Correlation Farrokh Alemi Ph D Kashif Haqqi M
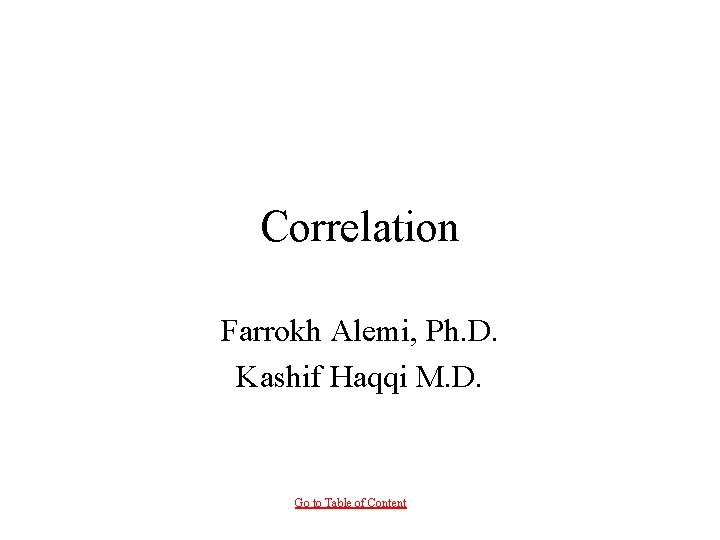
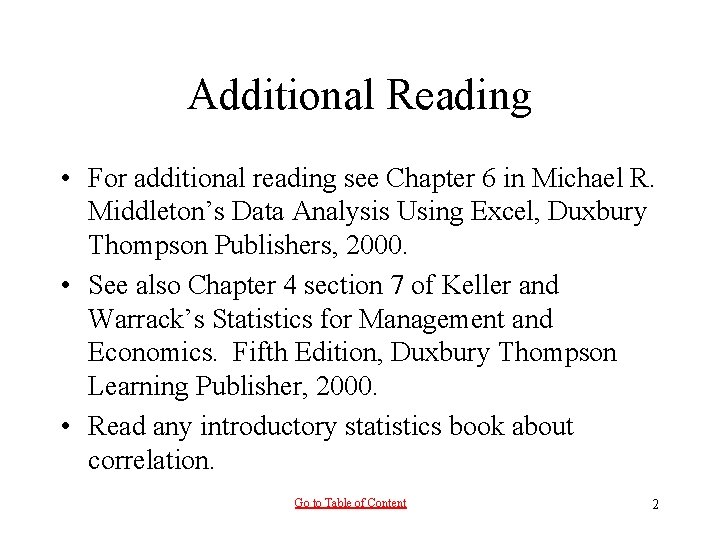
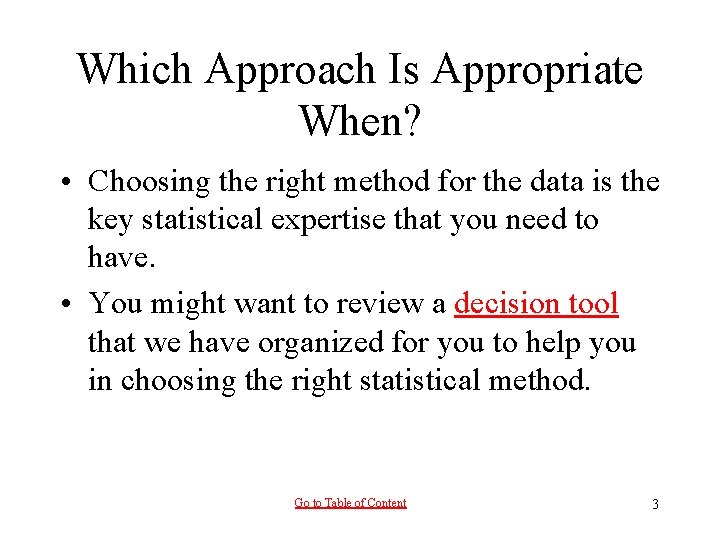
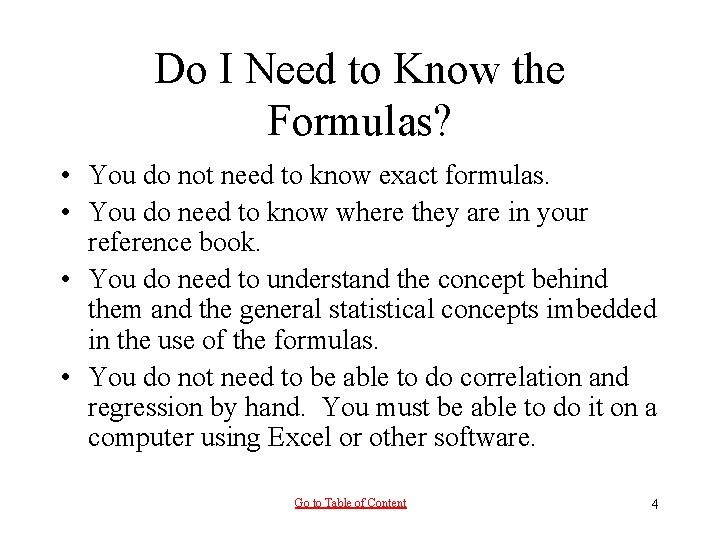
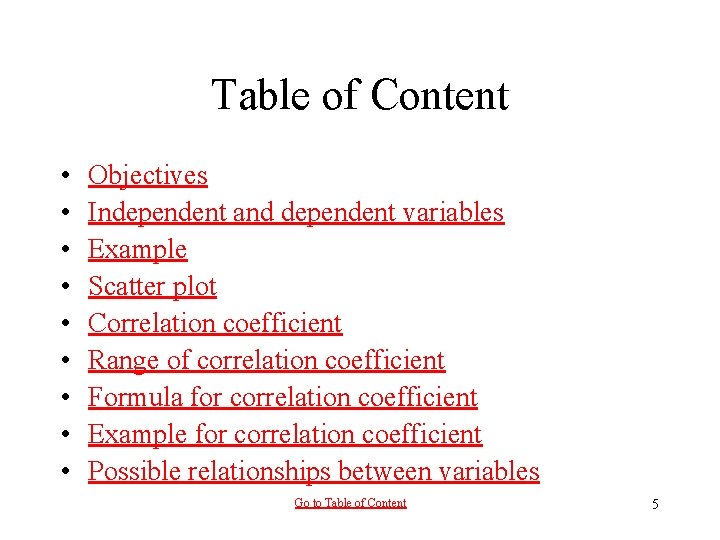
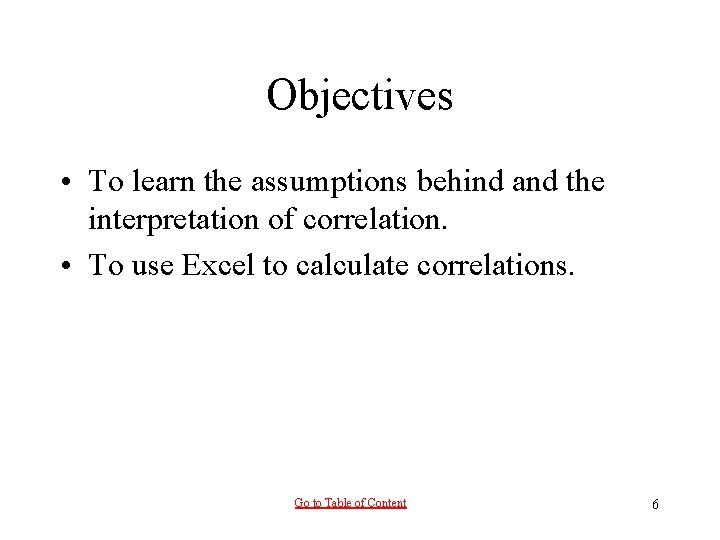
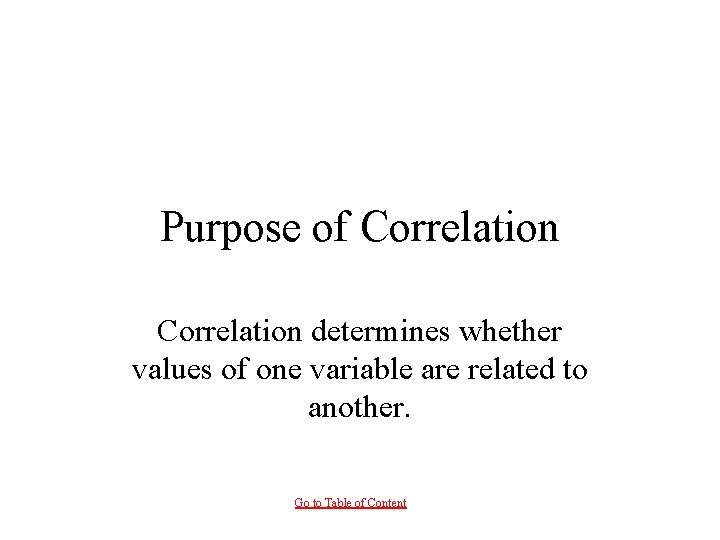
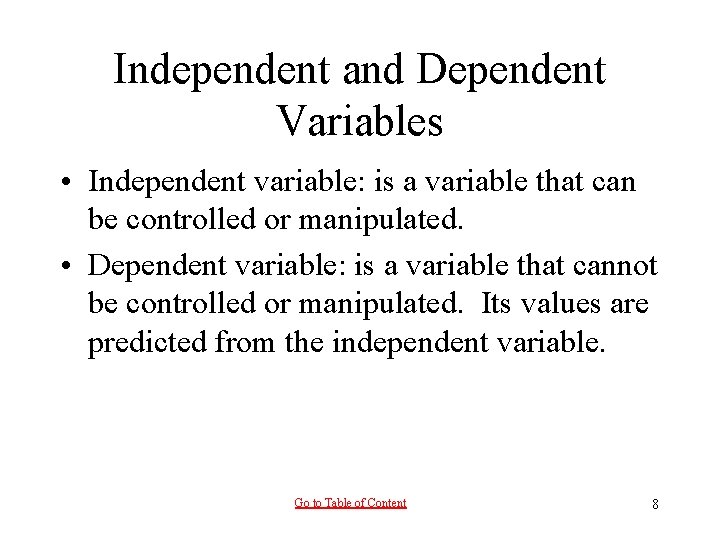
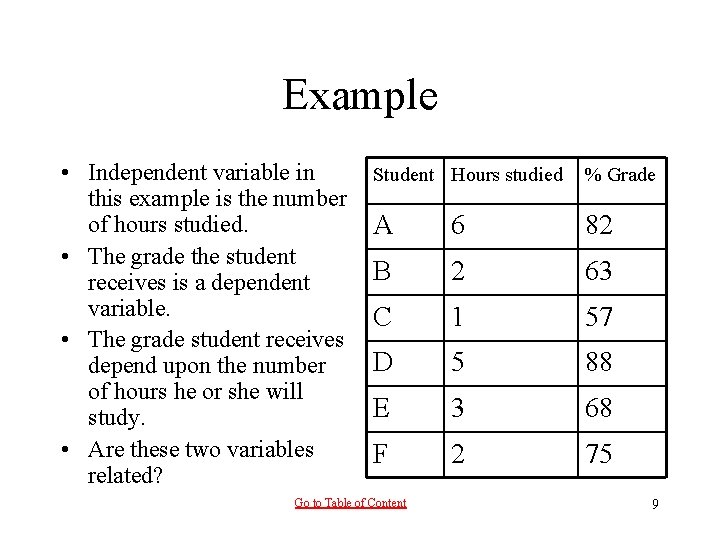
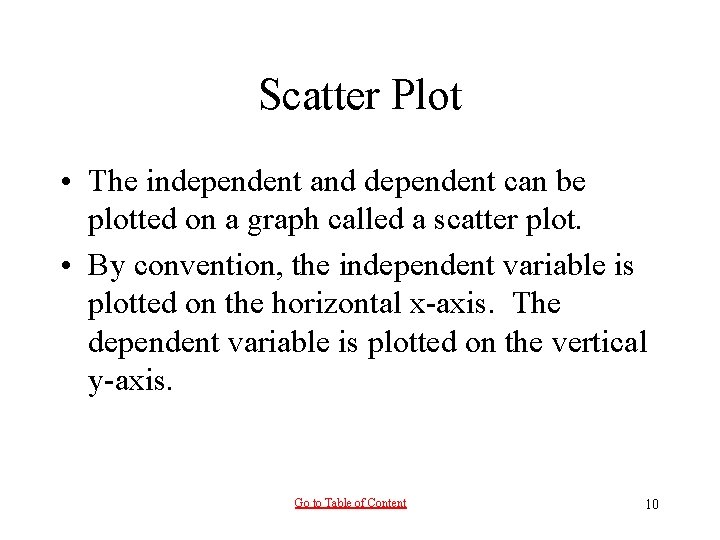
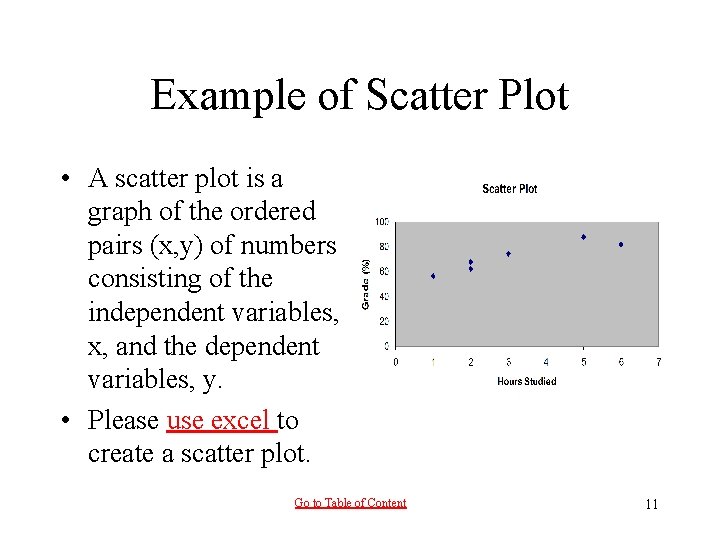
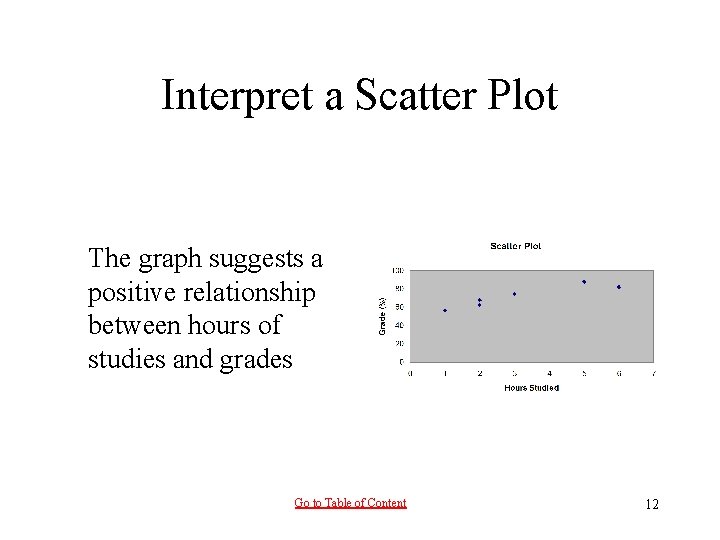
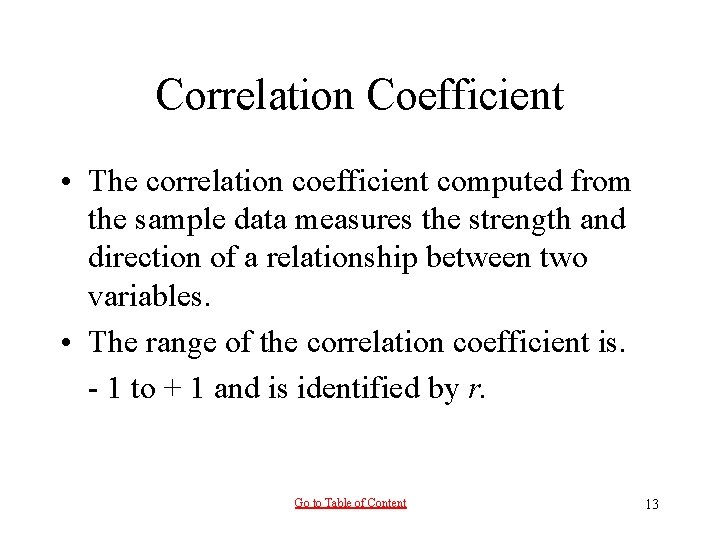
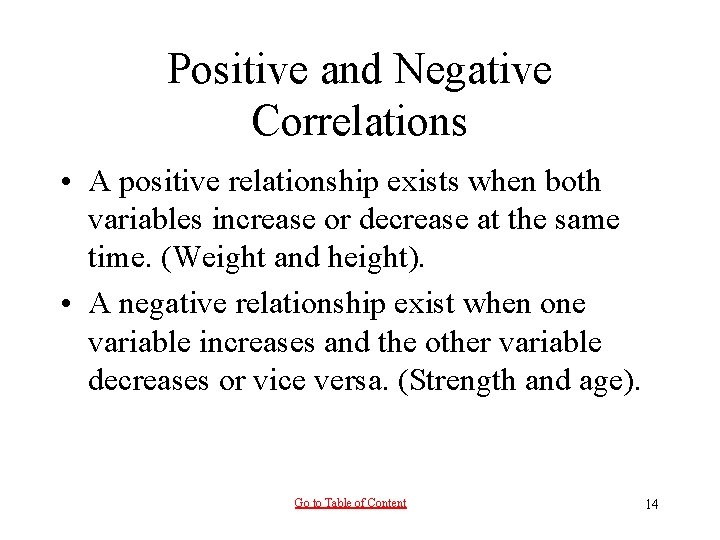
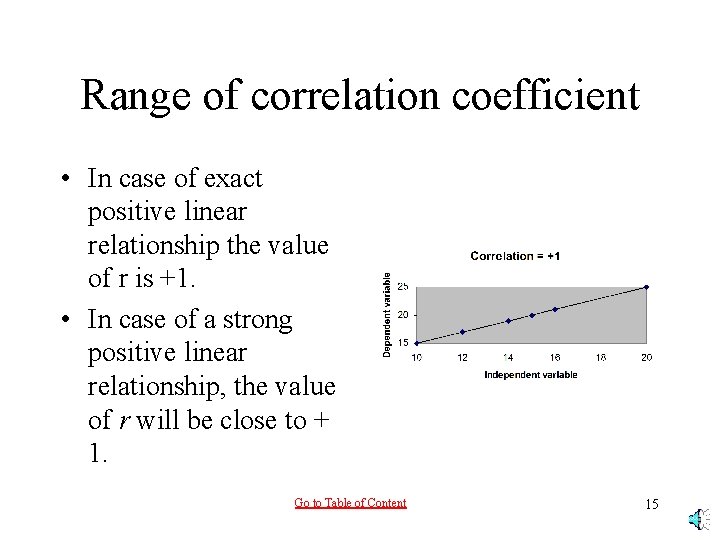
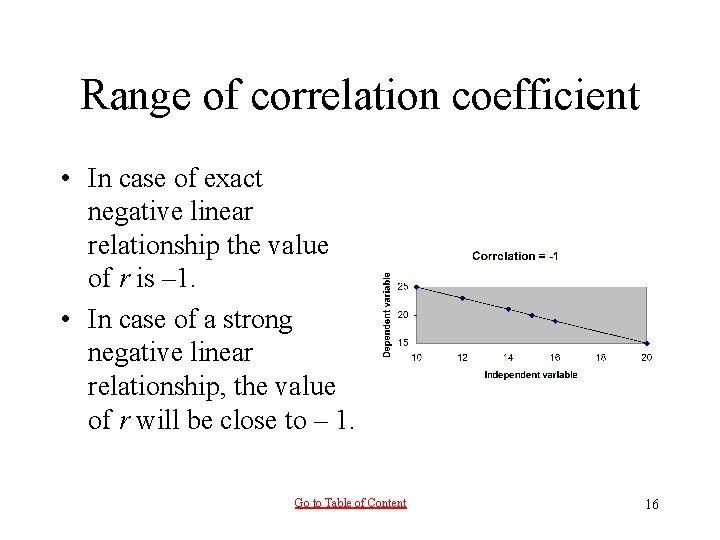
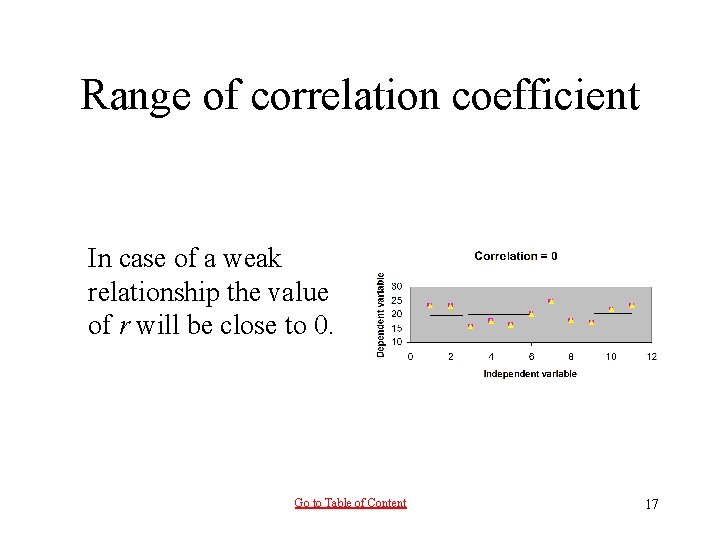
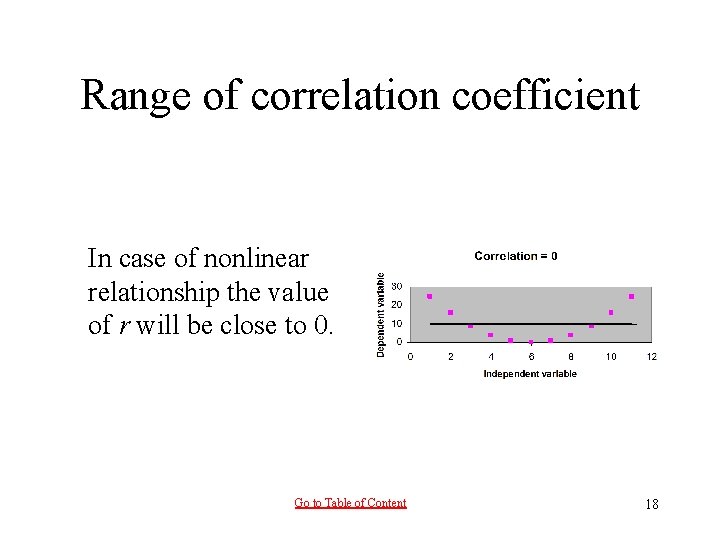
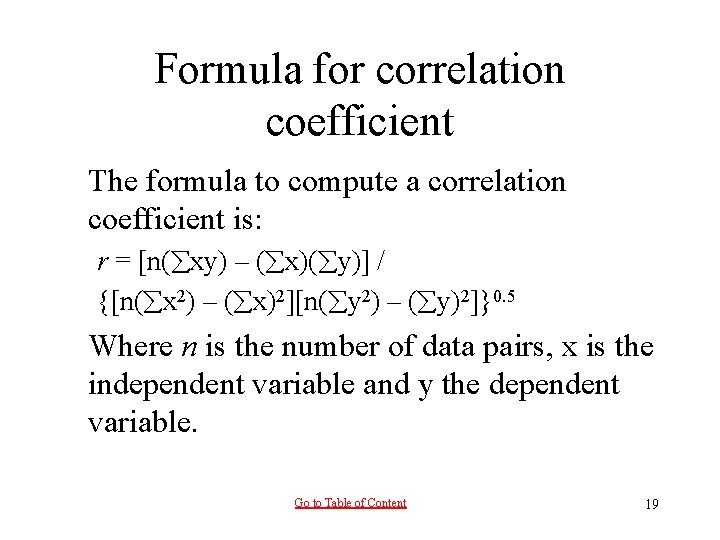
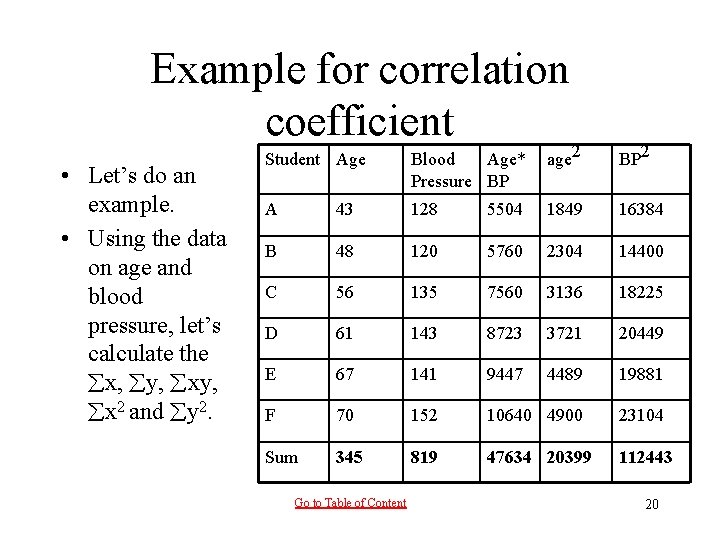
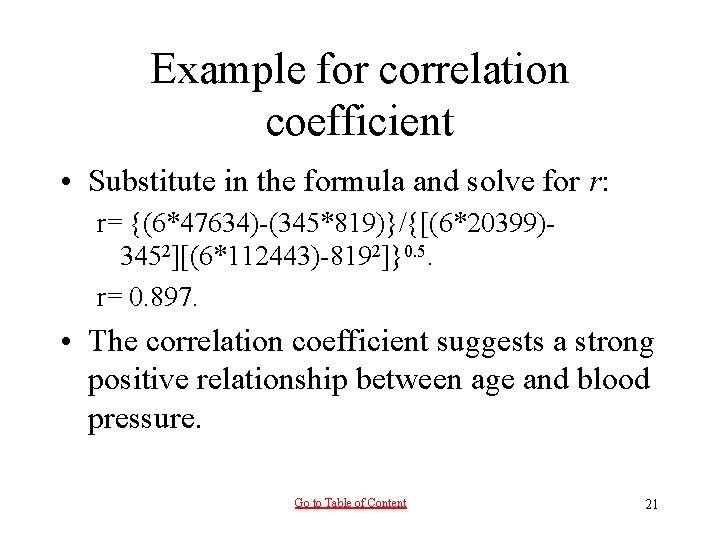
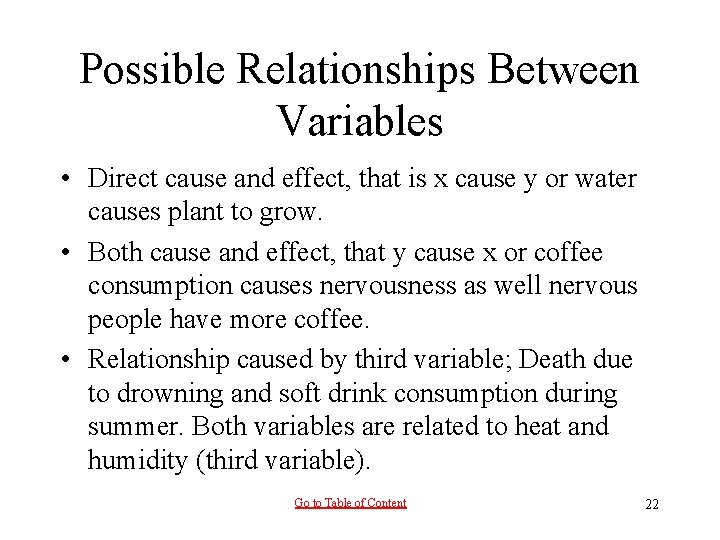
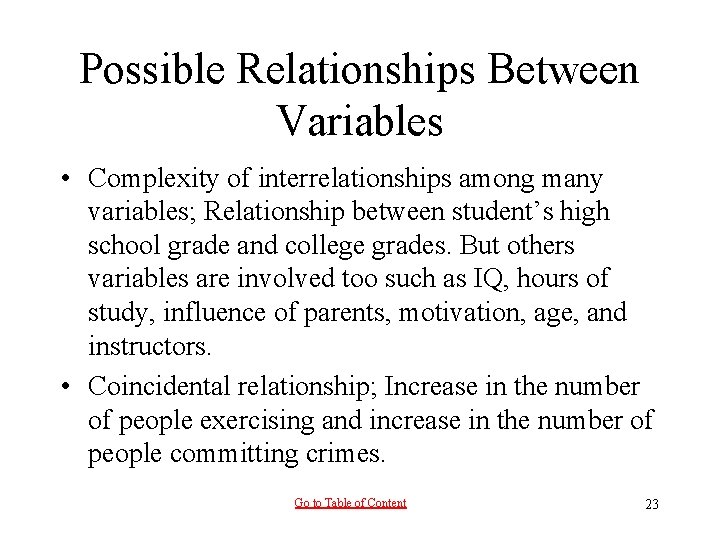
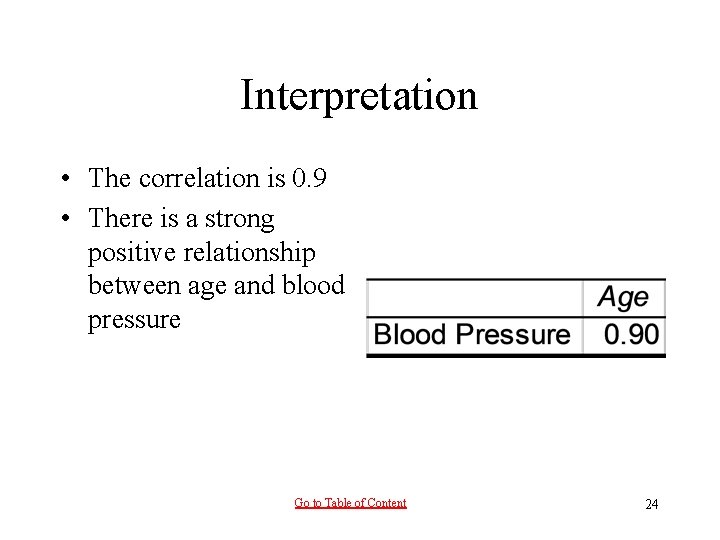
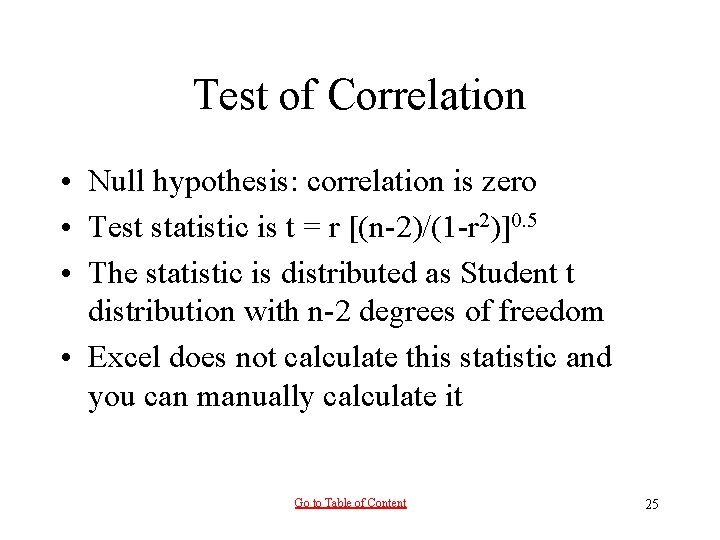
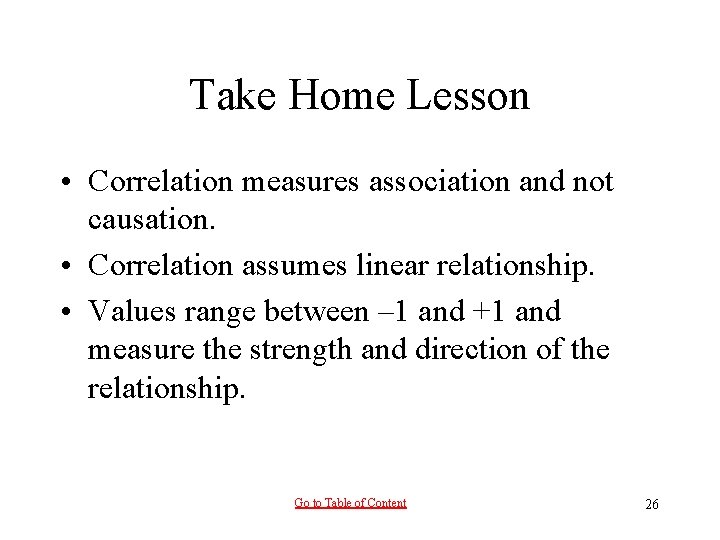
- Slides: 26
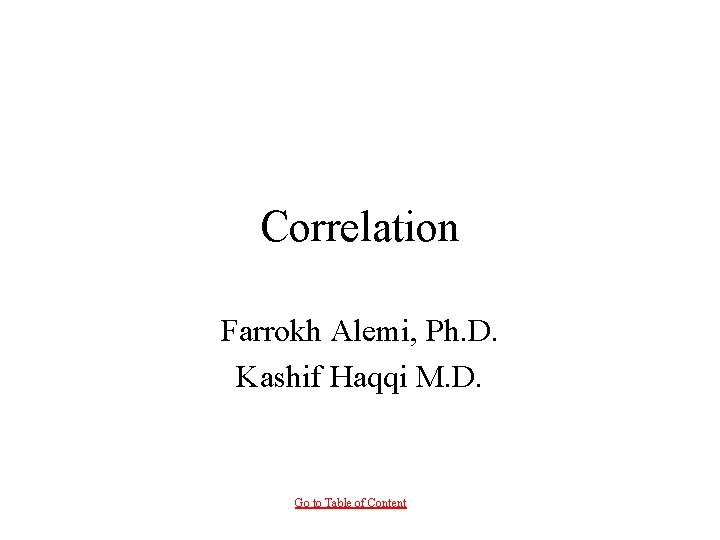
Correlation Farrokh Alemi, Ph. D. Kashif Haqqi M. D. Go to Table of Content
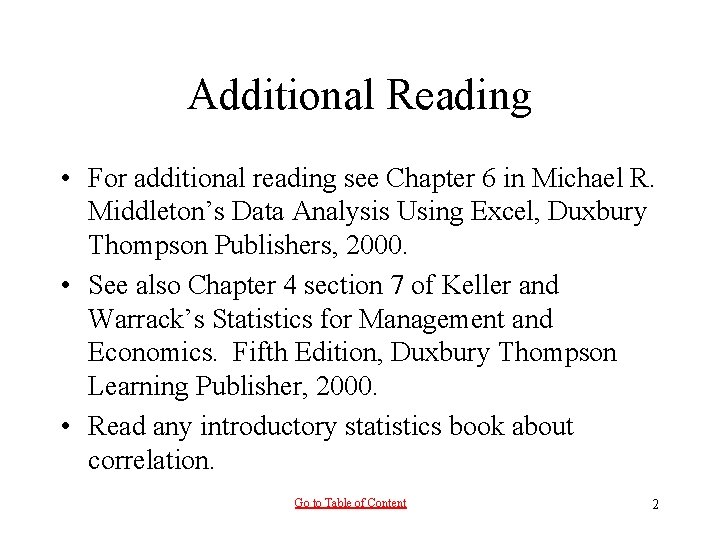
Additional Reading • For additional reading see Chapter 6 in Michael R. Middleton’s Data Analysis Using Excel, Duxbury Thompson Publishers, 2000. • See also Chapter 4 section 7 of Keller and Warrack’s Statistics for Management and Economics. Fifth Edition, Duxbury Thompson Learning Publisher, 2000. • Read any introductory statistics book about correlation. Go to Table of Content 2
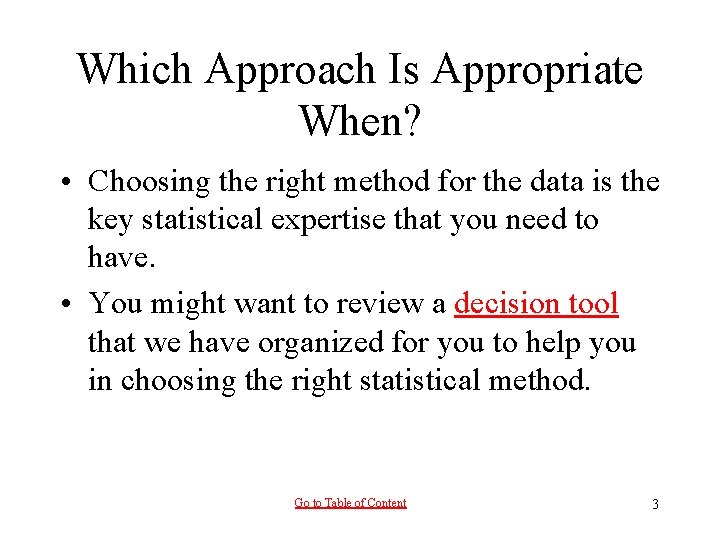
Which Approach Is Appropriate When? • Choosing the right method for the data is the key statistical expertise that you need to have. • You might want to review a decision tool that we have organized for you to help you in choosing the right statistical method. Go to Table of Content 3
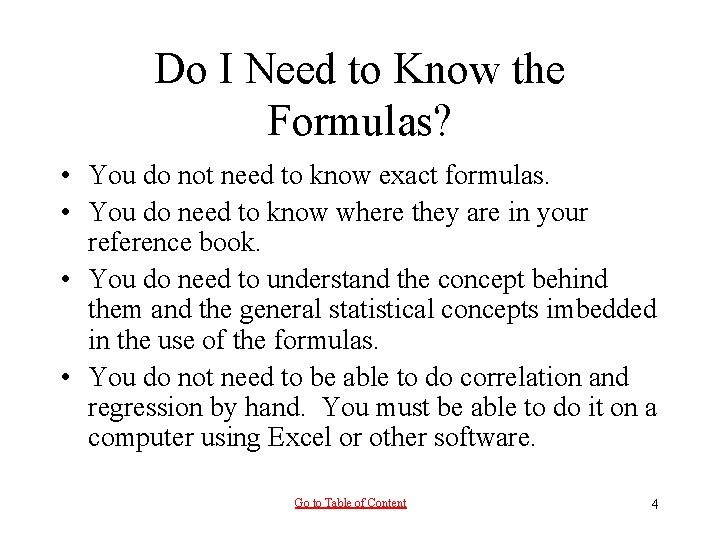
Do I Need to Know the Formulas? • You do not need to know exact formulas. • You do need to know where they are in your reference book. • You do need to understand the concept behind them and the general statistical concepts imbedded in the use of the formulas. • You do not need to be able to do correlation and regression by hand. You must be able to do it on a computer using Excel or other software. Go to Table of Content 4
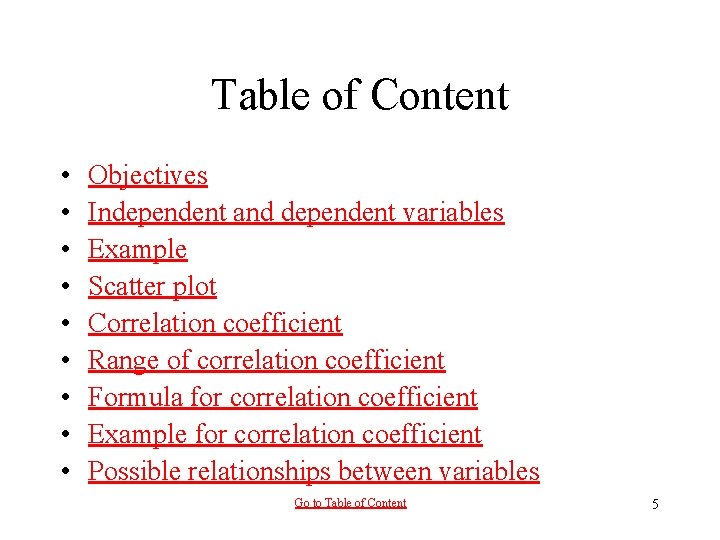
Table of Content • • • Objectives Independent and dependent variables Example Scatter plot Correlation coefficient Range of correlation coefficient Formula for correlation coefficient Example for correlation coefficient Possible relationships between variables Go to Table of Content 5
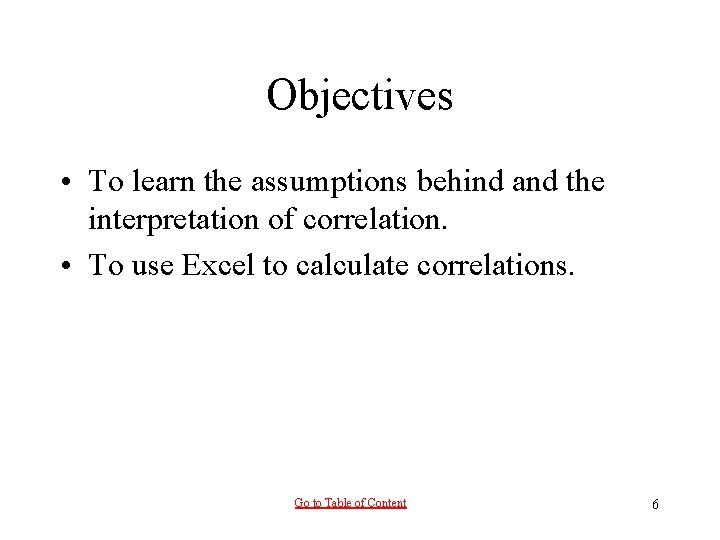
Objectives • To learn the assumptions behind and the interpretation of correlation. • To use Excel to calculate correlations. Go to Table of Content 6
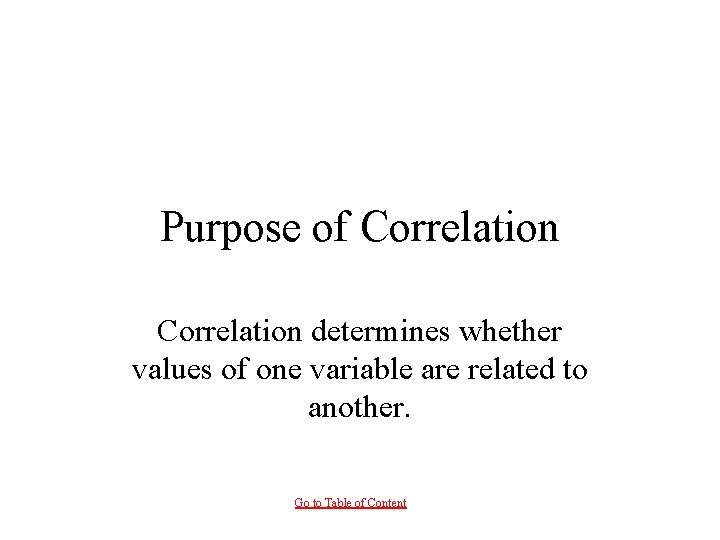
Purpose of Correlation determines whether values of one variable are related to another. Go to Table of Content
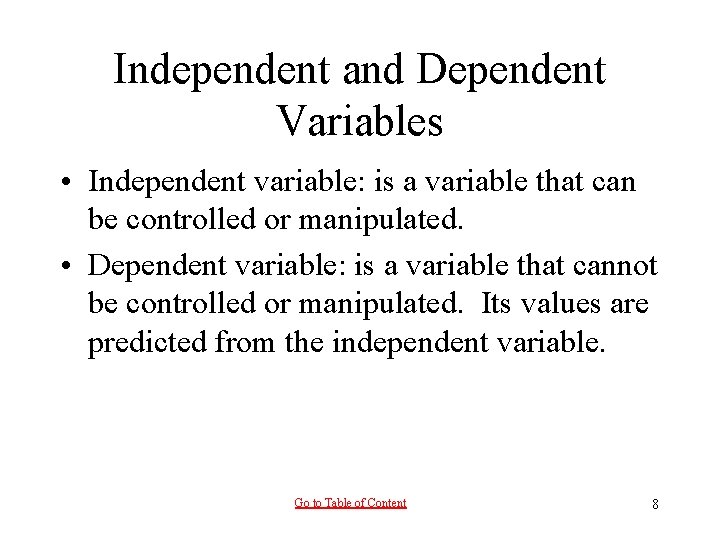
Independent and Dependent Variables • Independent variable: is a variable that can be controlled or manipulated. • Dependent variable: is a variable that cannot be controlled or manipulated. Its values are predicted from the independent variable. Go to Table of Content 8
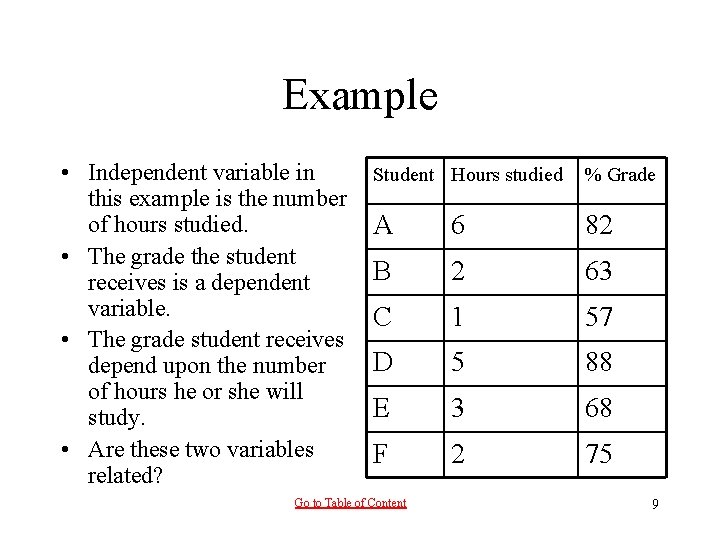
Example • Independent variable in this example is the number of hours studied. • The grade the student receives is a dependent variable. • The grade student receives depend upon the number of hours he or she will study. • Are these two variables related? Student Hours studied % Grade A 6 82 B 2 63 C 1 57 D 5 88 E 3 68 F 2 75 Go to Table of Content 9
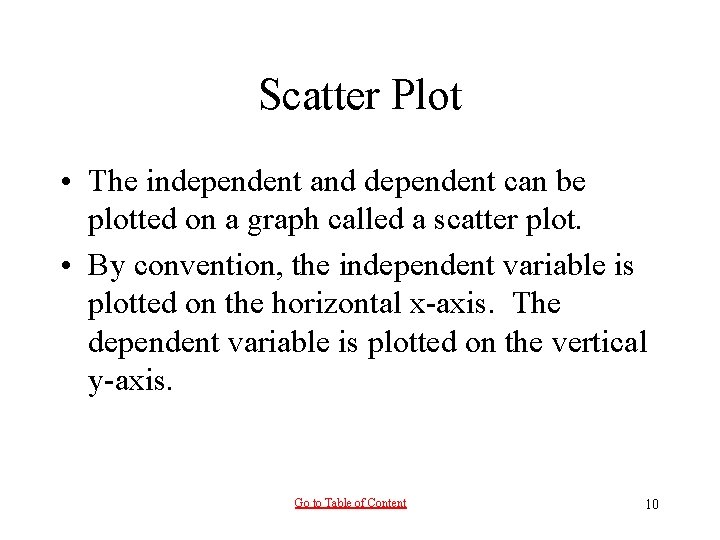
Scatter Plot • The independent and dependent can be plotted on a graph called a scatter plot. • By convention, the independent variable is plotted on the horizontal x-axis. The dependent variable is plotted on the vertical y-axis. Go to Table of Content 10
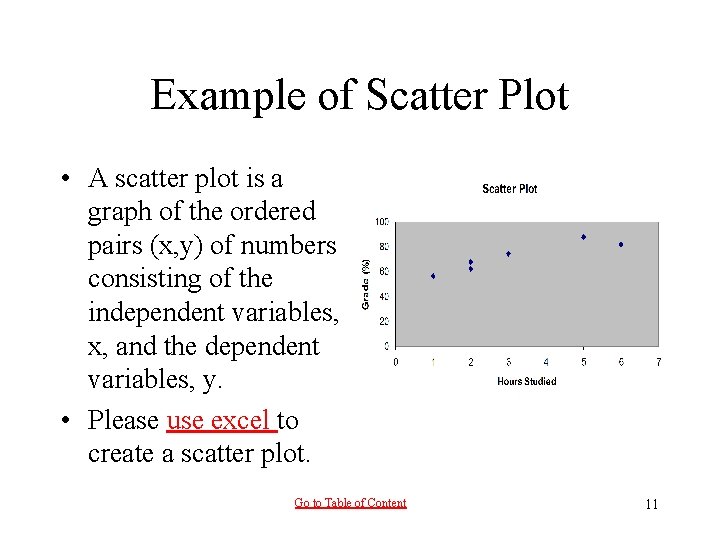
Example of Scatter Plot • A scatter plot is a graph of the ordered pairs (x, y) of numbers consisting of the independent variables, x, and the dependent variables, y. • Please use excel to create a scatter plot. Go to Table of Content 11
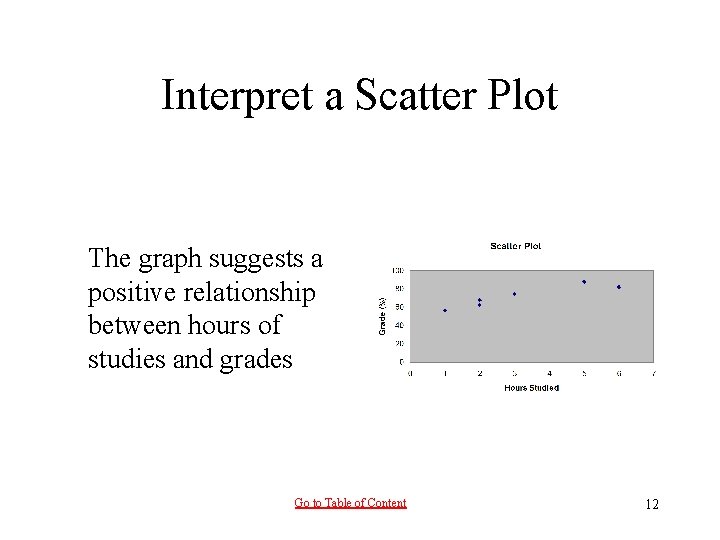
Interpret a Scatter Plot The graph suggests a positive relationship between hours of studies and grades Go to Table of Content 12
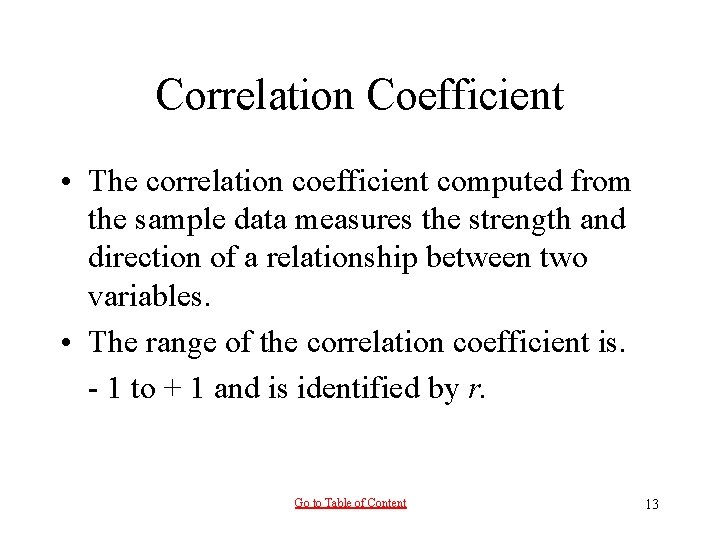
Correlation Coefficient • The correlation coefficient computed from the sample data measures the strength and direction of a relationship between two variables. • The range of the correlation coefficient is. - 1 to + 1 and is identified by r. Go to Table of Content 13
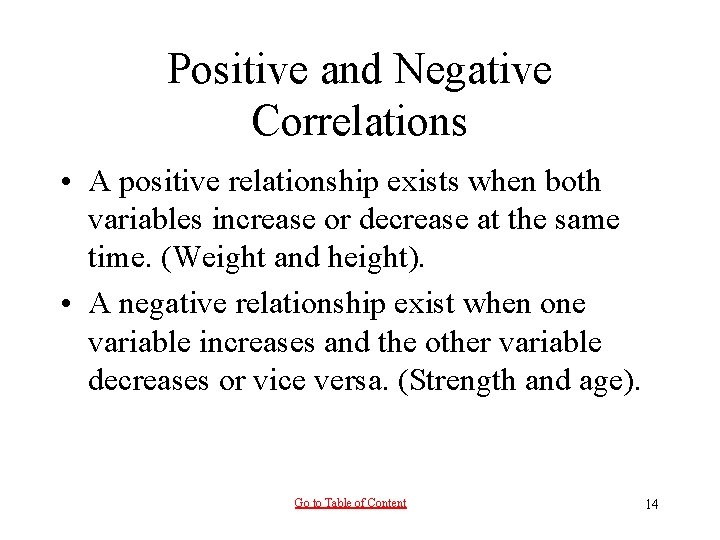
Positive and Negative Correlations • A positive relationship exists when both variables increase or decrease at the same time. (Weight and height). • A negative relationship exist when one variable increases and the other variable decreases or vice versa. (Strength and age). Go to Table of Content 14
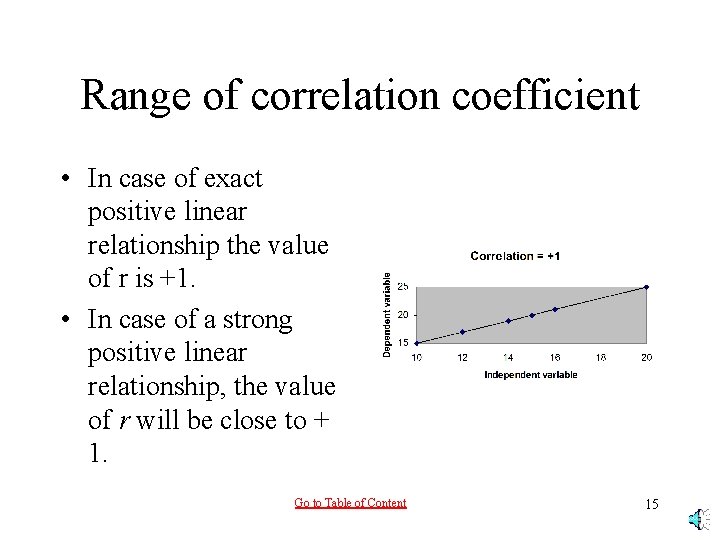
Range of correlation coefficient • In case of exact positive linear relationship the value of r is +1. • In case of a strong positive linear relationship, the value of r will be close to + 1. Go to Table of Content 15
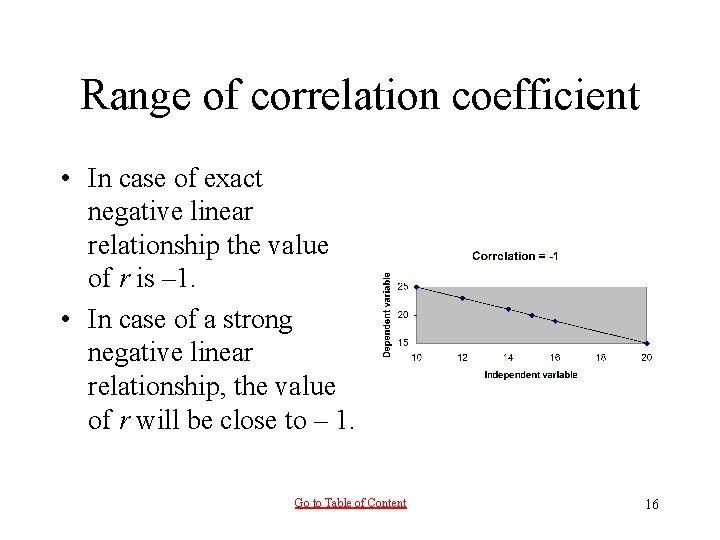
Range of correlation coefficient • In case of exact negative linear relationship the value of r is – 1. • In case of a strong negative linear relationship, the value of r will be close to – 1. Go to Table of Content 16
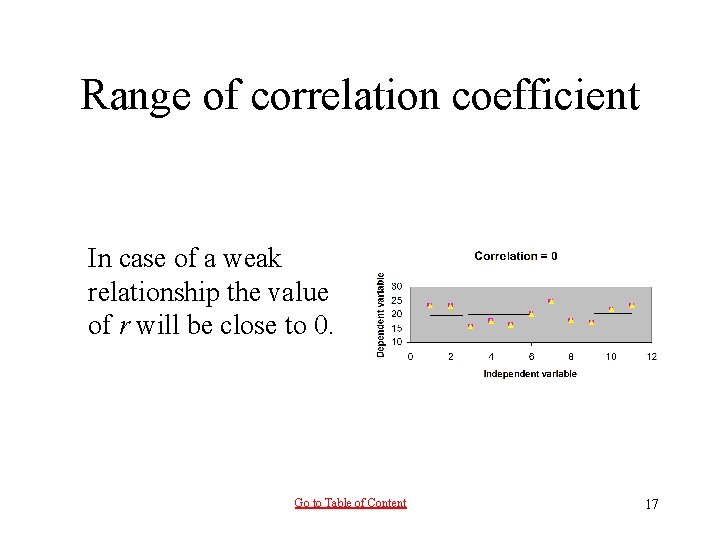
Range of correlation coefficient In case of a weak relationship the value of r will be close to 0. Go to Table of Content 17
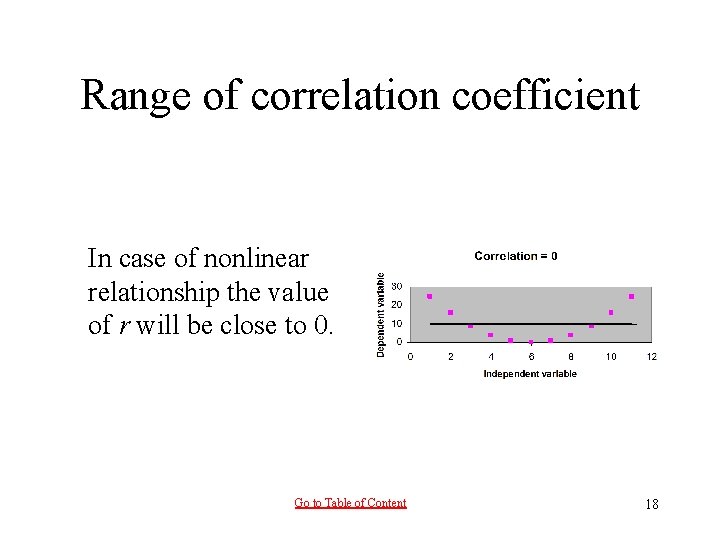
Range of correlation coefficient In case of nonlinear relationship the value of r will be close to 0. Go to Table of Content 18
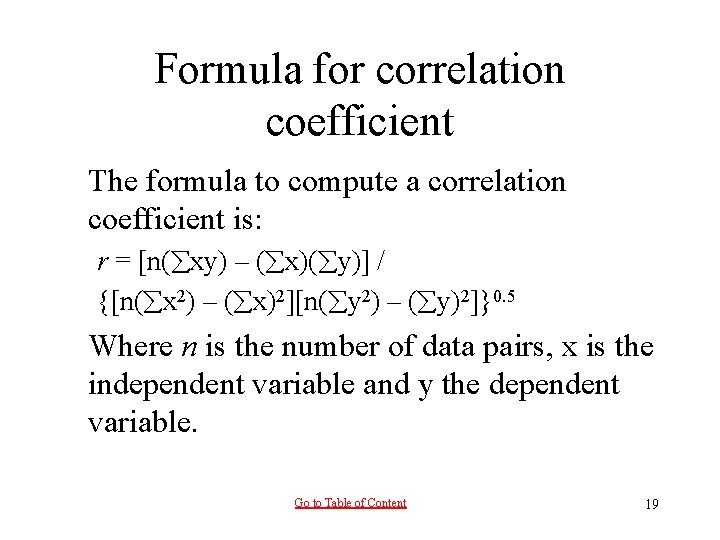
Formula for correlation coefficient The formula to compute a correlation coefficient is: r = [n( xy) – ( x)( y)] / {[n( x 2) – ( x)2][n( y 2) – ( y)2]}0. 5 Where n is the number of data pairs, x is the independent variable and y the dependent variable. Go to Table of Content 19
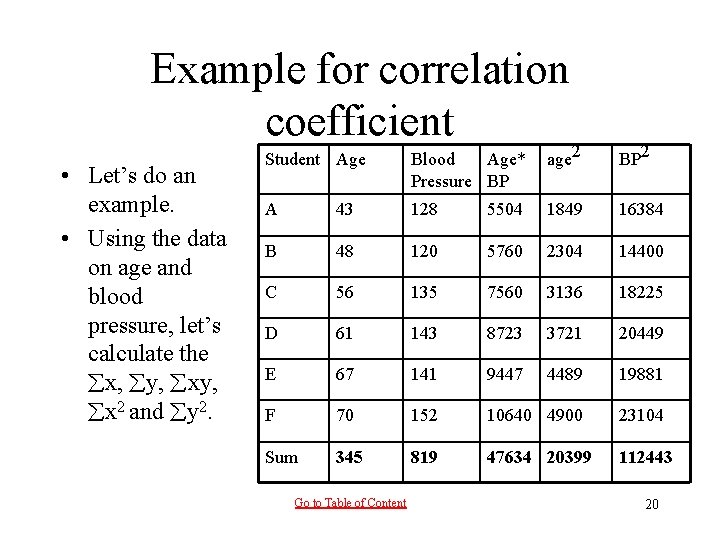
Example for correlation coefficient 2 • Let’s do an example. • Using the data on age and blood pressure, let’s calculate the x, y, x 2 and y 2. Student Age Blood Age* Pressure BP age BP 2 A 43 128 5504 1849 16384 B 48 120 5760 2304 14400 C 56 135 7560 3136 18225 D 61 143 8723 3721 20449 E 67 141 9447 4489 19881 F 70 152 10640 4900 23104 Sum 345 819 47634 20399 112443 Go to Table of Content 20
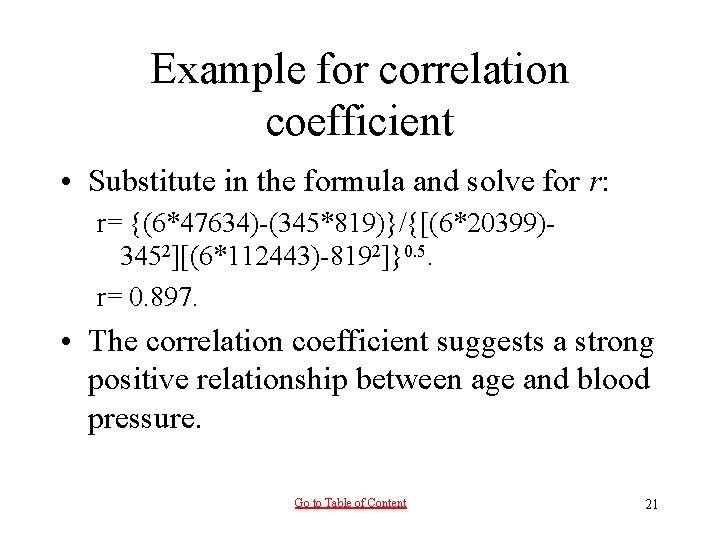
Example for correlation coefficient • Substitute in the formula and solve for r: r= {(6*47634)-(345*819)}/{[(6*20399)3452][(6*112443)-8192]}0. 5. r= 0. 897. • The correlation coefficient suggests a strong positive relationship between age and blood pressure. Go to Table of Content 21
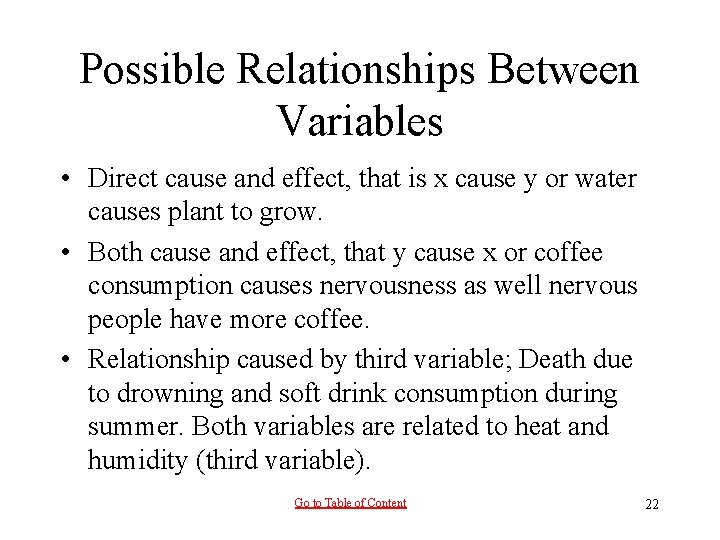
Possible Relationships Between Variables • Direct cause and effect, that is x cause y or water causes plant to grow. • Both cause and effect, that y cause x or coffee consumption causes nervousness as well nervous people have more coffee. • Relationship caused by third variable; Death due to drowning and soft drink consumption during summer. Both variables are related to heat and humidity (third variable). Go to Table of Content 22
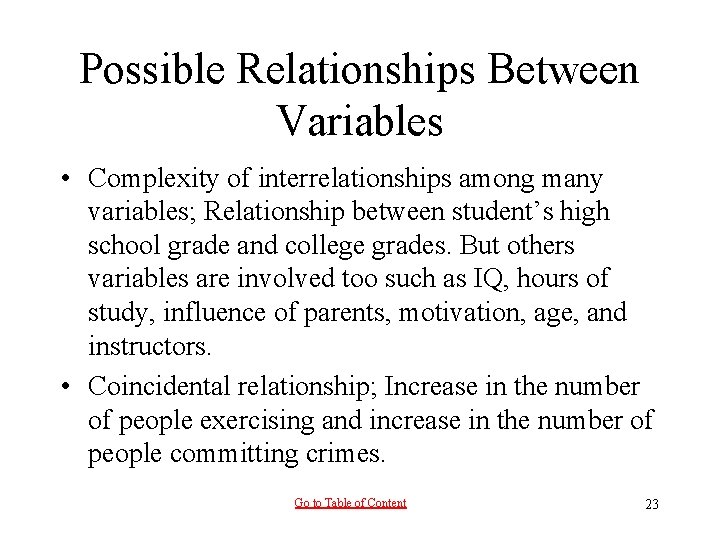
Possible Relationships Between Variables • Complexity of interrelationships among many variables; Relationship between student’s high school grade and college grades. But others variables are involved too such as IQ, hours of study, influence of parents, motivation, age, and instructors. • Coincidental relationship; Increase in the number of people exercising and increase in the number of people committing crimes. Go to Table of Content 23
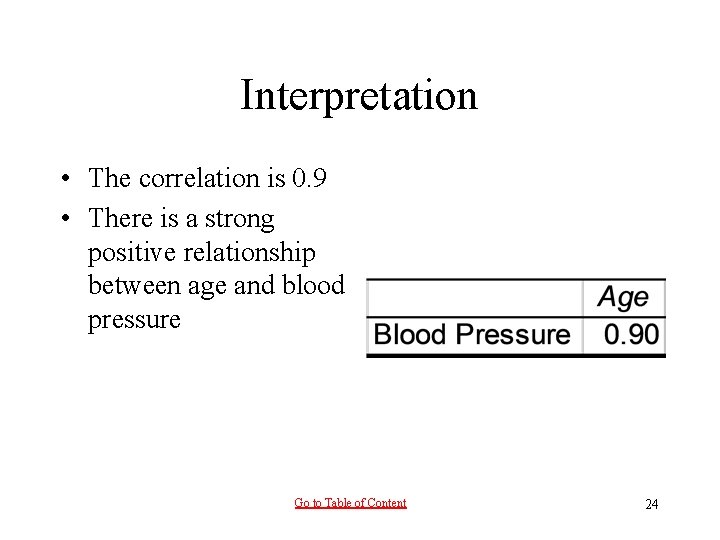
Interpretation • The correlation is 0. 9 • There is a strong positive relationship between age and blood pressure Go to Table of Content 24
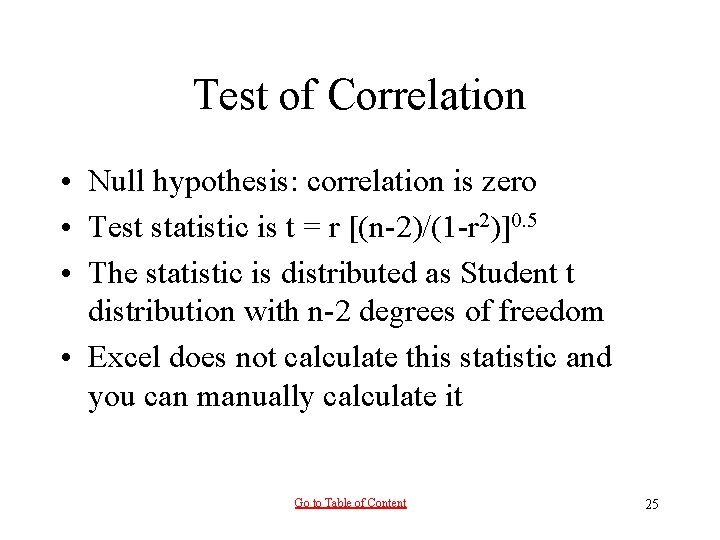
Test of Correlation • Null hypothesis: correlation is zero • Test statistic is t = r [(n-2)/(1 -r 2)]0. 5 • The statistic is distributed as Student t distribution with n-2 degrees of freedom • Excel does not calculate this statistic and you can manually calculate it Go to Table of Content 25
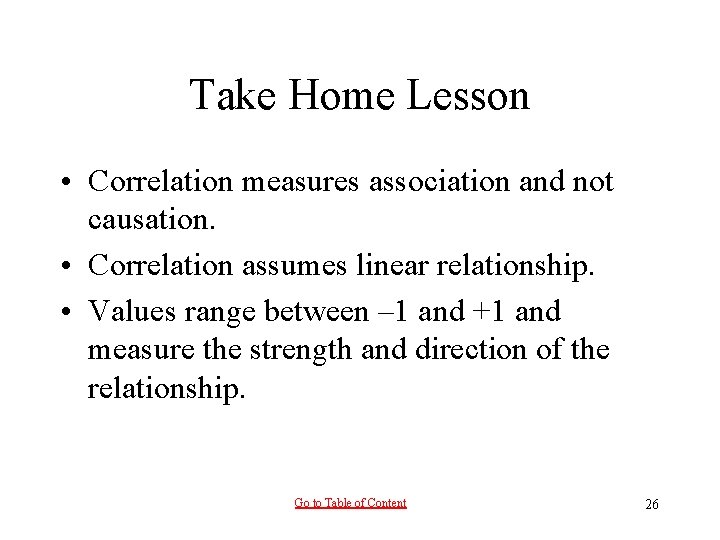
Take Home Lesson • Correlation measures association and not causation. • Correlation assumes linear relationship. • Values range between – 1 and +1 and measure the strength and direction of the relationship. Go to Table of Content 26
Kashif haqqi
Kashif haqqi
Kashif ghani
10 importance of health education
Fatima kashif
Negative and positive correlation
Positive correlation versus negative correlation
Kari skogland protitah
Kıbrıs akasyası
Monera alemi
Państwa roślinne
Cnler
Correlation coefficient
Canonical correlation assumptions
Clinical correlation
X and y axis dependent and independent
How many jelly beans
Which describes the correlation shown in the scatterplot?
Jumlah sampel minimal 30
Correlation research question example
Difference between correlation and regression
Correlation coefficient in analytical chemistry
Association vs correlation
Correlation diagram
Tabel spearman rank
Correlational descriptive
Correlation partielle