Comparisons of kinematical retrievals within a simulated supercell
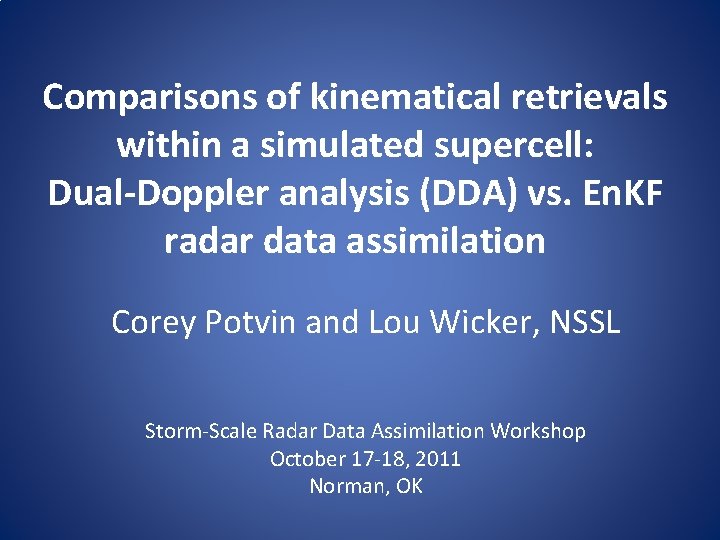
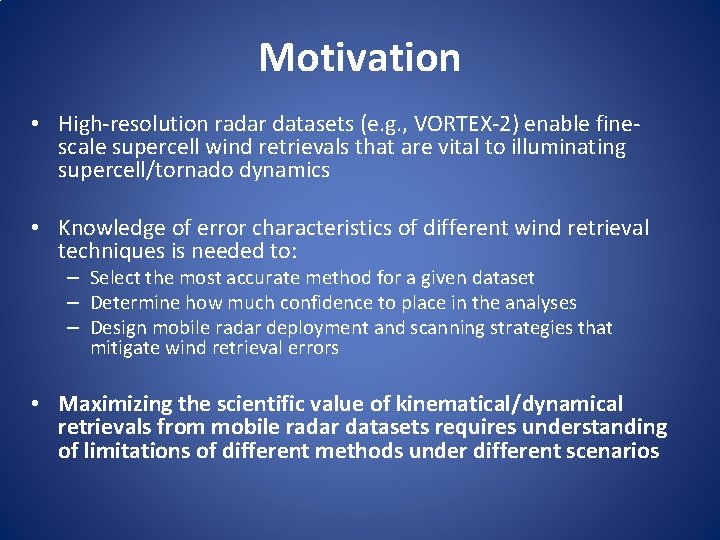
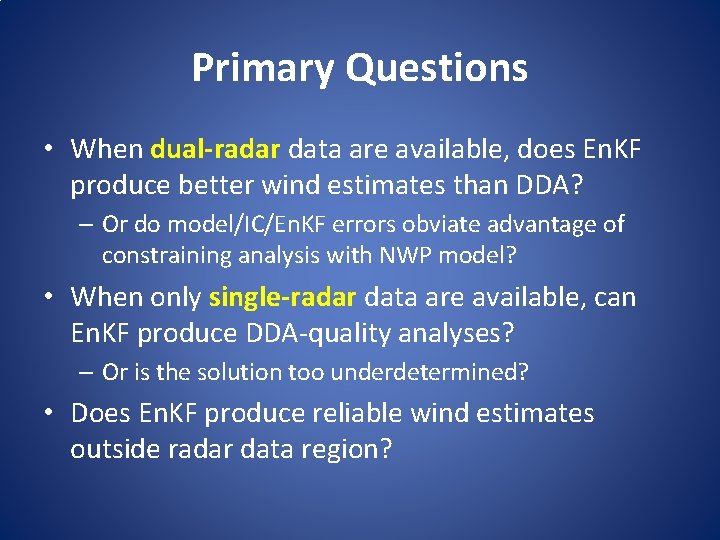
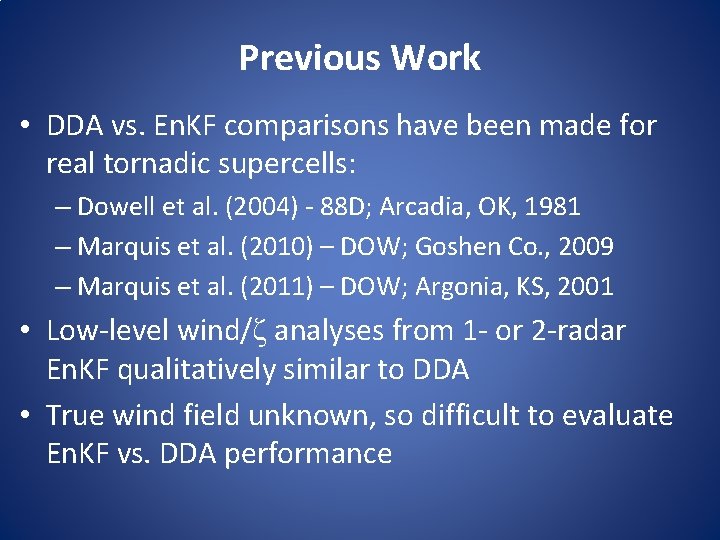
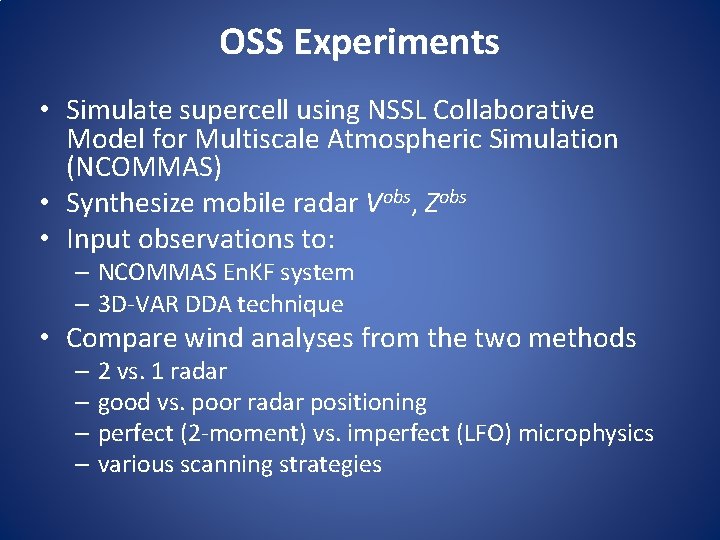
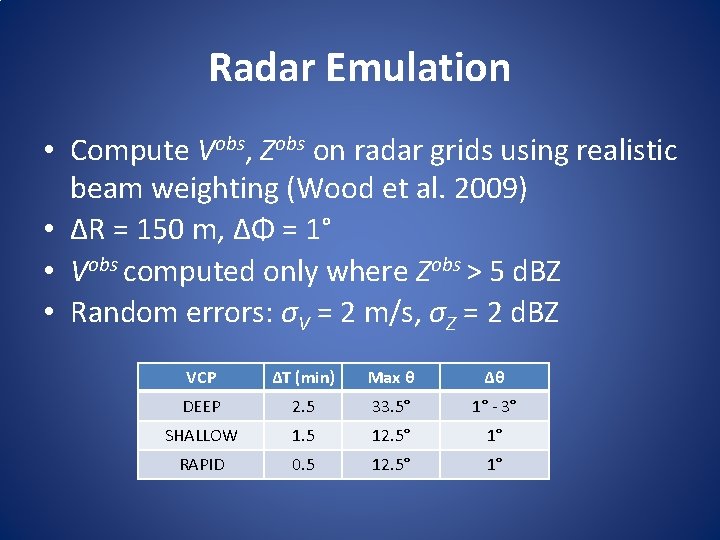
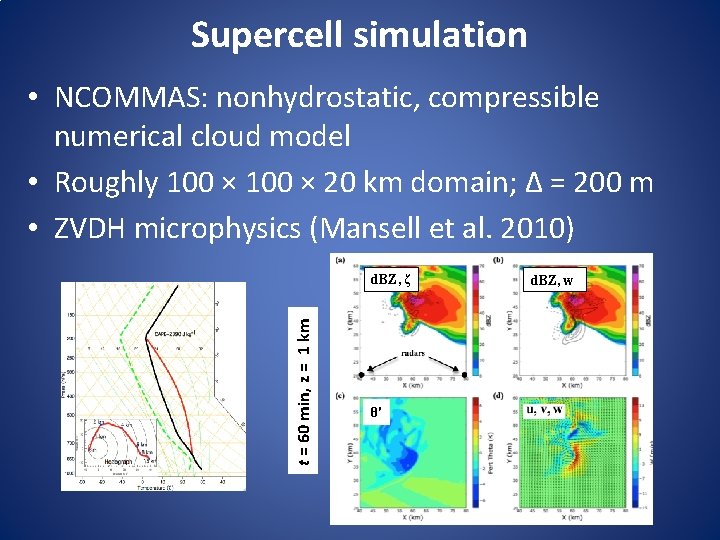
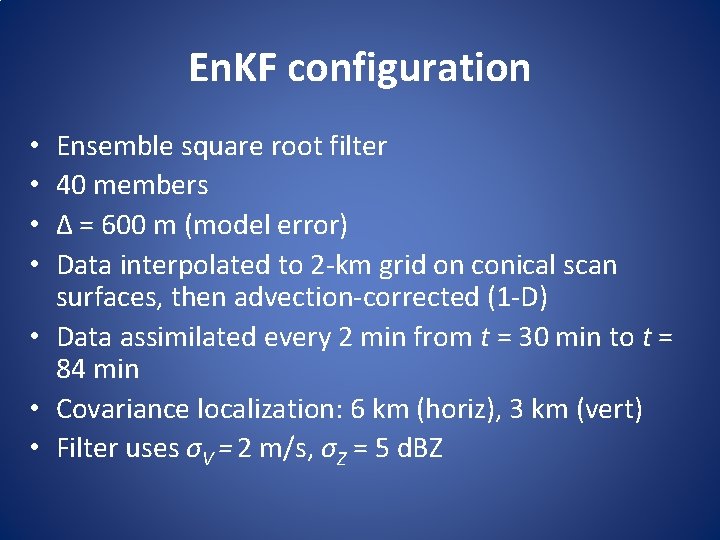
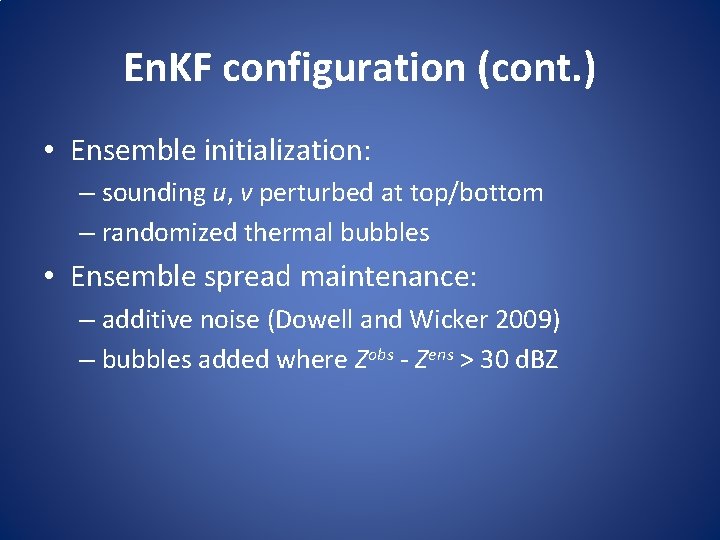
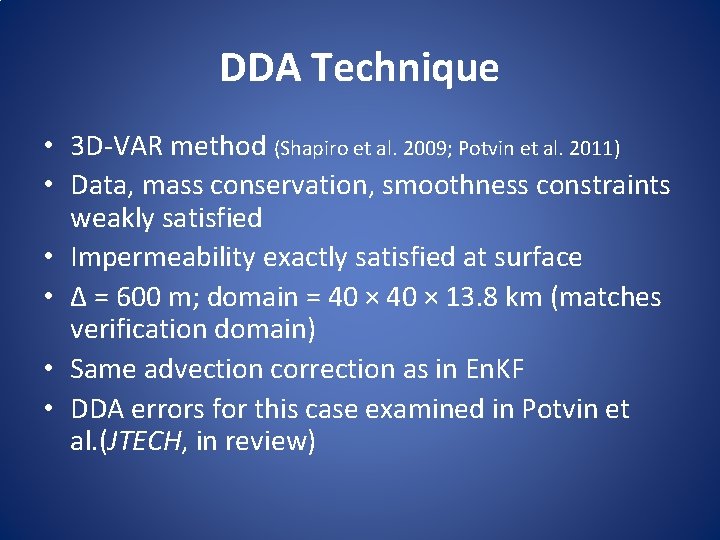
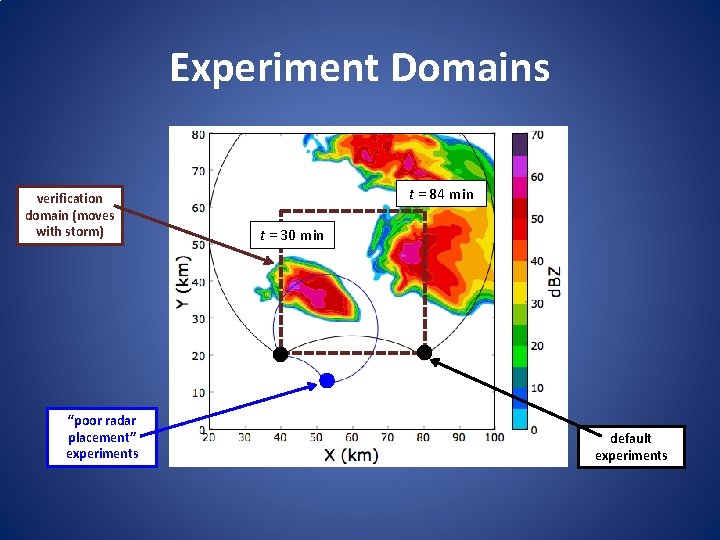
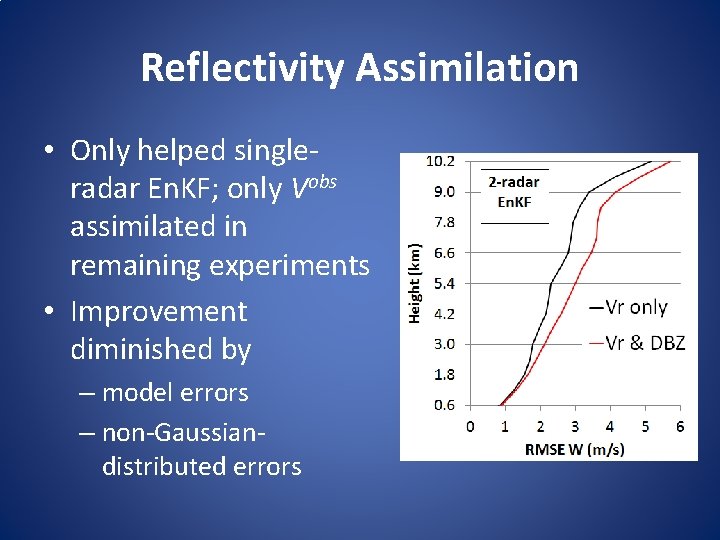
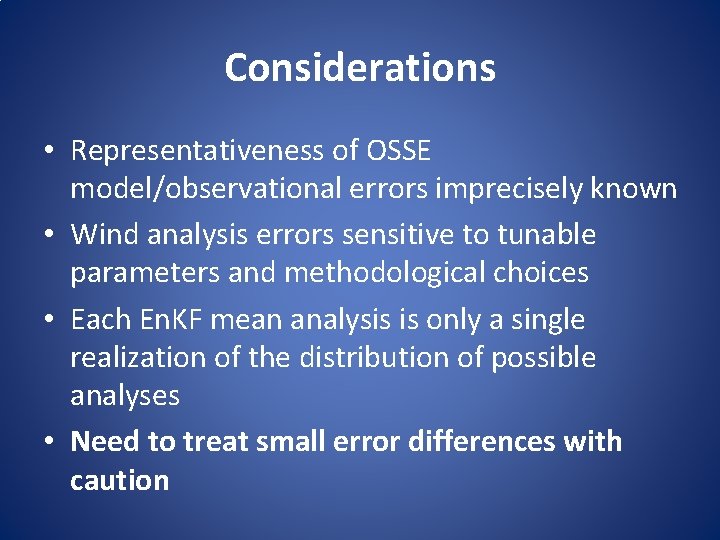
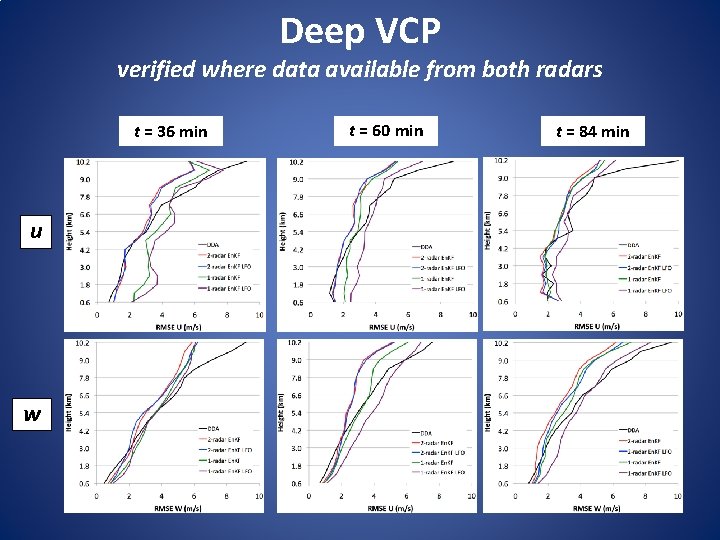
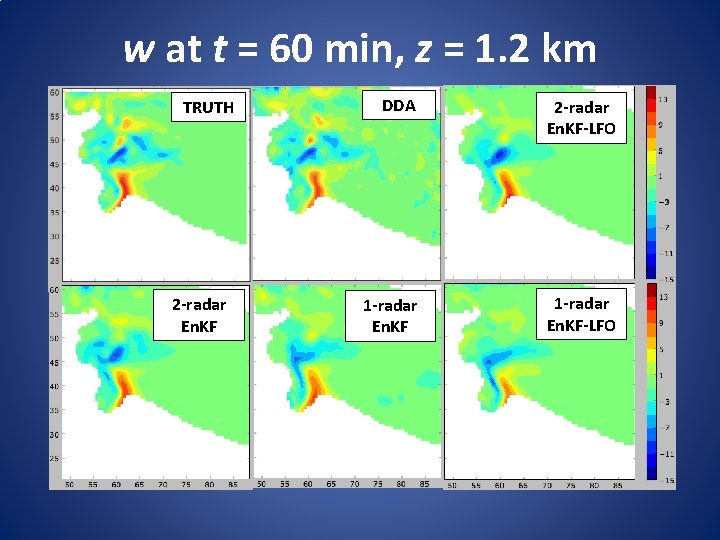
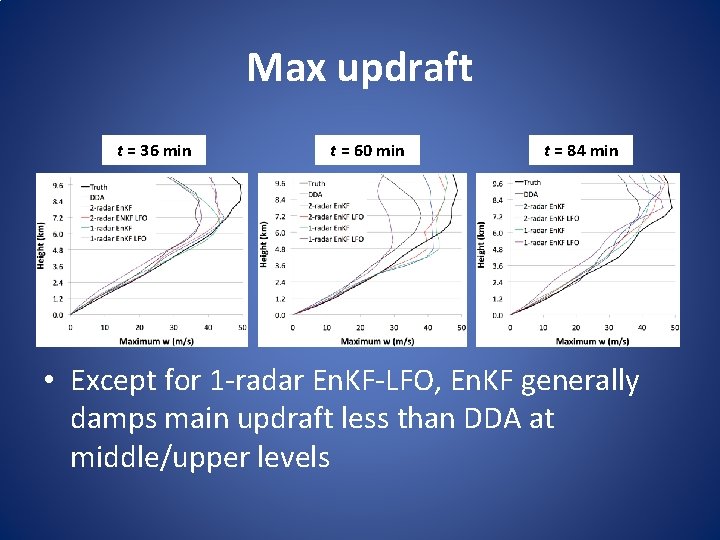
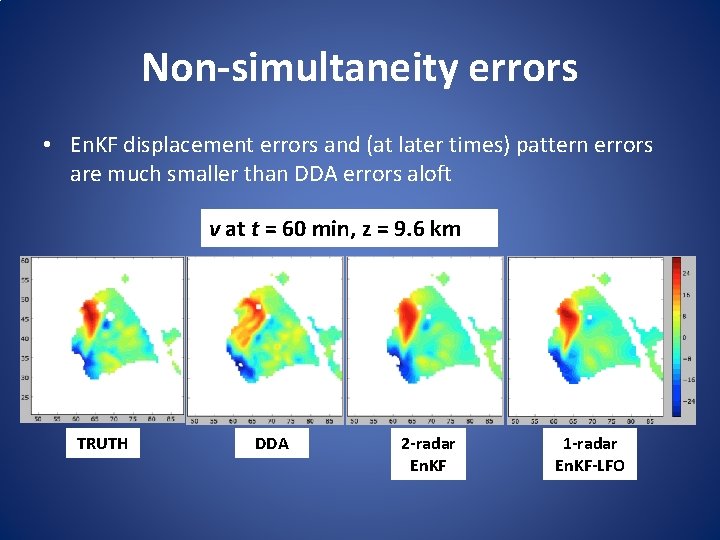
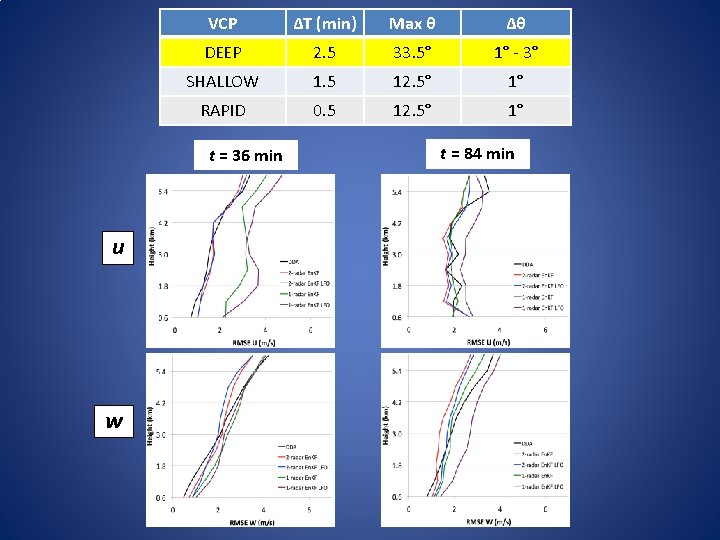
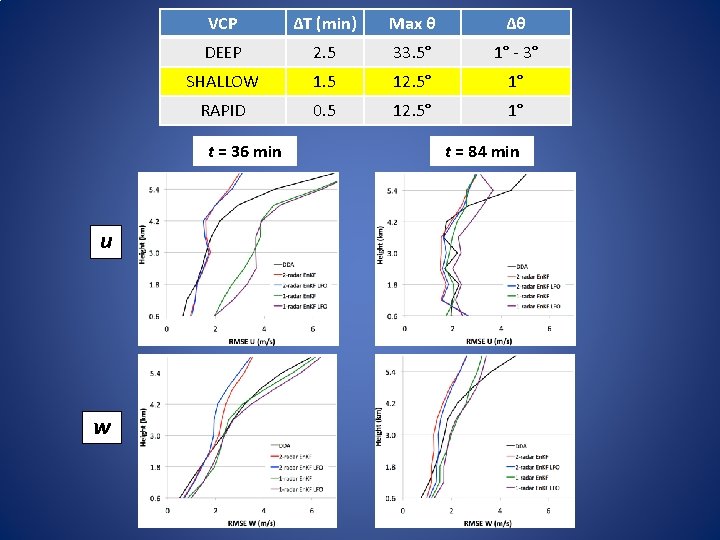
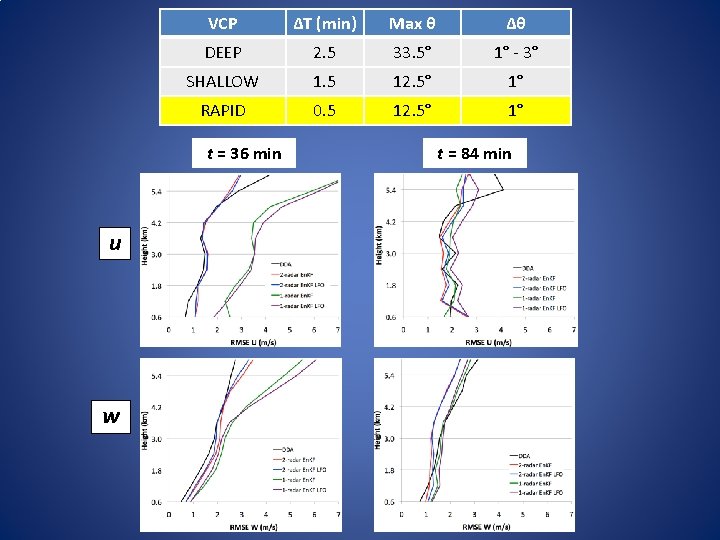
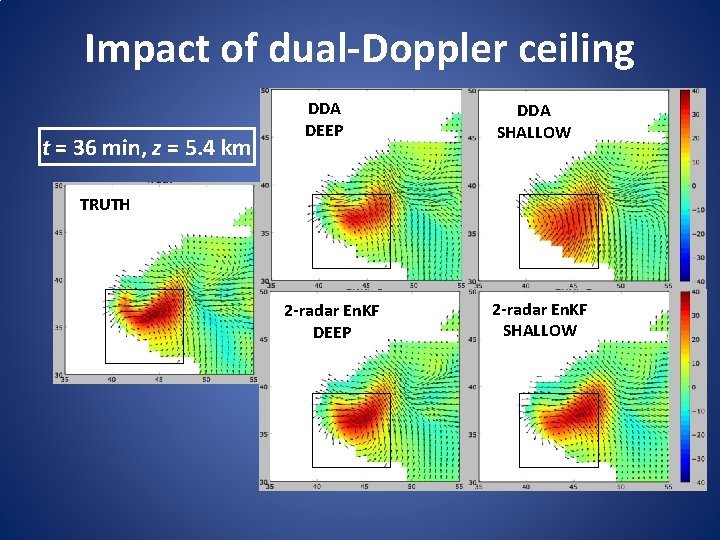
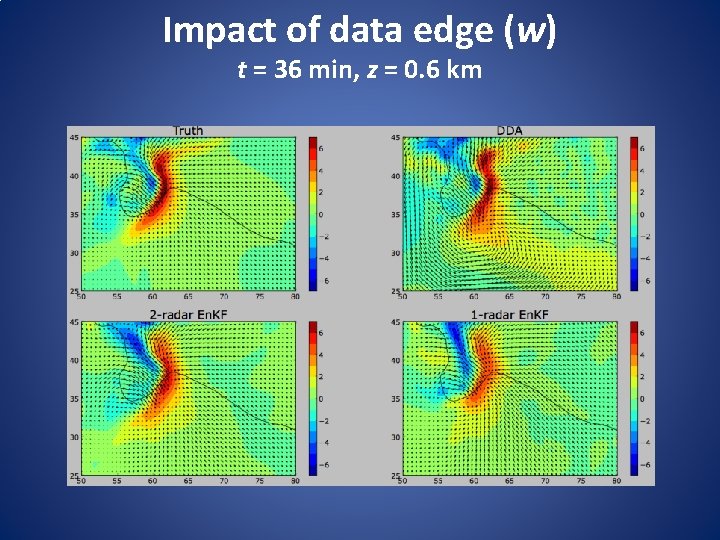
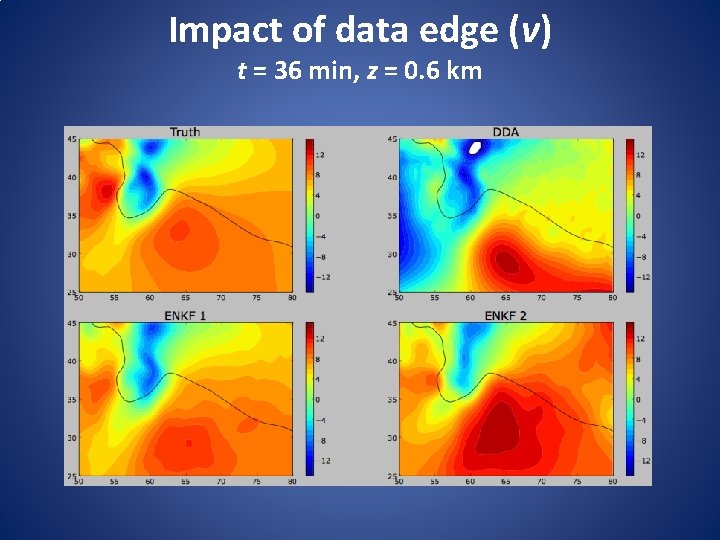
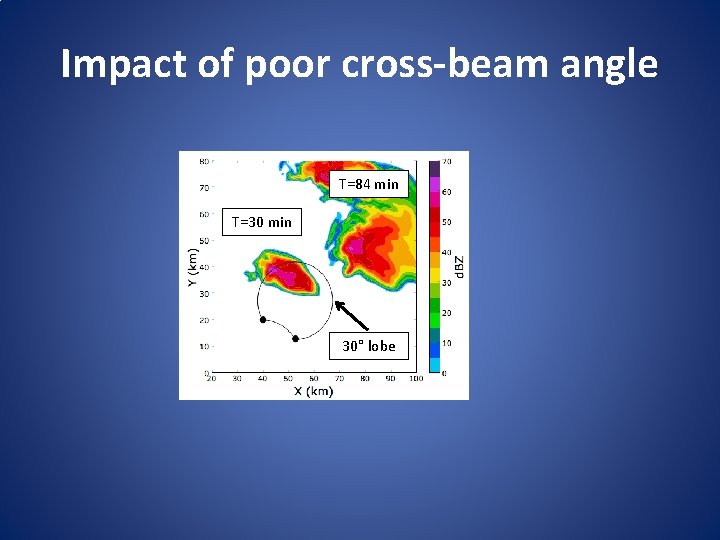
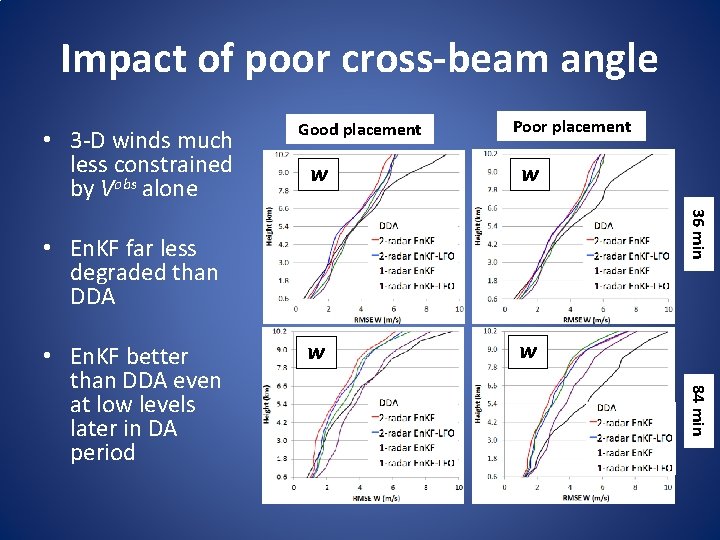
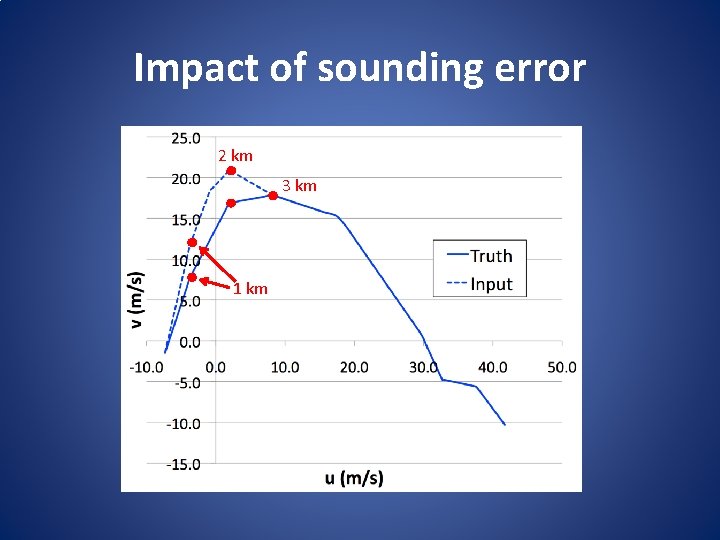
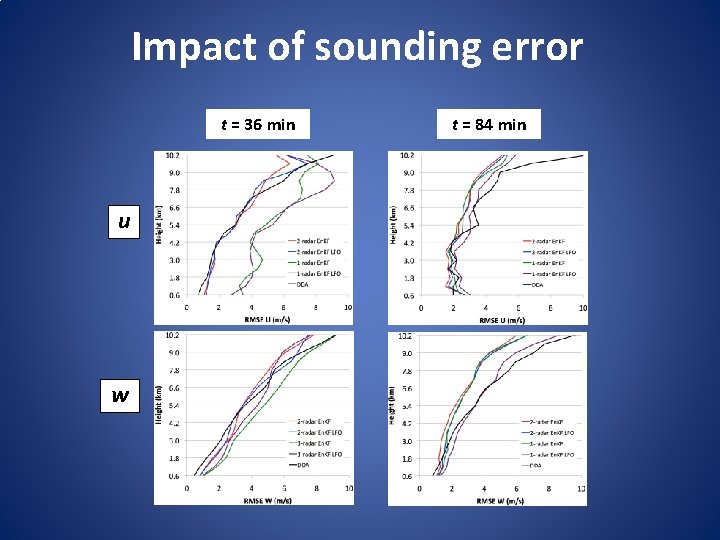
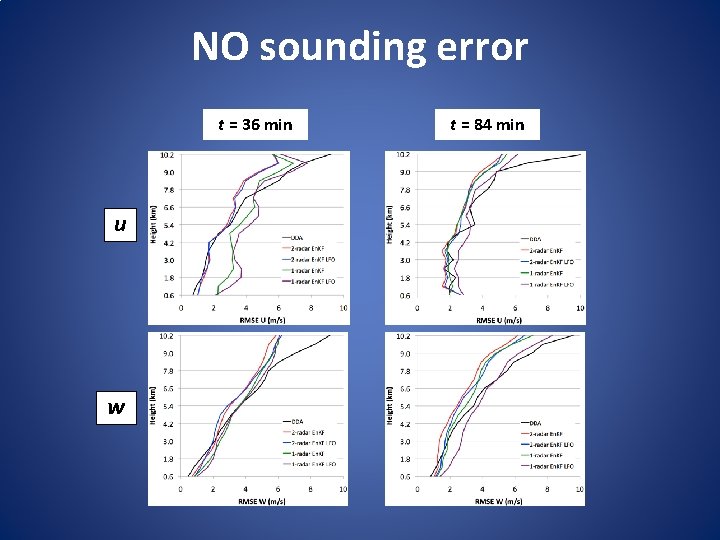
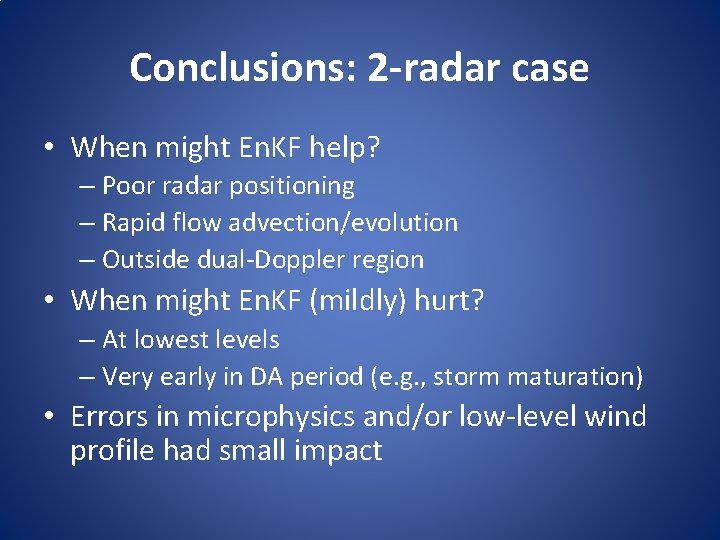
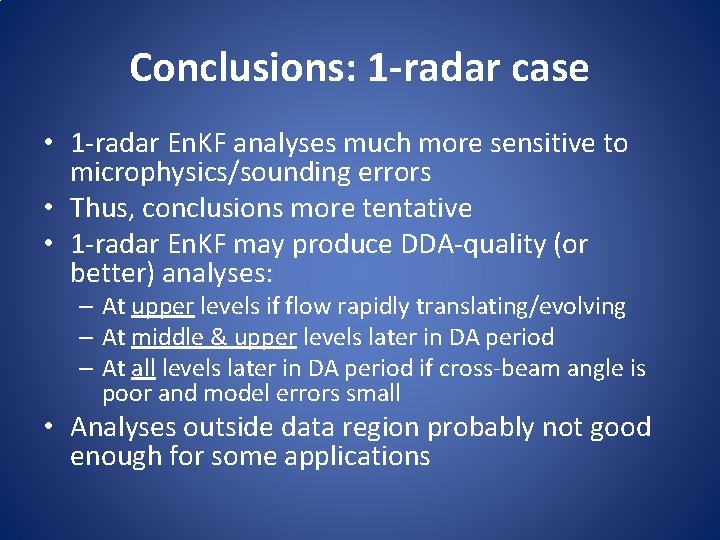
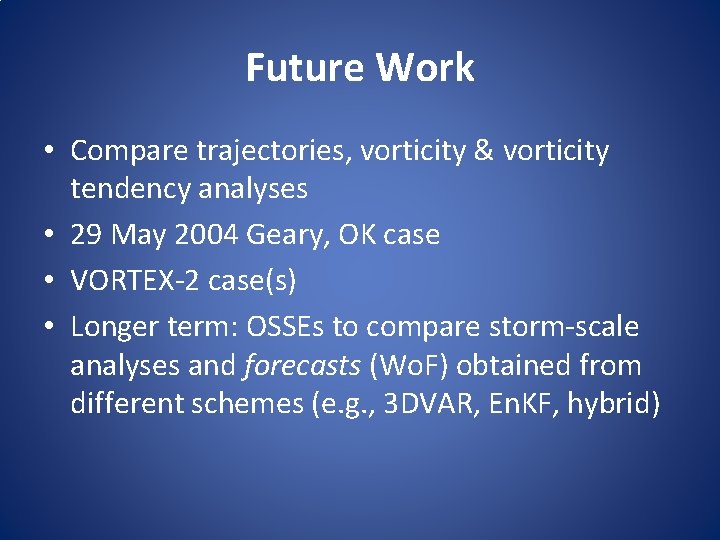
- Slides: 31
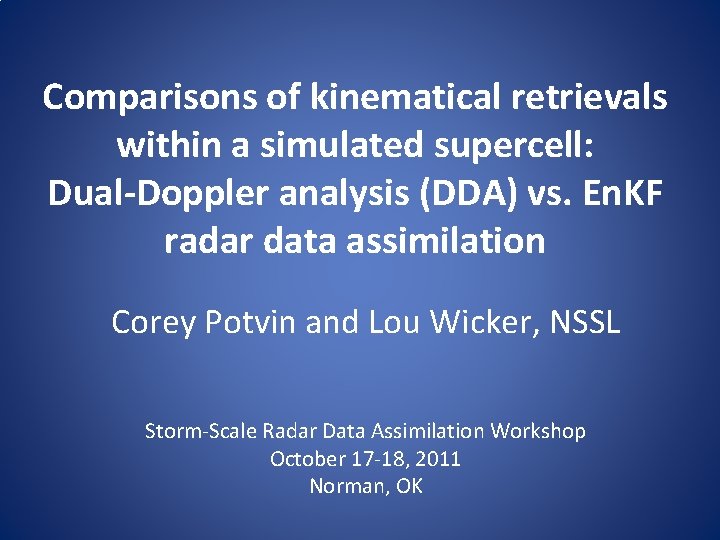
Comparisons of kinematical retrievals within a simulated supercell: Dual-Doppler analysis (DDA) vs. En. KF radar data assimilation Corey Potvin and Lou Wicker, NSSL Storm-Scale Radar Data Assimilation Workshop October 17 -18, 2011 Norman, OK
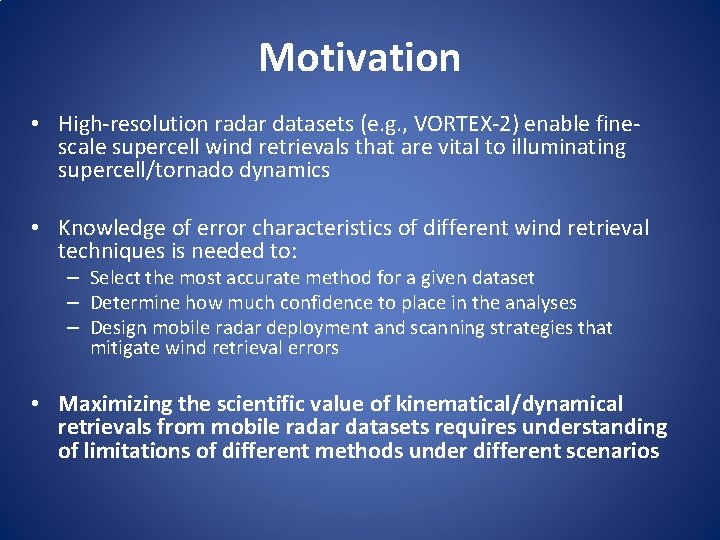
Motivation • High-resolution radar datasets (e. g. , VORTEX-2) enable finescale supercell wind retrievals that are vital to illuminating supercell/tornado dynamics • Knowledge of error characteristics of different wind retrieval techniques is needed to: – Select the most accurate method for a given dataset – Determine how much confidence to place in the analyses – Design mobile radar deployment and scanning strategies that mitigate wind retrieval errors • Maximizing the scientific value of kinematical/dynamical retrievals from mobile radar datasets requires understanding of limitations of different methods under different scenarios
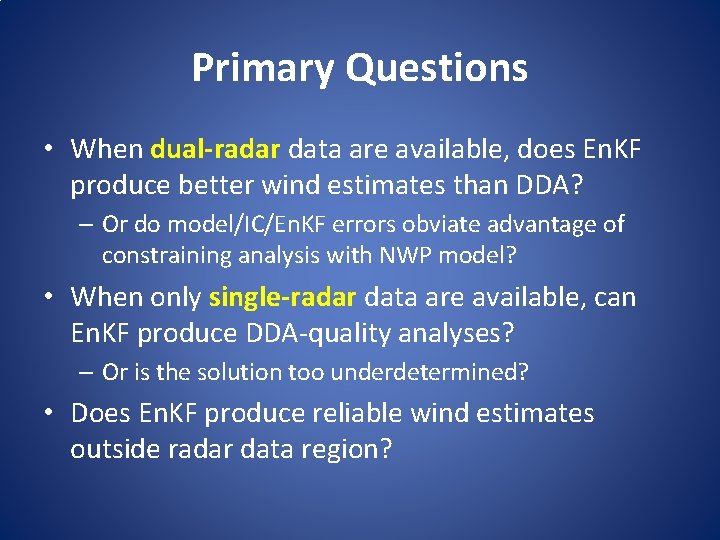
Primary Questions • When dual-radar data are available, does En. KF produce better wind estimates than DDA? – Or do model/IC/En. KF errors obviate advantage of constraining analysis with NWP model? • When only single-radar data are available, can En. KF produce DDA-quality analyses? – Or is the solution too underdetermined? • Does En. KF produce reliable wind estimates outside radar data region?
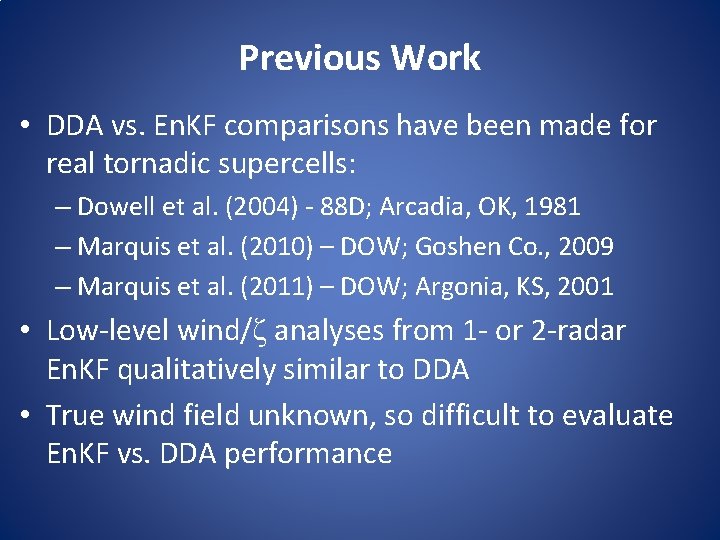
Previous Work • DDA vs. En. KF comparisons have been made for real tornadic supercells: – Dowell et al. (2004) - 88 D; Arcadia, OK, 1981 – Marquis et al. (2010) – DOW; Goshen Co. , 2009 – Marquis et al. (2011) – DOW; Argonia, KS, 2001 • Low-level wind/ζ analyses from 1 - or 2 -radar En. KF qualitatively similar to DDA • True wind field unknown, so difficult to evaluate En. KF vs. DDA performance
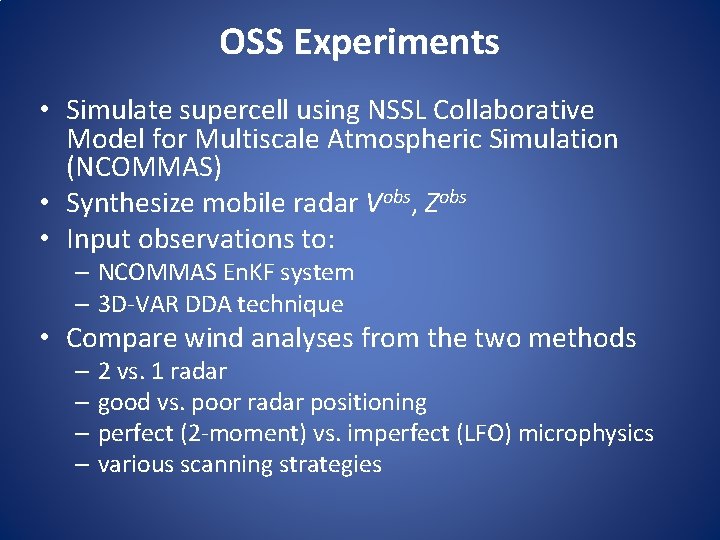
OSS Experiments • Simulate supercell using NSSL Collaborative Model for Multiscale Atmospheric Simulation (NCOMMAS) • Synthesize mobile radar Vobs, Zobs • Input observations to: – NCOMMAS En. KF system – 3 D-VAR DDA technique • Compare wind analyses from the two methods – 2 vs. 1 radar – good vs. poor radar positioning – perfect (2 -moment) vs. imperfect (LFO) microphysics – various scanning strategies
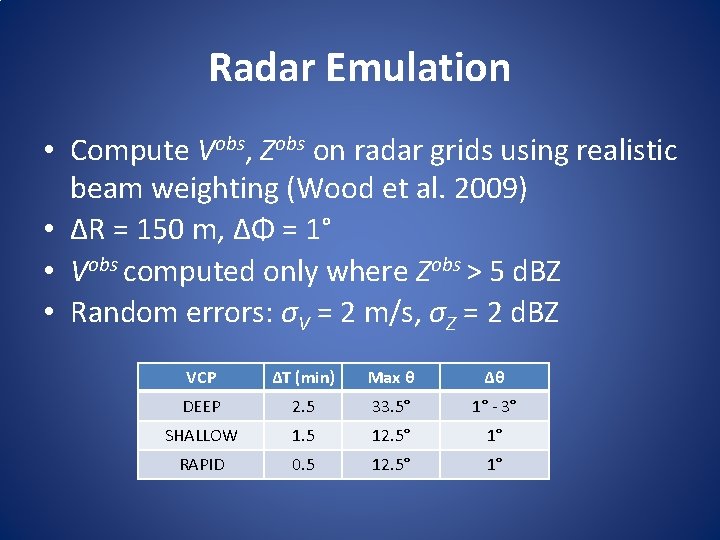
Radar Emulation • Compute Vobs, Zobs on radar grids using realistic beam weighting (Wood et al. 2009) • ΔR = 150 m, ΔΦ = 1° • Vobs computed only where Zobs > 5 d. BZ • Random errors: σV = 2 m/s, σZ = 2 d. BZ VCP ΔT (min) Max θ Δθ DEEP 2. 5 33. 5° 1° - 3° SHALLOW 1. 5 12. 5° 1° RAPID 0. 5 12. 5° 1°
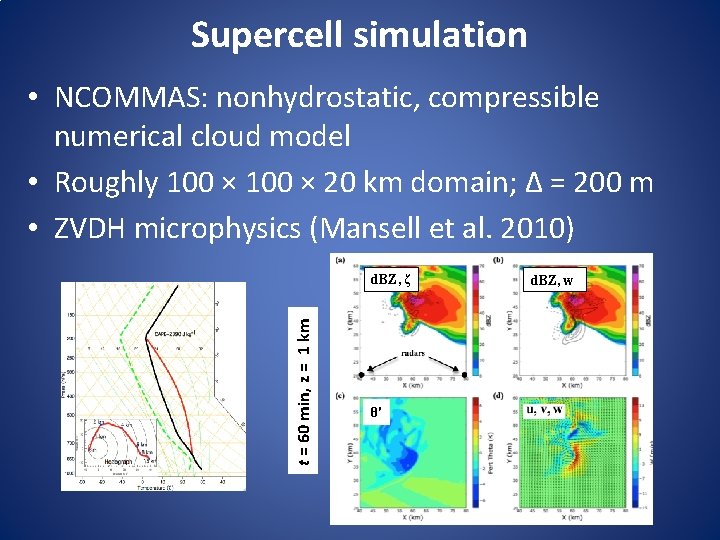
Supercell simulation • NCOMMAS: nonhydrostatic, compressible numerical cloud model • Roughly 100 × 20 km domain; Δ = 200 m • ZVDH microphysics (Mansell et al. 2010) t = 60 min, z = 1 km d. BZ, ζ θ' d. BZ, w
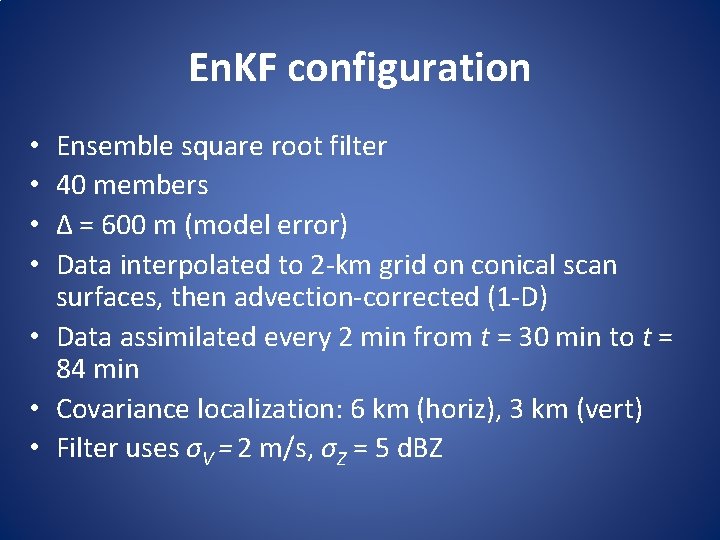
En. KF configuration Ensemble square root filter 40 members Δ = 600 m (model error) Data interpolated to 2 -km grid on conical scan surfaces, then advection-corrected (1 -D) • Data assimilated every 2 min from t = 30 min to t = 84 min • Covariance localization: 6 km (horiz), 3 km (vert) • Filter uses σV = 2 m/s, σZ = 5 d. BZ • •
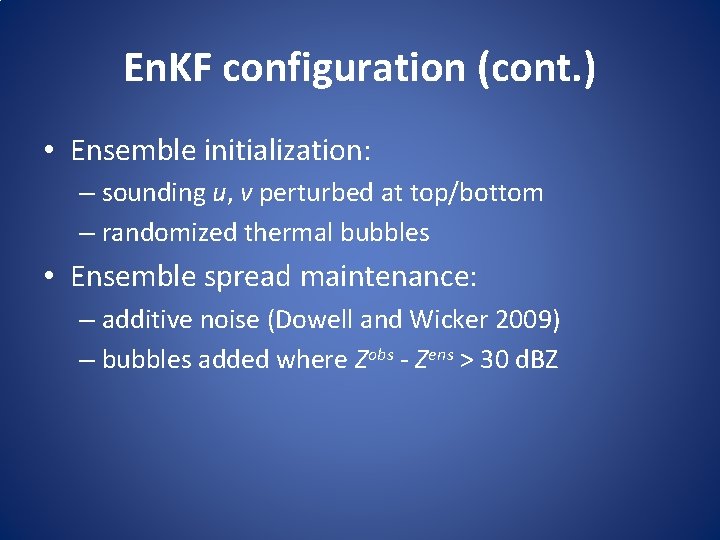
En. KF configuration (cont. ) • Ensemble initialization: – sounding u, v perturbed at top/bottom – randomized thermal bubbles • Ensemble spread maintenance: – additive noise (Dowell and Wicker 2009) – bubbles added where Zobs - Zens > 30 d. BZ
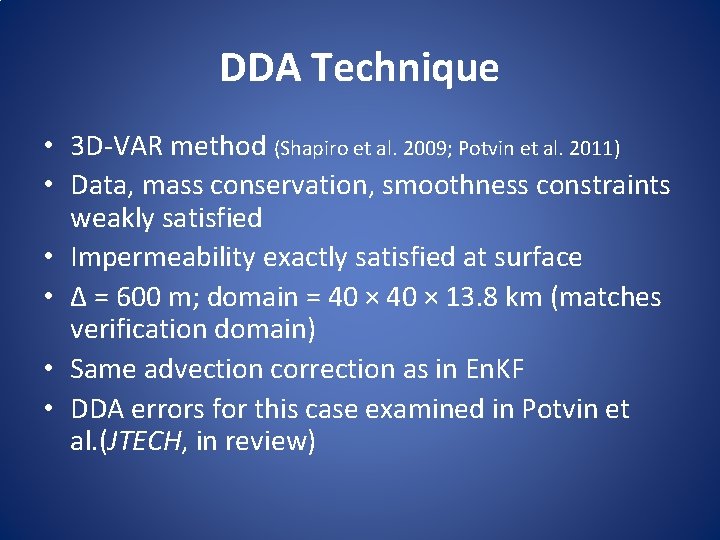
DDA Technique • 3 D-VAR method (Shapiro et al. 2009; Potvin et al. 2011) • Data, mass conservation, smoothness constraints weakly satisfied • Impermeability exactly satisfied at surface • Δ = 600 m; domain = 40 × 13. 8 km (matches verification domain) • Same advection correction as in En. KF • DDA errors for this case examined in Potvin et al. (JTECH, in review)
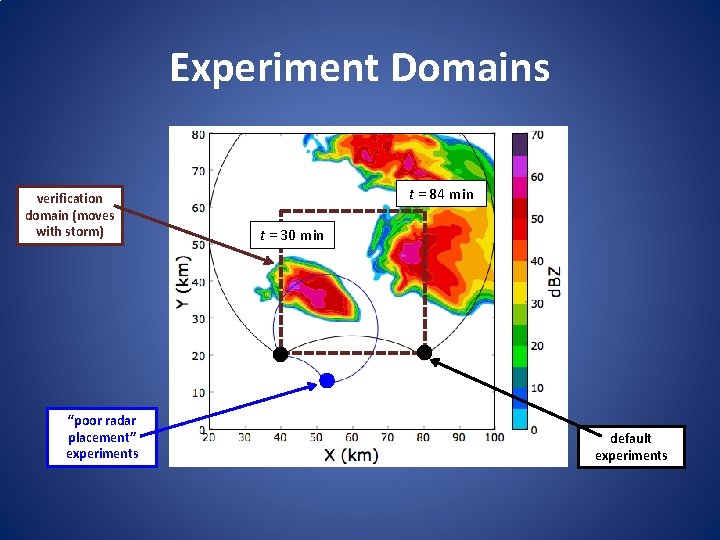
Experiment Domains verification domain (moves with storm) “poor radar placement” experiments t = 84 min t = 30 min default experiments
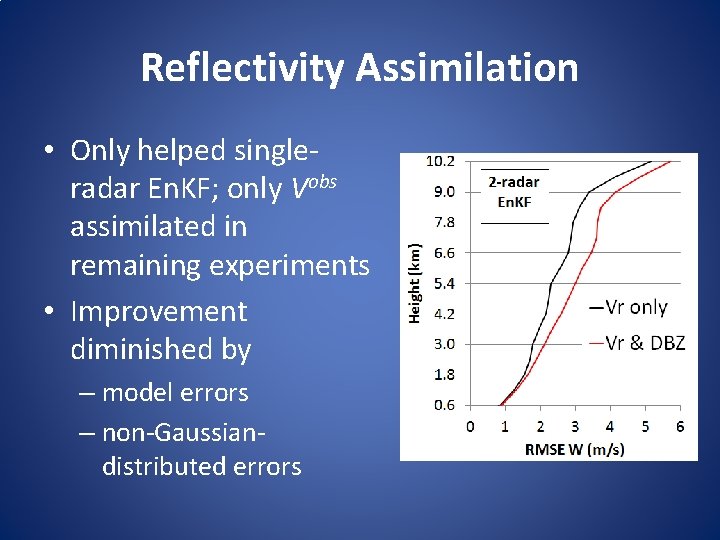
Reflectivity Assimilation • Only helped singleradar En. KF; only Vobs assimilated in remaining experiments • Improvement diminished by – model errors – non-Gaussiandistributed errors
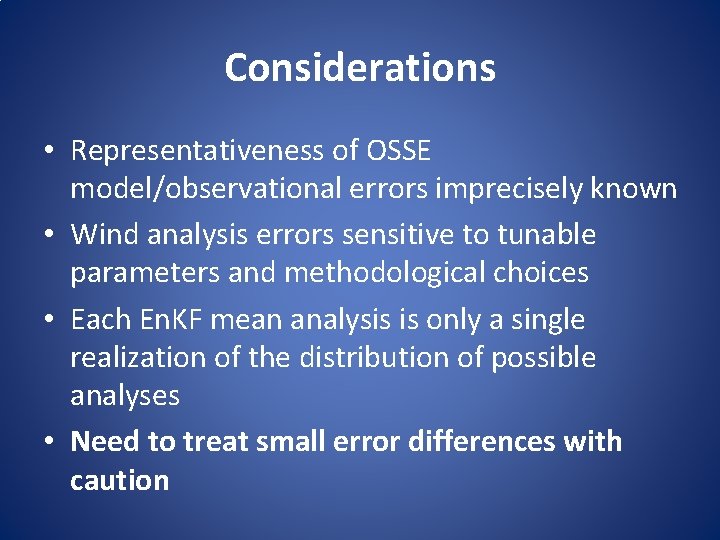
Considerations • Representativeness of OSSE model/observational errors imprecisely known • Wind analysis errors sensitive to tunable parameters and methodological choices • Each En. KF mean analysis is only a single realization of the distribution of possible analyses • Need to treat small error differences with caution
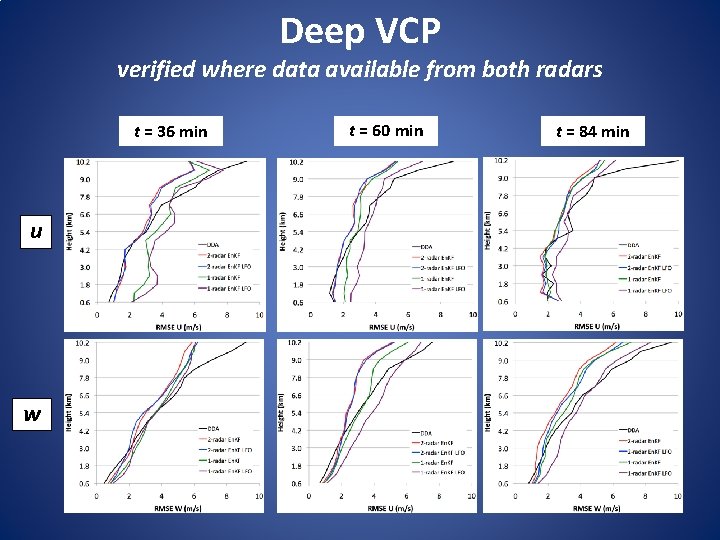
Deep VCP verified where data available from both radars t = 36 min u w t = 60 min t = 84 min
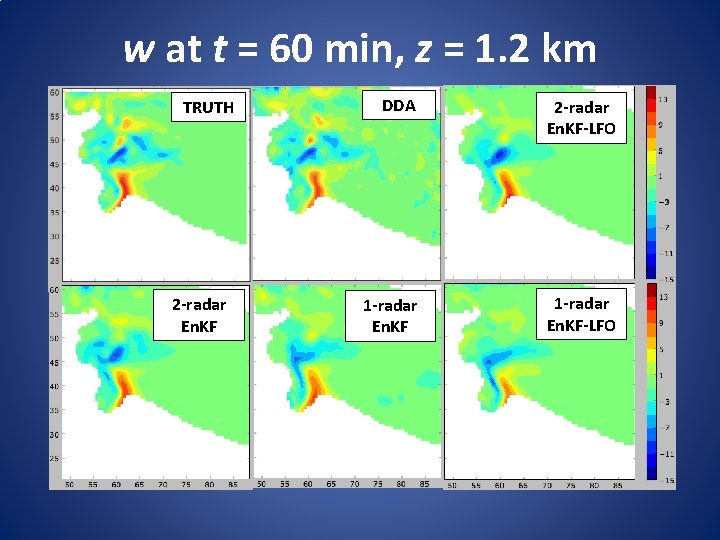
w at t = 60 min, z = 1. 2 km TRUTH 2 -radar En. KF DDA 2 -radar En. KF-LFO 1 -radar En. KF-LFO
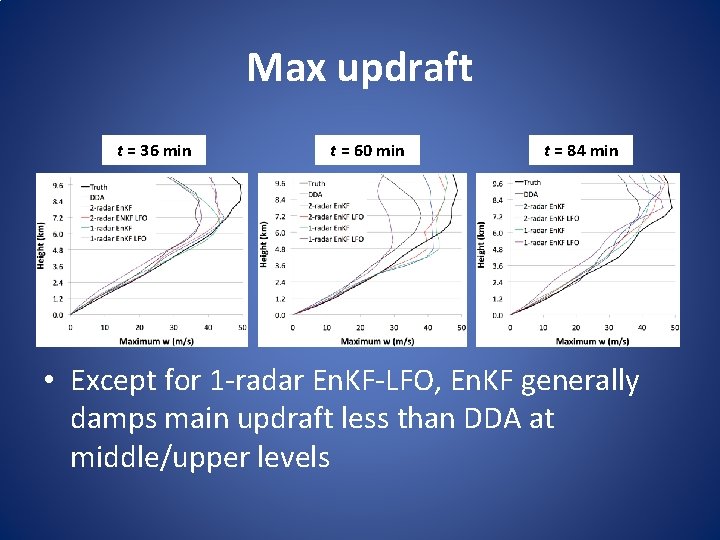
Max updraft t = 36 min t = 60 min t = 84 min • Except for 1 -radar En. KF-LFO, En. KF generally damps main updraft less than DDA at middle/upper levels
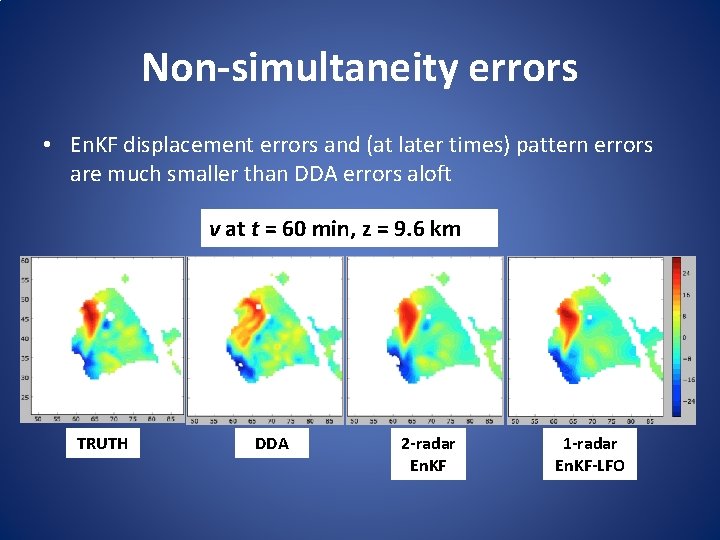
Non-simultaneity errors • En. KF displacement errors and (at later times) pattern errors are much smaller than DDA errors aloft v at t = 60 min, z = 9. 6 km TRUTH DDA 2 -radar En. KF 1 -radar En. KF-LFO
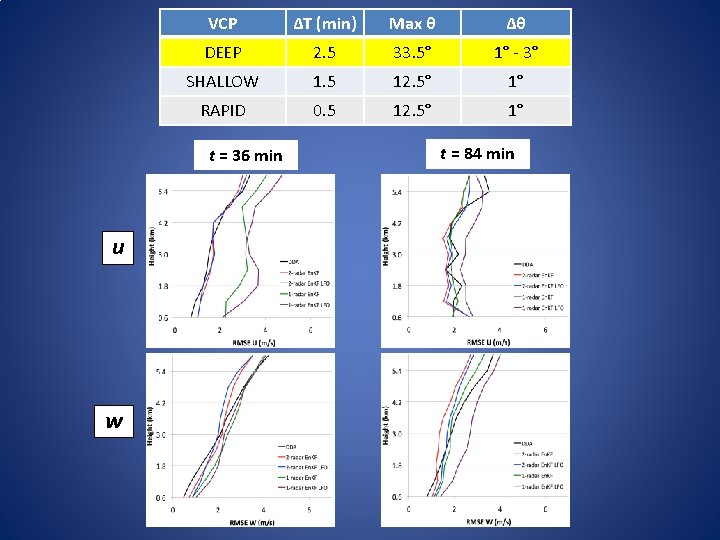
VCP DEEP SHALLOW t = 36 min w Max θ 2. 5 33. 5° Deep VCP RAPID u ΔT (min) Δθ 1° - 3° 1. 5 12. 5° 1° 0. 5 12. 5° 1° t = 84 min
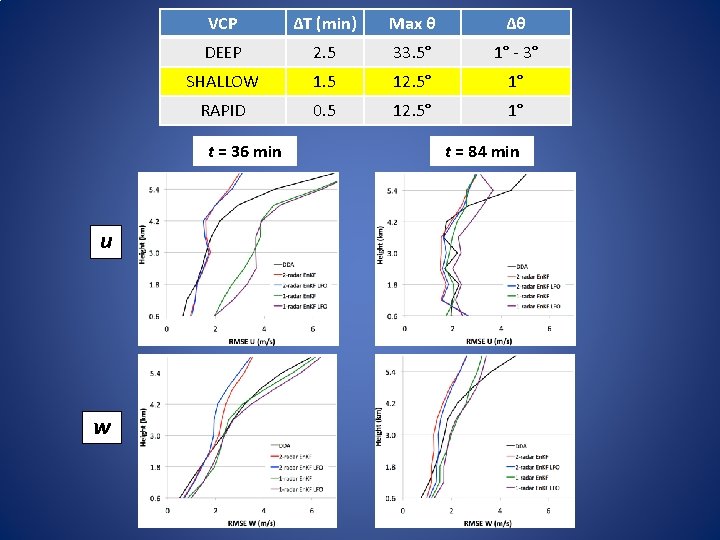
VCP ΔT (min) Max θ Δθ DEEP 2. 5 33. 5° 1° - 3° SHALLOW 1. 5 12. 5° 1° RAPID 0. 5 12. 5° 1° t = 36 min u w t = 84 min
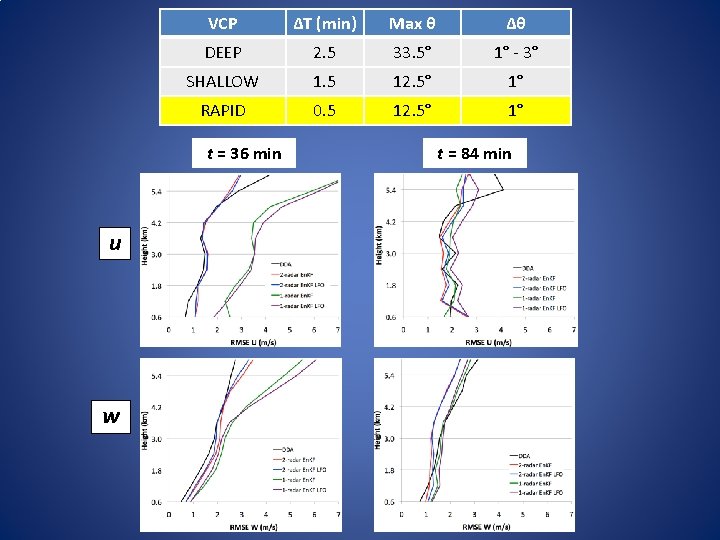
VCP ΔT (min) Max θ Δθ DEEP 2. 5 33. 5° 1° - 3° SHALLOW 1. 5 12. 5° 1° RAPID 0. 5 12. 5° 1° t = 36 min u w t = 84 min
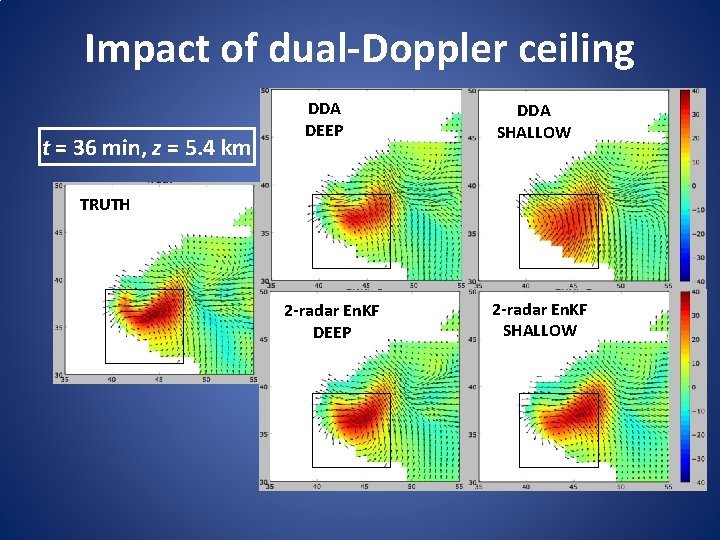
Impact of dual-Doppler ceiling t = 36 min, z = 5. 4 km DDA DEEP DDA SHALLOW TRUTH 2 -radar En. KF DEEP 2 -radar En. KF SHALLOW
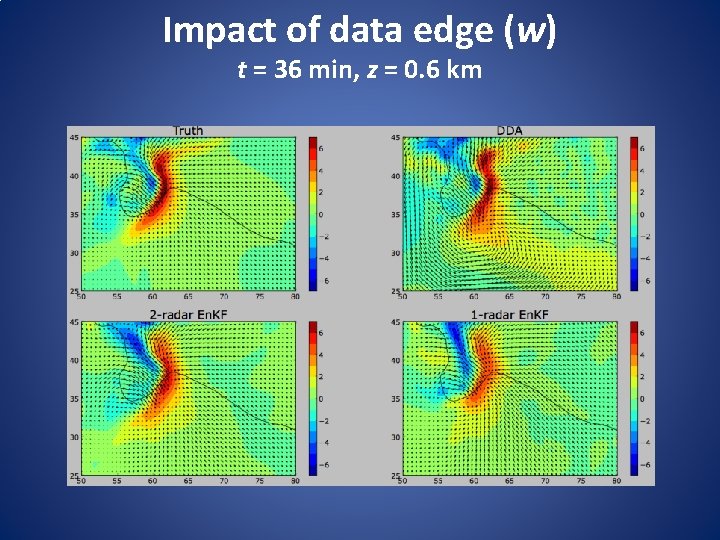
Impact of data edge (w) t = 36 min, z = 0. 6 km
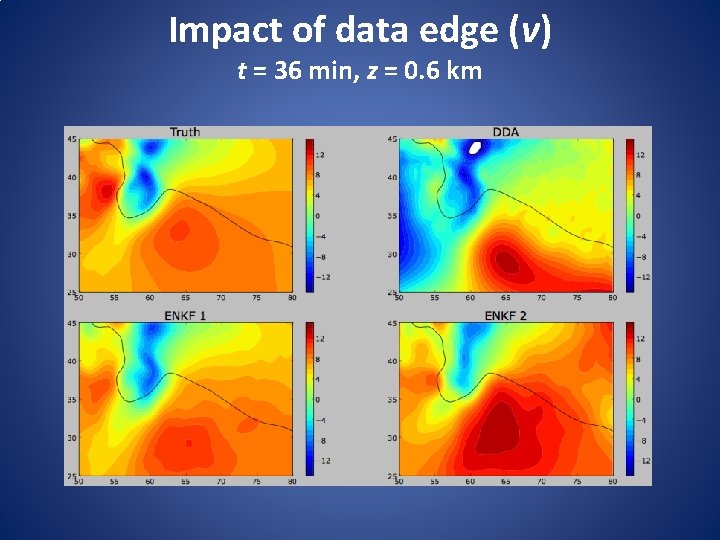
Impact of data edge (v) t = 36 min, z = 0. 6 km
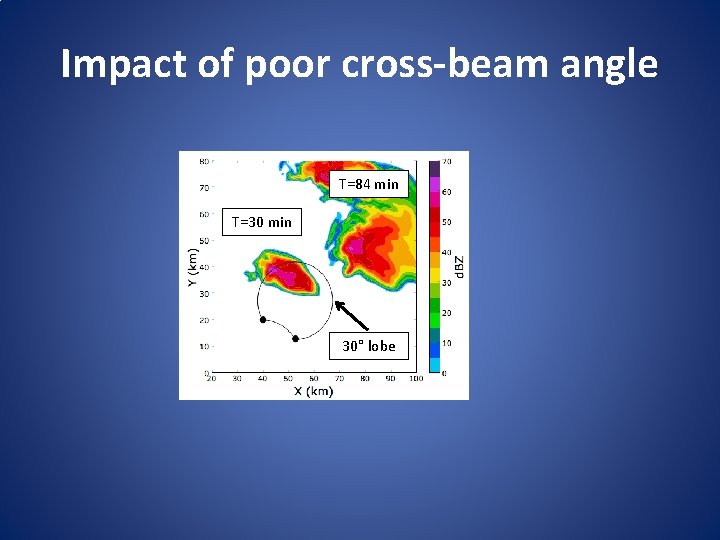
Impact of poor cross-beam angle T=84 min T=30 min 30° lobe
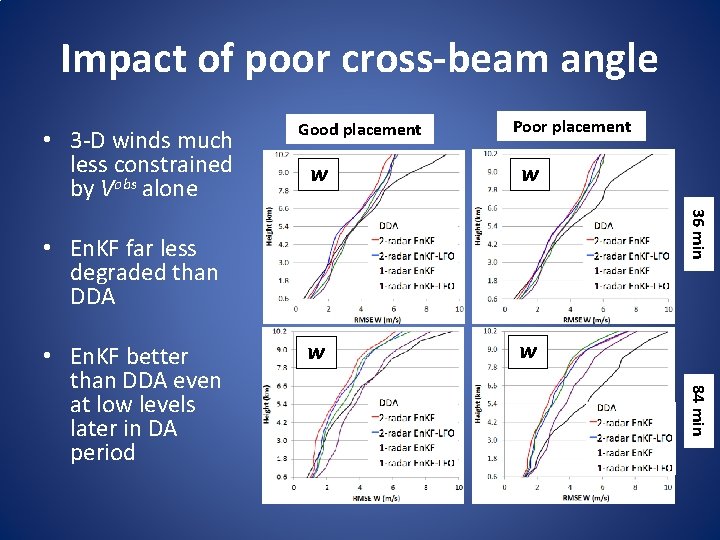
Impact of poor cross-beam angle • 3 -D winds much less constrained by Vobs alone Good placement Poor placement w w w 36 min w • En. KF far less degraded than DDA 84 min • En. KF better than DDA even at low levels later in DA period
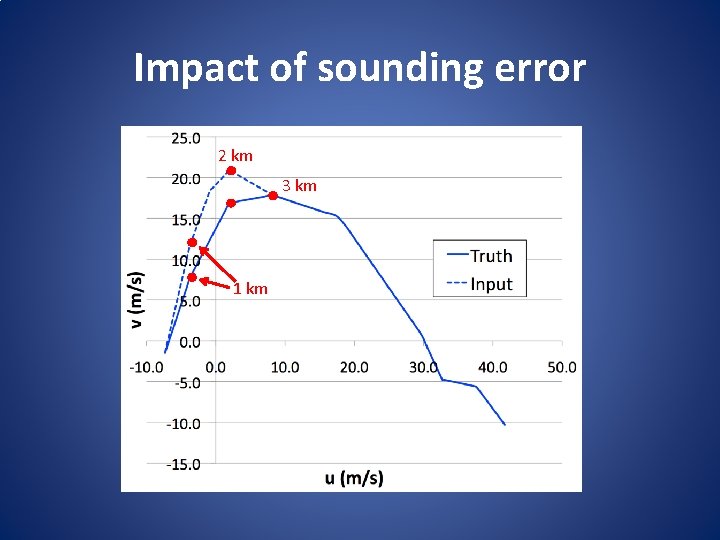
Impact of sounding error 2 km 3 km 1 km
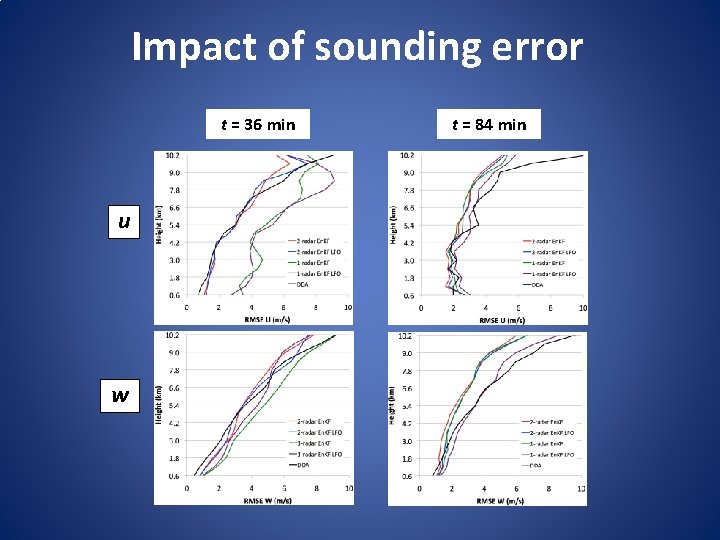
Impact of sounding error t = 36 min u w t = 84 min
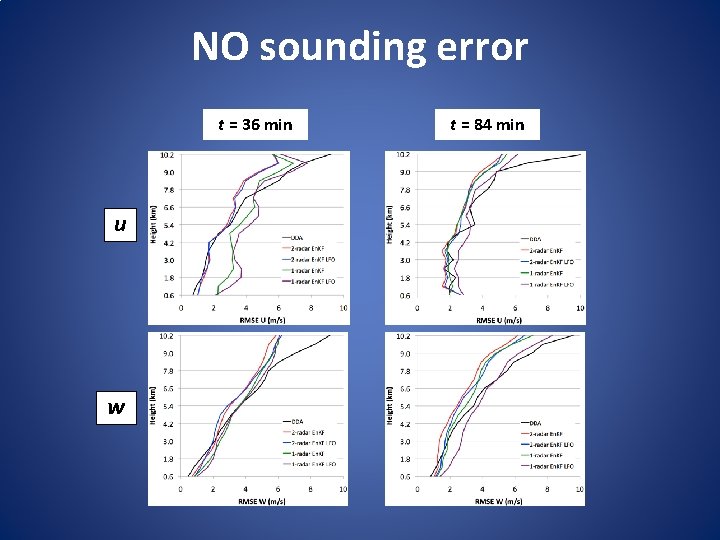
NO sounding error t = 36 min u w t = 84 min
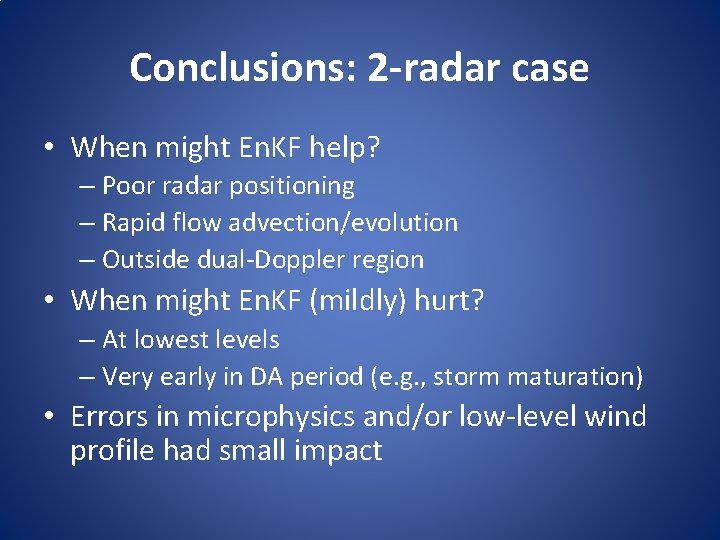
Conclusions: 2 -radar case • When might En. KF help? – Poor radar positioning – Rapid flow advection/evolution – Outside dual-Doppler region • When might En. KF (mildly) hurt? – At lowest levels – Very early in DA period (e. g. , storm maturation) • Errors in microphysics and/or low-level wind profile had small impact
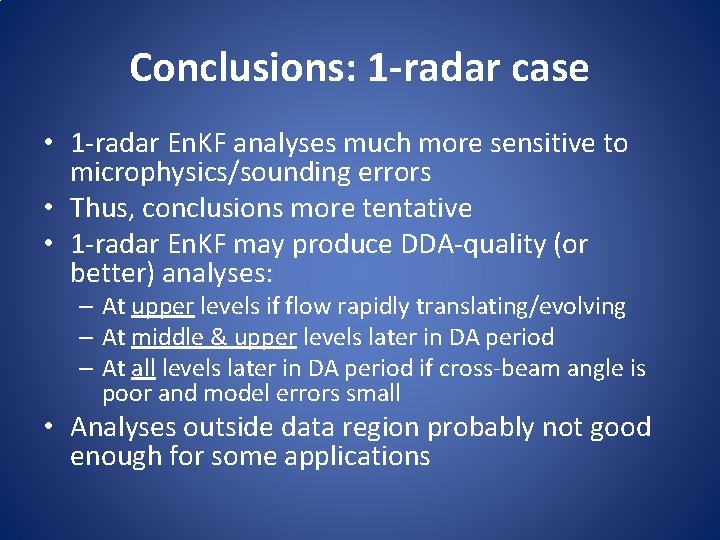
Conclusions: 1 -radar case • 1 -radar En. KF analyses much more sensitive to microphysics/sounding errors • Thus, conclusions more tentative • 1 -radar En. KF may produce DDA-quality (or better) analyses: – At upper levels if flow rapidly translating/evolving – At middle & upper levels later in DA period – At all levels later in DA period if cross-beam angle is poor and model errors small • Analyses outside data region probably not good enough for some applications
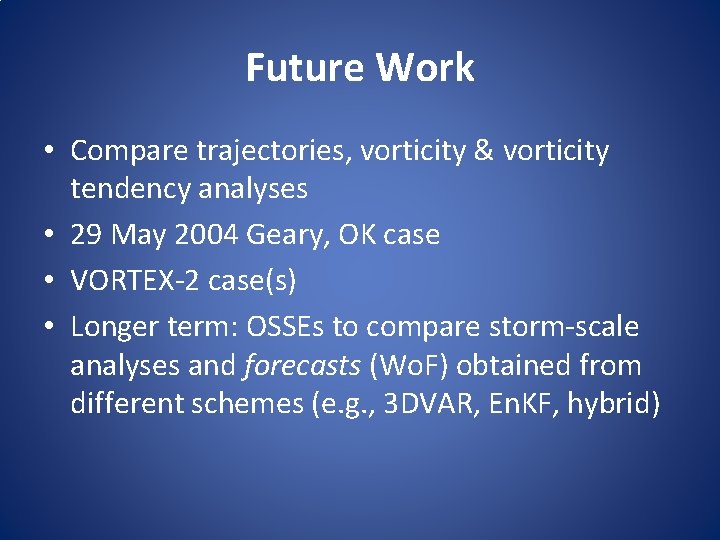
Future Work • Compare trajectories, vorticity & vorticity tendency analyses • 29 May 2004 Geary, OK case • VORTEX-2 case(s) • Longer term: OSSEs to compare storm-scale analyses and forecasts (Wo. F) obtained from different schemes (e. g. , 3 DVAR, En. KF, hybrid)
Society for worldwide interbank financial telecommunication
Kinematical diffraction
List and describe the stages of a single cell thunderstorm
Supercell storm
Frontogenesis and frontolysis
Supercell tutorial
Simulated annealing pseudo code
English test background
Exodeviation
Tone value
Simulated annealing algoritmo
Contoh simulated annealing
The actual surface feel of the simulated appearance
London letters for handwriting examination
Simulated killing philosophy
What is
Simulated forgery definition
The tactile quality of a surface
Types of emphasis in art
Texture actual
Actual, simulated, abstract, and invented texture.
Equality and inequality ejemplos
Irregular comparisons
Realidades 2 capitulo 1b making comparisons answers
Quantifiers to make comparisons
Comparatives equality
Cmpt 225
Two figures of speech that involve comparisons are *
As + adjective/adverb + as
Comparatives cold
What is a double comparison
Comparisons of equality ejemplos