Audiovisual Event Detection Recognition Audiovisual speech recognition Manifold
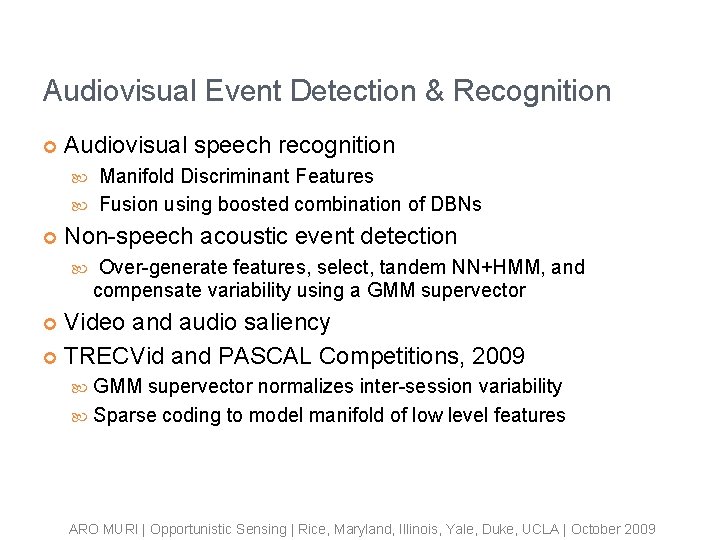
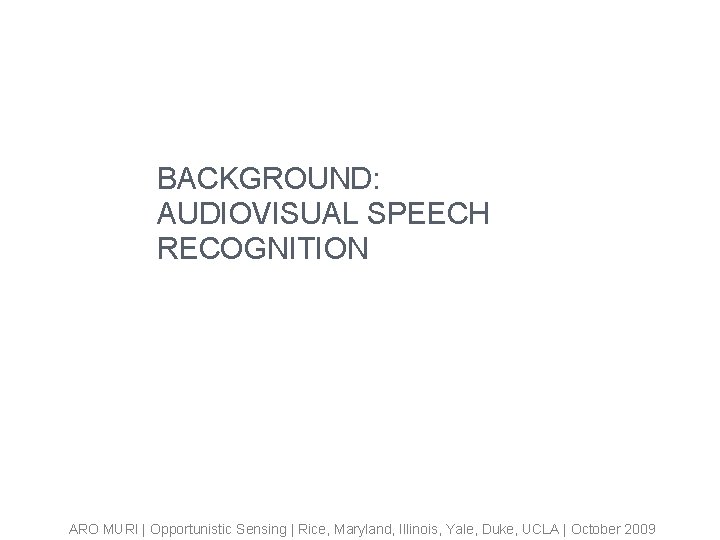
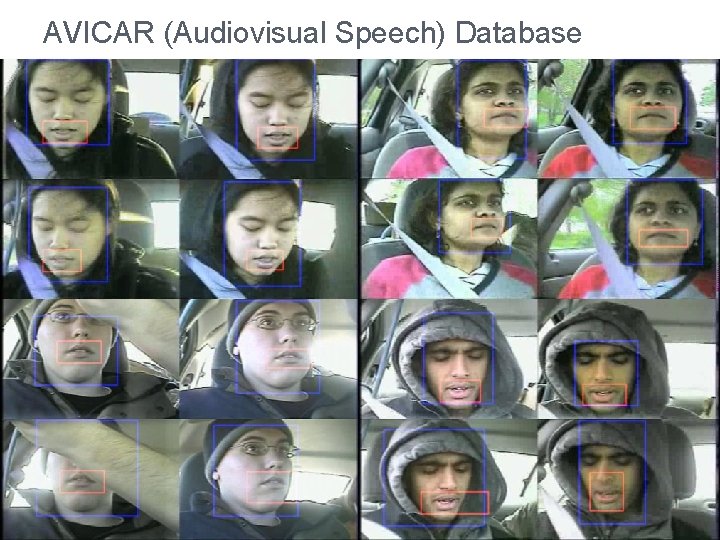
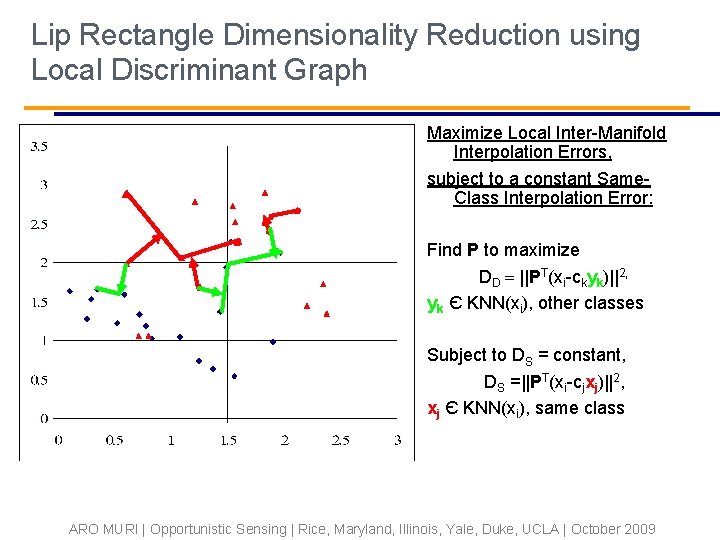
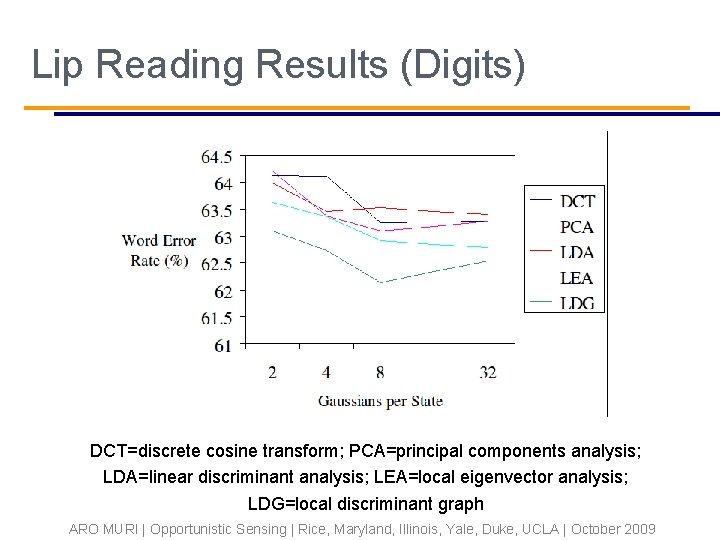
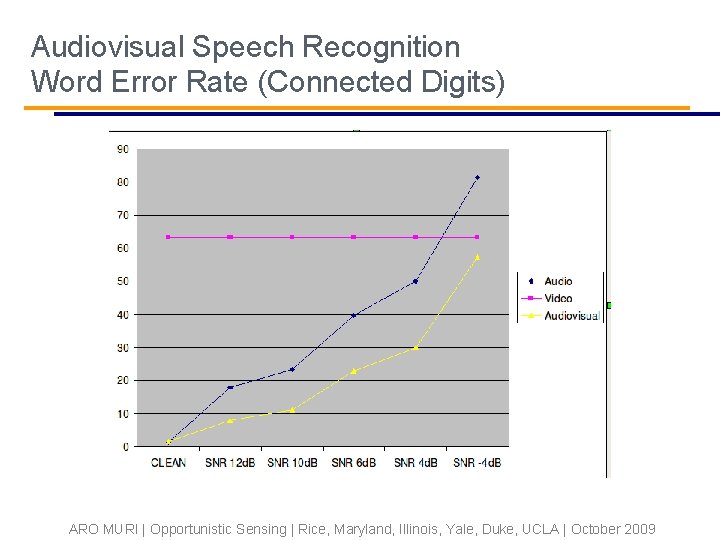
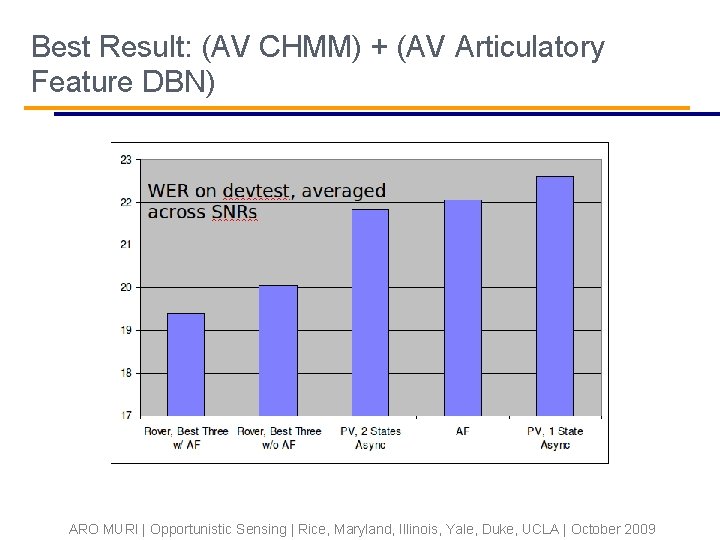
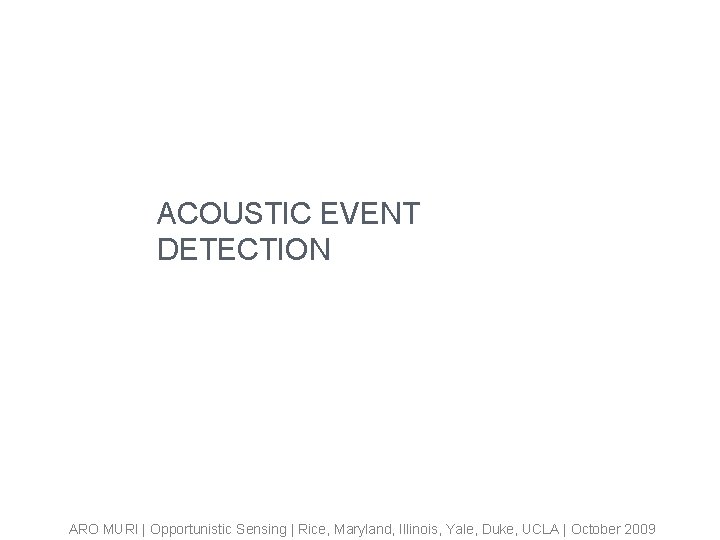
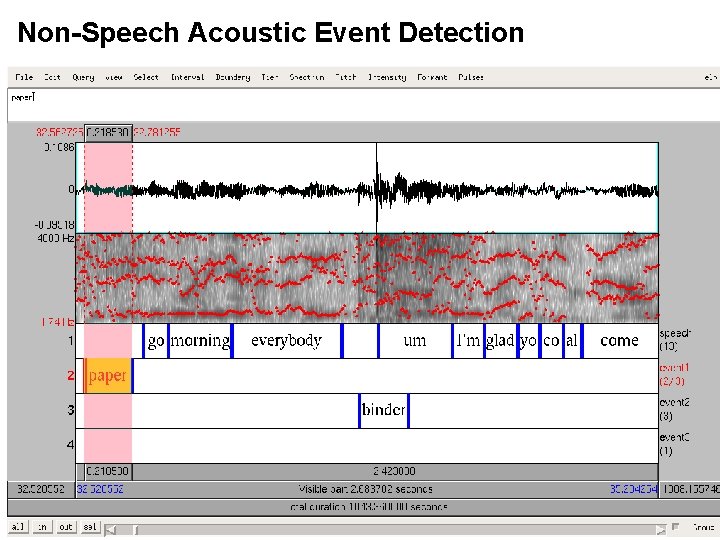
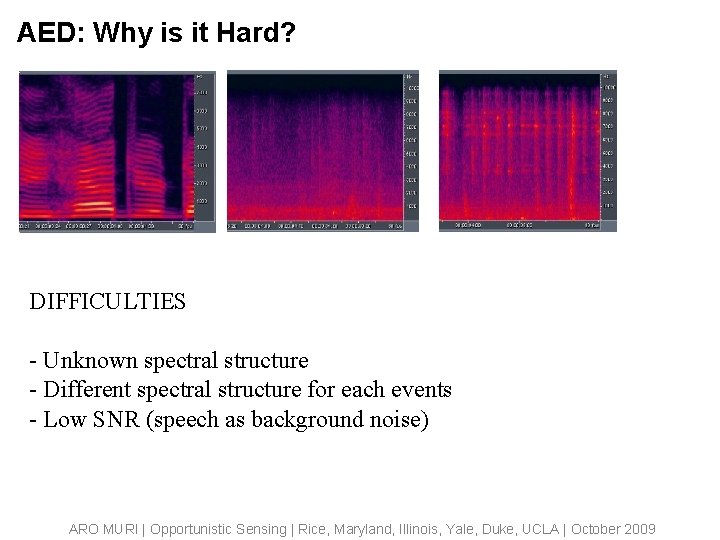
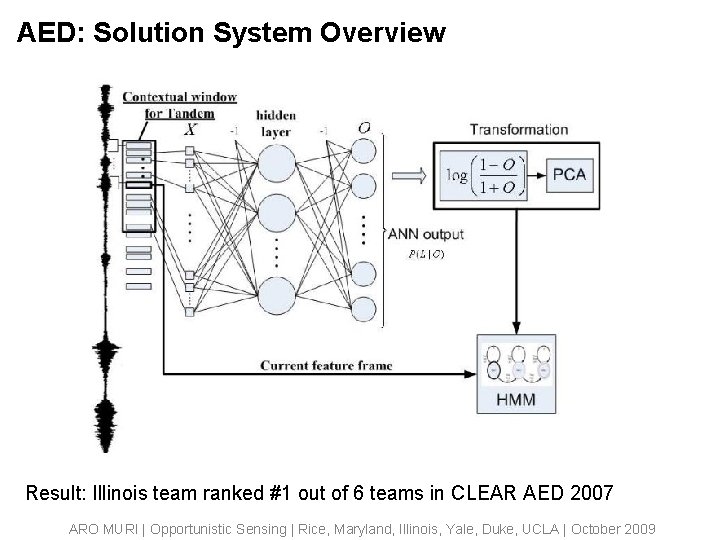
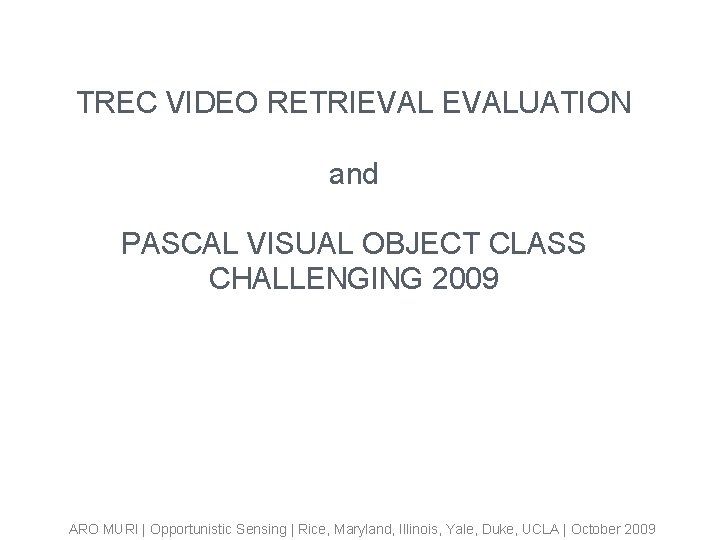
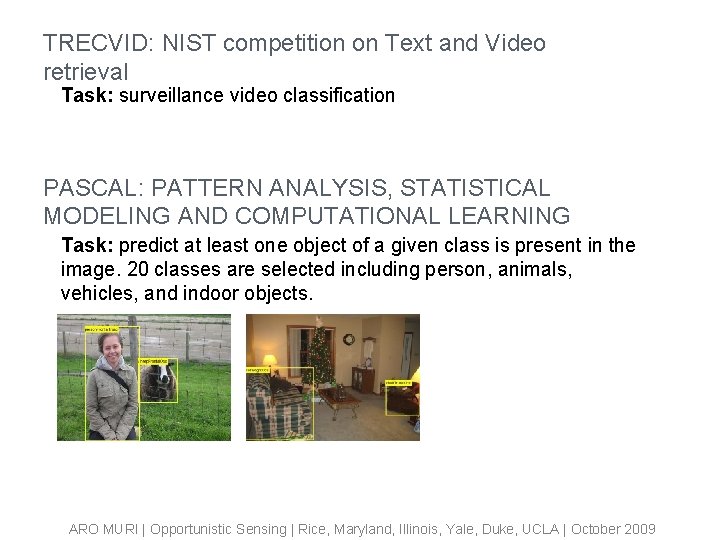
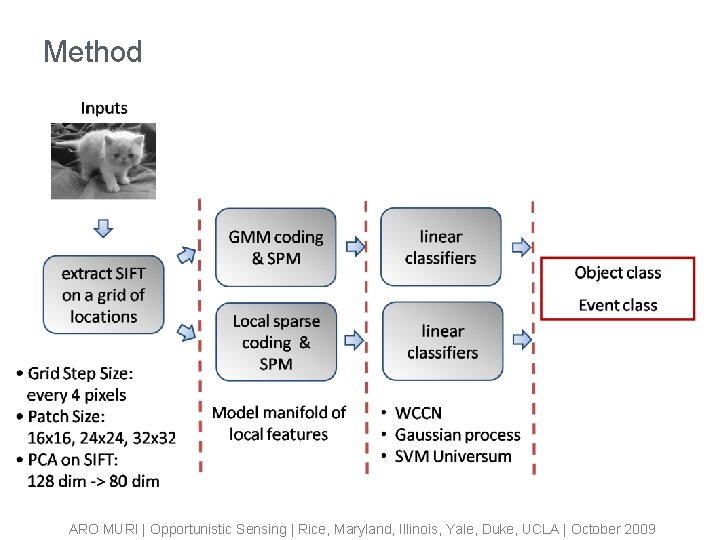
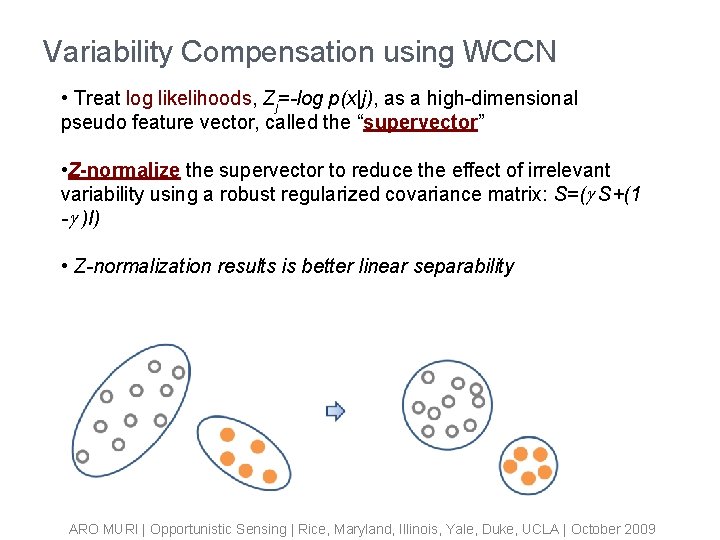
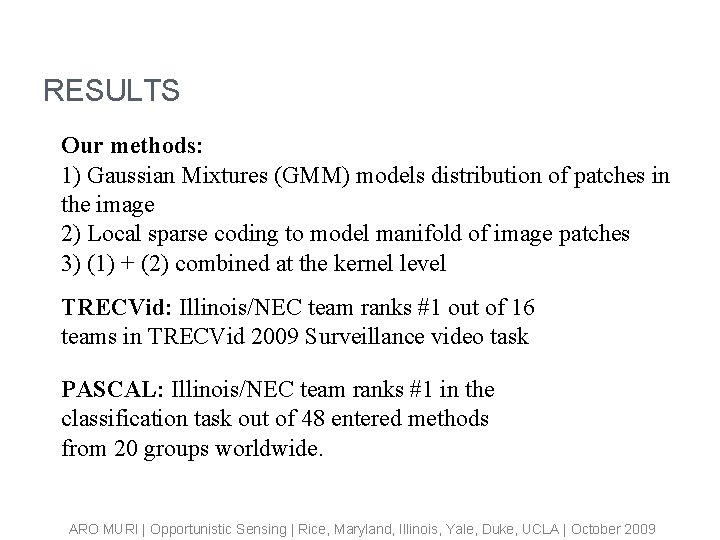
- Slides: 16
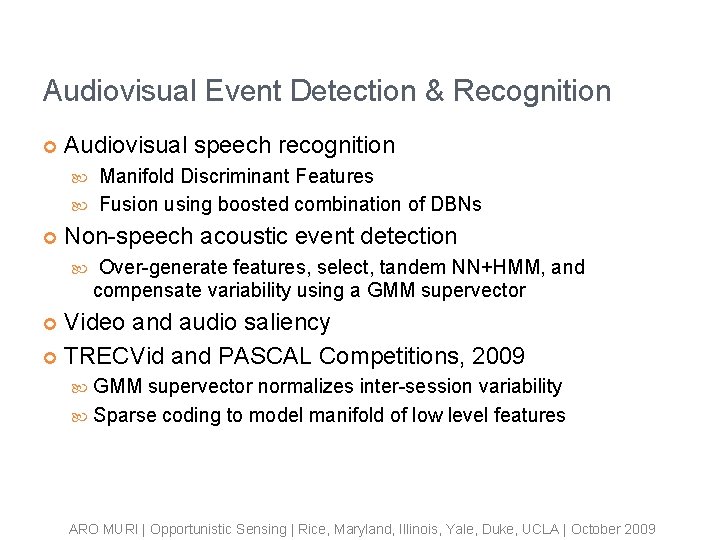
Audiovisual Event Detection & Recognition Audiovisual speech recognition Manifold Discriminant Features Fusion using boosted combination of DBNs Non-speech acoustic event detection Over-generate features, select, tandem NN+HMM, and compensate variability using a GMM supervector Video and audio saliency TRECVid and PASCAL Competitions, 2009 GMM supervector normalizes inter-session variability Sparse coding to model manifold of low level features ARO MURI | Opportunistic Sensing | Rice, Maryland, Illinois, Yale, Duke, UCLA | October 2009
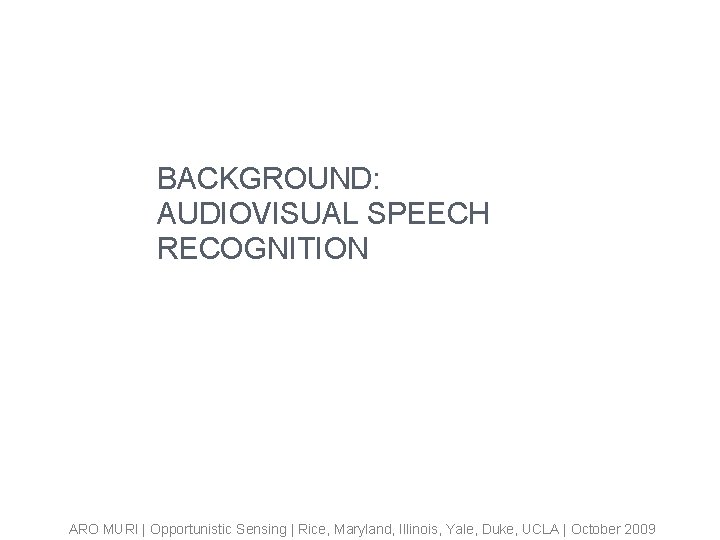
BACKGROUND: AUDIOVISUAL SPEECH RECOGNITION ARO MURI | Opportunistic Sensing | Rice, Maryland, Illinois, Yale, Duke, UCLA | October 2009
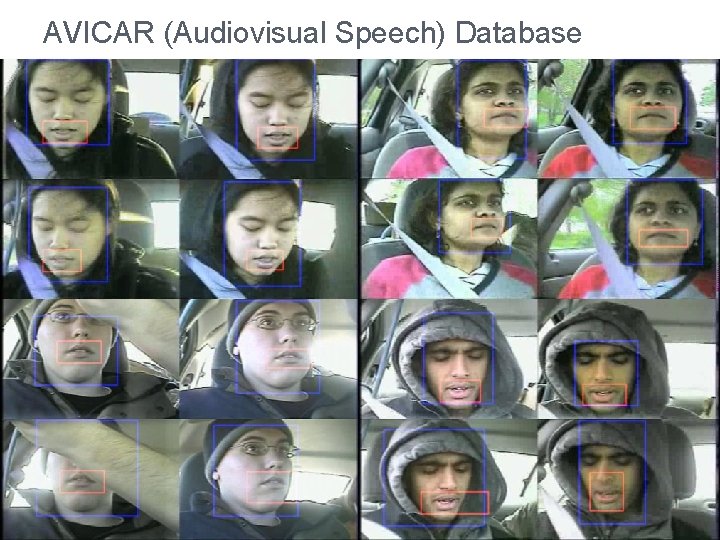
AVICAR (Audiovisual Speech) Database ARO MURI | Opportunistic Sensing | Rice, Maryland, Illinois, Yale, Duke, UCLA | October 2009
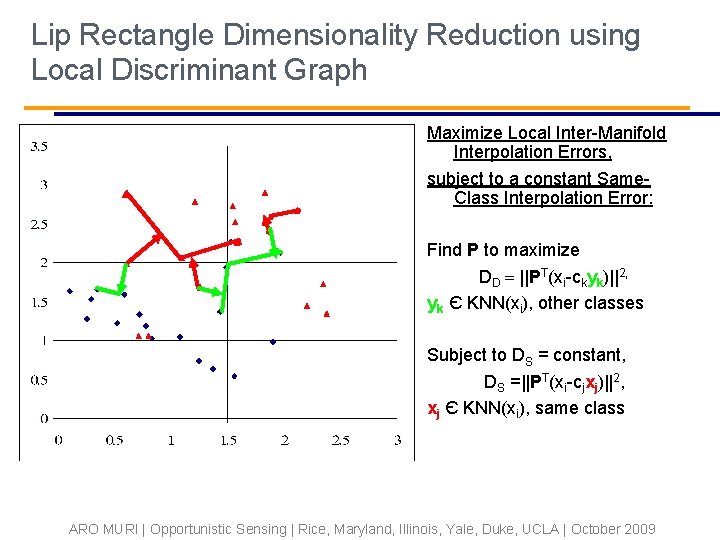
Lip Rectangle Dimensionality Reduction using Local Discriminant Graph Maximize Local Inter-Manifold Interpolation Errors, subject to a constant Same. Class Interpolation Error: Find P to maximize DD ||PT(xi-ckyk)||2, yk Є KNN(xi), other classes Subject to DS = constant, DS =||PT(xi-cjxj)||2, xj Є KNN(xi), same class ARO MURI | Opportunistic Sensing | Rice, Maryland, Illinois, Yale, Duke, UCLA | October 2009
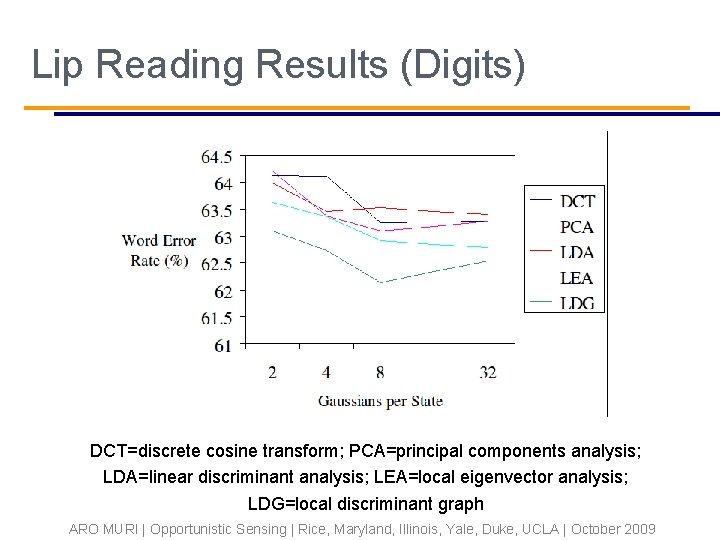
Lip Reading Results (Digits) DCT=discrete cosine transform; PCA=principal components analysis; LDA=linear discriminant analysis; LEA=local eigenvector analysis; LDG=local discriminant graph ARO MURI | Opportunistic Sensing | Rice, Maryland, Illinois, Yale, Duke, UCLA | October 2009
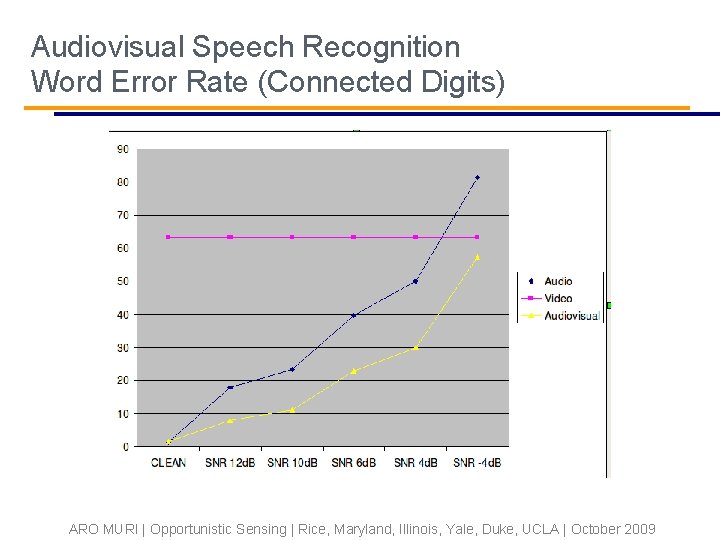
Audiovisual Speech Recognition Word Error Rate (Connected Digits) ARO MURI | Opportunistic Sensing | Rice, Maryland, Illinois, Yale, Duke, UCLA | October 2009
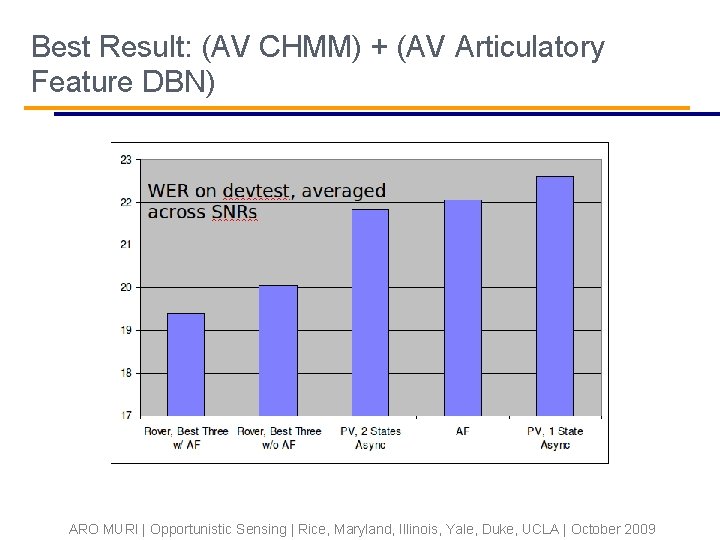
Best Result: (AV CHMM) + (AV Articulatory Feature DBN) ARO MURI | Opportunistic Sensing | Rice, Maryland, Illinois, Yale, Duke, UCLA | October 2009
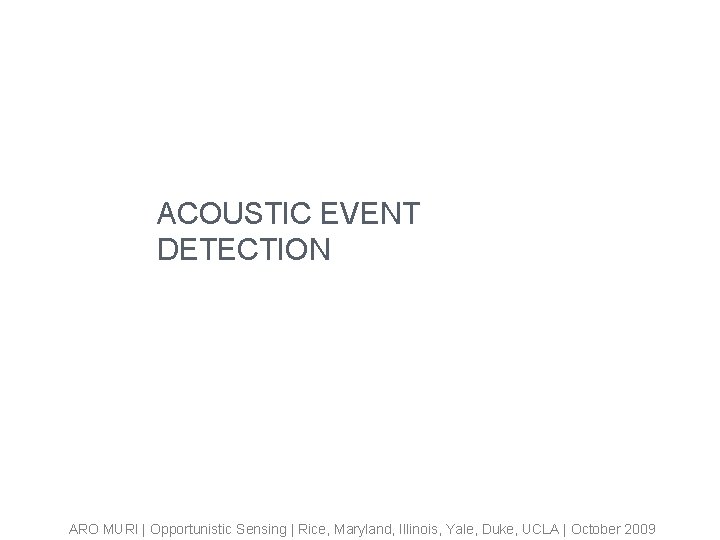
ACOUSTIC EVENT DETECTION ARO MURI | Opportunistic Sensing | Rice, Maryland, Illinois, Yale, Duke, UCLA | October 2009
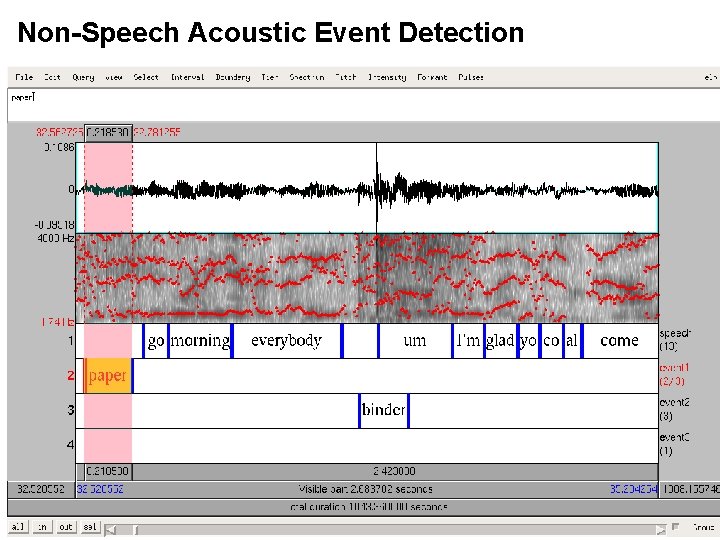
Non-Speech Acoustic Event Detection ARO MURI | Opportunistic Sensing | Rice, Maryland, Illinois, Yale, Duke, UCLA | October 2009
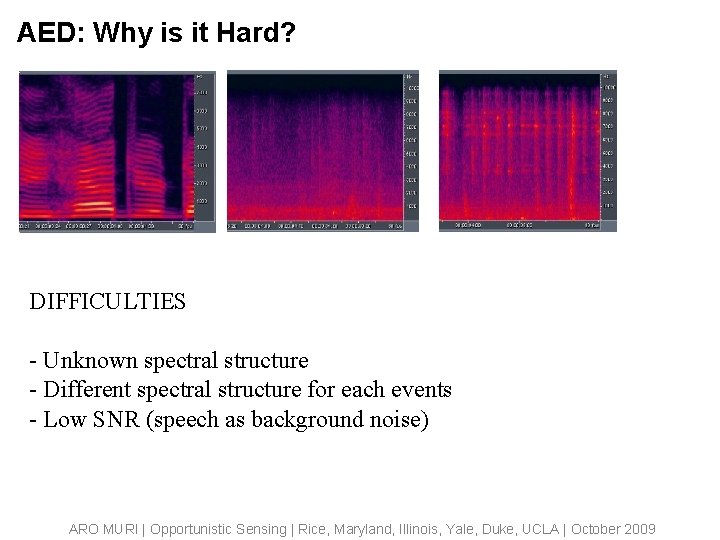
AED: Why is it Hard? DIFFICULTIES - Unknown spectral structure - Different spectral structure for each events - Low SNR (speech as background noise) ARO MURI | Opportunistic Sensing | Rice, Maryland, Illinois, Yale, Duke, UCLA | October 2009
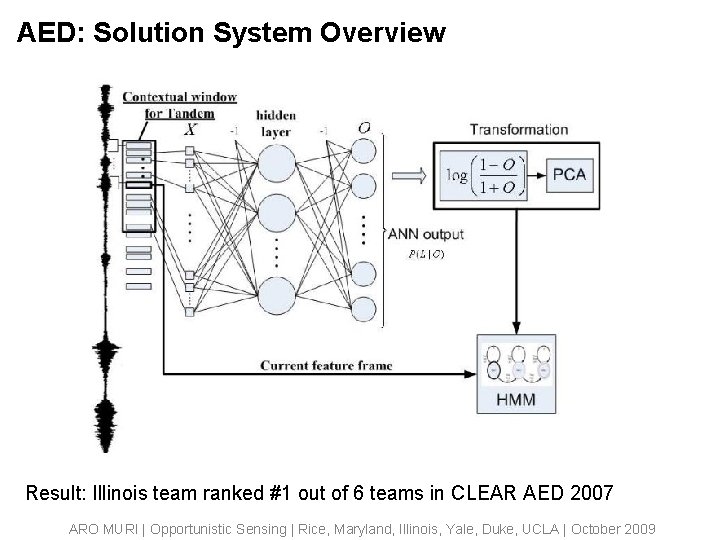
AED: Solution System Overview Result: Illinois team ranked #1 out of 6 teams in CLEAR AED 2007 ARO MURI | Opportunistic Sensing | Rice, Maryland, Illinois, Yale, Duke, UCLA | October 2009
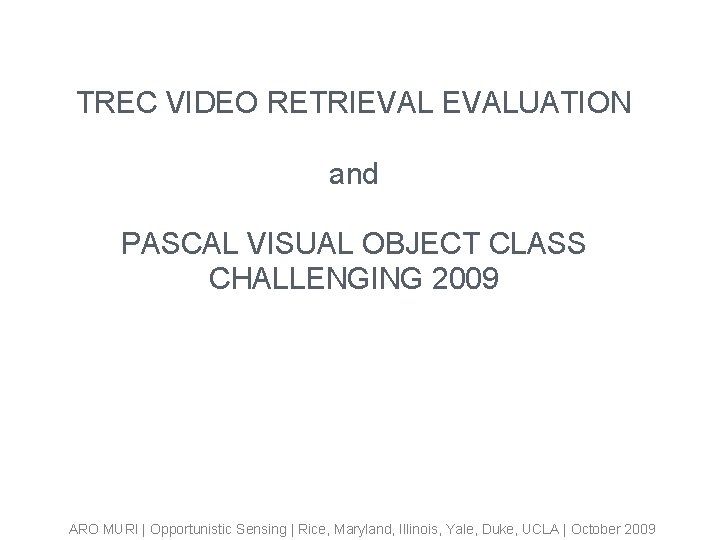
TREC VIDEO RETRIEVALUATION and PASCAL VISUAL OBJECT CLASS CHALLENGING 2009 ARO MURI | Opportunistic Sensing | Rice, Maryland, Illinois, Yale, Duke, UCLA | October 2009
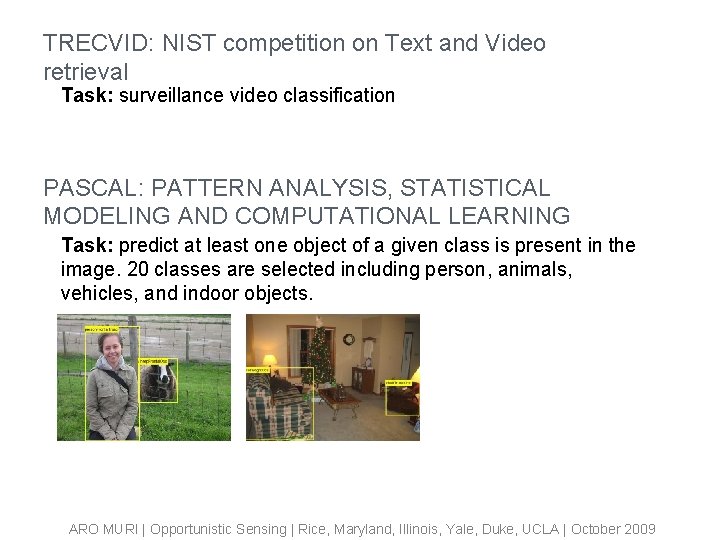
TRECVID: NIST competition on Text and Video retrieval Task: surveillance video classification PASCAL: PATTERN ANALYSIS, STATISTICAL MODELING AND COMPUTATIONAL LEARNING Task: predict at least one object of a given class is present in the image. 20 classes are selected including person, animals, vehicles, and indoor objects. ARO MURI | Opportunistic Sensing | Rice, Maryland, Illinois, Yale, Duke, UCLA | October 2009
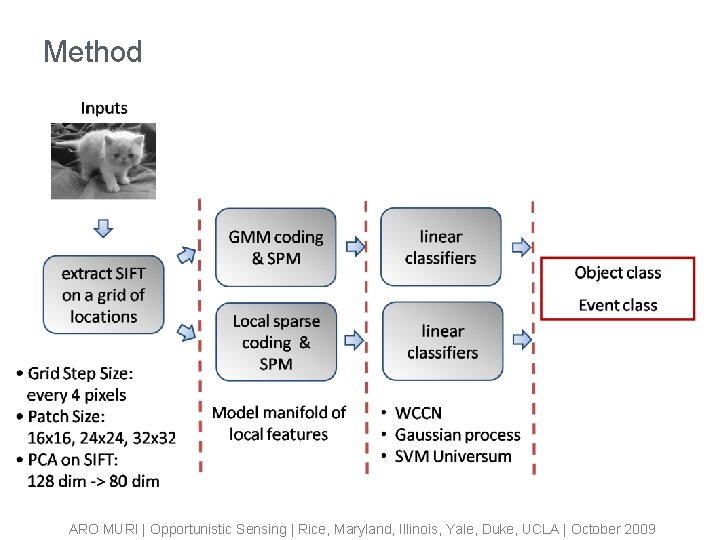
Method ARO MURI | Opportunistic Sensing | Rice, Maryland, Illinois, Yale, Duke, UCLA | October 2009
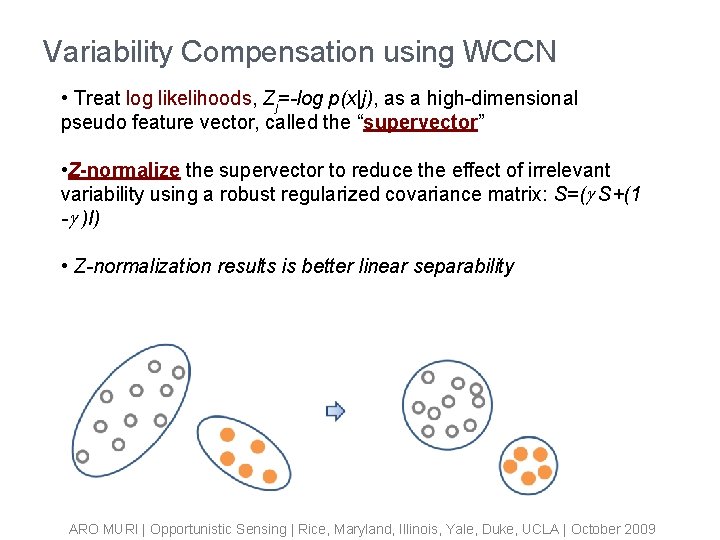
Variability Compensation using WCCN • Treat log likelihoods, Zj=-log p(x|j), as a high-dimensional pseudo feature vector, called the “supervector” • Z-normalize the supervector to reduce the effect of irrelevant variability using a robust regularized covariance matrix: S=(g S+(1 -g )I) • Z-normalization results is better linear separability ARO MURI | Opportunistic Sensing | Rice, Maryland, Illinois, Yale, Duke, UCLA | October 2009
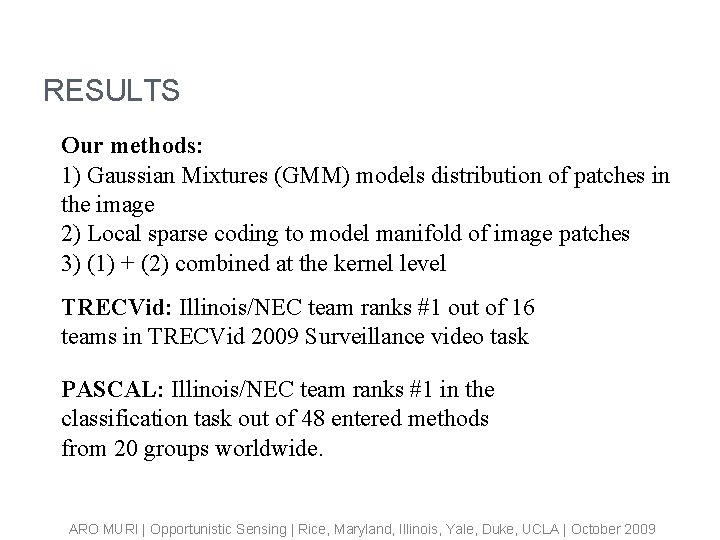
RESULTS Our methods: 1) Gaussian Mixtures (GMM) models distribution of patches in the image 2) Local sparse coding to model manifold of image patches 3) (1) + (2) combined at the kernel level TRECVid: Illinois/NEC team ranks #1 out of 16 teams in TRECVid 2009 Surveillance video task PASCAL: Illinois/NEC team ranks #1 in the classification task out of 48 entered methods from 20 groups worldwide. ARO MURI | Opportunistic Sensing | Rice, Maryland, Illinois, Yale, Duke, UCLA | October 2009
Independent or dependent
Chaernobyl
Independent event vs dependent event
Near miss adverse event sentinel event
Swot event management
Simple event and compound event
Bridge breaks in central java the text tells us about
Intake manifold design theory
Steam tracing
Split manifold plug-in type
A typical map compares the vacuum in the intake manifold to
Tri tech medical
Manifold hypothesis
D mpfi system uses
One dimensional manifold
Manifold ug
Simple classification and manifold classification