Chapter 16 Oneway Analysis of Variance Confidence Limits
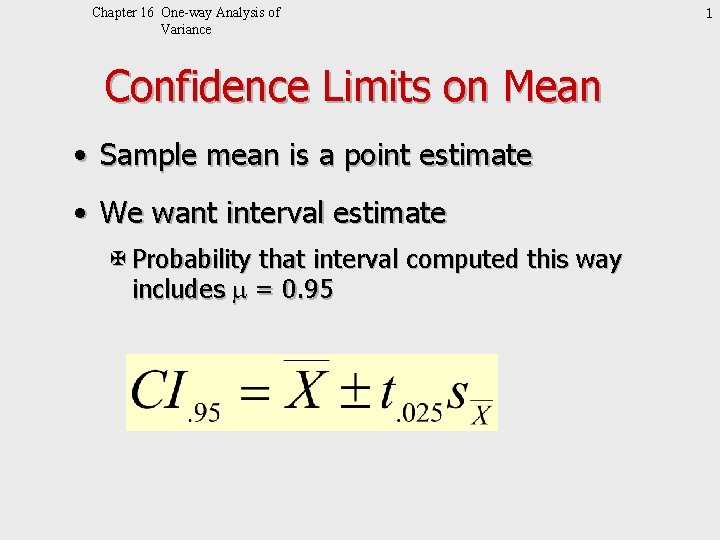
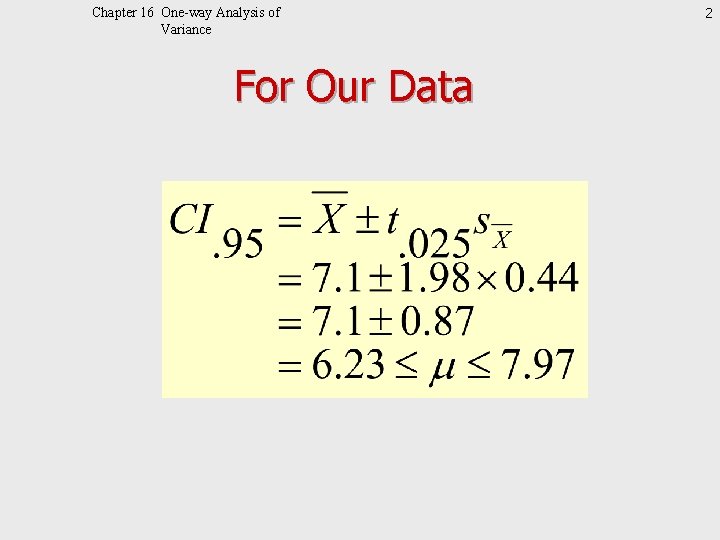
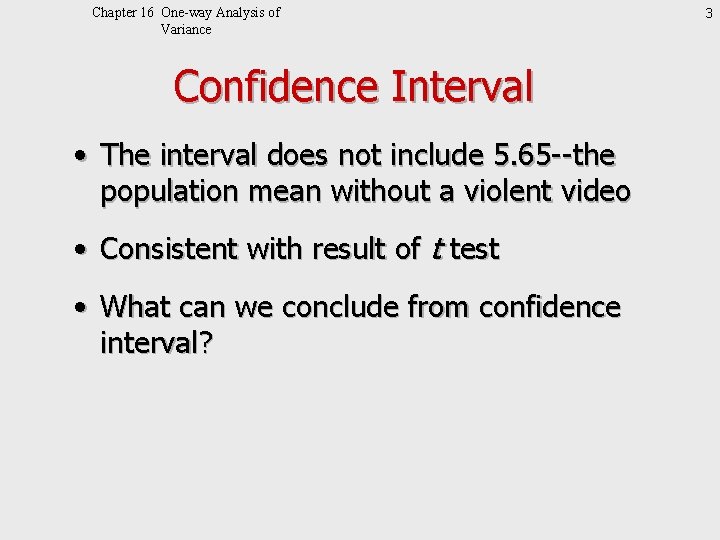
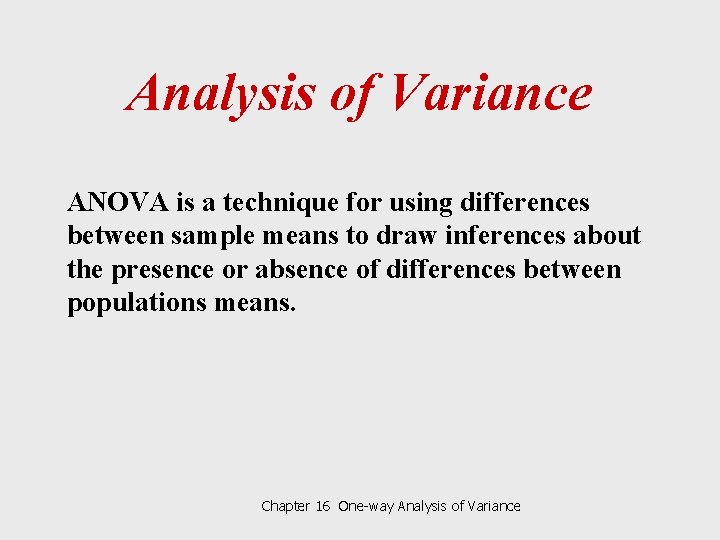
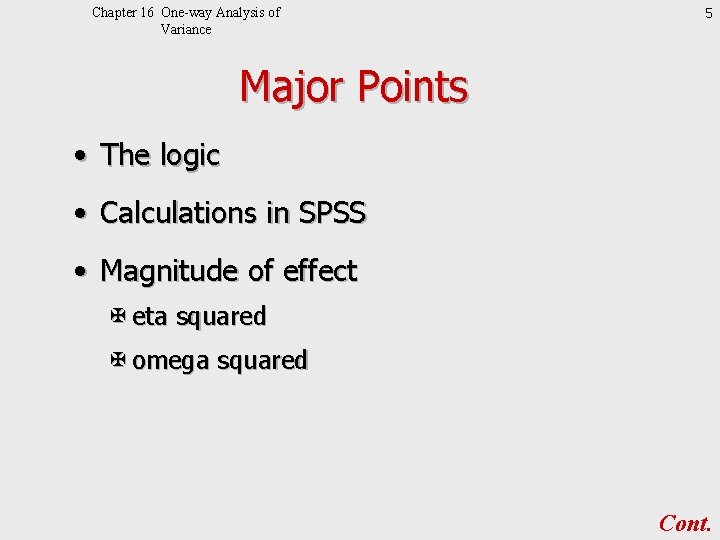
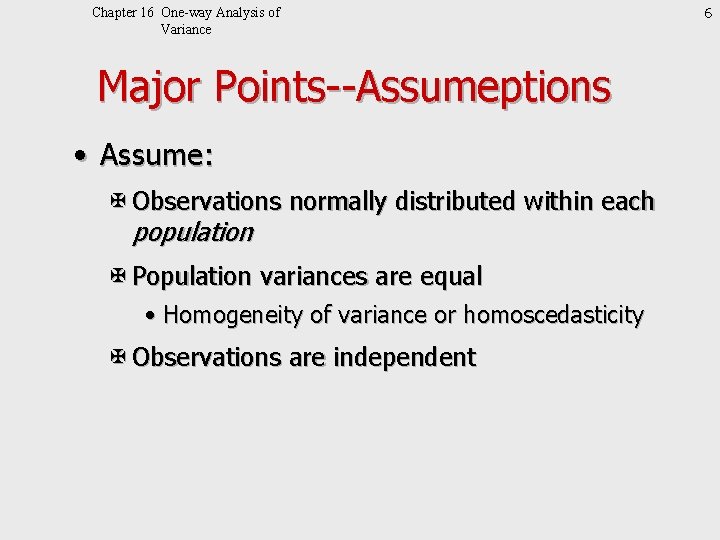
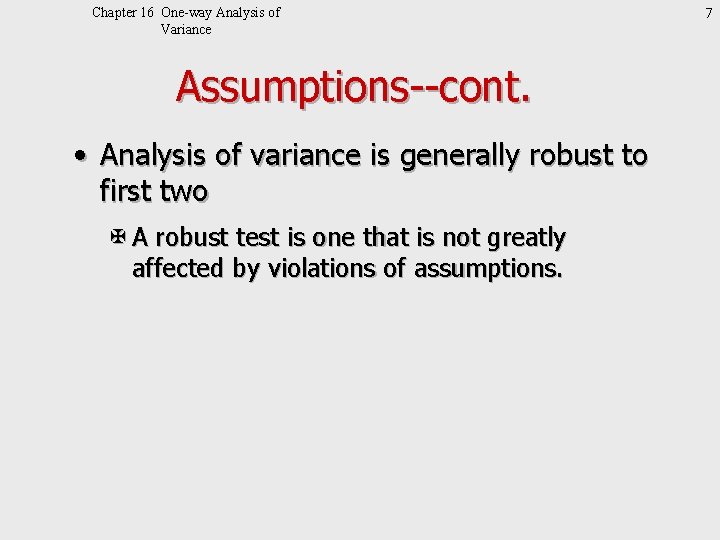
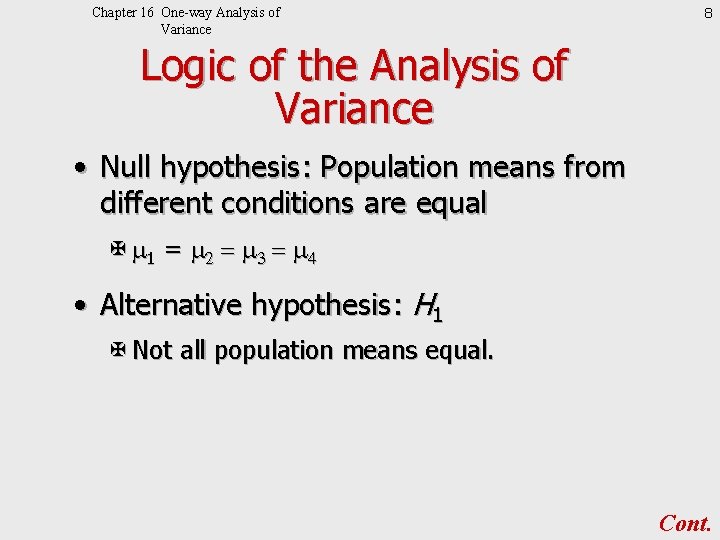
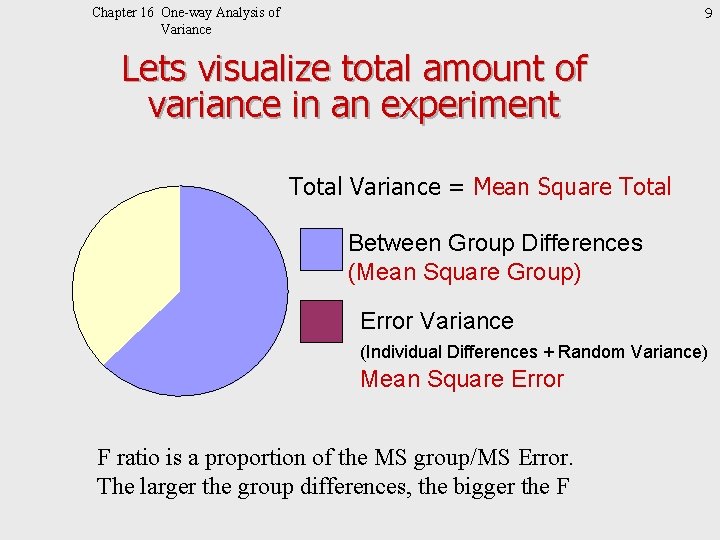
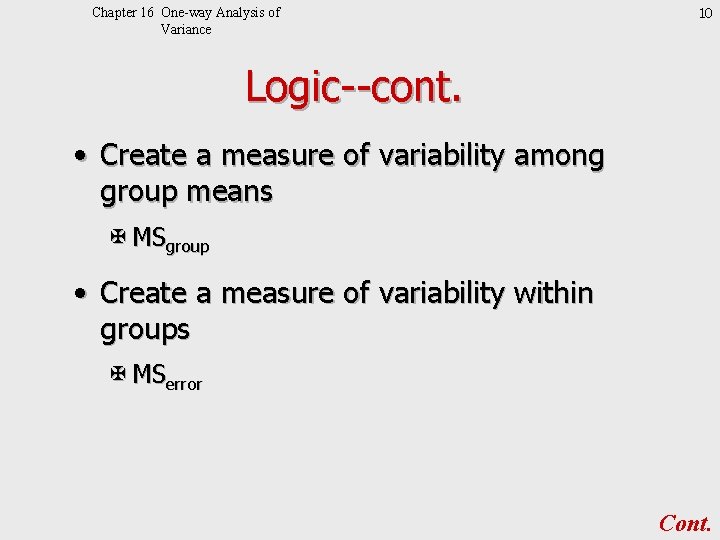
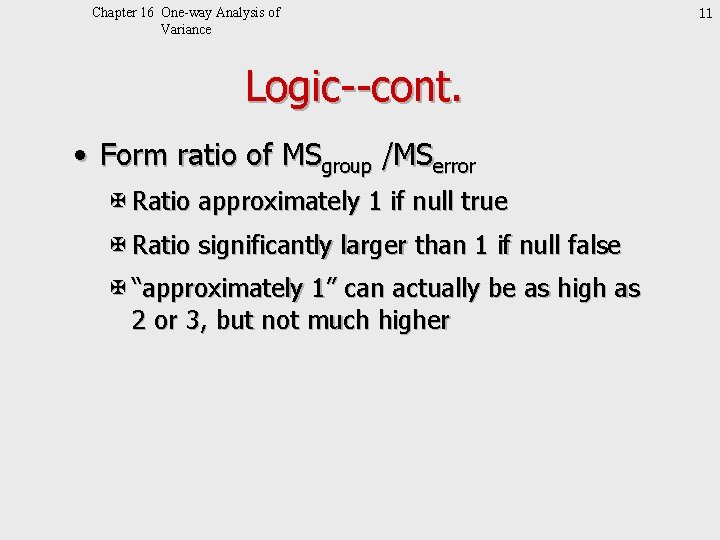
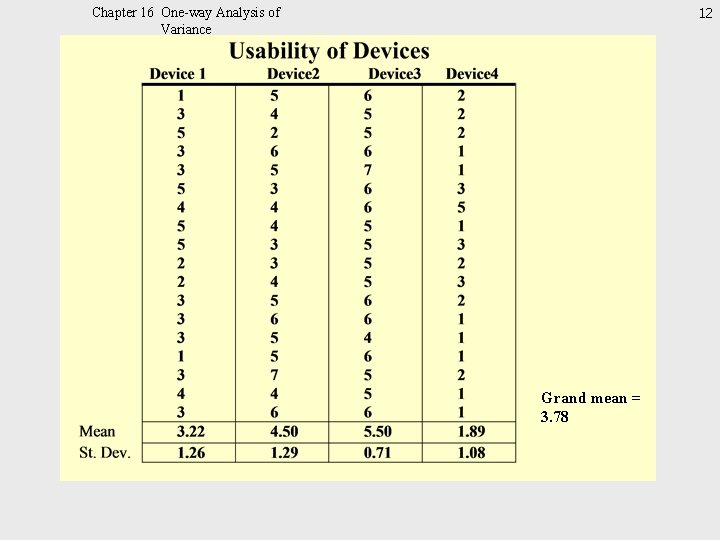
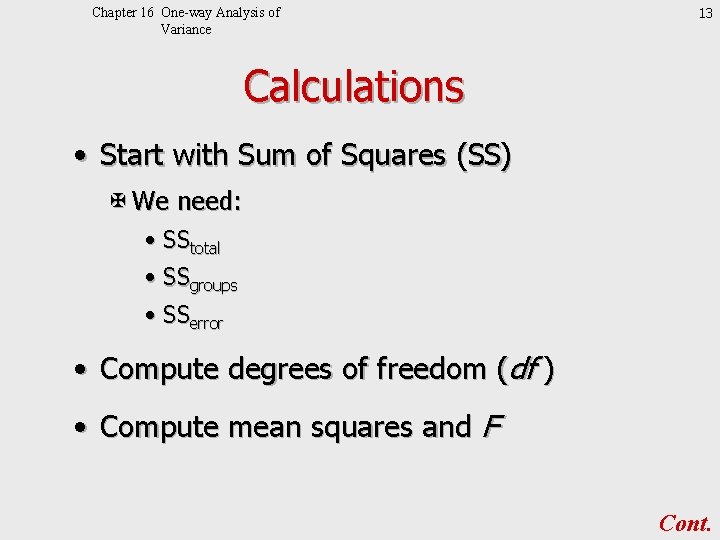
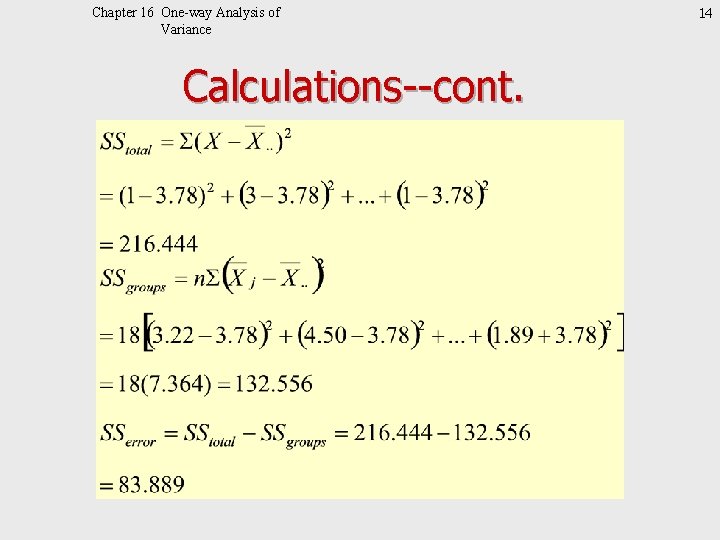
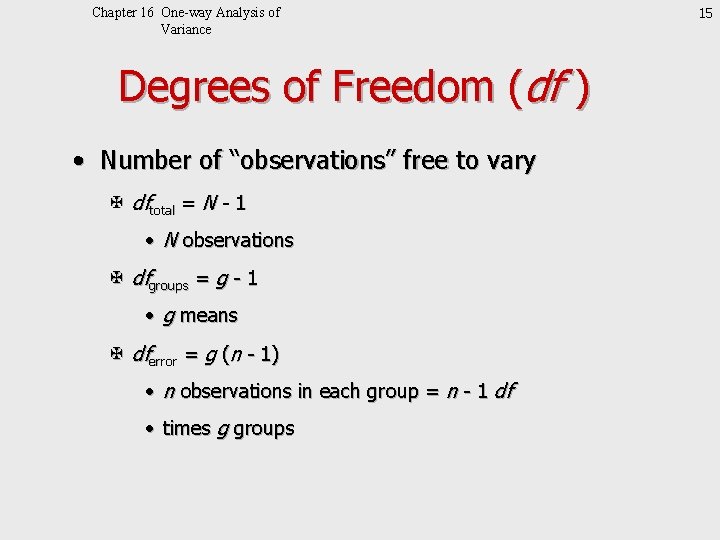
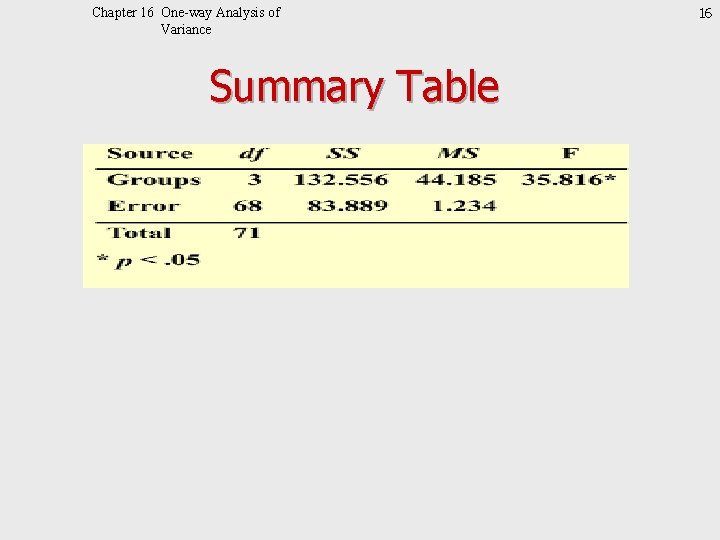
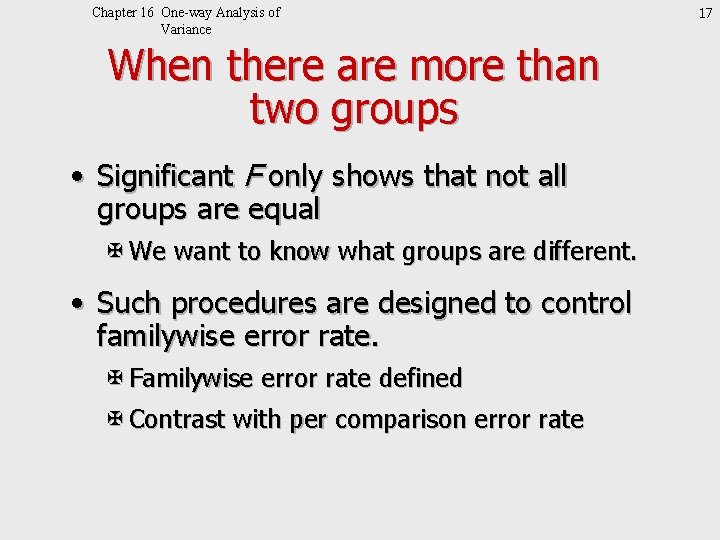
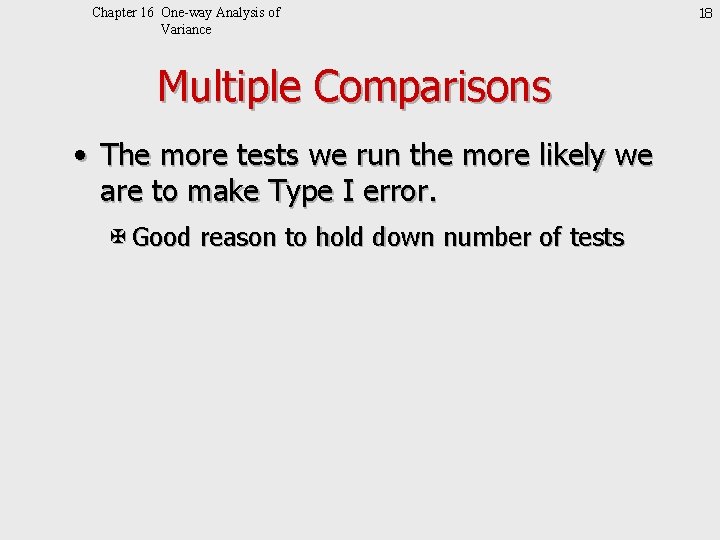
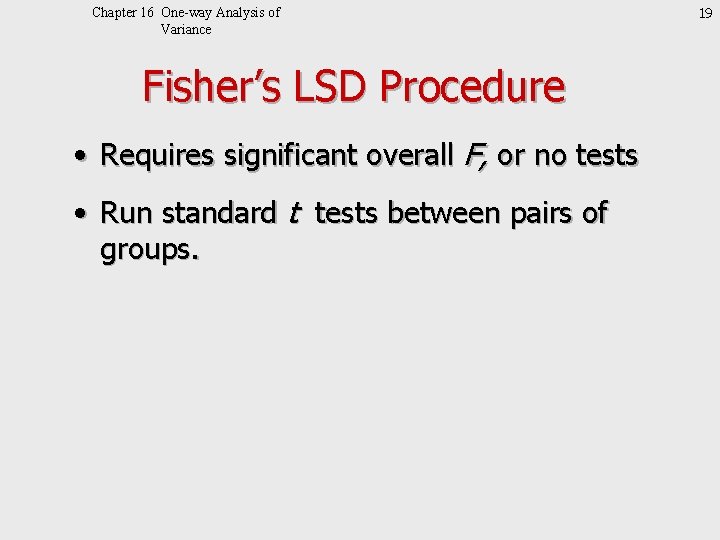
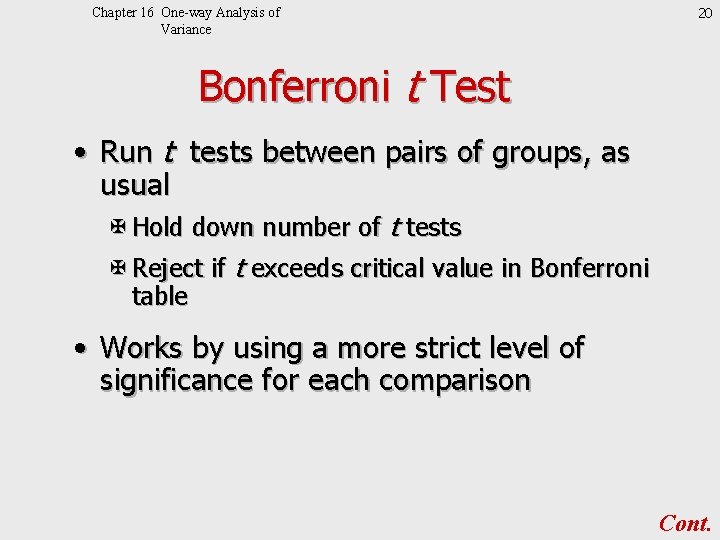
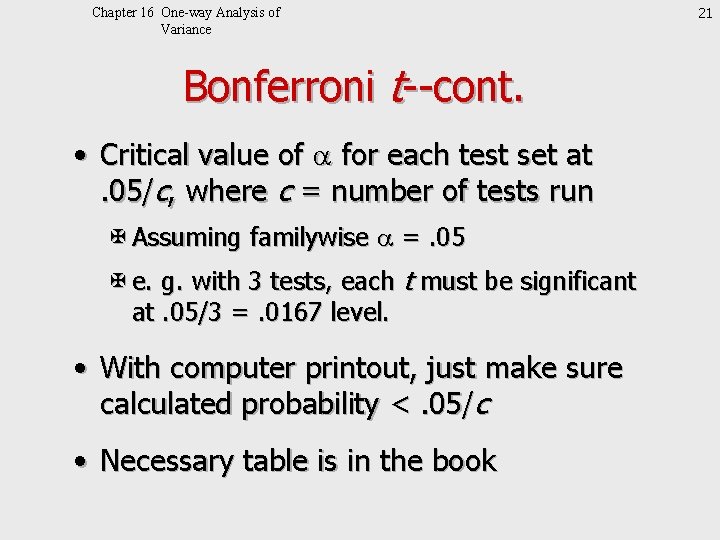
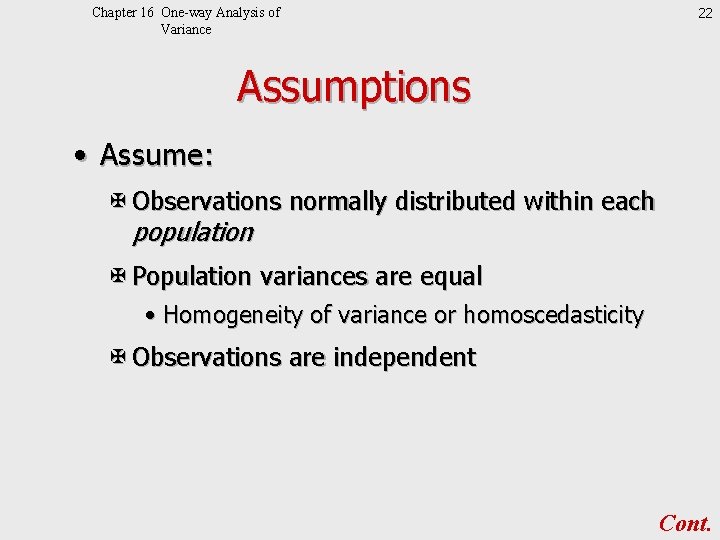
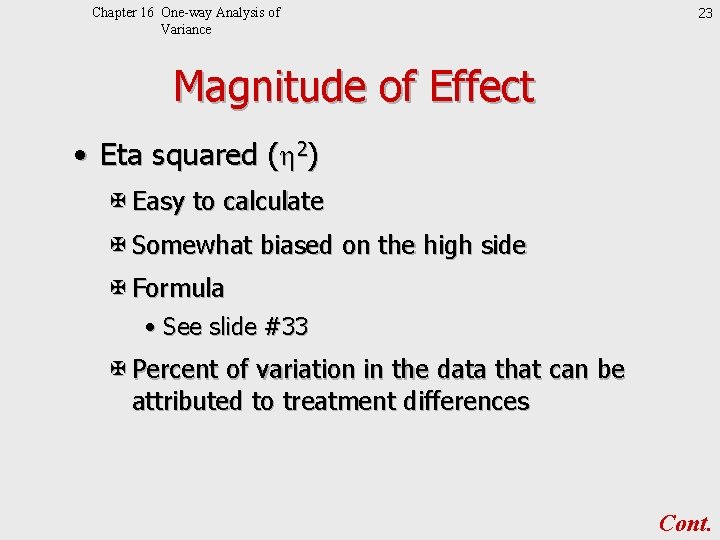
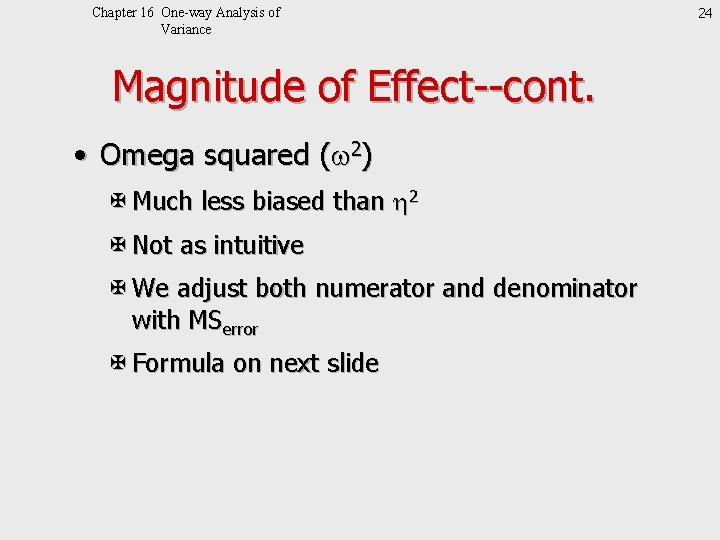
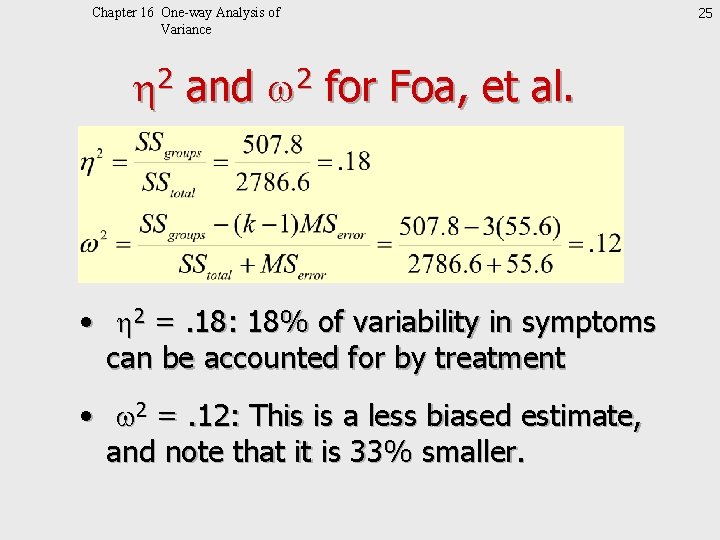
- Slides: 25
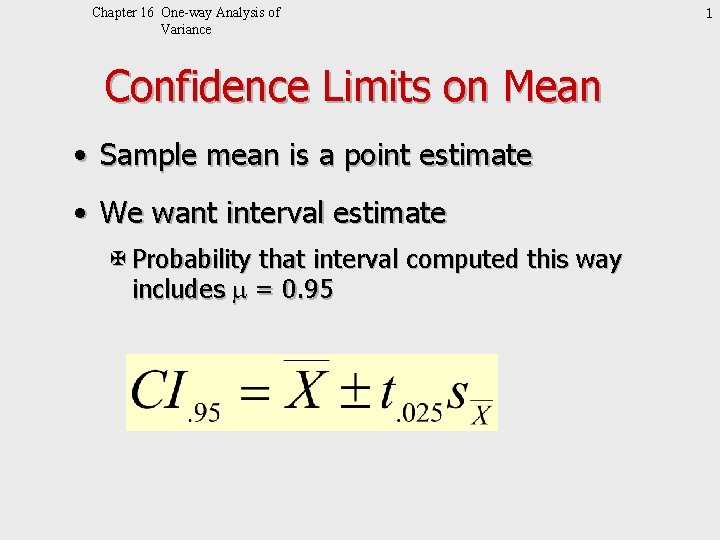
Chapter 16 One-way Analysis of Variance Confidence Limits on Mean • Sample mean is a point estimate • We want interval estimate X Probability that interval computed this way includes m = 0. 95 1
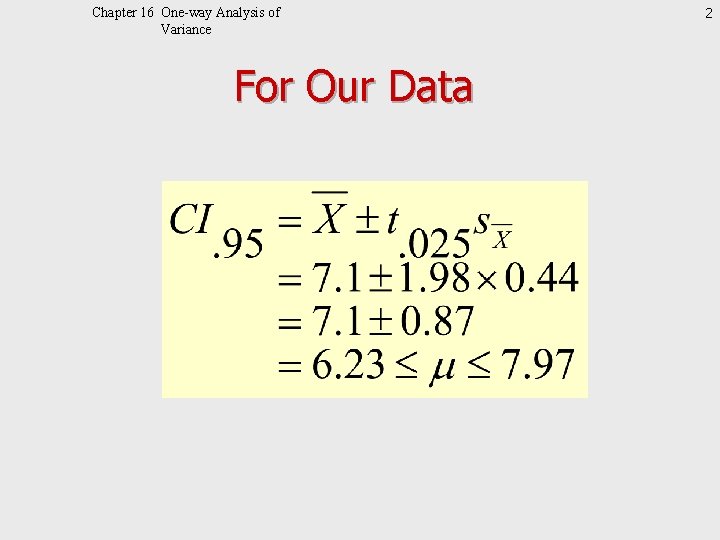
Chapter 16 One-way Analysis of Variance For Our Data 2
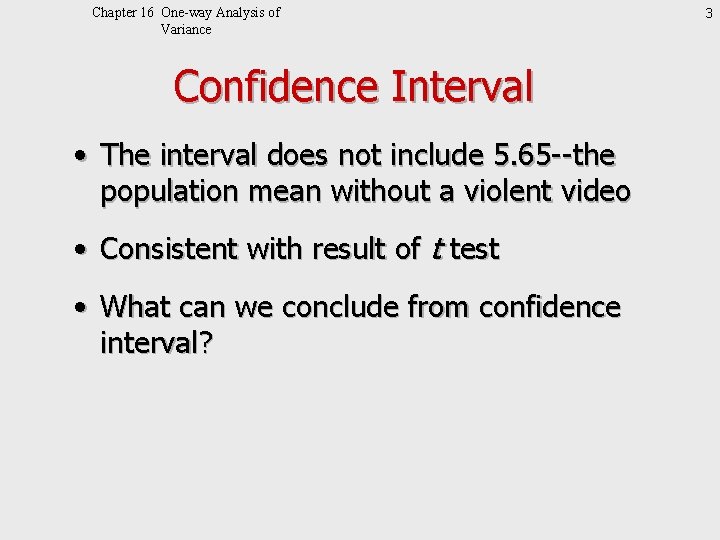
Chapter 16 One-way Analysis of Variance Confidence Interval • The interval does not include 5. 65 --the population mean without a violent video • Consistent with result of t test • What can we conclude from confidence interval? 3
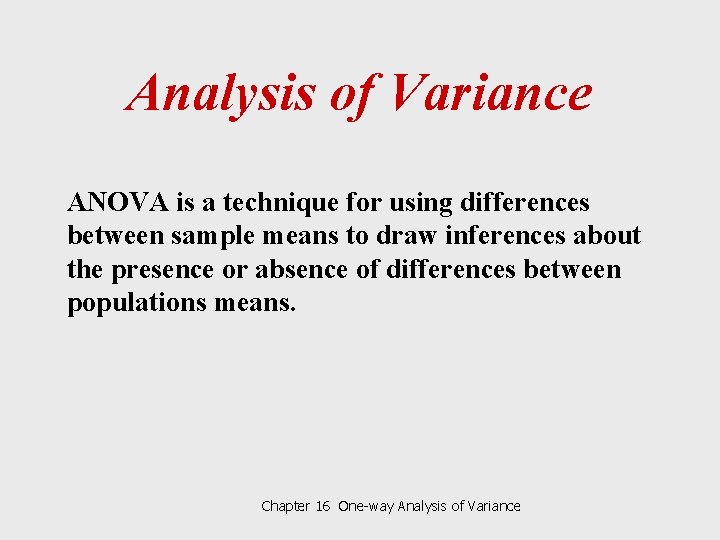
Analysis of Variance ANOVA is a technique for using differences between sample means to draw inferences about the presence or absence of differences between populations means. Chapter 16 One-way Analysis of Variance
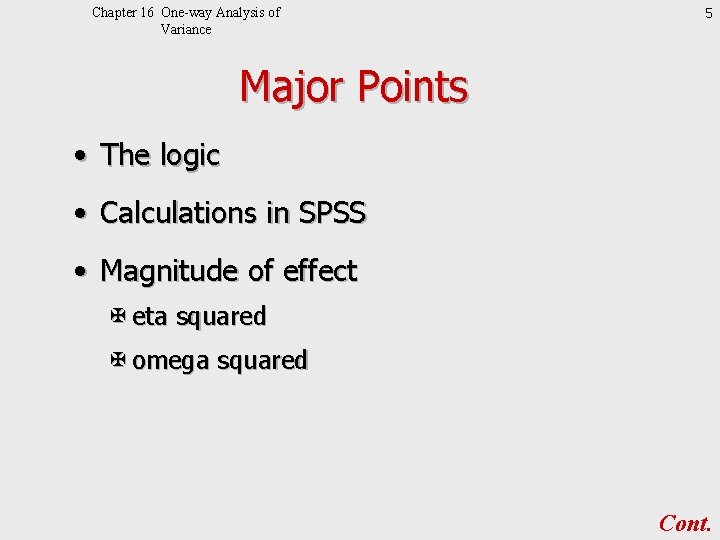
Chapter 16 One-way Analysis of Variance 5 Major Points • The logic • Calculations in SPSS • Magnitude of effect X eta squared X omega squared Cont.
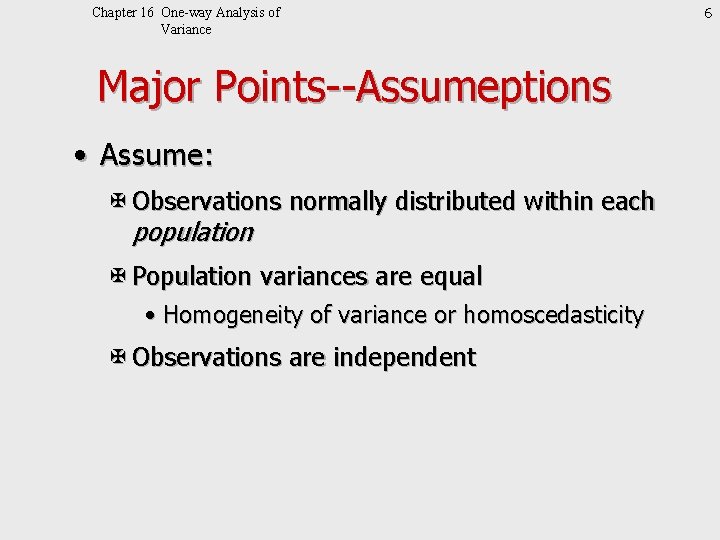
Chapter 16 One-way Analysis of Variance Major Points--Assumeptions • Assume: X Observations normally distributed within each population X Population variances are equal • Homogeneity of variance or homoscedasticity X Observations are independent 6
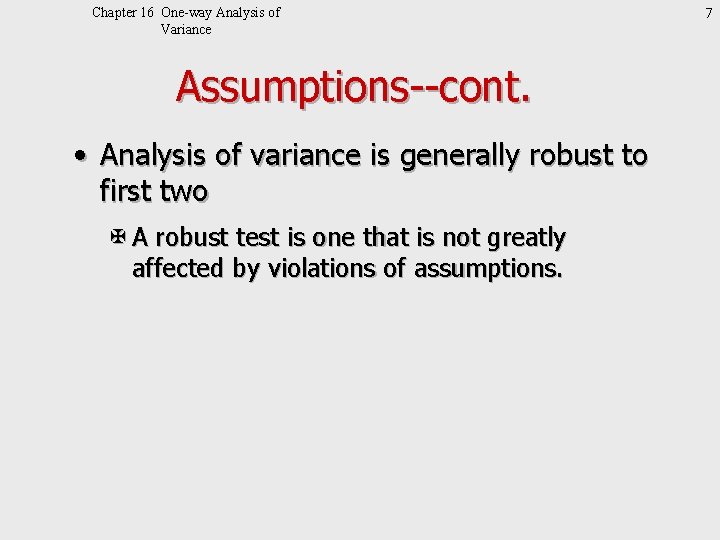
Chapter 16 One-way Analysis of Variance Assumptions--cont. • Analysis of variance is generally robust to first two X A robust test is one that is not greatly affected by violations of assumptions. 7
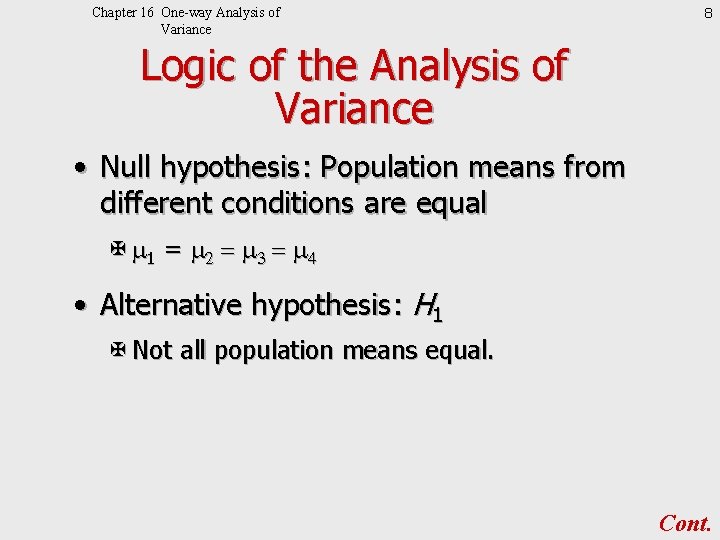
Chapter 16 One-way Analysis of Variance 8 Logic of the Analysis of Variance • Null hypothesis: Population means from different conditions are equal X m 1 = m 2 = m 3 = m 4 • Alternative hypothesis: H 1 X Not all population means equal. Cont.
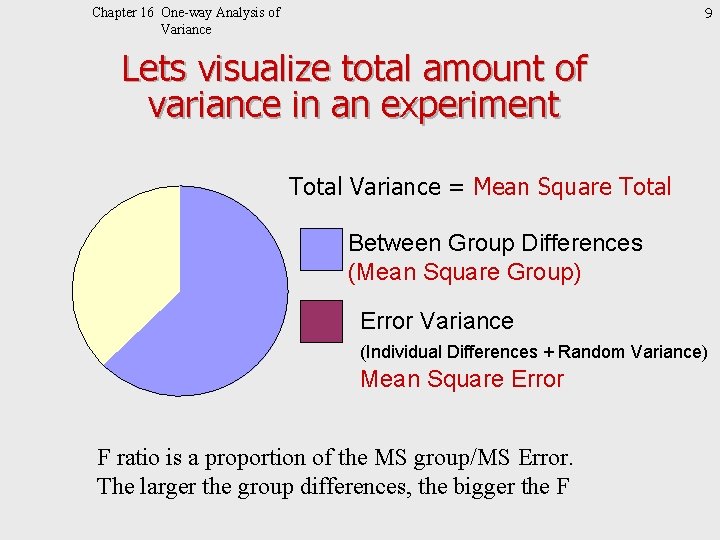
9 Chapter 16 One-way Analysis of Variance Lets visualize total amount of variance in an experiment Total Variance = Mean Square Total Between Group Differences (Mean Square Group) Error Variance (Individual Differences + Random Variance) Mean Square Error F ratio is a proportion of the MS group/MS Error. The larger the group differences, the bigger the F
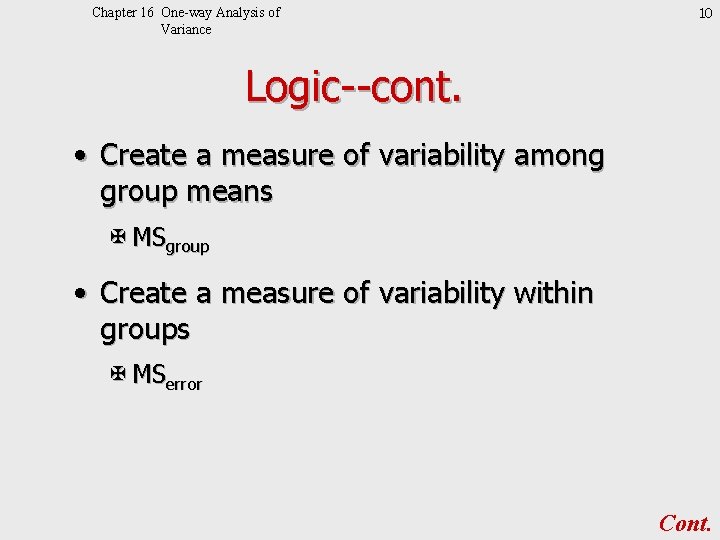
Chapter 16 One-way Analysis of Variance 10 Logic--cont. • Create a measure of variability among group means X MSgroup • Create a measure of variability within groups X MSerror Cont.
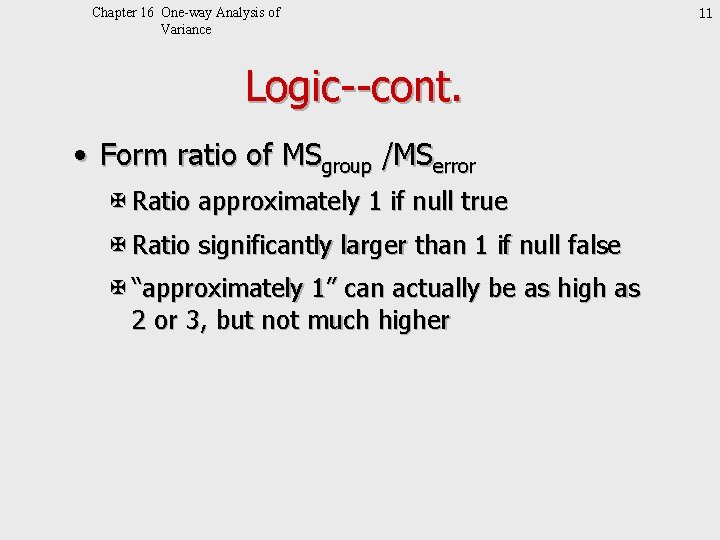
Chapter 16 One-way Analysis of Variance Logic--cont. • Form ratio of MSgroup /MSerror X Ratio approximately 1 if null true X Ratio significantly larger than 1 if null false X “approximately 1” can actually be as high as 2 or 3, but not much higher 11
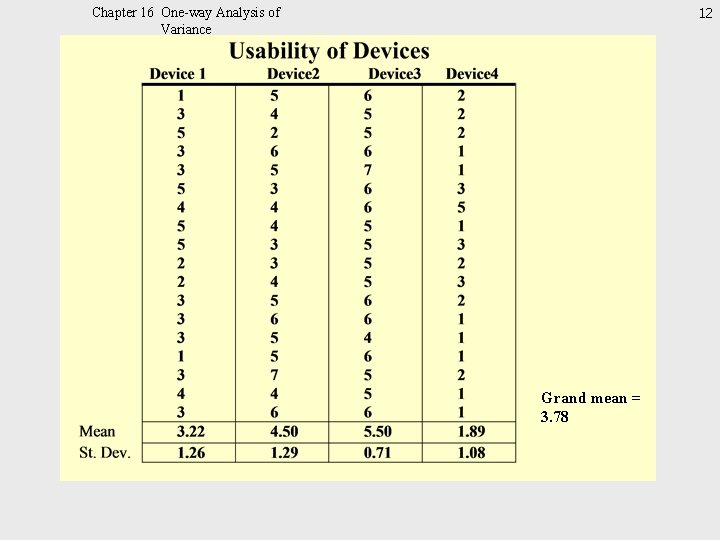
12 Chapter 16 One-way Analysis of Variance Grand mean = 3. 78
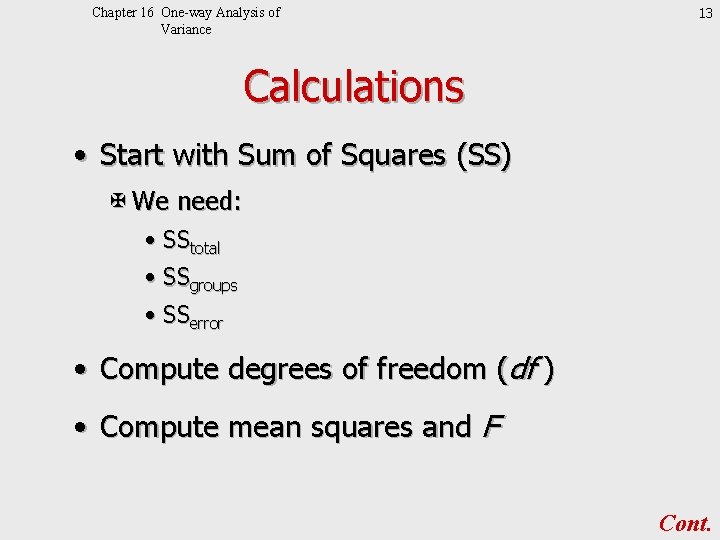
Chapter 16 One-way Analysis of Variance 13 Calculations • Start with Sum of Squares (SS) X We need: • SStotal • SSgroups • SSerror • Compute degrees of freedom (df ) • Compute mean squares and F Cont.
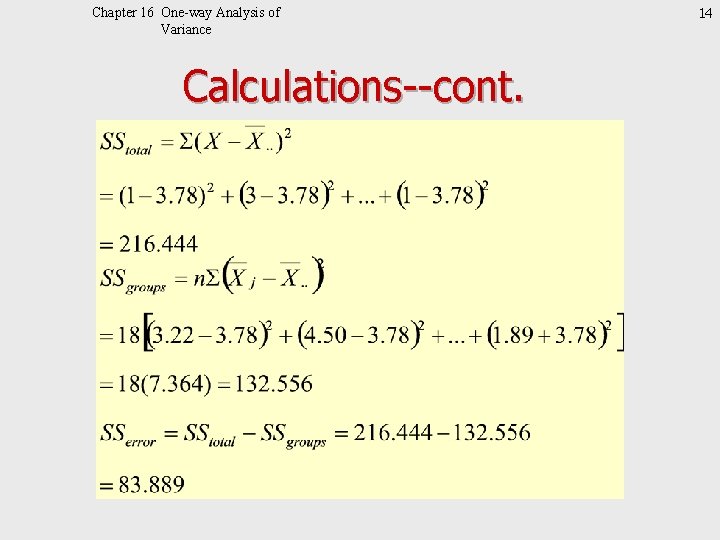
Chapter 16 One-way Analysis of Variance Calculations--cont. 14
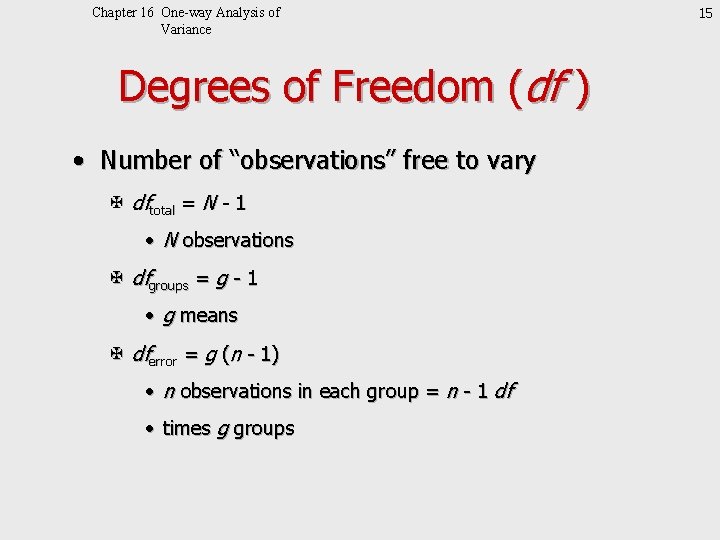
Chapter 16 One-way Analysis of Variance Degrees of Freedom (df ) • Number of “observations” free to vary X dftotal = N - 1 • N observations X dfgroups = g - 1 • g means X dferror = g (n - 1) • n observations in each group = n - 1 df • times g groups 15
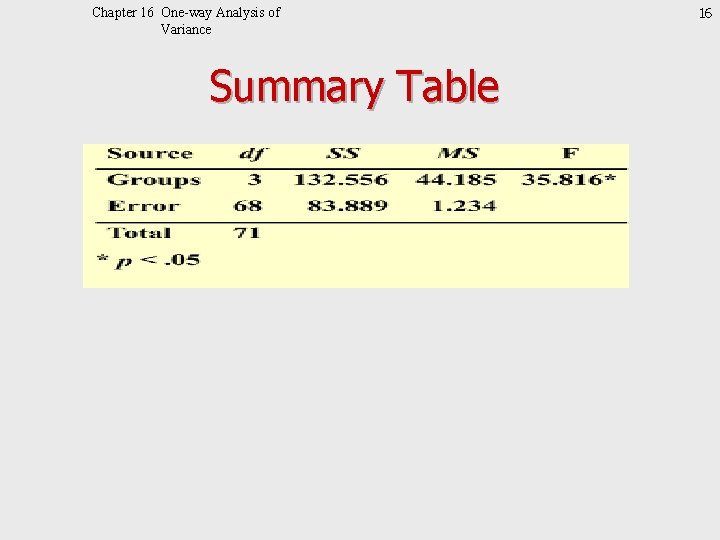
Chapter 16 One-way Analysis of Variance Summary Table 16
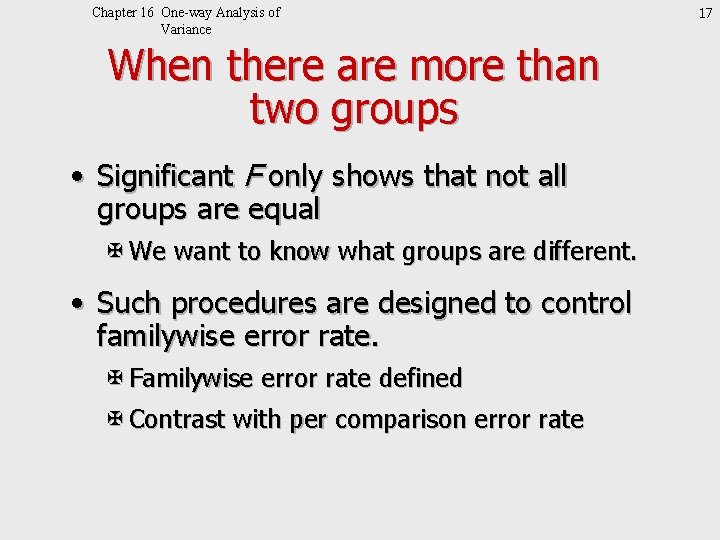
Chapter 16 One-way Analysis of Variance When there are more than two groups • Significant F only shows that not all groups are equal X We want to know what groups are different. • Such procedures are designed to control familywise error rate. X Familywise error rate defined X Contrast with per comparison error rate 17
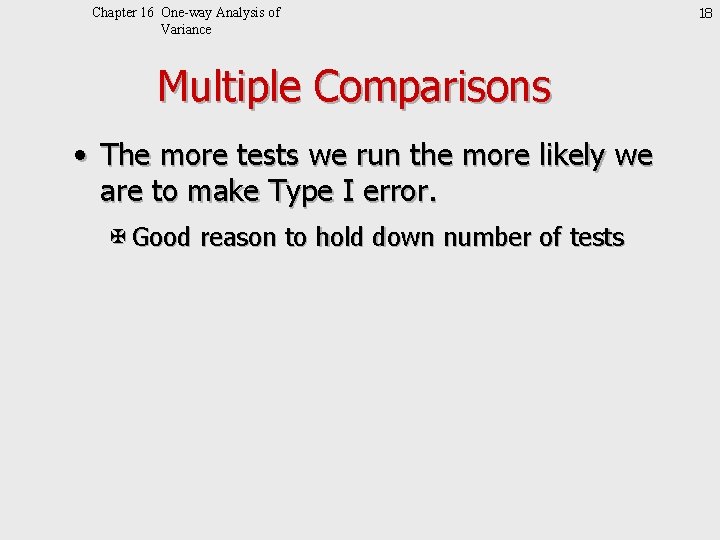
Chapter 16 One-way Analysis of Variance Multiple Comparisons • The more tests we run the more likely we are to make Type I error. X Good reason to hold down number of tests 18
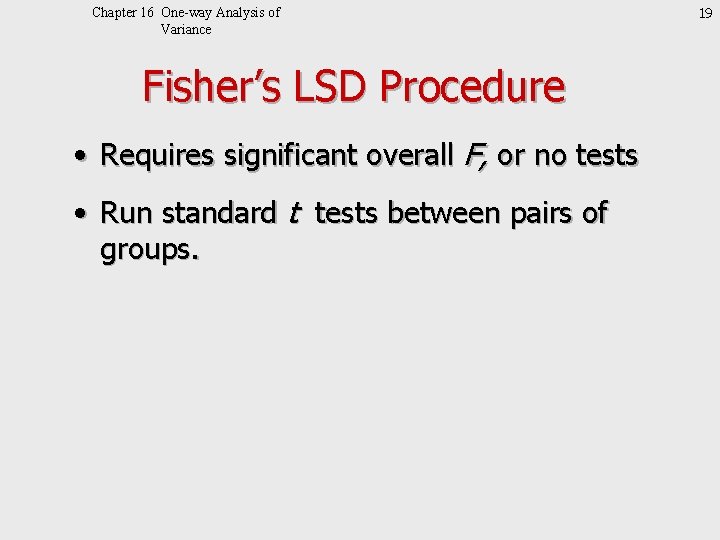
Chapter 16 One-way Analysis of Variance Fisher’s LSD Procedure • Requires significant overall F, or no tests • Run standard t tests between pairs of groups. 19
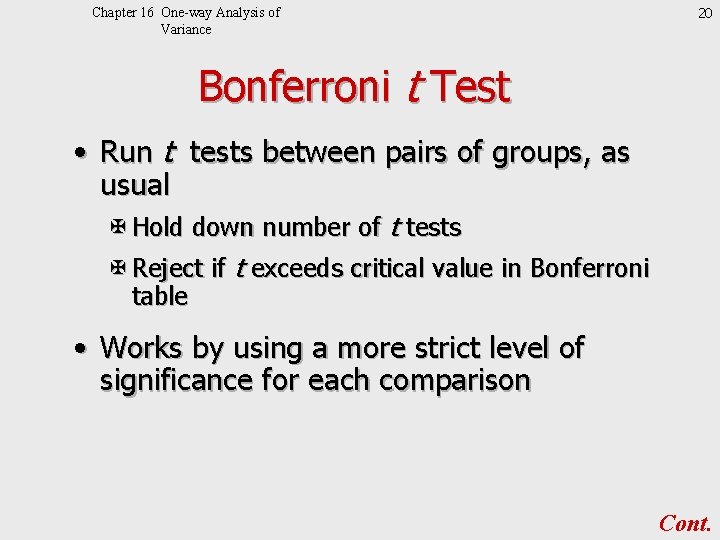
Chapter 16 One-way Analysis of Variance 20 Bonferroni t Test • Run t tests between pairs of groups, as usual X Hold down number of t tests X Reject if t exceeds critical value in Bonferroni table • Works by using a more strict level of significance for each comparison Cont.
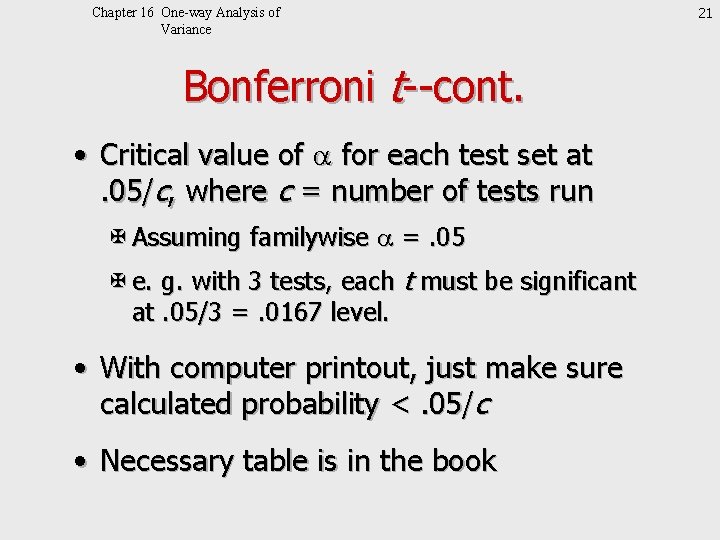
Chapter 16 One-way Analysis of Variance Bonferroni t--cont. • Critical value of a for each test set at. 05/c, where c = number of tests run X Assuming familywise a =. 05 X e. g. with 3 tests, each t must be significant at. 05/3 =. 0167 level. • With computer printout, just make sure calculated probability <. 05/c • Necessary table is in the book 21
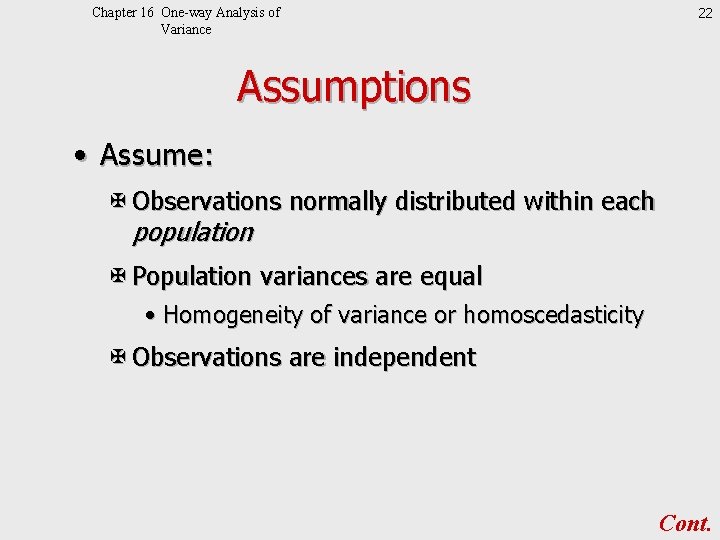
Chapter 16 One-way Analysis of Variance 22 Assumptions • Assume: X Observations normally distributed within each population X Population variances are equal • Homogeneity of variance or homoscedasticity X Observations are independent Cont.
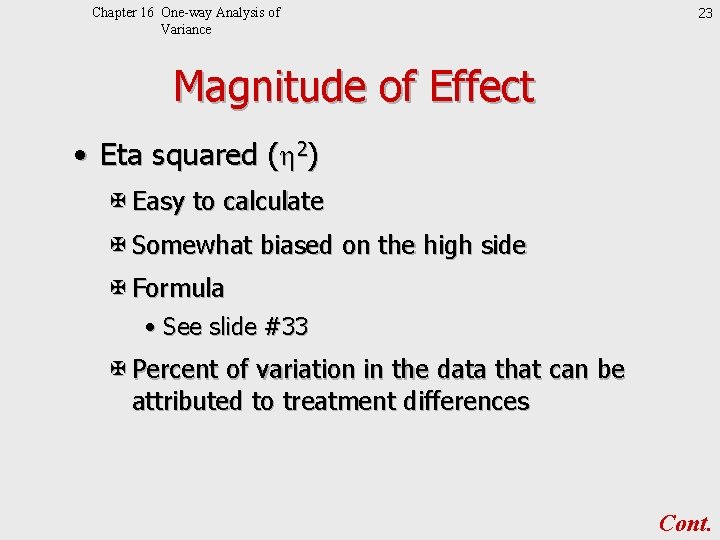
Chapter 16 One-way Analysis of Variance 23 Magnitude of Effect • Eta squared (h 2) X Easy to calculate X Somewhat biased on the high side X Formula • See slide #33 X Percent of variation in the data that can be attributed to treatment differences Cont.
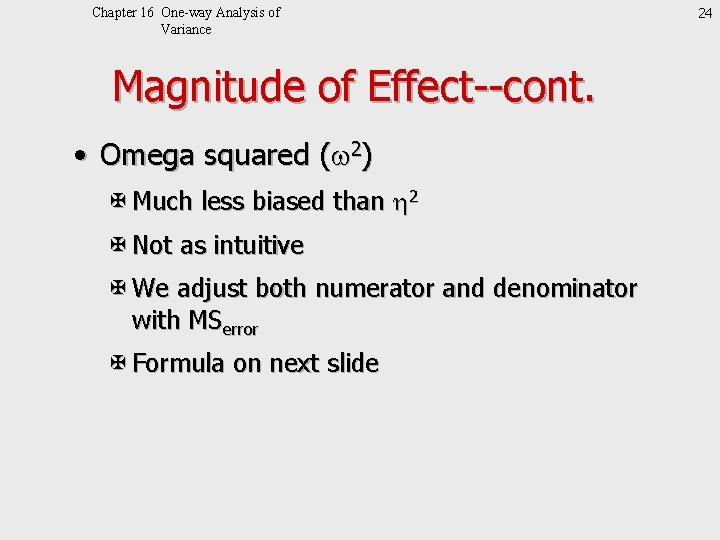
Chapter 16 One-way Analysis of Variance Magnitude of Effect--cont. • Omega squared (w 2) X Much less biased than h 2 X Not as intuitive X We adjust both numerator and denominator with MSerror X Formula on next slide 24
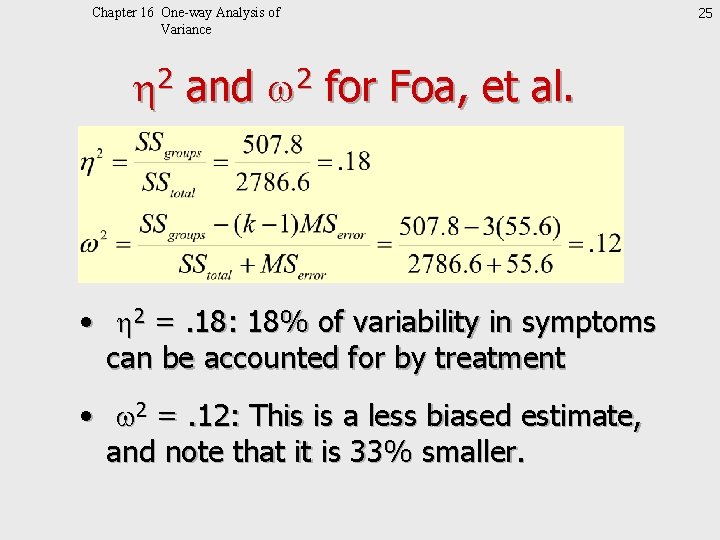
Chapter 16 One-way Analysis of Variance h 2 and w 2 for Foa, et al. • h 2 =. 18: 18% of variability in symptoms can be accounted for by treatment • w 2 =. 12: This is a less biased estimate, and note that it is 33% smaller. 25
Stata oneway
Jeff boote
Oneway hash
Limits involving infinity
Histogram polygon graph
Material yield variance formula
Confidence interval for population variance formula
Confidence interval for variance
Sample size
Confident
Confidence interval vs confidence level
How to interpret confidence intervals example
Static budget variance
The variance analysis cycle
Budget variance formula
Standard costing and variance analysis formulas
Manova definition
Direct materials variances
Multivariate analysis of variance and covariance
Standard cost vs budgeted cost
Job cost variance
Mixed analysis of variance
Variance analysis in nursing
Sales activity variance
Introduction to analysis of variance
Analysis of variance and covariance