Chapter 5 Critically Appraising Quantitative Evidence for Clinical
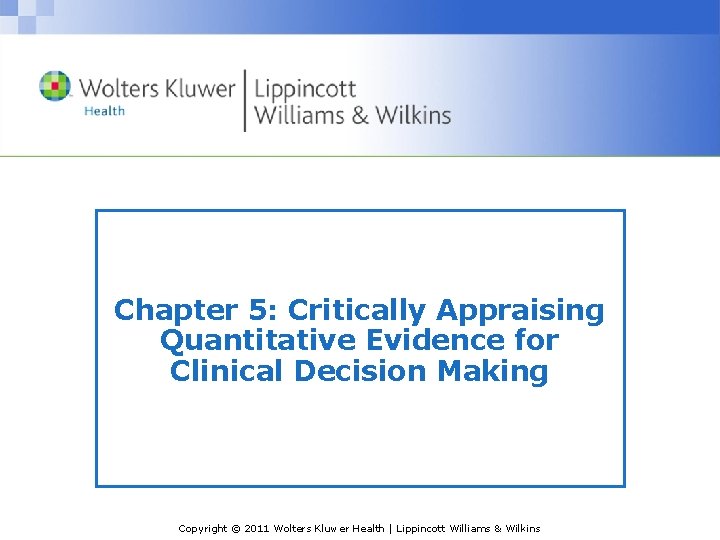
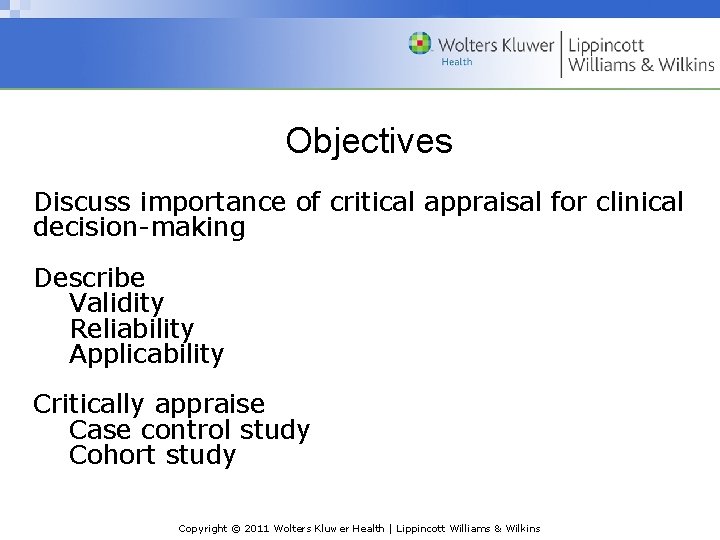
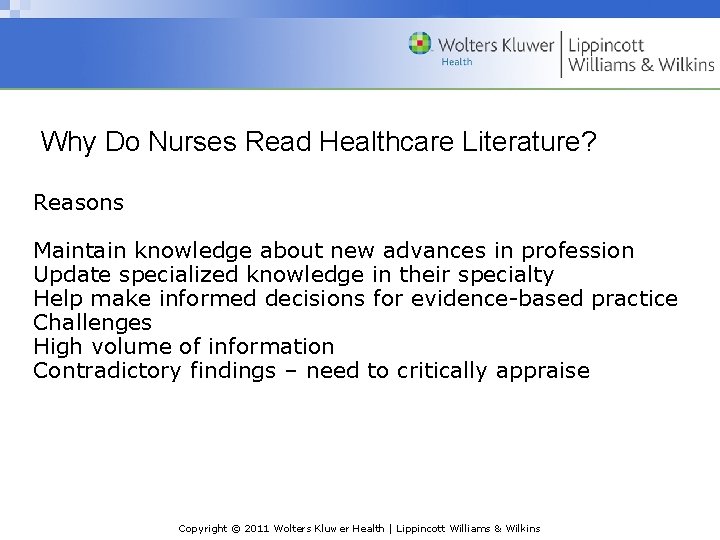
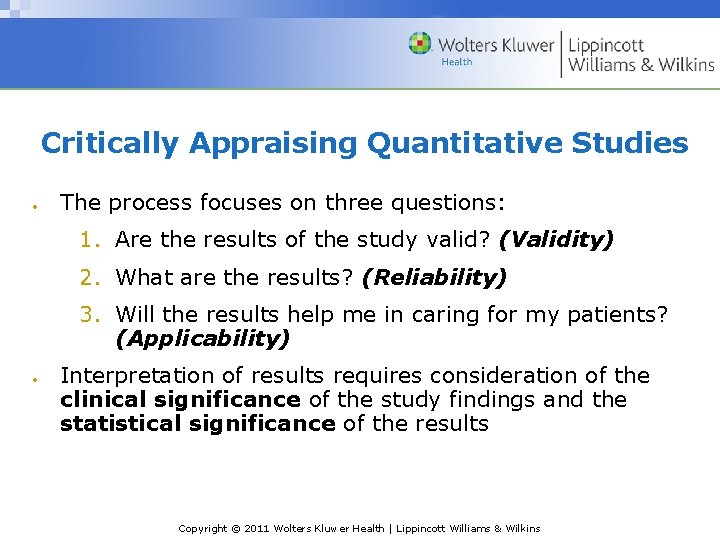
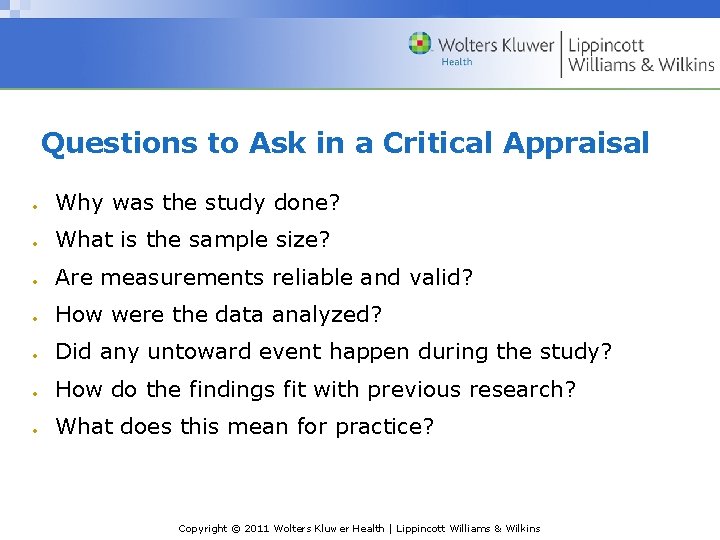
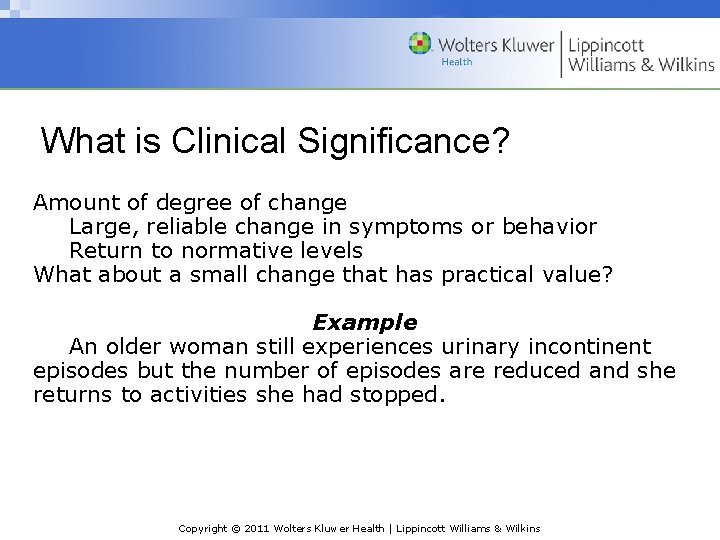
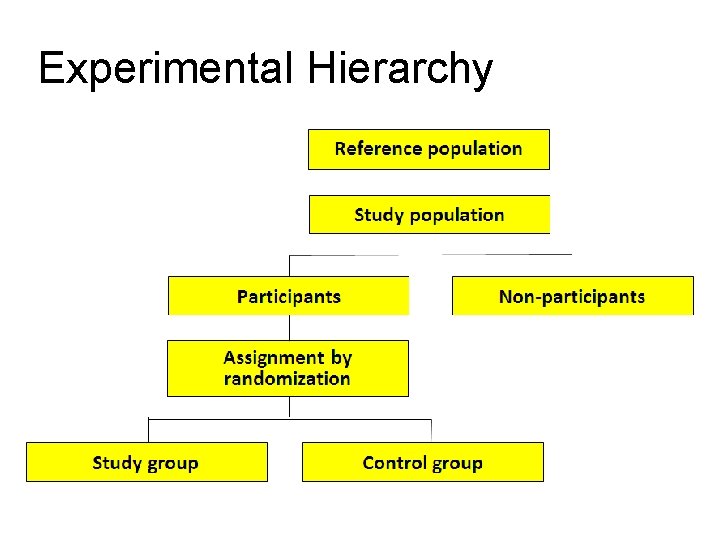
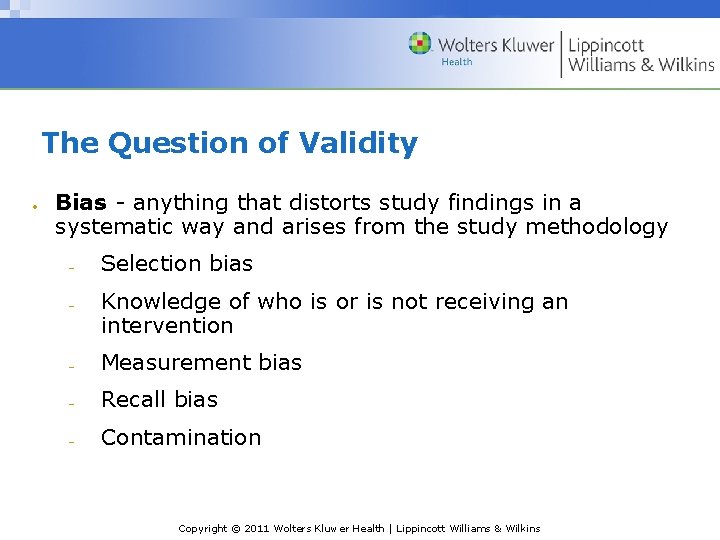
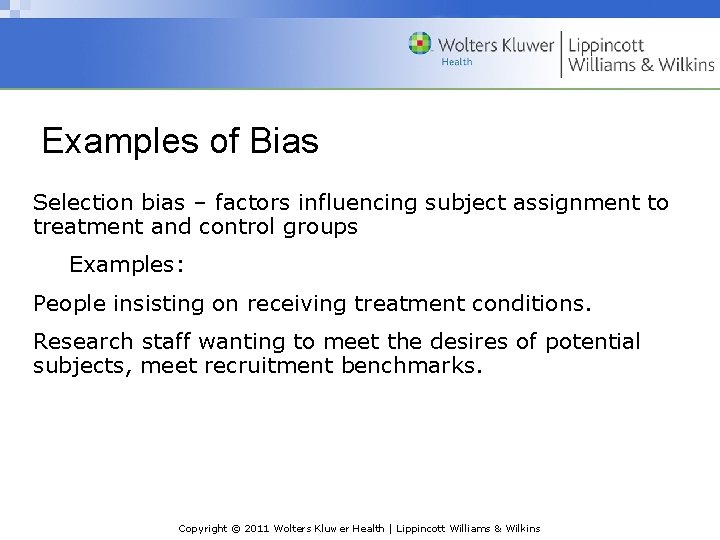
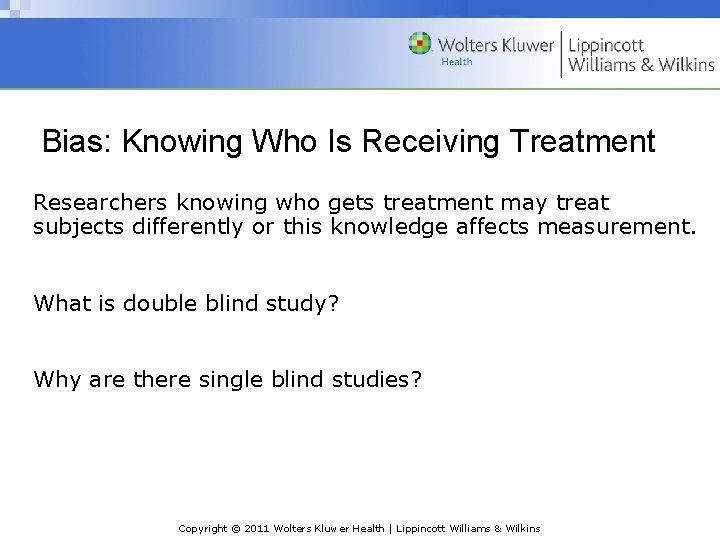
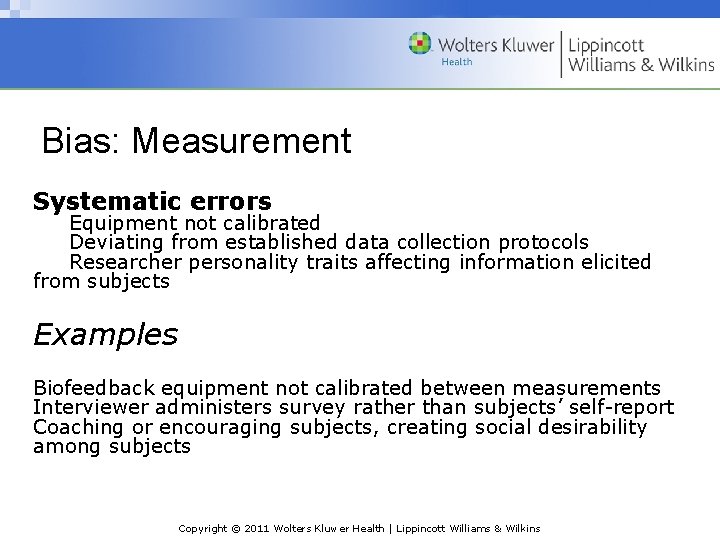
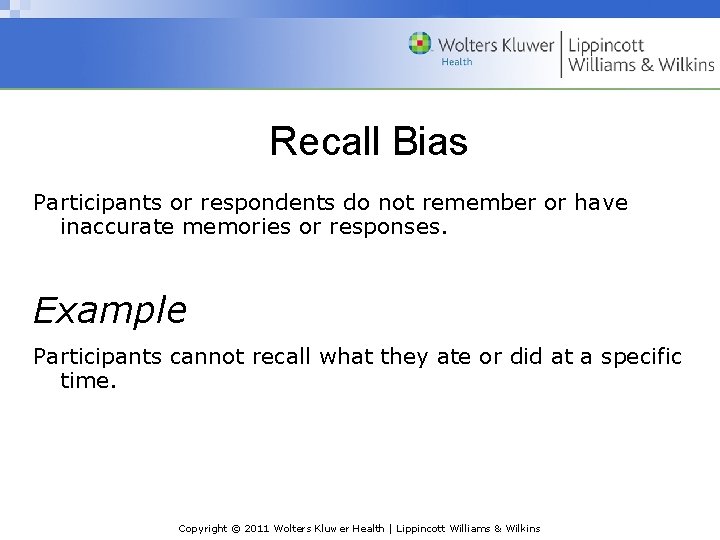
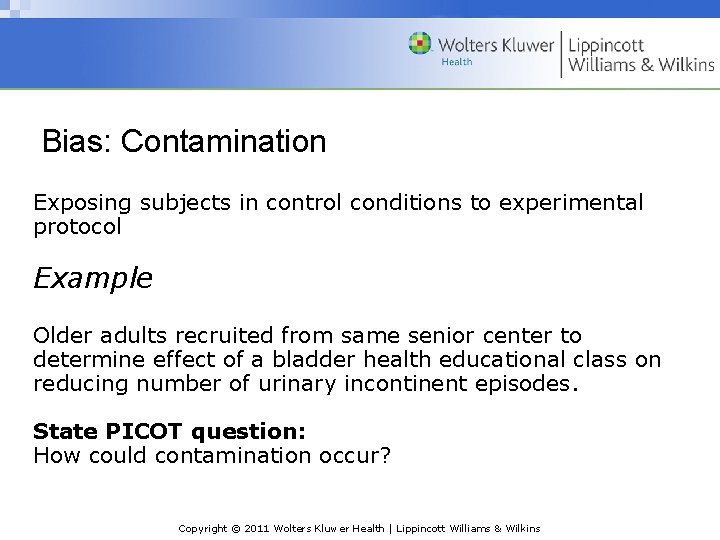
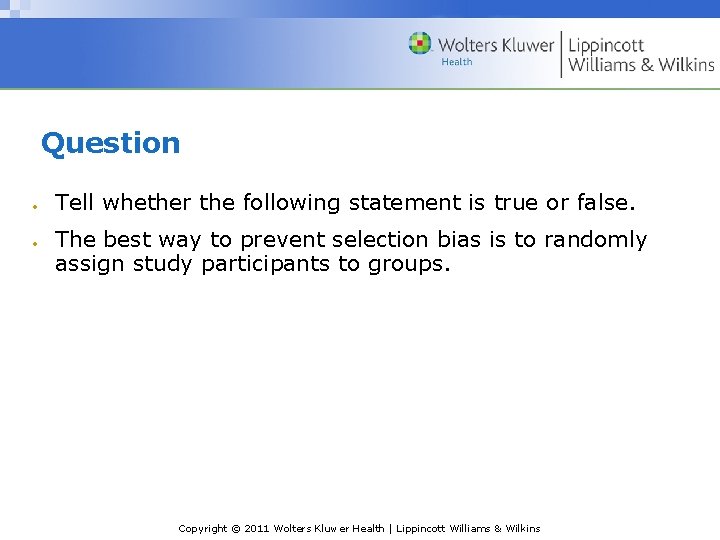
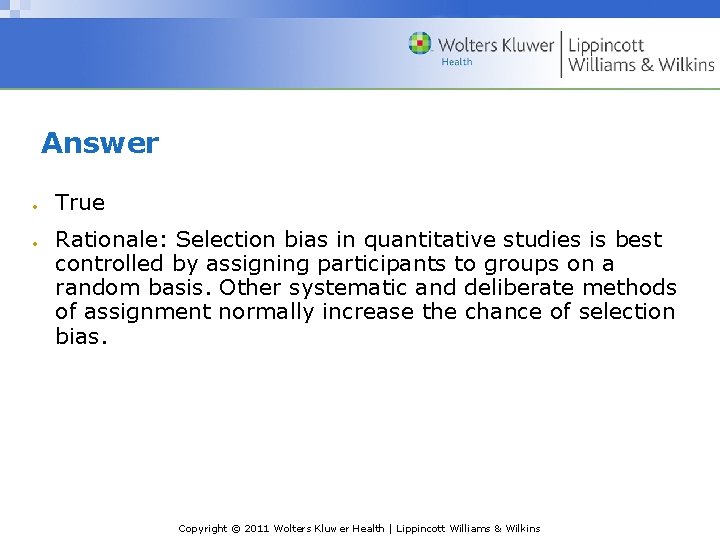
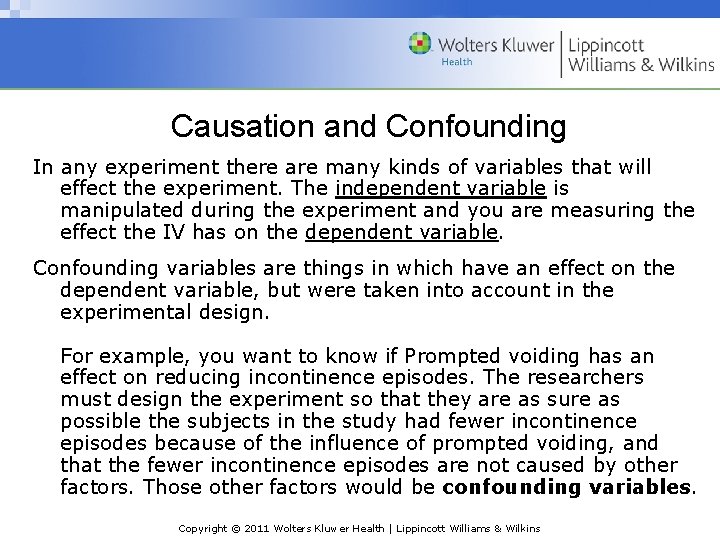
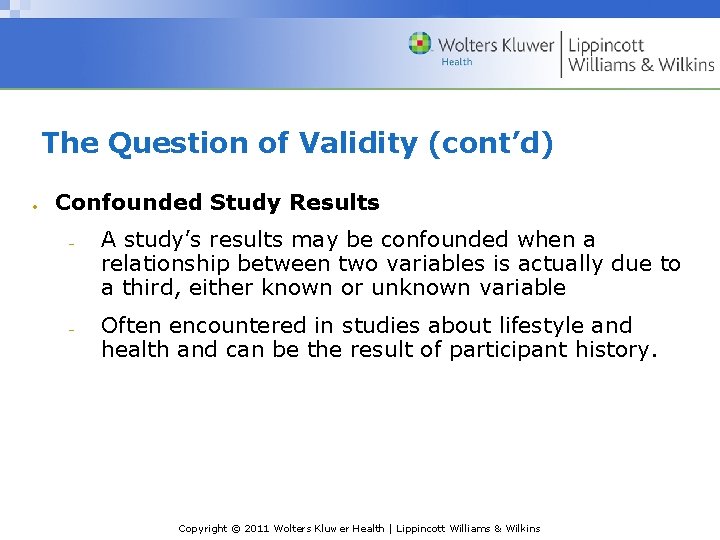
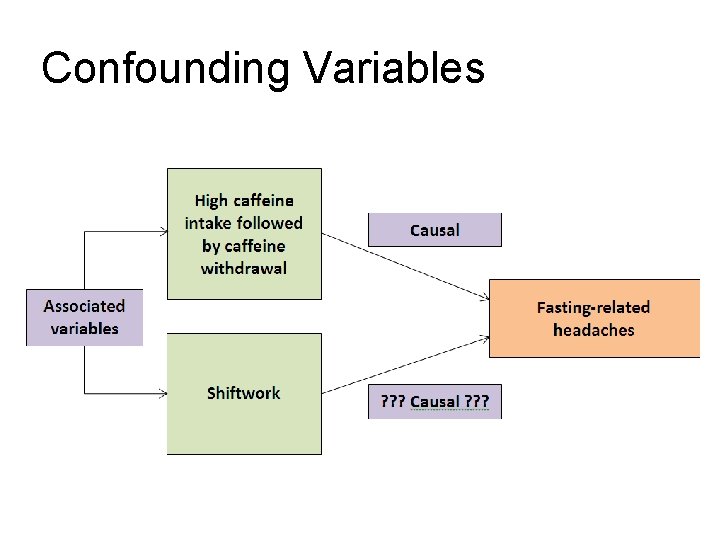
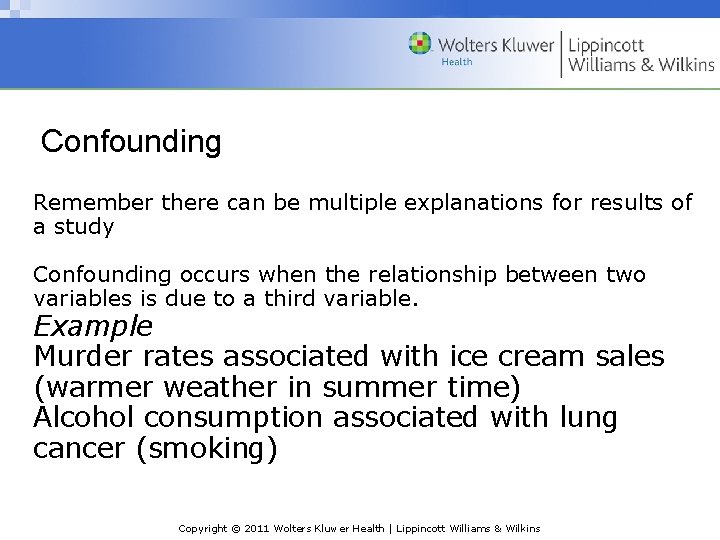
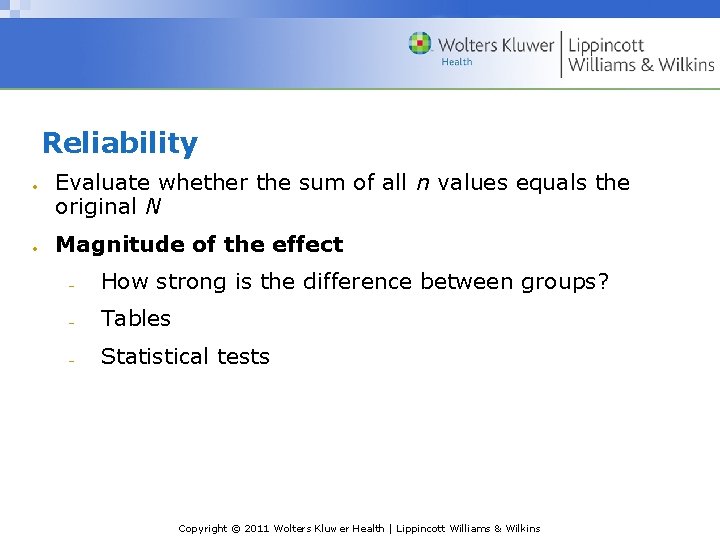
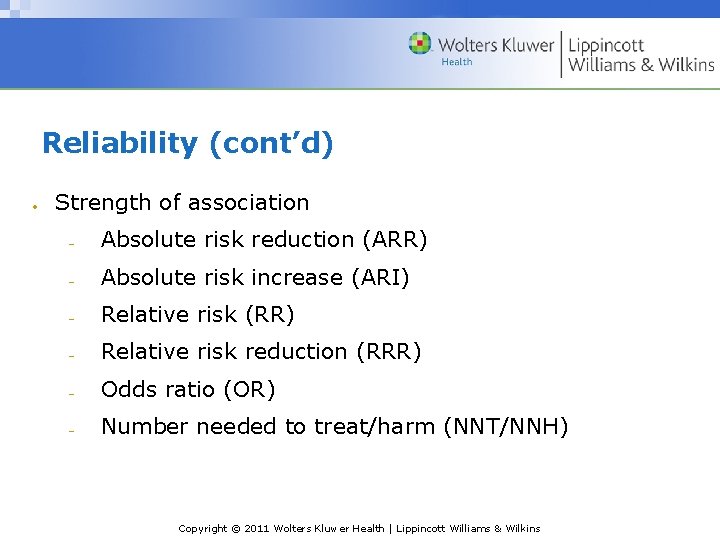
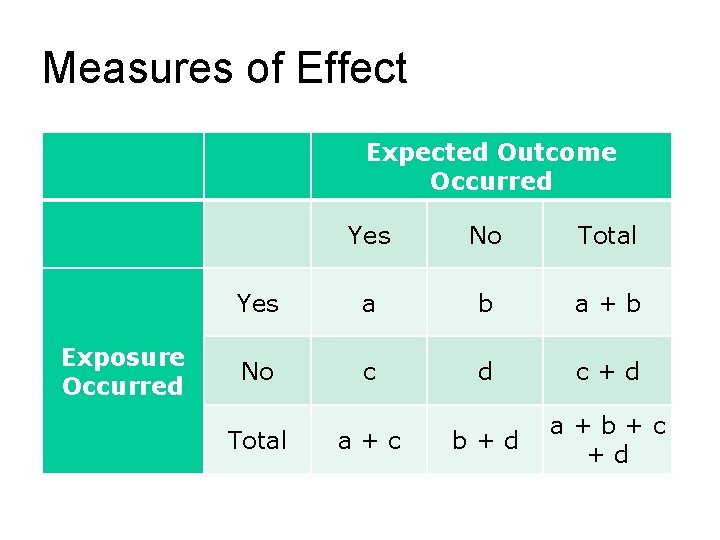
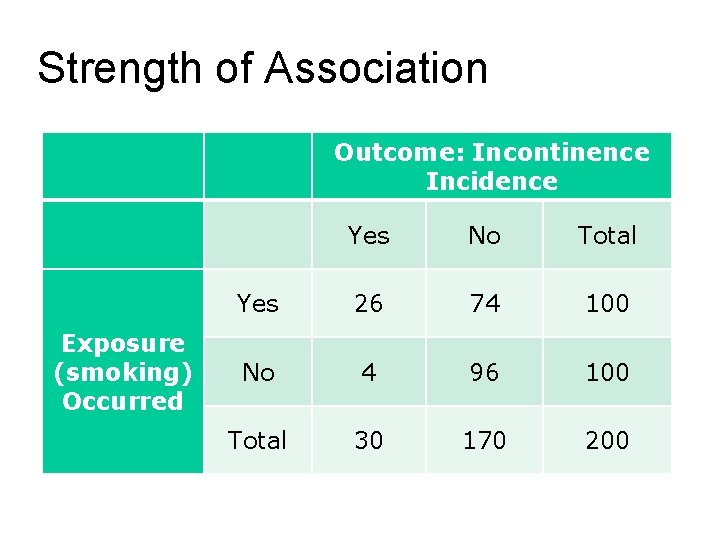
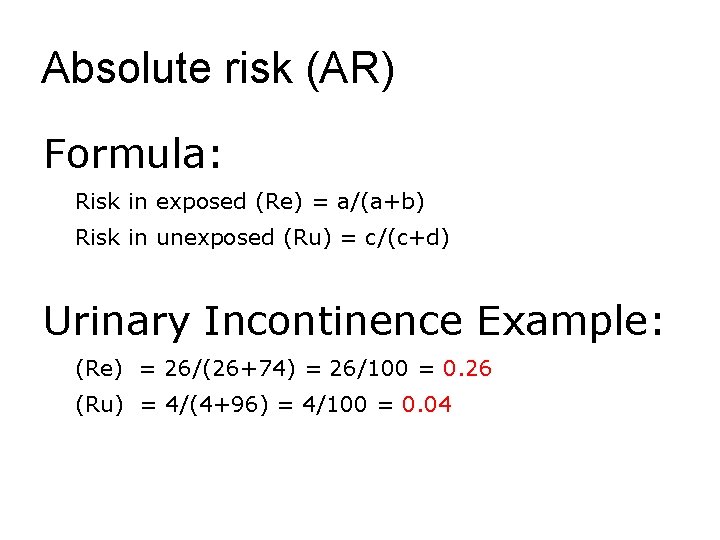
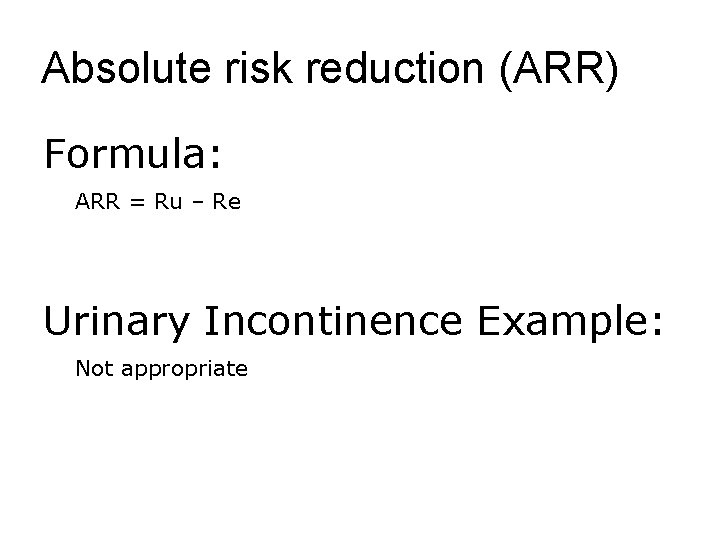
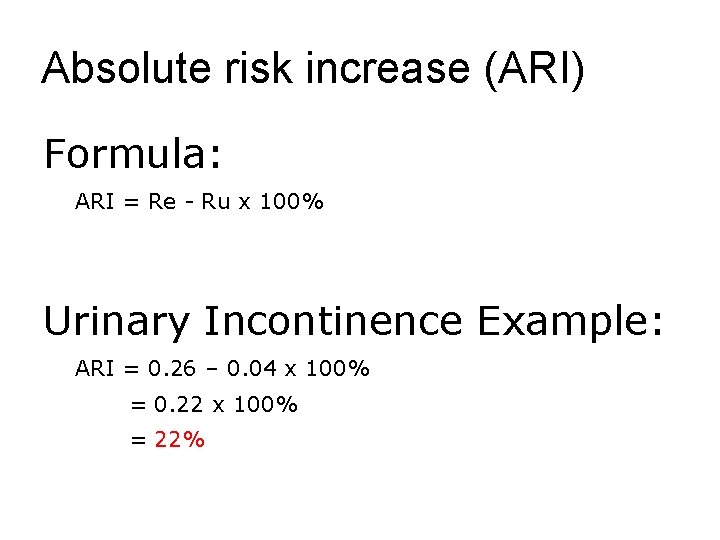
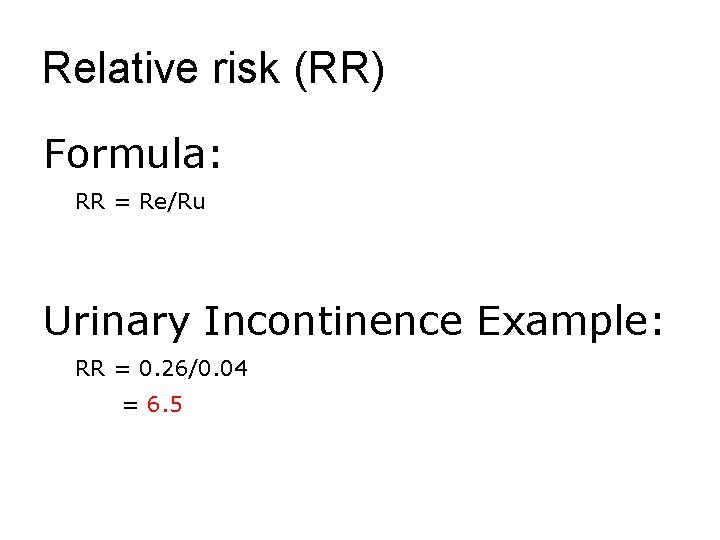
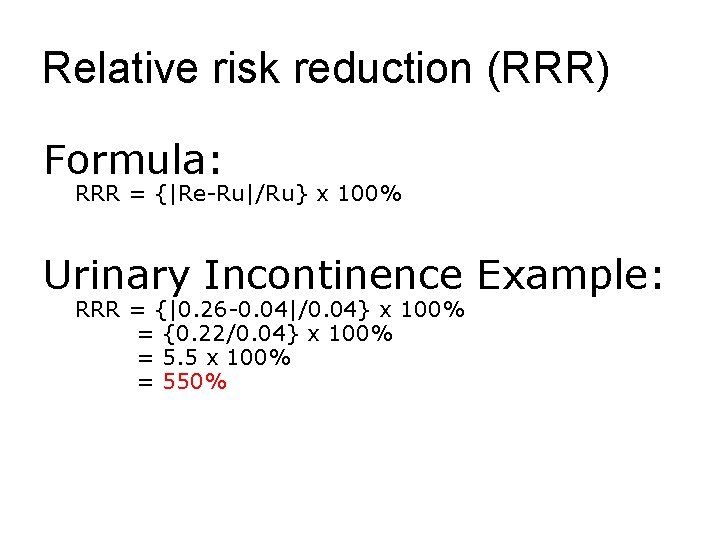
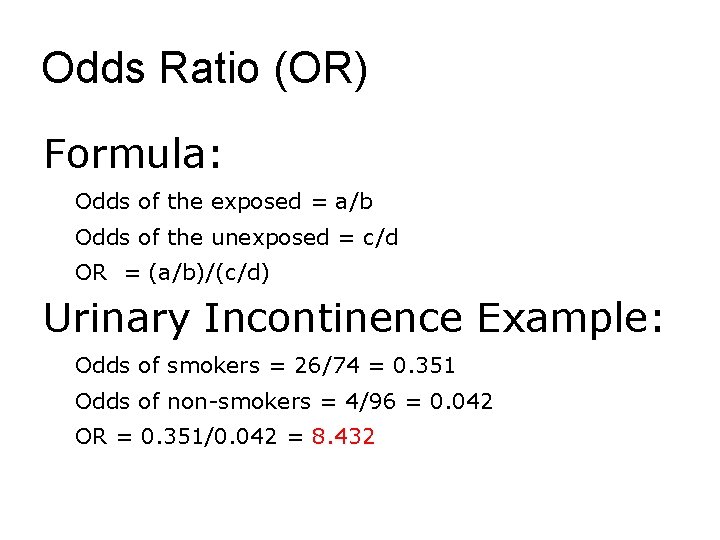
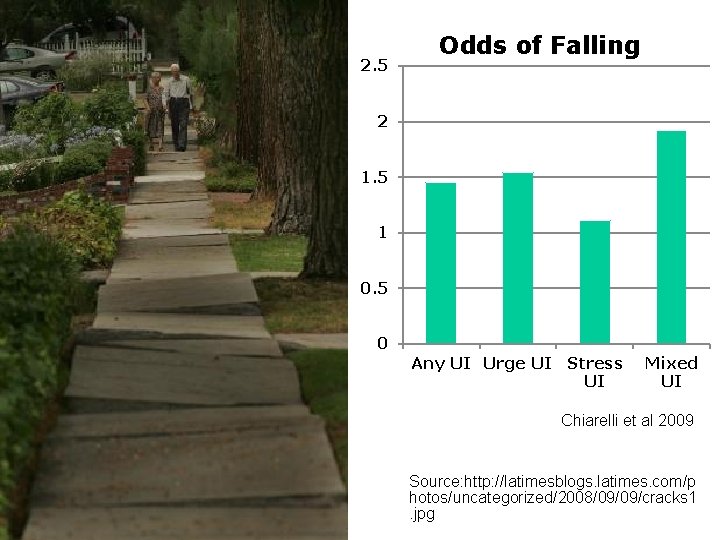
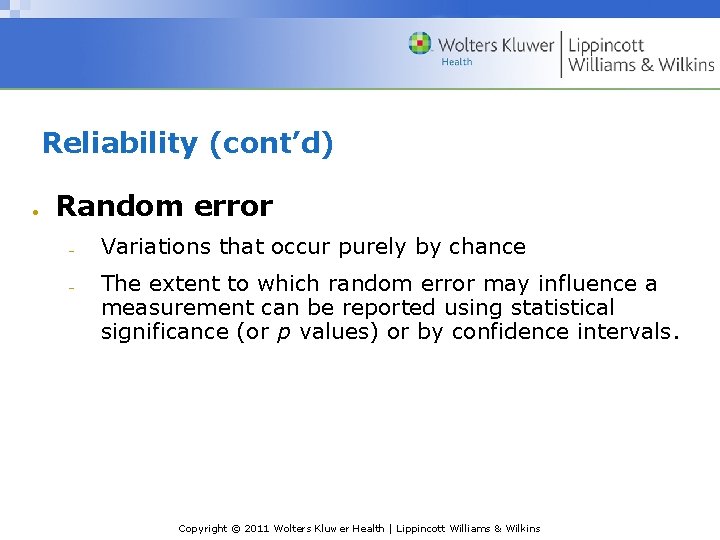
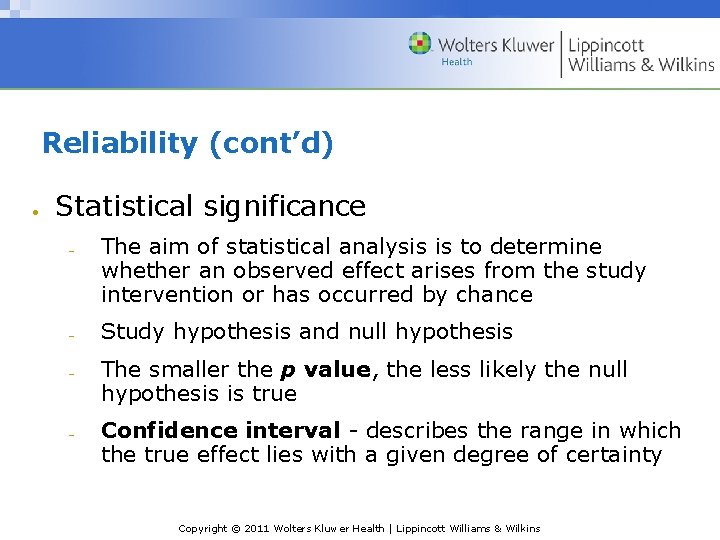
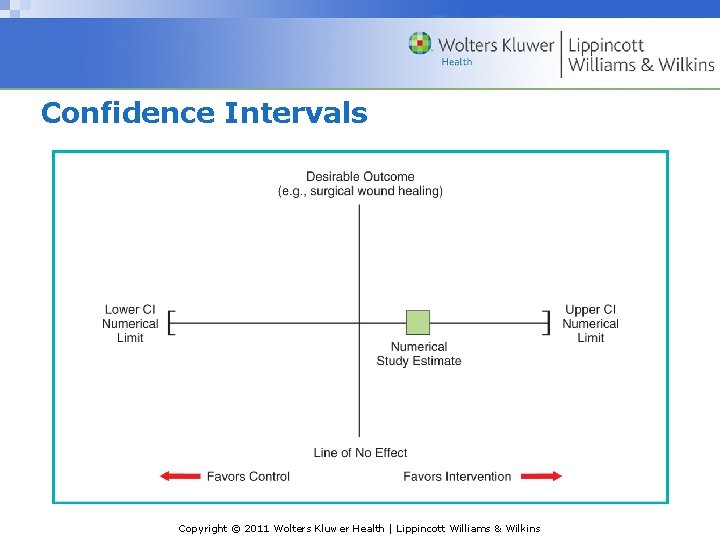
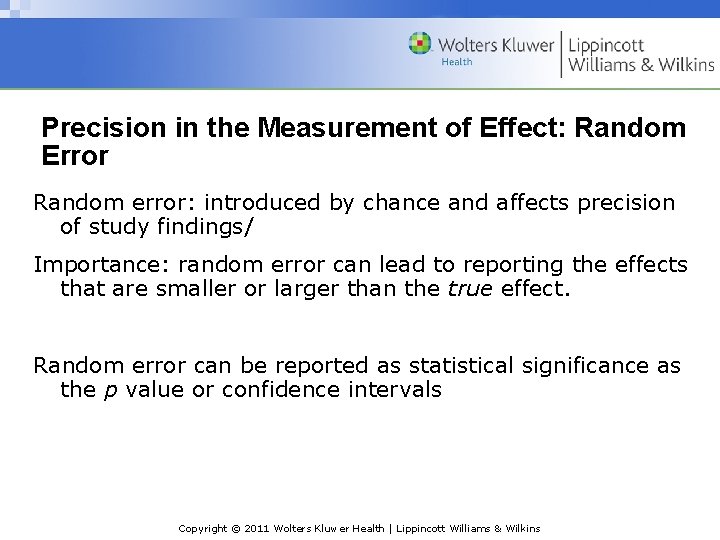
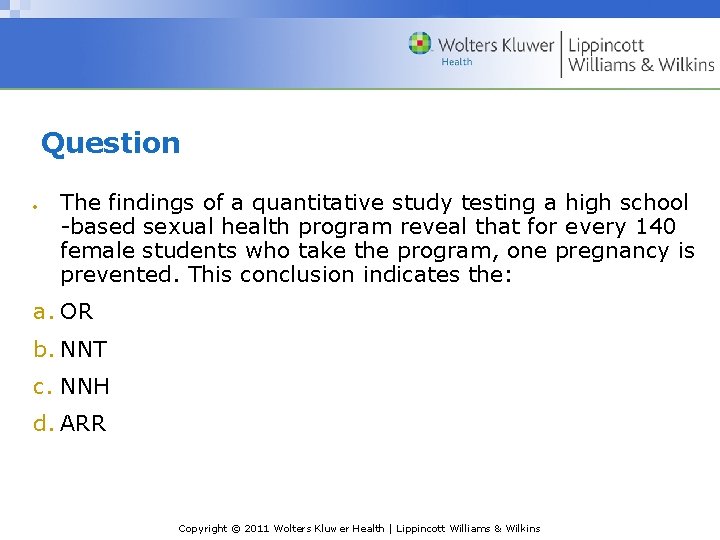
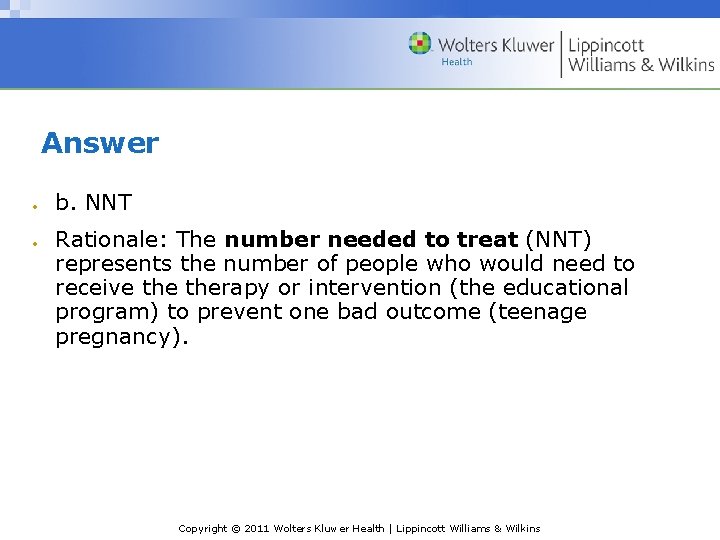
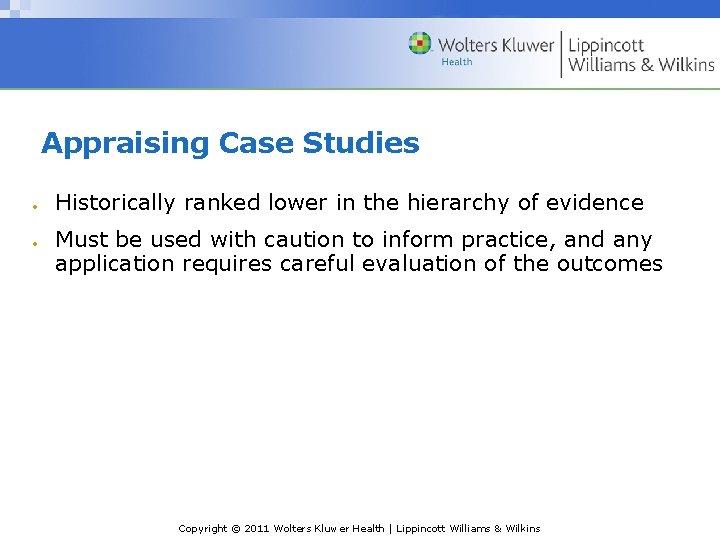
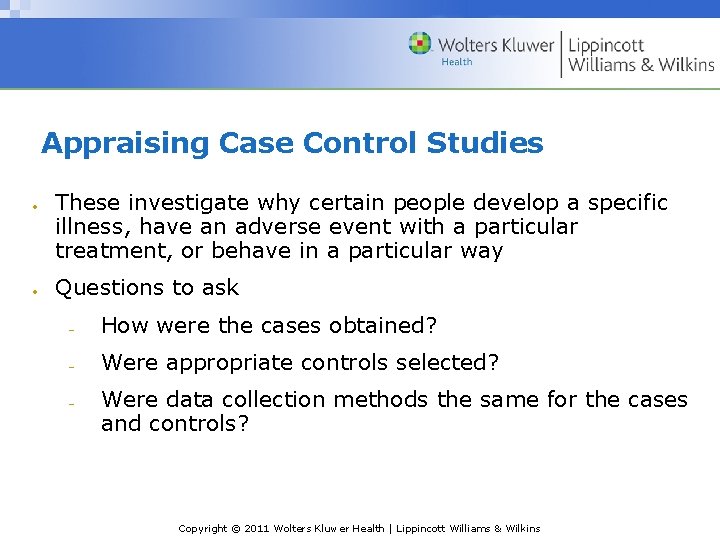
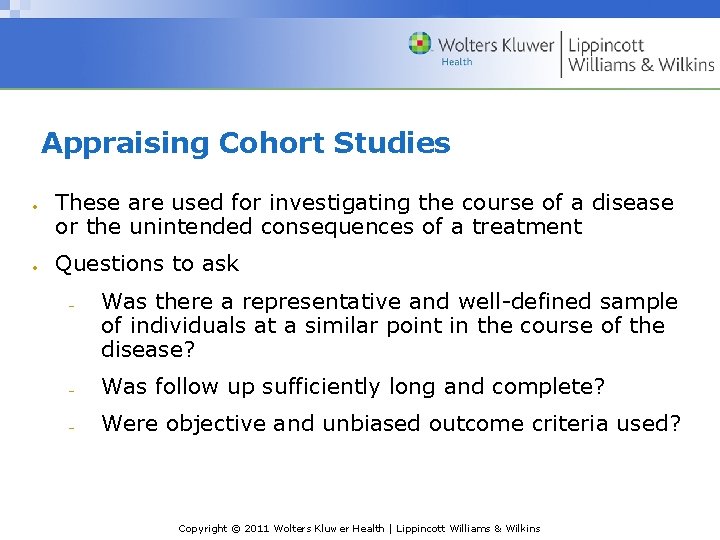
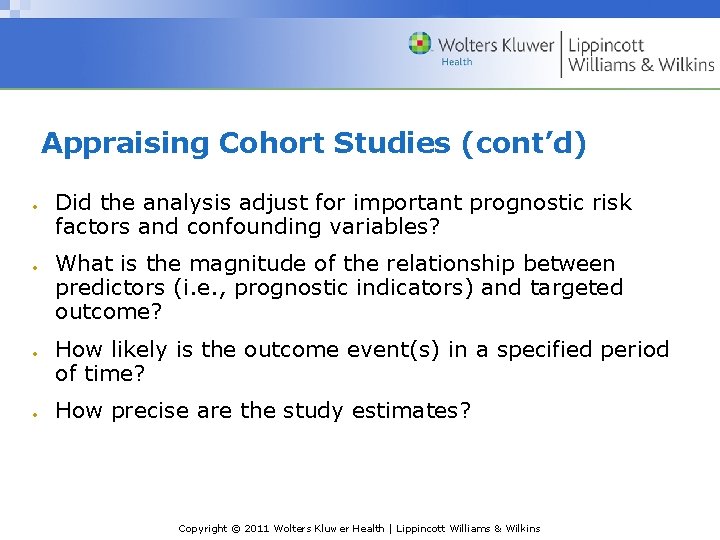
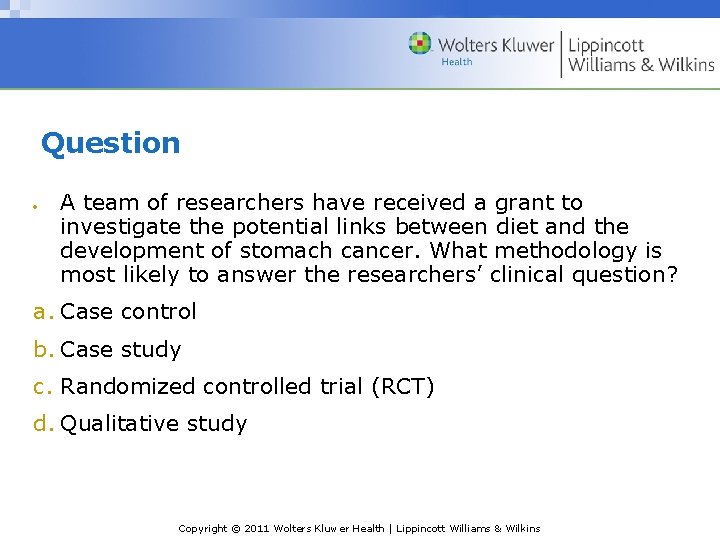
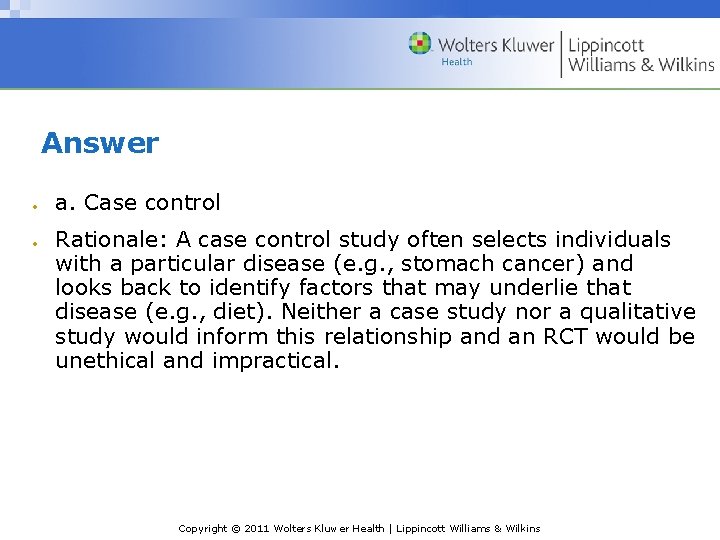
- Slides: 42
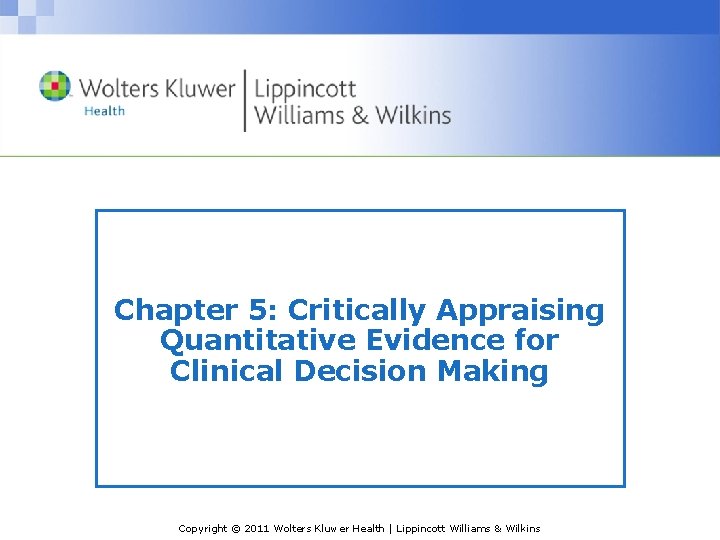
Chapter 5: Critically Appraising Quantitative Evidence for Clinical Decision Making Copyright © 2011 Wolters Kluwer Health | Lippincott Williams & Wilkins
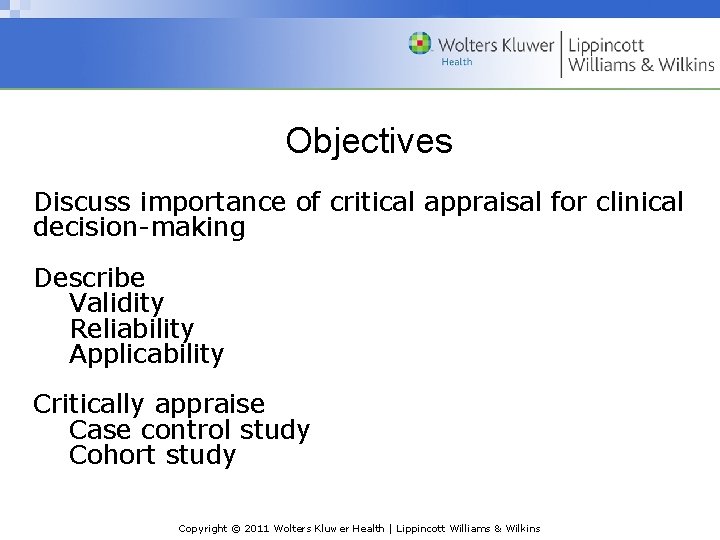
Objectives Discuss importance of critical appraisal for clinical decision-making Describe Validity Reliability Applicability Critically appraise Case control study Cohort study Copyright © 2011 Wolters Kluwer Health | Lippincott Williams & Wilkins
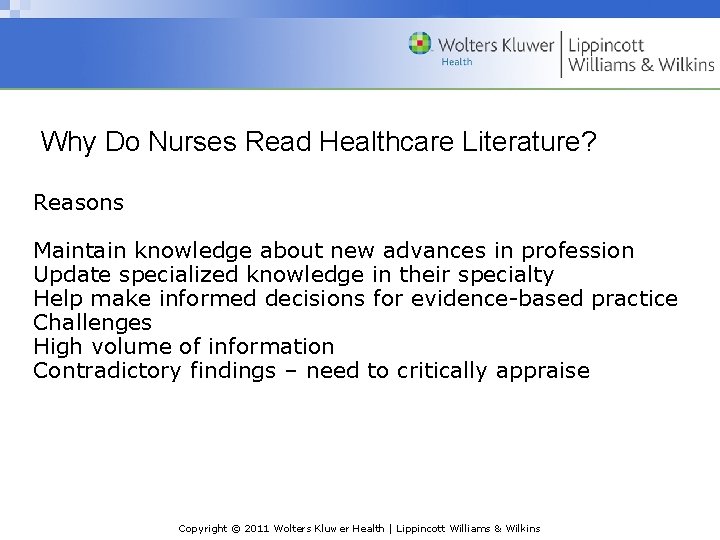
Why Do Nurses Read Healthcare Literature? Reasons Maintain knowledge about new advances in profession Update specialized knowledge in their specialty Help make informed decisions for evidence-based practice Challenges High volume of information Contradictory findings – need to critically appraise Copyright © 2011 Wolters Kluwer Health | Lippincott Williams & Wilkins
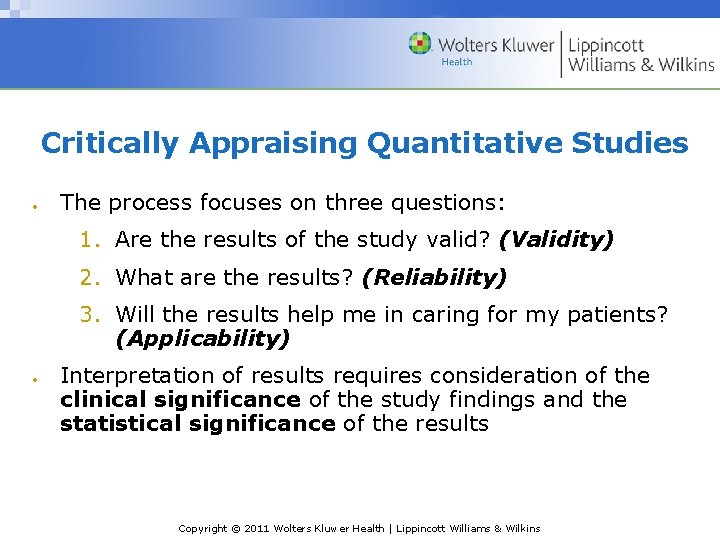
Critically Appraising Quantitative Studies The process focuses on three questions: 1. Are the results of the study valid? (Validity) 2. What are the results? (Reliability) 3. Will the results help me in caring for my patients? (Applicability) Interpretation of results requires consideration of the clinical significance of the study findings and the statistical significance of the results Copyright © 2011 Wolters Kluwer Health | Lippincott Williams & Wilkins
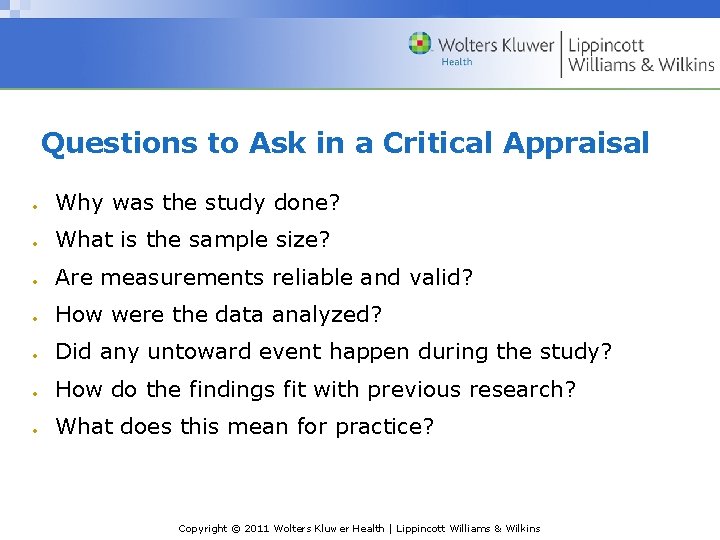
Questions to Ask in a Critical Appraisal Why was the study done? What is the sample size? Are measurements reliable and valid? How were the data analyzed? Did any untoward event happen during the study? How do the findings fit with previous research? What does this mean for practice? Copyright © 2011 Wolters Kluwer Health | Lippincott Williams & Wilkins
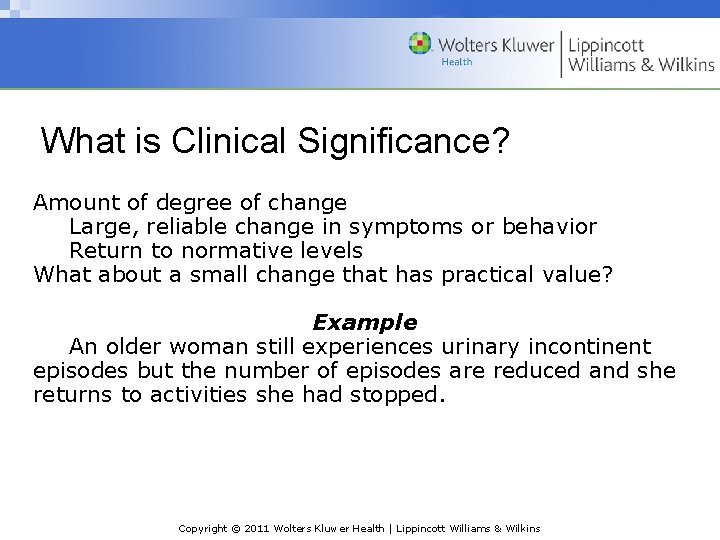
What is Clinical Significance? Amount of degree of change Large, reliable change in symptoms or behavior Return to normative levels What about a small change that has practical value? Example An older woman still experiences urinary incontinent episodes but the number of episodes are reduced and she returns to activities she had stopped. Copyright © 2011 Wolters Kluwer Health | Lippincott Williams & Wilkins
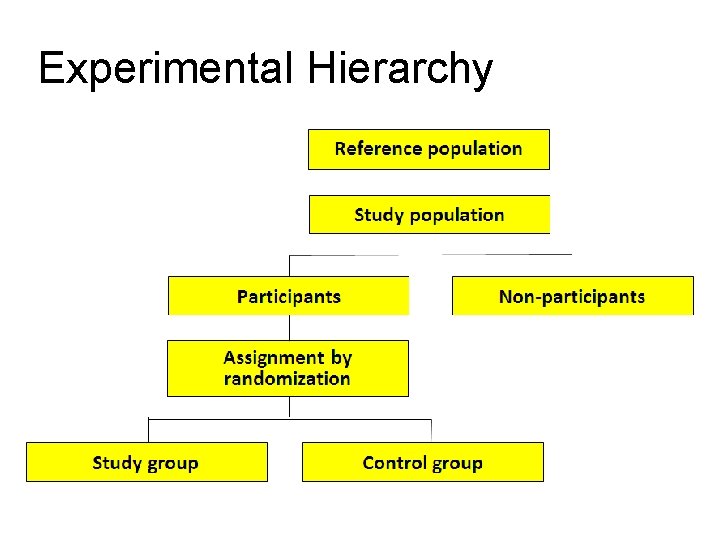
Experimental Hierarchy
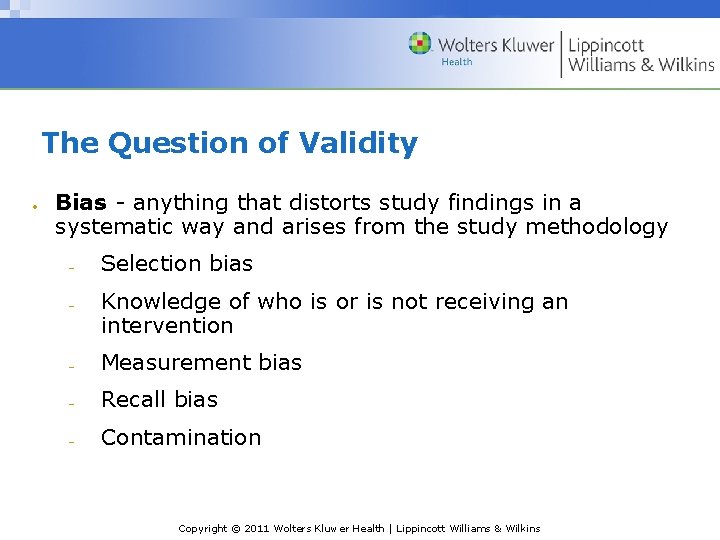
The Question of Validity Bias - anything that distorts study findings in a systematic way and arises from the study methodology Selection bias Knowledge of who is or is not receiving an intervention Measurement bias Recall bias Contamination Copyright © 2011 Wolters Kluwer Health | Lippincott Williams & Wilkins
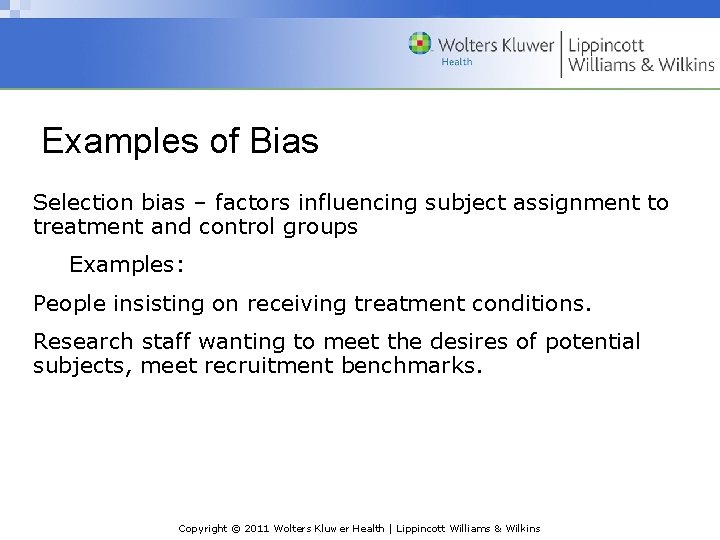
Examples of Bias Selection bias – factors influencing subject assignment to treatment and control groups Examples: People insisting on receiving treatment conditions. Research staff wanting to meet the desires of potential subjects, meet recruitment benchmarks. Copyright © 2011 Wolters Kluwer Health | Lippincott Williams & Wilkins
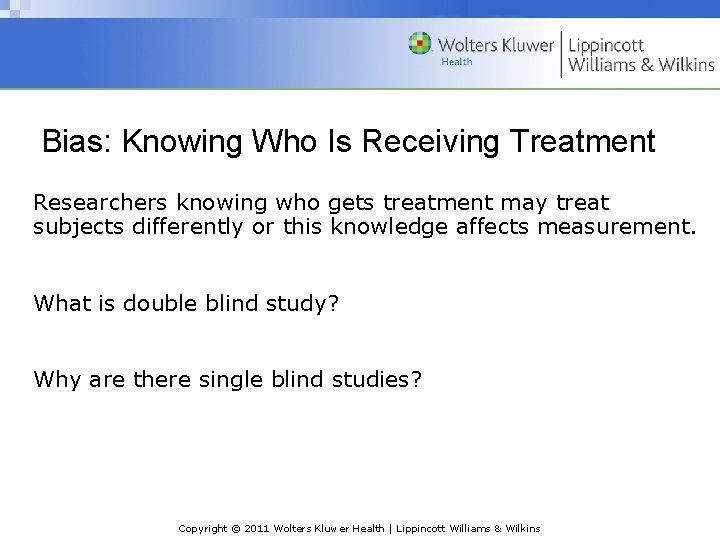
Bias: Knowing Who Is Receiving Treatment Researchers knowing who gets treatment may treat subjects differently or this knowledge affects measurement. What is double blind study? Why are there single blind studies? Copyright © 2011 Wolters Kluwer Health | Lippincott Williams & Wilkins
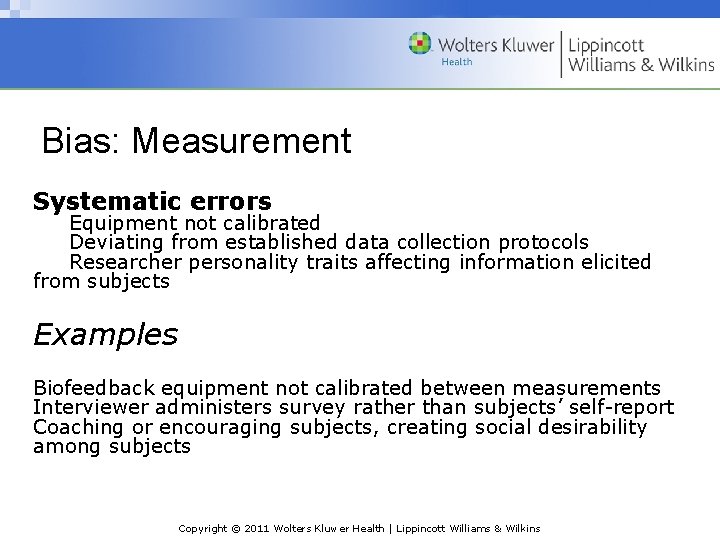
Bias: Measurement Systematic errors Equipment not calibrated Deviating from established data collection protocols Researcher personality traits affecting information elicited from subjects Examples Biofeedback equipment not calibrated between measurements Interviewer administers survey rather than subjects’ self-report Coaching or encouraging subjects, creating social desirability among subjects Copyright © 2011 Wolters Kluwer Health | Lippincott Williams & Wilkins
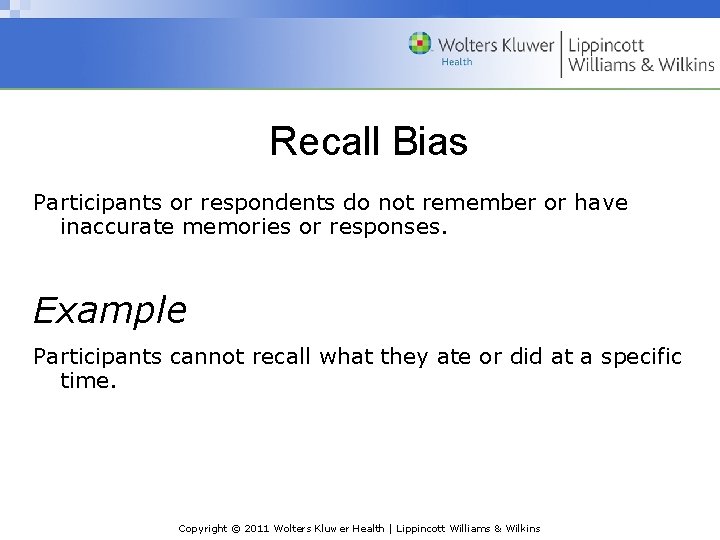
Recall Bias Participants or respondents do not remember or have inaccurate memories or responses. Example Participants cannot recall what they ate or did at a specific time. Copyright © 2011 Wolters Kluwer Health | Lippincott Williams & Wilkins
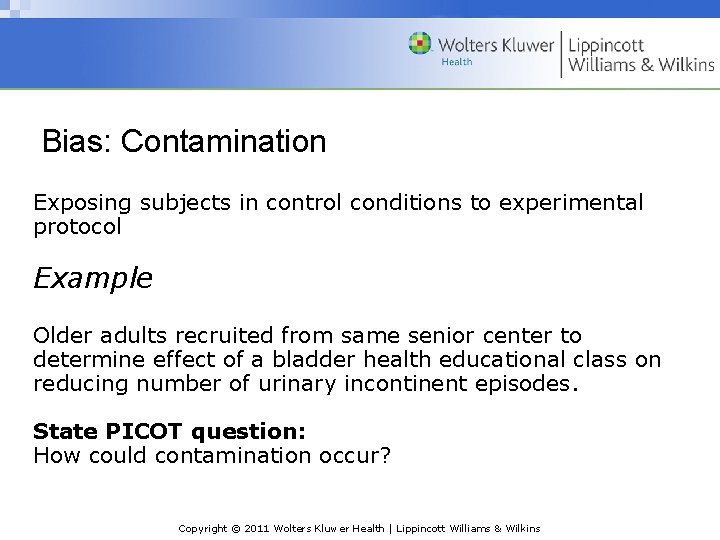
Bias: Contamination Exposing subjects in control conditions to experimental protocol Example Older adults recruited from same senior center to determine effect of a bladder health educational class on reducing number of urinary incontinent episodes. State PICOT question: How could contamination occur? Copyright © 2011 Wolters Kluwer Health | Lippincott Williams & Wilkins
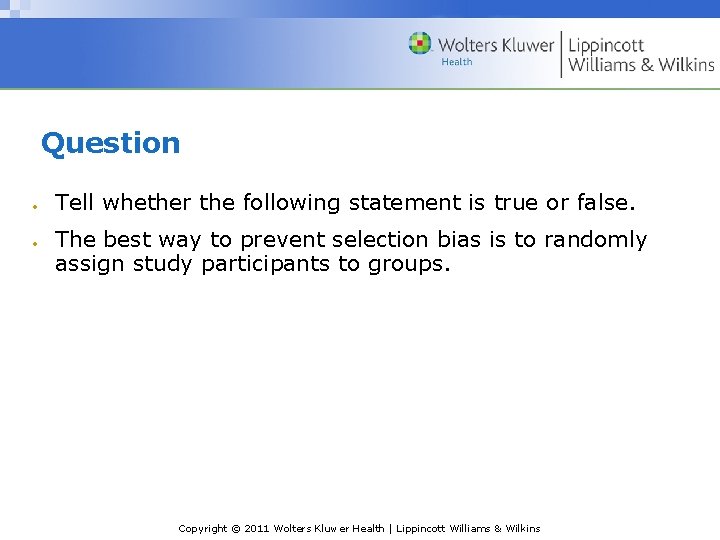
Question Tell whether the following statement is true or false. The best way to prevent selection bias is to randomly assign study participants to groups. Copyright © 2011 Wolters Kluwer Health | Lippincott Williams & Wilkins
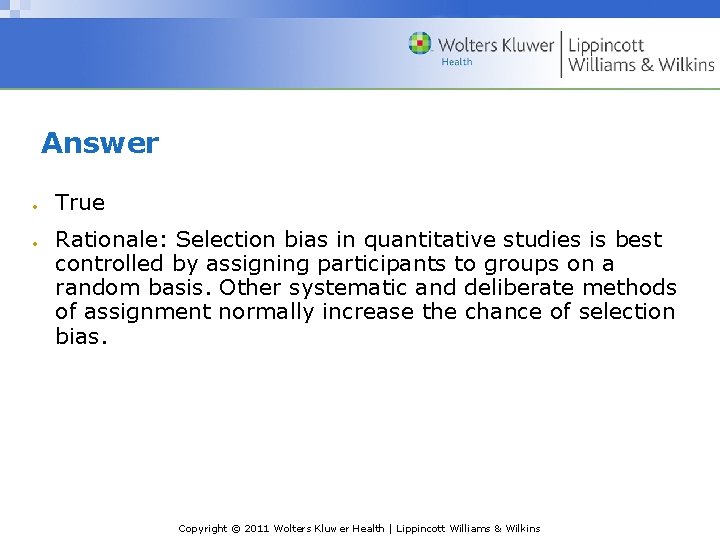
Answer True Rationale: Selection bias in quantitative studies is best controlled by assigning participants to groups on a random basis. Other systematic and deliberate methods of assignment normally increase the chance of selection bias. Copyright © 2011 Wolters Kluwer Health | Lippincott Williams & Wilkins
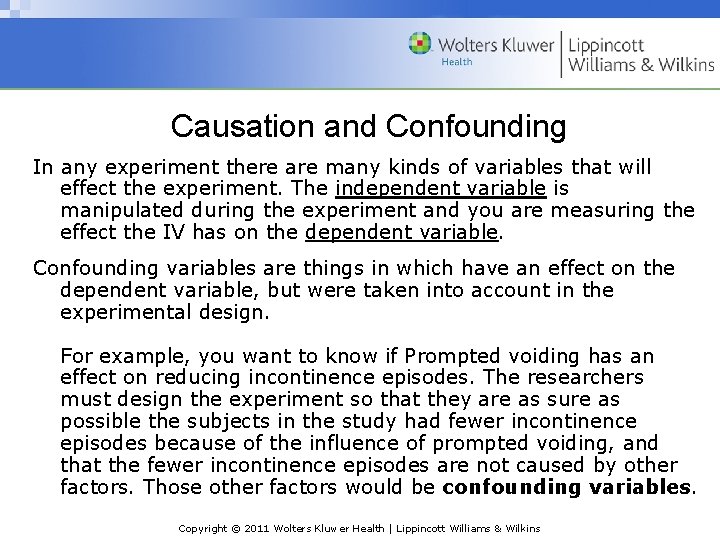
Causation and Confounding In any experiment there are many kinds of variables that will effect the experiment. The independent variable is manipulated during the experiment and you are measuring the effect the IV has on the dependent variable. Confounding variables are things in which have an effect on the dependent variable, but were taken into account in the experimental design. For example, you want to know if Prompted voiding has an effect on reducing incontinence episodes. The researchers must design the experiment so that they are as sure as possible the subjects in the study had fewer incontinence episodes because of the influence of prompted voiding, and that the fewer incontinence episodes are not caused by other factors. Those other factors would be confounding variables. Copyright © 2011 Wolters Kluwer Health | Lippincott Williams & Wilkins
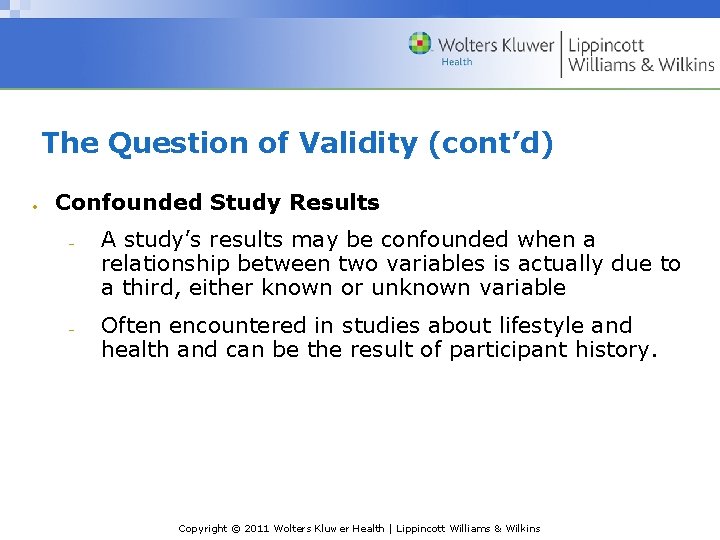
The Question of Validity (cont’d) Confounded Study Results A study’s results may be confounded when a relationship between two variables is actually due to a third, either known or unknown variable Often encountered in studies about lifestyle and health and can be the result of participant history. Copyright © 2011 Wolters Kluwer Health | Lippincott Williams & Wilkins
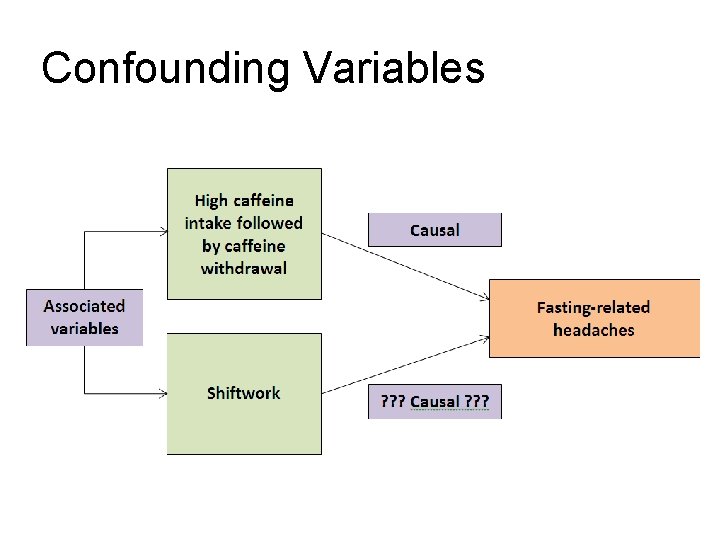
Confounding Variables
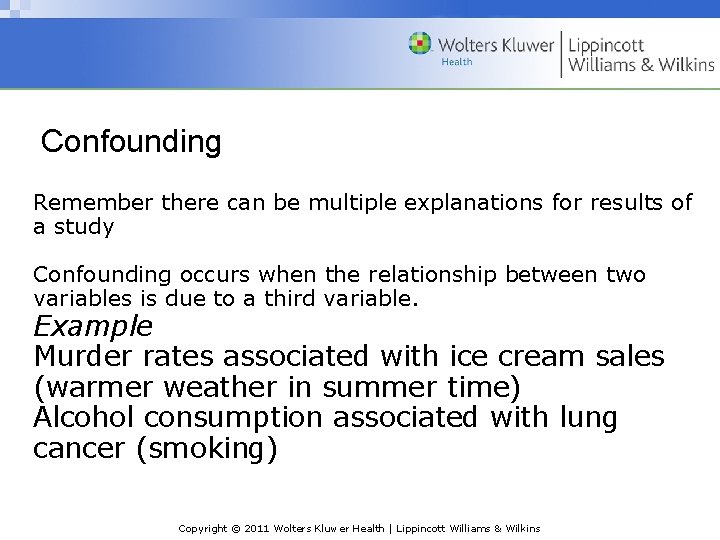
Confounding Remember there can be multiple explanations for results of a study Confounding occurs when the relationship between two variables is due to a third variable. Example Murder rates associated with ice cream sales (warmer weather in summer time) Alcohol consumption associated with lung cancer (smoking) Copyright © 2011 Wolters Kluwer Health | Lippincott Williams & Wilkins
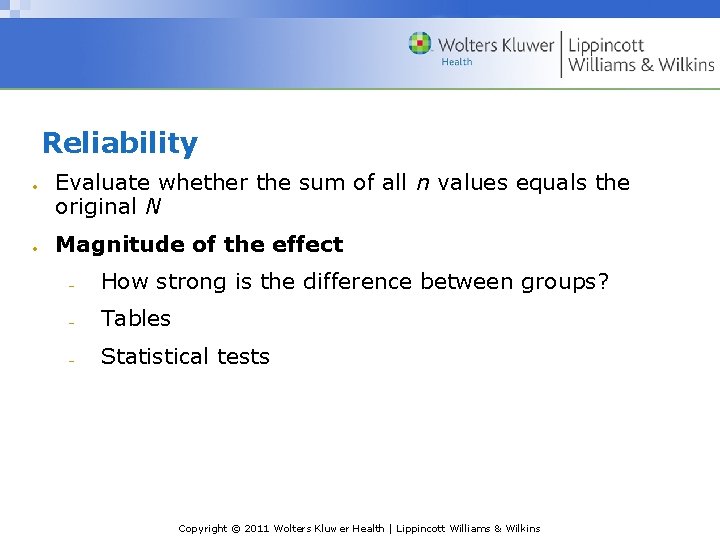
Reliability Evaluate whether the sum of all n values equals the original N Magnitude of the effect How strong is the difference between groups? Tables Statistical tests Copyright © 2011 Wolters Kluwer Health | Lippincott Williams & Wilkins
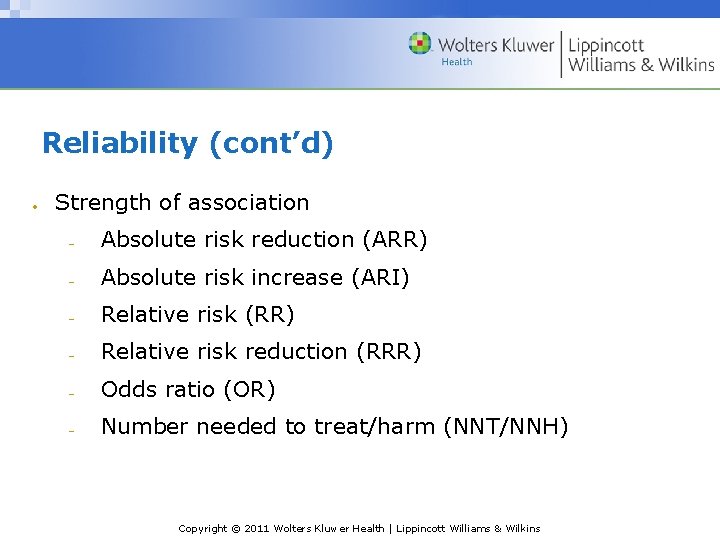
Reliability (cont’d) Strength of association Absolute risk reduction (ARR) Absolute risk increase (ARI) Relative risk (RR) Relative risk reduction (RRR) Odds ratio (OR) Number needed to treat/harm (NNT/NNH) Copyright © 2011 Wolters Kluwer Health | Lippincott Williams & Wilkins
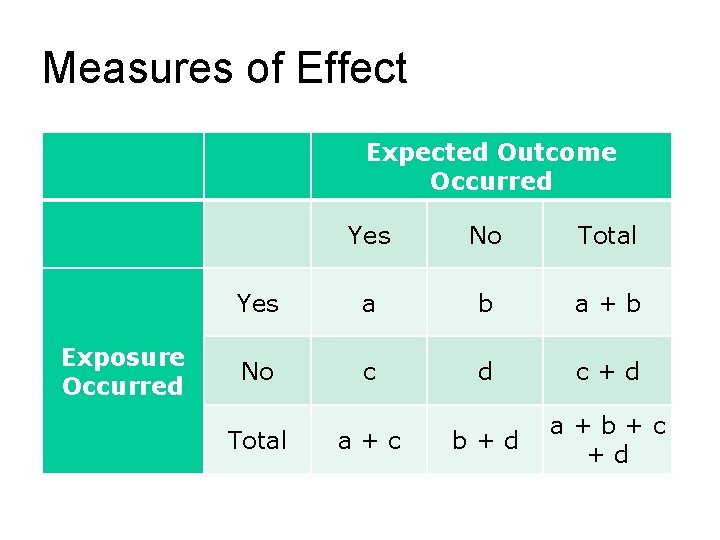
Measures of Effect Expected Outcome Occurred Yes No Total Yes a b a + b No c d c + d b + d a + b + c + d Exposure Occurred Total a + c
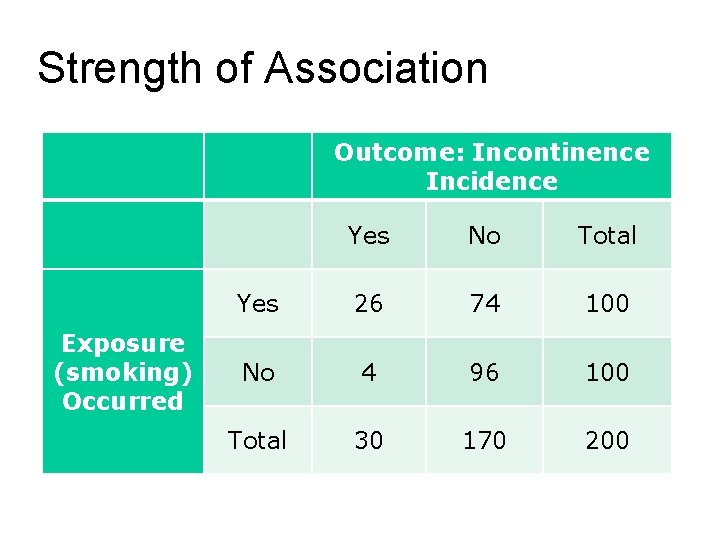
Strength of Association Outcome: Incontinence Incidence Yes No Total Yes 26 74 100 No 4 96 100 Total 30 170 200 Exposure (smoking) Occurred
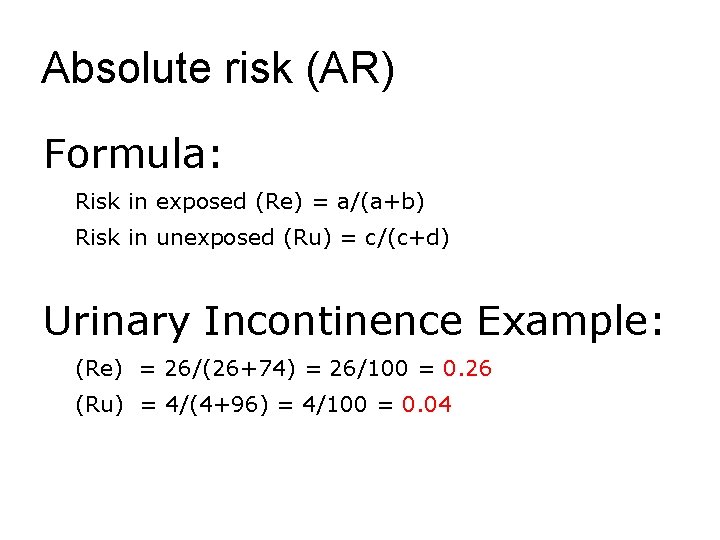
Absolute risk (AR) Formula: Risk in exposed (Re) = a/(a+b) Risk in unexposed (Ru) = c/(c+d) Urinary Incontinence Example: (Re) = 26/(26+74) = 26/100 = 0. 26 (Ru) = 4/(4+96) = 4/100 = 0. 04
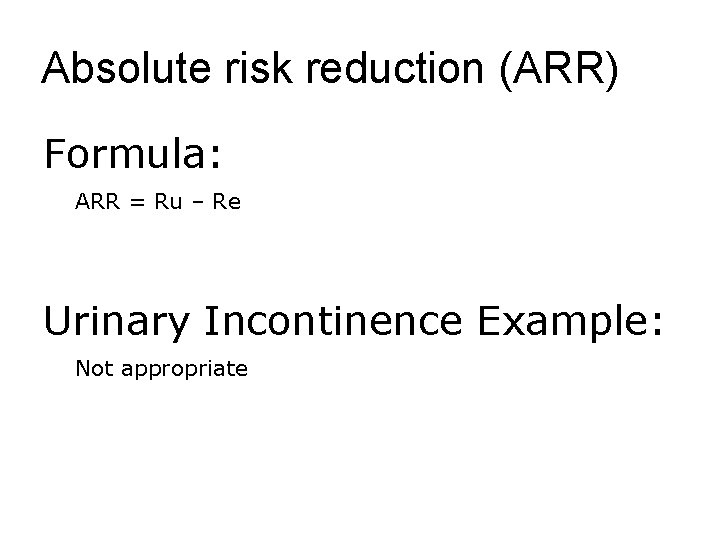
Absolute risk reduction (ARR) Formula: ARR = Ru – Re Urinary Incontinence Example: Not appropriate
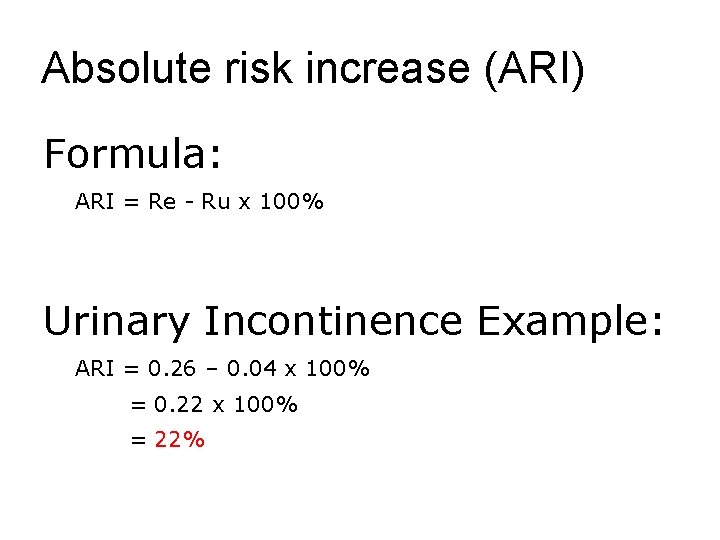
Absolute risk increase (ARI) Formula: ARI = Re - Ru x 100% Urinary Incontinence Example: ARI = 0. 26 – 0. 04 x 100% = 0. 22 x 100% = 22%
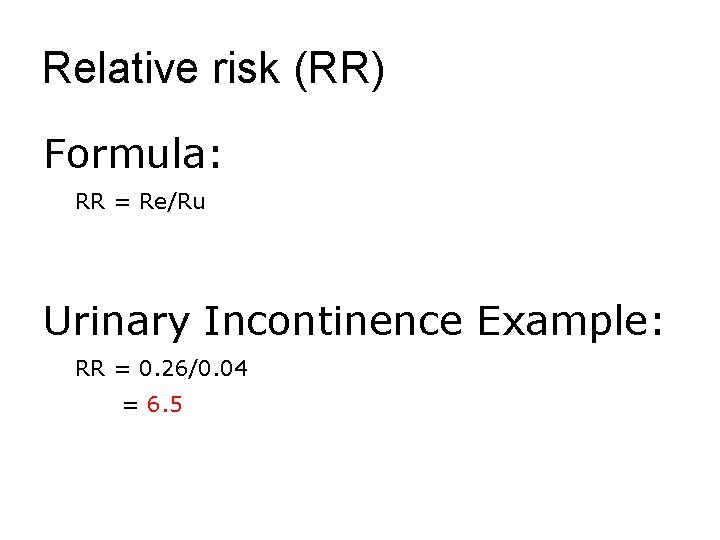
Relative risk (RR) Formula: RR = Re/Ru Urinary Incontinence Example: RR = 0. 26/0. 04 = 6. 5
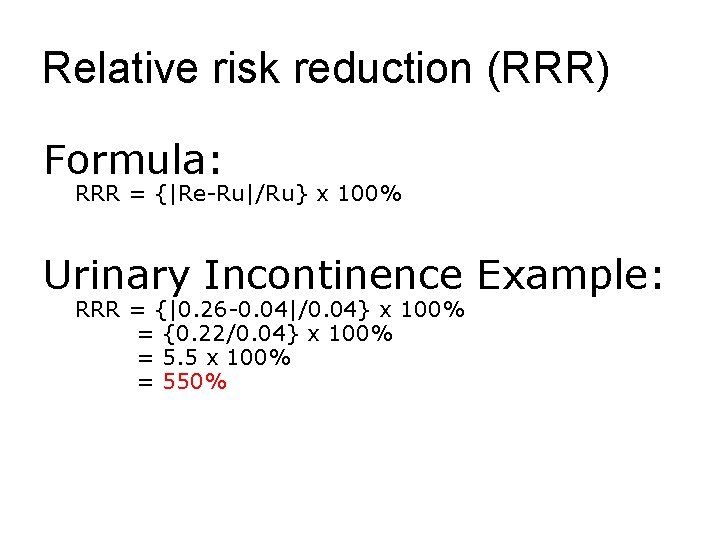
Relative risk reduction (RRR) Formula: RRR = {|Re-Ru|/Ru} x 100% Urinary Incontinence Example: RRR = {|0. 26 -0. 04|/0. 04} x 100% = {0. 22/0. 04} x 100% = 5. 5 x 100% = 550%
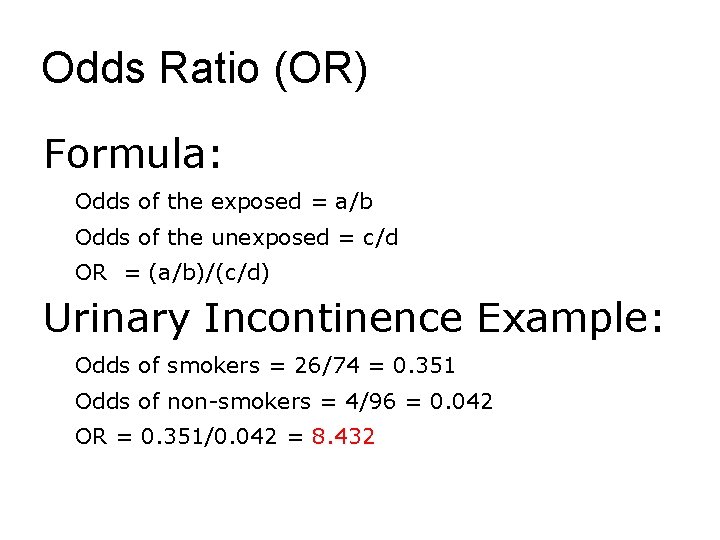
Odds Ratio (OR) Formula: Odds of the exposed = a/b Odds of the unexposed = c/d OR = (a/b)/(c/d) Urinary Incontinence Example: Odds of smokers = 26/74 = 0. 351 Odds of non-smokers = 4/96 = 0. 042 OR = 0. 351/0. 042 = 8. 432
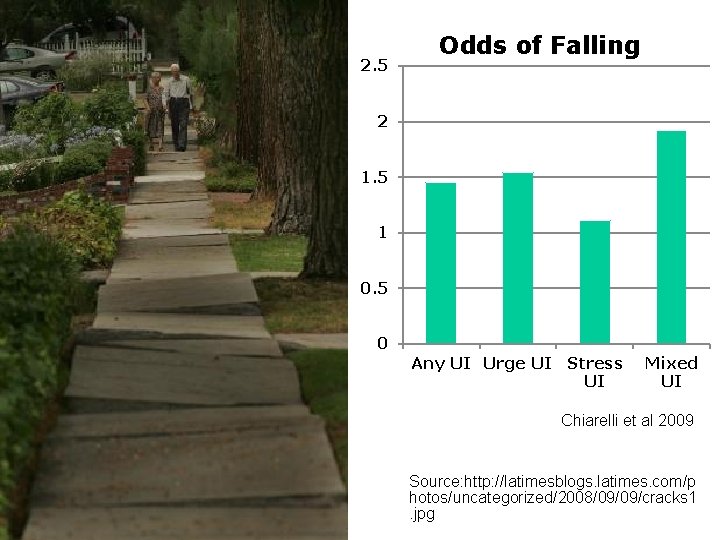
2. 5 Odds of Falling 2 1. 5 1 0. 5 0 Any UI Urge UI Stress UI Mixed UI Chiarelli et al 2009 Source: http: //latimesblogs. latimes. com/p hotos/uncategorized/2008/09/09/cracks 1. jpg
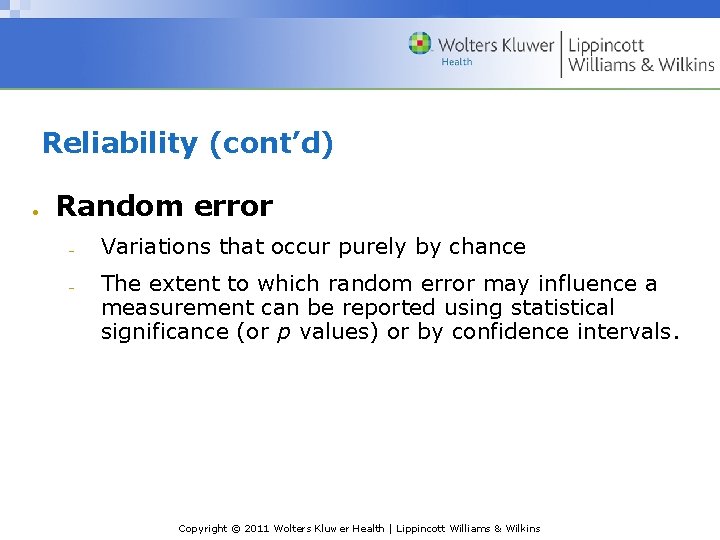
Reliability (cont’d) Random error Variations that occur purely by chance The extent to which random error may influence a measurement can be reported using statistical significance (or p values) or by confidence intervals. Copyright © 2011 Wolters Kluwer Health | Lippincott Williams & Wilkins
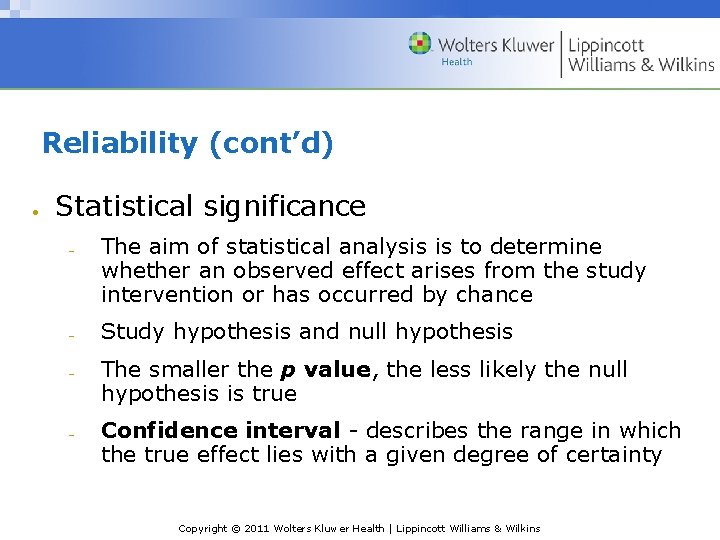
Reliability (cont’d) Statistical significance The aim of statistical analysis is to determine whether an observed effect arises from the study intervention or has occurred by chance Study hypothesis and null hypothesis The smaller the p value, the less likely the null hypothesis is true Confidence interval - describes the range in which the true effect lies with a given degree of certainty Copyright © 2011 Wolters Kluwer Health | Lippincott Williams & Wilkins
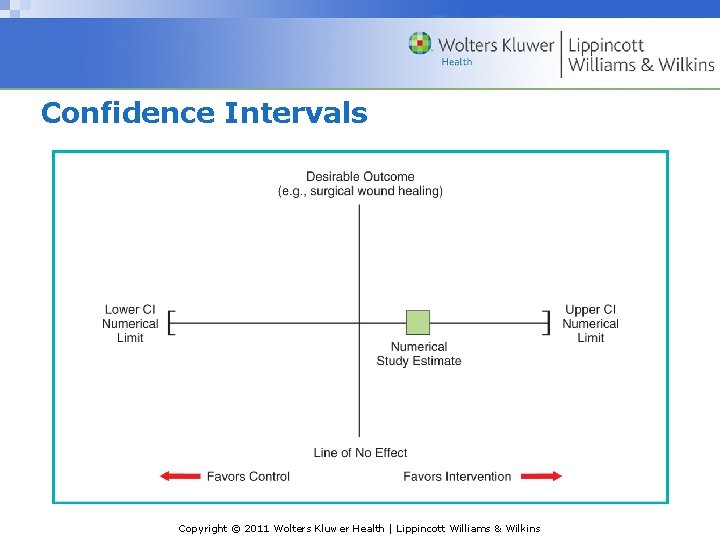
Confidence Intervals Copyright © 2011 Wolters Kluwer Health | Lippincott Williams & Wilkins
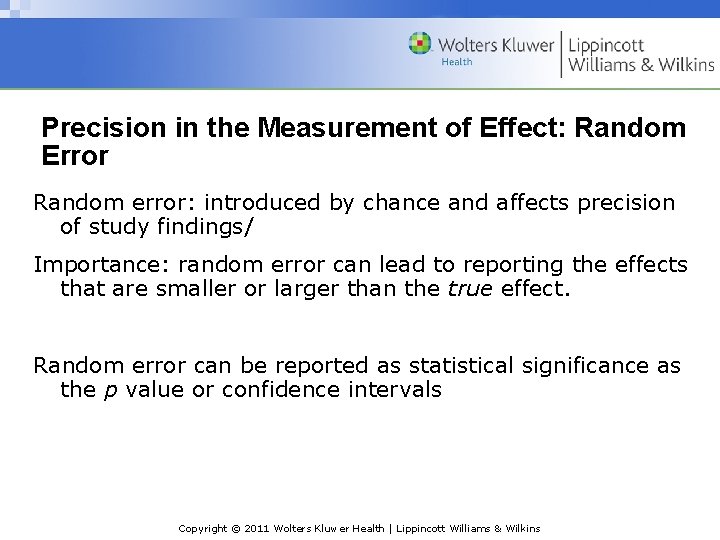
Precision in the Measurement of Effect: Random Error Random error: introduced by chance and affects precision of study findings/ Importance: random error can lead to reporting the effects that are smaller or larger than the true effect. Random error can be reported as statistical significance as the p value or confidence intervals Copyright © 2011 Wolters Kluwer Health | Lippincott Williams & Wilkins
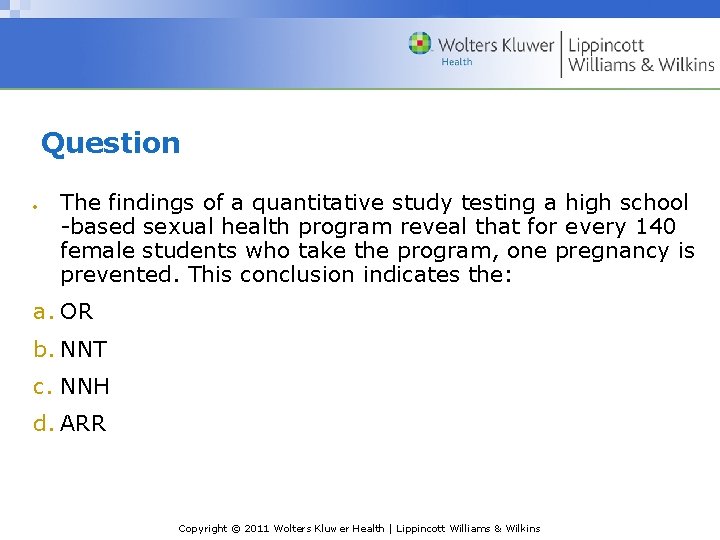
Question The findings of a quantitative study testing a high school -based sexual health program reveal that for every 140 female students who take the program, one pregnancy is prevented. This conclusion indicates the: a. OR b. NNT c. NNH d. ARR Copyright © 2011 Wolters Kluwer Health | Lippincott Williams & Wilkins
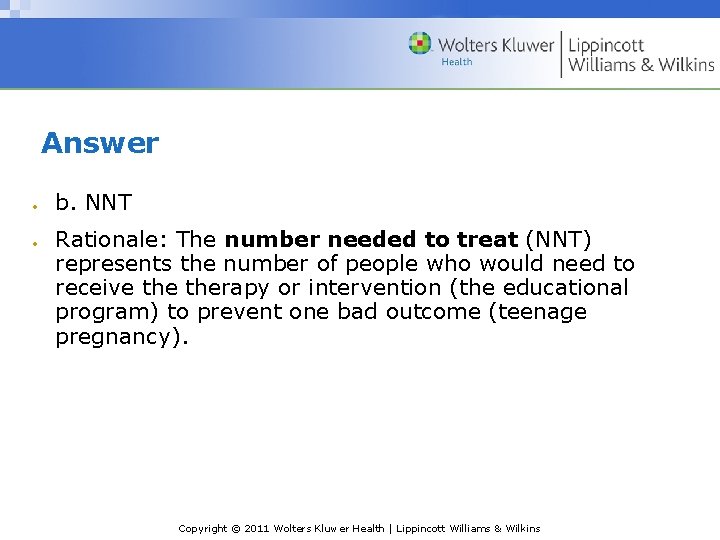
Answer b. NNT Rationale: The number needed to treat (NNT) represents the number of people who would need to receive therapy or intervention (the educational program) to prevent one bad outcome (teenage pregnancy). Copyright © 2011 Wolters Kluwer Health | Lippincott Williams & Wilkins
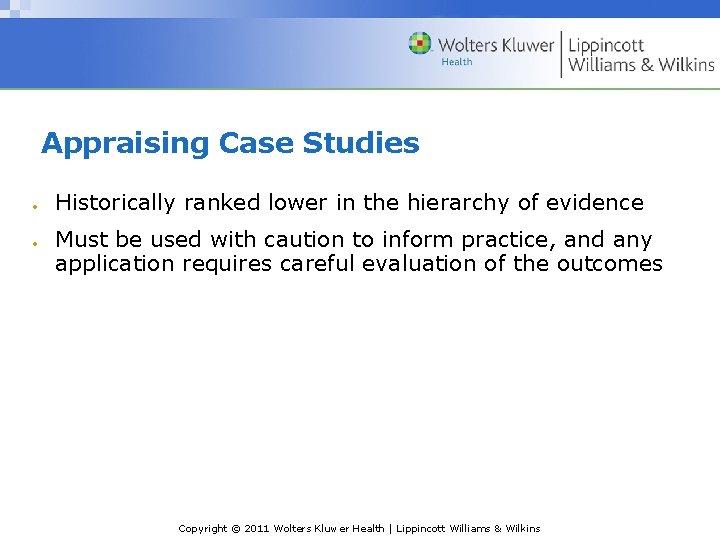
Appraising Case Studies Historically ranked lower in the hierarchy of evidence Must be used with caution to inform practice, and any application requires careful evaluation of the outcomes Copyright © 2011 Wolters Kluwer Health | Lippincott Williams & Wilkins
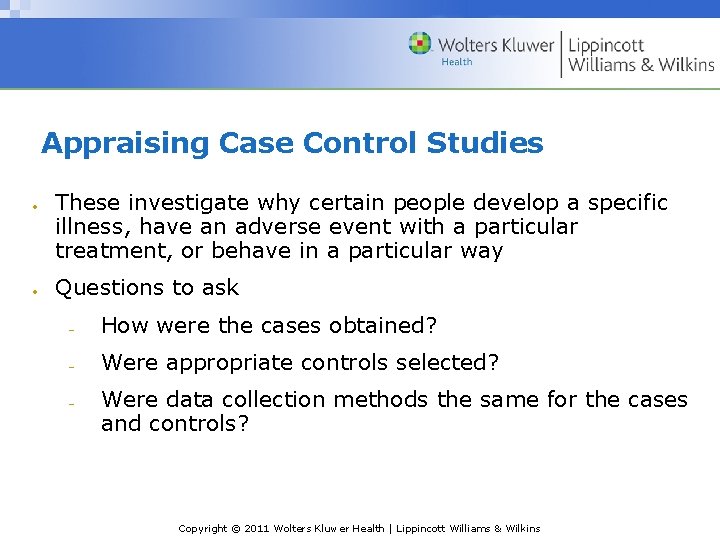
Appraising Case Control Studies These investigate why certain people develop a specific illness, have an adverse event with a particular treatment, or behave in a particular way Questions to ask How were the cases obtained? Were appropriate controls selected? Were data collection methods the same for the cases and controls? Copyright © 2011 Wolters Kluwer Health | Lippincott Williams & Wilkins
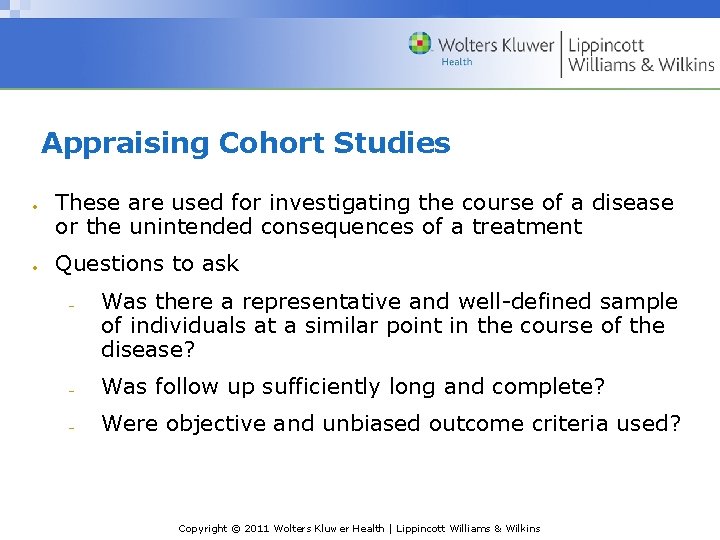
Appraising Cohort Studies These are used for investigating the course of a disease or the unintended consequences of a treatment Questions to ask Was there a representative and well-defined sample of individuals at a similar point in the course of the disease? Was follow up sufficiently long and complete? Were objective and unbiased outcome criteria used? Copyright © 2011 Wolters Kluwer Health | Lippincott Williams & Wilkins
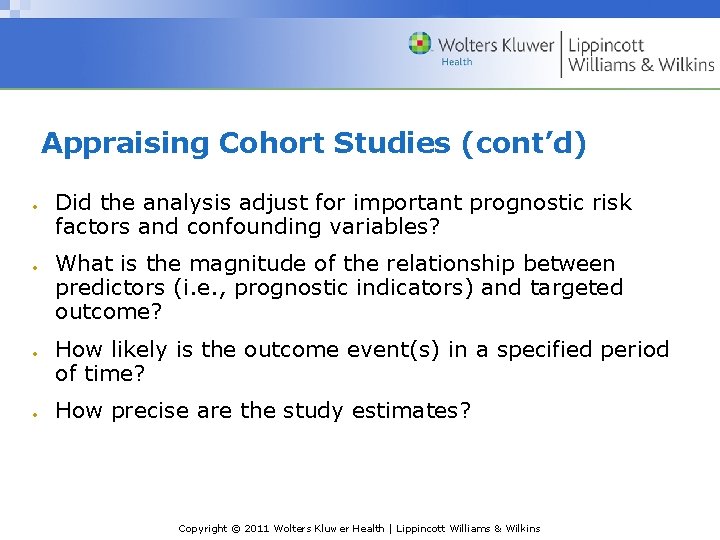
Appraising Cohort Studies (cont’d) Did the analysis adjust for important prognostic risk factors and confounding variables? What is the magnitude of the relationship between predictors (i. e. , prognostic indicators) and targeted outcome? How likely is the outcome event(s) in a specified period of time? How precise are the study estimates? Copyright © 2011 Wolters Kluwer Health | Lippincott Williams & Wilkins
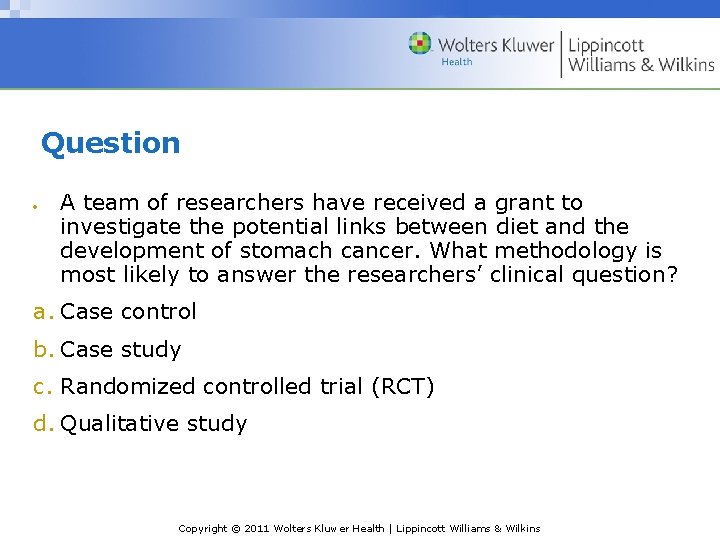
Question A team of researchers have received a grant to investigate the potential links between diet and the development of stomach cancer. What methodology is most likely to answer the researchers’ clinical question? a. Case control b. Case study c. Randomized controlled trial (RCT) d. Qualitative study Copyright © 2011 Wolters Kluwer Health | Lippincott Williams & Wilkins
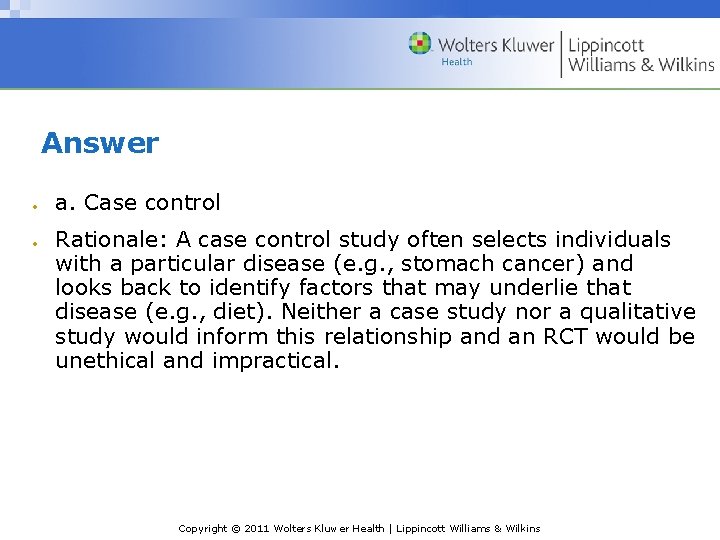
Answer a. Case control Rationale: A case control study often selects individuals with a particular disease (e. g. , stomach cancer) and looks back to identify factors that may underlie that disease (e. g. , diet). Neither a case study nor a qualitative study would inform this relationship and an RCT would be unethical and impractical. Copyright © 2011 Wolters Kluwer Health | Lippincott Williams & Wilkins
Appraising and rewarding performance
Appraising performance of office employees
Process of acquiring training appraising and compensating
Superfluous strengths examples
Listening and appraising music
Garfield assessment
Listening critically
Thinking critically with psychological science
Pico cat voorbeeld
Care of the critically ill surgical patient
Nasogastrio
Thinking critically with psychological science
Micro critical aseptic field
Thinking critically
Thinking critically
Importance of critical thinking in nursing process
Sonnet 18 ppt
Negative issues
Thinking critically with psychological science answer key
Micro critical aseptic field
Primary evidence vs secondary evidence
Primary evidence vs secondary evidence
Secondary sources
Primary evidence vs secondary evidence
Primary evidence vs secondary evidence
Fiber evidence can have probative value.
Class evidence vs individual evidence
How can class evidence be useful
Class and individual evidence
Ecological fallacy definition
Iso 22301 utbildning
Typiska novell drag
Nationell inriktning för artificiell intelligens
Vad står k.r.å.k.a.n för
Varför kallas perioden 1918-1939 för mellankrigstiden?
En lathund för arbete med kontinuitetshantering
Underlag för särskild löneskatt på pensionskostnader
Tidböcker
A gastrica
Förklara densitet för barn
Datorkunskap för nybörjare
Boverket ka
Hur skriver man en debattartikel