Chapter 16 Oneway Analysis of Concept Map Variance
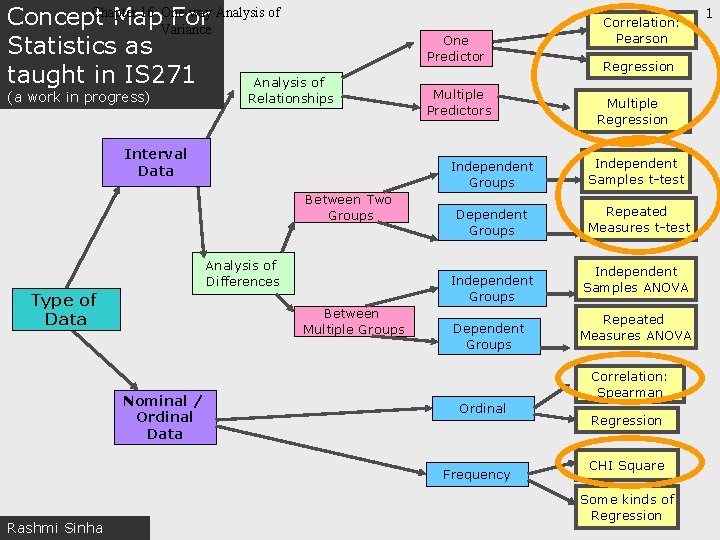
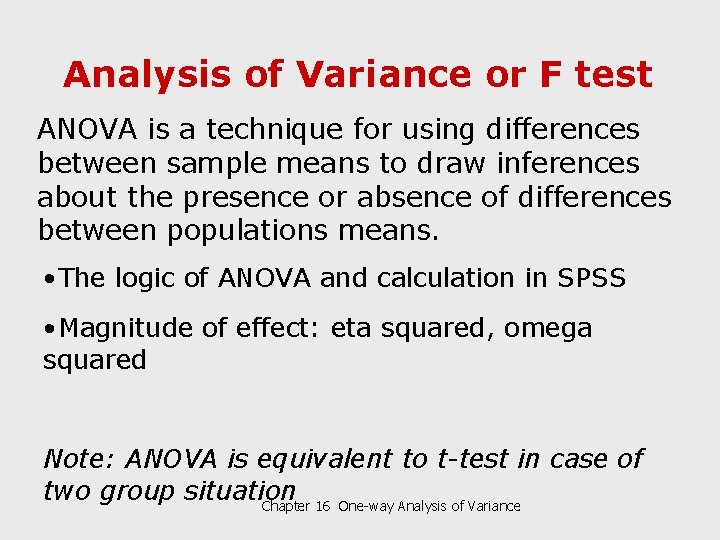
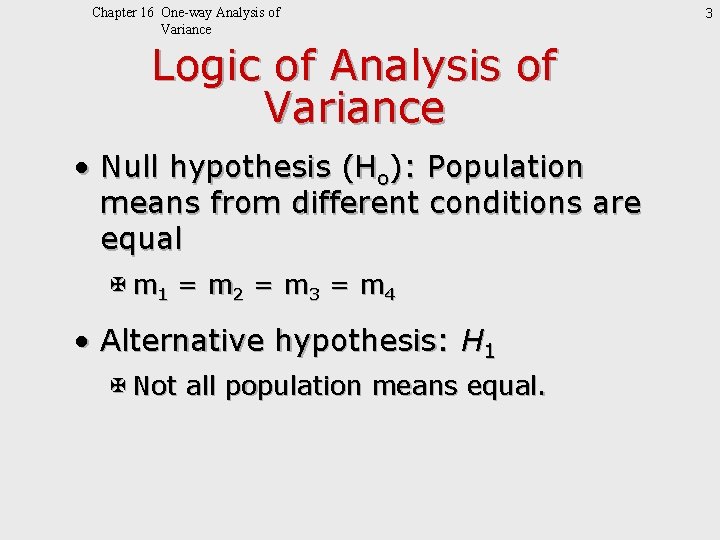
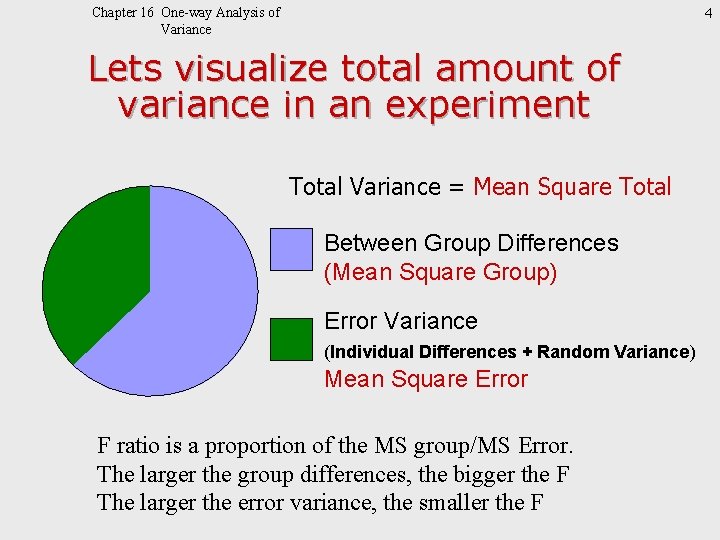
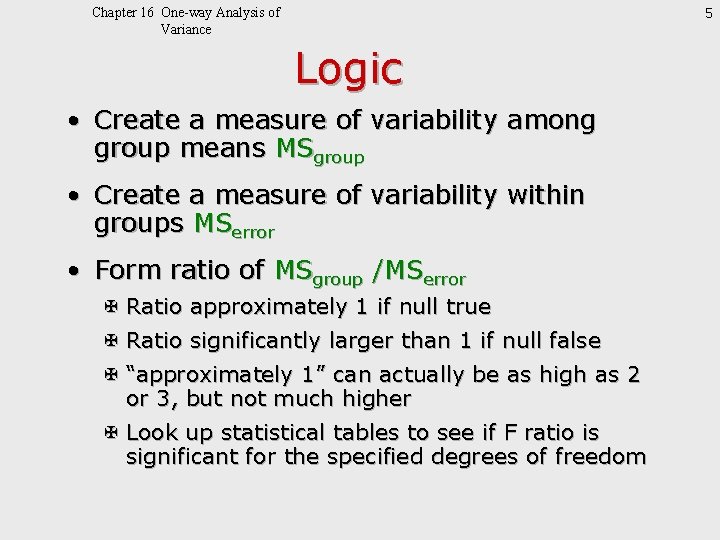
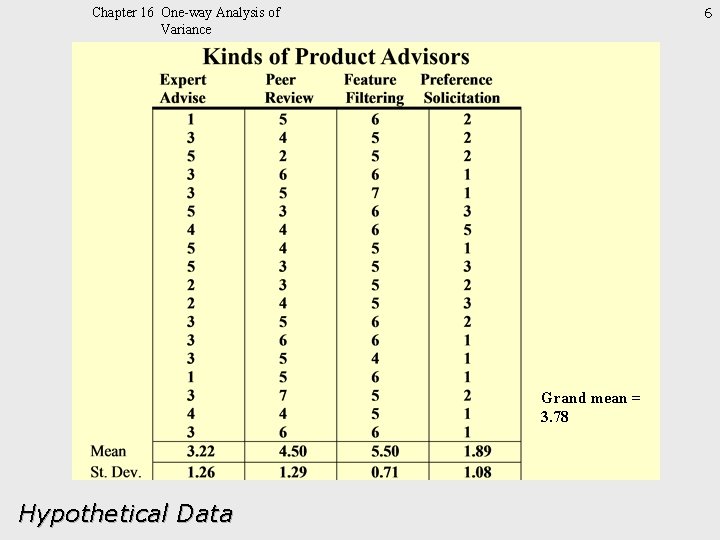
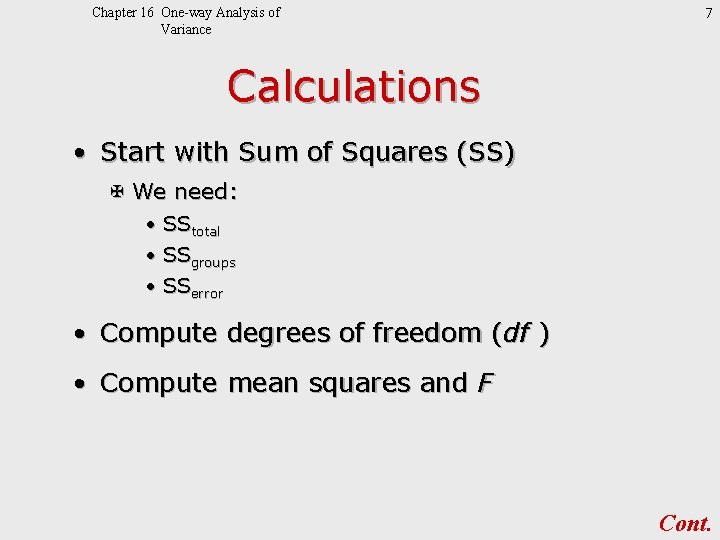
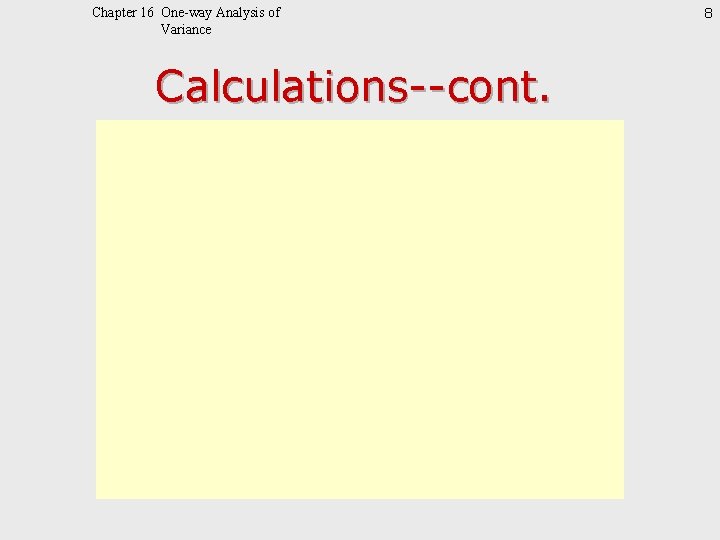
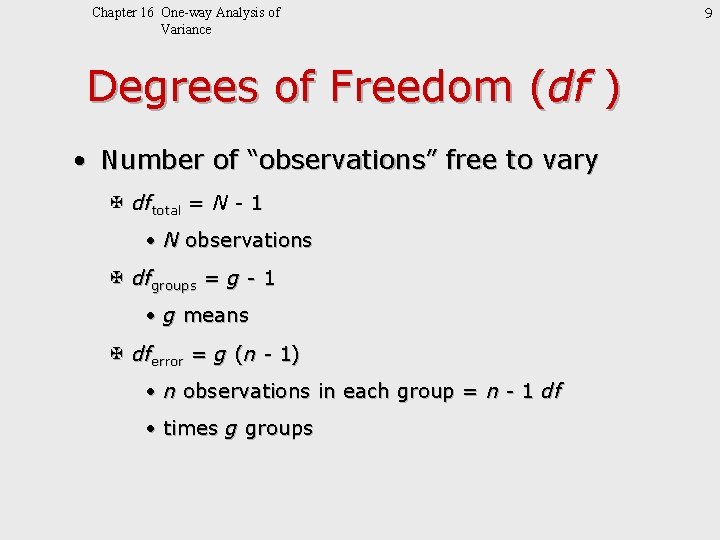
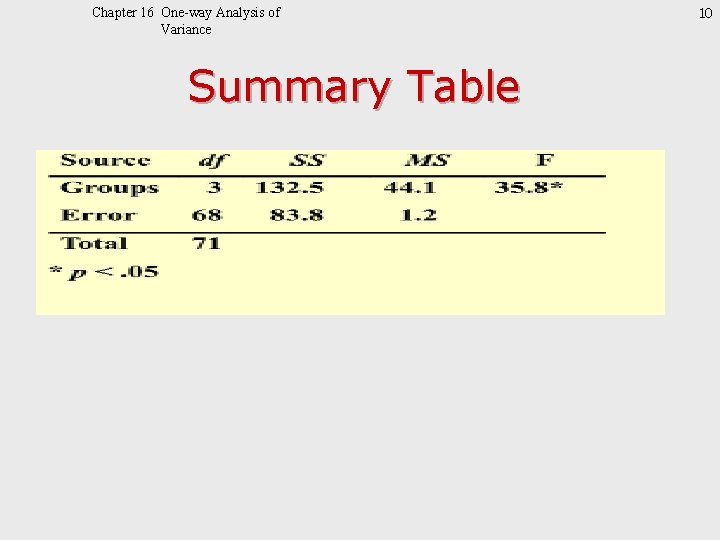
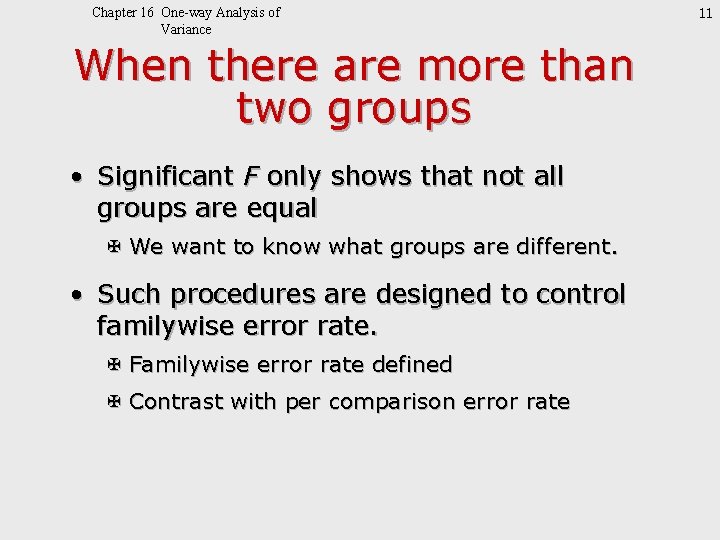
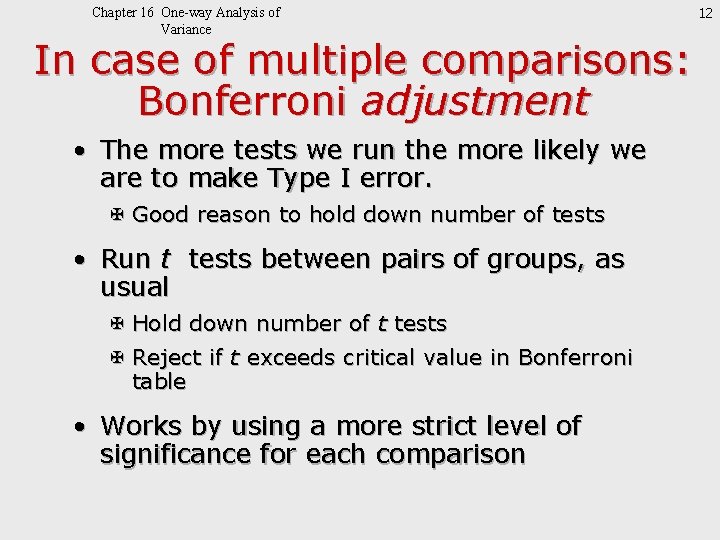
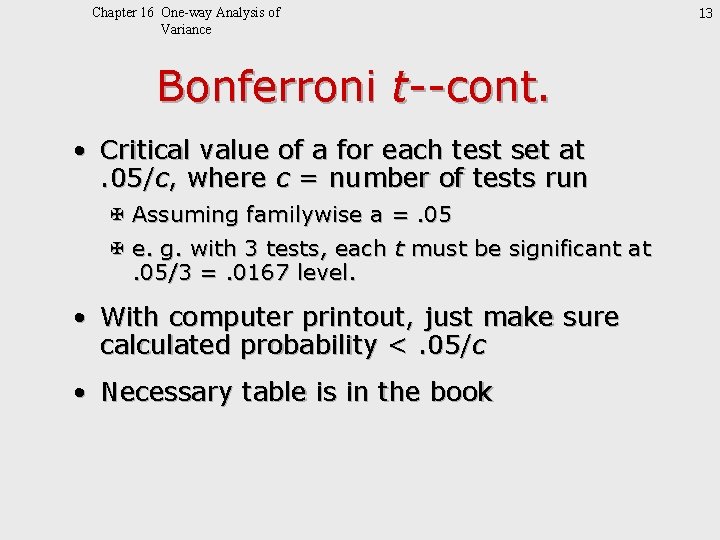
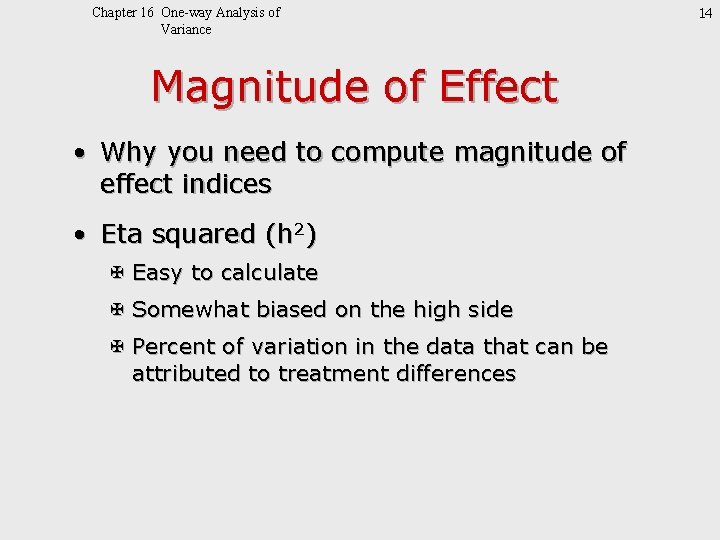
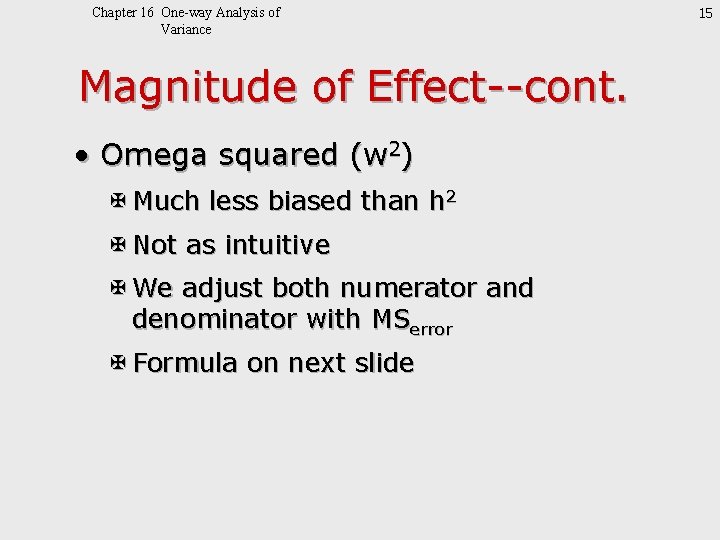
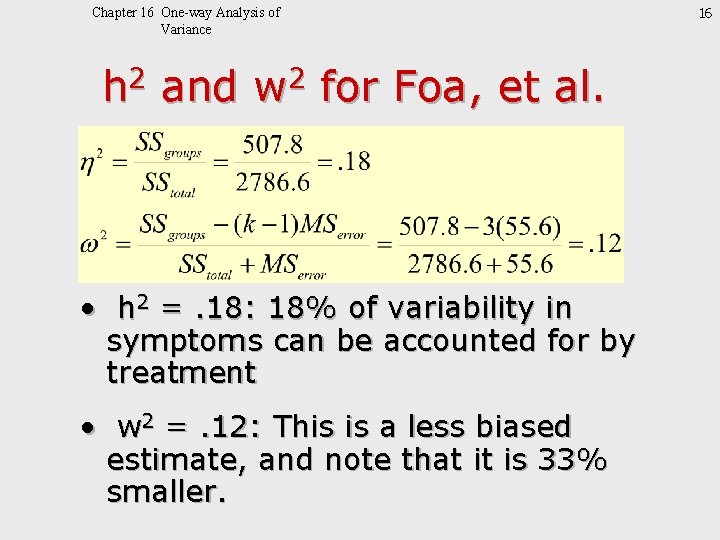
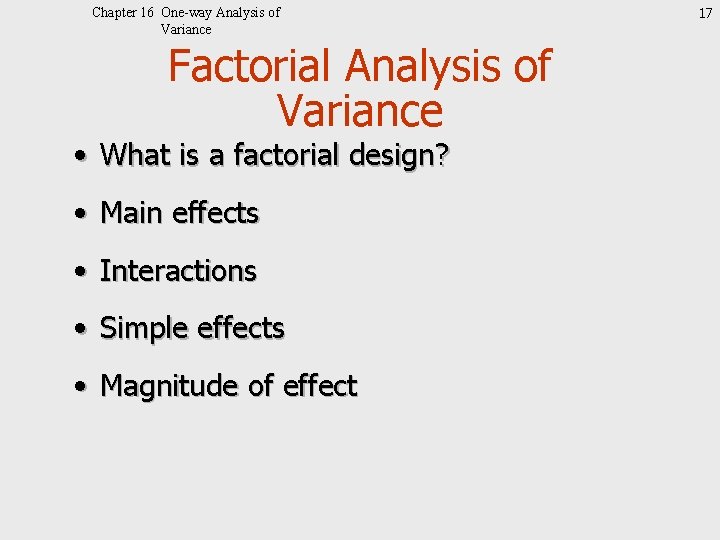
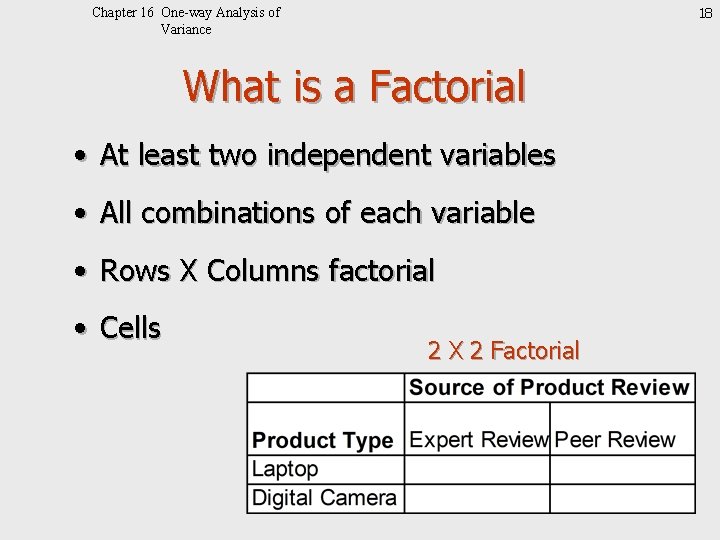
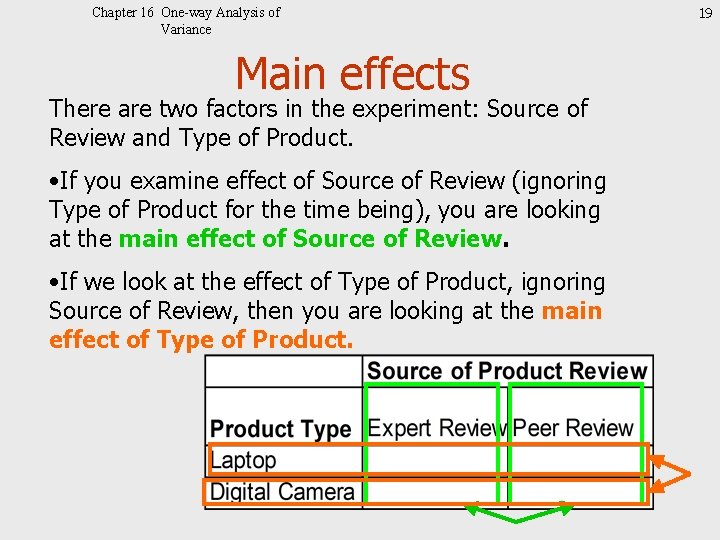
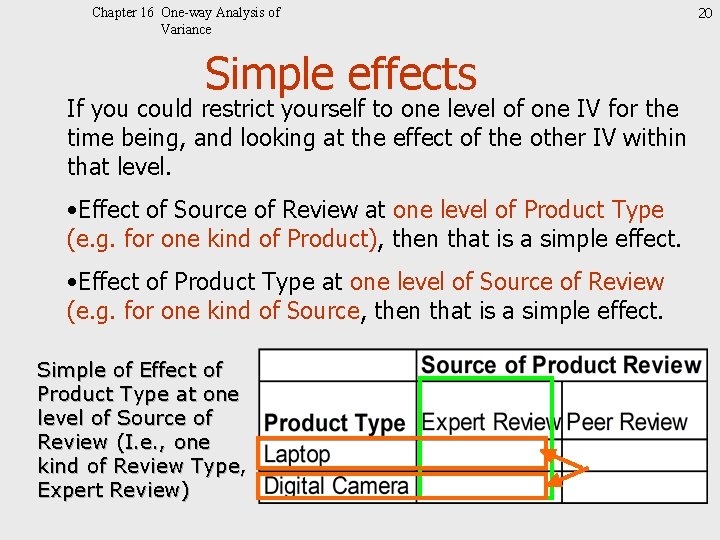
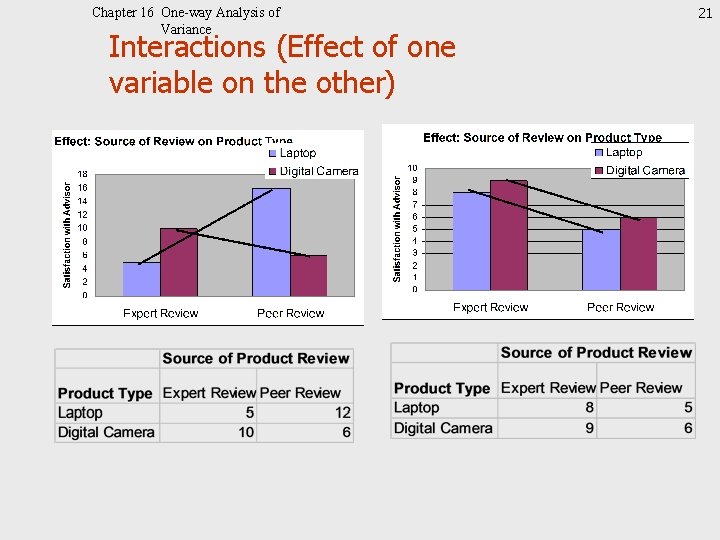
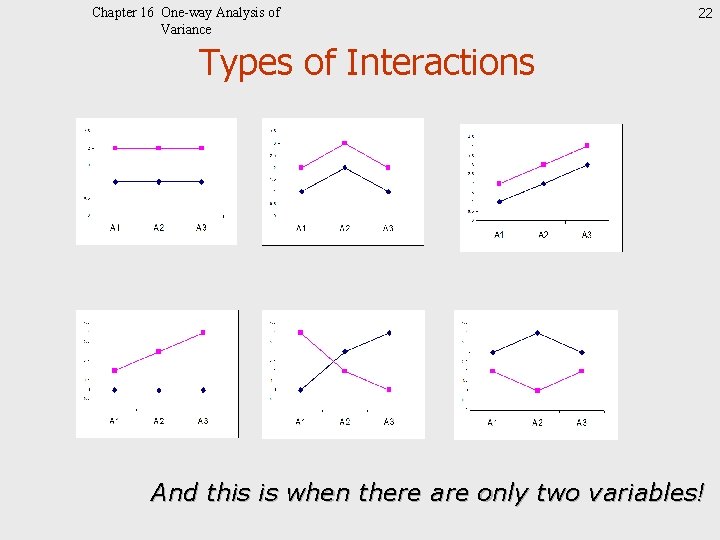
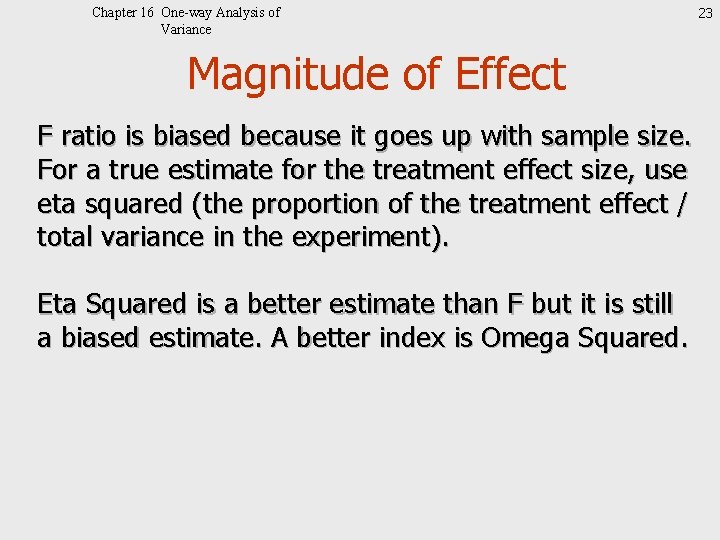
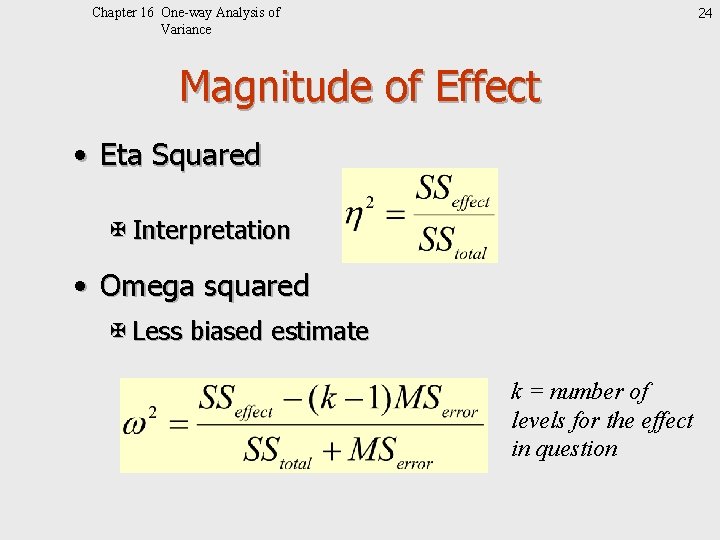
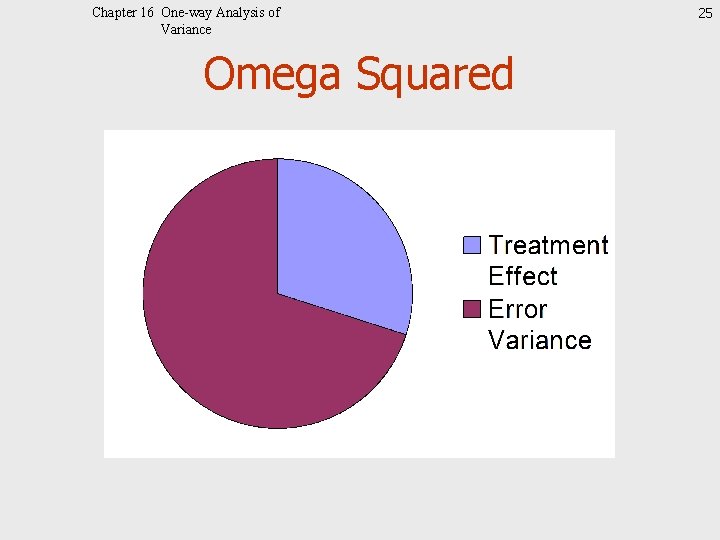
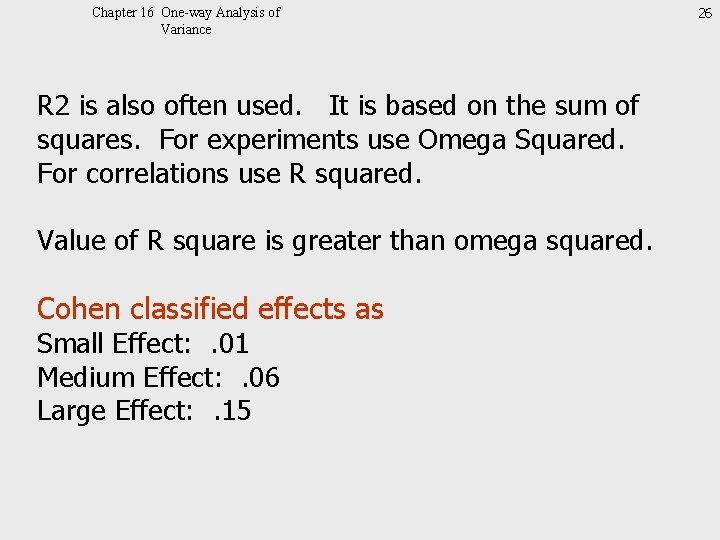
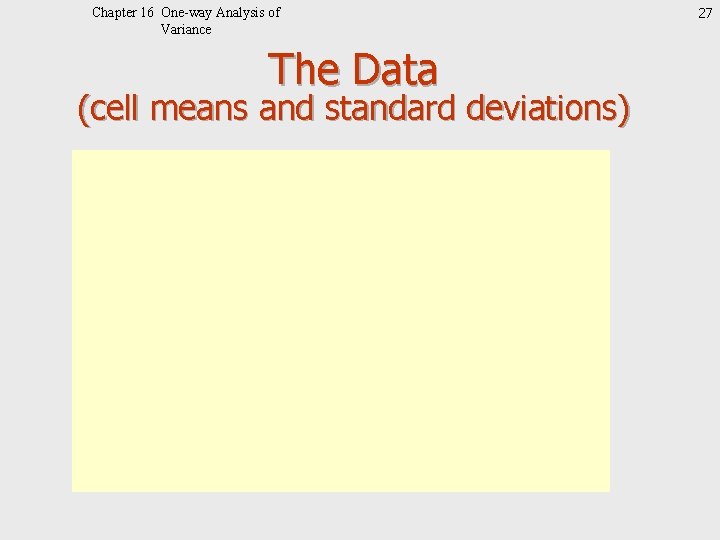
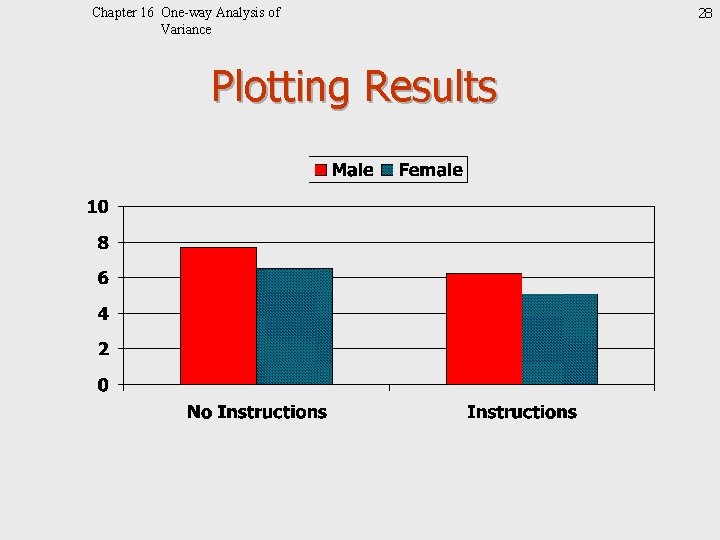
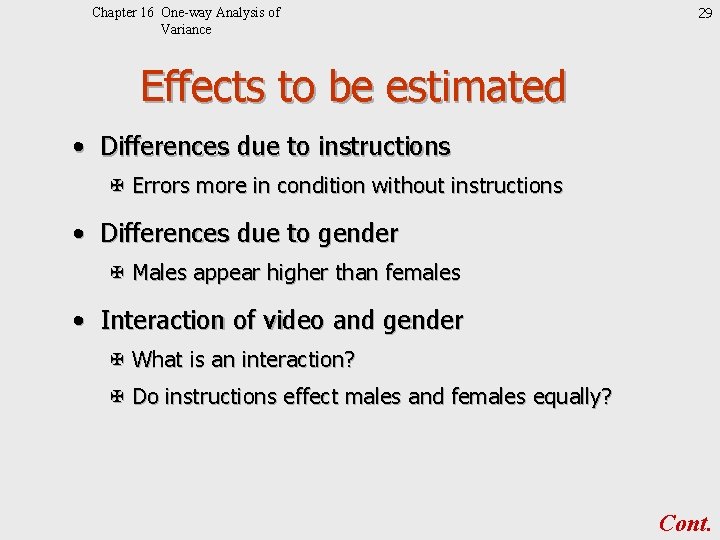
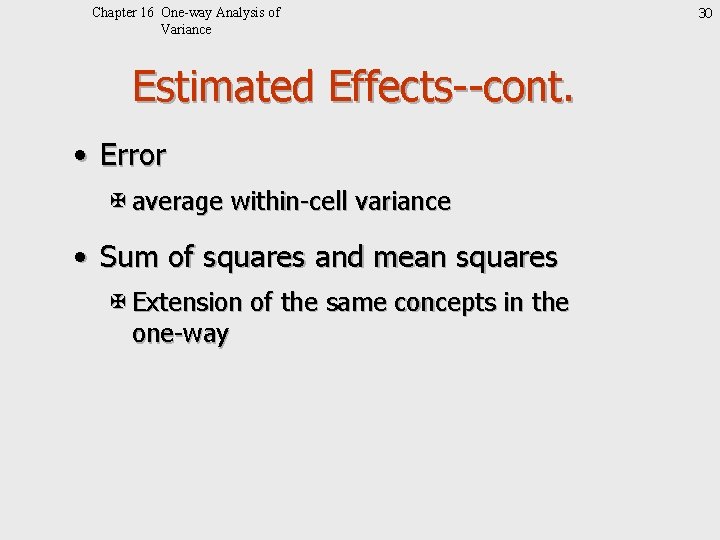
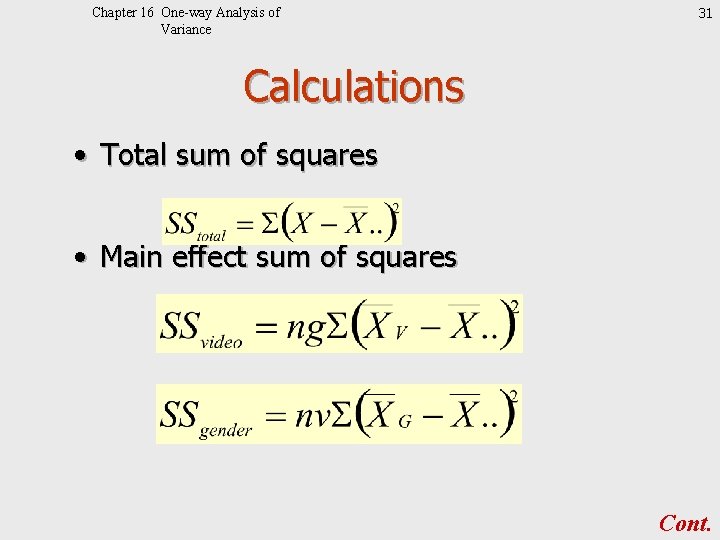
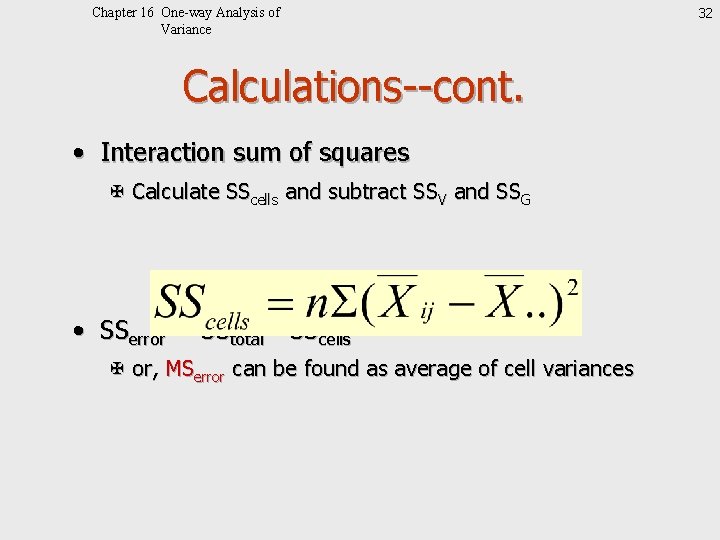
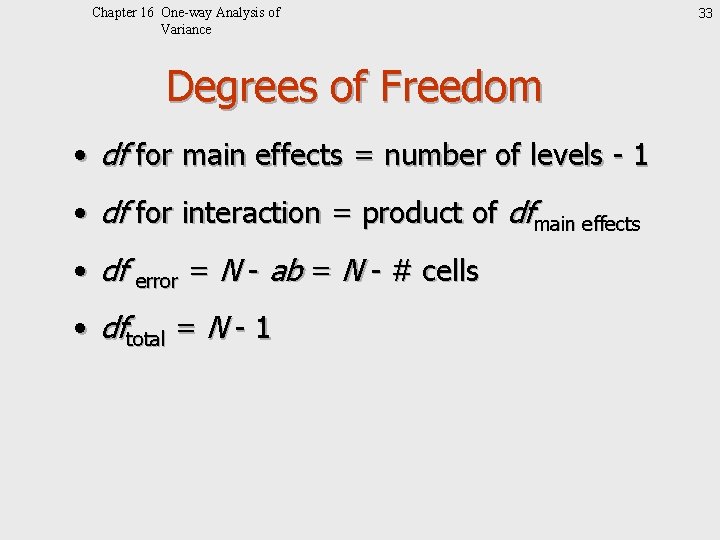
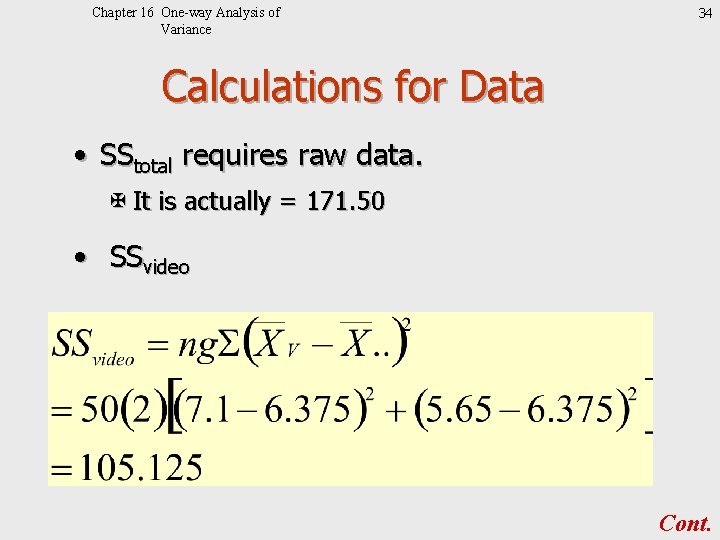
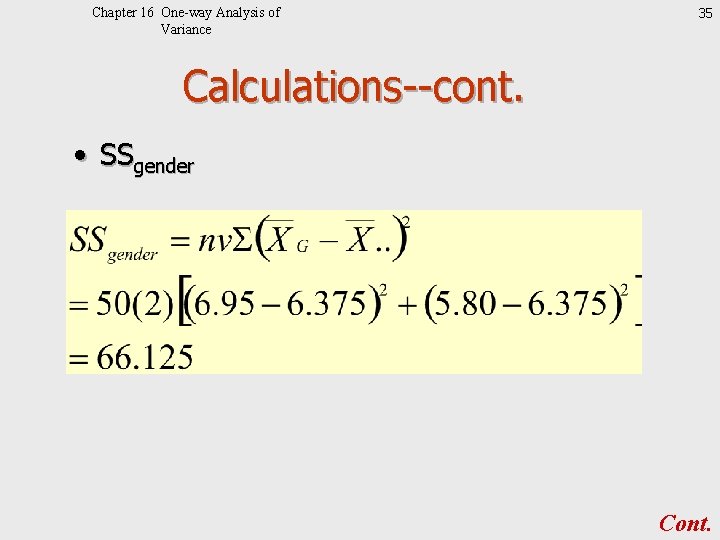
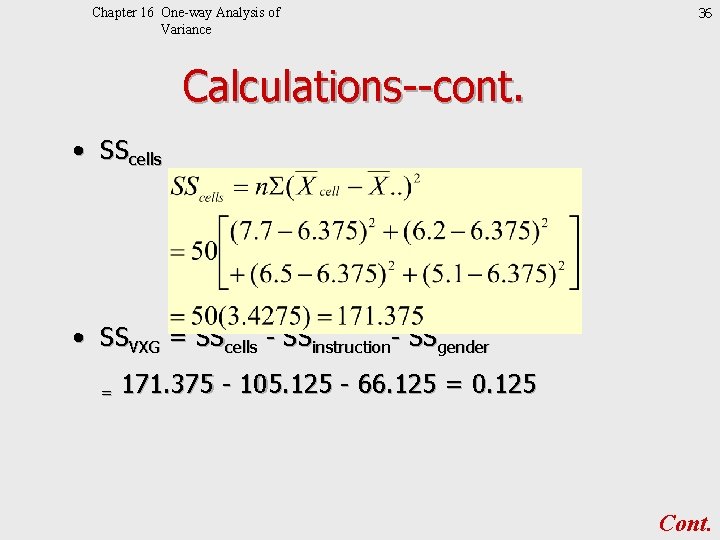
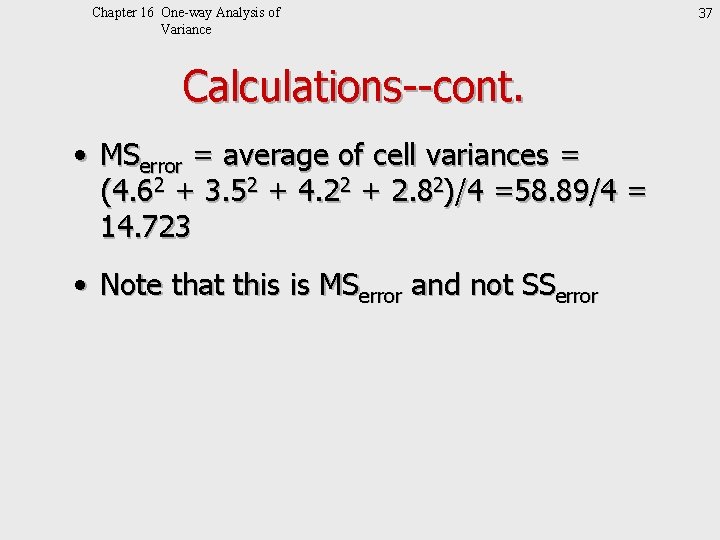
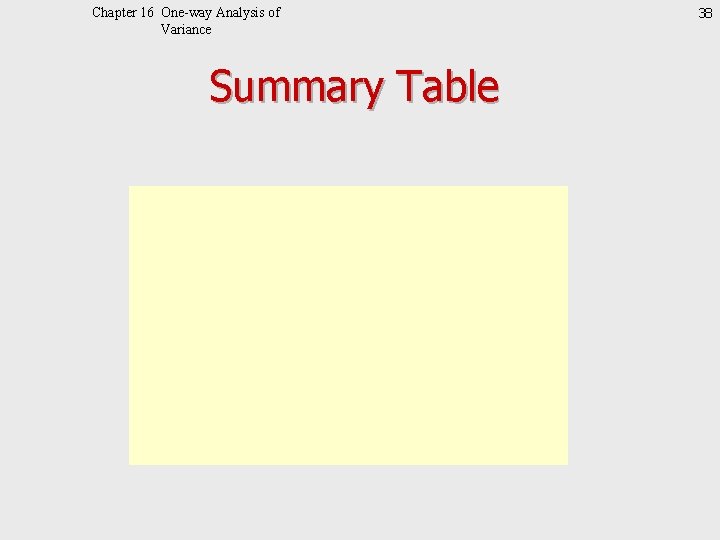
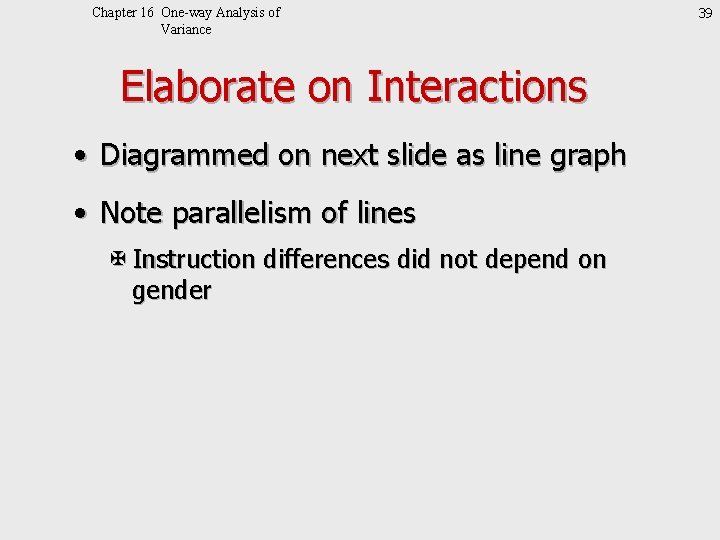
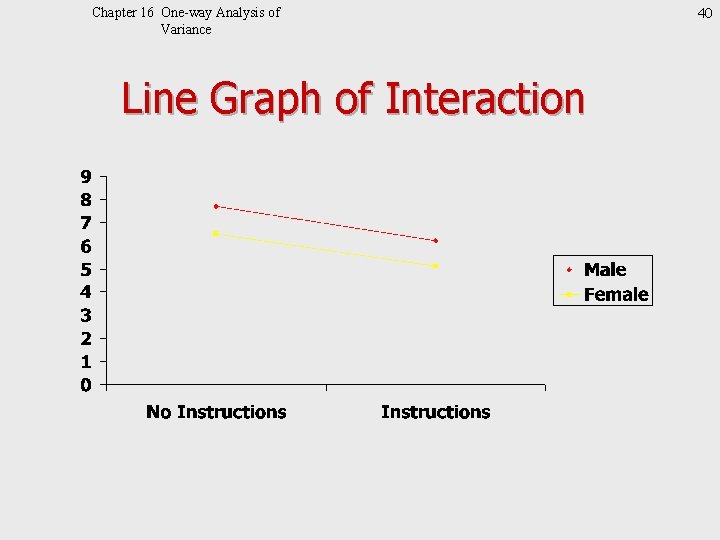
- Slides: 40
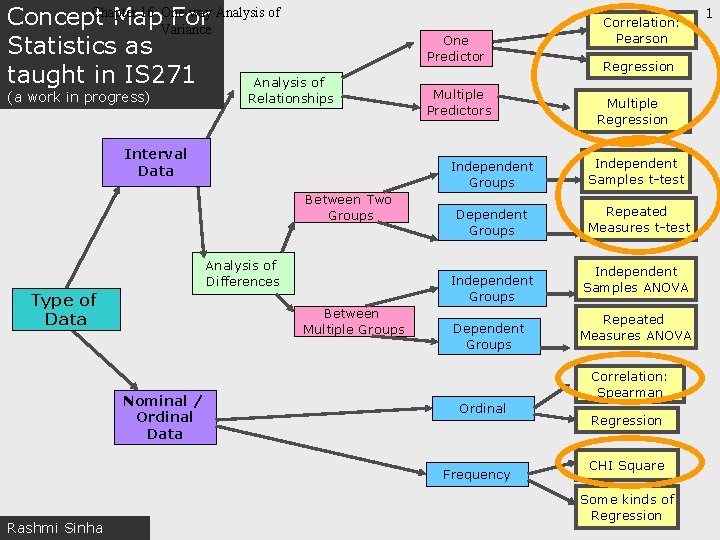
Chapter 16 One-way Analysis of Concept Map. Variance For Statistics as taught in IS 271 Analysis of (a work in progress) Relationships Interval Data Between Two Groups Analysis of Differences Type of Data Multiple Predictors Multiple Regression Independent Samples t-test Dependent Groups Repeated Measures t-test Dependent Groups Independent Samples ANOVA Repeated Measures ANOVA Correlation: Spearman Ordinal Frequency Rashmi Sinha Regression Independent Groups Between Multiple Groups Nominal / Ordinal Data One Predictor Correlation: Pearson Regression CHI Square Some kinds of Regression 1
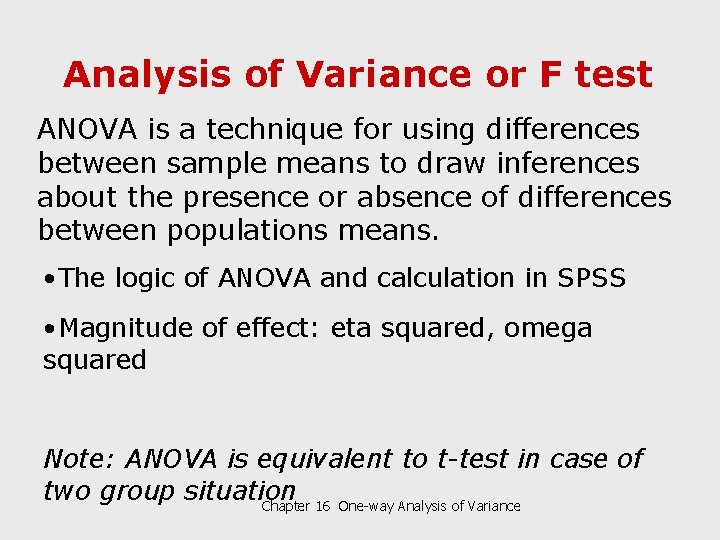
Analysis of Variance or F test ANOVA is a technique for using differences between sample means to draw inferences about the presence or absence of differences between populations means. • The logic of ANOVA and calculation in SPSS • Magnitude of effect: eta squared, omega squared Note: ANOVA is equivalent to t-test in case of two group situation Chapter 16 One-way Analysis of Variance
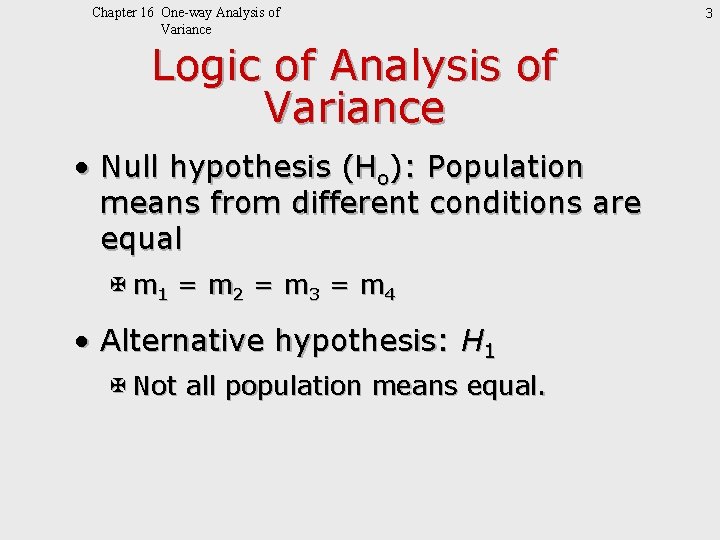
Chapter 16 One-way Analysis of Variance Logic of Analysis of Variance • Null hypothesis (Ho): Population means from different conditions are equal X m 1 = m 2 = m 3 = m 4 • Alternative hypothesis: H 1 X Not all population means equal. 3
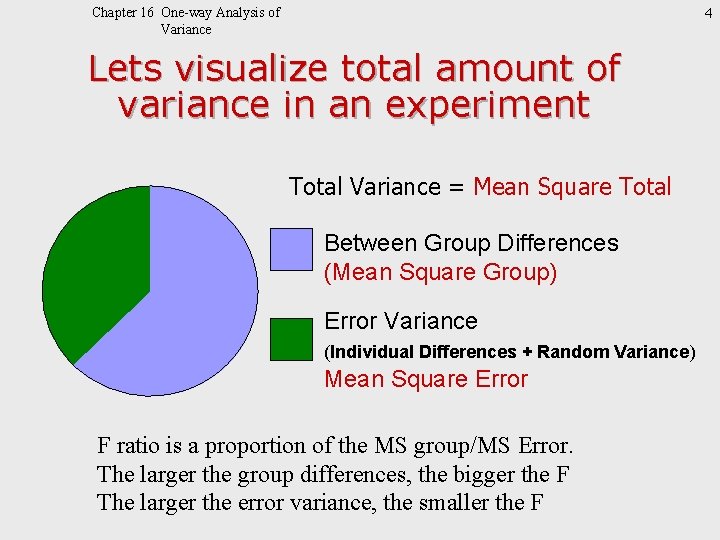
4 Chapter 16 One-way Analysis of Variance Lets visualize total amount of variance in an experiment Total Variance = Mean Square Total Between Group Differences (Mean Square Group) Error Variance (Individual Differences + Random Variance) Mean Square Error F ratio is a proportion of the MS group/MS Error. The larger the group differences, the bigger the F The larger the error variance, the smaller the F
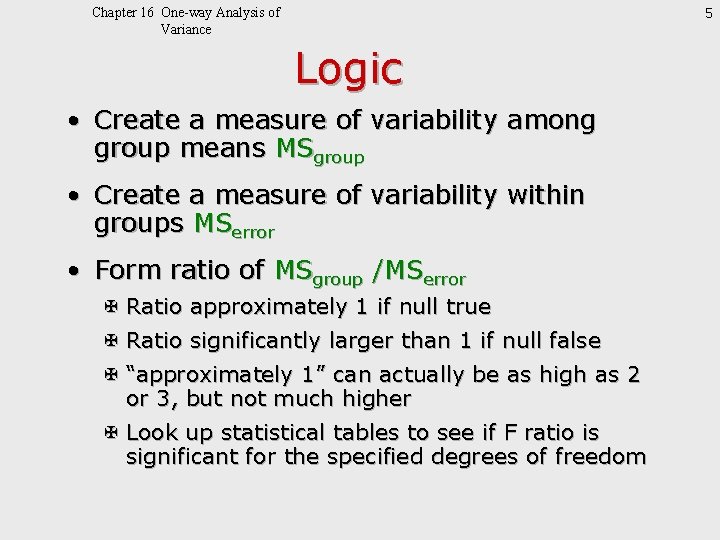
5 Chapter 16 One-way Analysis of Variance Logic • Create a measure of variability among group means MSgroup • Create a measure of variability within groups MSerror • Form ratio of MSgroup /MSerror X Ratio approximately 1 if null true X Ratio significantly larger than 1 if null false X “approximately 1” can actually be as high as 2 or 3, but not much higher X Look up statistical tables to see if F ratio is significant for the specified degrees of freedom
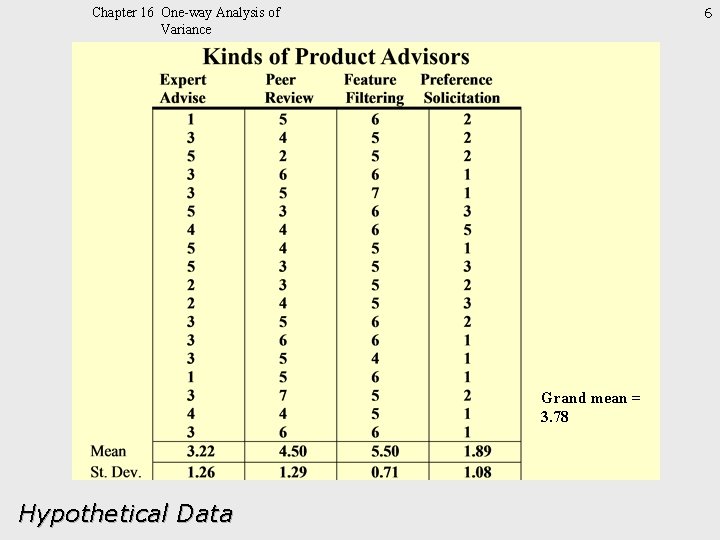
6 Chapter 16 One-way Analysis of Variance Grand mean = 3. 78 Hypothetical Data
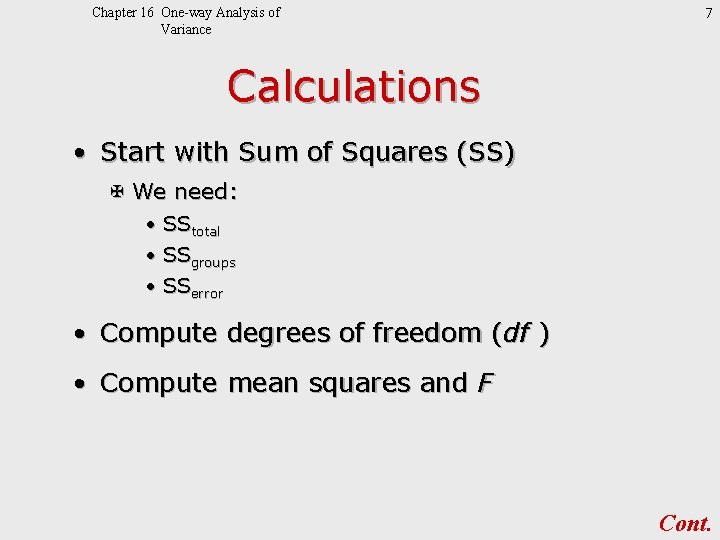
Chapter 16 One-way Analysis of Variance 7 Calculations • Start with Sum of Squares (SS) X We need: • SStotal • SSgroups • SSerror • Compute degrees of freedom (df ) • Compute mean squares and F Cont.
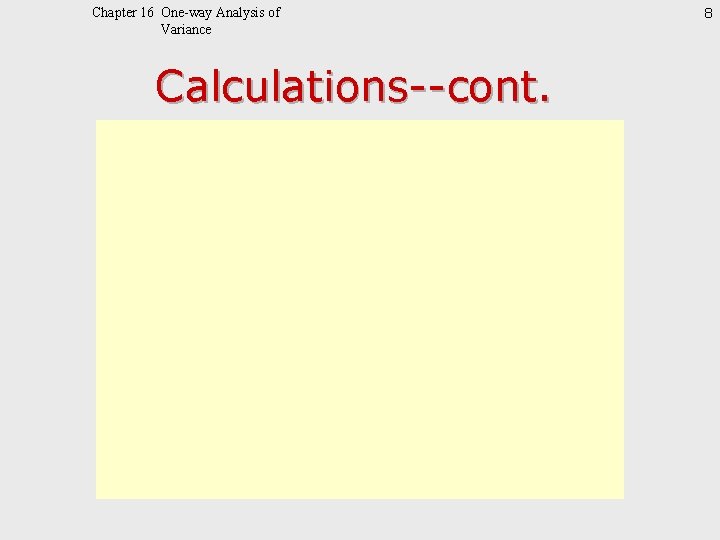
Chapter 16 One-way Analysis of Variance Calculations--cont. 8
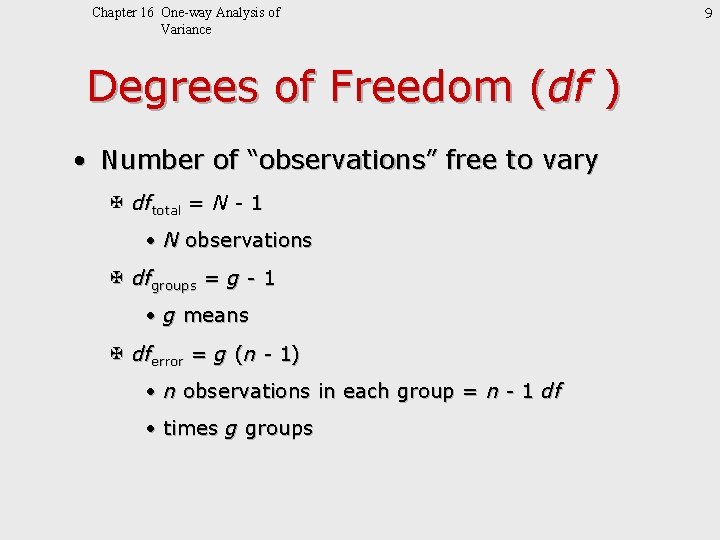
Chapter 16 One-way Analysis of Variance Degrees of Freedom (df ) • Number of “observations” free to vary X dftotal = N - 1 • N observations X dfgroups = g - 1 • g means X dferror = g (n - 1) • n observations in each group = n - 1 df • times g groups 9
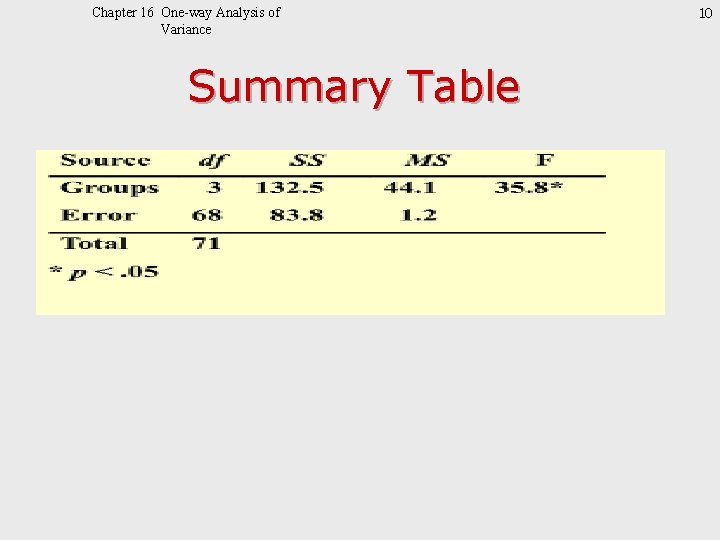
Chapter 16 One-way Analysis of Variance Summary Table 10
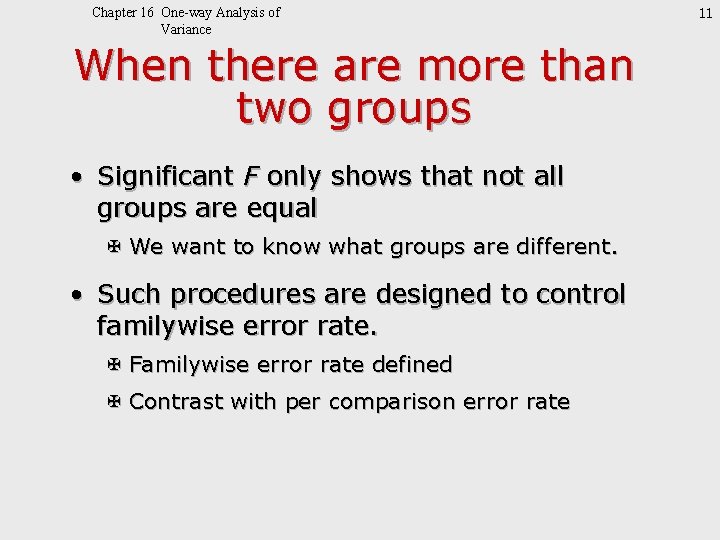
Chapter 16 One-way Analysis of Variance When there are more than two groups • Significant F only shows that not all groups are equal X We want to know what groups are different. • Such procedures are designed to control familywise error rate. X Familywise error rate defined X Contrast with per comparison error rate 11
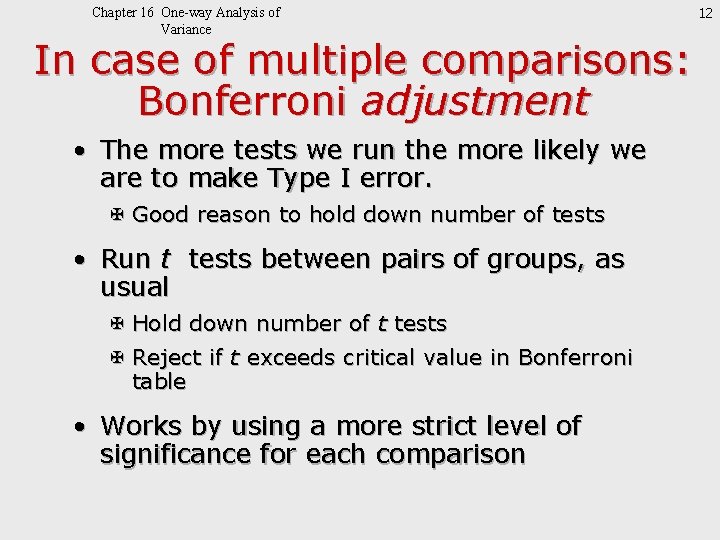
Chapter 16 One-way Analysis of Variance In case of multiple comparisons: Bonferroni adjustment • The more tests we run the more likely we are to make Type I error. X Good reason to hold down number of tests • Run t tests between pairs of groups, as usual X Hold down number of t tests X Reject if t exceeds critical value in Bonferroni table • Works by using a more strict level of significance for each comparison 12
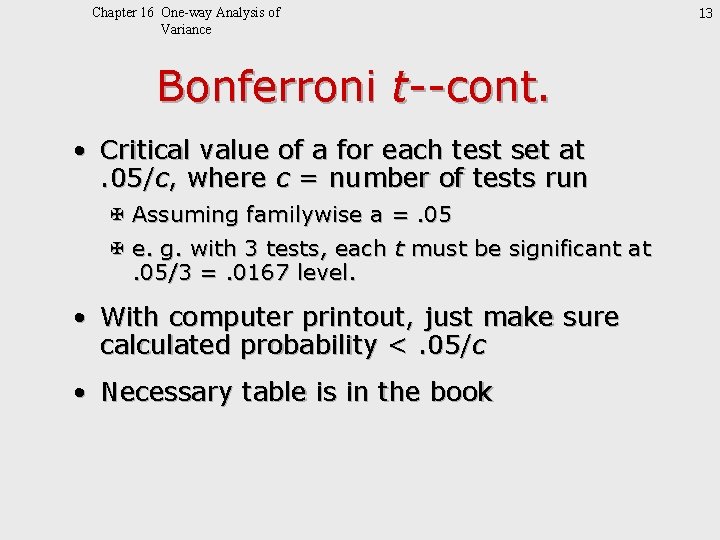
Chapter 16 One-way Analysis of Variance Bonferroni t--cont. • Critical value of a for each test set at. 05/c, where c = number of tests run X Assuming familywise a =. 05 X e. g. with 3 tests, each t must be significant at. 05/3 =. 0167 level. • With computer printout, just make sure calculated probability <. 05/c • Necessary table is in the book 13
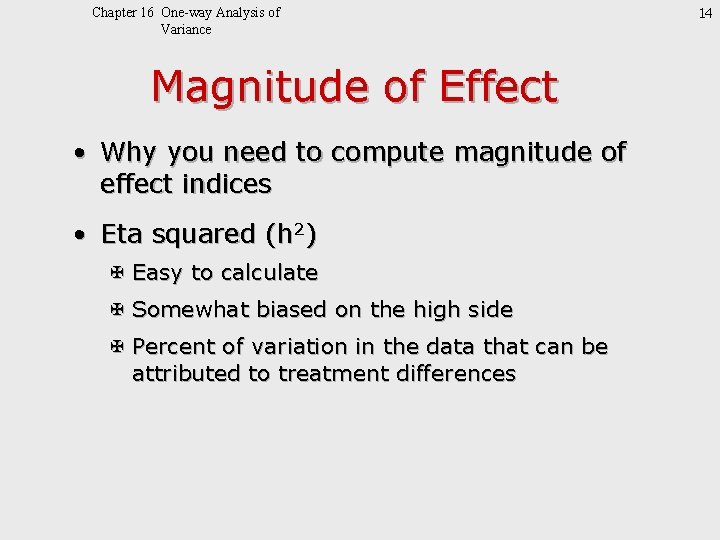
Chapter 16 One-way Analysis of Variance Magnitude of Effect • Why you need to compute magnitude of effect indices • Eta squared (h 2) X Easy to calculate X Somewhat biased on the high side X Percent of variation in the data that can be attributed to treatment differences 14
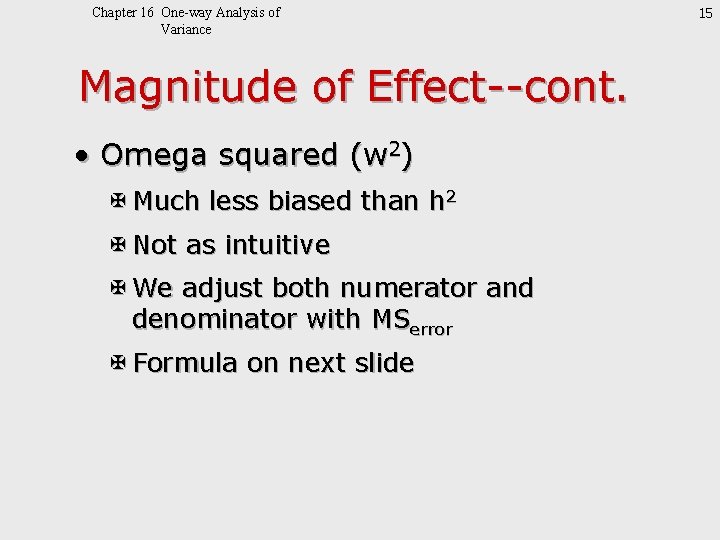
Chapter 16 One-way Analysis of Variance Magnitude of Effect--cont. • Omega squared (w 2) X Much less biased than h 2 X Not as intuitive X We adjust both numerator and denominator with MSerror X Formula on next slide 15
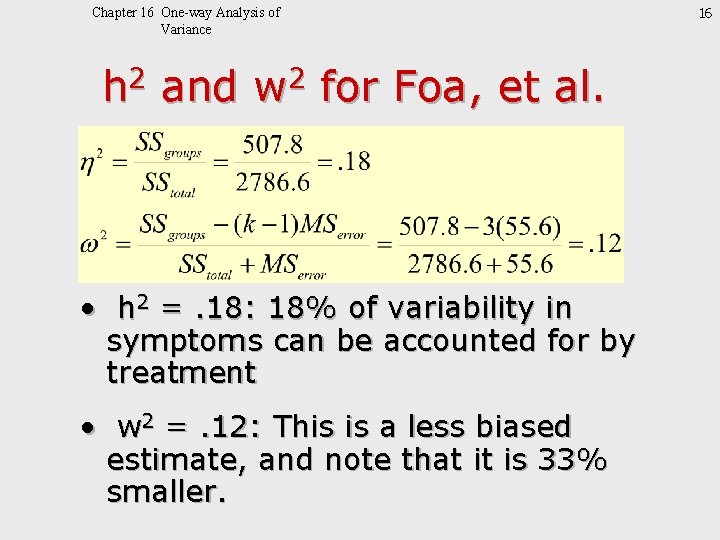
Chapter 16 One-way Analysis of Variance h 2 and w 2 for Foa, et al. • h 2 =. 18: 18% of variability in symptoms can be accounted for by treatment • w 2 =. 12: This is a less biased estimate, and note that it is 33% smaller. 16
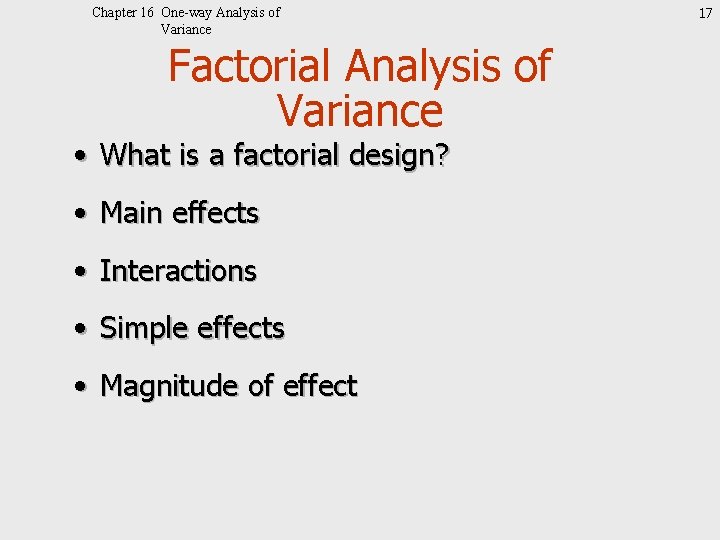
Chapter 16 One-way Analysis of Variance Factorial Analysis of Variance • What is a factorial design? • Main effects • Interactions • Simple effects • Magnitude of effect 17
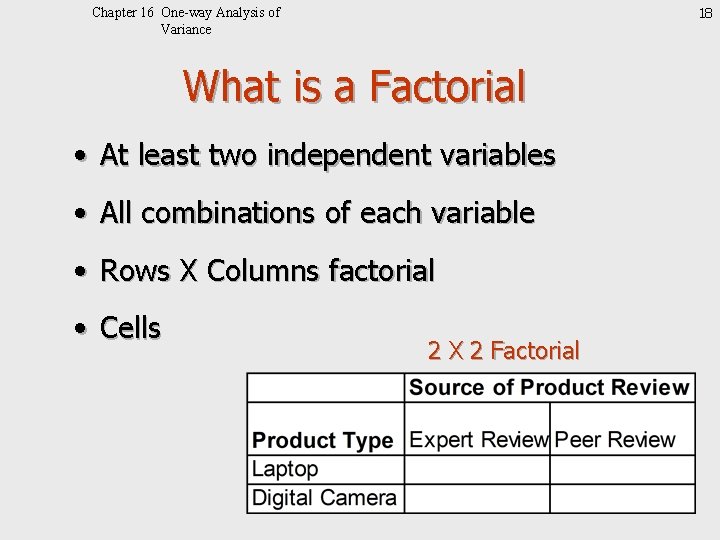
18 Chapter 16 One-way Analysis of Variance What is a Factorial • At least two independent variables • All combinations of each variable • Rows X Columns factorial • Cells 2 X 2 Factorial
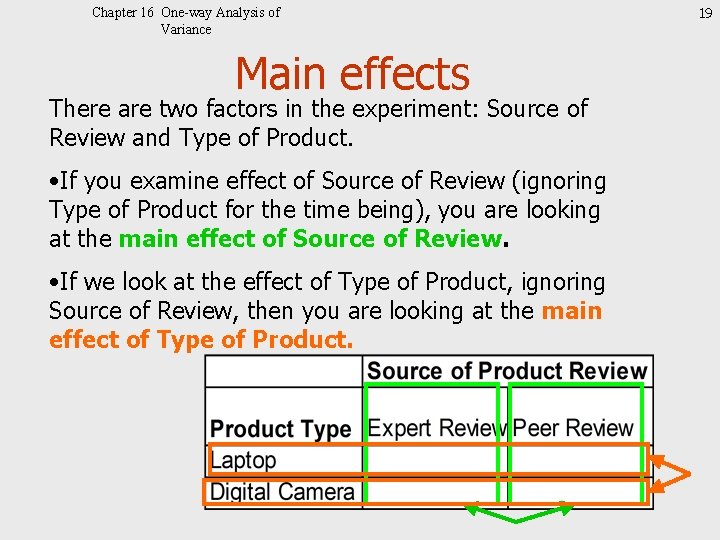
Chapter 16 One-way Analysis of Variance Main effects There are two factors in the experiment: Source of Review and Type of Product. • If you examine effect of Source of Review (ignoring Type of Product for the time being), you are looking at the main effect of Source of Review. • If we look at the effect of Type of Product, ignoring Source of Review, then you are looking at the main effect of Type of Product. 19
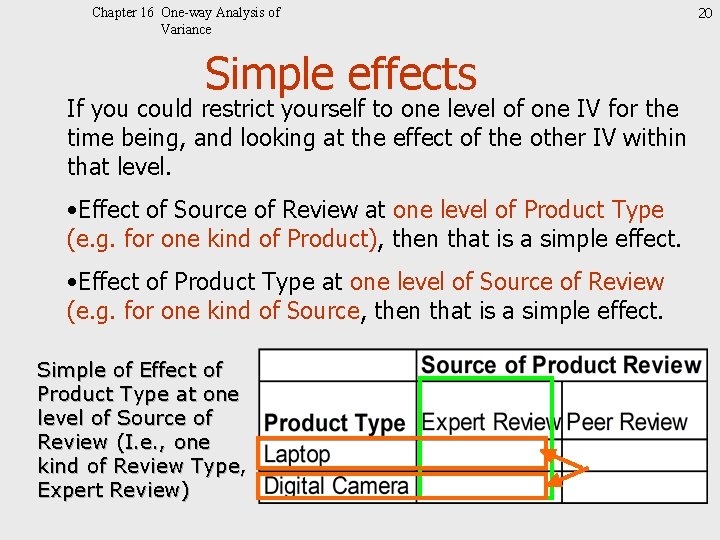
Chapter 16 One-way Analysis of Variance Simple effects If you could restrict yourself to one level of one IV for the time being, and looking at the effect of the other IV within that level. • Effect of Source of Review at one level of Product Type (e. g. for one kind of Product), then that is a simple effect. • Effect of Product Type at one level of Source of Review (e. g. for one kind of Source, then that is a simple effect. Simple of Effect of Product Type at one level of Source of Review (I. e. , one kind of Review Type, Expert Review) 20
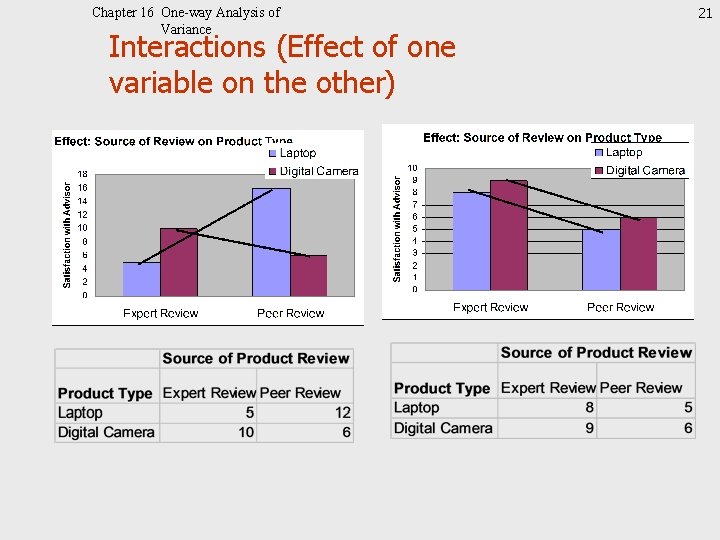
Chapter 16 One-way Analysis of Variance Interactions (Effect of one variable on the other) 21
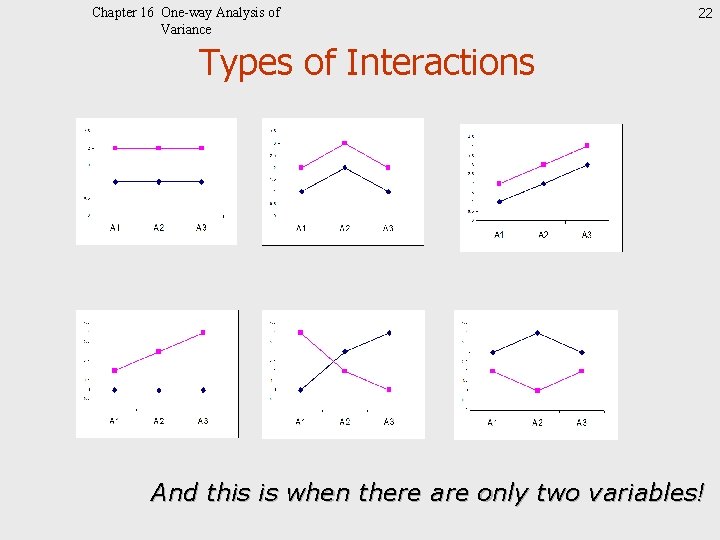
Chapter 16 One-way Analysis of Variance 22 Types of Interactions And this is when there are only two variables!
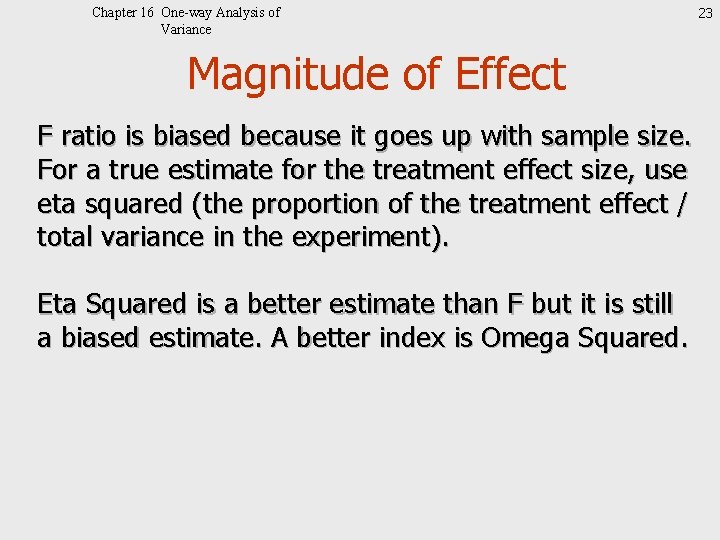
Chapter 16 One-way Analysis of Variance Magnitude of Effect F ratio is biased because it goes up with sample size. For a true estimate for the treatment effect size, use eta squared (the proportion of the treatment effect / total variance in the experiment). Eta Squared is a better estimate than F but it is still a biased estimate. A better index is Omega Squared. 23
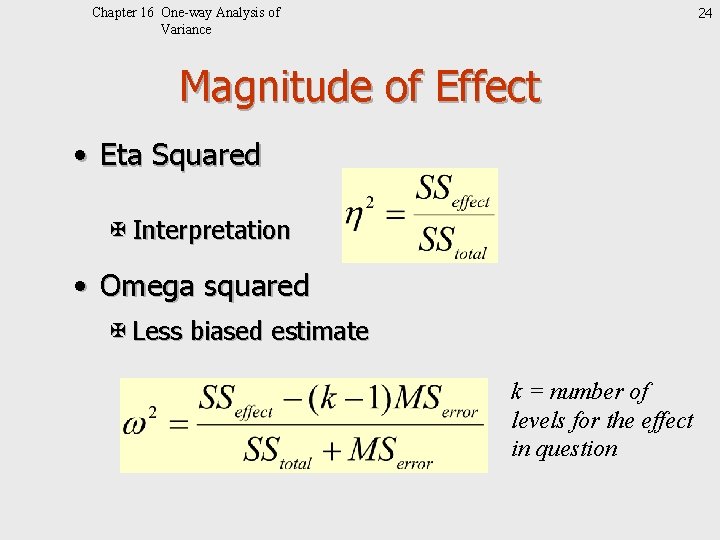
24 Chapter 16 One-way Analysis of Variance Magnitude of Effect • Eta Squared X Interpretation • Omega squared X Less biased estimate k = number of levels for the effect in question
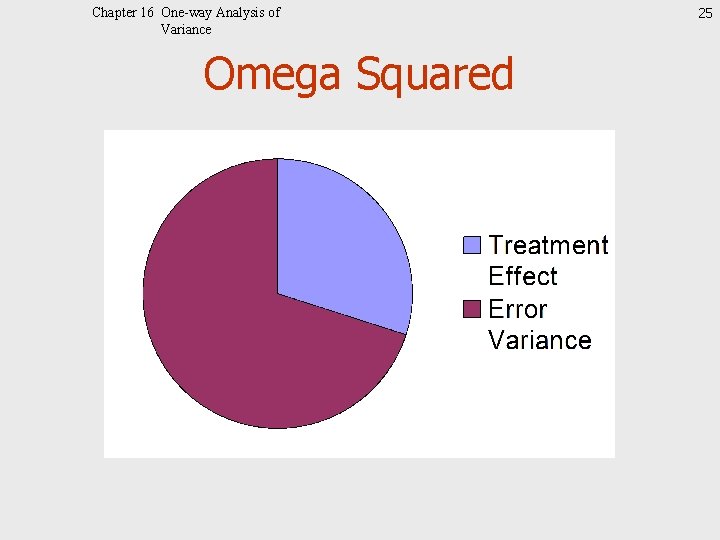
Chapter 16 One-way Analysis of Variance Omega Squared 25
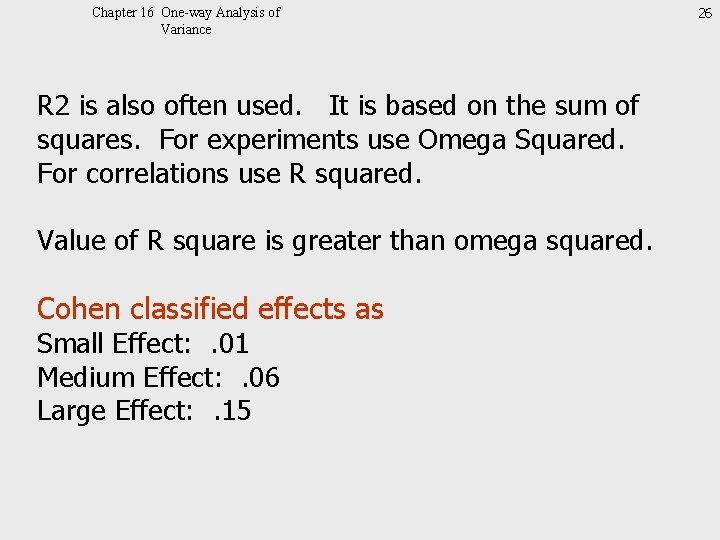
Chapter 16 One-way Analysis of Variance R 2 is also often used. It is based on the sum of squares. For experiments use Omega Squared. For correlations use R squared. Value of R square is greater than omega squared. Cohen classified effects as Small Effect: . 01 Medium Effect: . 06 Large Effect: . 15 26
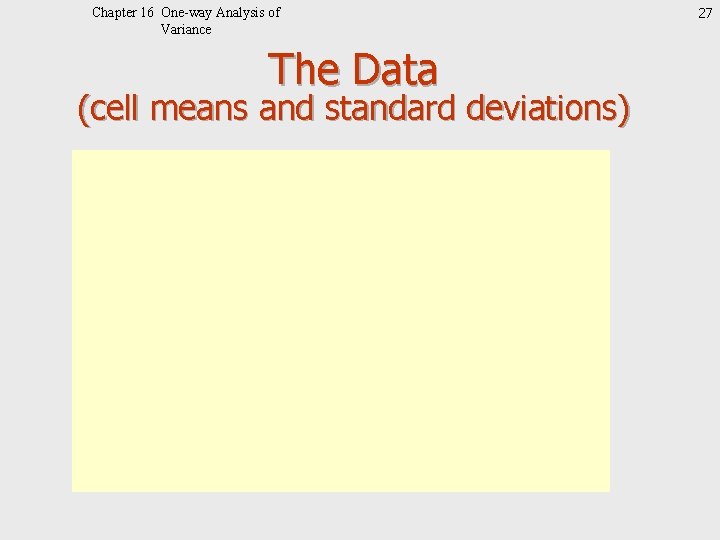
Chapter 16 One-way Analysis of Variance The Data (cell means and standard deviations) 27
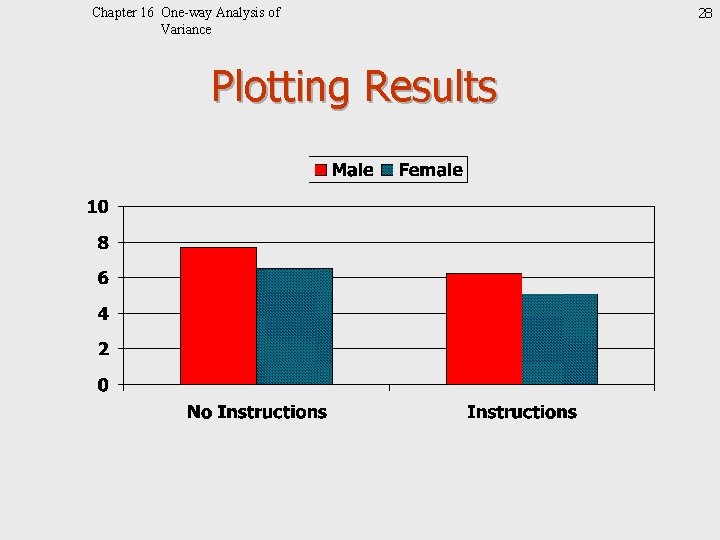
Chapter 16 One-way Analysis of Variance Plotting Results 28
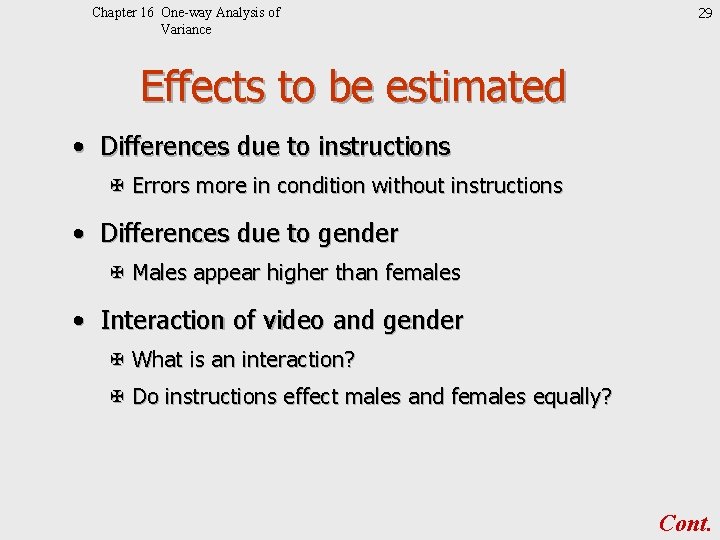
Chapter 16 One-way Analysis of Variance 29 Effects to be estimated • Differences due to instructions X Errors more in condition without instructions • Differences due to gender X Males appear higher than females • Interaction of video and gender X What is an interaction? X Do instructions effect males and females equally? Cont.
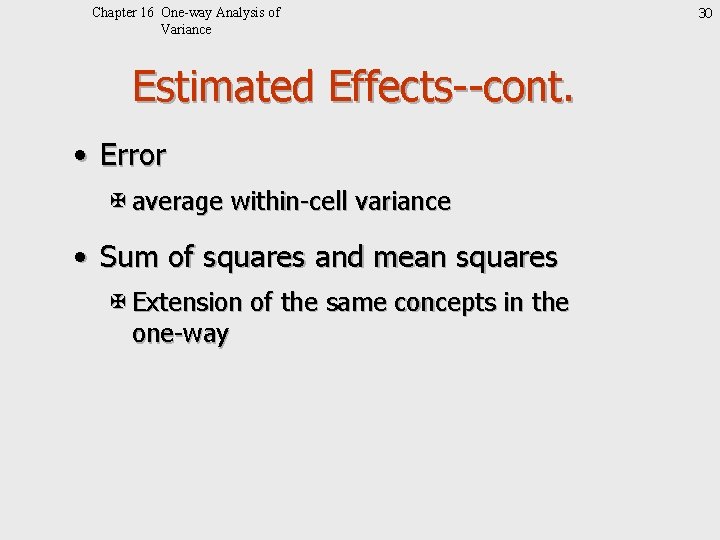
Chapter 16 One-way Analysis of Variance Estimated Effects--cont. • Error X average within-cell variance • Sum of squares and mean squares X Extension of the same concepts in the one-way 30
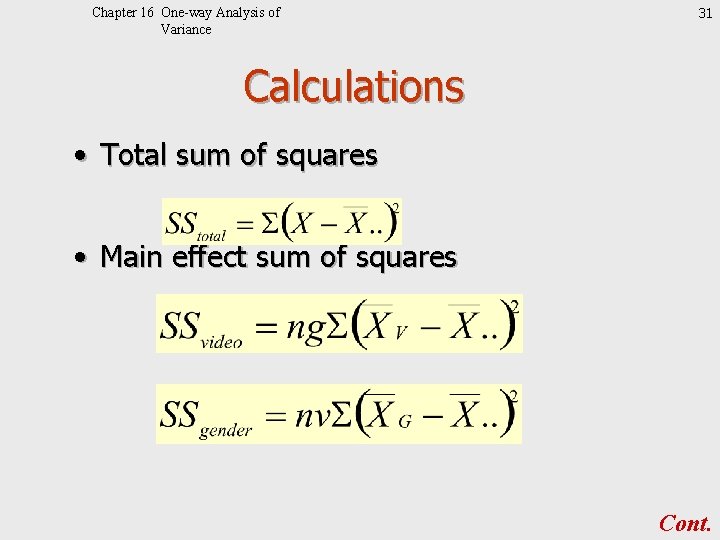
Chapter 16 One-way Analysis of Variance 31 Calculations • Total sum of squares • Main effect sum of squares Cont.
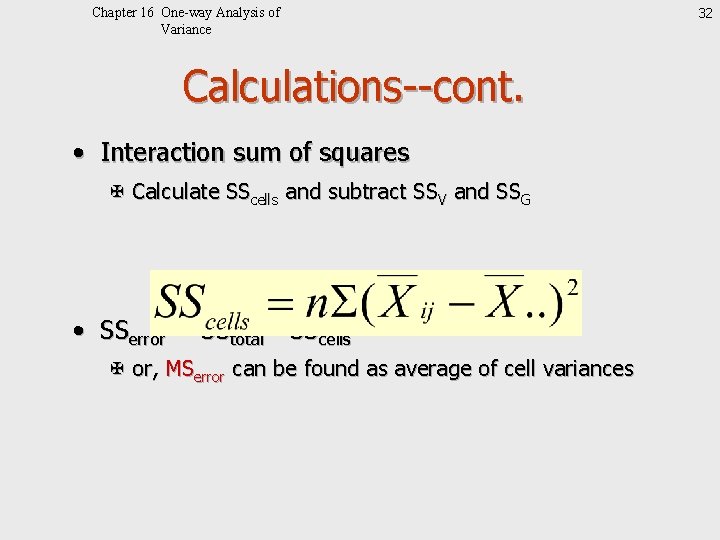
Chapter 16 One-way Analysis of Variance Calculations--cont. • Interaction sum of squares X Calculate SScells and subtract SSV and SSG • SSerror = SStotal - SScells X or, MSerror can be found as average of cell variances 32
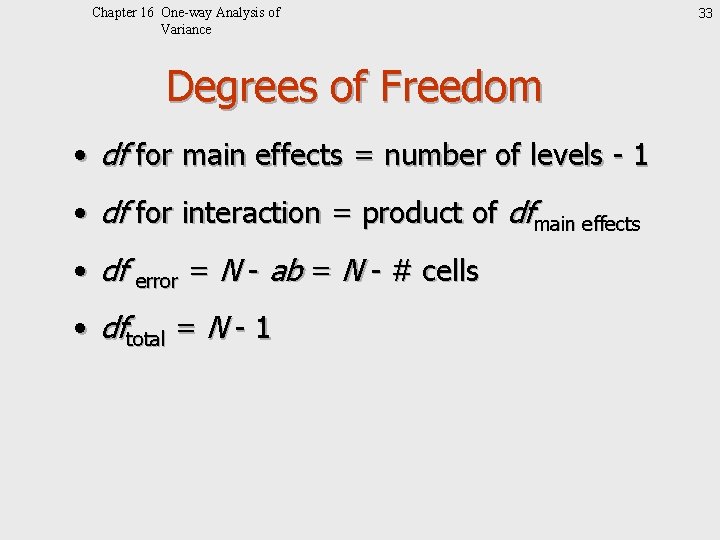
Chapter 16 One-way Analysis of Variance Degrees of Freedom • df for main effects = number of levels - 1 • df for interaction = product of dfmain effects • df error = N - ab = N - # cells • dftotal = N - 1 33
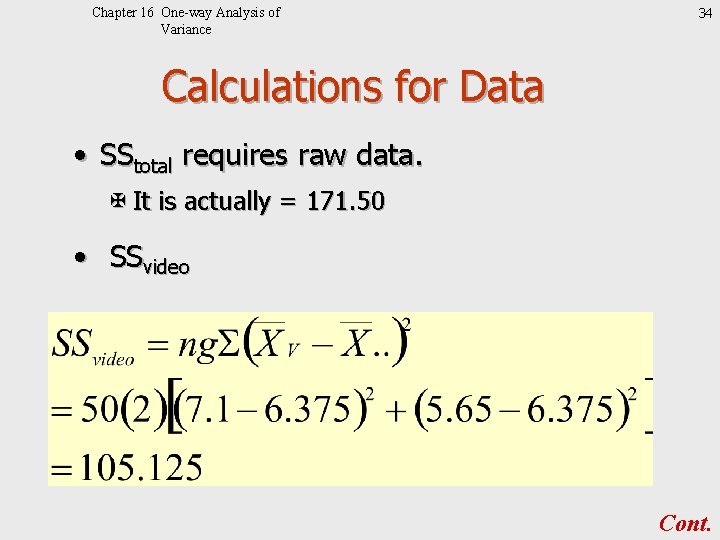
Chapter 16 One-way Analysis of Variance 34 Calculations for Data • SStotal requires raw data. X It is actually = 171. 50 • SSvideo Cont.
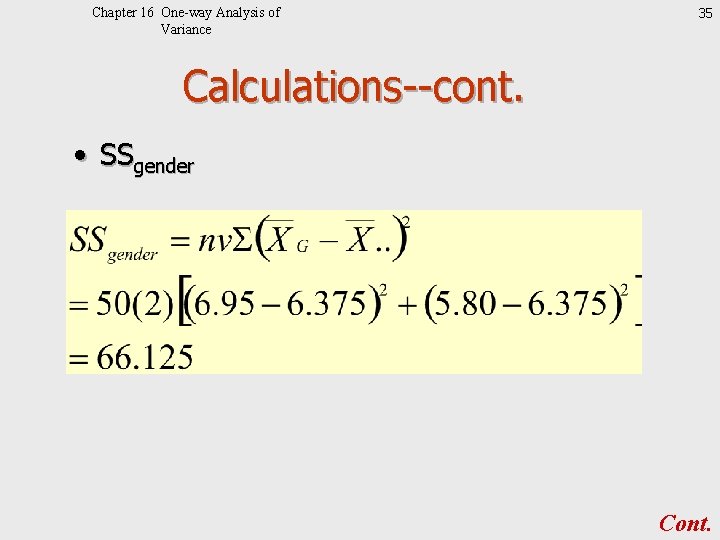
Chapter 16 One-way Analysis of Variance 35 Calculations--cont. • SSgender Cont.
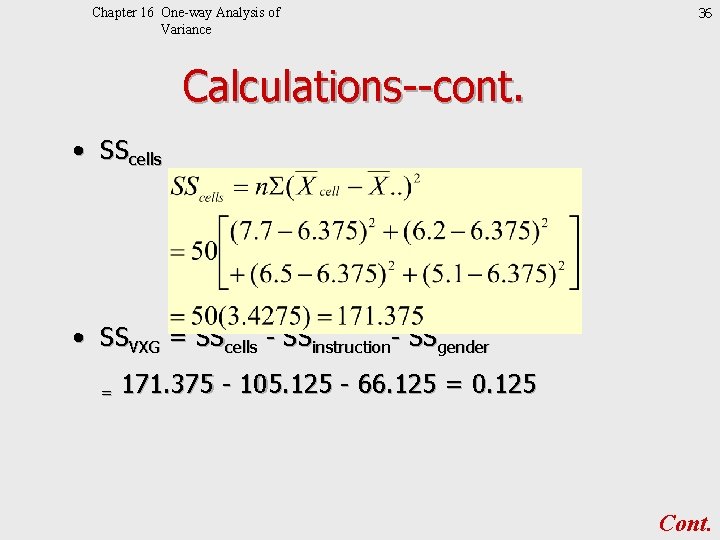
Chapter 16 One-way Analysis of Variance 36 Calculations--cont. • SScells • SSVXG = SScells - SSinstruction- SSgender = 171. 375 - 105. 125 - 66. 125 = 0. 125 Cont.
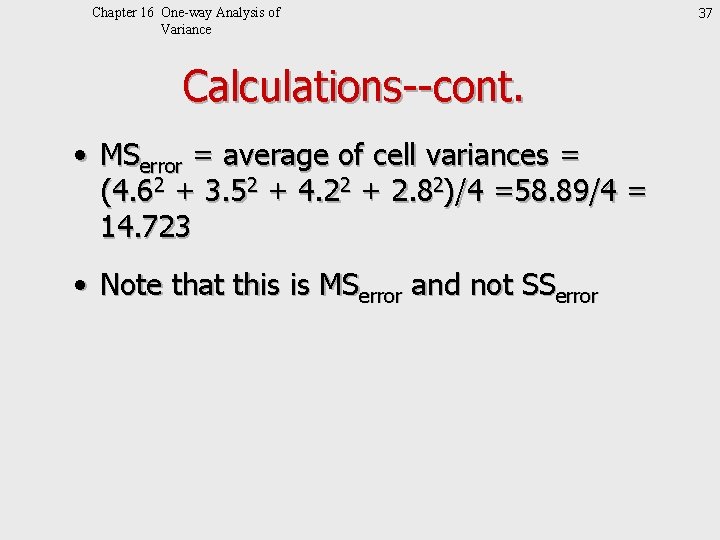
Chapter 16 One-way Analysis of Variance Calculations--cont. • MSerror = average of cell variances = (4. 62 + 3. 52 + 4. 22 + 2. 82)/4 =58. 89/4 = 14. 723 • Note that this is MSerror and not SSerror 37
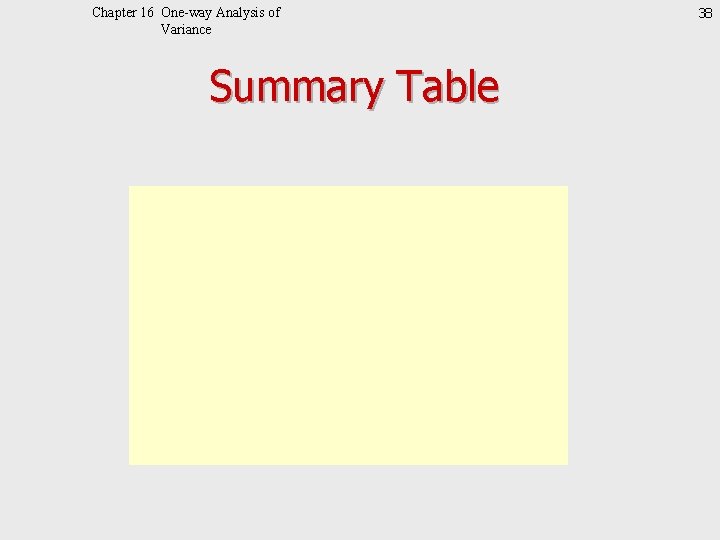
Chapter 16 One-way Analysis of Variance Summary Table 38
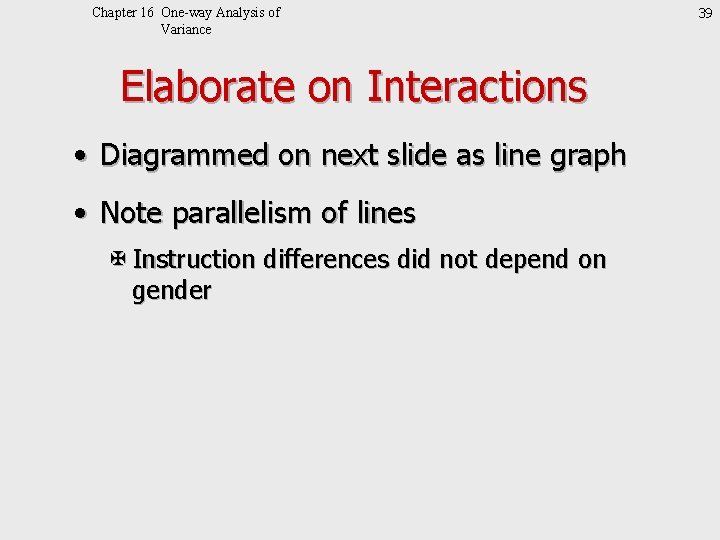
Chapter 16 One-way Analysis of Variance Elaborate on Interactions • Diagrammed on next slide as line graph • Note parallelism of lines X Instruction differences did not depend on gender 39
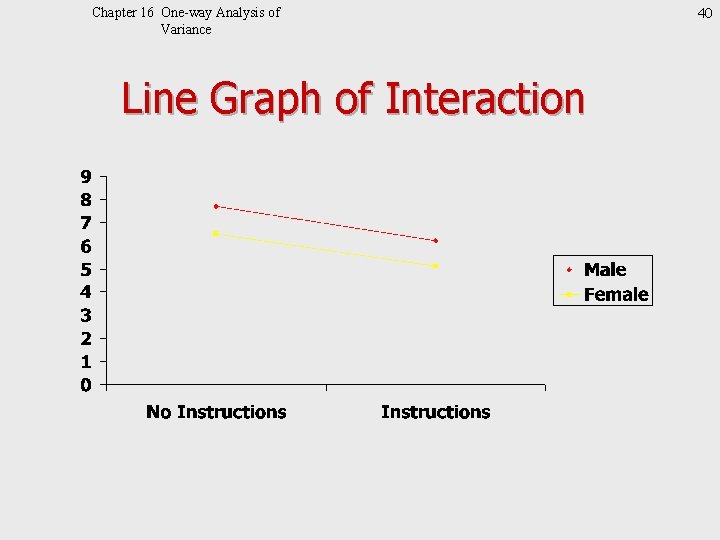
Chapter 16 One-way Analysis of Variance Line Graph of Interaction 40
Stata oneway
Owamp
Oneway hash
Time variance
Variance map
Static budget example
Variance analysis cycle
Budget variance formula
Kaizen costing formula
Multivariate analysis of variance
Direct materials variance
Multivariate analysis of variance and covariance
The formula for usage variance is (aq - sq) * sp.
Variance analysis definition
Spss mixed model
Variance analysis in nursing
Flexible budget variance example
Introduction to analysis of variance
Analysis of variance and covariance
Kaizen costing vs standard costing
Job cost analysis
Manufacturing cost variance
Variance accounting meaning
Mancova
Real self vs ideal self essay
Perbedaan pemasaran dan penjualan
Thermoisoplethendiagramm tropen
Properties of water concept map
Concept map for igneous rocks
Concept map of rocks
Igneous rock concept map
Jessica gurevitch
Properties of matter concept map
Recall mapping marketing
What is this process called
Concept map of covalent bond
Halimbawa ng sistematikong pananaliksik
Concept map ng pagsulat
Packing mind map
Concept map multiple sclerosis
Nucleic acids concept map