Sentiment Analysis Farrokh Alemi Ph D Wednesday April
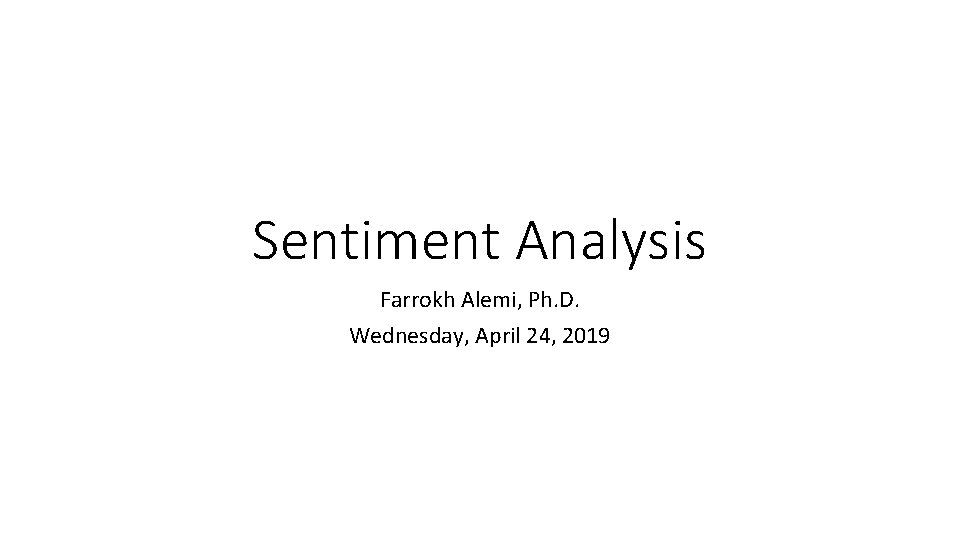
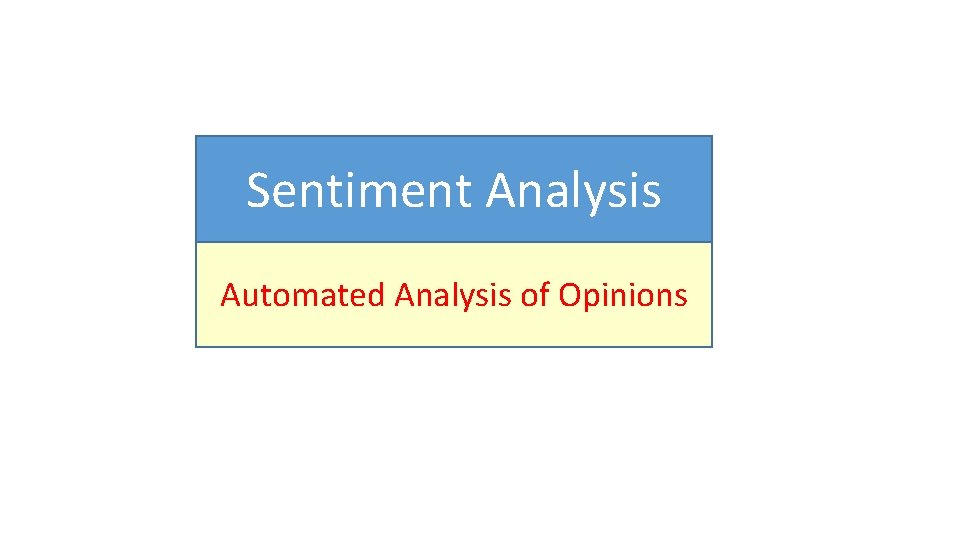
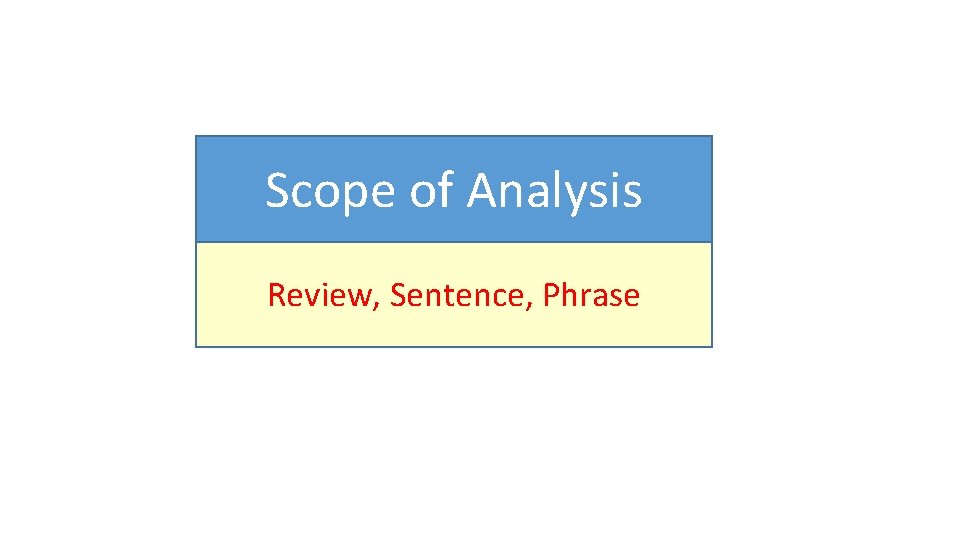
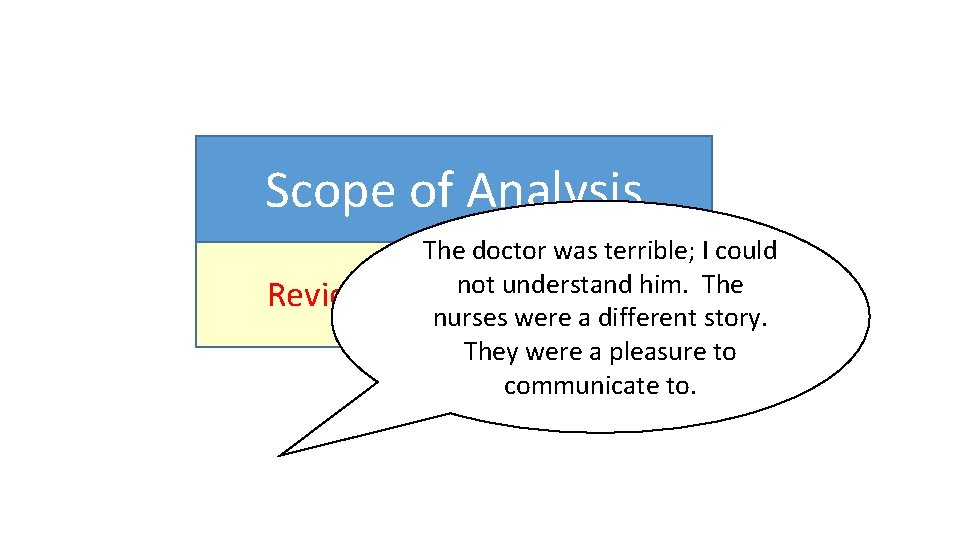
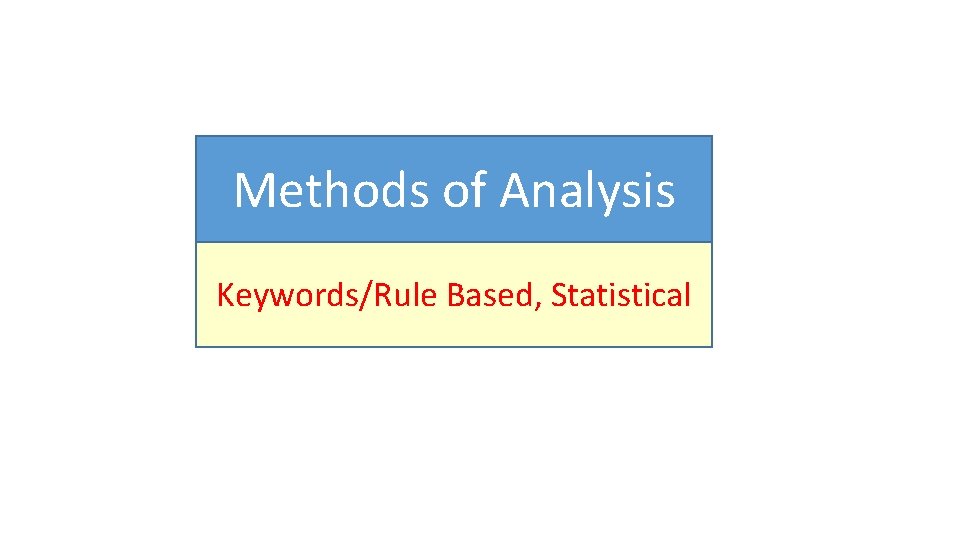
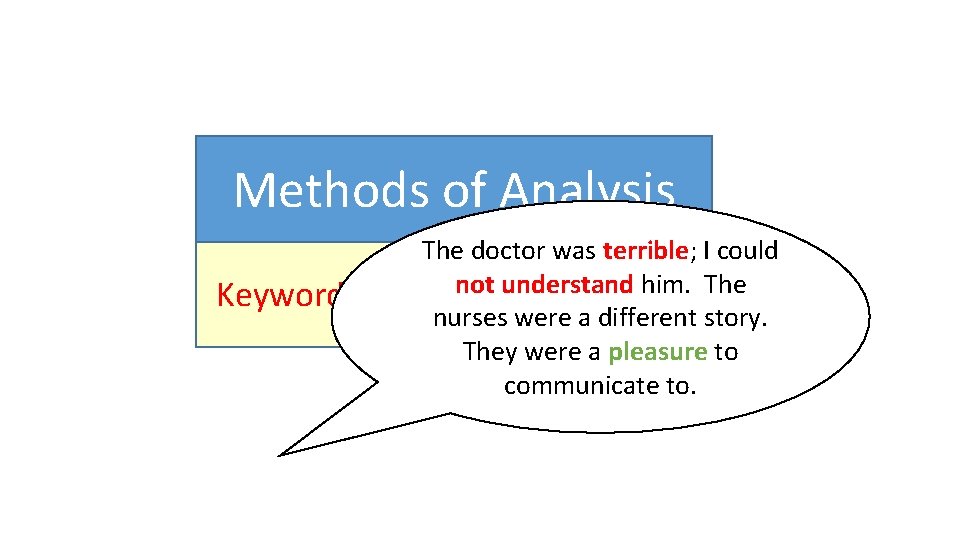
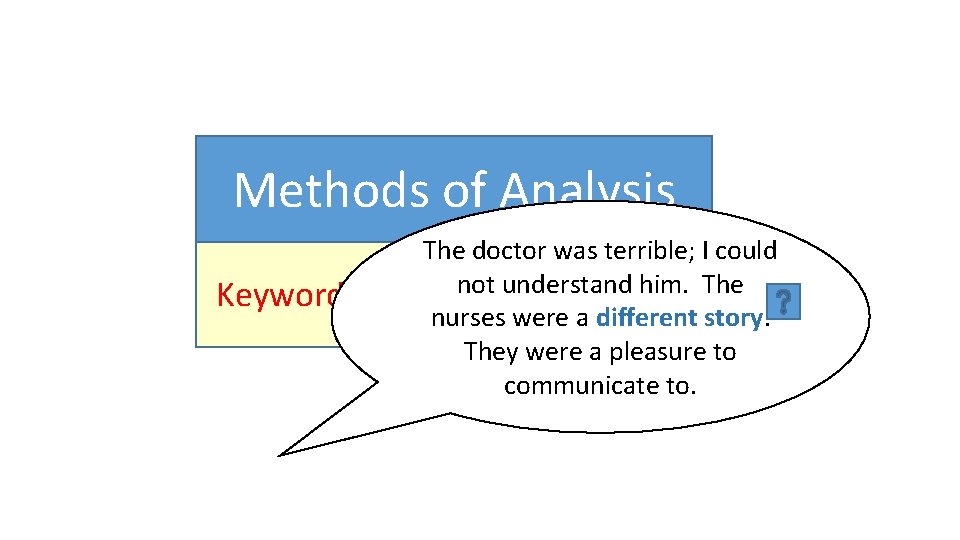
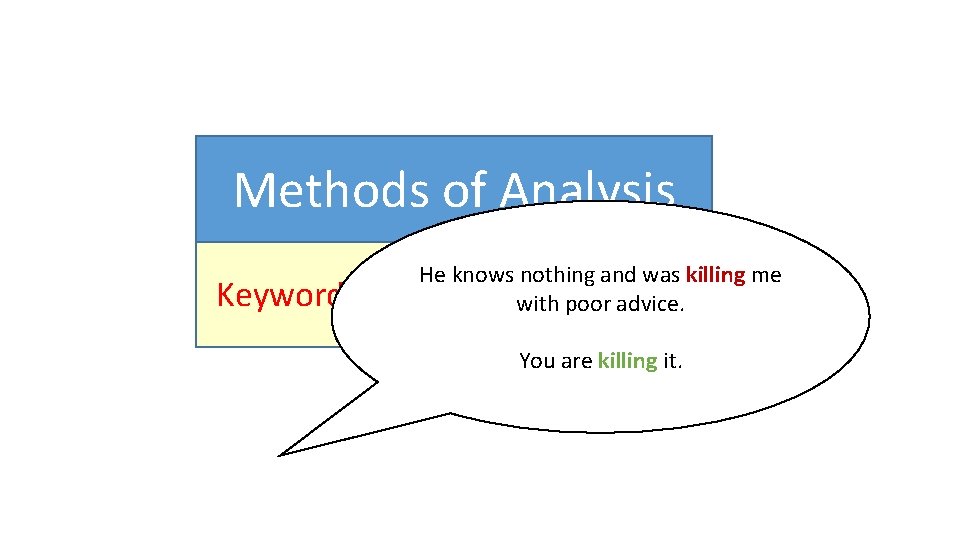
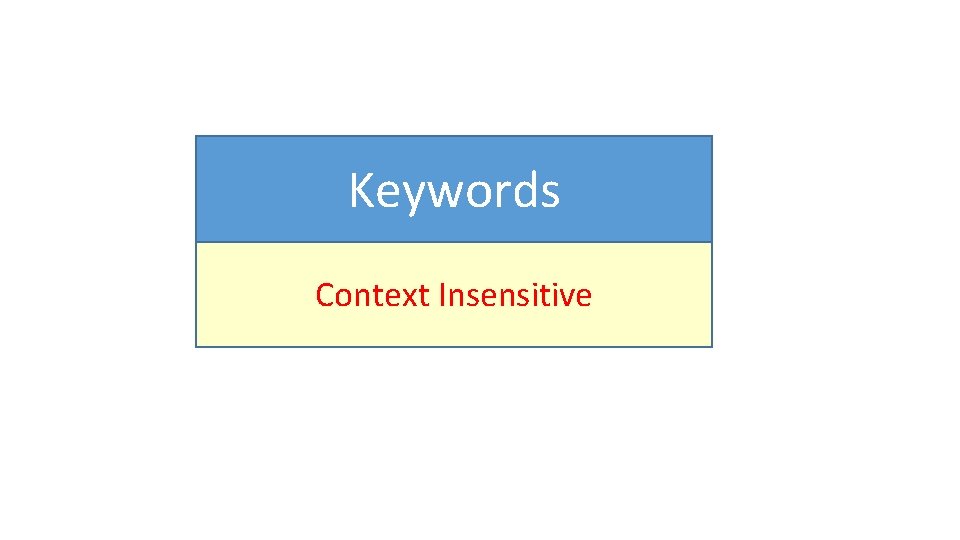
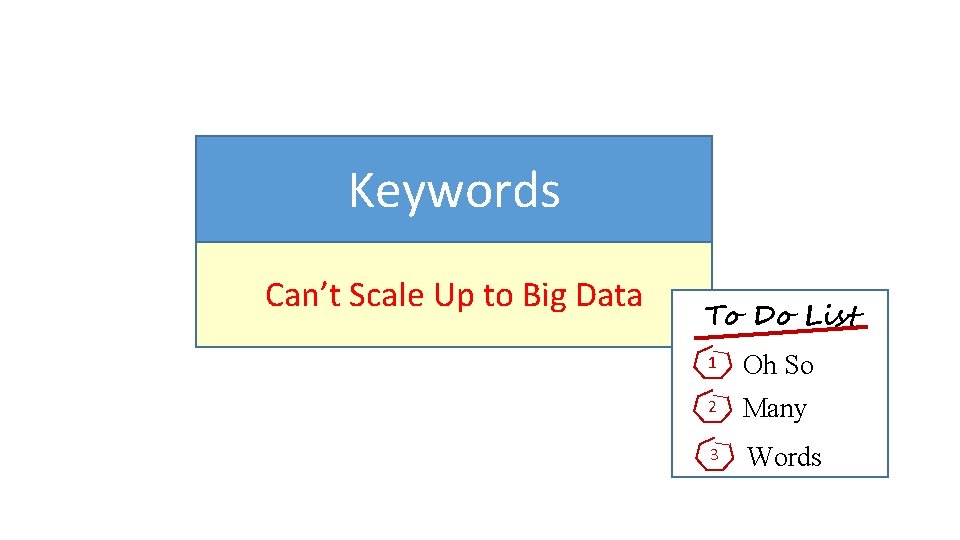
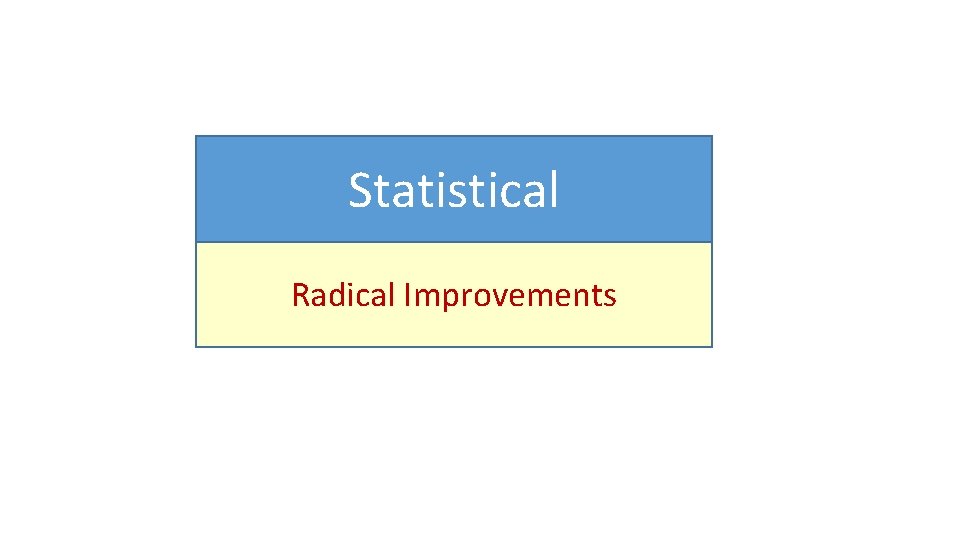
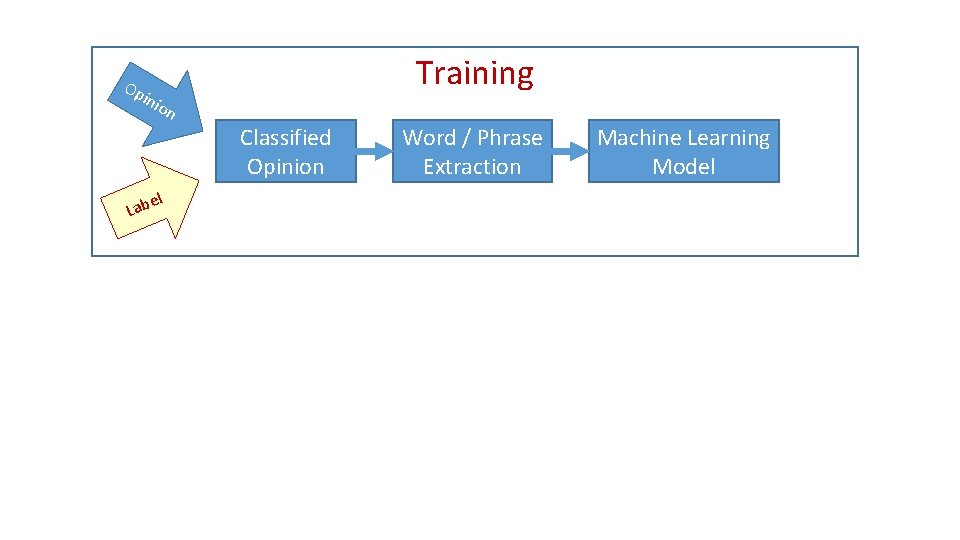
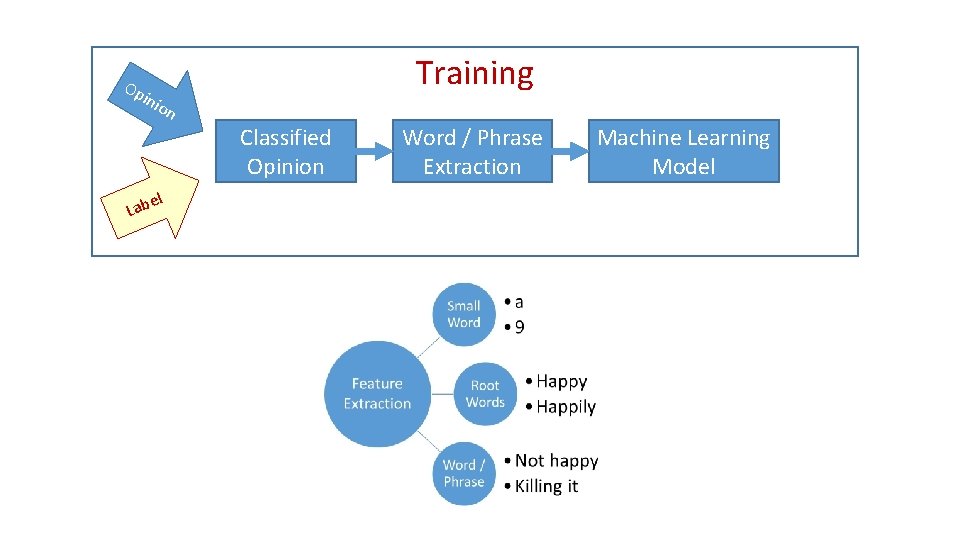
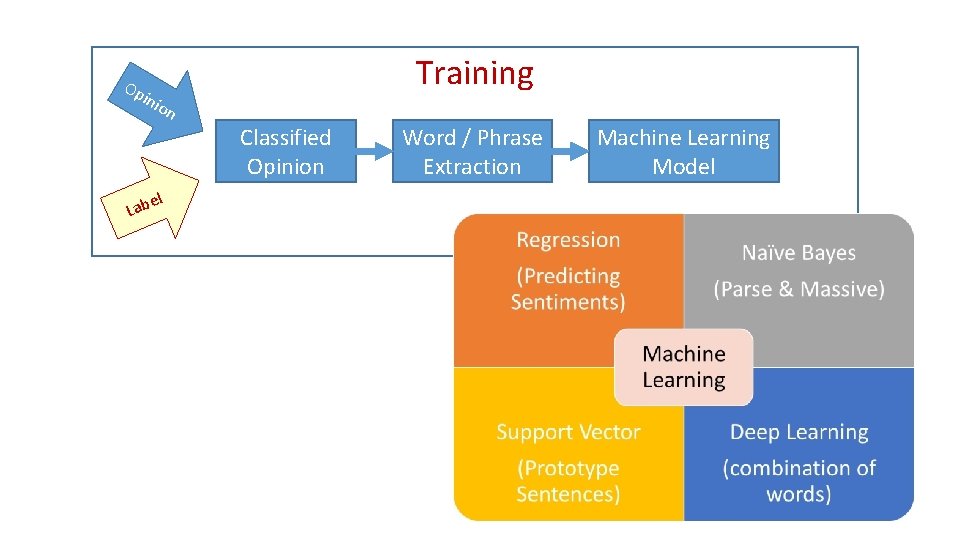
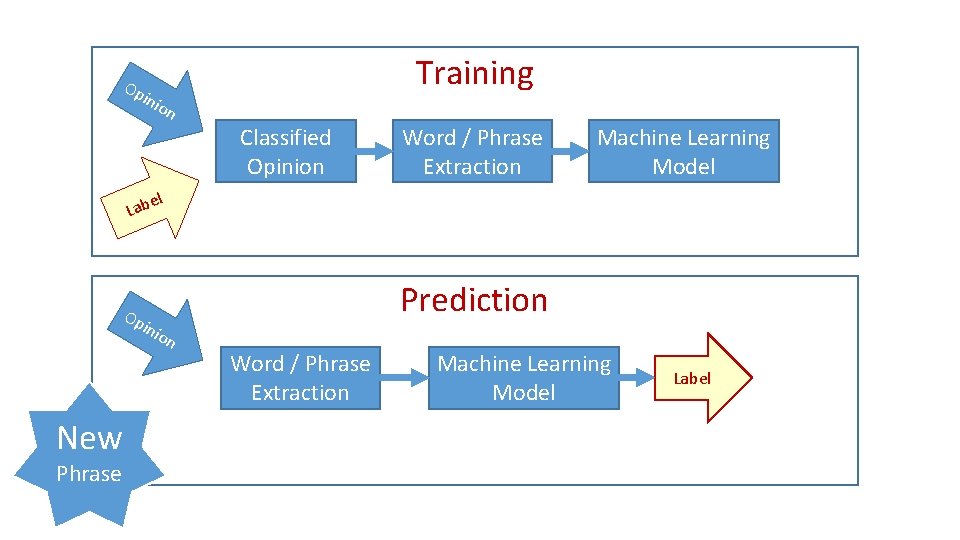
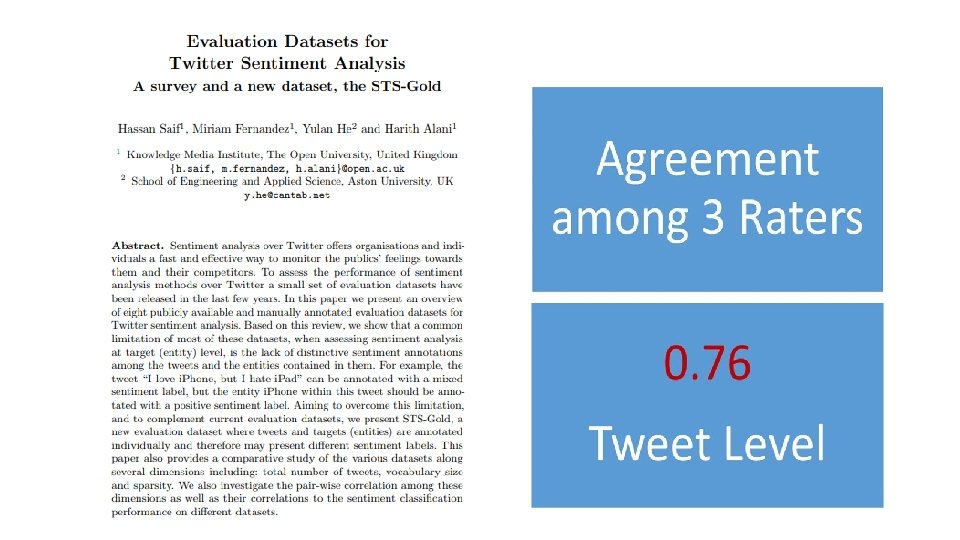
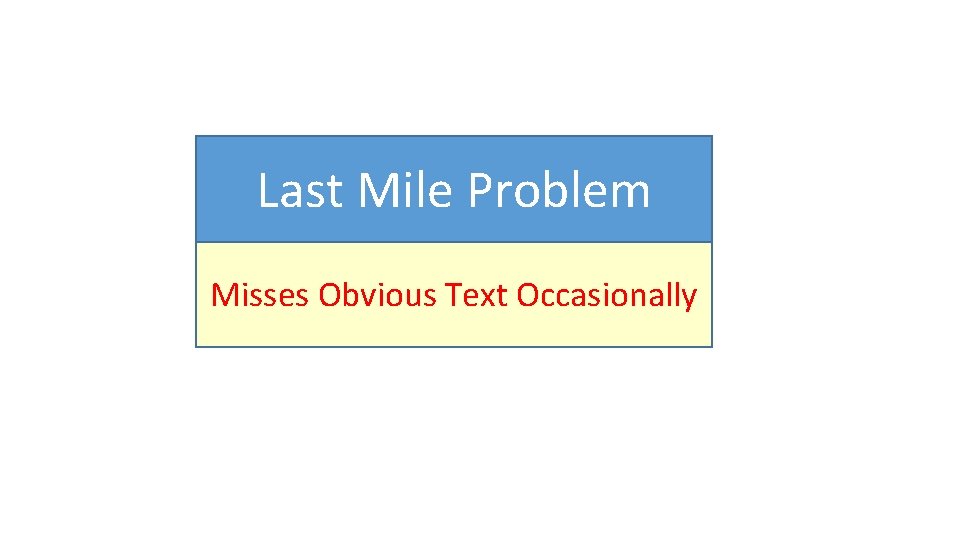
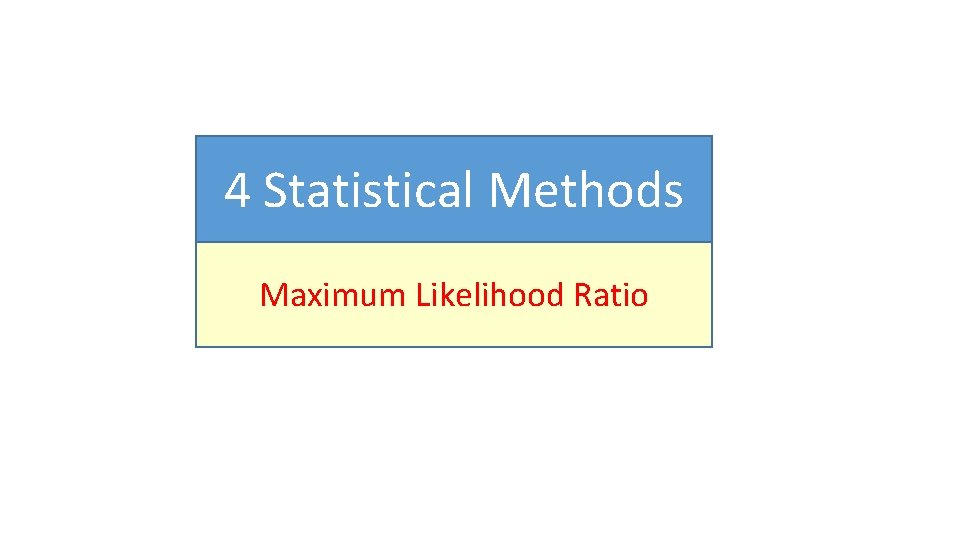
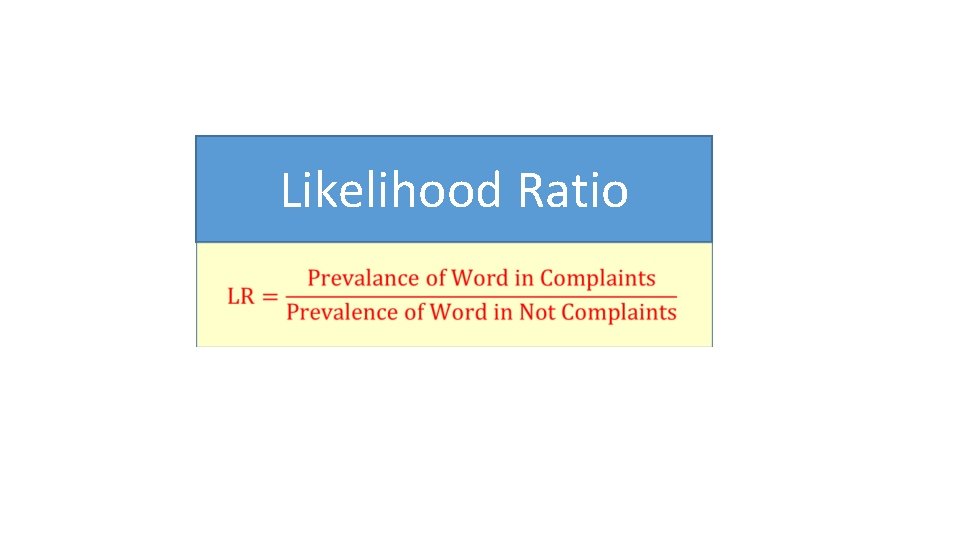
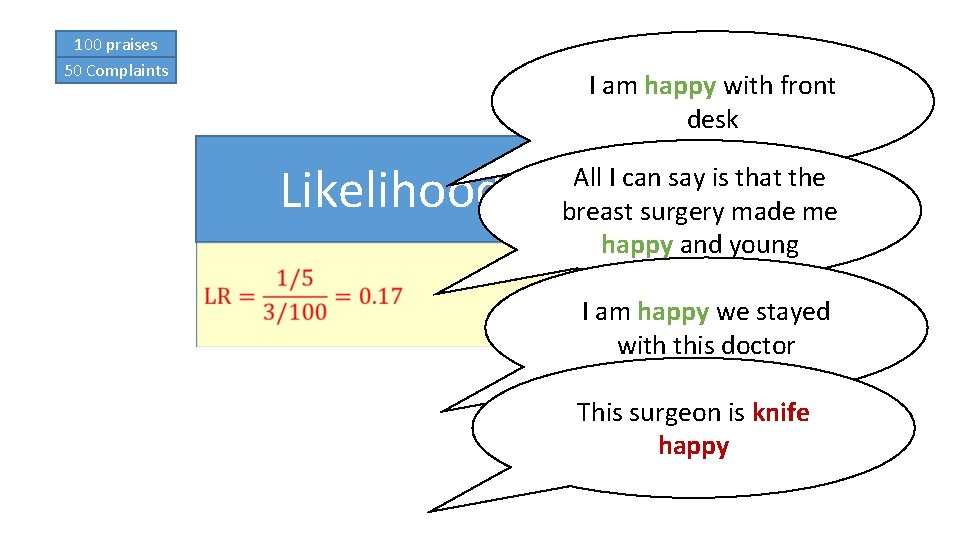
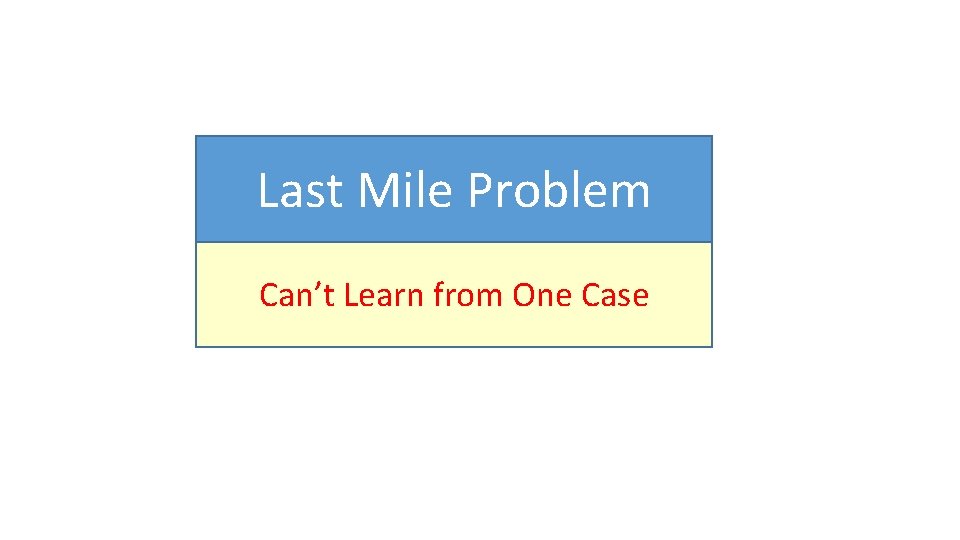
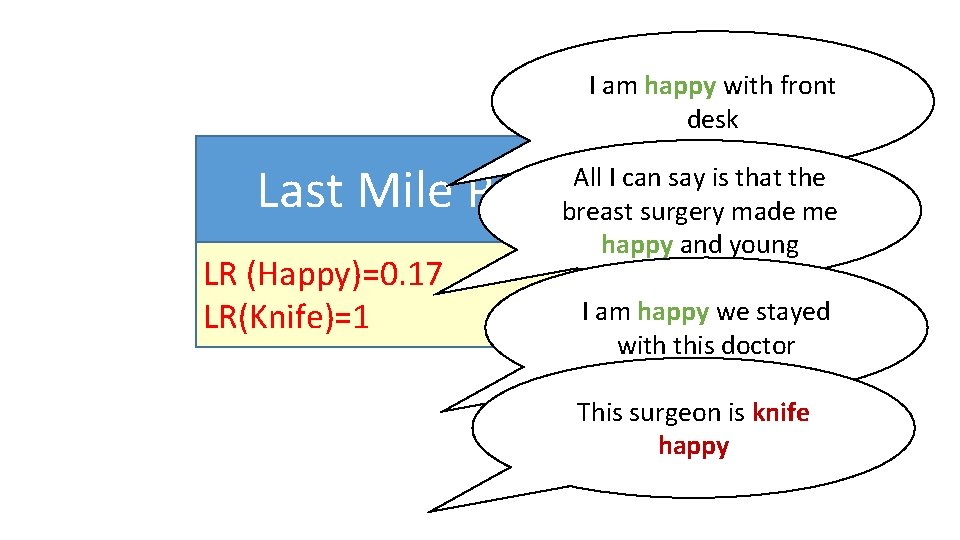
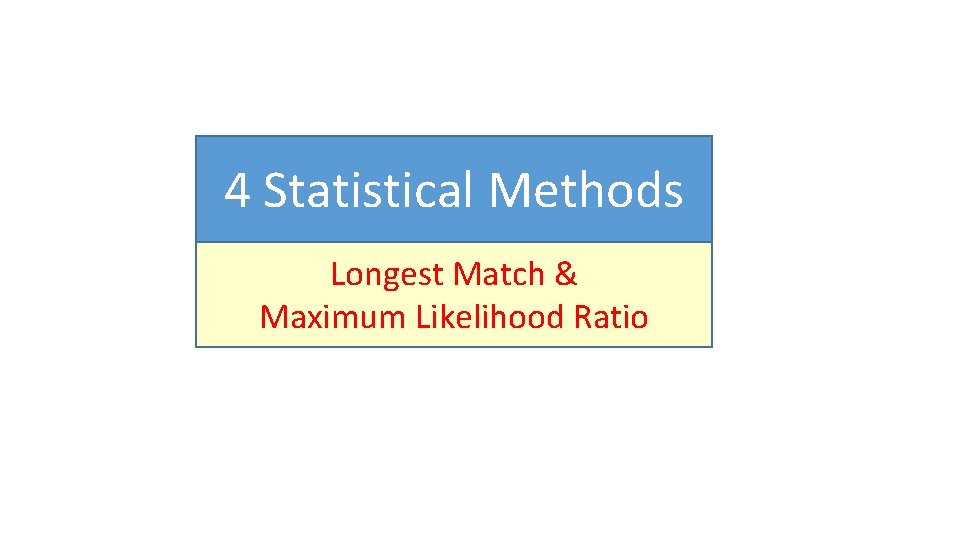
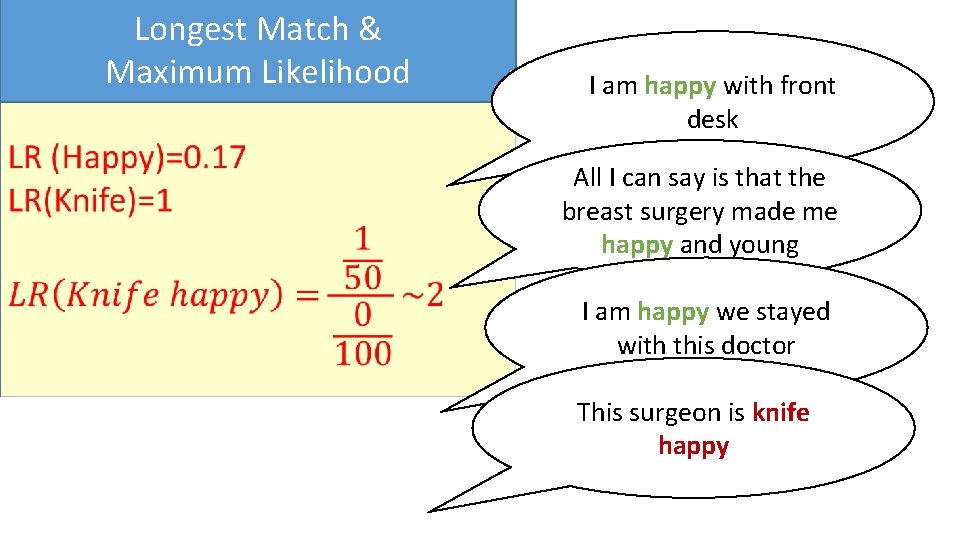
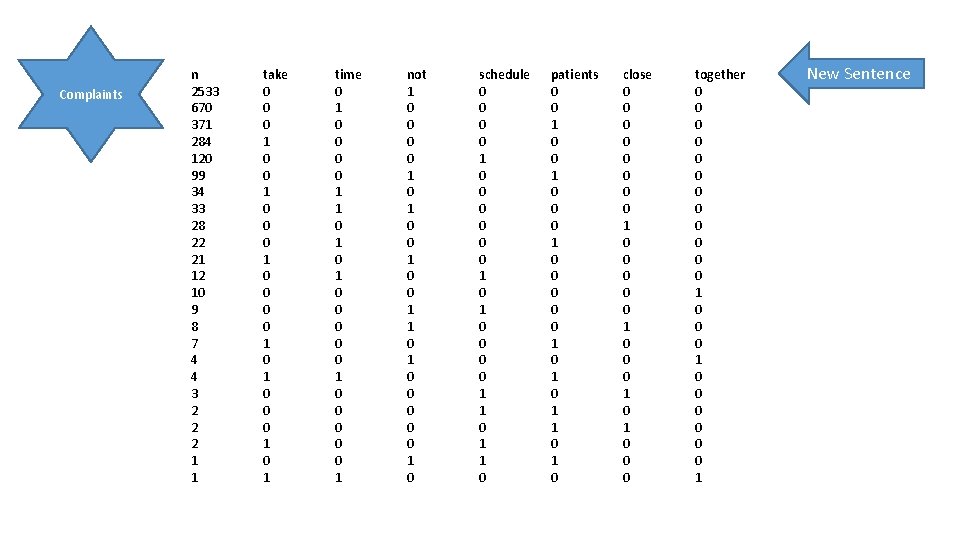
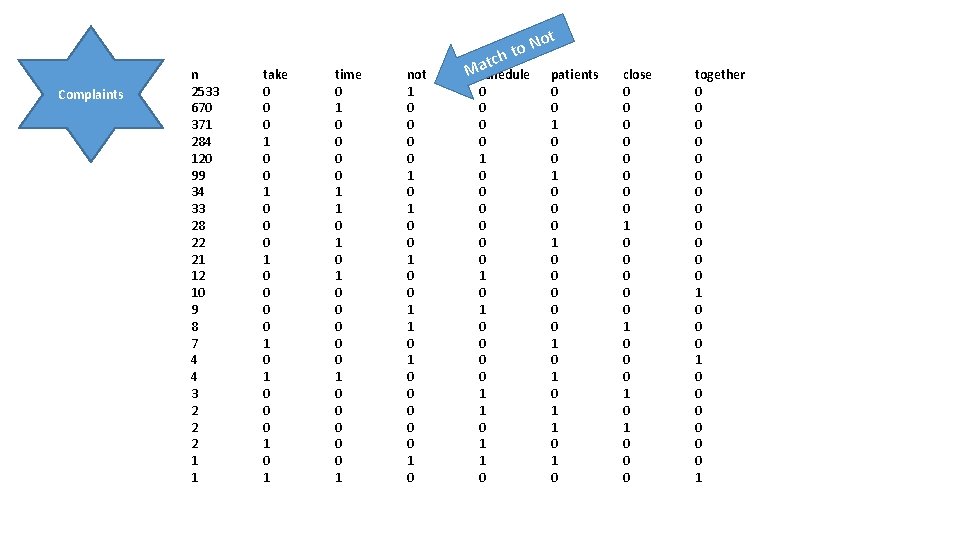
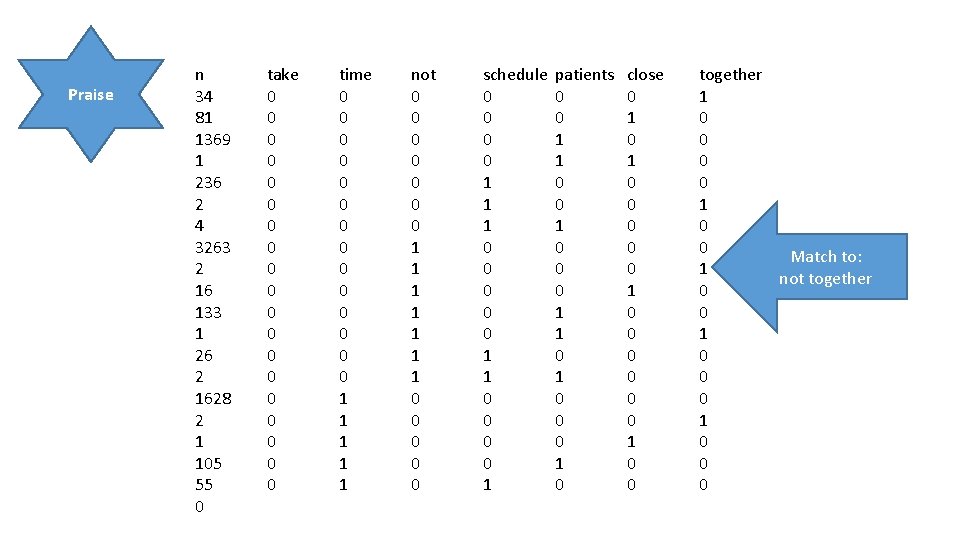
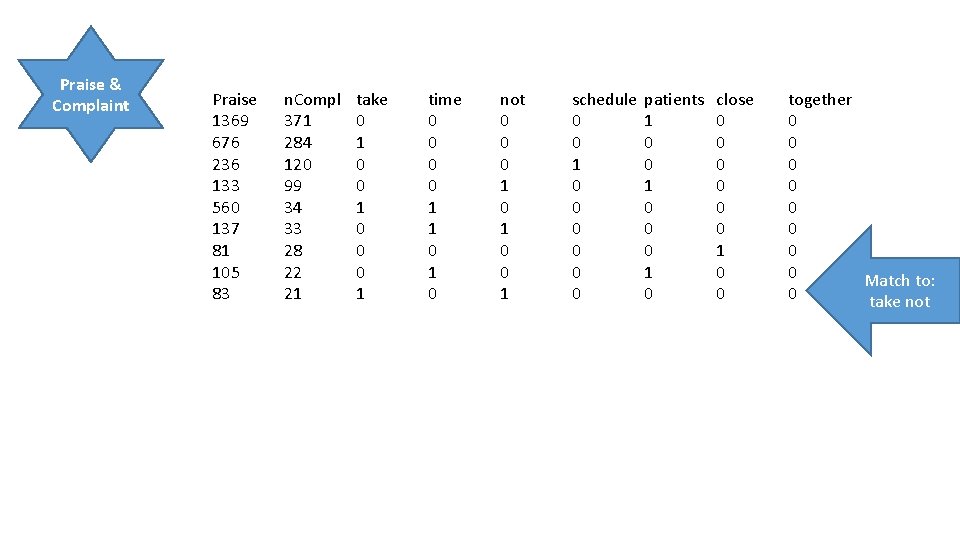
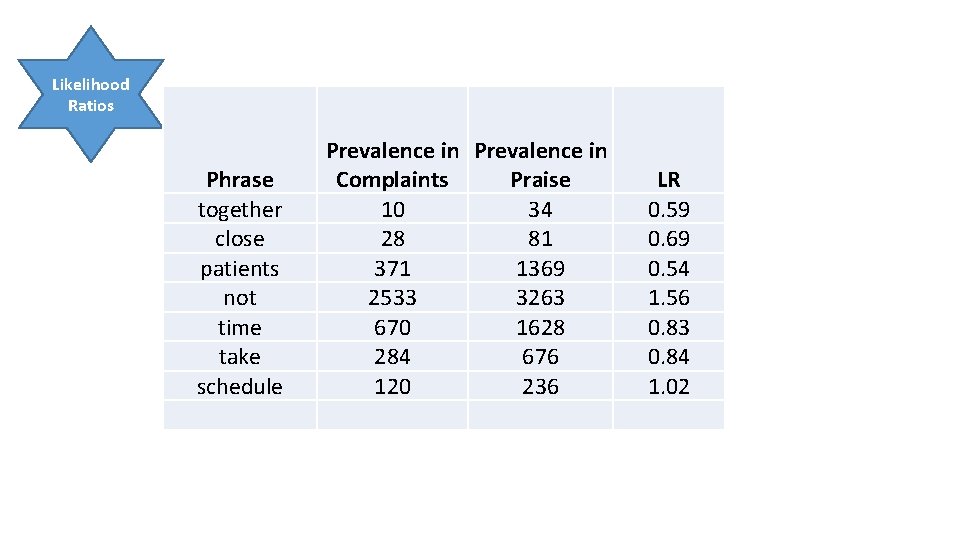
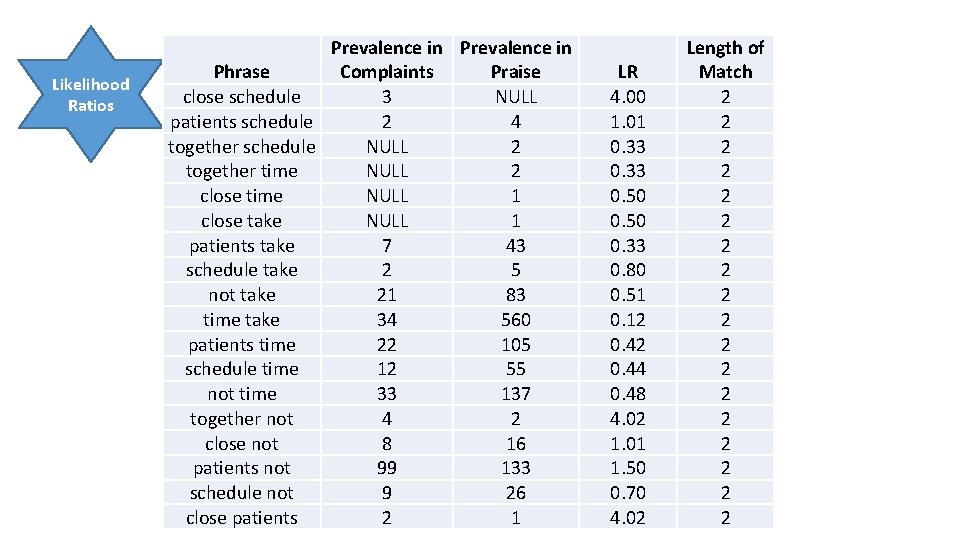
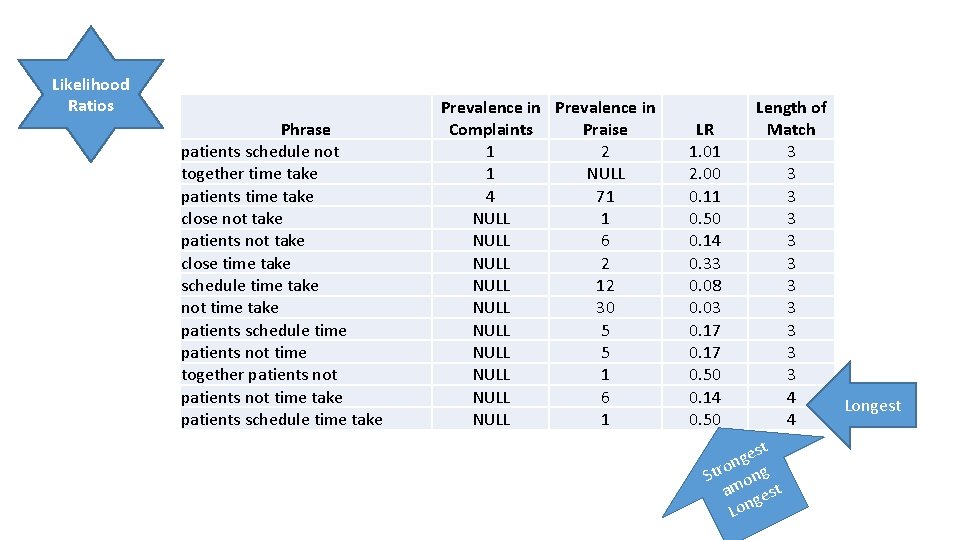
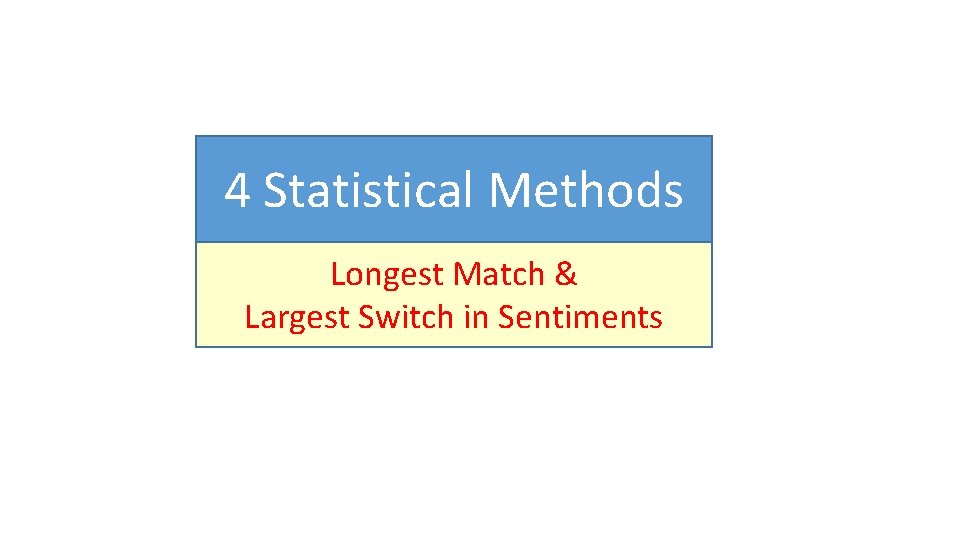
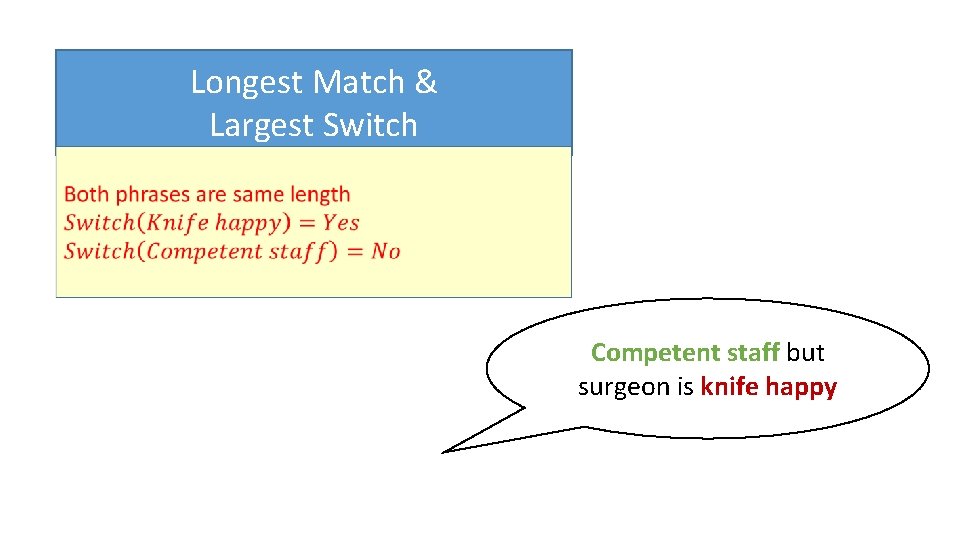
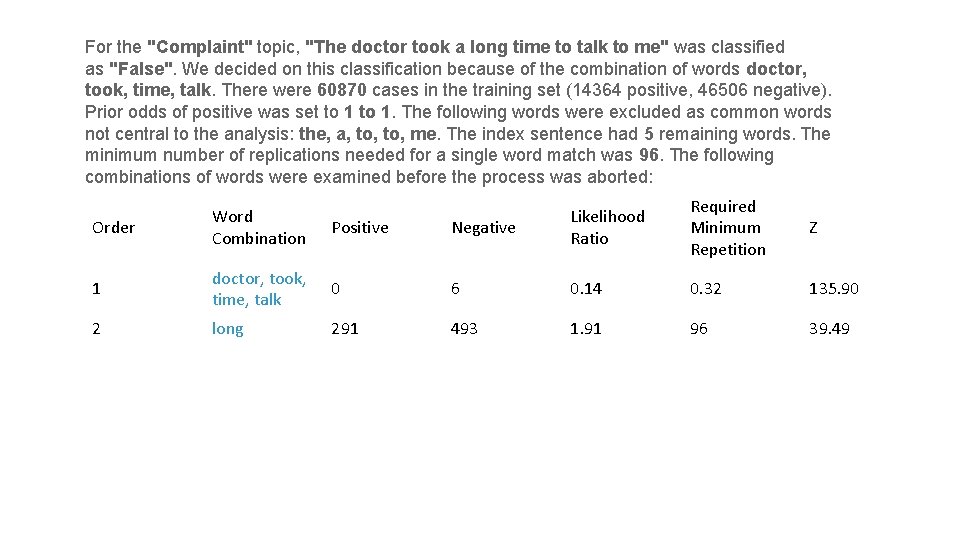
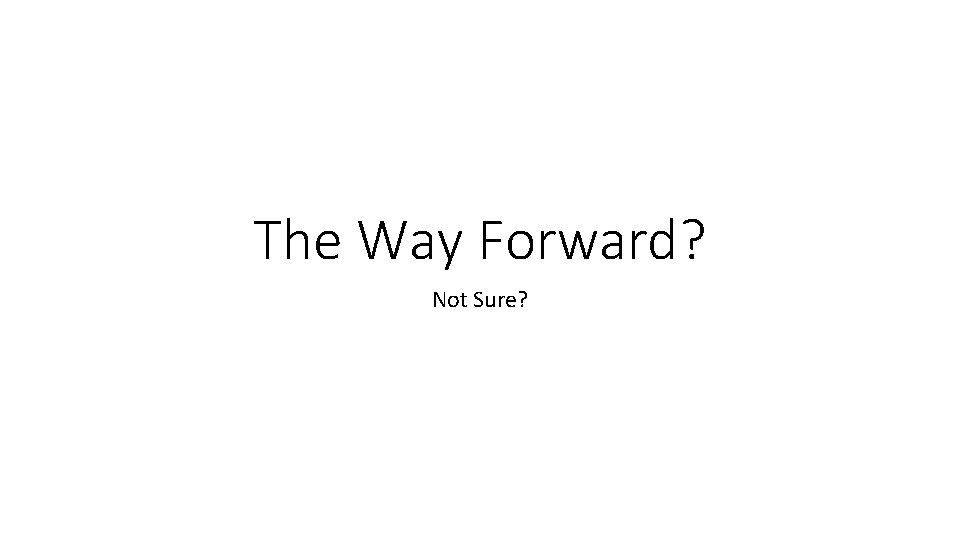
- Slides: 35
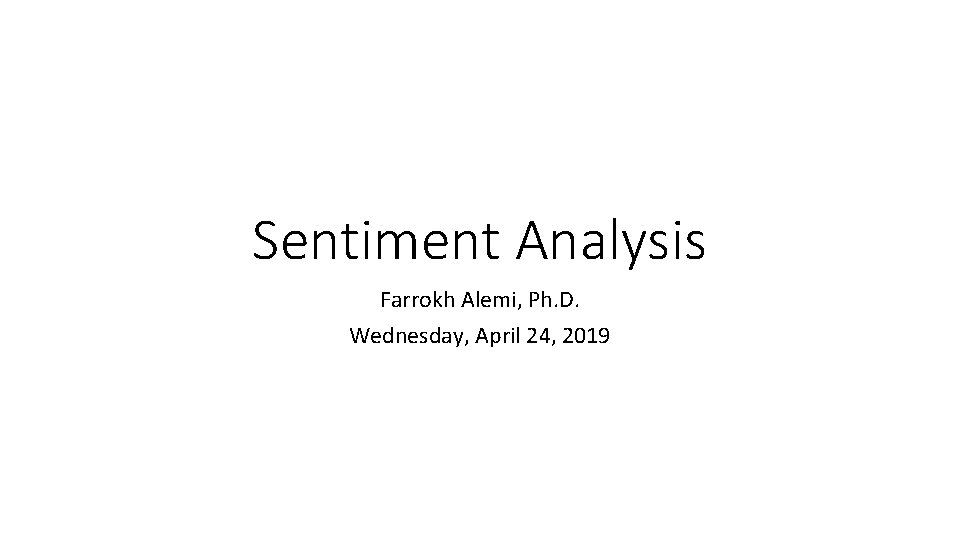
Sentiment Analysis Farrokh Alemi, Ph. D. Wednesday, April 24, 2019
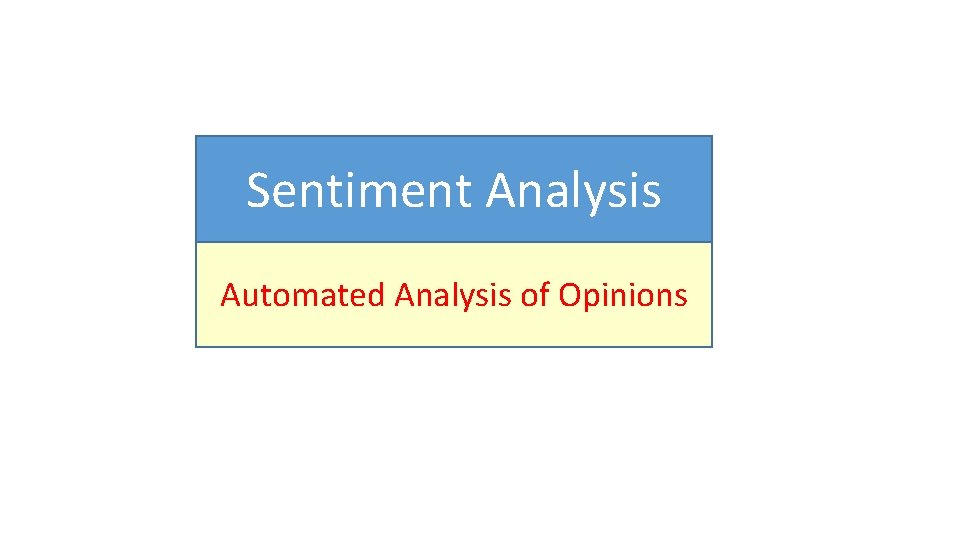
Sentiment Analysis Automated Analysis of Opinions
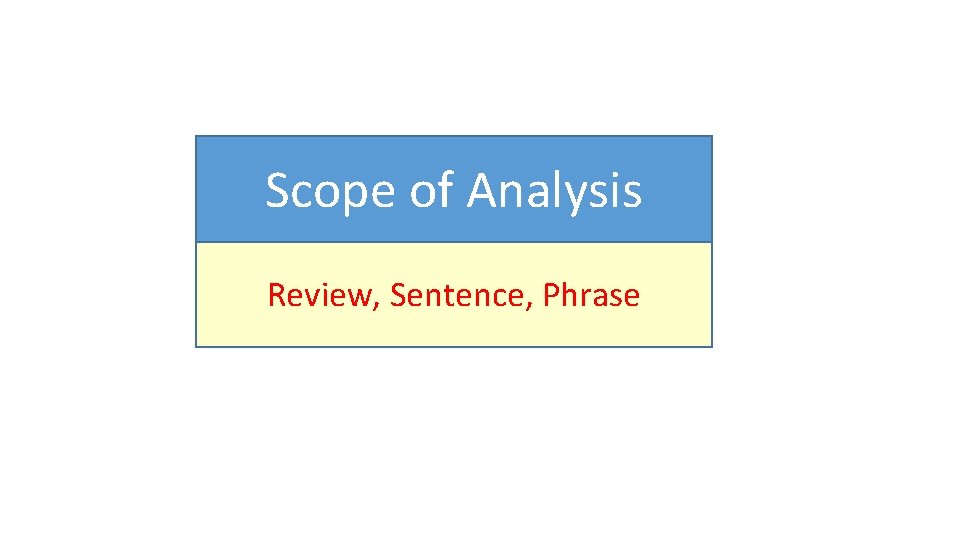
Scope of Analysis Review, Sentence, Phrase
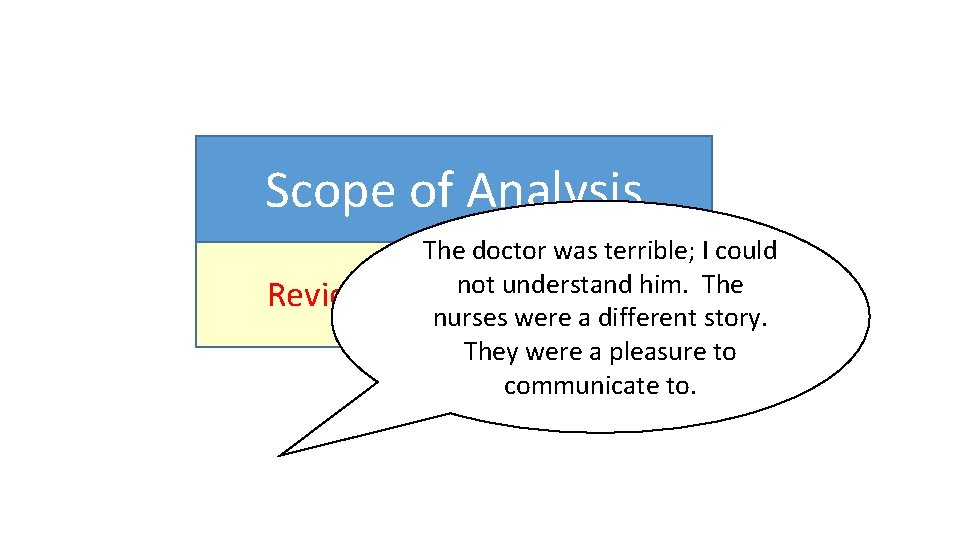
Scope of Analysis The doctor was terrible; I could not understand him. The Review, Sentence, Phrase nurses were a different story. They were a pleasure to communicate to.
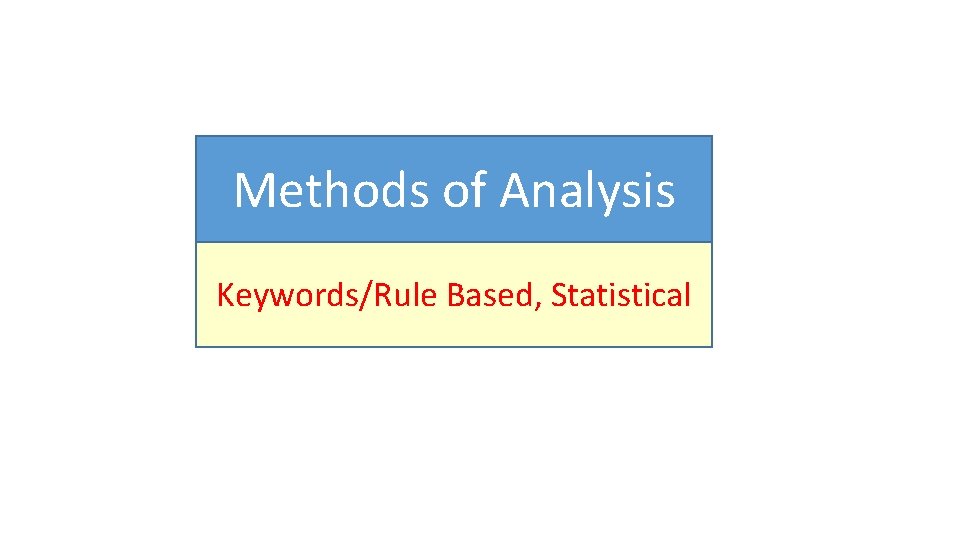
Methods of Analysis Keywords/Rule Based, Statistical
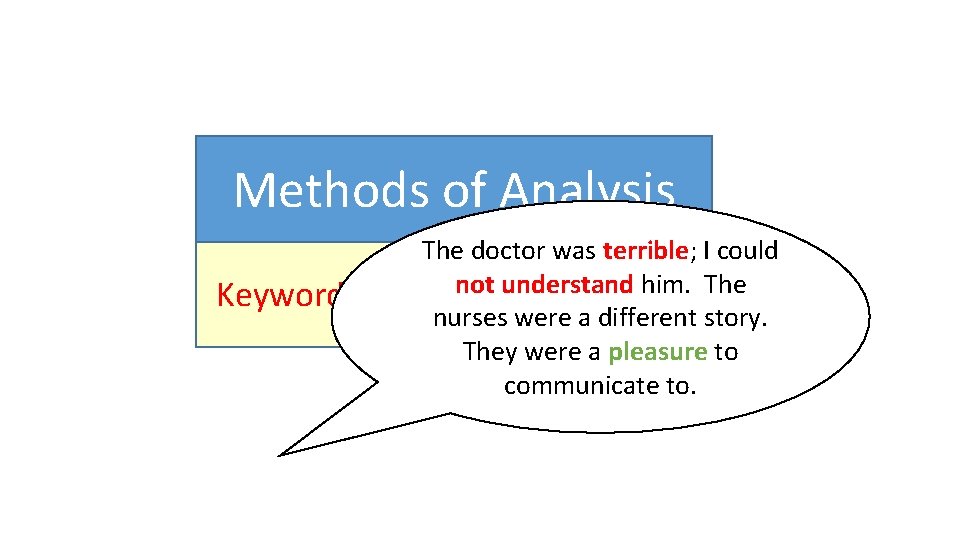
Methods of Analysis The doctor was terrible; I could not understand him. The Keywords/Rule Based, Statistical nurses were a different story. They were a pleasure to communicate to.
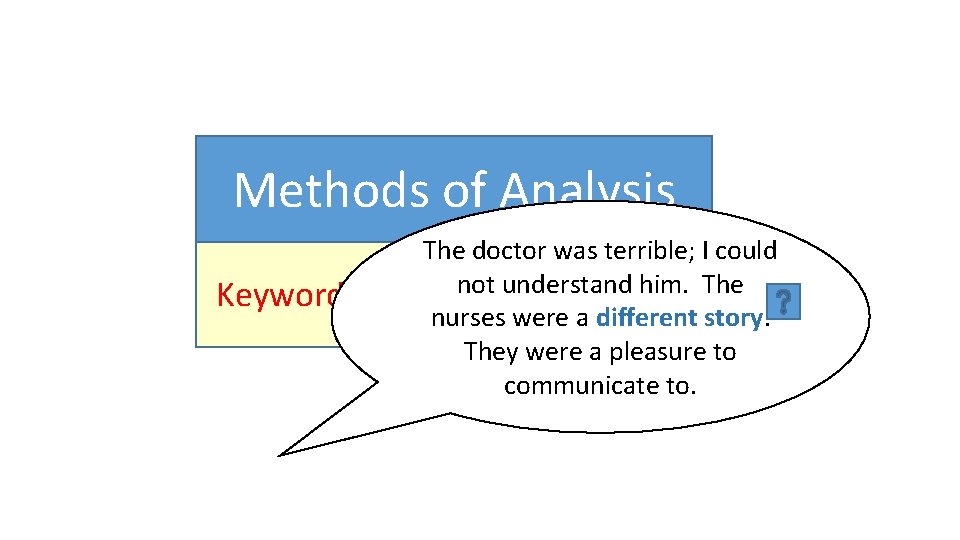
Methods of Analysis The doctor was terrible; I could not understand him. The Keywords/Rule Based, Statistical nurses were a different story. They were a pleasure to communicate to.
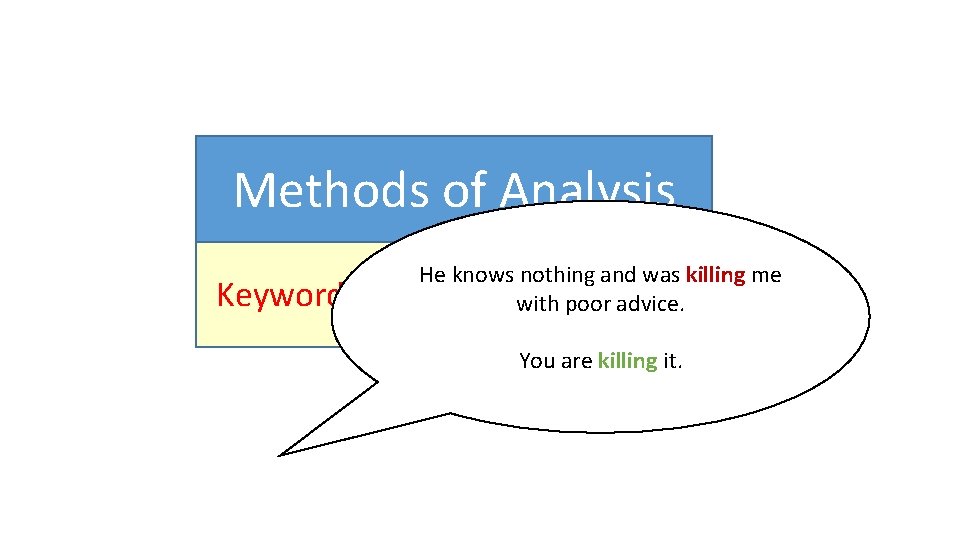
Methods of Analysis He knows nothing and was killing me Keywords/Rule Based, Statistical with poor advice. You are killing it.
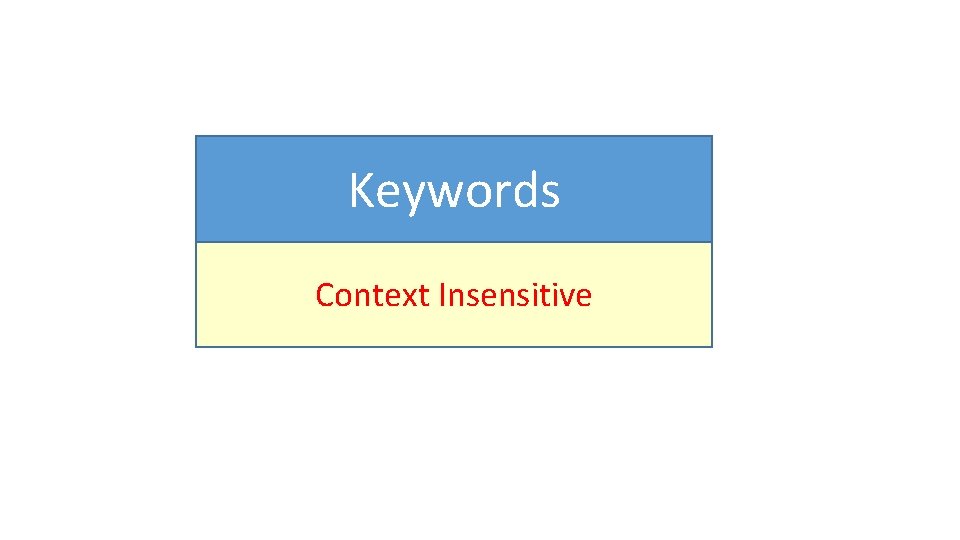
Keywords Context Insensitive
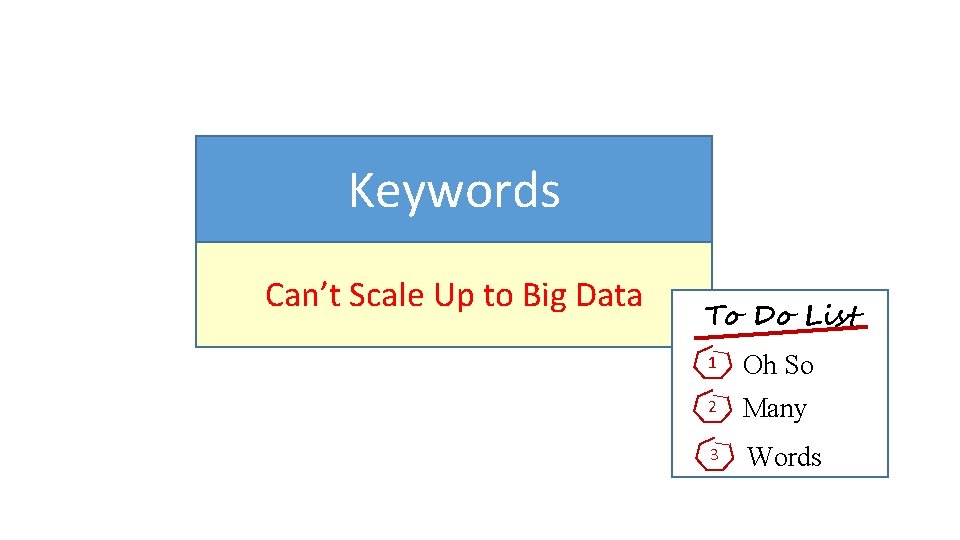
Keywords Can’t Scale Up to Big Data To Do List 1 Oh So 2 Many 3 Words
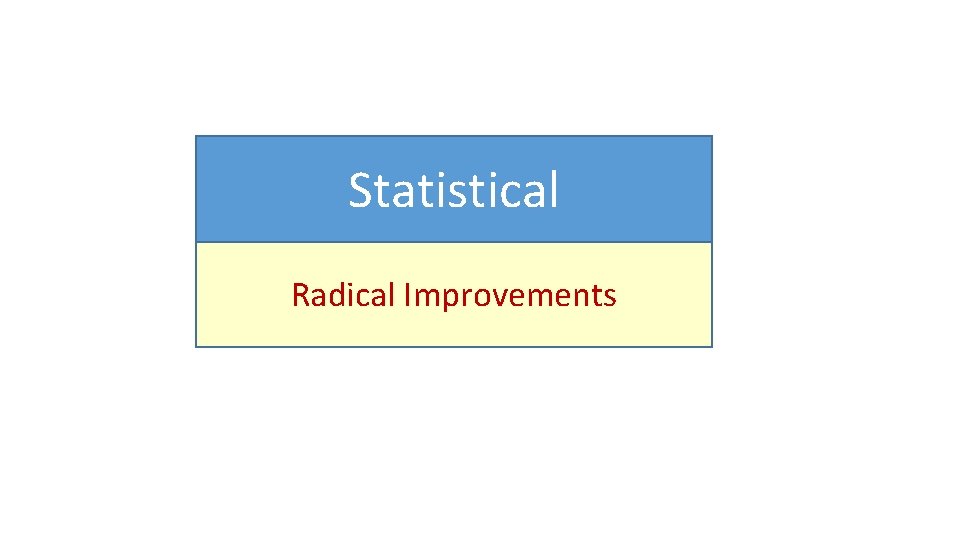
Statistical Radical Improvements
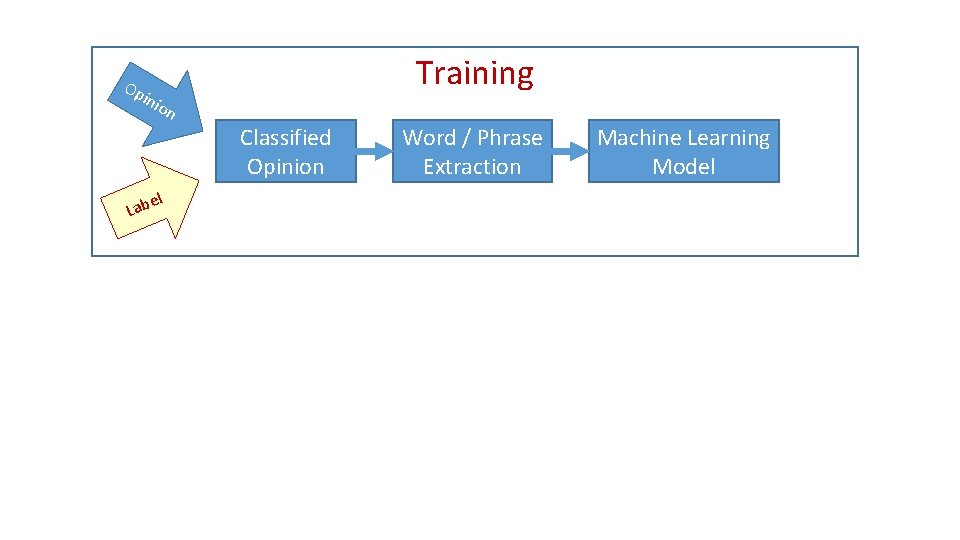
Op Training ini on Classified Opinion l e Lab Word / Phrase Extraction Machine Learning Model
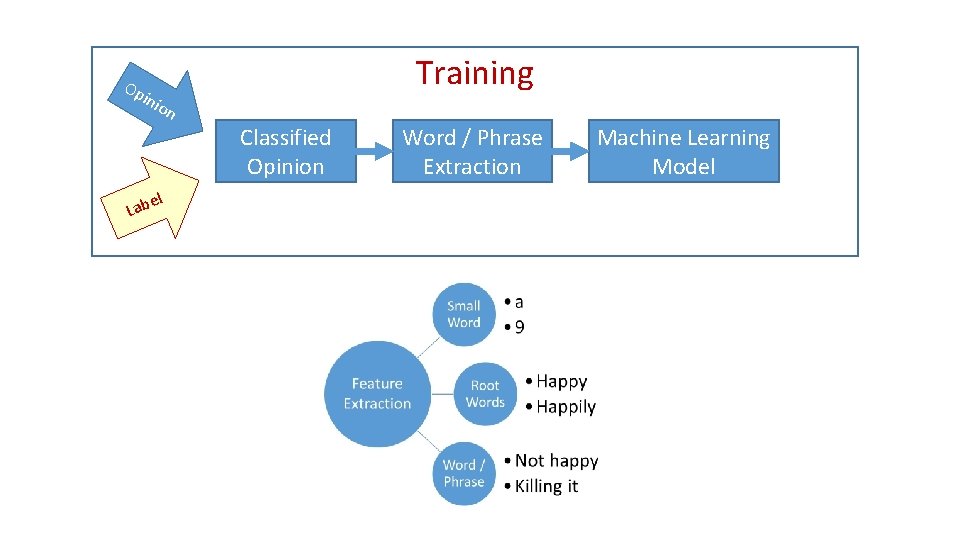
Op Training ini on Classified Opinion l e Lab Word / Phrase Extraction Machine Learning Model
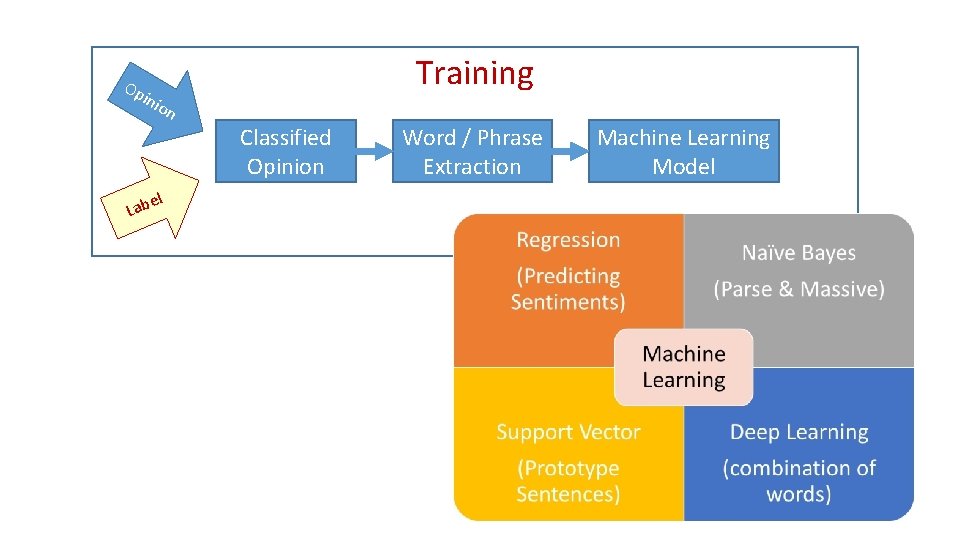
Op Training ini on Classified Opinion l e Lab Word / Phrase Extraction Machine Learning Model
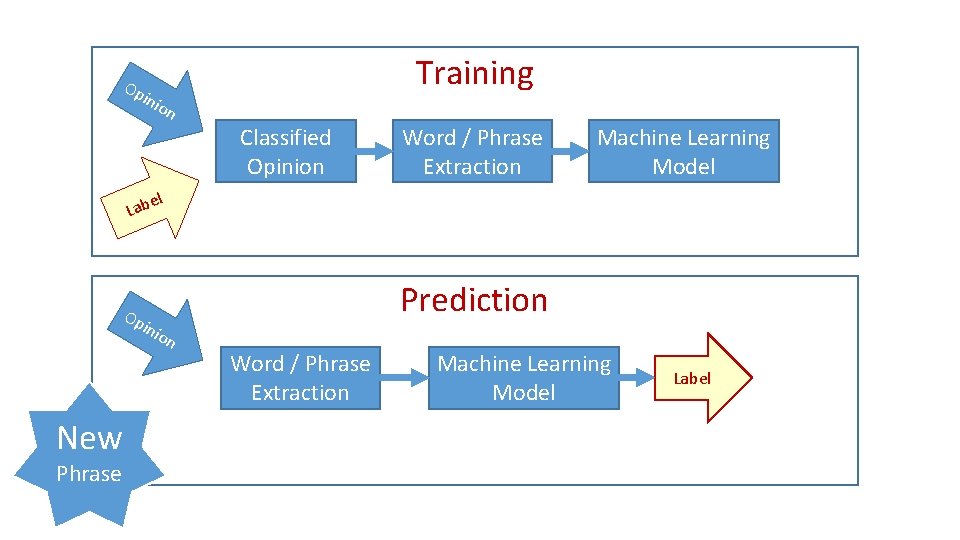
Op Training ini on Classified Opinion Word / Phrase Extraction Machine Learning Model l e Lab Op Prediction ini New Phrase on Word / Phrase Extraction Machine Learning Model Label
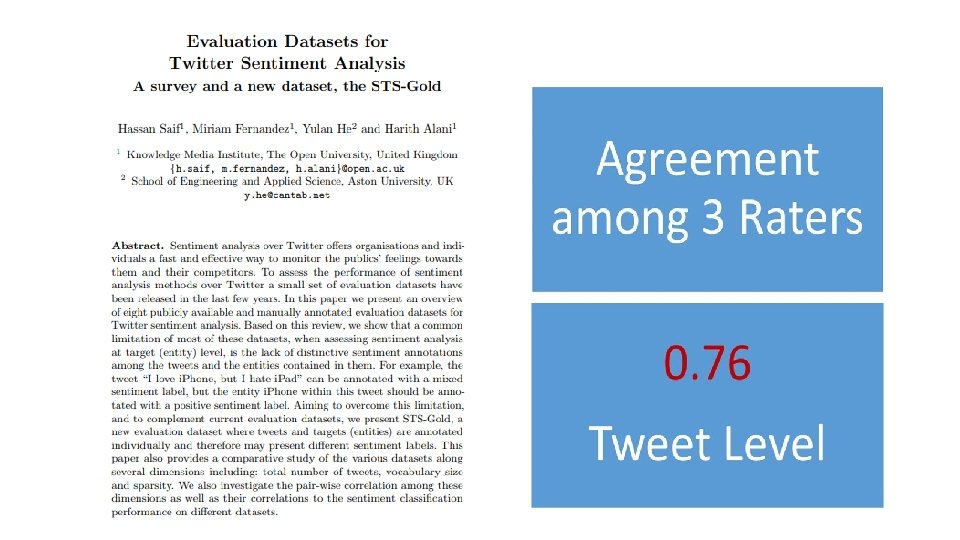
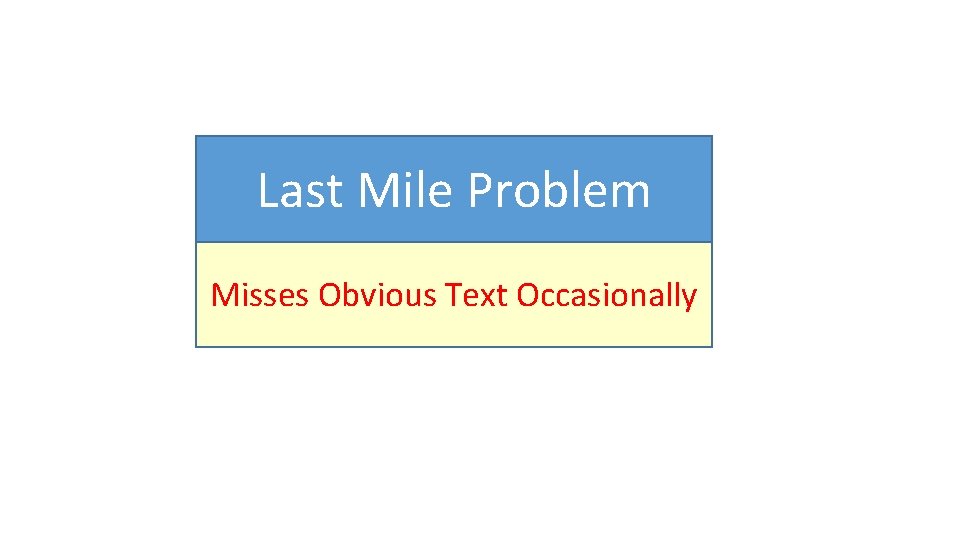
Last Mile Problem Misses Obvious Text Occasionally
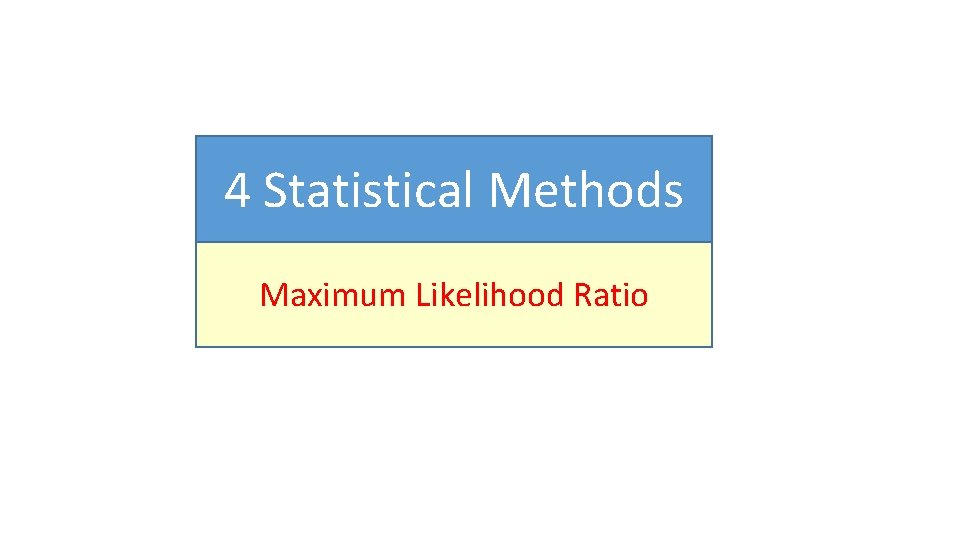
4 Statistical Methods Maximum Likelihood Ratio
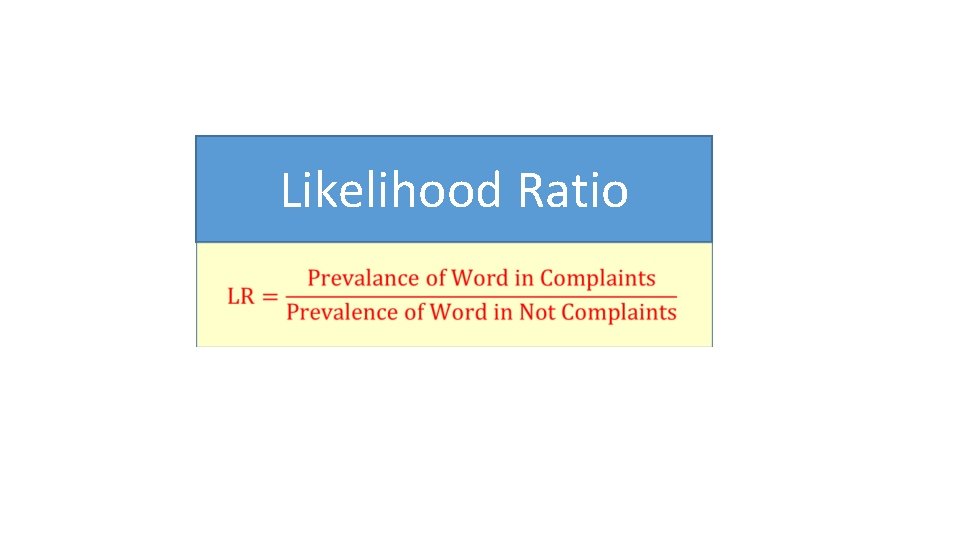
Likelihood Ratio
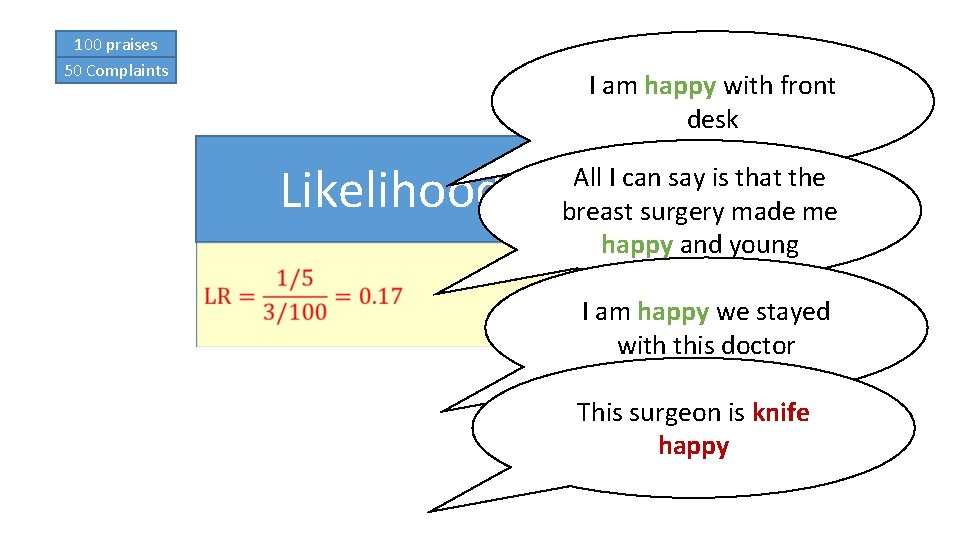
100 praises 50 Complaints I am happy with front desk Likelihood Ratio All I can say is that the breast surgery made me happy and young I am happy we stayed with this doctor This surgeon is knife happy
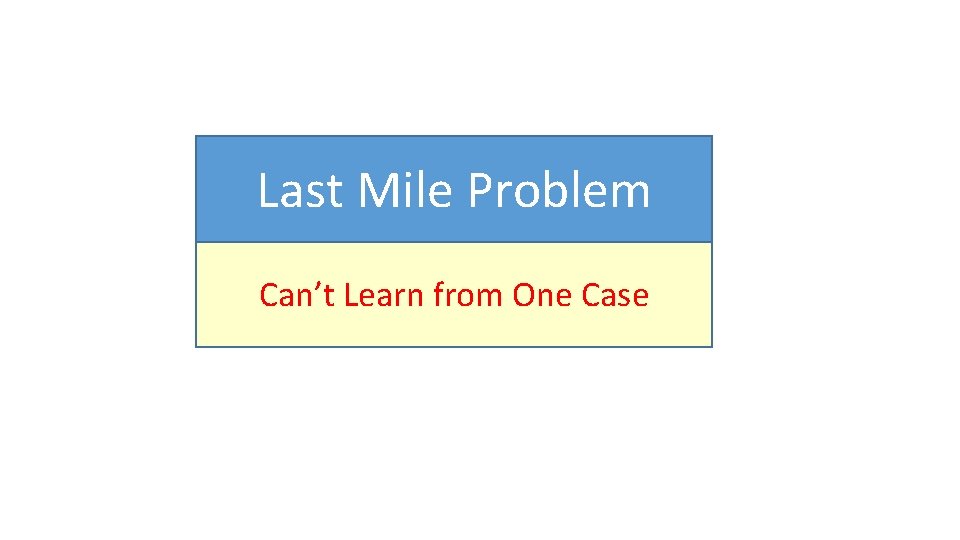
Last Mile Problem Can’t Learn from One Case
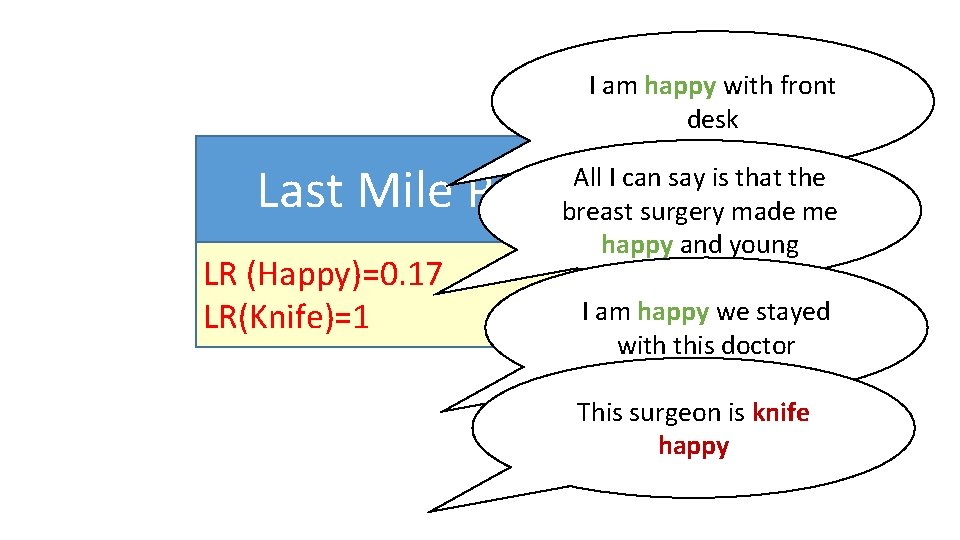
I am happy with front desk Last Mile Problem LR (Happy)=0. 17 LR(Knife)=1 All I can say is that the breast surgery made me happy and young I am happy we stayed with this doctor This surgeon is knife happy
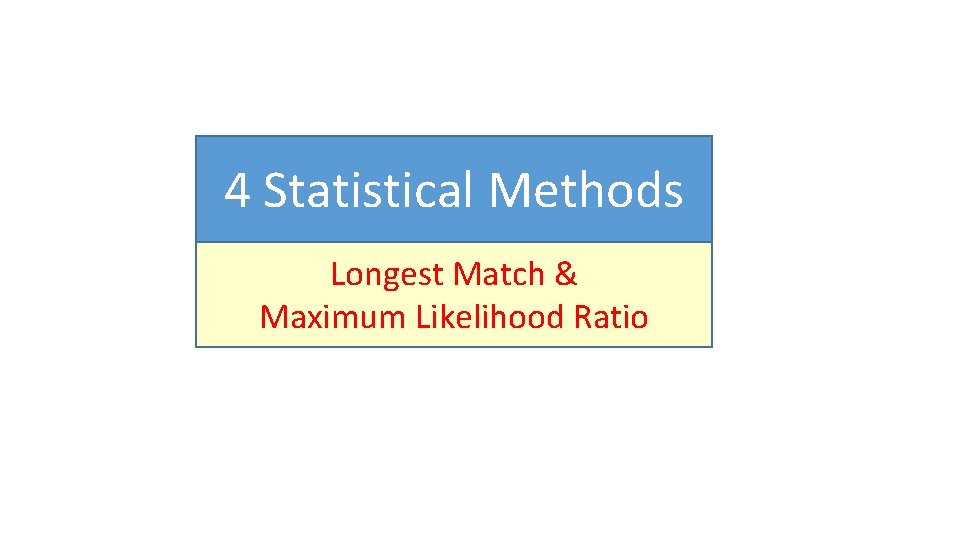
4 Statistical Methods Longest Match & Maximum Likelihood Ratio
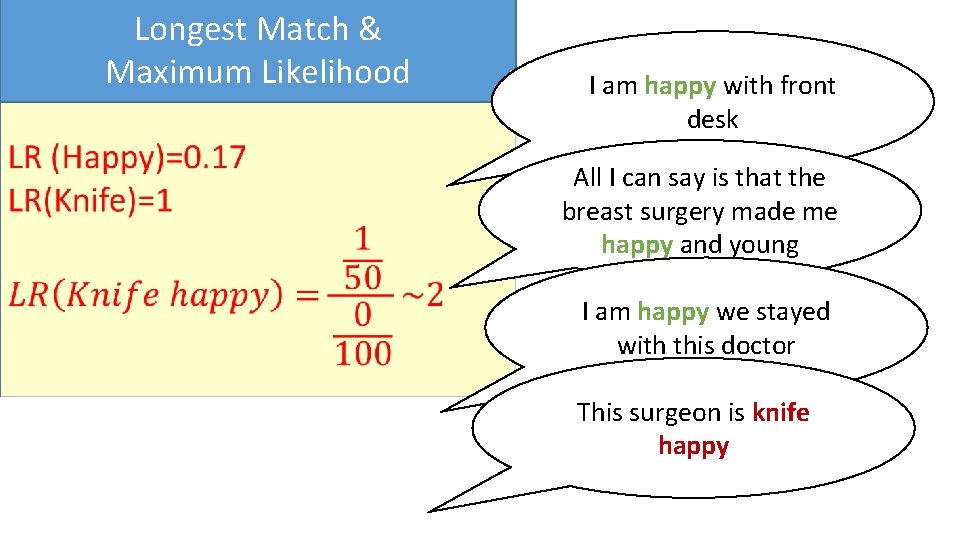
Longest Match & Maximum Likelihood I am happy with front desk All I can say is that the breast surgery made me happy and young I am happy we stayed with this doctor This surgeon is knife happy
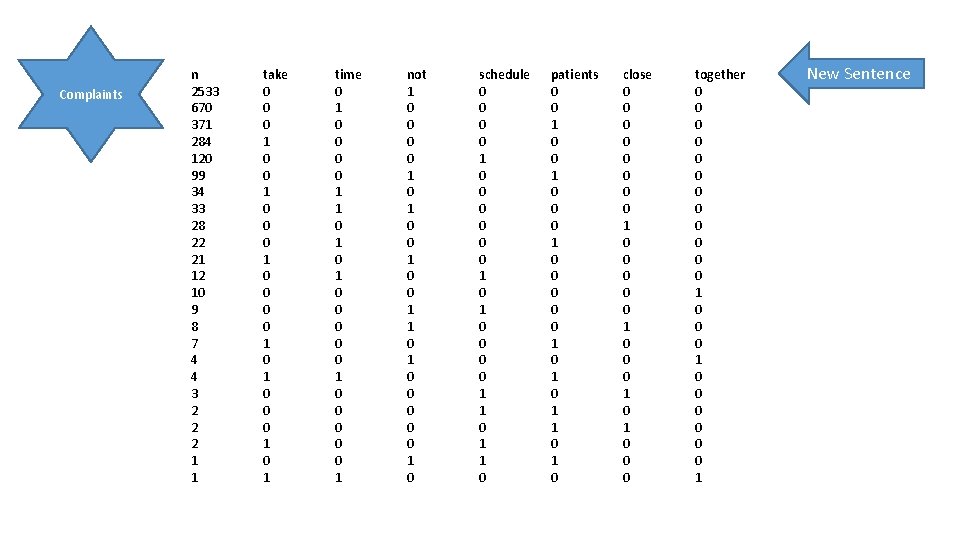
Complaints n 2533 670 371 284 120 99 34 33 28 22 21 12 10 9 8 7 4 4 3 2 2 2 1 1 take 0 0 0 1 0 1 0 0 0 1 time 0 1 0 0 1 1 0 1 0 0 0 0 0 1 not 1 0 0 1 0 0 1 1 0 0 0 1 0 schedule 0 0 1 0 0 0 0 1 1 0 patients 0 0 1 0 0 0 1 0 1 1 0 close 0 0 0 0 1 0 0 0 1 0 0 0 together 0 0 0 0 0 0 1 New Sentence
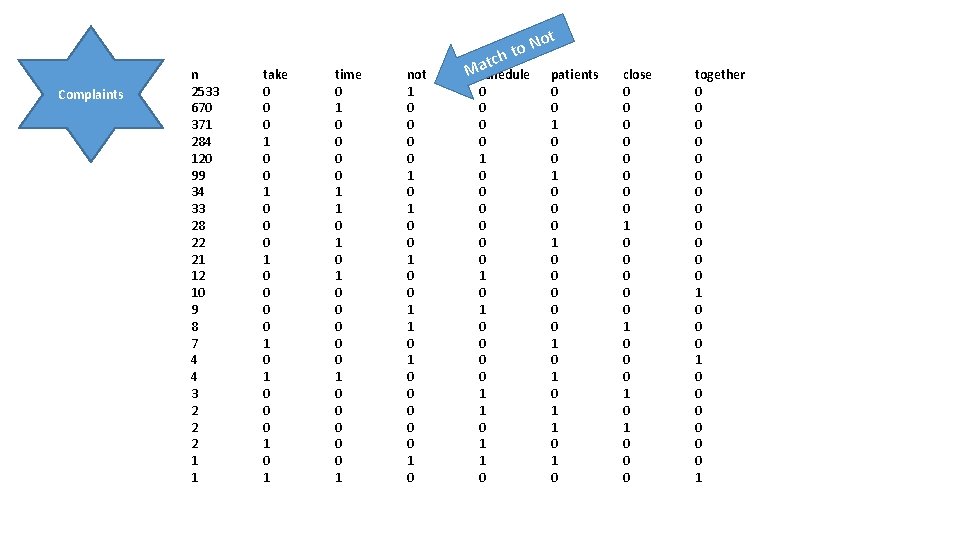
ot Complaints n 2533 670 371 284 120 99 34 33 28 22 21 12 10 9 8 7 4 4 3 2 2 2 1 1 take 0 0 0 1 0 1 0 0 0 1 time 0 1 0 0 1 1 0 1 0 0 0 0 0 1 not 1 0 0 1 0 0 1 1 0 0 0 1 0 o N t h c at Mschedule 0 0 1 0 0 0 0 1 1 0 patients 0 0 1 0 0 0 1 0 1 1 0 close 0 0 0 0 1 0 0 0 1 0 0 0 together 0 0 0 0 0 0 1
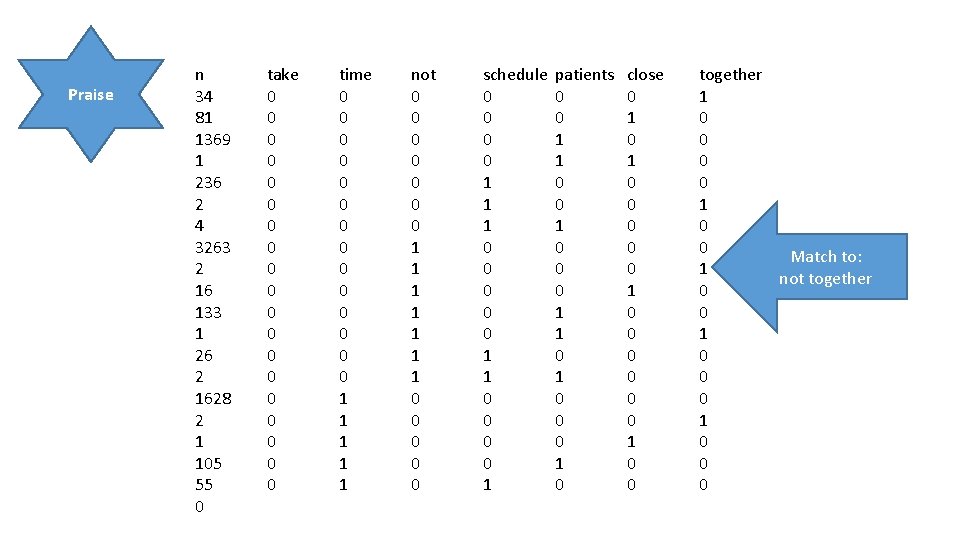
Praise n 34 81 1369 1 236 2 4 3263 2 16 133 1 26 2 1628 2 1 105 55 0 take 0 0 0 0 0 time 0 0 0 0 1 1 1 not 0 0 0 0 1 1 1 1 0 0 0 schedule 0 0 1 1 1 0 0 0 1 1 0 0 1 patients 0 0 1 1 0 0 0 1 0 close 0 1 0 0 0 0 0 1 0 0 together 1 0 0 Match to: 1 not together 0 0 1 0 0 0
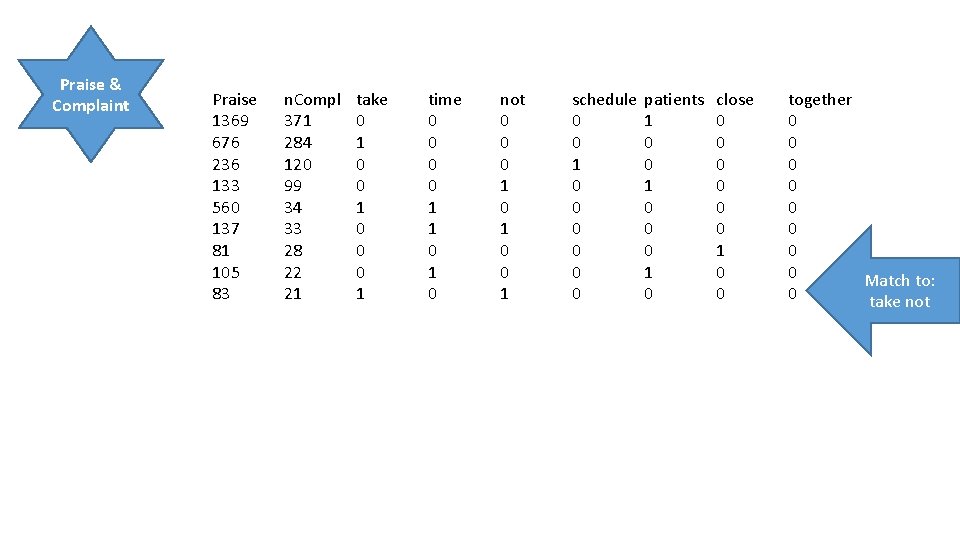
Praise & Complaint Praise 1369 676 236 133 560 137 81 105 83 n. Compl 371 284 120 99 34 33 28 22 21 take 0 1 0 0 0 1 time 0 0 1 1 0 not 0 0 0 1 schedule 0 0 1 0 0 0 patients 1 0 0 0 1 0 close 0 0 0 1 0 0 together 0 0 0 0 Match to: 0 take not
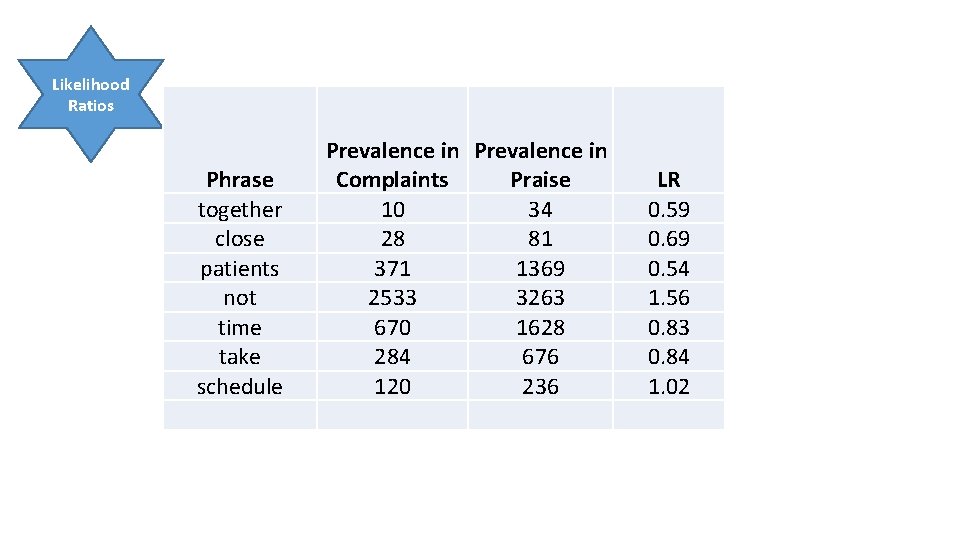
Likelihood Ratios Phrase together close patients not time take schedule Prevalence in Complaints Praise 10 34 28 81 371 1369 2533 3263 670 1628 284 676 120 236 LR 0. 59 0. 69 0. 54 1. 56 0. 83 0. 84 1. 02
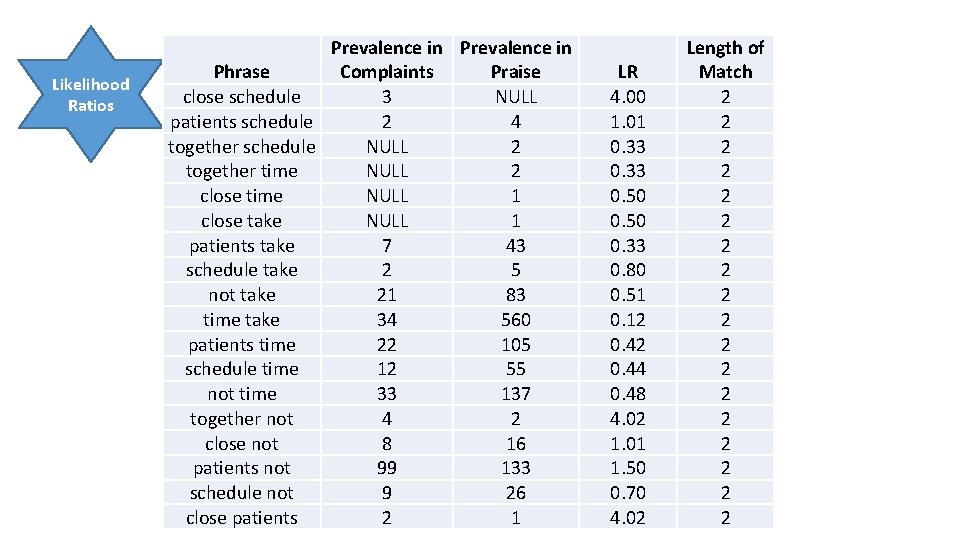
Likelihood Ratios Prevalence in Phrase Complaints Praise close schedule 3 NULL patients schedule 2 4 together schedule NULL 2 together time NULL 2 close time NULL 1 close take NULL 1 patients take 7 43 schedule take 2 5 not take 21 83 time take 34 560 patients time 22 105 schedule time 12 55 not time 33 137 together not 4 2 close not 8 16 patients not 99 133 schedule not 9 26 close patients 2 1 LR 4. 00 1. 01 0. 33 0. 50 0. 33 0. 80 0. 51 0. 12 0. 44 0. 48 4. 02 1. 01 1. 50 0. 70 4. 02 Length of Match 2 2 2 2 2
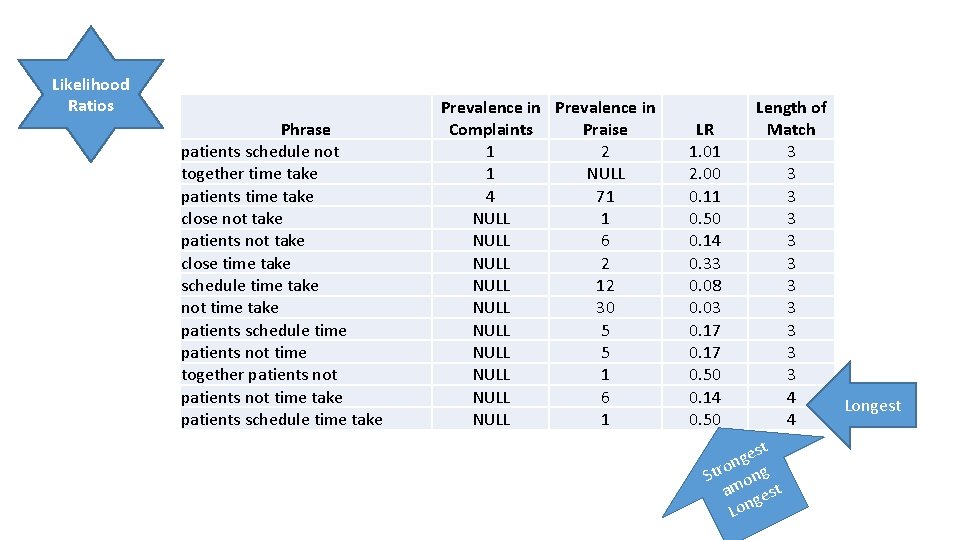
Likelihood Ratios Phrase patients schedule not together time take patients time take close not take patients not take close time take schedule time take not time take patients schedule time patients not time together patients not time take patients schedule time take Prevalence in Complaints Praise 1 2 1 NULL 4 71 NULL 6 NULL 2 NULL 12 NULL 30 NULL 5 NULL 1 NULL 6 NULL 1 Length of LR Match 1. 01 3 2. 00 3 0. 11 3 0. 50 3 0. 14 3 0. 33 3 0. 08 3 0. 03 3 0. 17 3 0. 50 3 0. 14 4 Longest 0. 50 4 t s e ong g r t S on t m a es g n Lo
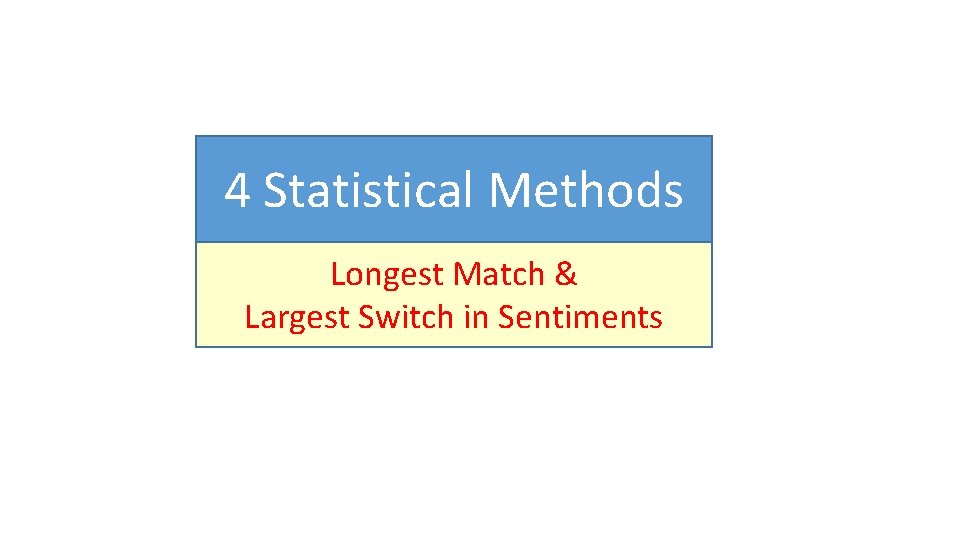
4 Statistical Methods Longest Match & Largest Switch in Sentiments
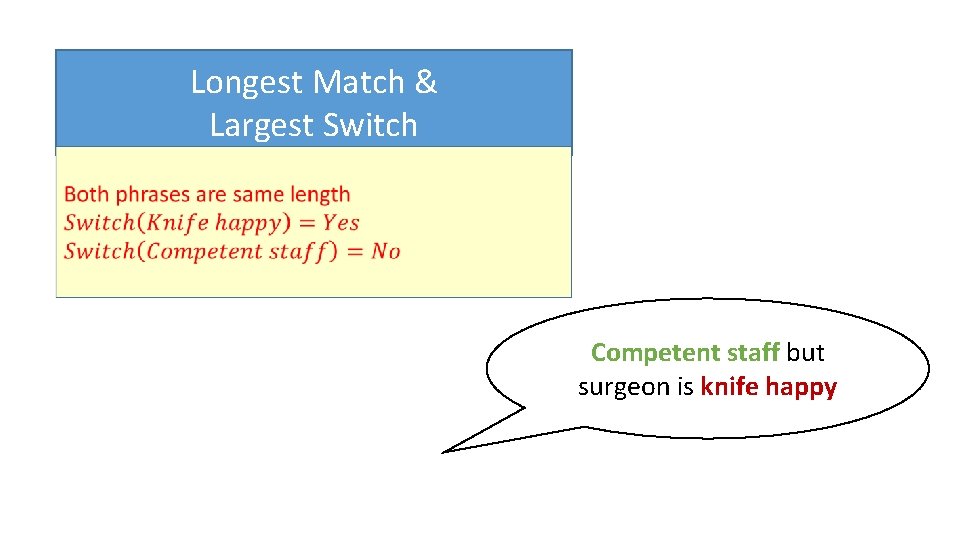
Longest Match & Largest Switch Competent staff but surgeon is knife happy
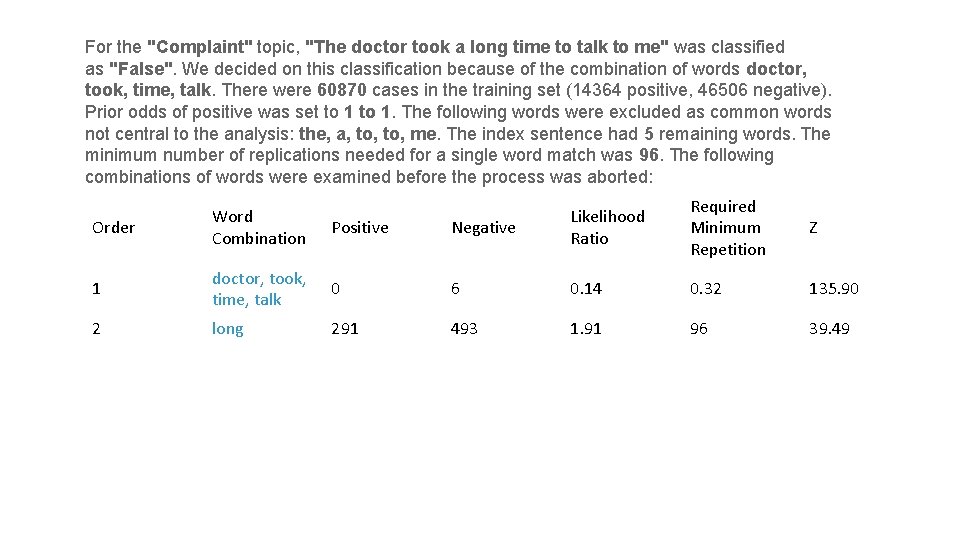
For the "Complaint" topic, "The doctor took a long time to talk to me" was classified as "False". We decided on this classification because of the combination of words doctor, took, time, talk. There were 60870 cases in the training set (14364 positive, 46506 negative). Prior odds of positive was set to 1. The following words were excluded as common words not central to the analysis: the, a, to, me. The index sentence had 5 remaining words. The minimum number of replications needed for a single word match was 96. The following combinations of words were examined before the process was aborted: Negative Likelihood Ratio Required Minimum Repetition Z 0 6 0. 14 0. 32 135. 90 291 493 1. 91 96 39. 49 Order Word Combination Positive 1 doctor, took, time, talk 2 long
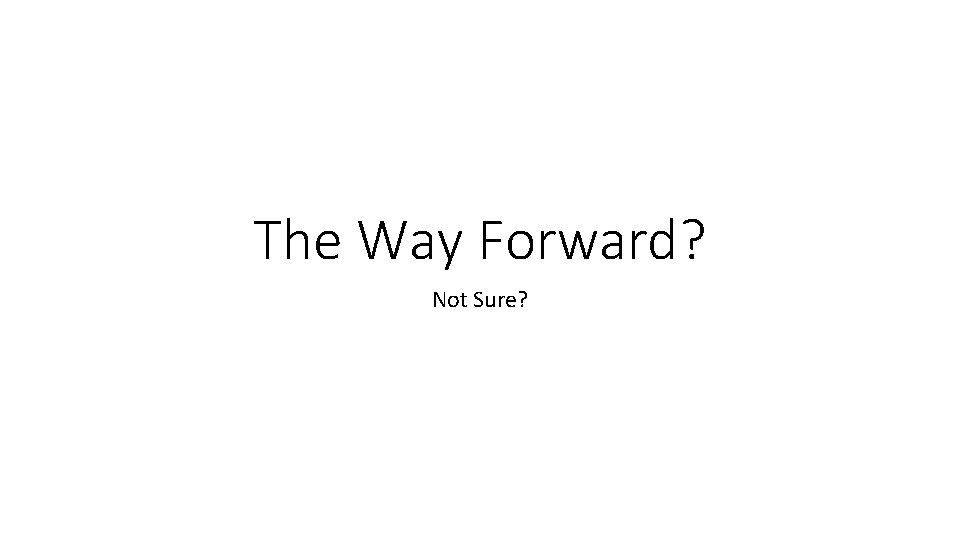
The Way Forward? Not Sure?
Chenghua lin
Albizza
Flora bölgeleri
Cnler
Protsta
Monera alemi
Analysis twitter mdausfishkinsparktoro
W62vr
Subjectivity in sentiment analysis
Aspect based sentiment analysis using bert
Bing liu sentiment analysis
What is nvivo used for
Sentiment analysis tools comparison
Moontae lee
Sentiment analysis for hotel reviews
Turkish sentiment analysis
Turkish sentiment analysis
Azure twitter sentiment analysis
Slide to doc.com
Sentiment analysis with deep learning using bert
Sentiment analysis conclusion
Sentiment analysis
Monday tuesday wednesday thursday friday calendar
Wednesday evening prayer
Wednesday seminar
Ib grades to percentage
Web analytics wednesday
How to write wednesday
Happy wednesday february
Monday my favorite day
Skinny wednesday
Flmsg download
Tuesday wednesday saturday
Happy wednesday
Happy wednesday march
Monday tuesday wednesday thursday friday saturday sunday