Analyzing p K data OverComplete Measurements and partialwave
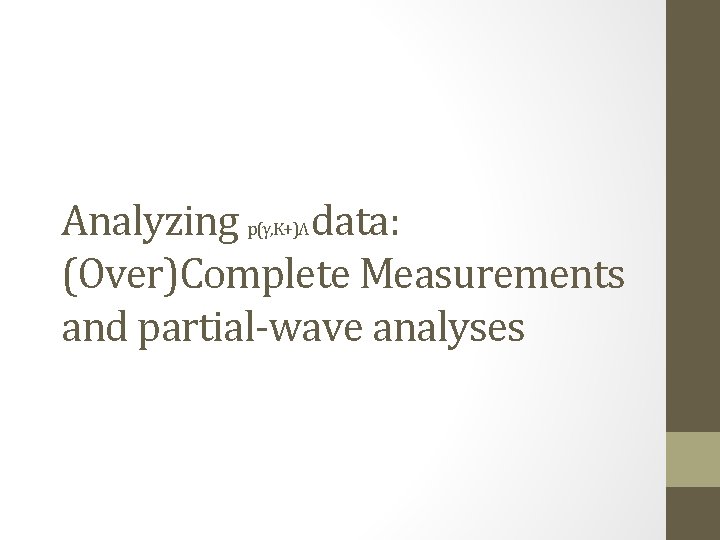
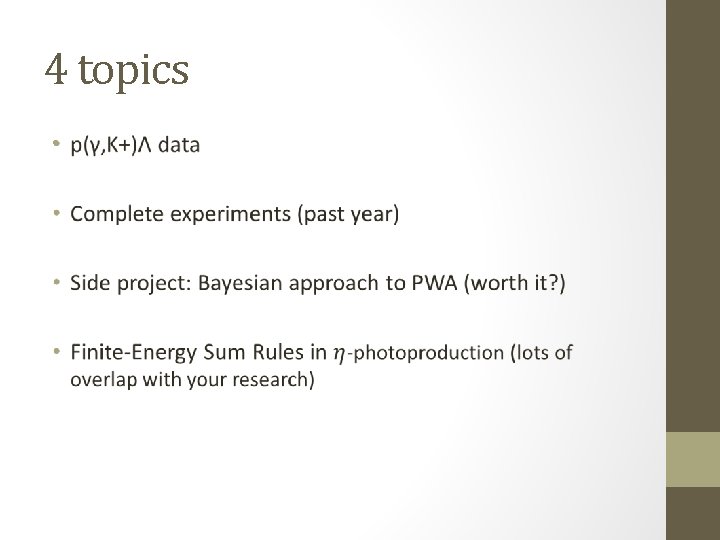
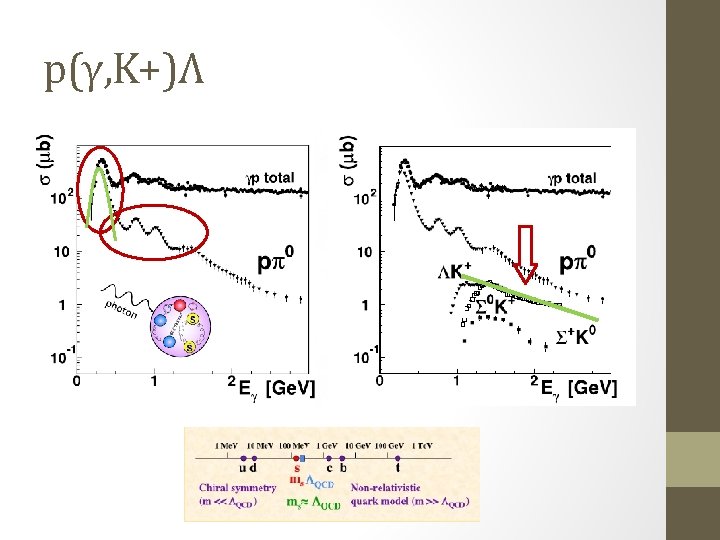
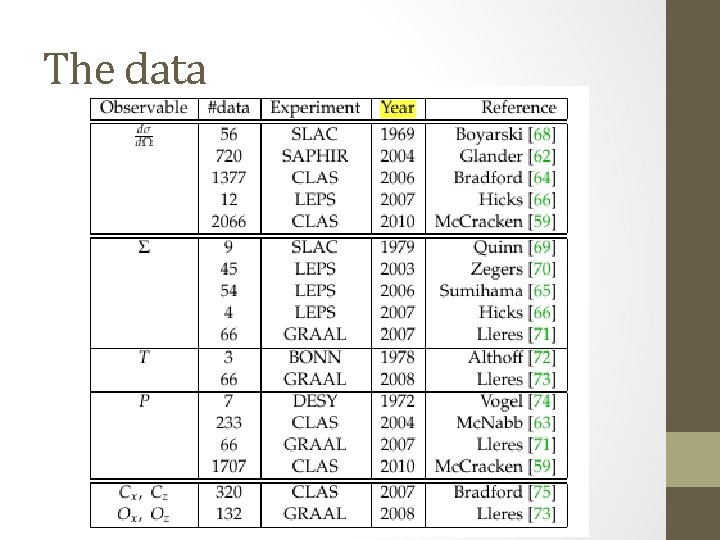
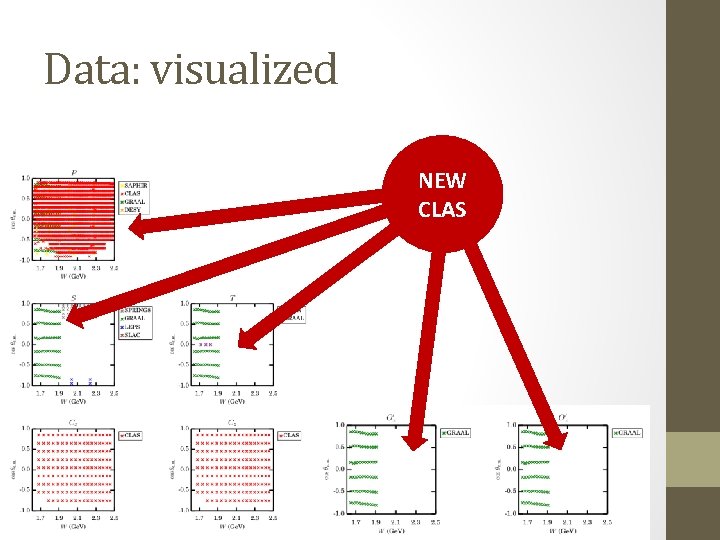
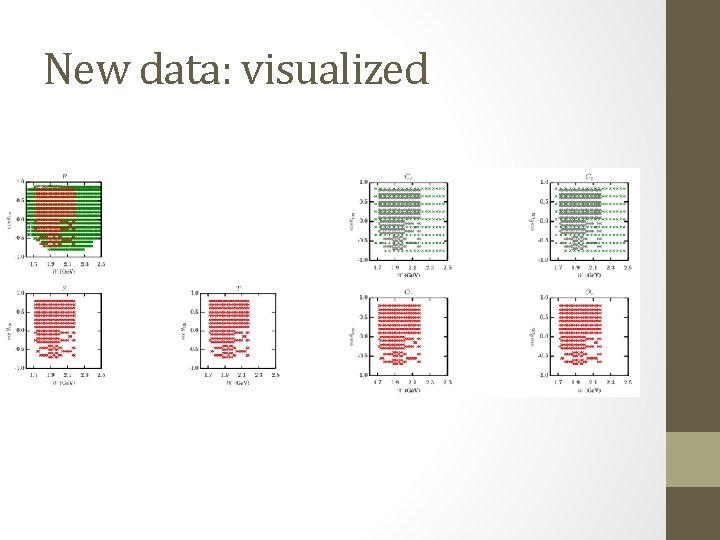
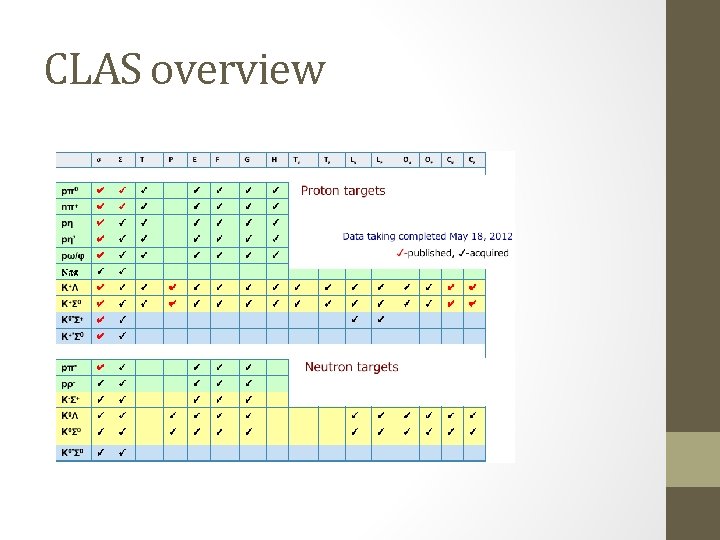
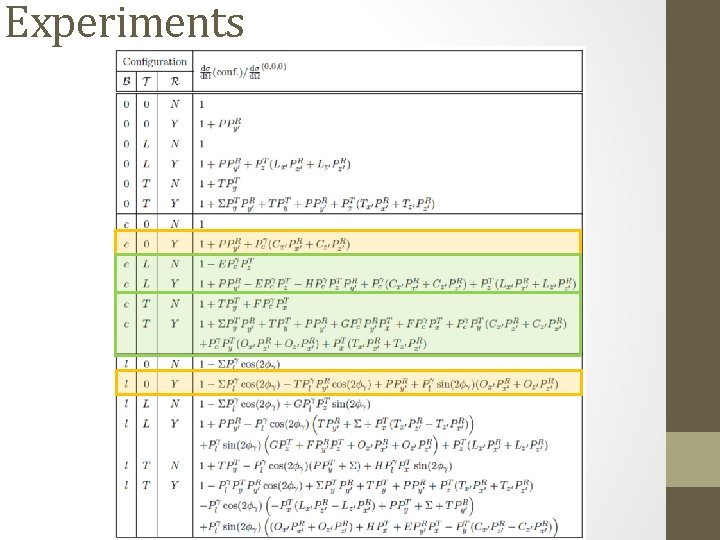
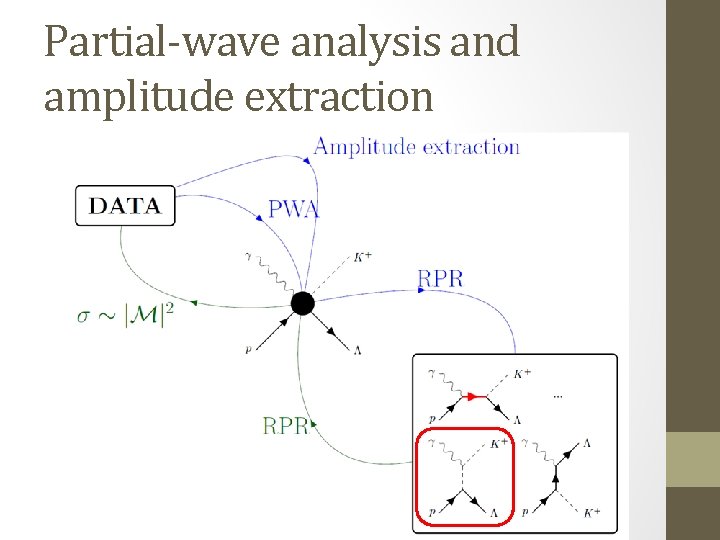
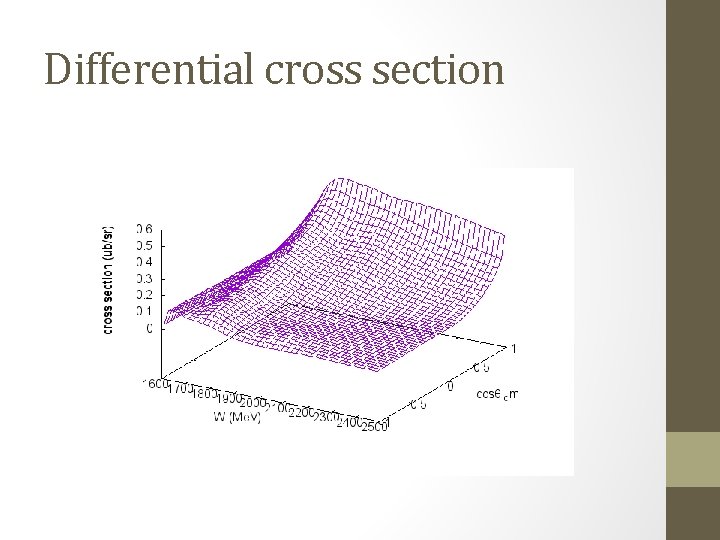
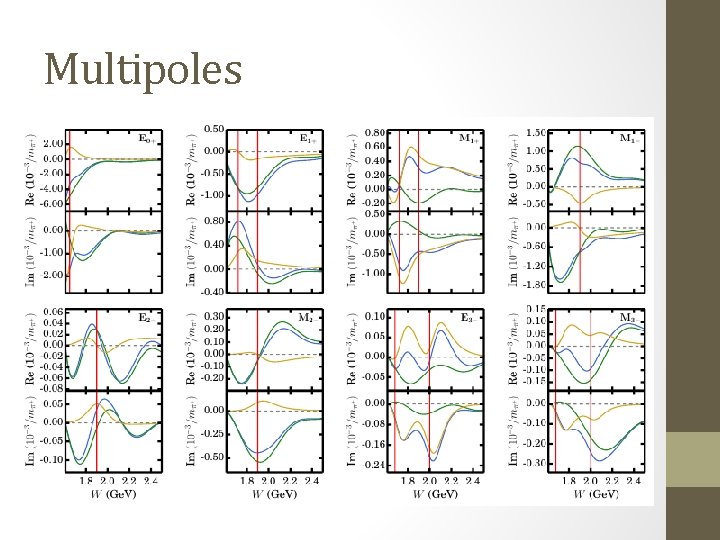
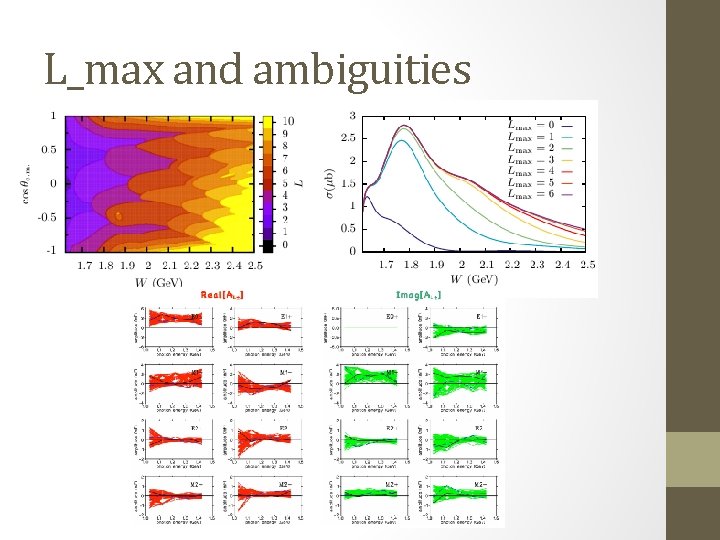
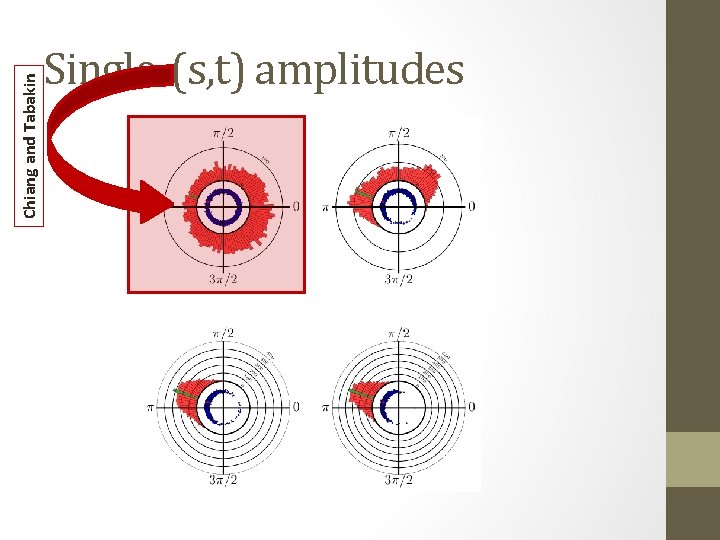
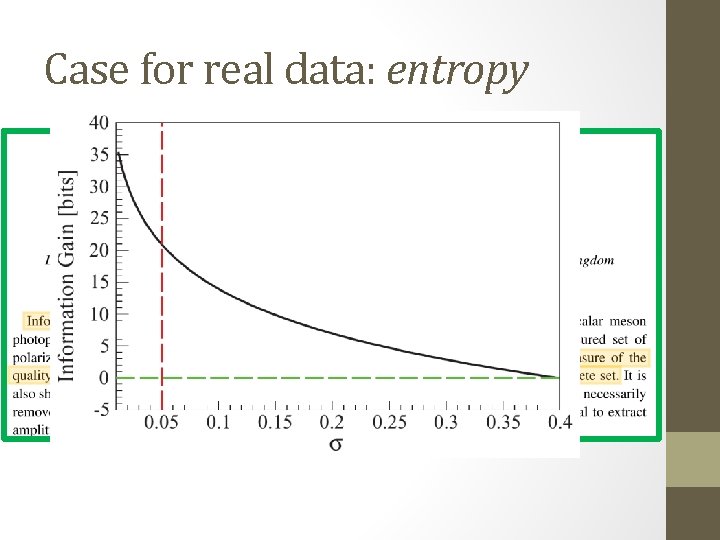
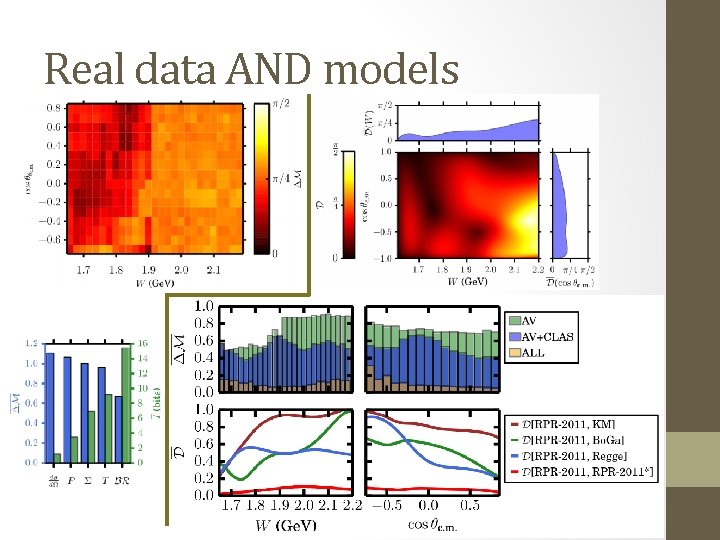
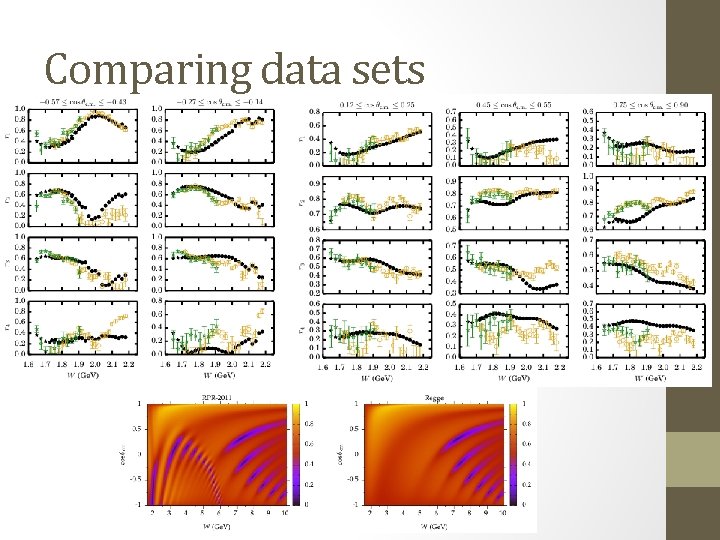
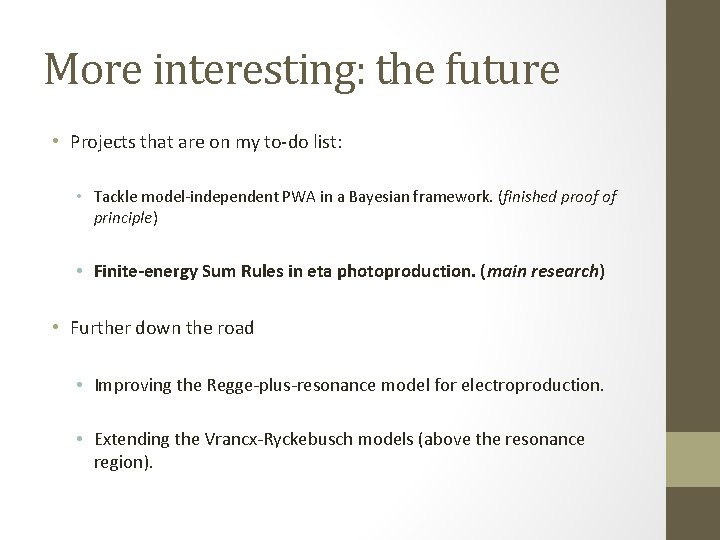
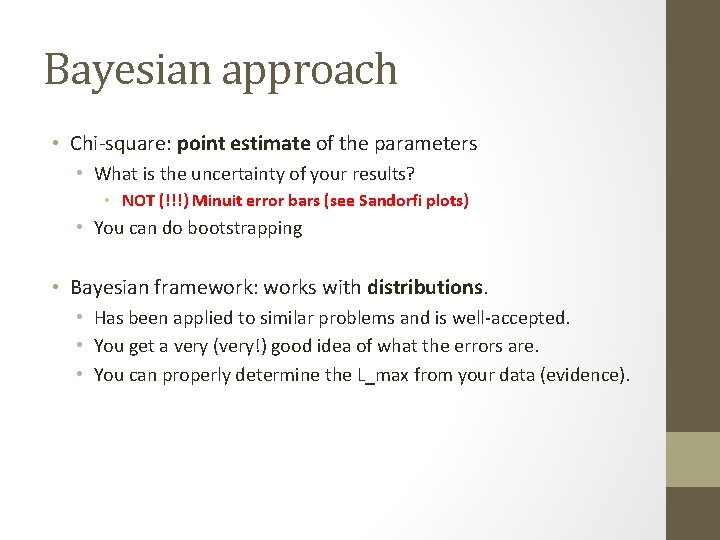
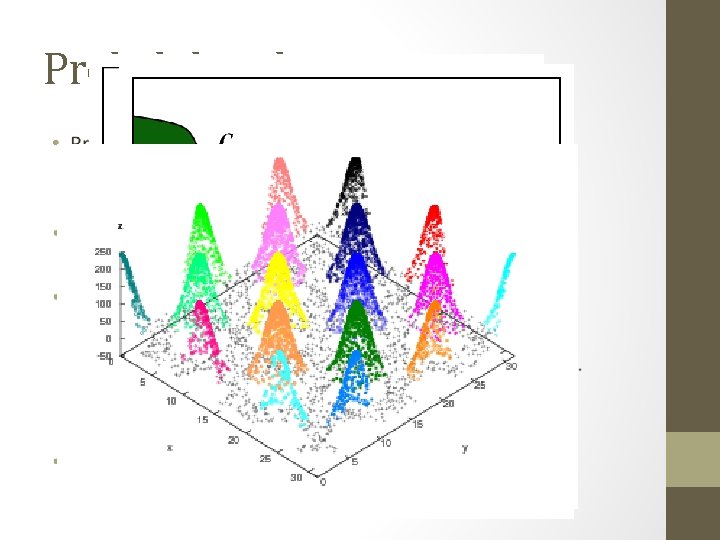
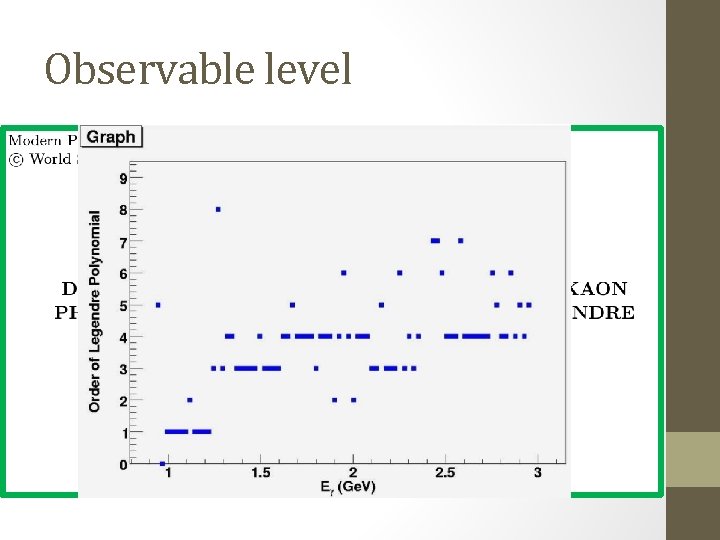
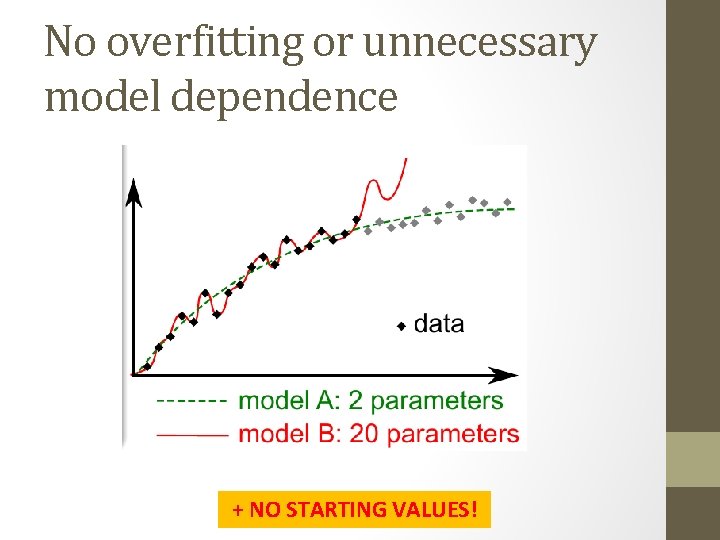
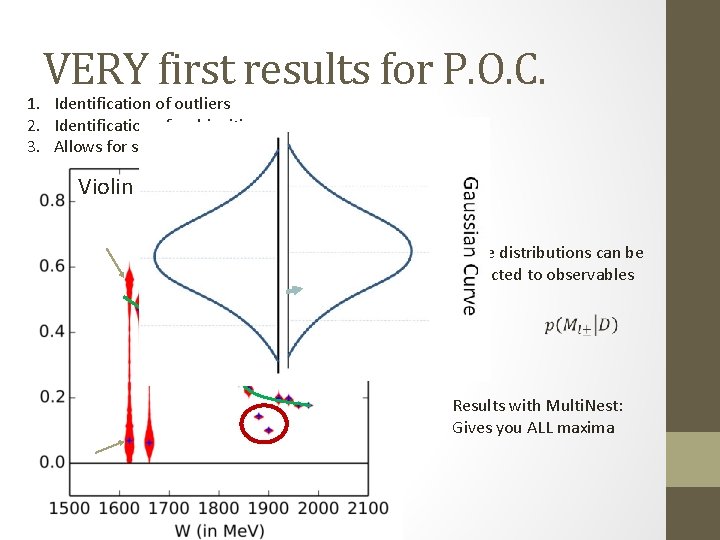
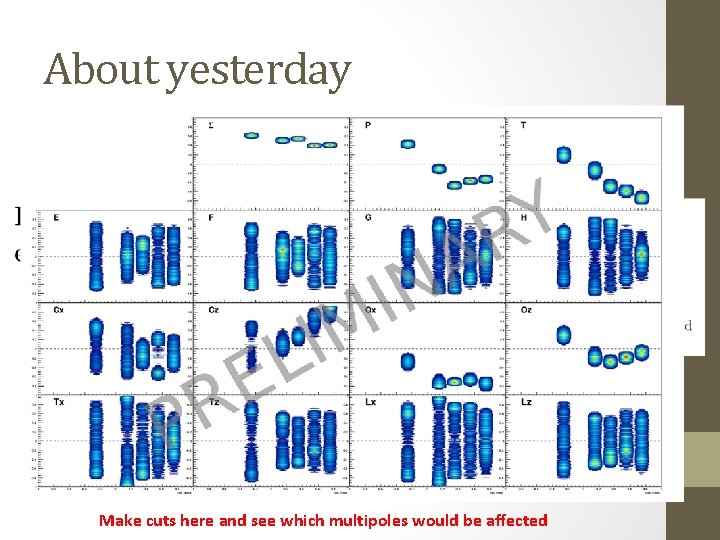
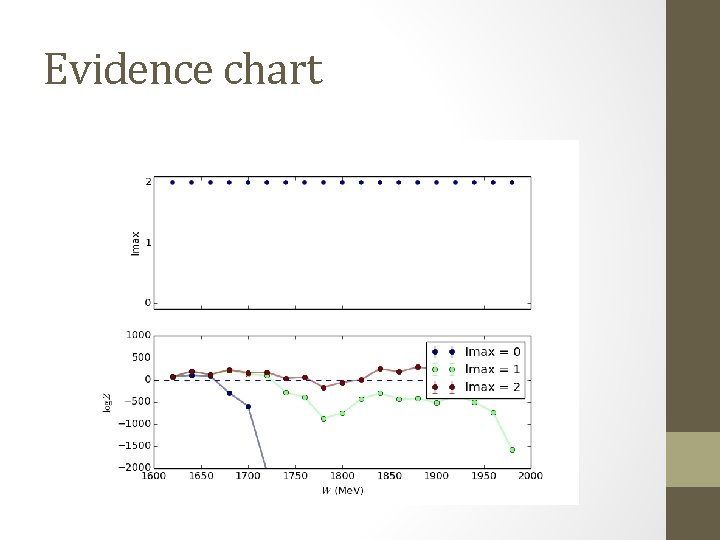
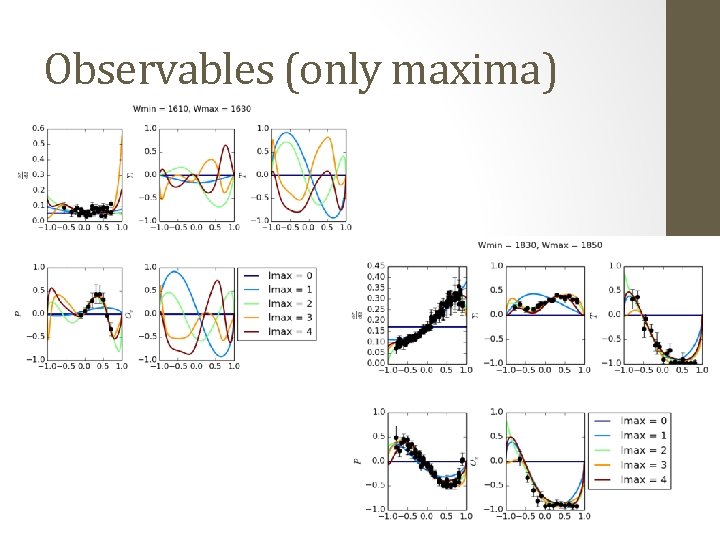
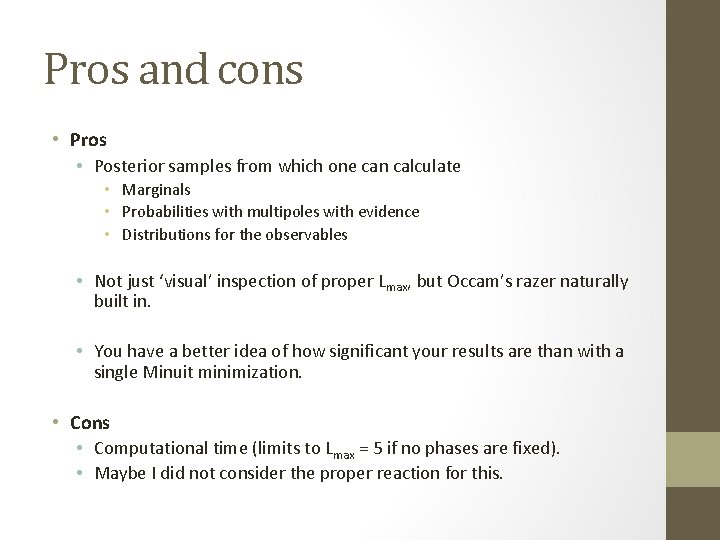
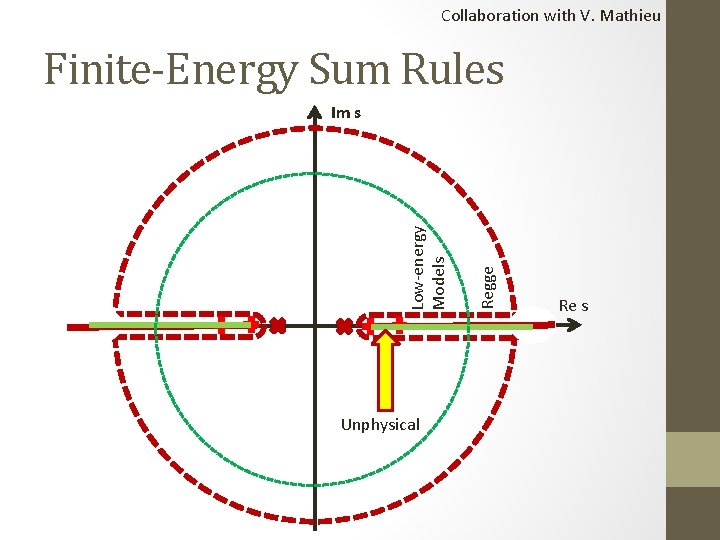
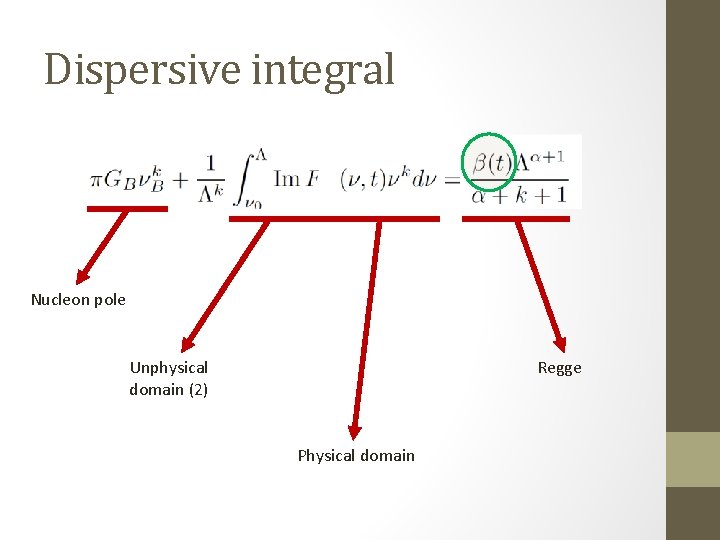
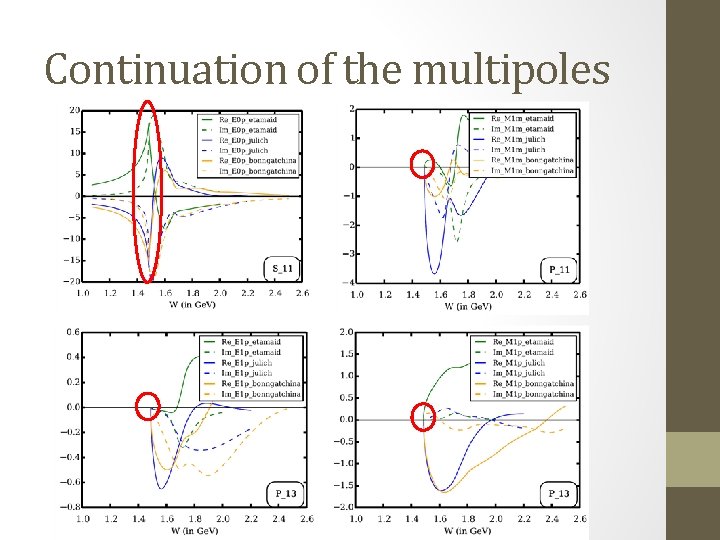
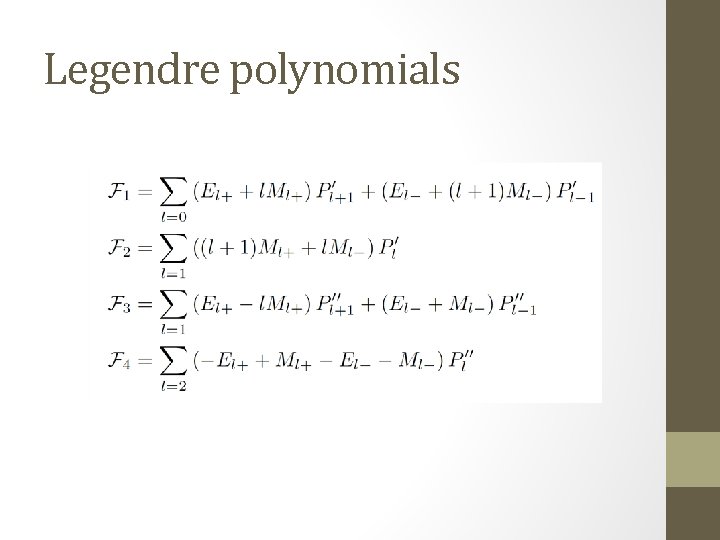
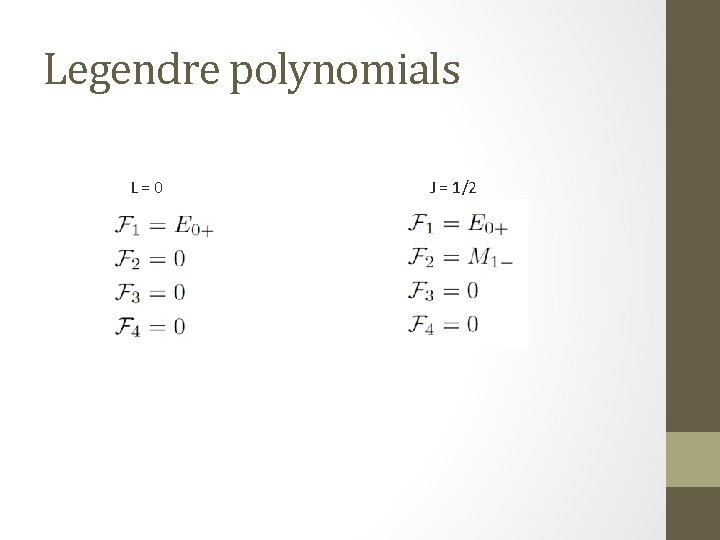
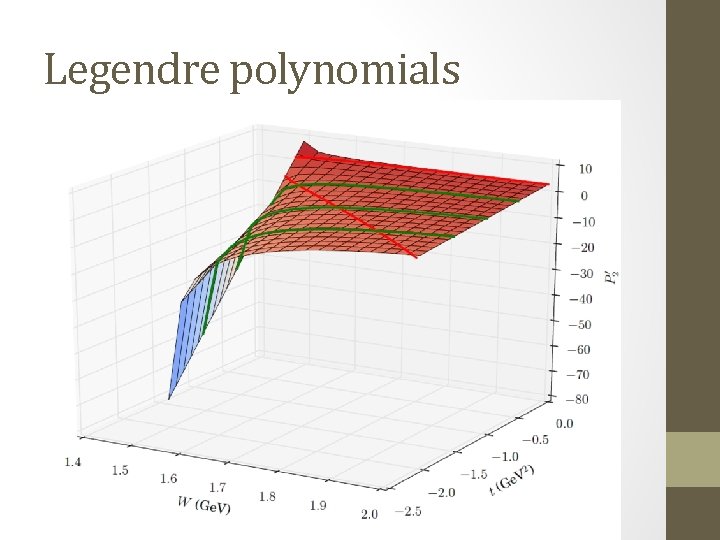
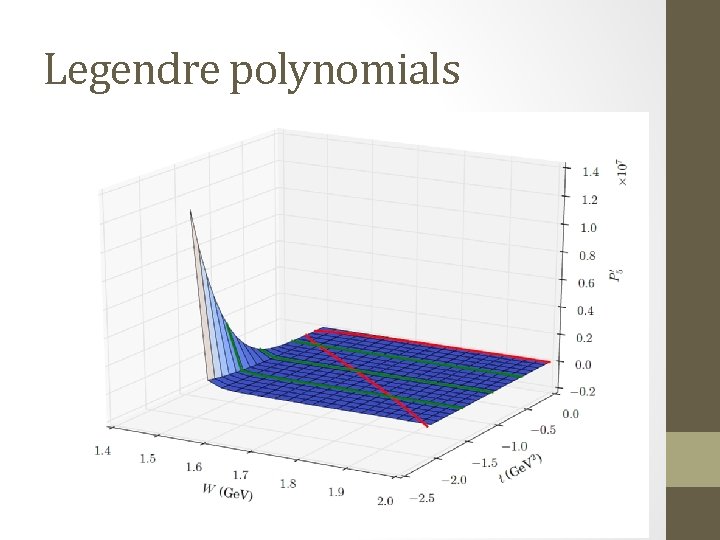
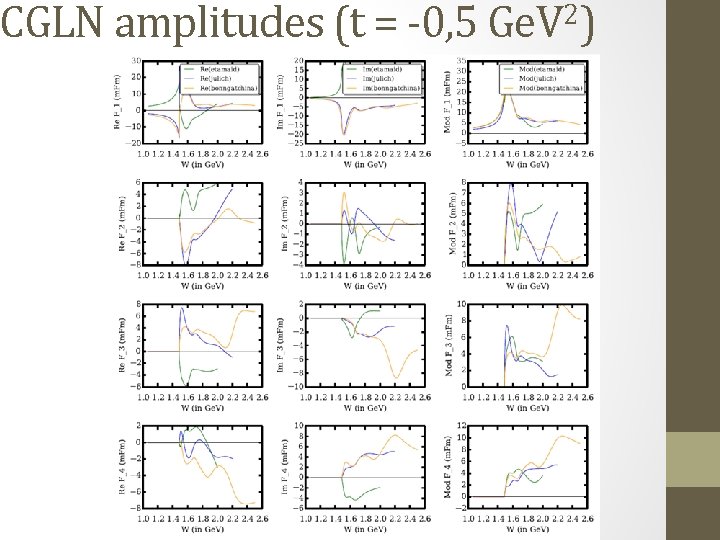
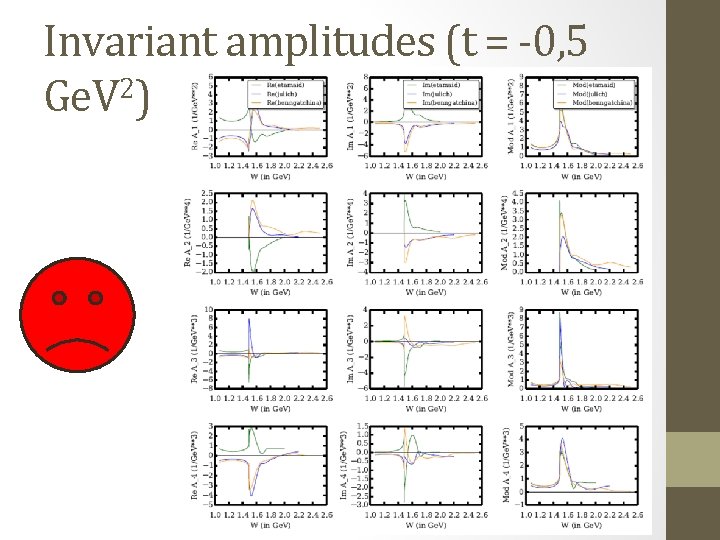
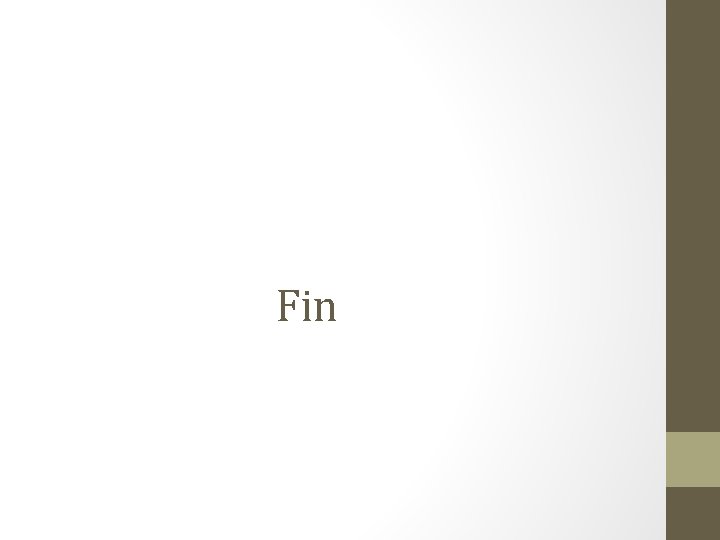
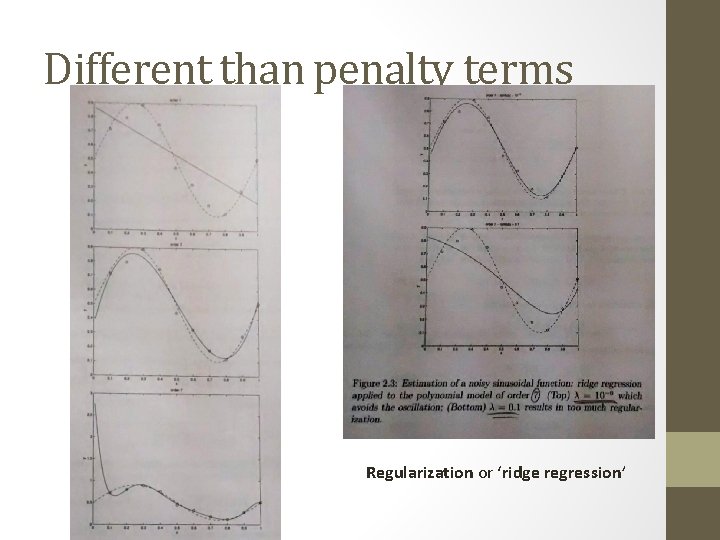
- Slides: 37
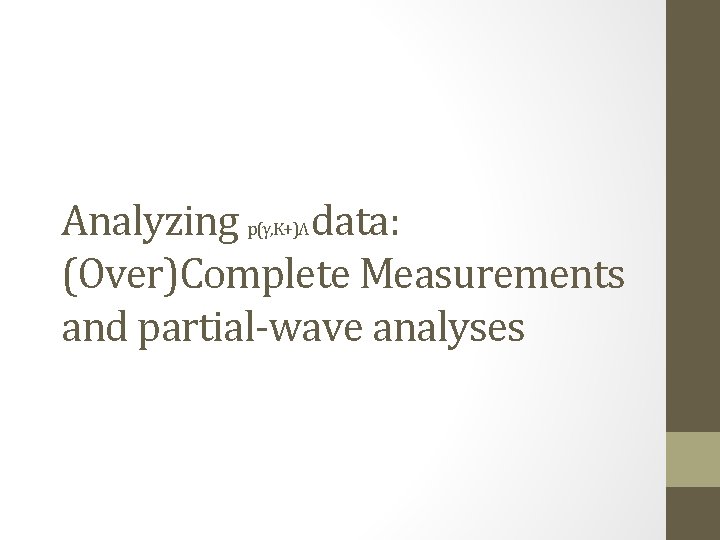
Analyzing p(γ, K+)Λ data: (Over)Complete Measurements and partial-wave analyses
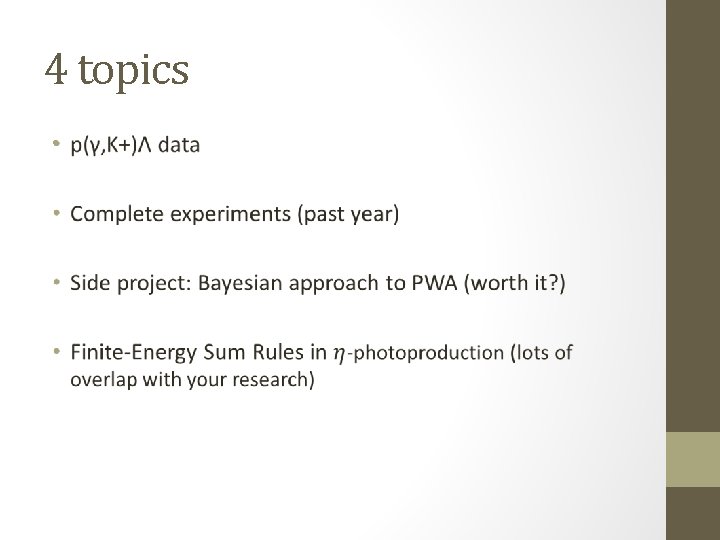
4 topics •
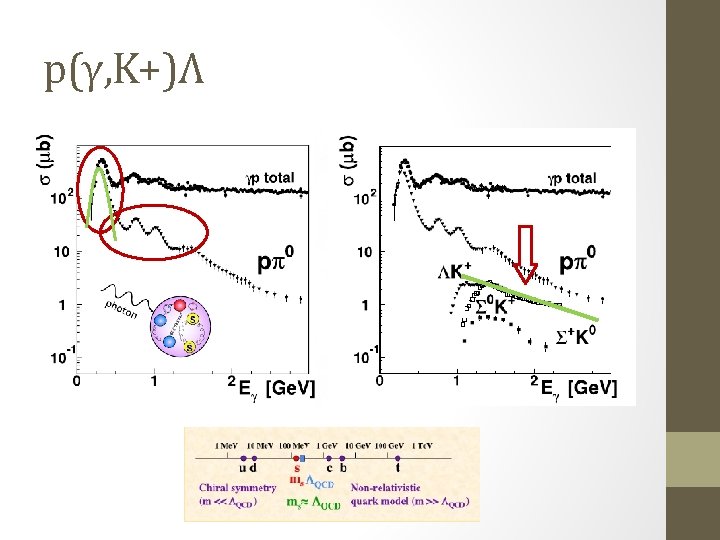
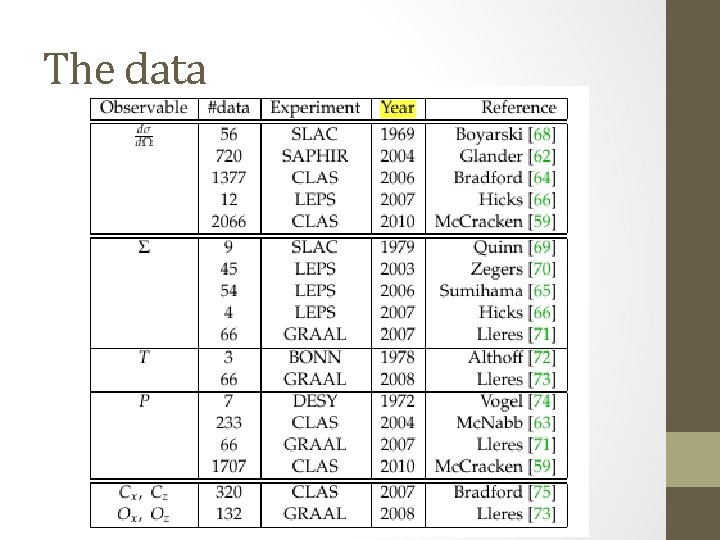
The data
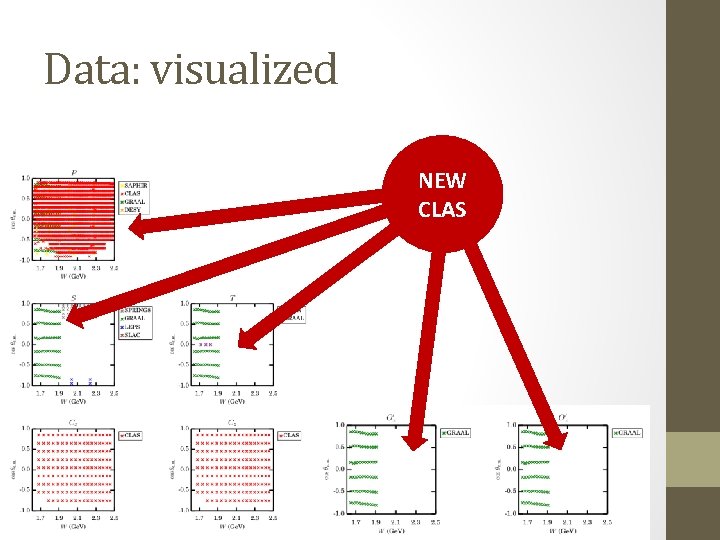
Data: visualized NEW CLAS
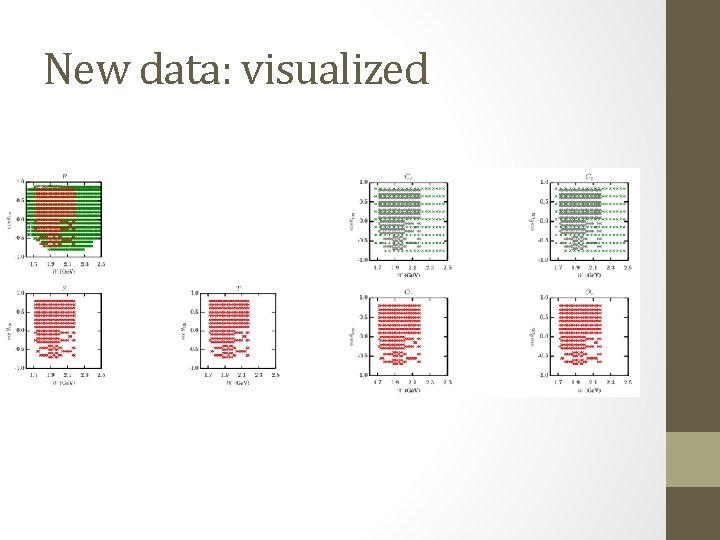
New data: visualized
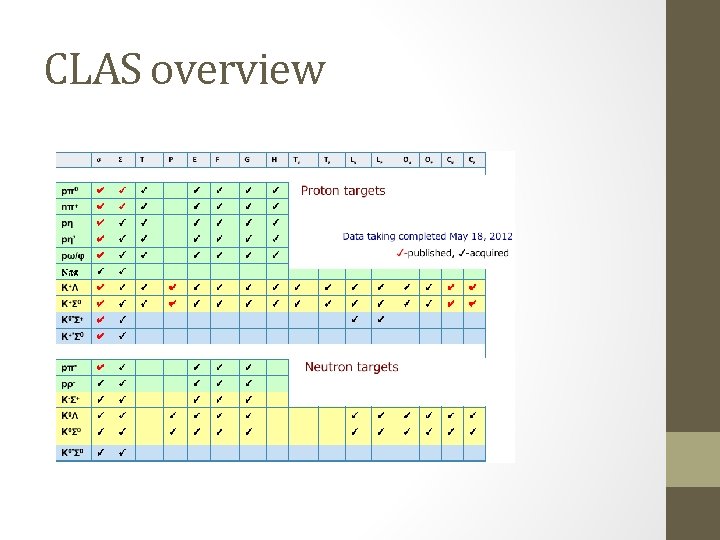
CLAS overview
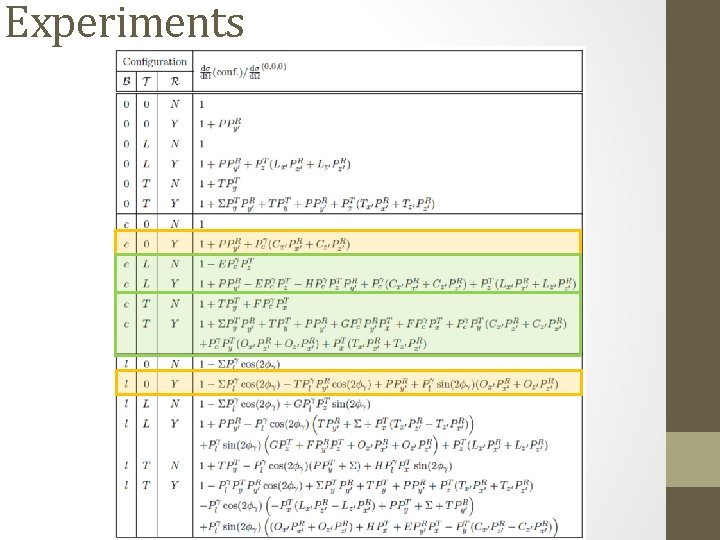
Experiments
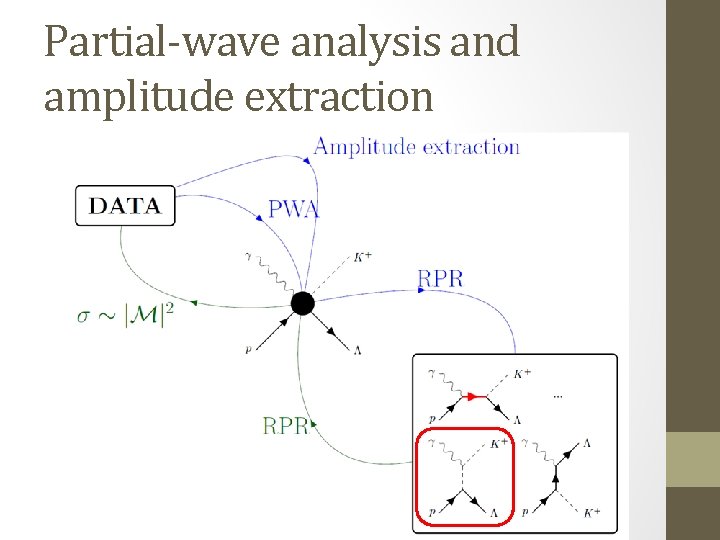
Partial-wave analysis and amplitude extraction
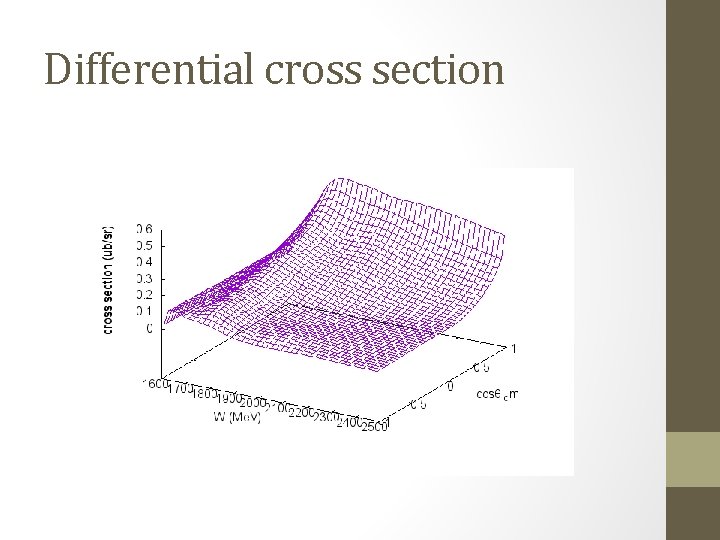
Differential cross section
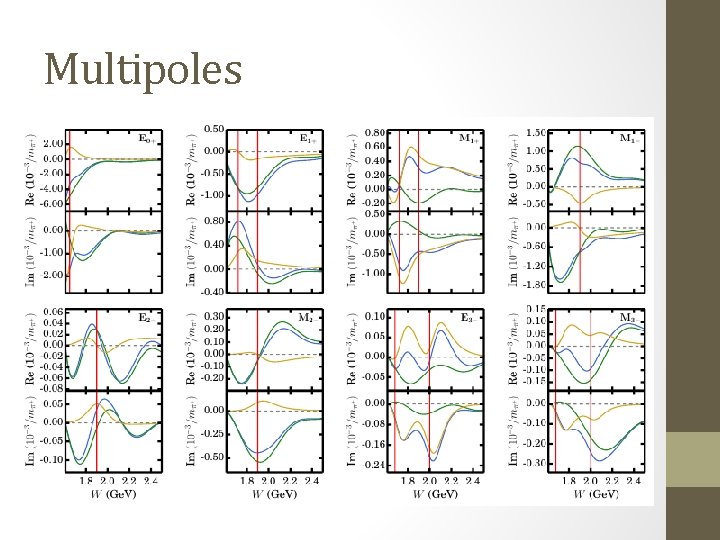
Multipoles
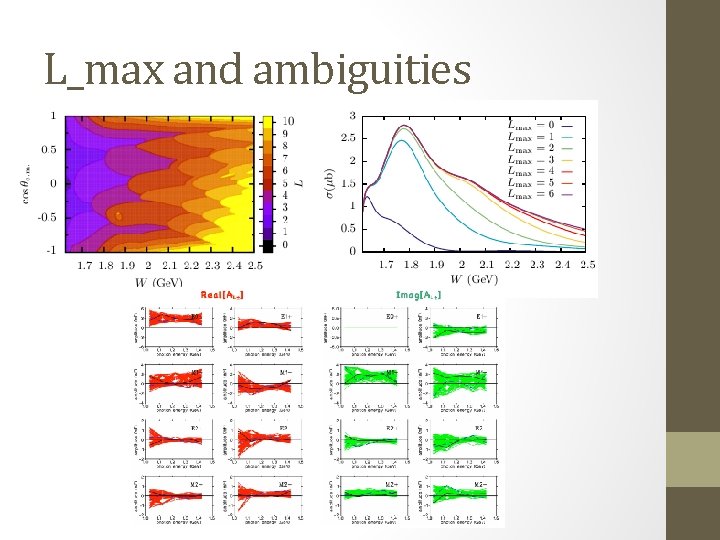
L_max and ambiguities
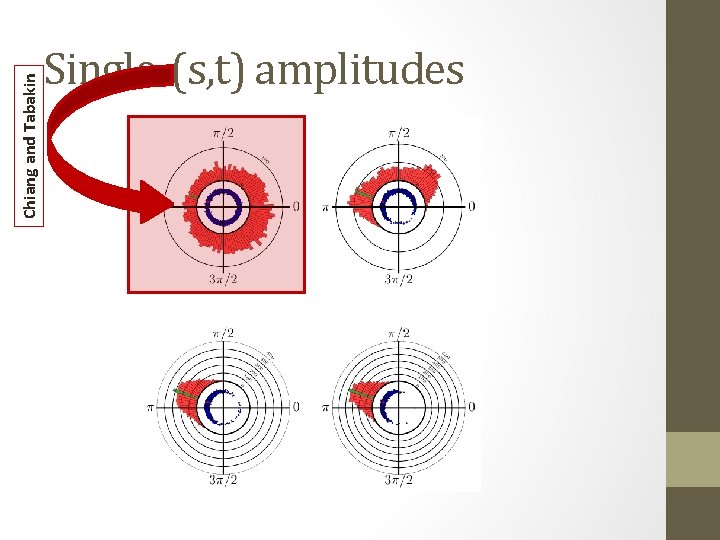
Chiang and Tabakin Single-(s, t) amplitudes
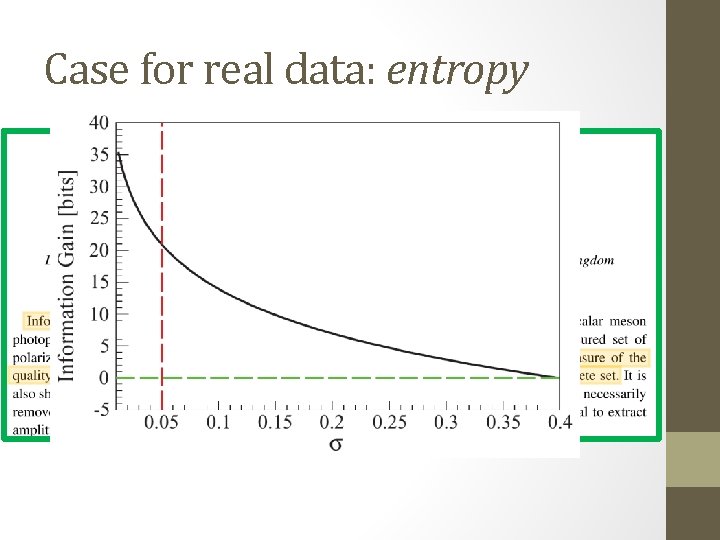
Case for real data: entropy
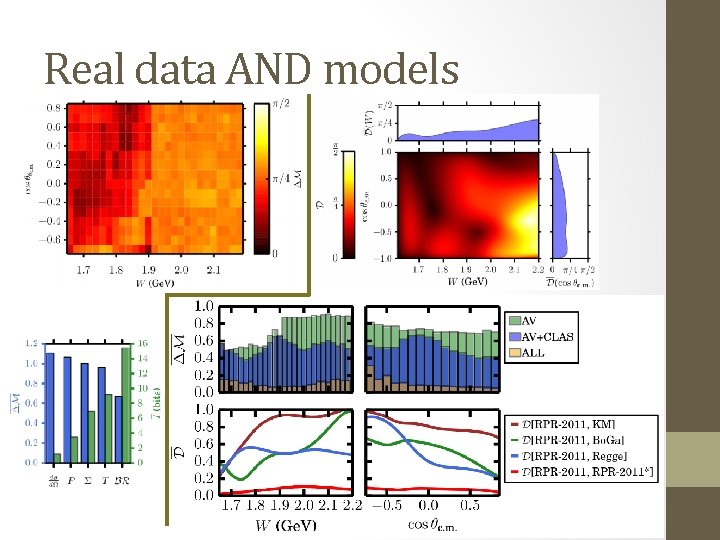
Real data AND models
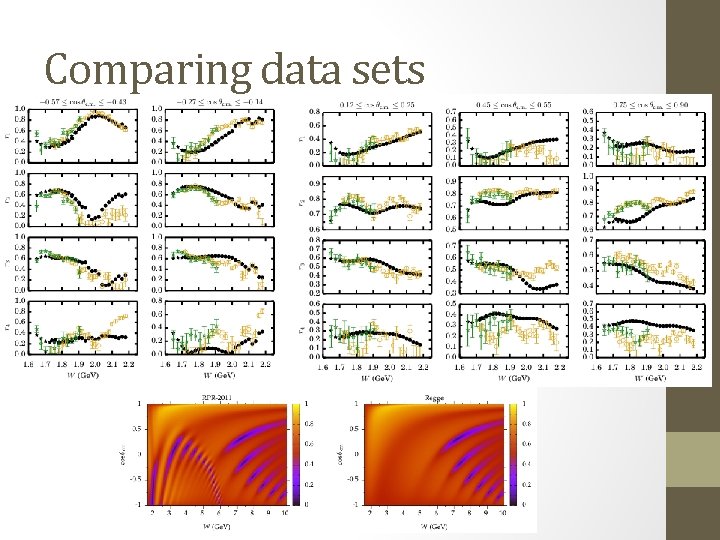
Comparing data sets
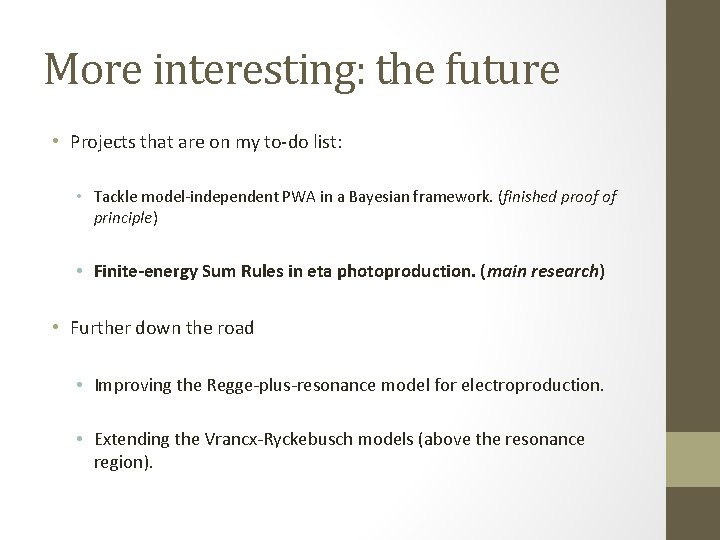
More interesting: the future • Projects that are on my to-do list: • Tackle model-independent PWA in a Bayesian framework. (finished proof of principle) • Finite-energy Sum Rules in eta photoproduction. (main research) • Further down the road • Improving the Regge-plus-resonance model for electroproduction. • Extending the Vrancx-Ryckebusch models (above the resonance region).
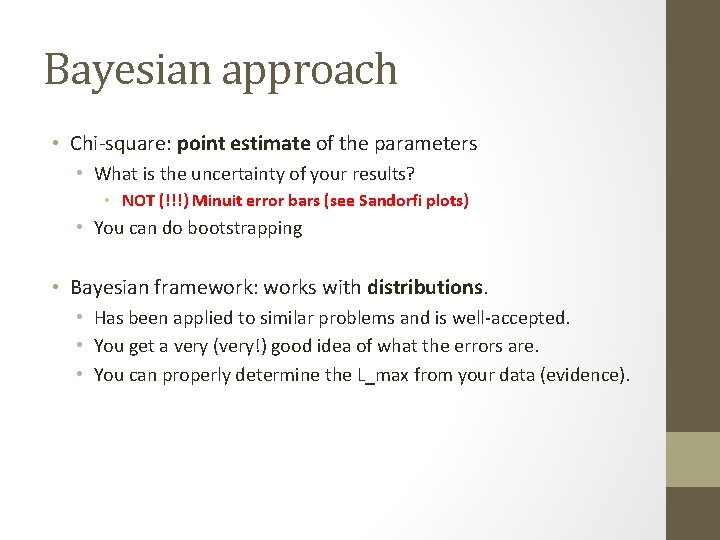
Bayesian approach • Chi-square: point estimate of the parameters • What is the uncertainty of your results? • NOT (!!!) Minuit error bars (see Sandorfi plots) • You can do bootstrapping • Bayesian framework: works with distributions. • Has been applied to similar problems and is well-accepted. • You get a very (very!) good idea of what the errors are. • You can properly determine the L_max from your data (evidence).
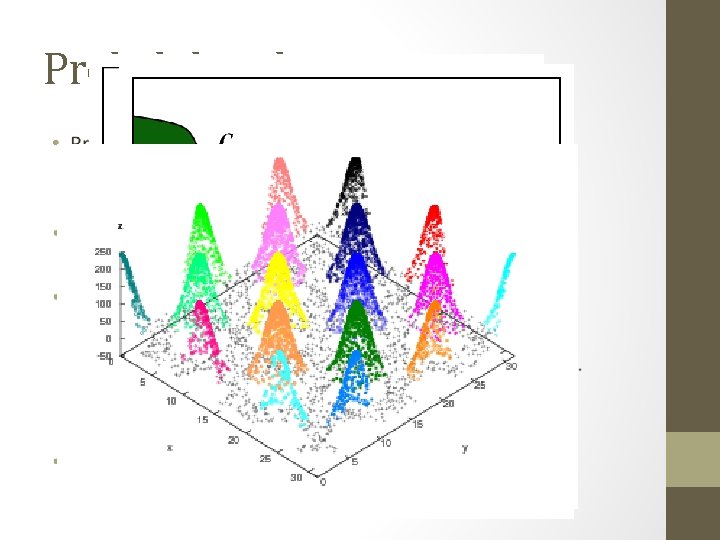
Probability theory •
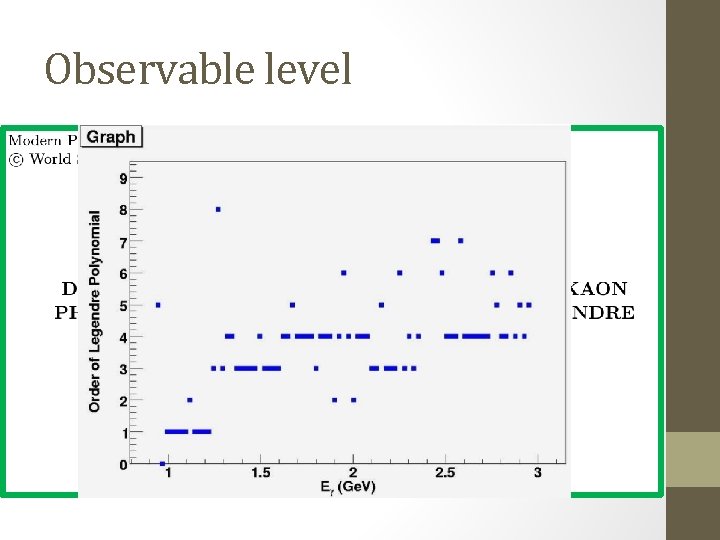
Observable level
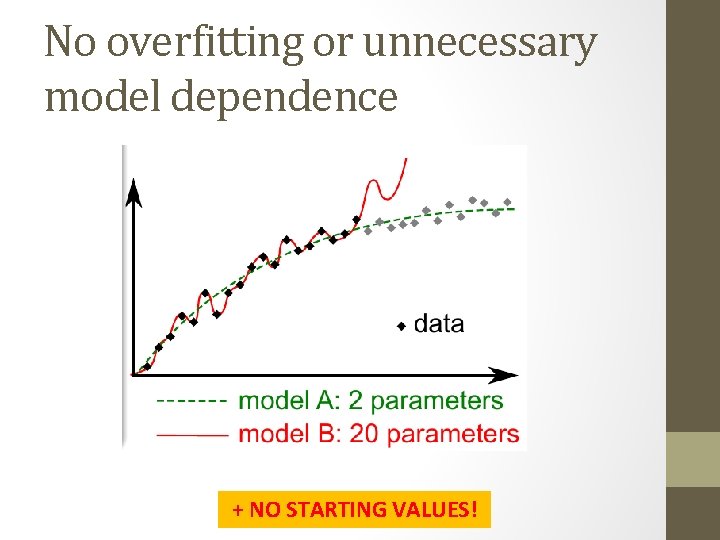
No overfitting or unnecessary model dependence + NO STARTING VALUES!
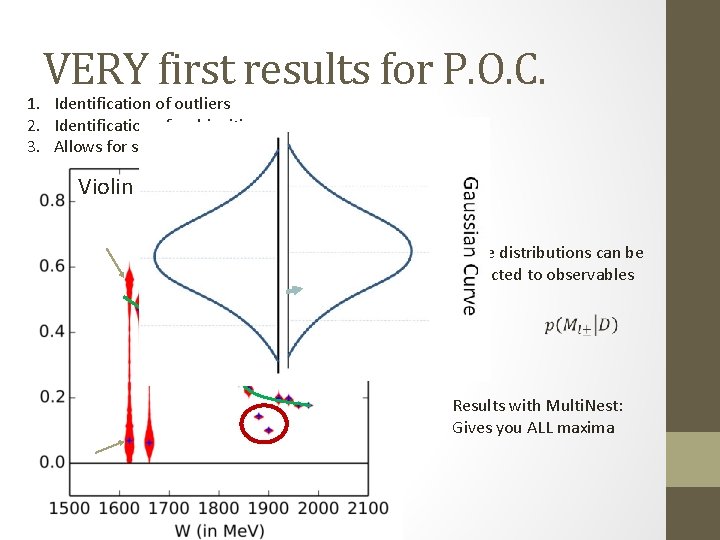
VERY first results for P. O. C. 1. Identification of outliers 2. Identification of ambiguities 3. Allows for smooth connections (continuous distributions!) Violin plot for Lmax = 1, Re E 0+ These distributions can be projected to observables Results with Multi. Nest: Gives you ALL maxima
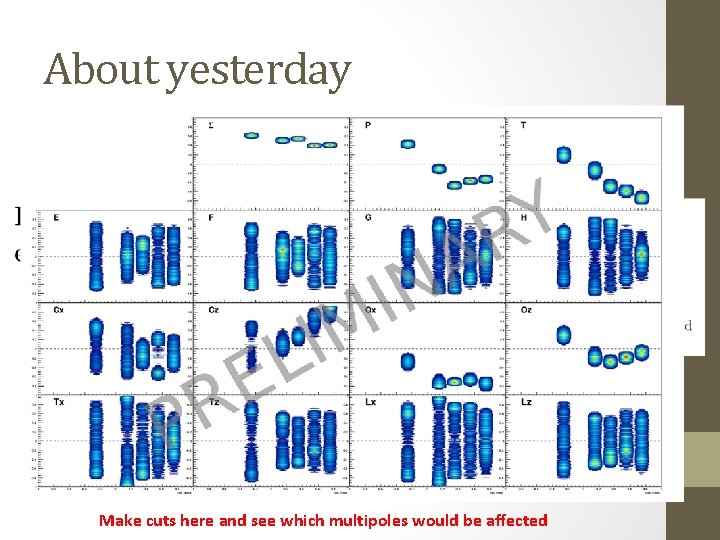
About yesterday Make cuts here and see which multipoles would be affected
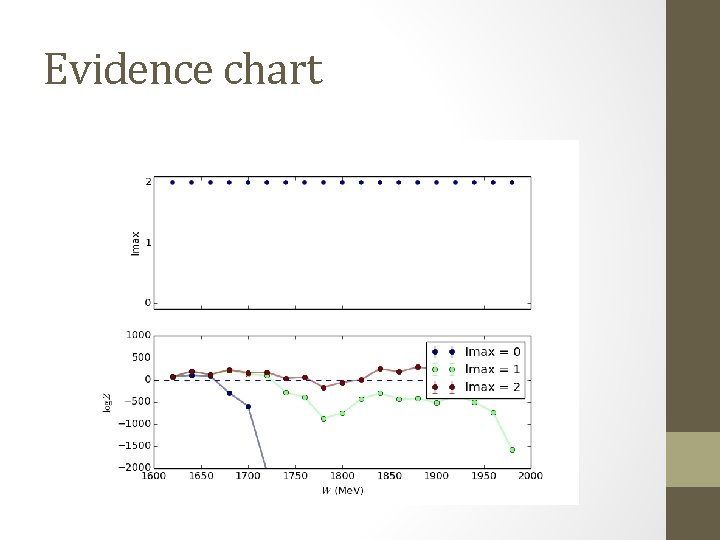
Evidence chart
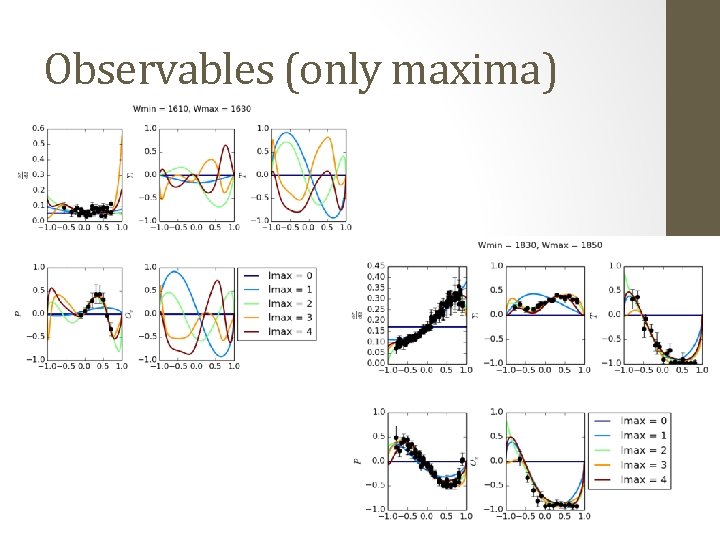
Observables (only maxima)
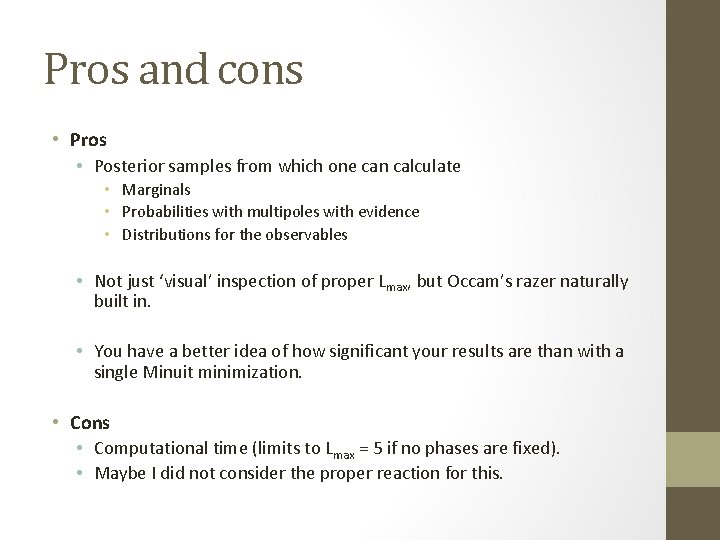
Pros and cons • Pros • Posterior samples from which one can calculate • Marginals • Probabilities with multipoles with evidence • Distributions for the observables • Not just ‘visual’ inspection of proper Lmax, but Occam’s razer naturally built in. • You have a better idea of how significant your results are than with a single Minuit minimization. • Cons • Computational time (limits to Lmax = 5 if no phases are fixed). • Maybe I did not consider the proper reaction for this.
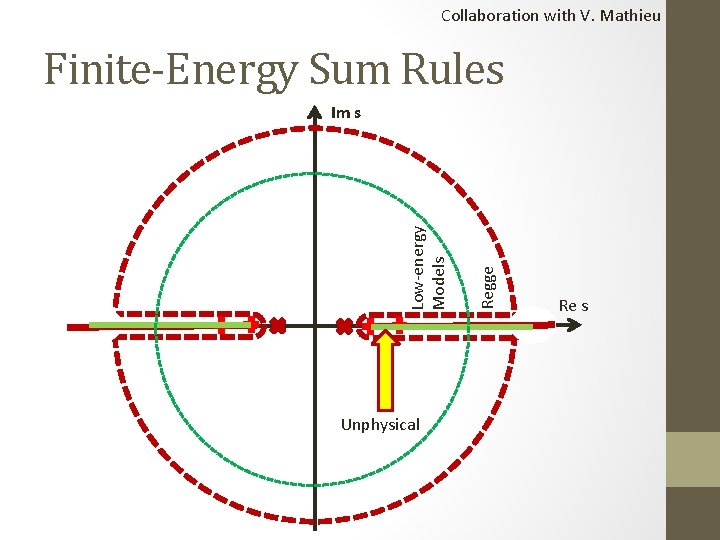
Collaboration with V. Mathieu Finite-Energy Sum Rules Unphysical Regge Low-energy Models Im s Re s
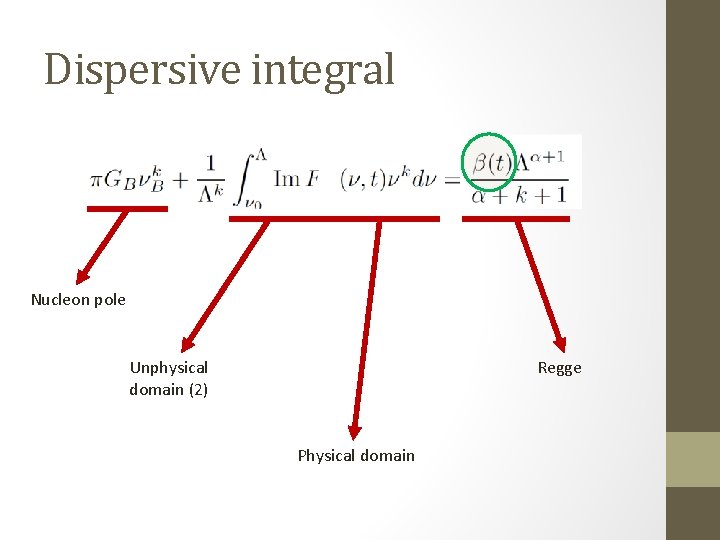
Dispersive integral Nucleon pole Regge Unphysical domain (2) Physical domain
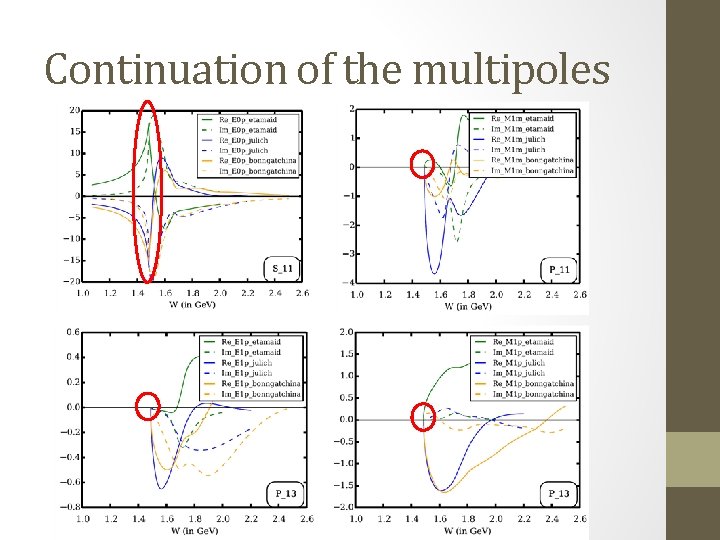
Continuation of the multipoles
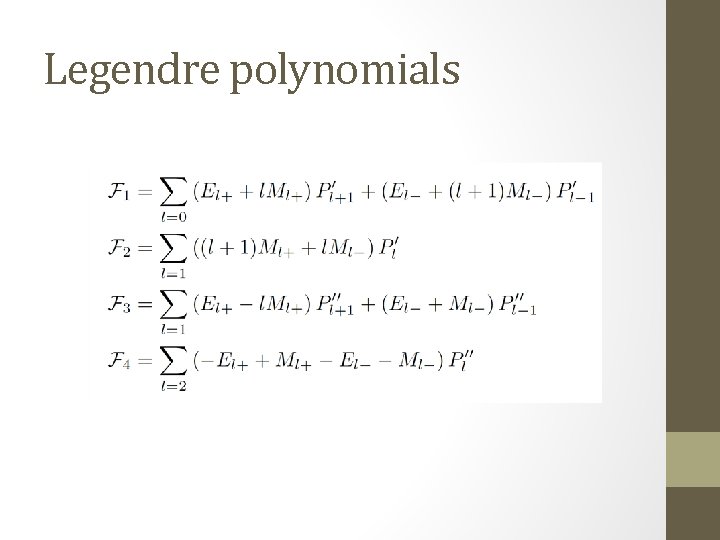
Legendre polynomials
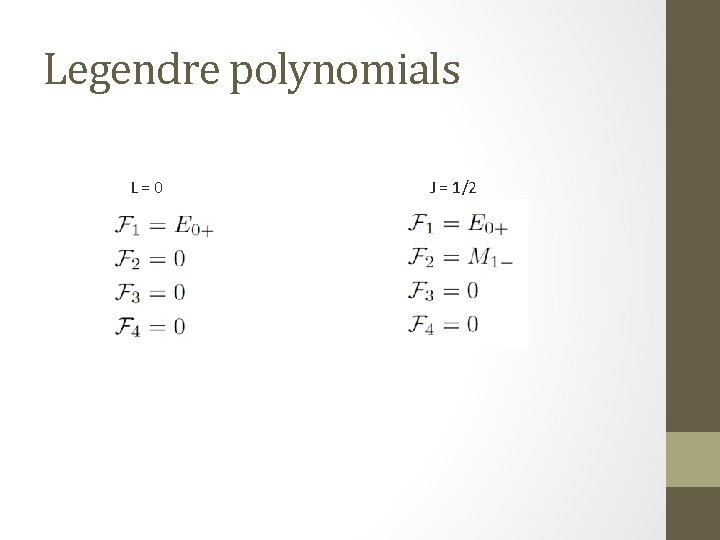
Legendre polynomials L=0 J = 1/2
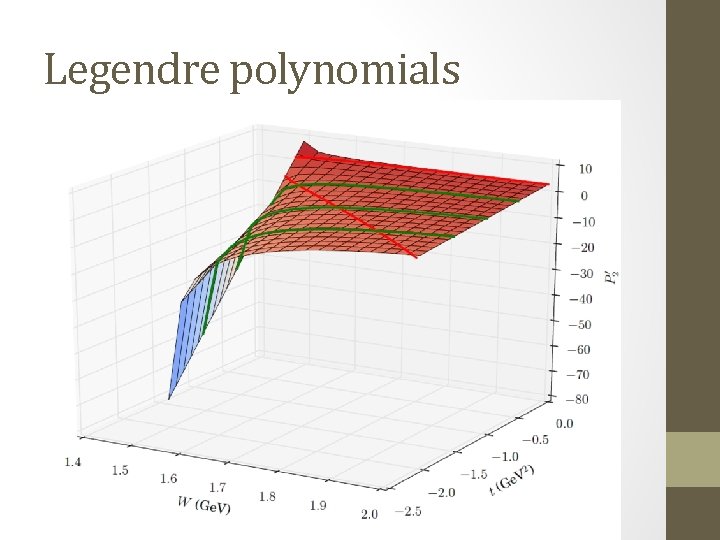
Legendre polynomials
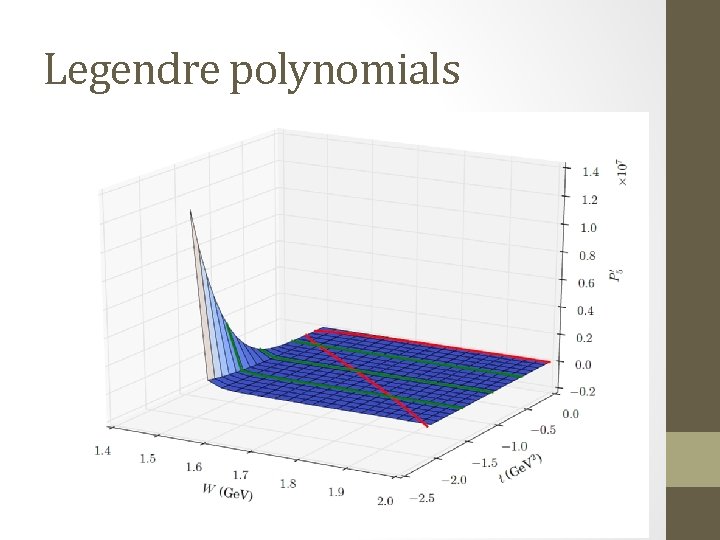
Legendre polynomials
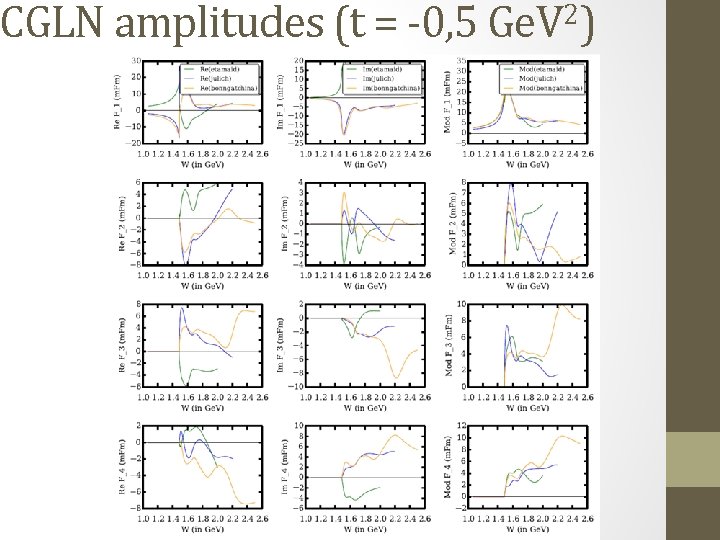
2 CGLN amplitudes (t = -0, 5 Ge. V )
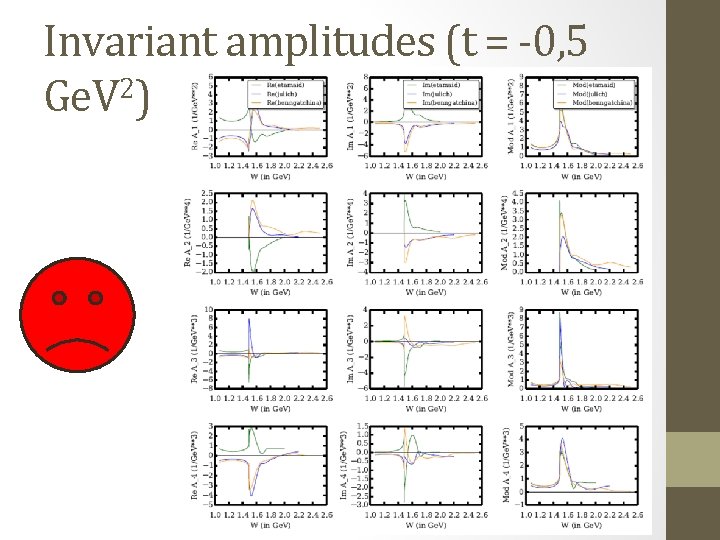
Invariant amplitudes (t = -0, 5 Ge. V 2)
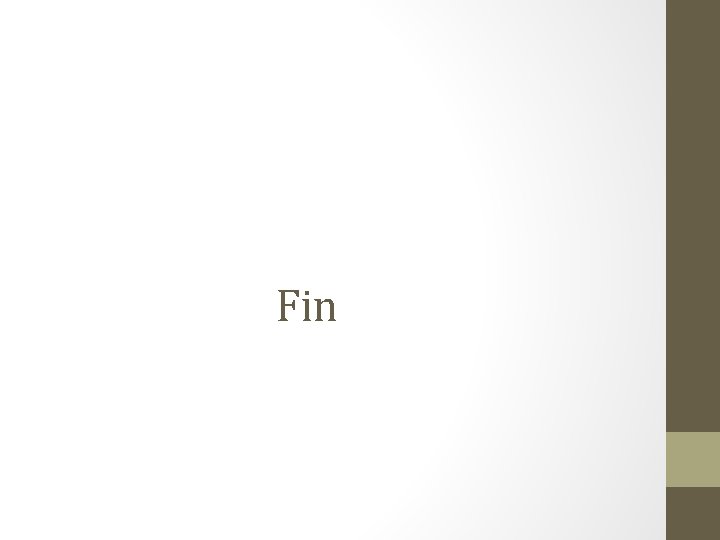
Fin
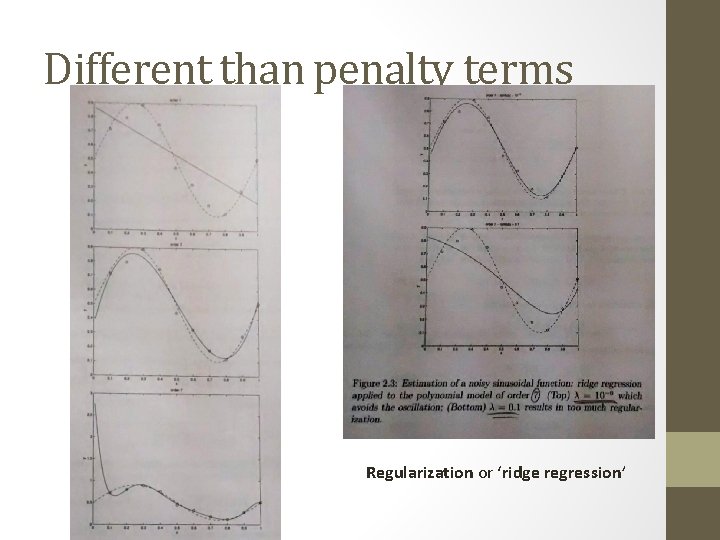
Different than penalty terms Regularization or ‘ridge regression’
What conclusion can you draw out from the pie graph
Analyzing and visualizing data with microsoft power bi
Interpreting quantitative data
Research design meaning
Analyzing and interpreting data in research
Analyzing and interpreting data in research
Analyzing and interpreting data in research
Analyzing and interpreting data in research
Ngss analyzing and interpreting data
Analyzing ethnographic data
Chapter 2 analyzing data
Chapter 2 analyzing data answer key
Analyzing systems using data dictionaries
Analyzing categorical data
Analyzing one variable data
Analyzing data
Analyzing categorical data
Measurements equivalents and adjustments
Chapter 2 measurements and calculations
Force and torque measurements
Ee8403 measurements and instrumentation
Anthropometric measurement includes vital signs
Vital signs and body measurements quiz
Angle measures and segment lengths
Orthostatic vital signs
Vital signs and anthropometric measurements
Measurement topic
Scientific measurements
Pharmaceutical measurements
Diamtral
Metrology and measurements subject code
Tablespoon abbreviation
Percent error example
Pharmaceutical measurements and calculations
5 physical quantities and their si units
Using and expressing measurements
Unit 2 lesson 2 scientific notation
Weights and measure training