Direct and Indirect Matching of Schema Elements for
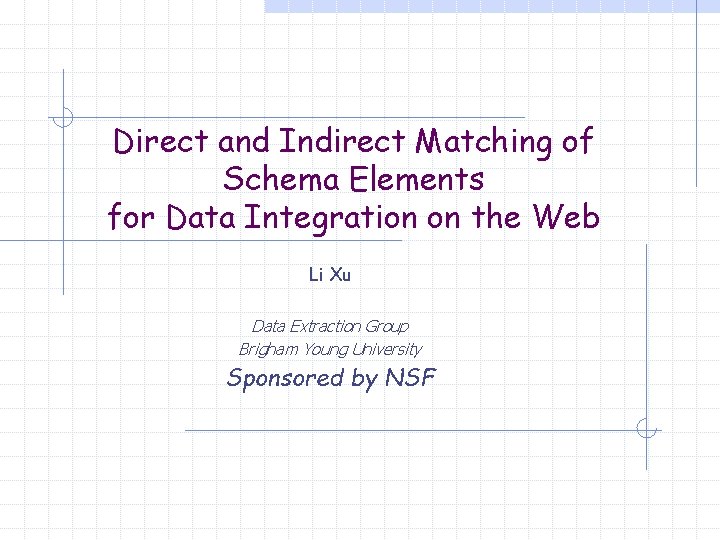
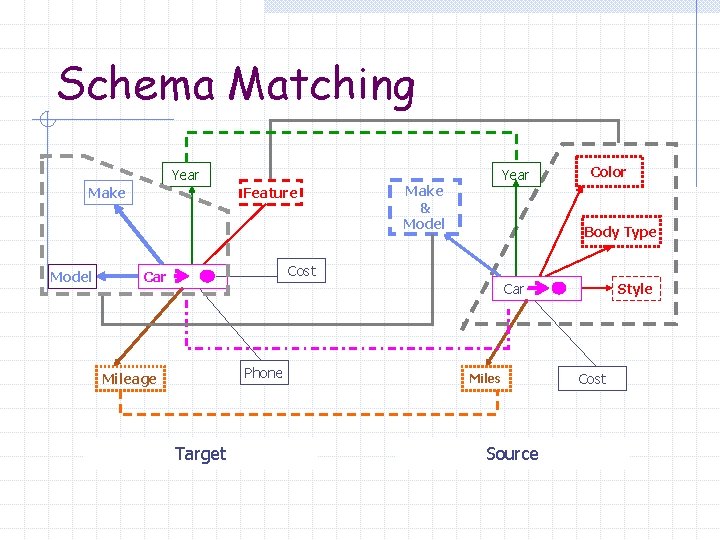
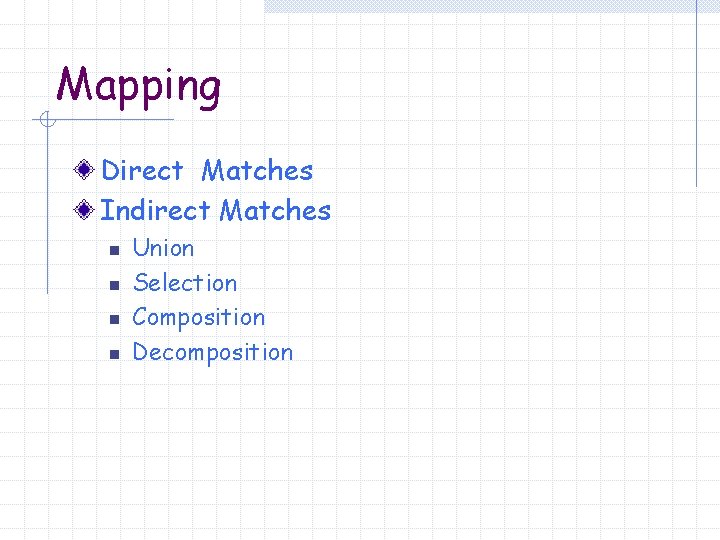
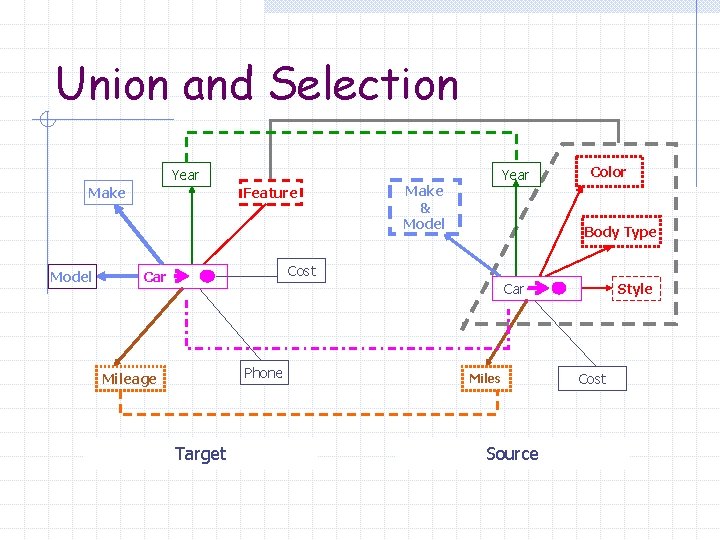
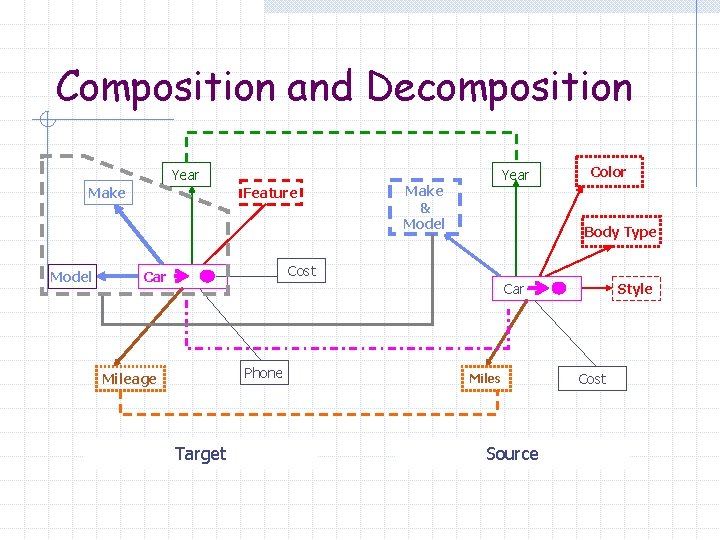
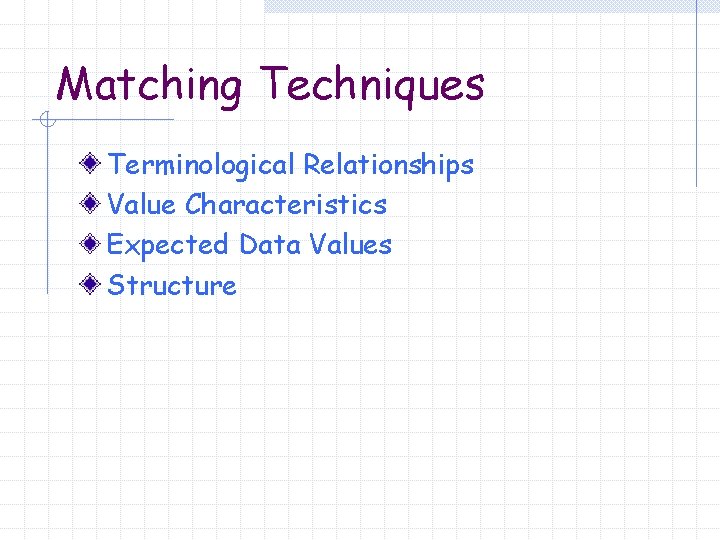
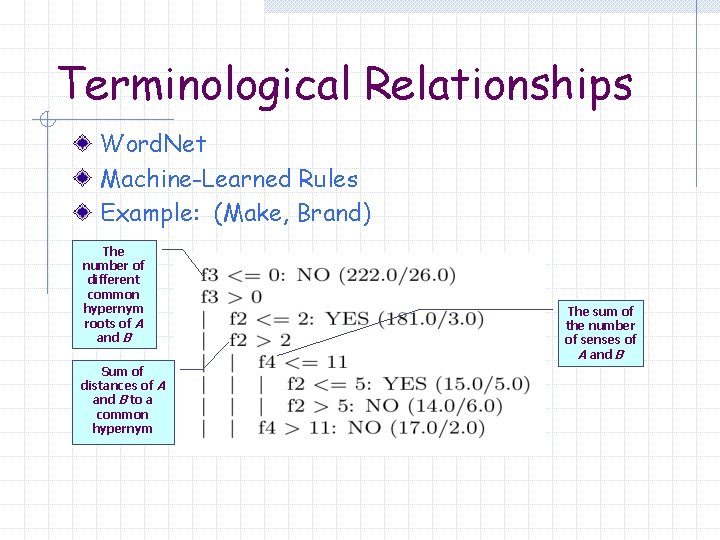
![Value Characteristics n Machine Learning n Features [LC 94] n String length, numeric ratio, Value Characteristics n Machine Learning n Features [LC 94] n String length, numeric ratio,](https://slidetodoc.com/presentation_image_h/21fbab55fff0564d3d402e4fbe42766a/image-8.jpg)
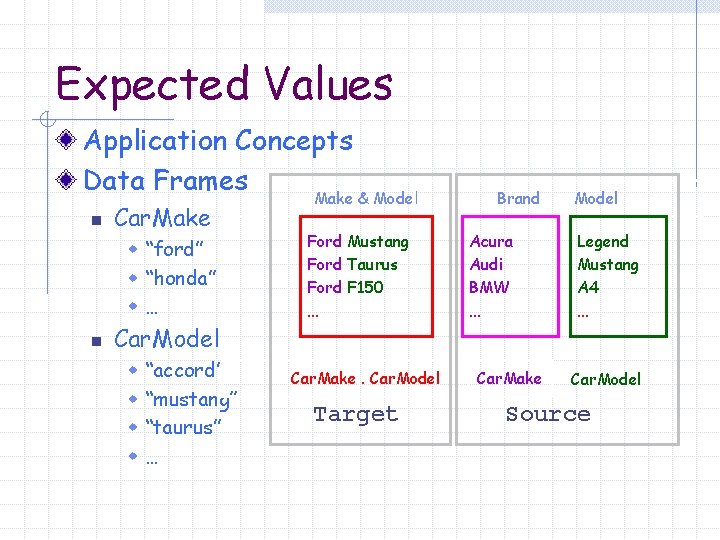
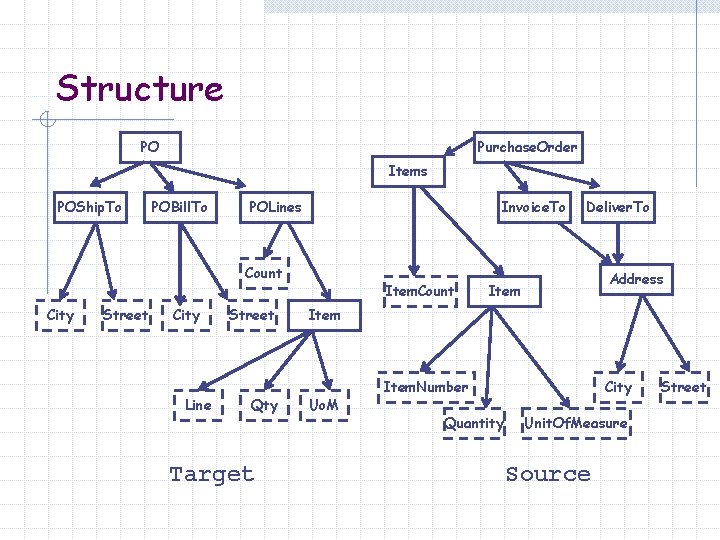
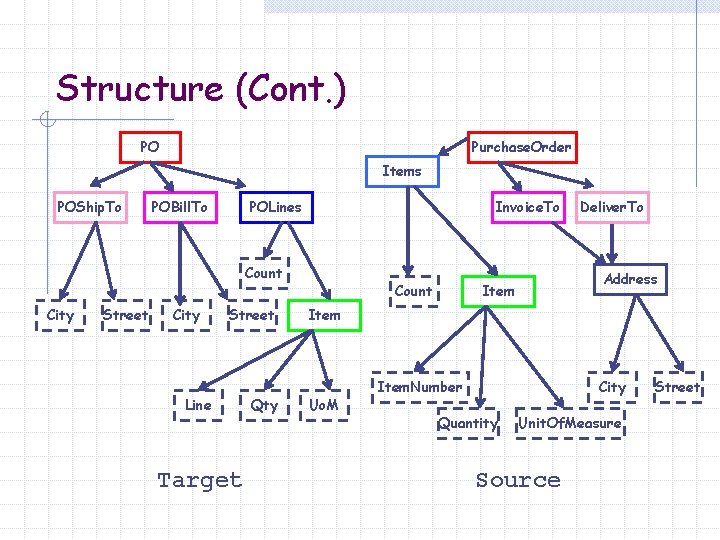
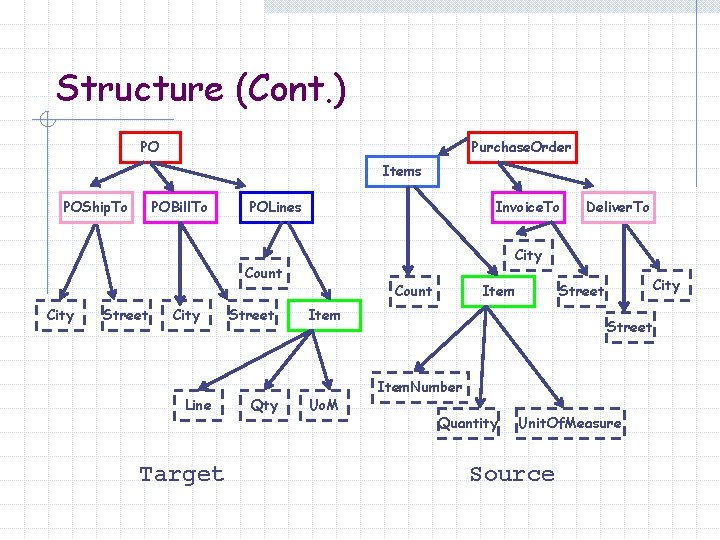
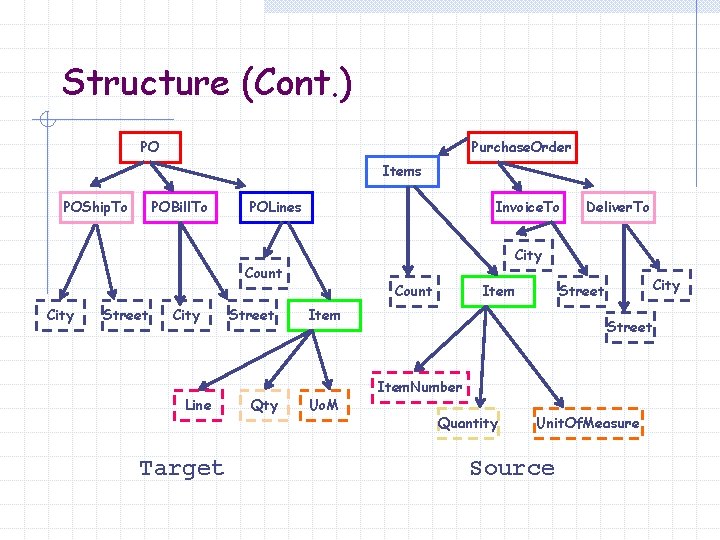
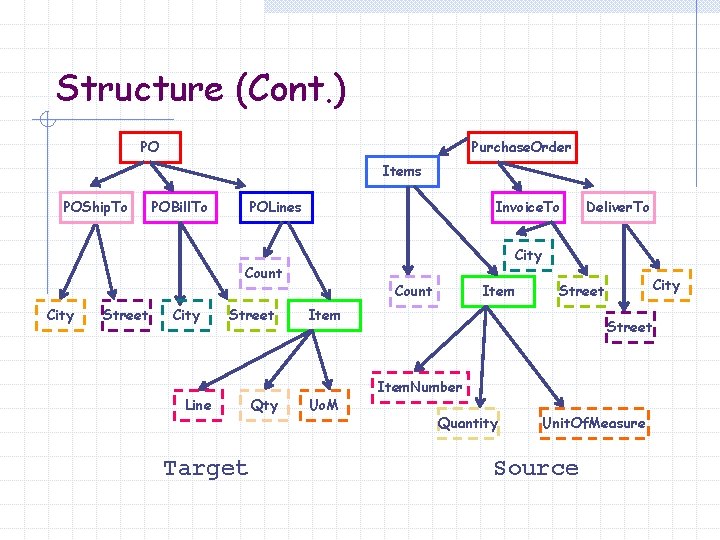
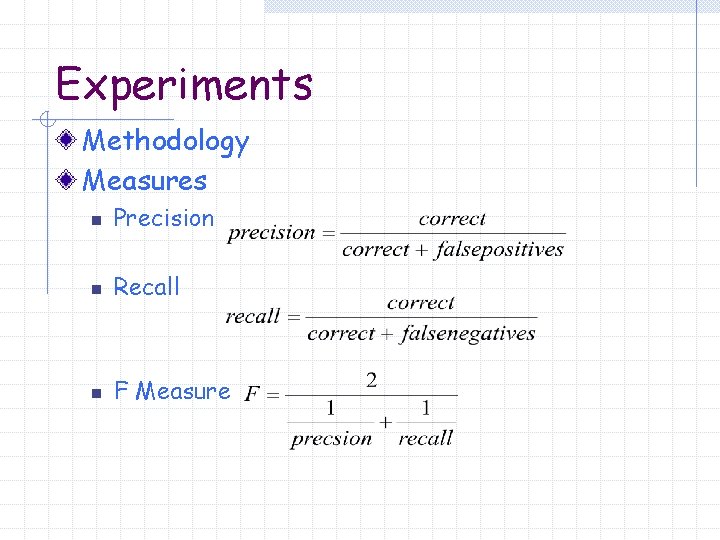
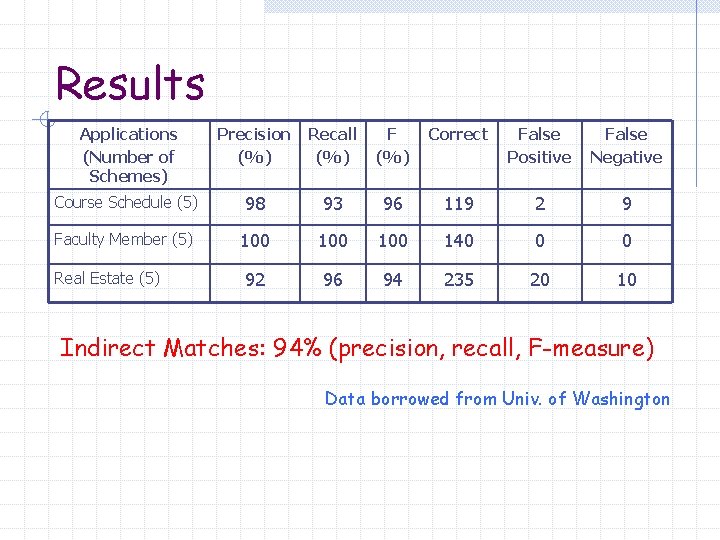
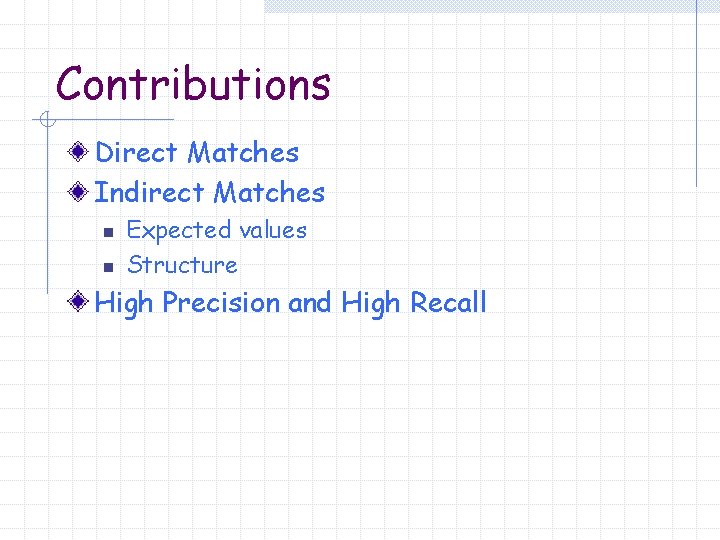
- Slides: 17
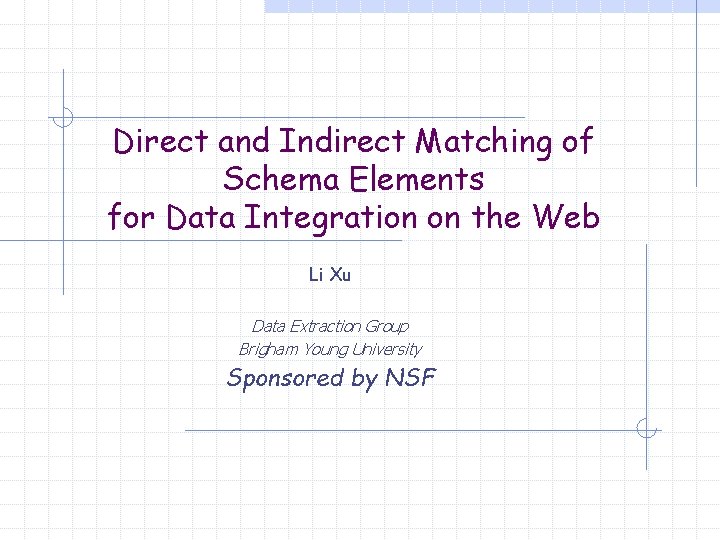
Direct and Indirect Matching of Schema Elements for Data Integration on the Web Li Xu Data Extraction Group Brigham Young University Sponsored by NSF
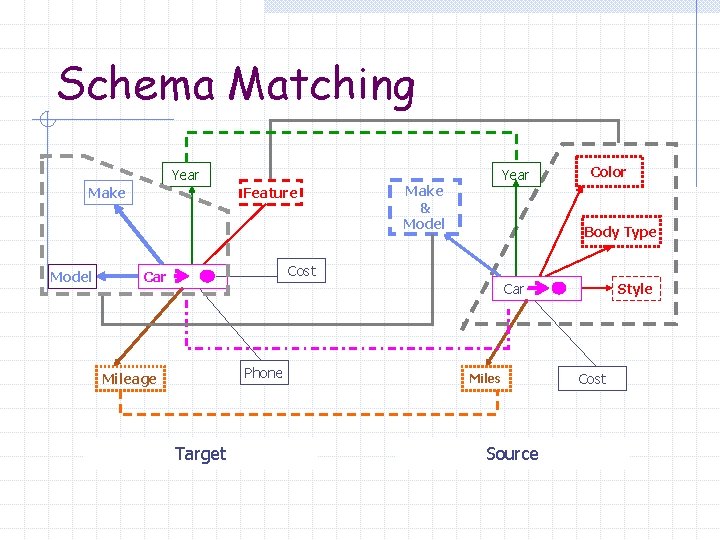
Schema Matching Year Make Model Feature Year Make & Model Color Body Type Cost Car Style Car Phone Mileage Target Miles Source Cost
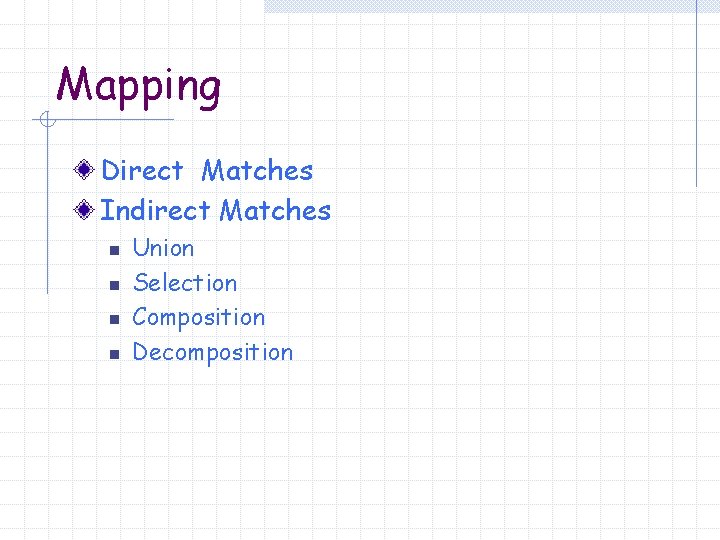
Mapping Direct Matches Indirect Matches n n Union Selection Composition Decomposition
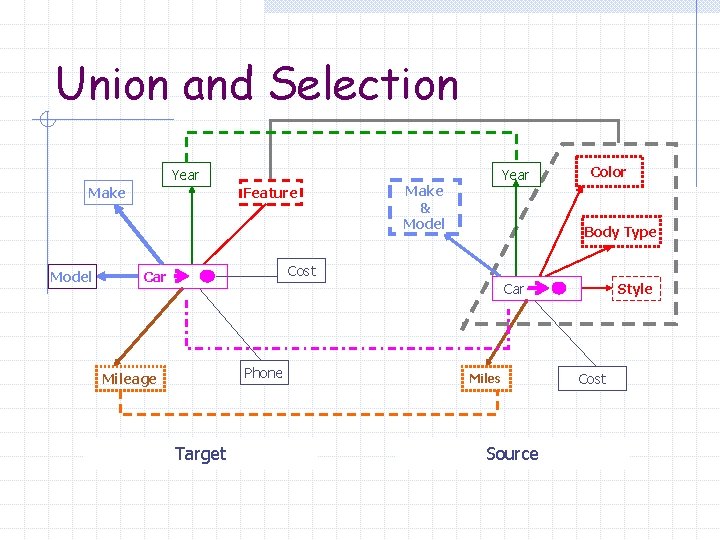
Union and Selection Year Make Model Feature Year Make & Model Color Body Type Cost Car Style Car Phone Mileage Target Miles Source Cost
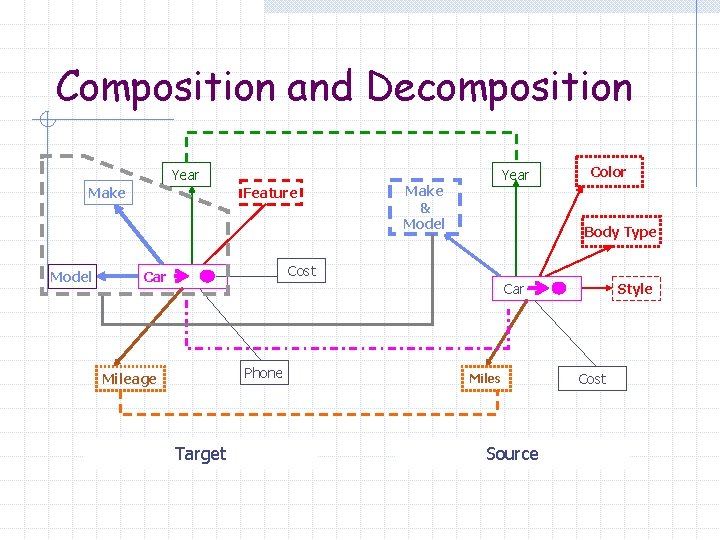
Composition and Decomposition Year Make Model Feature Year Make & Model Color Body Type Cost Car Style Car Phone Mileage Target Miles Source Cost
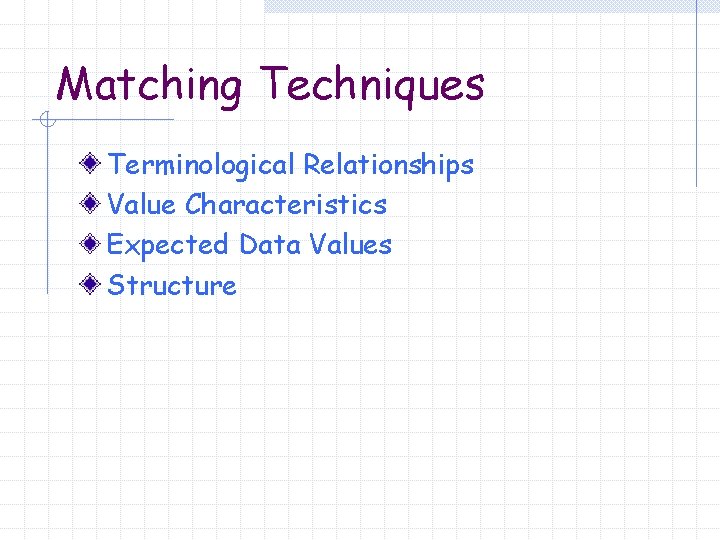
Matching Techniques Terminological Relationships Value Characteristics Expected Data Values Structure
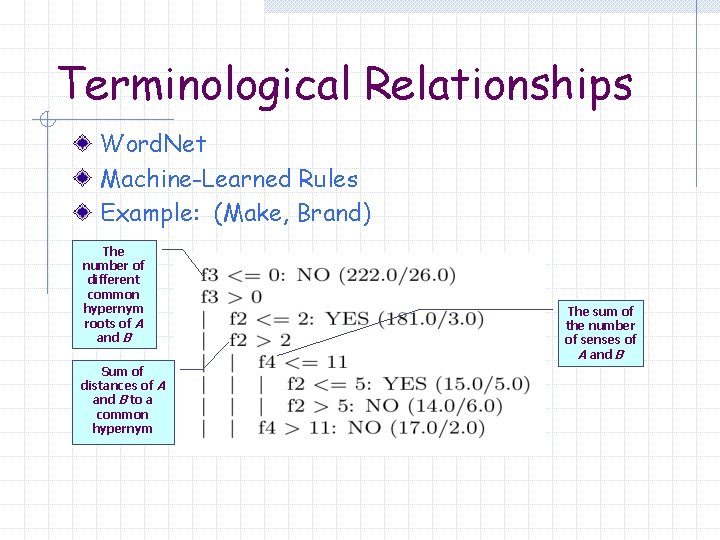
Terminological Relationships Word. Net Machine-Learned Rules Example: (Make, Brand) The number of different common hypernym roots of A and B Sum of distances of A and B to a common hypernym The sum of the number of senses of A and B
![Value Characteristics n Machine Learning n Features LC 94 n String length numeric ratio Value Characteristics n Machine Learning n Features [LC 94] n String length, numeric ratio,](https://slidetodoc.com/presentation_image_h/21fbab55fff0564d3d402e4fbe42766a/image-8.jpg)
Value Characteristics n Machine Learning n Features [LC 94] n String length, numeric ratio, space ratio. Mean, variation, coefficient variation, standard deviation; n
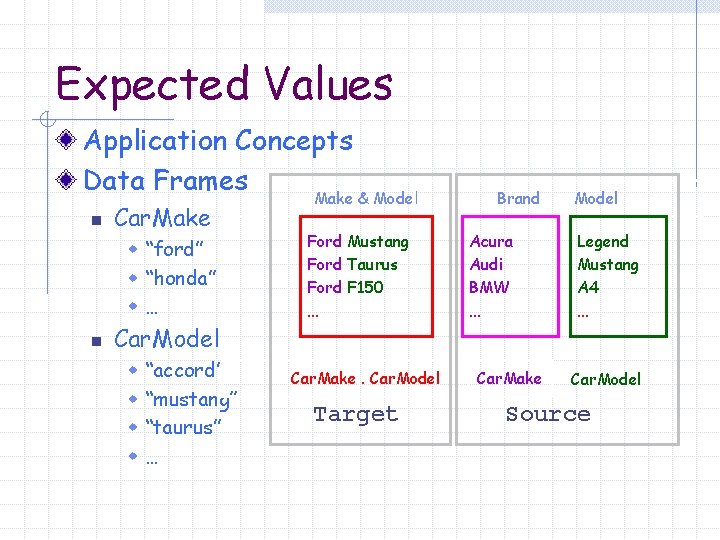
Expected Values Application Concepts Data Frames Make & Model n Car. Make w “ford” w “honda” w… n Car. Model w w “accord” “mustang” “taurus” … Ford Mustang Ford Taurus Ford F 150 … Car. Make. Car. Model Target Brand Acura Audi BMW … Car. Make Model Legend Mustang A 4 … Car. Model Source
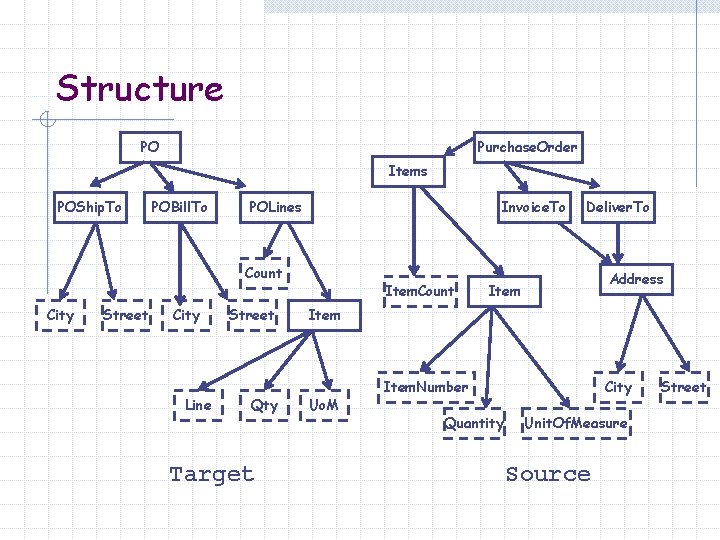
Structure PO Purchase. Order Items POShip. To POBill. To POLines Invoice. To Count City Street City Line Street Qty Target Item. Count Deliver. To Address Item Uo. M Item. Number Quantity City Unit. Of. Measure Source Street
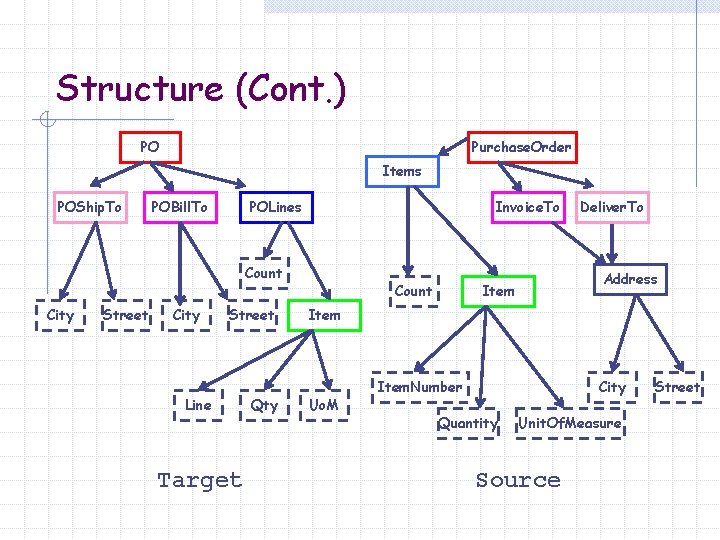
Structure (Cont. ) PO Purchase. Order Items POShip. To POBill. To POLines Invoice. To Count City Street Line Target Qty Count Deliver. To Address Item Uo. M Item. Number City Quantity Unit. Of. Measure Source Street
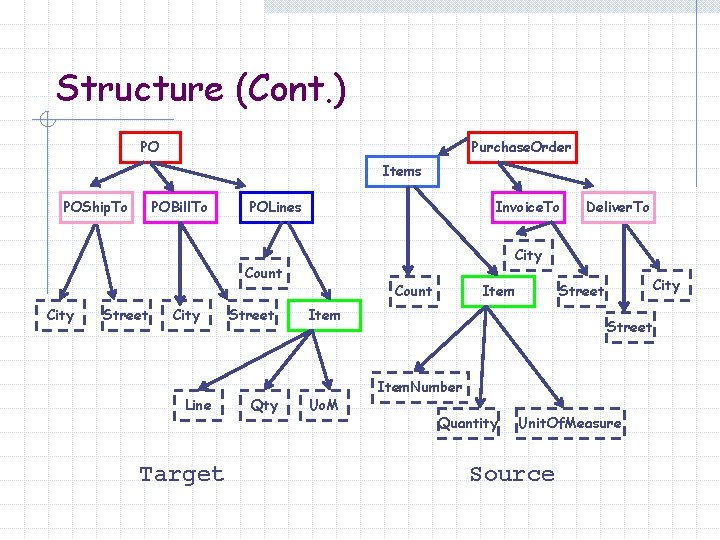
Structure (Cont. ) PO Purchase. Order Items POShip. To POBill. To POLines Invoice. To City Count City Street City Line Target Street Qty Deliver. To Count Item Uo. M City Street Item. Number Quantity Unit. Of. Measure Source
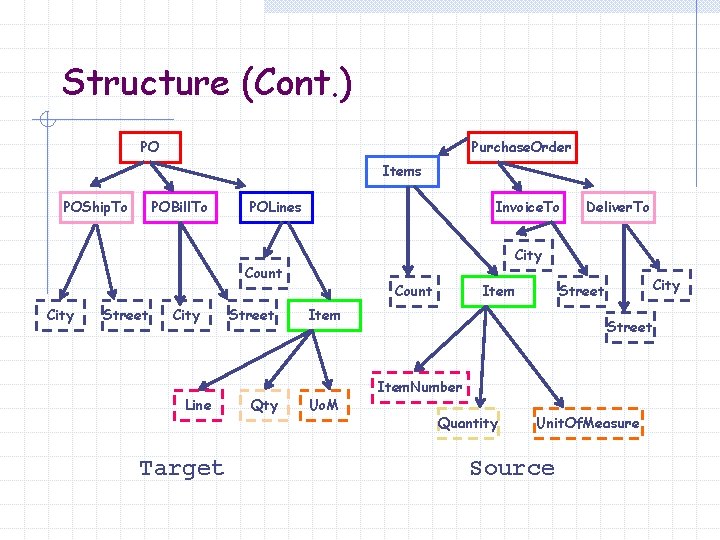
Structure (Cont. ) PO Purchase. Order Items POShip. To POBill. To POLines Invoice. To City Count City Street City Line Target Street Qty Deliver. To Count Item Uo. M City Street Item. Number Quantity Unit. Of. Measure Source
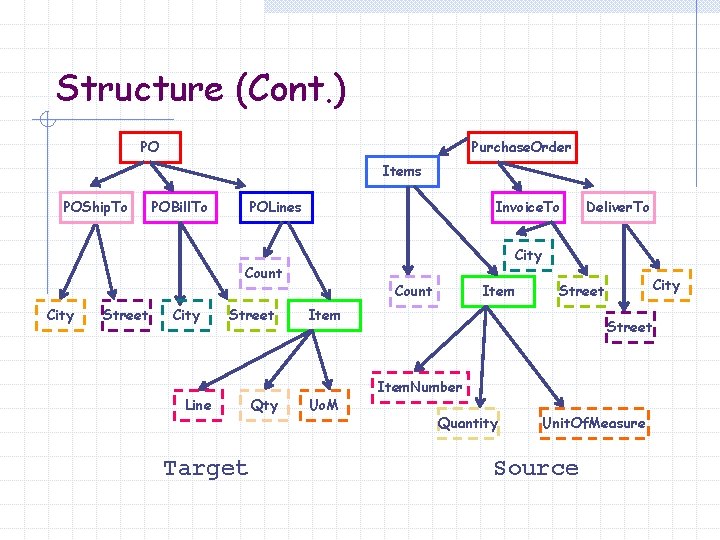
Structure (Cont. ) PO Purchase. Order Items POShip. To POBill. To POLines Invoice. To City Count City Street Line Target Qty Deliver. To Count Item Uo. M City Street Item. Number Quantity Unit. Of. Measure Source
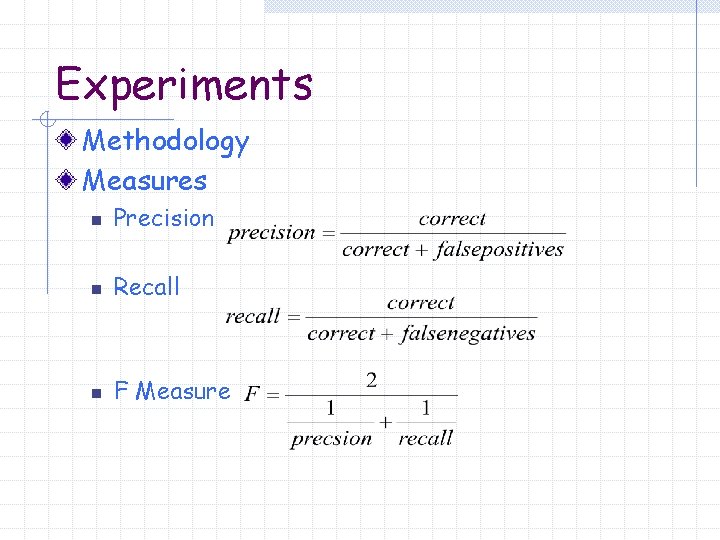
Experiments Methodology Measures n Precision n Recall n F Measure
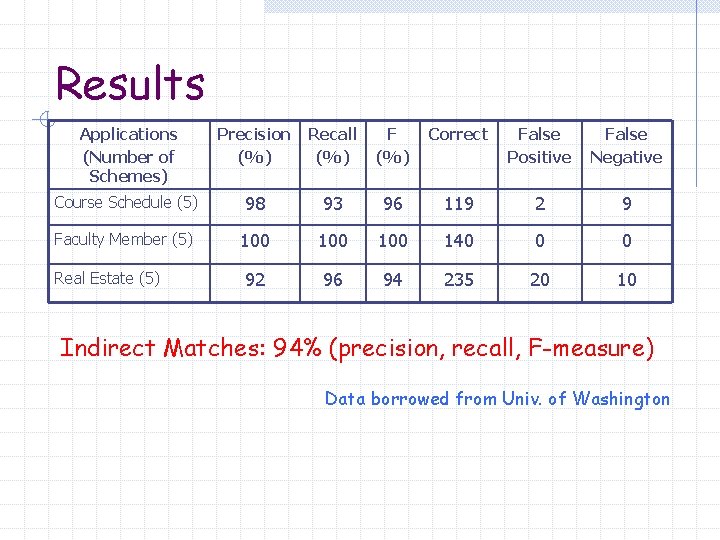
Results Applications (Number of Schemes) Precision (%) Recall (%) F (%) Correct False Positive False Negative Course Schedule (5) 98 93 96 119 2 9 Faculty Member (5) 100 100 140 0 0 92 96 94 235 20 10 Real Estate (5) Indirect Matches: 94% (precision, recall, F-measure) Data borrowed from Univ. of Washington
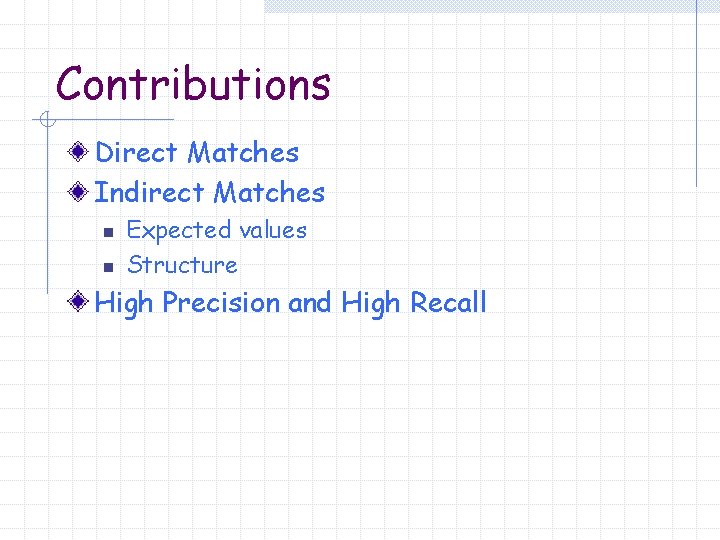
Contributions Direct Matches Indirect Matches n n Expected values Structure High Precision and High Recall
Jingles are musical messages written around the brand.
What is direct characterization
What is charactization
Direct proof and indirect proof
Direct and indirect band gap semiconductors
Indirect and direct characterization
Combined gas law direct or indirect
Explicit and implicit performatives
John mandy is at home
Imperative sentence direct speech
Direct and indirect measurement
Direct or indirect speech act
Direct observation
Direct and indirect observation examples
Whats a dangling modifier
Direct and indirect competitors examples
Direct proof and indirect proof
Learning outcomes of direct and indirect speech