference in general: linking information pieces
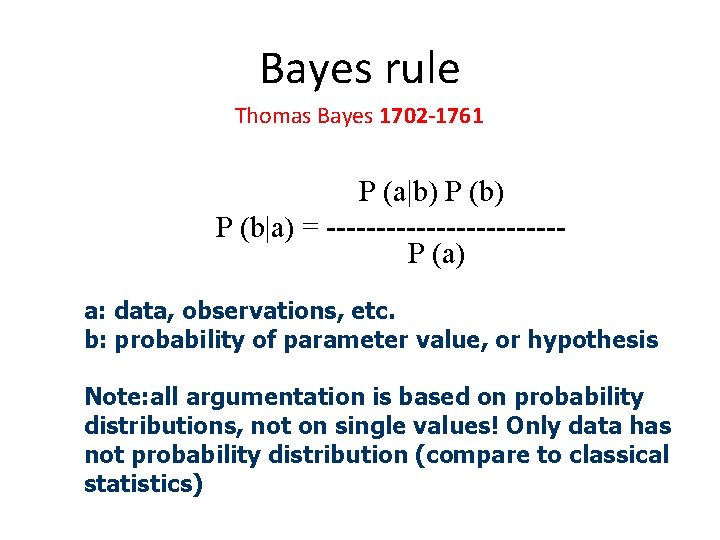
Bayes rule Thomas Bayes 1702 -1761 P (a|b) P (b|a) = ------------P (a) a: data, observations, etc. b: probability of parameter value, or hypothesis Note: all argumentation is based on probability distributions, not on single values! Only data has not probability distribution (compare to classical statistics)
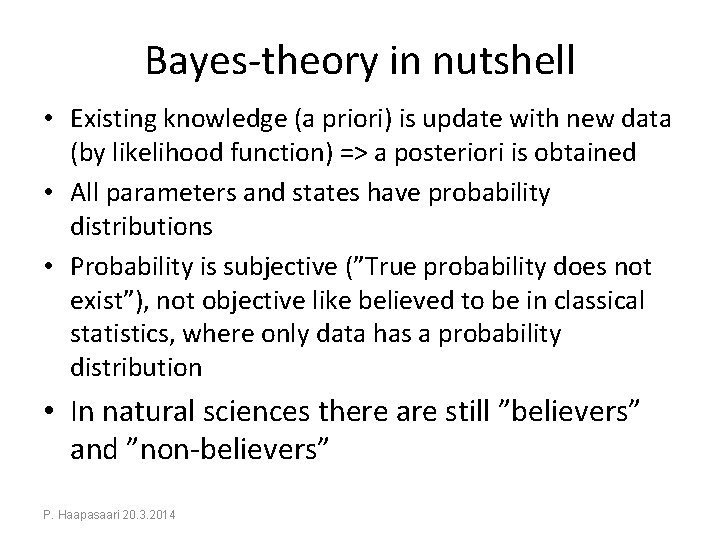
Bayes-theory in nutshell • Existing knowledge (a priori) is update with new data (by likelihood function) => a posteriori is obtained • All parameters and states have probability distributions • Probability is subjective (”True probability does not exist”), not objective like believed to be in classical statistics, where only data has a probability distribution • In natural sciences there are still ”believers” and ”non-believers” P. Haapasaari 20. 3. 2014
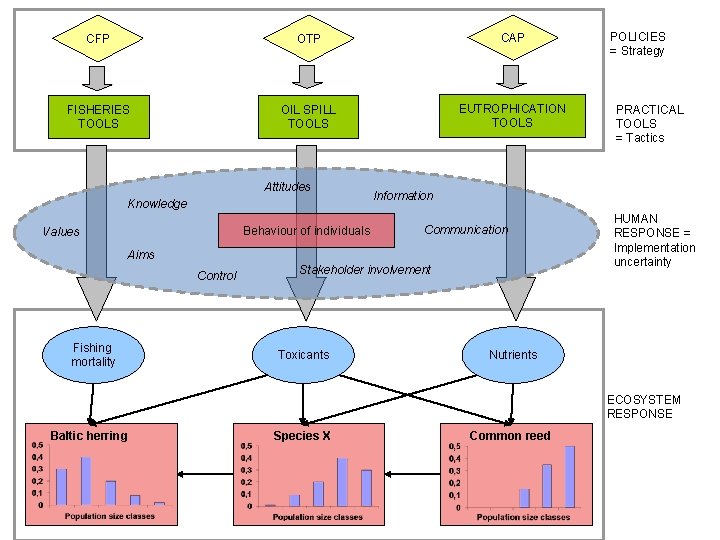
CFP OTP CAP FISHERIES TOOLS OIL SPILL TOOLS EUTROPHICATION TOOLS Attitudes Knowledge Behaviour of individuals Values Fishing mortality Communication Stakeholder involvement Toxicants PRACTICAL TOOLS = Tactics Information Aims Control POLICIES = Strategy HUMAN RESPONSE = Implementation uncertainty Nutrients ECOSYSTEM RESPONSE Baltic herring Species X Common reed
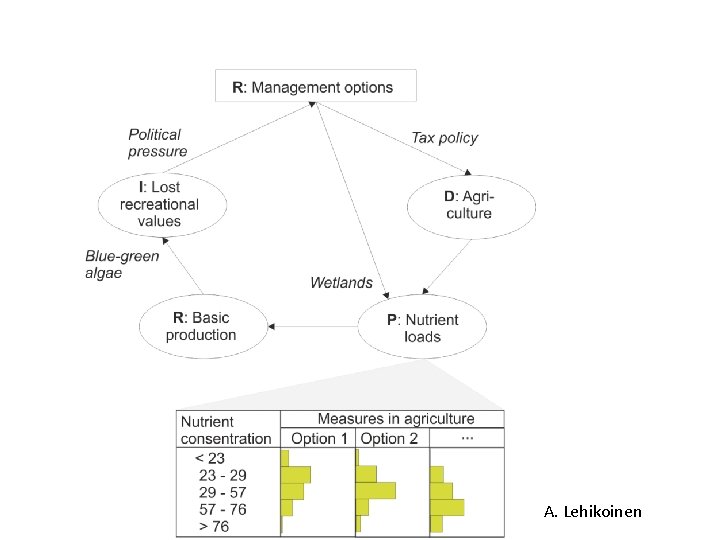
A. Lehikoinen
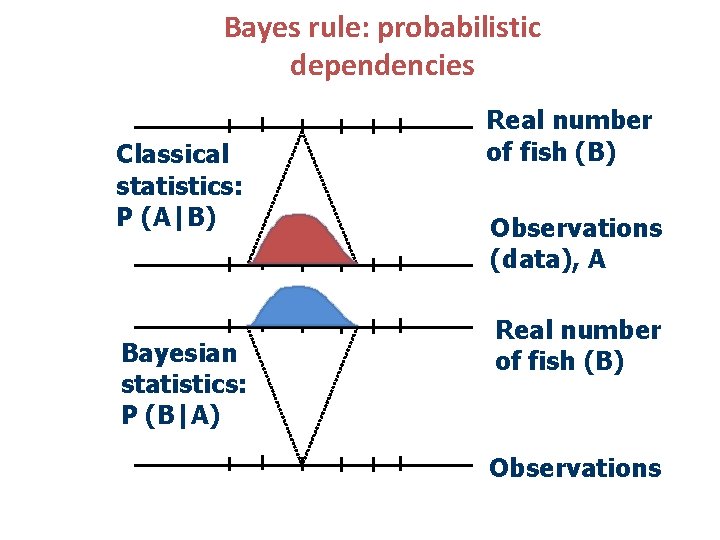
Bayes rule: probabilistic dependencies Classical statistics: P (A|B) Bayesian statistics: P (B|A) Real number of fish (B) Observations (data), A Real number of fish (B) Observations
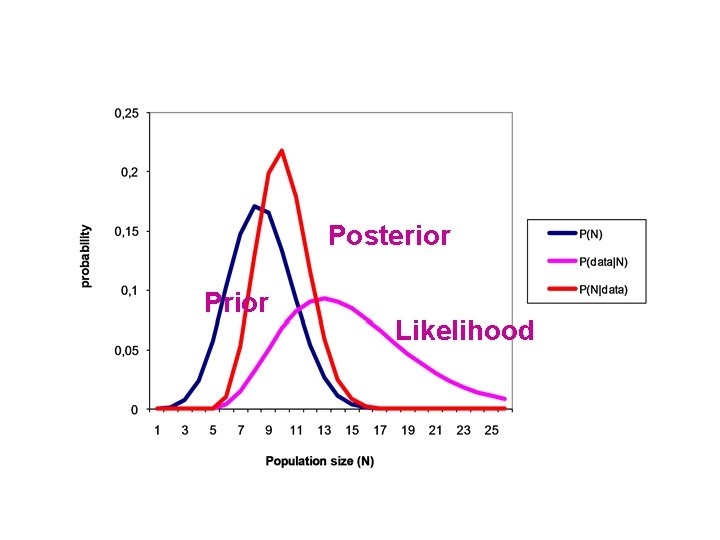
Posterior Prior Likelihood
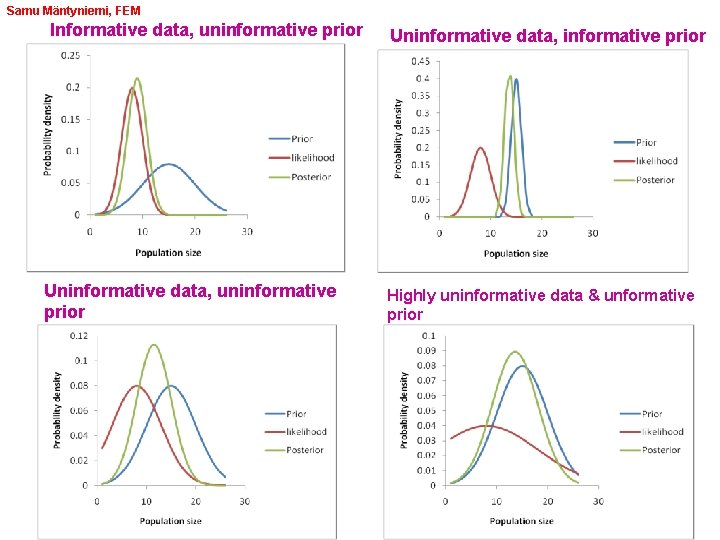
Samu Mäntyniemi, FEM Informative data, uninformative prior Uninformative data, informative prior Highly uninformative data & unformative prior
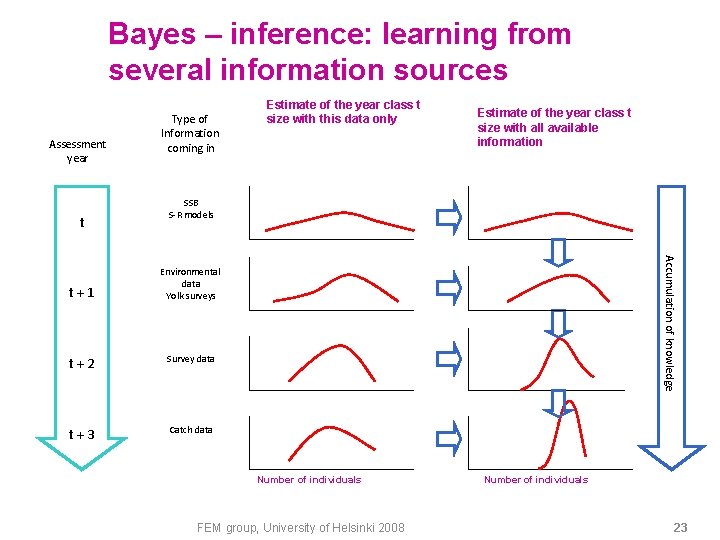
Bayes – inference: learning from several information sources Assessment year t Estimate of the year class t size with this data only Estimate of the year class t size with all available information SSB S-R models Accumulation of knowledge t+1 Type of Information coming in Environmental data Yolk surveys t+2 Survey data t+3 Catch data Number of individuals FEM group, University of Helsinki 2008 Number of individuals 23
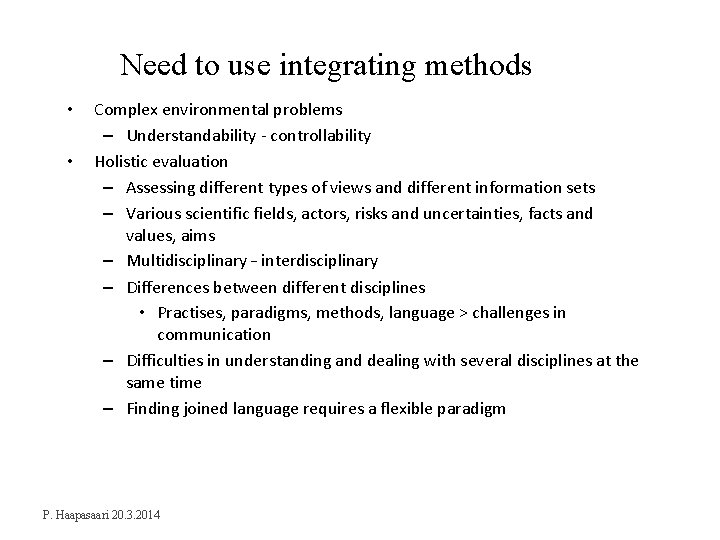
Need to use integrating methods • • Complex environmental problems – Understandability - controllability Holistic evaluation – Assessing different types of views and different information sets – Various scientific fields, actors, risks and uncertainties, facts and values, aims – Multidisciplinary – interdisciplinary – Differences between different disciplines • Practises, paradigms, methods, language > challenges in communication – Difficulties in understanding and dealing with several disciplines at the same time – Finding joined language requires a flexible paradigm P. Haapasaari 20. 3. 2014
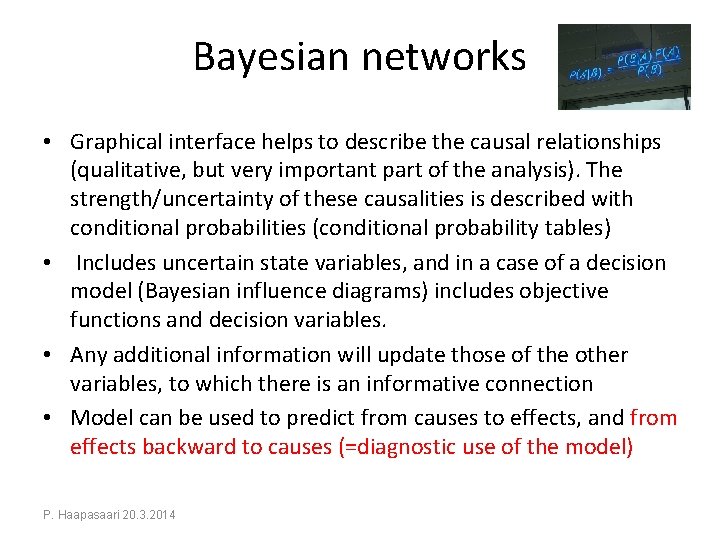
Bayesian networks • Graphical interface helps to describe the causal relationships (qualitative, but very important part of the analysis). The strength/uncertainty of these causalities is described with conditional probabilities (conditional probability tables) • Includes uncertain state variables, and in a case of a decision model (Bayesian influence diagrams) includes objective functions and decision variables. • Any additional information will update those of the other variables, to which there is an informative connection • Model can be used to predict from causes to effects, and from effects backward to causes (=diagnostic use of the model) P. Haapasaari 20. 3. 2014
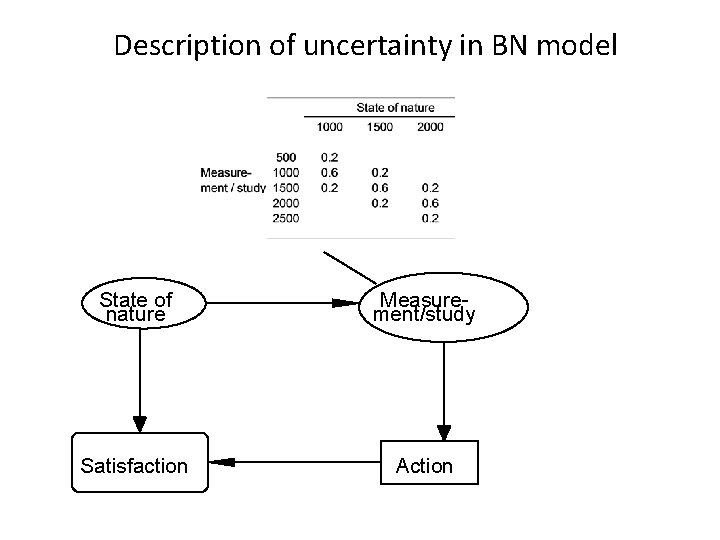
Description of uncertainty in BN model State of nature Measurement/study Satisfaction Action
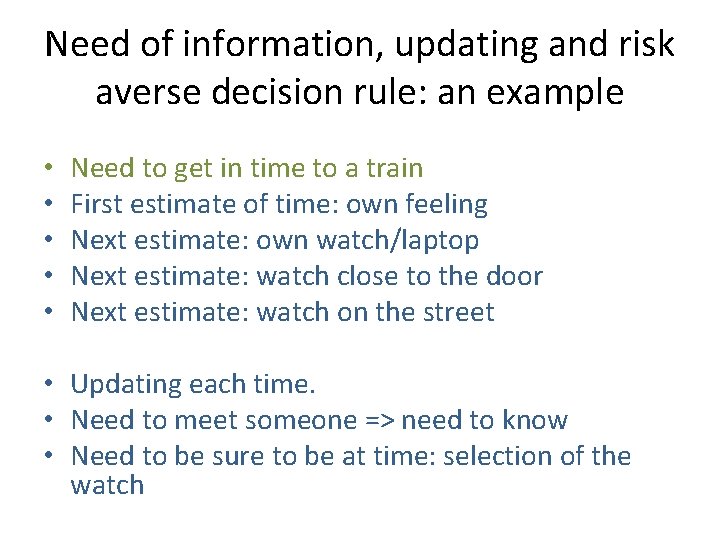
Need of information, updating and risk averse decision rule: an example • • • Need to get in time to a train First estimate of time: own feeling Next estimate: own watch/laptop Next estimate: watch close to the door Next estimate: watch on the street • Updating each time. • Need to meet someone => need to know • Need to be sure to be at time: selection of the watch
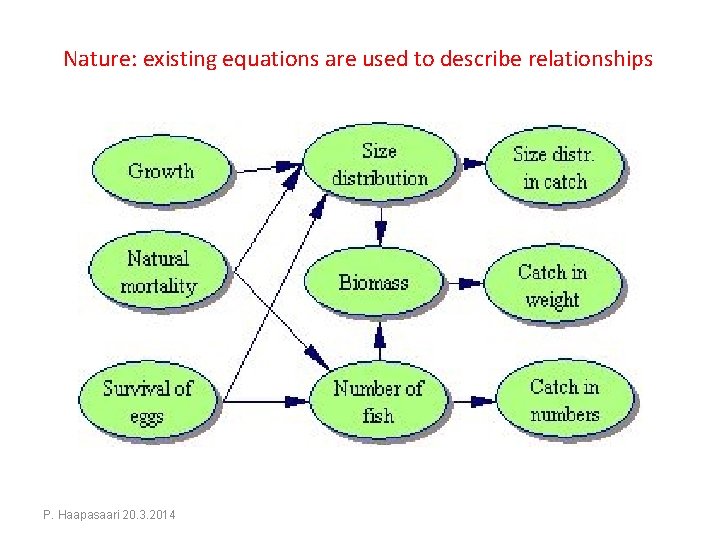
Nature: existing equations are used to describe relationships P. Haapasaari 20. 3. 2014
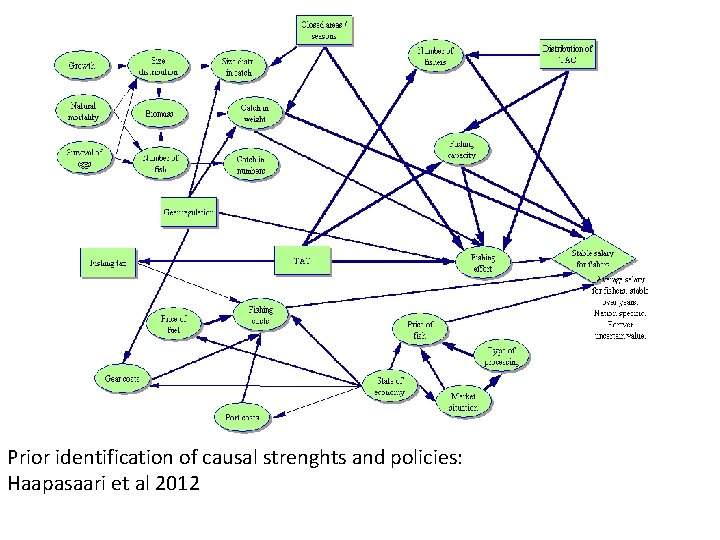
Prior identification of causal strenghts and policies: Haapasaari et al 2012
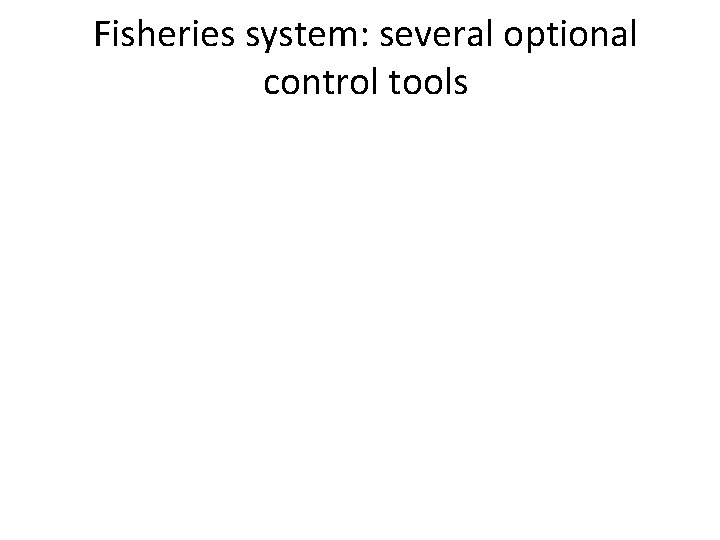
Fisheries system: several optional control tools
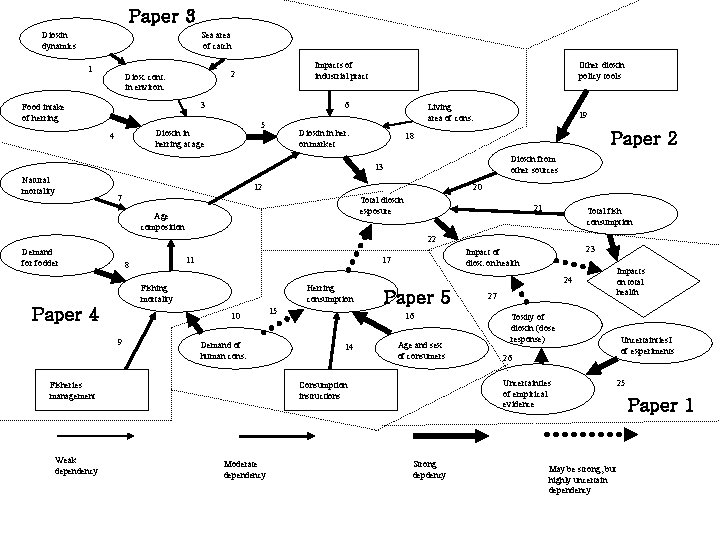
Paper 3 Dioxin dynamics Sea area of catch 1 Impacts of industrial pract 2 Diox. cont. in environ. 3 Food intake of herring 6 5 Dioxin in herring at age 4 Other dioxin policy tools Living area of cons. Dioxin in her. on market 19 Paper 2 18 Dioxin from other sources 13 Natural mortality 12 20 7 Total dioxin exposure Age composition 21 Total fish consumption 22 Demand for fodder 11 8 Fishing mortality Paper 4 Herring consumption 10 9 Demand of human cons. Fisheries management Weak dependency 15 24 Paper 5 16 14 Age and sex of consumers 27 Toxity of dioxin (dose response) 26 Uncertainties of empirical evidence Consumption instructions Moderate dependency 23 Impact of diox. on health 17 Strong depdency May be strong, but highly uncertain dependency Impacts on total health Uncertainties. I of experiments 25 Paper 1
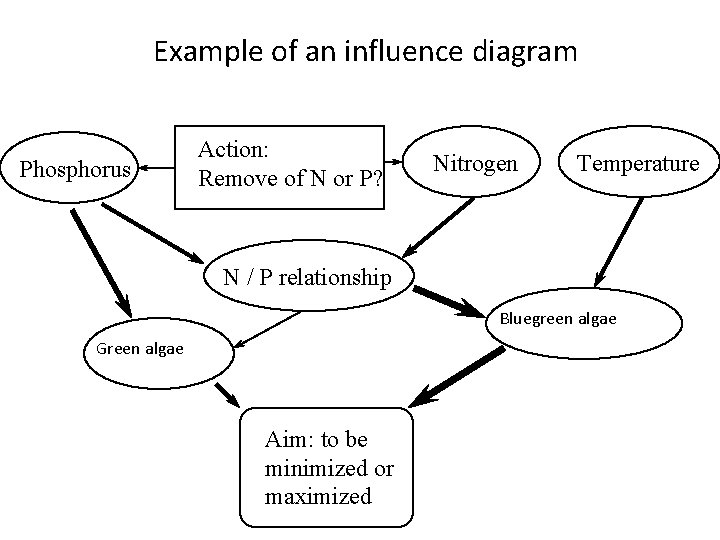
Example of an influence diagram Phosphorus Action: Remove of N or P? Nitrogen Temperature N / P relationship Bluegreen algae Green algae Aim: to be minimized or maximized
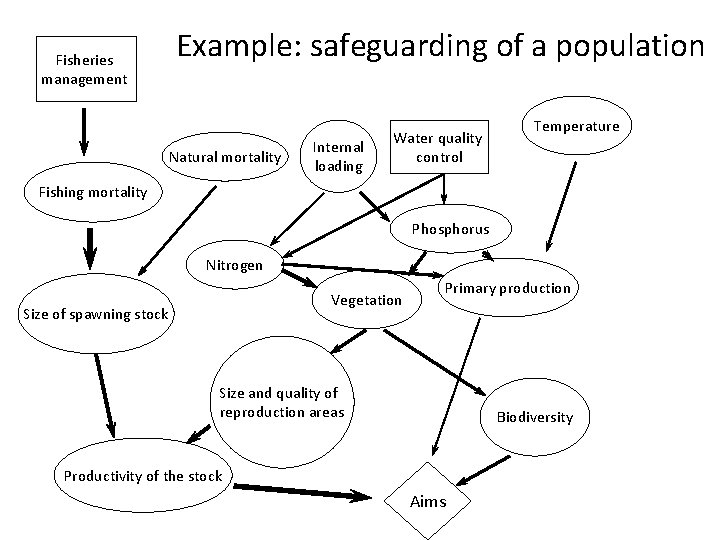
Fisheries management Example: safeguarding of a population Natural mortality Internal loading Water quality control Temperature Fishing mortality Phosphorus Nitrogen Vegetation Size of spawning stock Primary production Size and quality of reproduction areas Biodiversity Productivity of the stock Aims
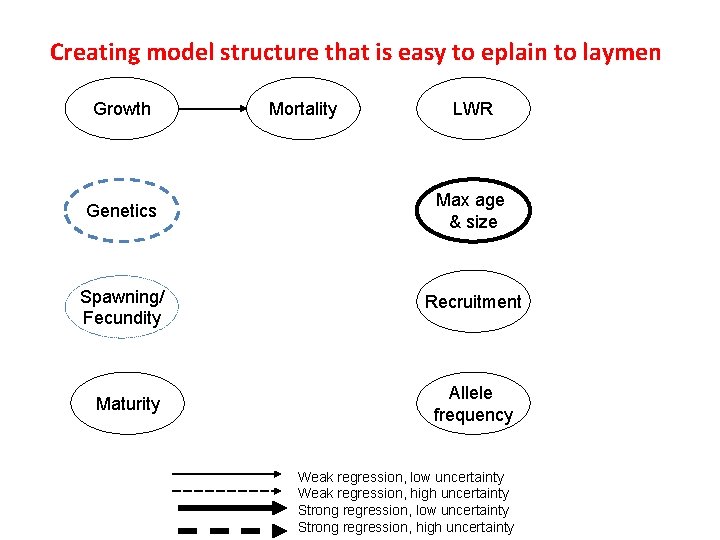
Creating model structure that is easy to eplain to laymen Growth Mortality LWR Genetics Max age & size Spawning/ Fecundity Recruitment Maturity Allele frequency Weak regression, low uncertainty Weak regression, high uncertainty Strong regression, low uncertainty Strong regression, high uncertainty
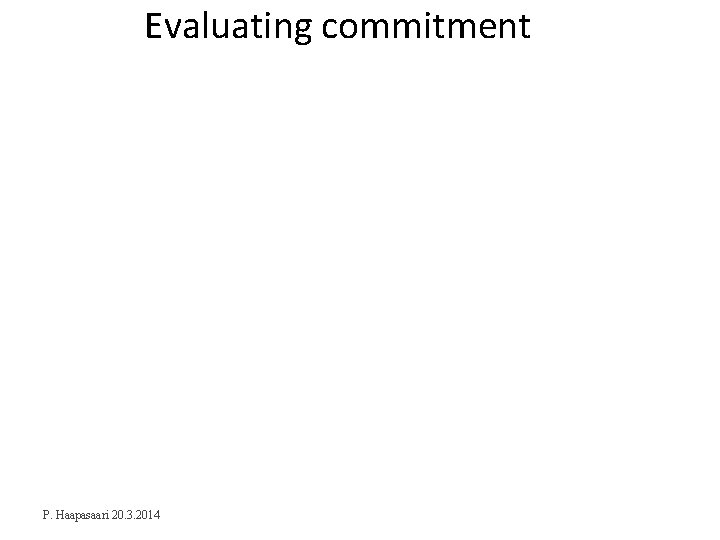
Evaluating commitment P. Haapasaari 20. 3. 2014
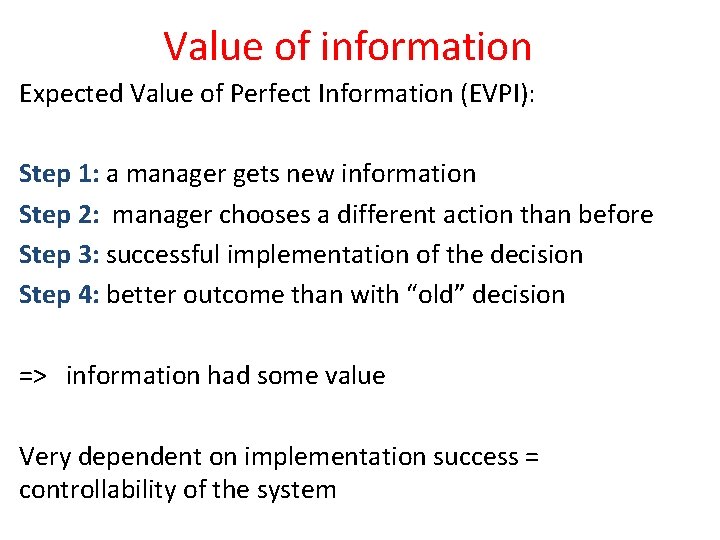
Value of information Expected Value of Perfect Information (EVPI): Step 1: a manager gets new information Step 2: manager chooses a different action than before Step 3: successful implementation of the decision Step 4: better outcome than with “old” decision => information had some value Very dependent on implementation success = controllability of the system
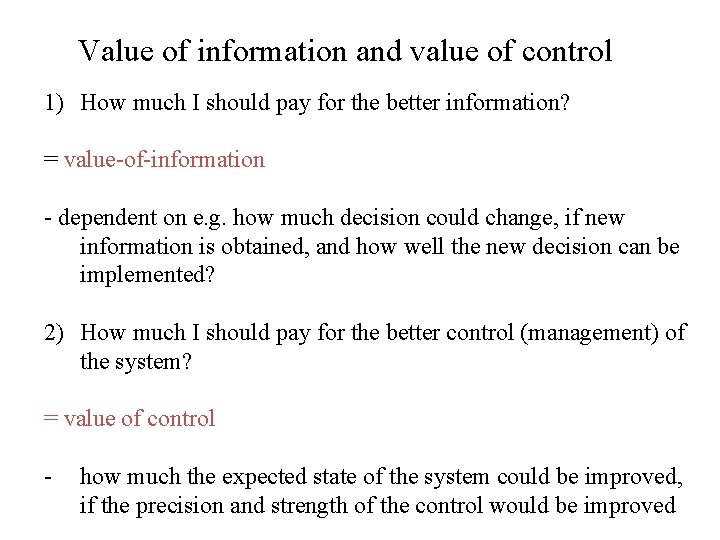
Value of information and value of control 1) How much I should pay for the better information? = value-of-information - dependent on e. g. how much decision could change, if new information is obtained, and how well the new decision can be implemented? 2) How much I should pay for the better control (management) of the system? = value of control - how much the expected state of the system could be improved, if the precision and strength of the control would be improved
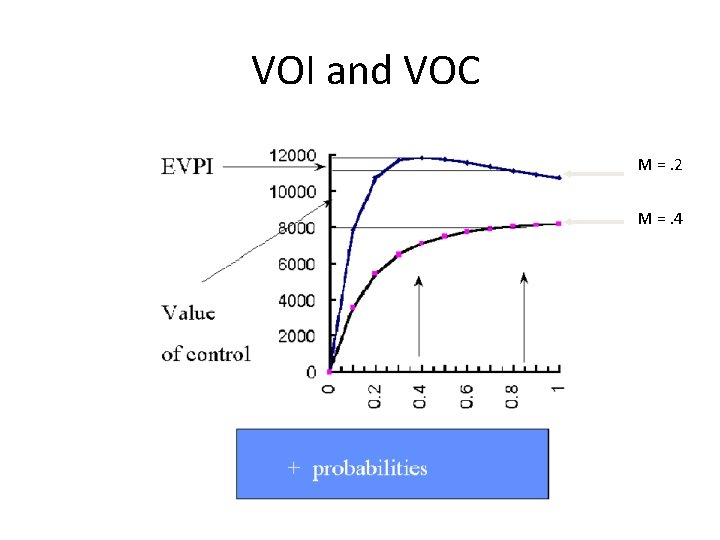
VOI and VOC M =. 2 M =. 4
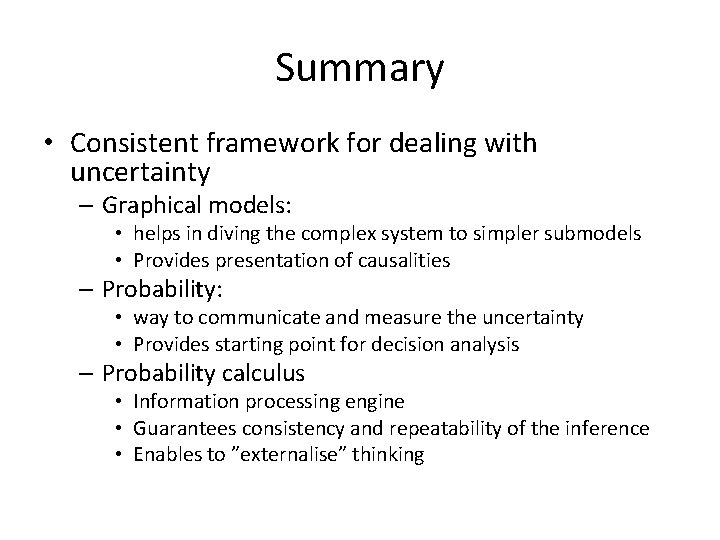
Summary • Consistent framework for dealing with uncertainty – Graphical models: • helps in diving the complex system to simpler submodels • Provides presentation of causalities – Probability: • way to communicate and measure the uncertainty • Provides starting point for decision analysis – Probability calculus • Information processing engine • Guarantees consistency and repeatability of the inference • Enables to ”externalise” thinking
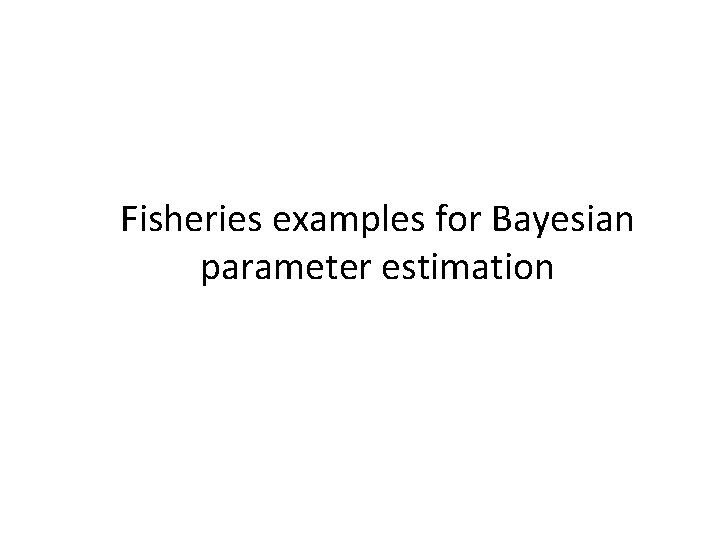
Fisheries examples for Bayesian parameter estimation
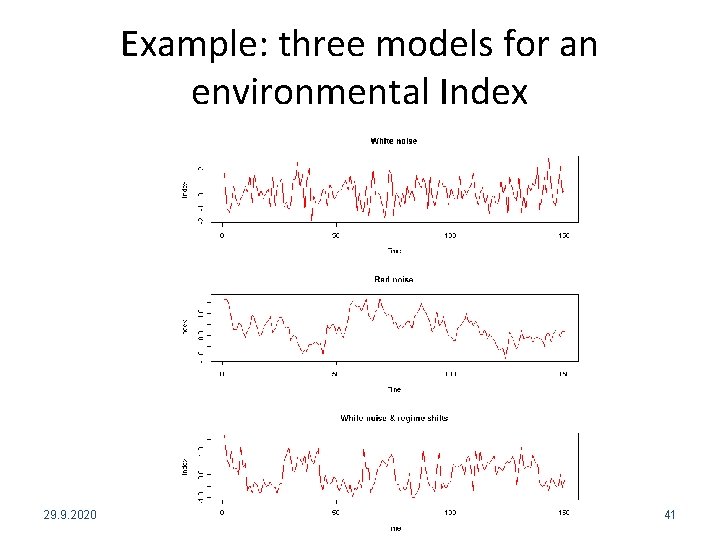
Example: three models for an environmental Index 29. 9. 2020 Biotieteellinen tiedekunta / Henkilön nimi / Esityksen nimi 41
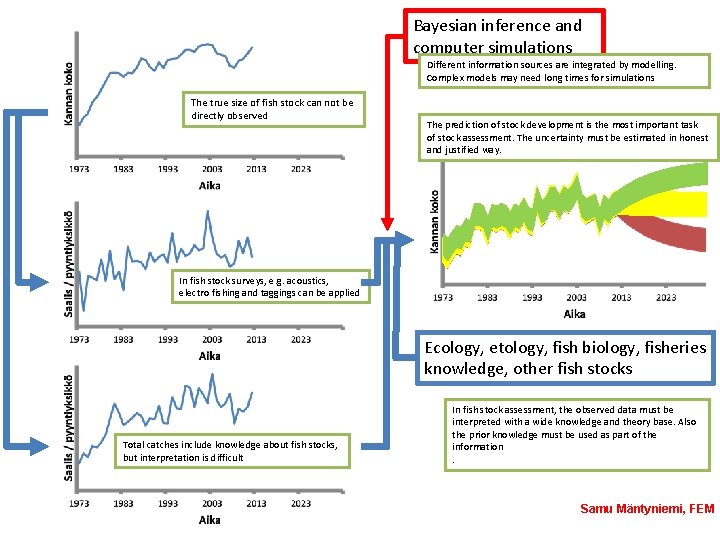
Bayesian inference and computer simulations Different information sources are integrated by modelling. Complex models may need long times for simulations The true size of fish stock can not be directly observed The prediction of stock development is the most important task of stock assessment. The uncertainty must be estimated in honest and justified way. In fish stock surveys, e. g. acoustics, electro fishing and taggings can be applied Ecology, etology, fish biology, fisheries knowledge, other fish stocks Total catches include knowledge about fish stocks, but interpretation is difficult In fish stock assessment, the observed data must be interpreted with a wide knowledge and theory base. Also the prior knowledge must be used as part of the information. Samu Mäntyniemi, FEM
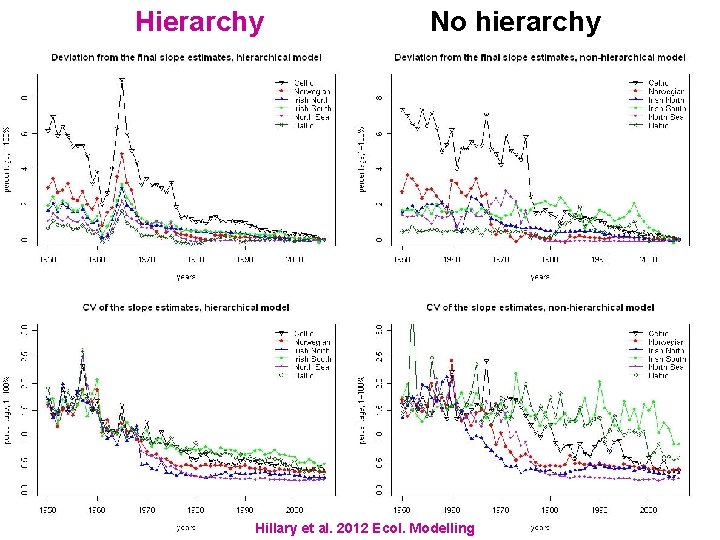
Hierarchical Hierarchy Bayesian model No No hierarchy Hillary et al. 2012 Ecol. Modelling
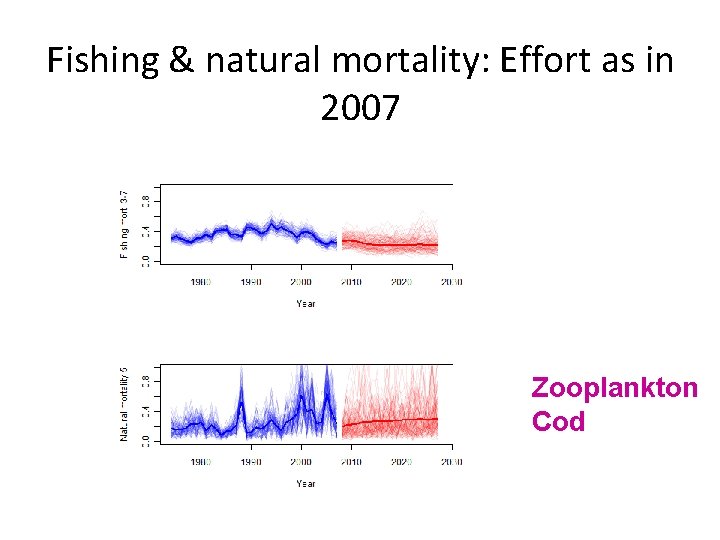
Fishing & natural mortality: Effort as in 2007 Zooplankton Cod
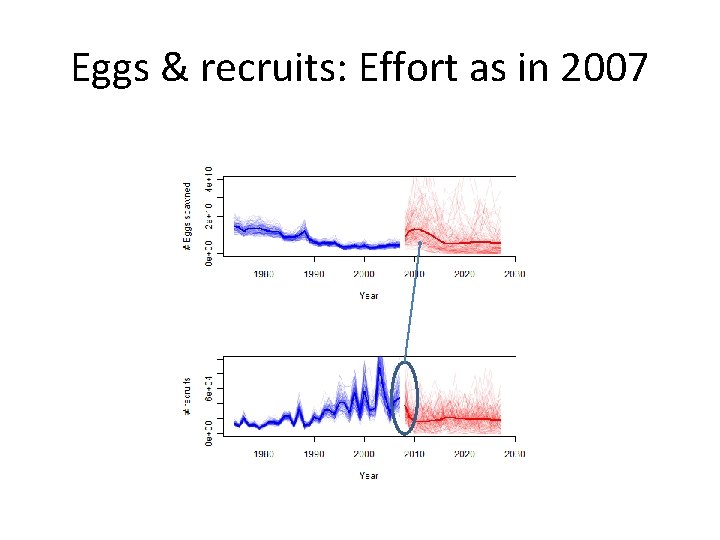
Eggs & recruits: Effort as in 2007
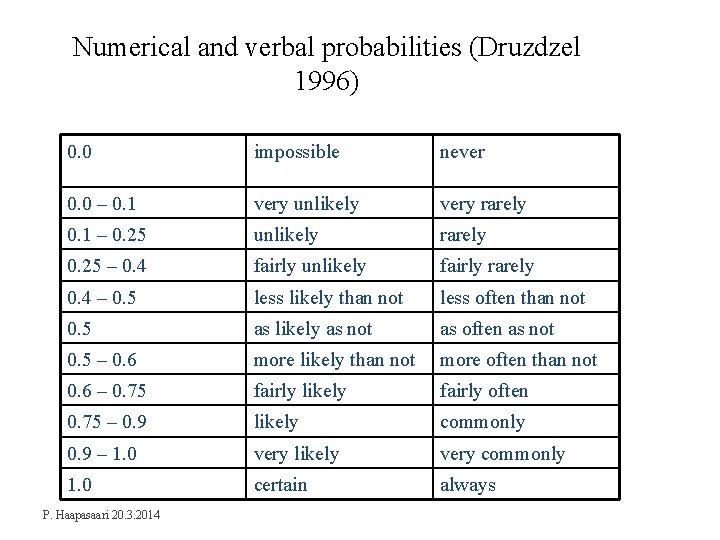
Numerical and verbal probabilities (Druzdzel 1996) 0. 0 impossible never 0. 0 – 0. 1 very unlikely very rarely 0. 1 – 0. 25 unlikely rarely 0. 25 – 0. 4 fairly unlikely fairly rarely 0. 4 – 0. 5 less likely than not less often than not 0. 5 as likely as not as often as not 0. 5 – 0. 6 more likely than not more often than not 0. 6 – 0. 75 fairly likely fairly often 0. 75 – 0. 9 likely commonly 0. 9 – 1. 0 very likely very commonly 1. 0 certain always P. Haapasaari 20. 3. 2014
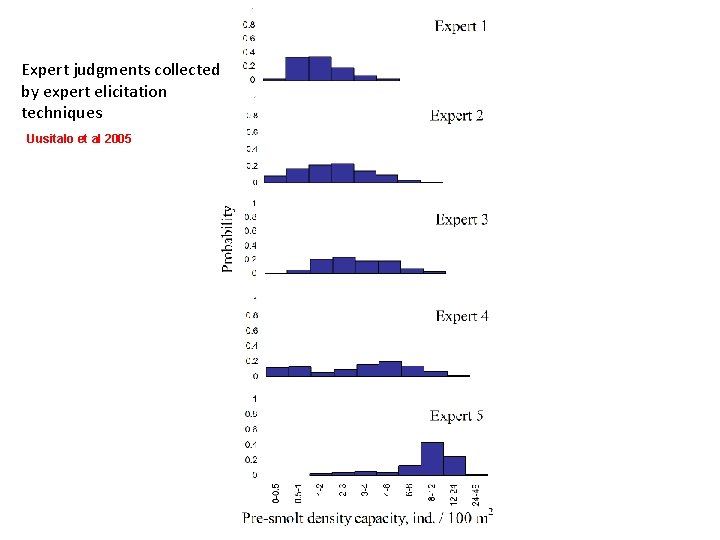
Expert judgments collected by expert elicitation techniques Uusitalo et al 2005
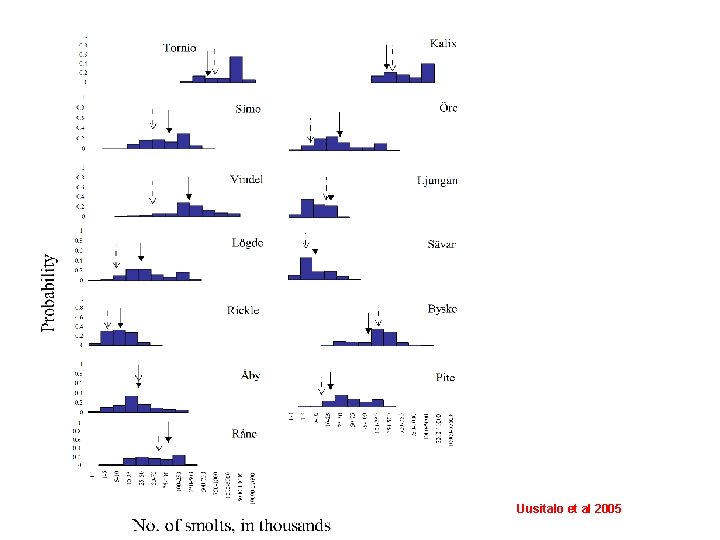
Uusitalo et al 2005
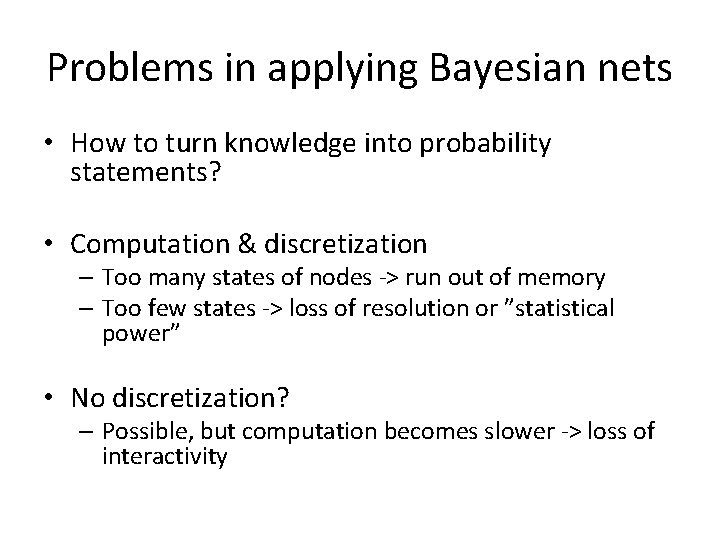
Problems in applying Bayesian nets • How to turn knowledge into probability statements? • Computation & discretization – Too many states of nodes -> run out of memory – Too few states -> loss of resolution or ”statistical power” • No discretization? – Possible, but computation becomes slower -> loss of interactivity
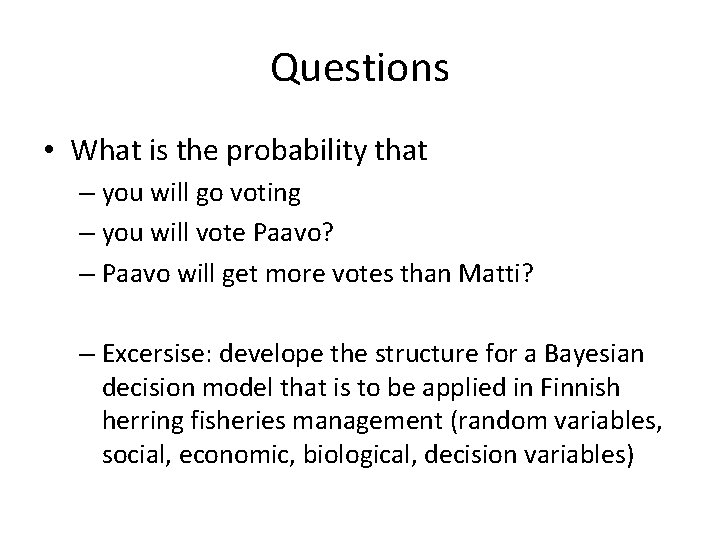
Questions • What is the probability that – you will go voting – you will vote Paavo? – Paavo will get more votes than Matti? – Excersise: develope the structure for a Bayesian decision model that is to be applied in Finnish herring fisheries management (random variables, social, economic, biological, decision variables)
Ecgs
Nom preeclampsia
017
Fisheries management
Market risk assessment
Talk, read talk write template
Amateurs talk strategy professionals talk logistics
Problem talk vs solution talk
Key risk indicators financial risk management
Risk map risk management
Mifco logo
Frafra v boakye
Certified fisheries professional
Southeast fisheries science center
сплит institute of oceanography and fisheries
Small-scale fisheries
Dg fisheries
Tea directorate
Northwest fisheries
Classification of fisheries
Institute of oceanography and fisheries
Ocean fisheries
Pearson prentice hall
Microscopic algae
Alaska fisheries development foundation
General fisheries commission for the mediterranean
National fisheries service
Ministry of food agriculture and fisheries denmark
Allingham v minister of agriculture and fisheries
Boat security system
Apex fisheries
Atlantic fisheries fund
Tea directorate kenya
Hazard identification card
Risk projection attempts to rate each risk in two ways
Risk management avoidance
Relative risk calculation
Residual risk and secondary risk pmp
Inherent risk vs control risk
Absolute risk vs relative risk
Smg stock split
Risk classification system
Pembiayaan risiko (risk financing)
The biggest risk is not taking any risk
Business risk audit
Business risk and financial risk leverage
Relative risk and attributable risk
Measure of association formula
Toolbox talk fatigue
Waste management toolbox talk
Pyramid levels of management