An Introduction to Compressive Sensing Speaker YingJou Chen
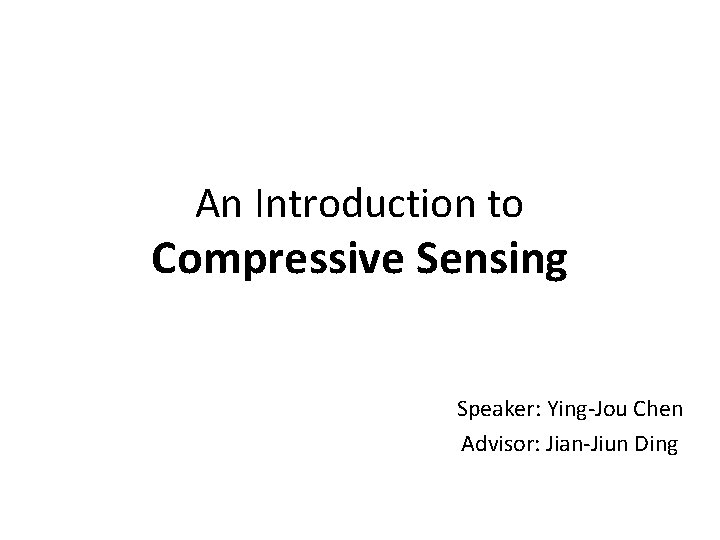
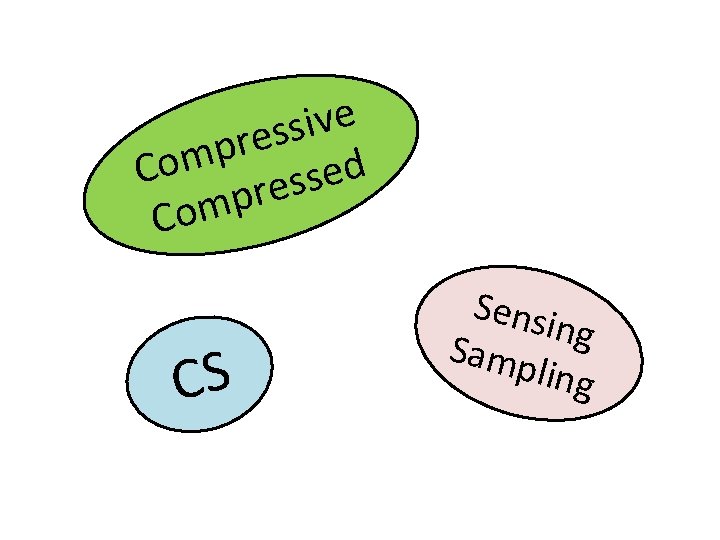
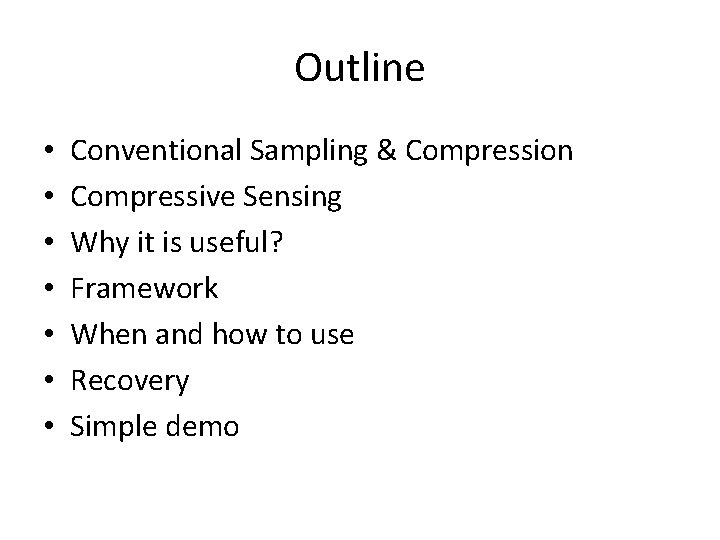
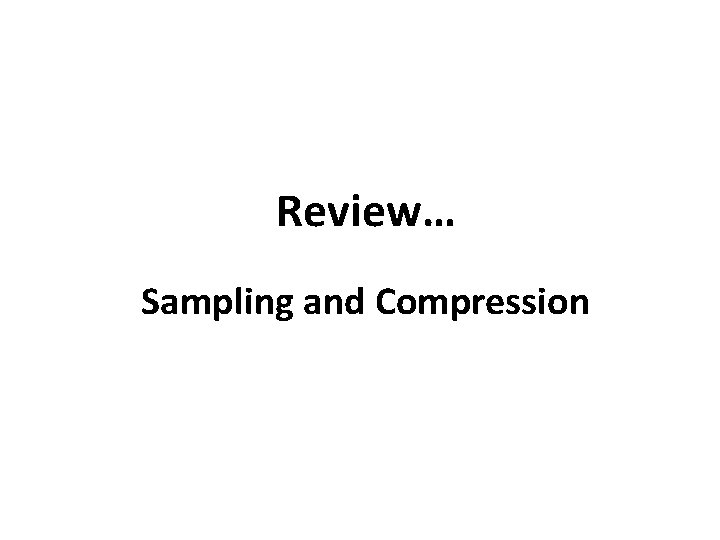
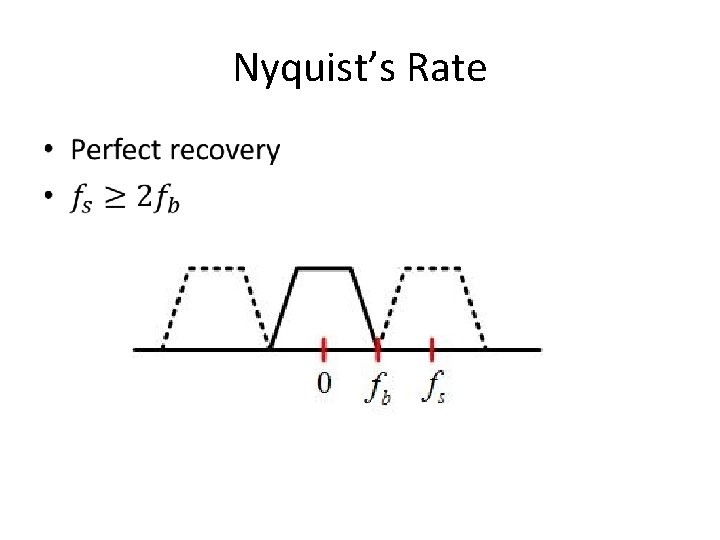
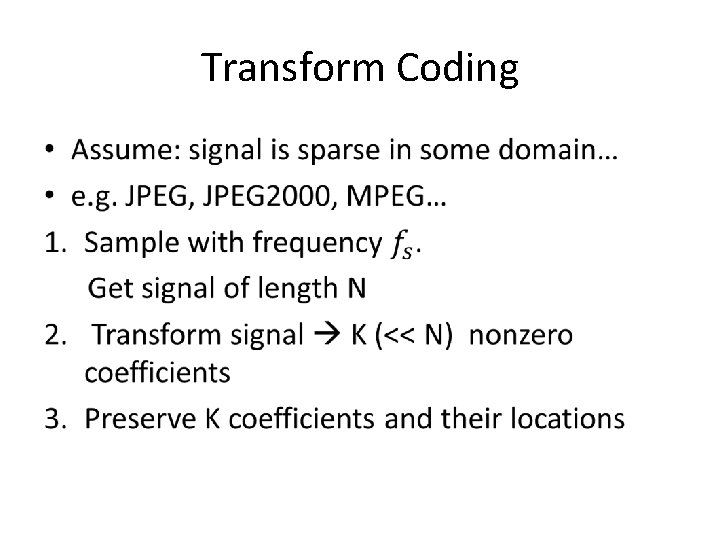
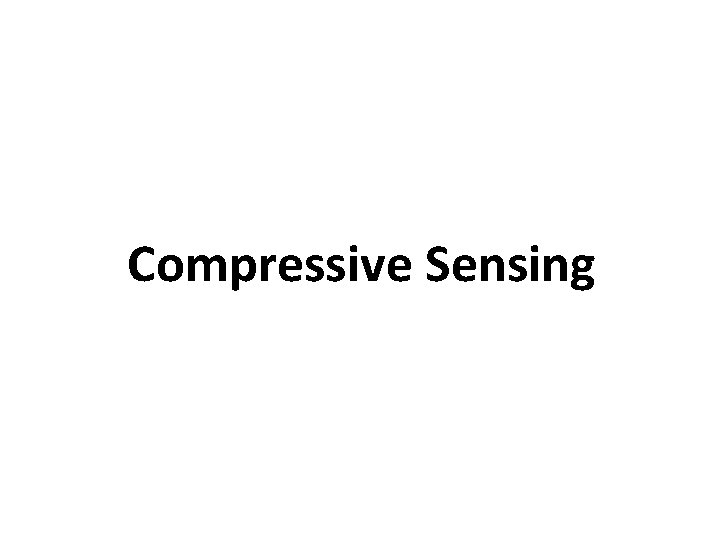
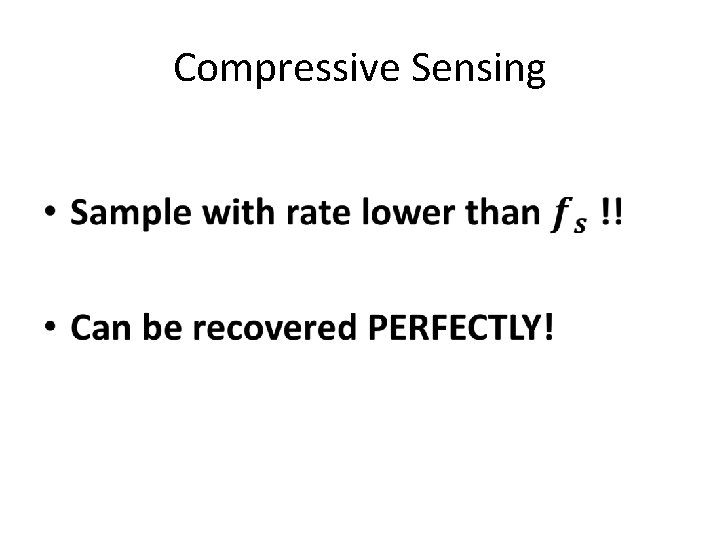
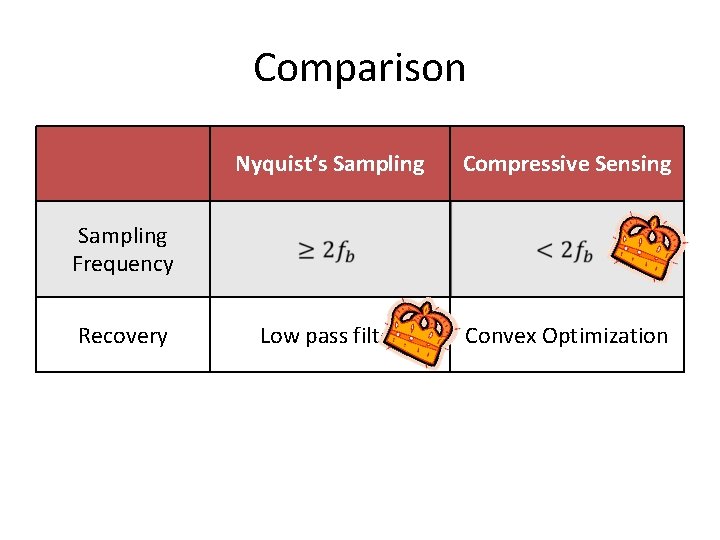
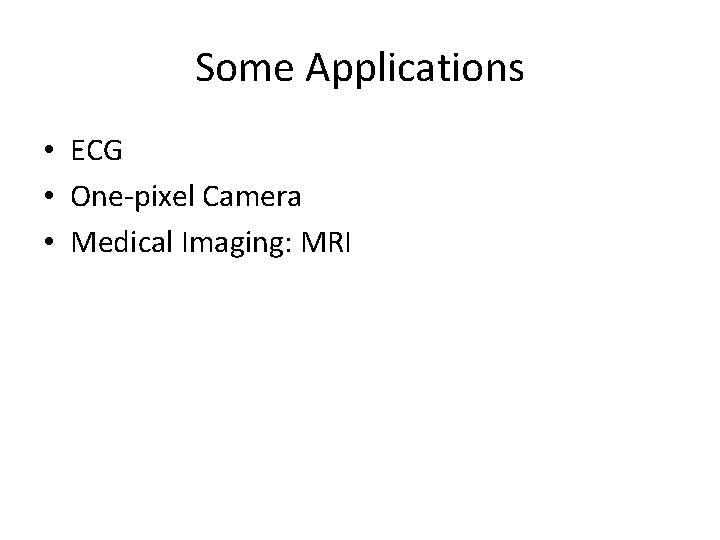
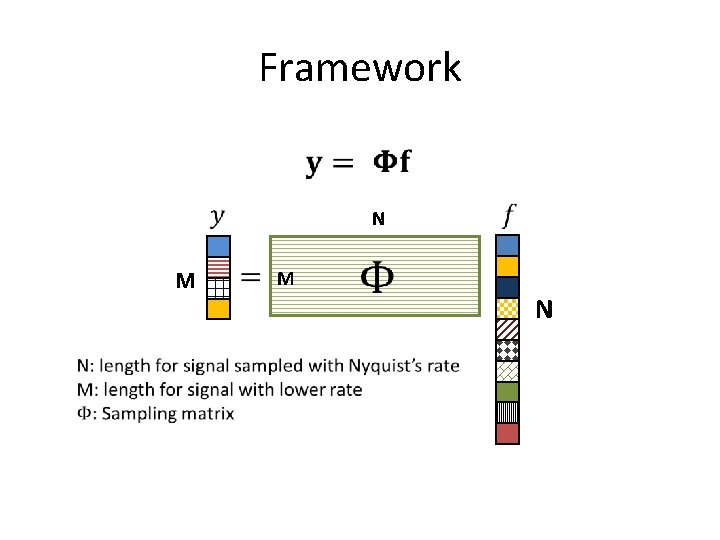
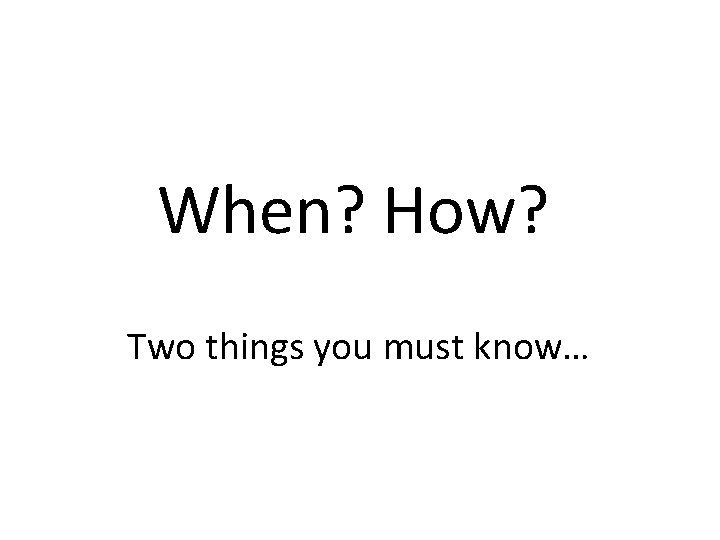
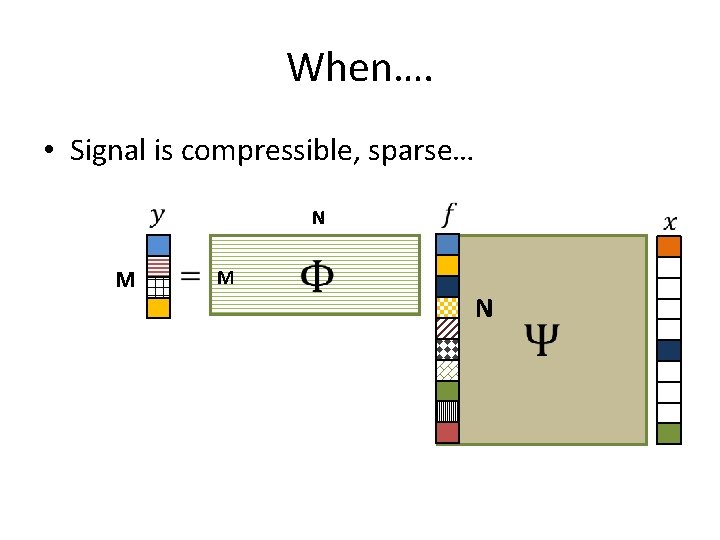
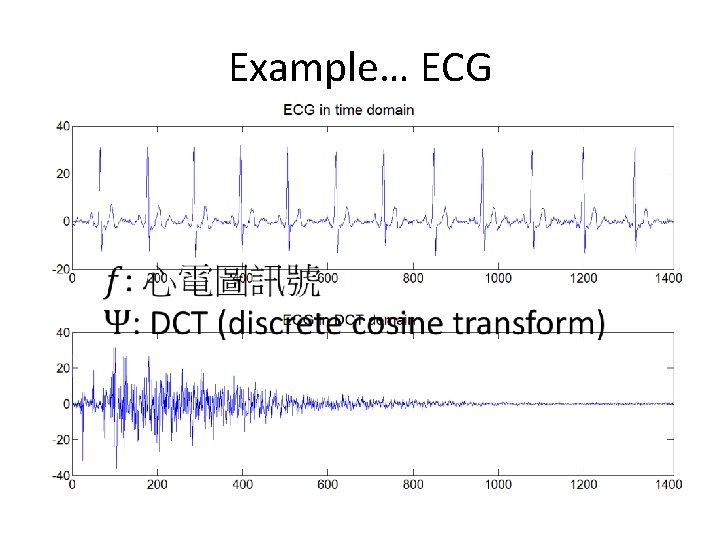
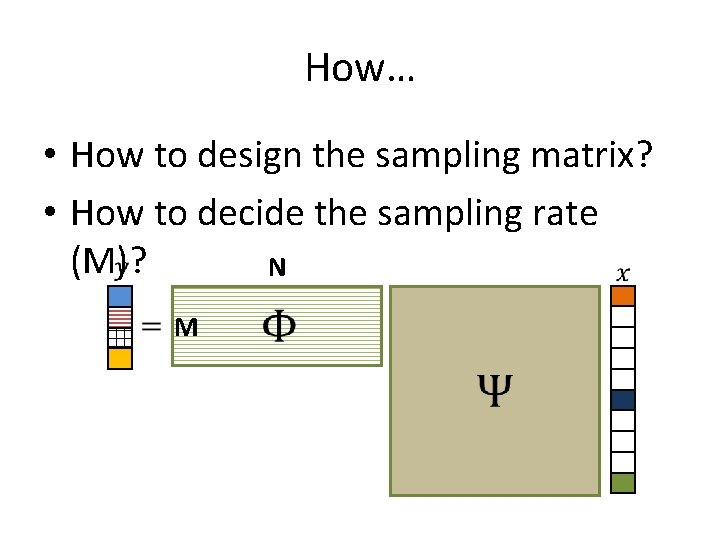
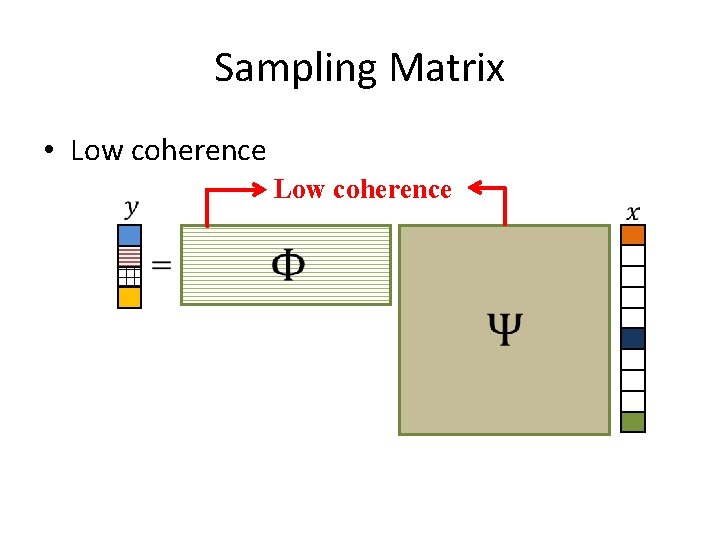
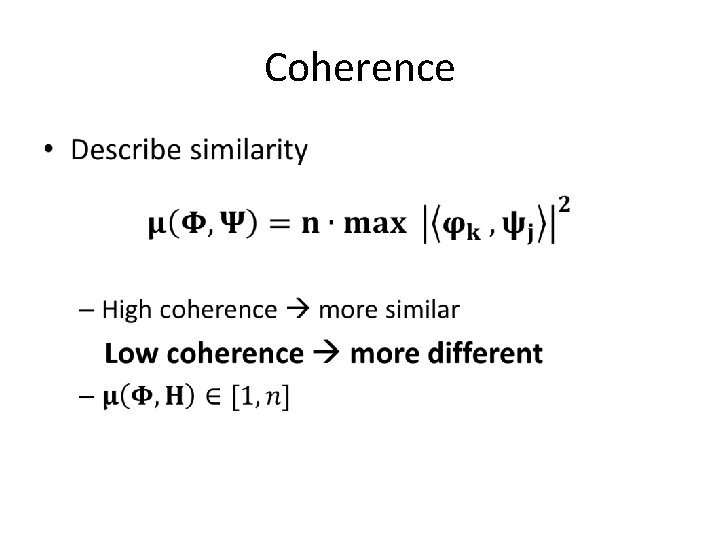
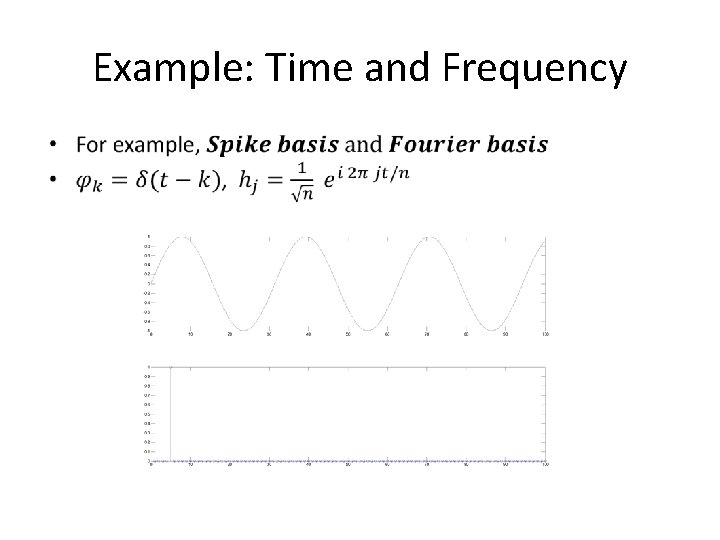
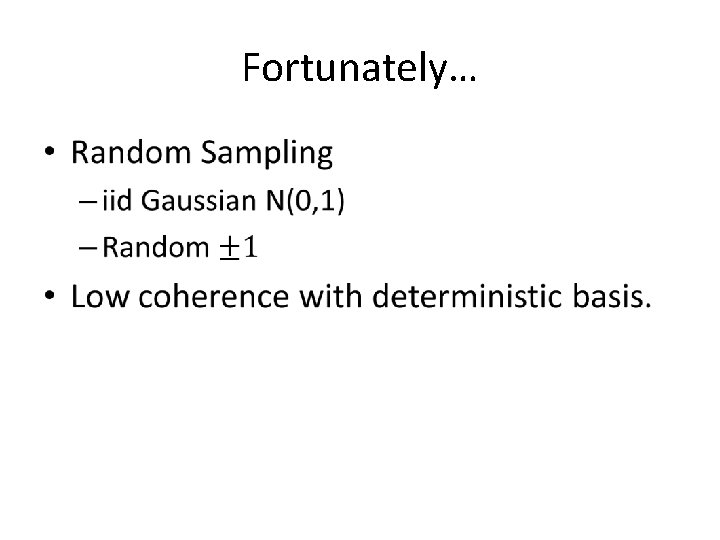
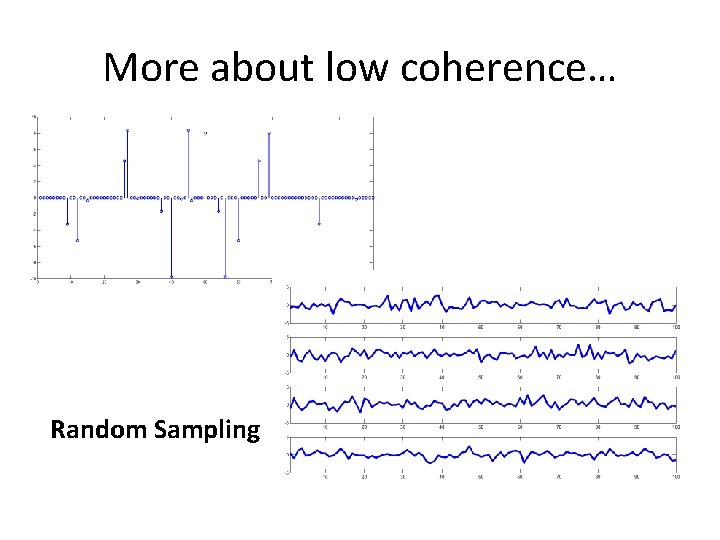
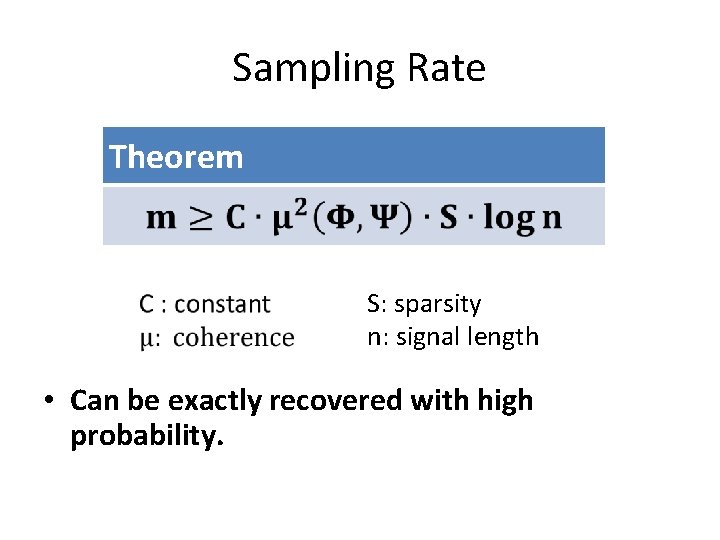
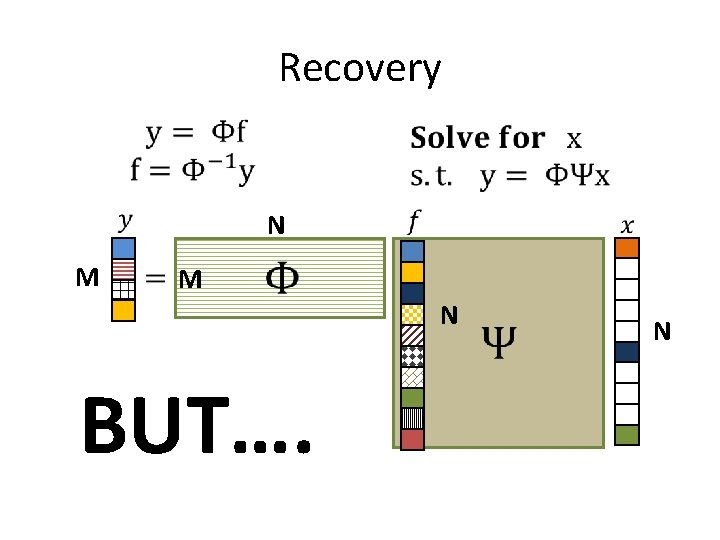
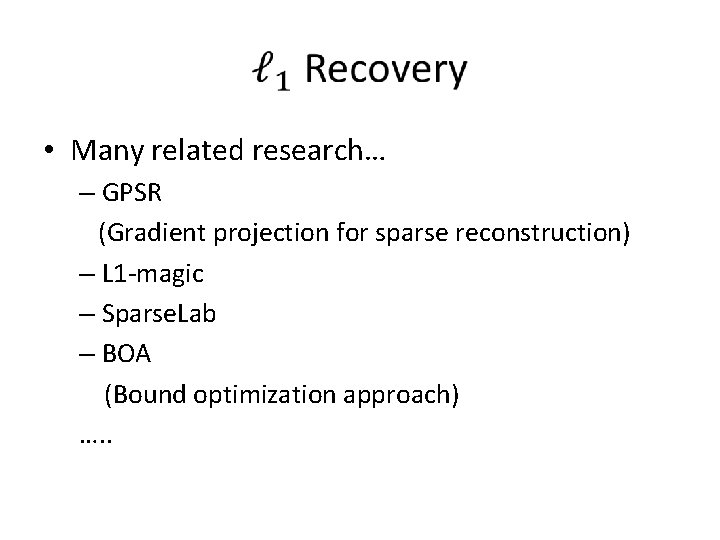
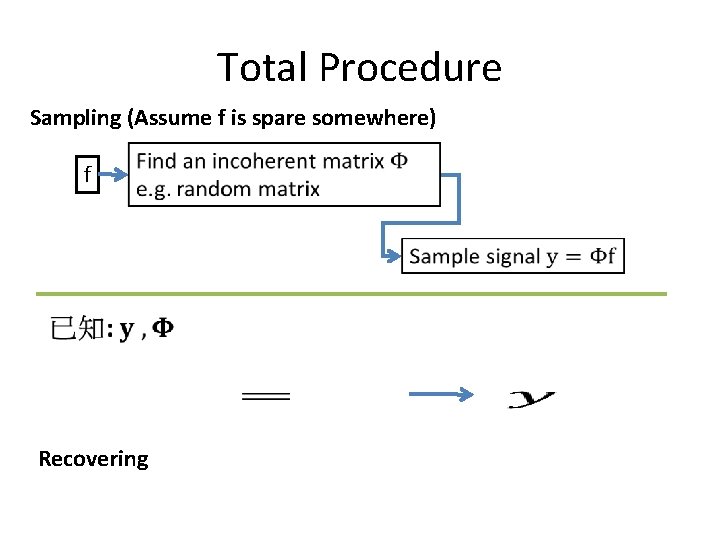
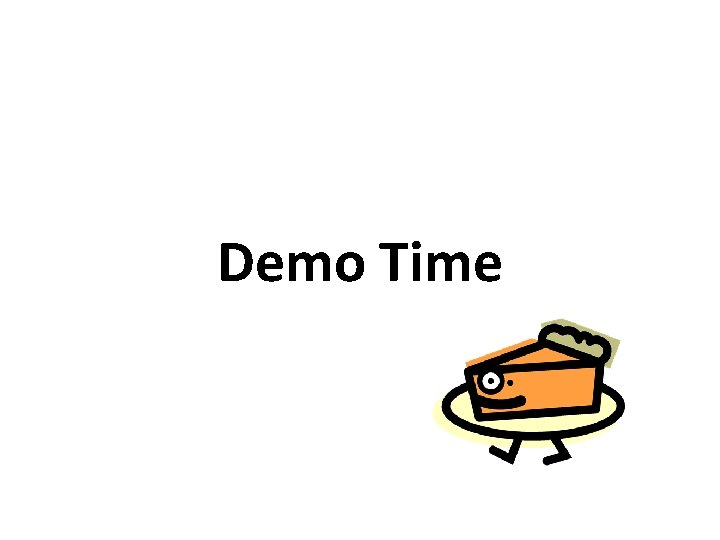
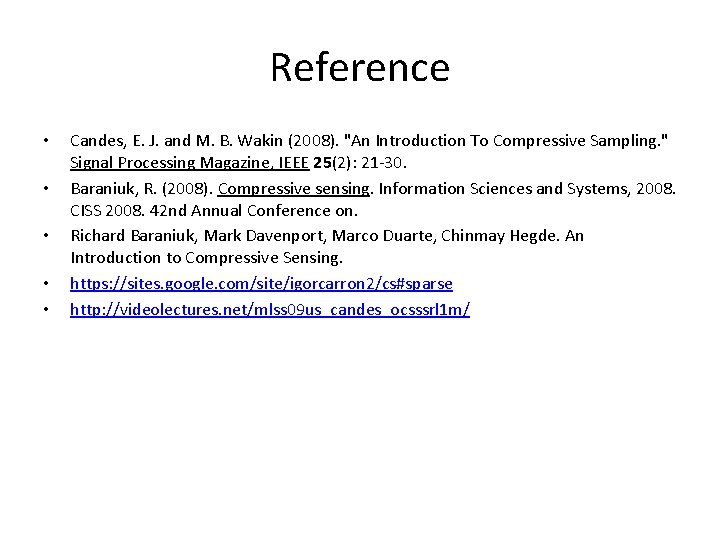
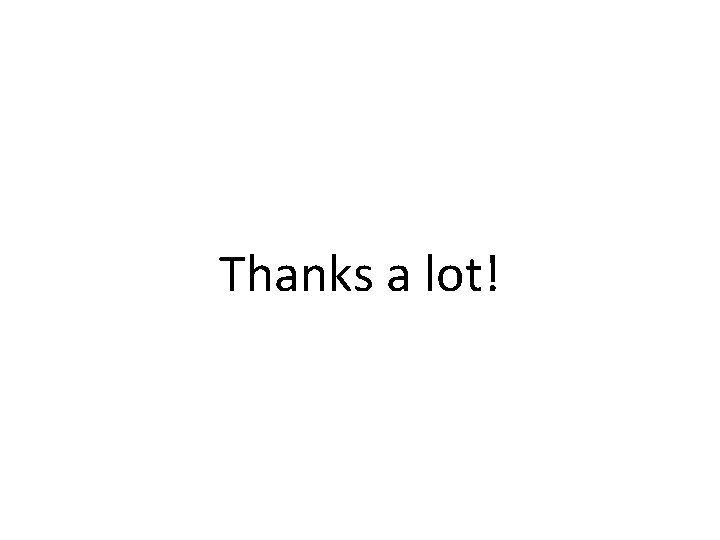
- Slides: 27
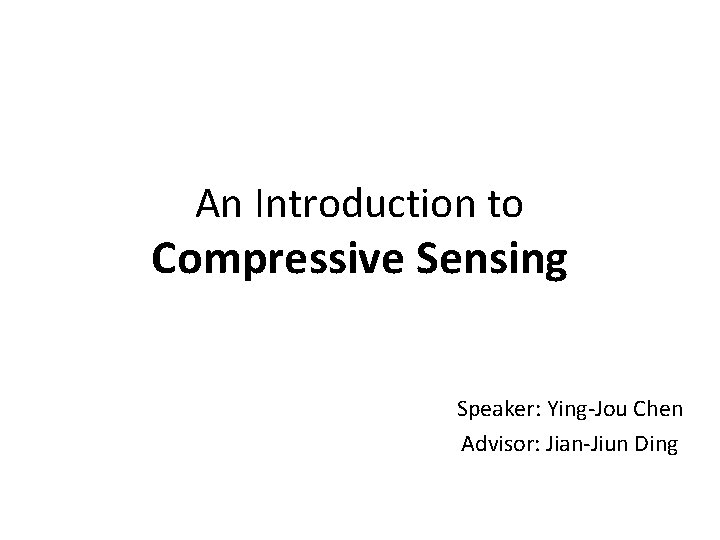
An Introduction to Compressive Sensing Speaker: Ying-Jou Chen Advisor: Jian-Jiun Ding
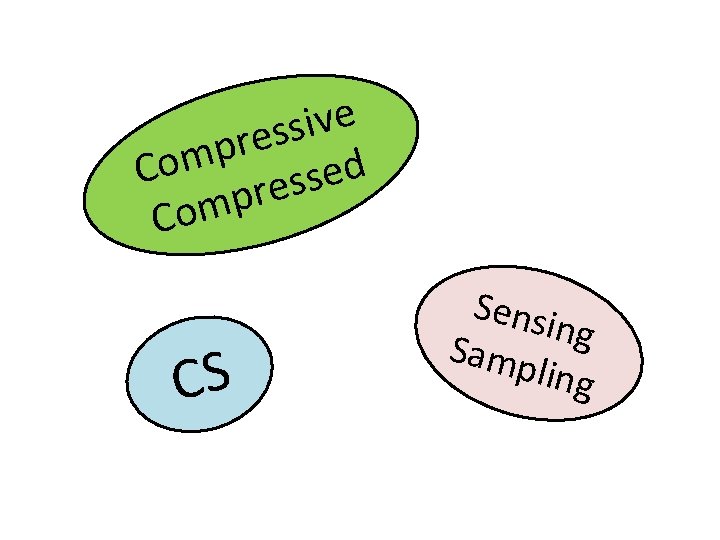
e v i s s e r p m d Co e s s e r p m o C CS Sensi ng Samp ling
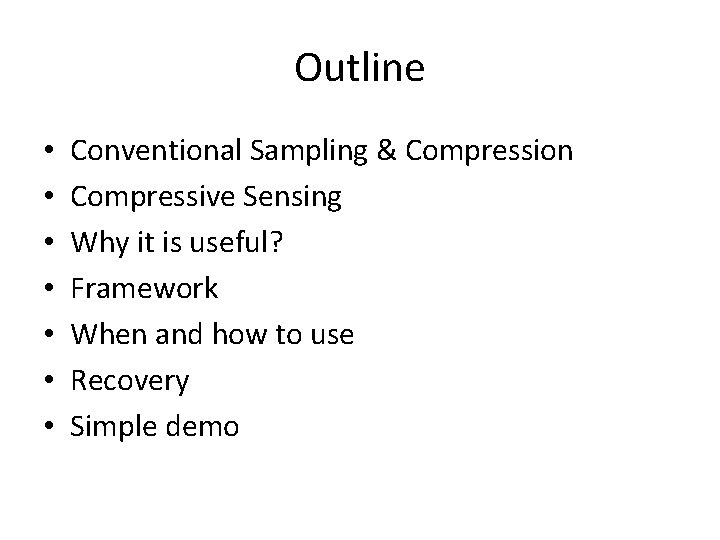
Outline • • Conventional Sampling & Compression Compressive Sensing Why it is useful? Framework When and how to use Recovery Simple demo
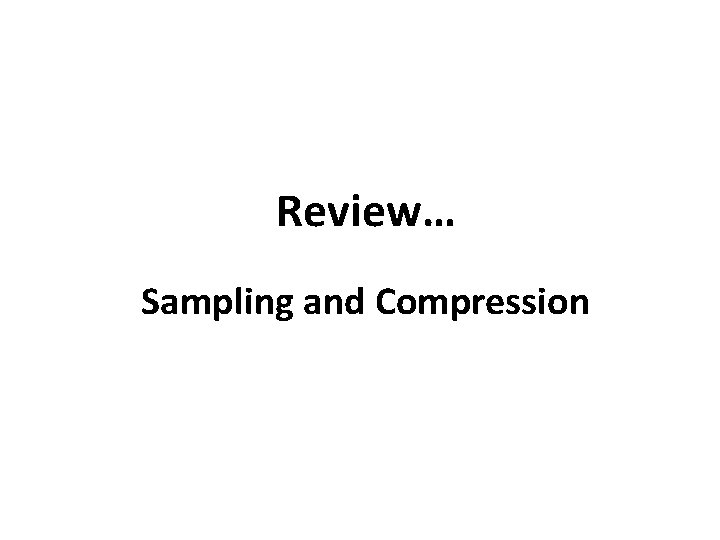
Review… Sampling and Compression
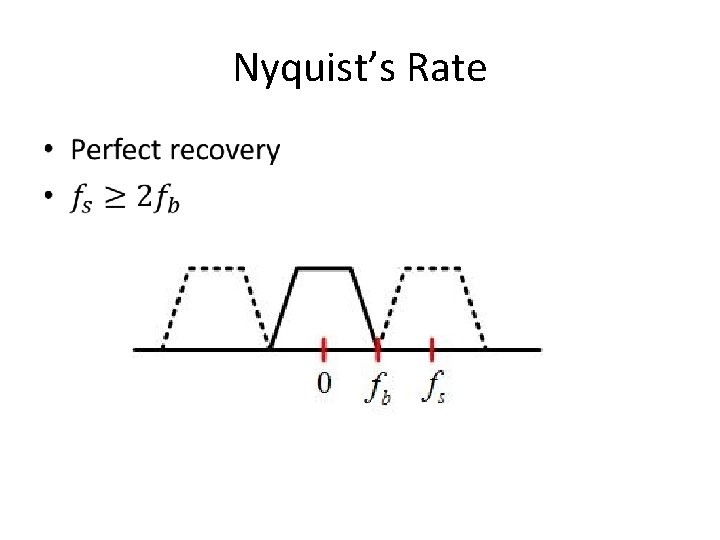
Nyquist’s Rate •
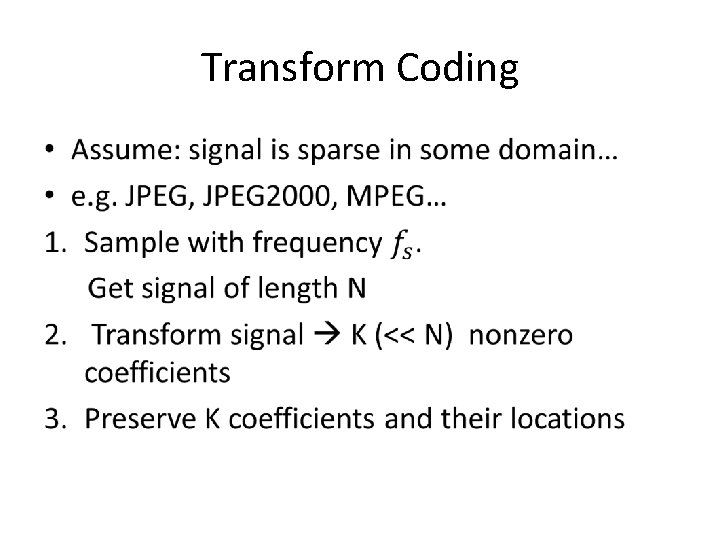
Transform Coding •
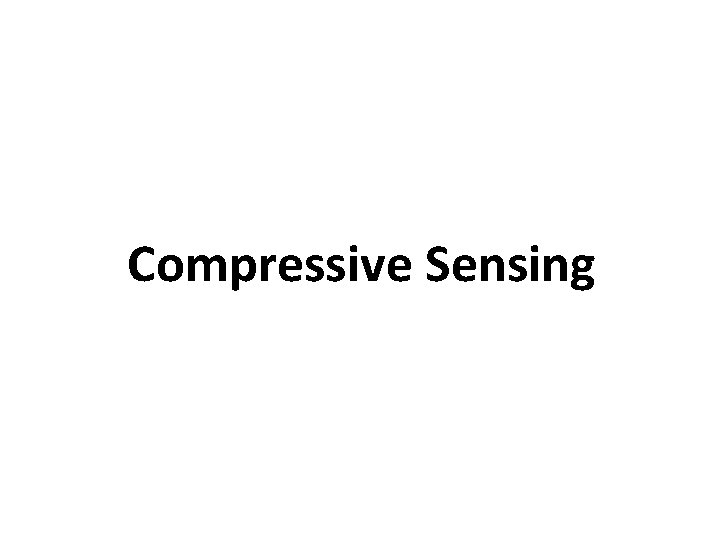
Compressive Sensing
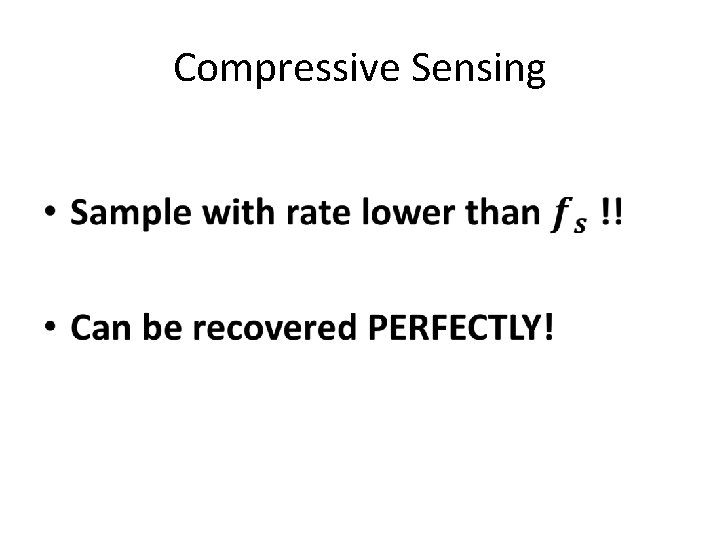
Compressive Sensing •
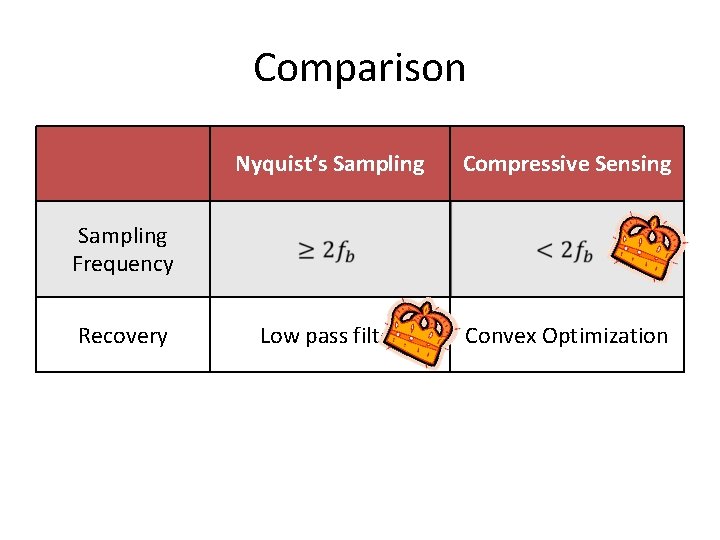
Comparison Nyquist’s Sampling Compressive Sensing Low pass filter Convex Optimization Sampling Frequency Recovery
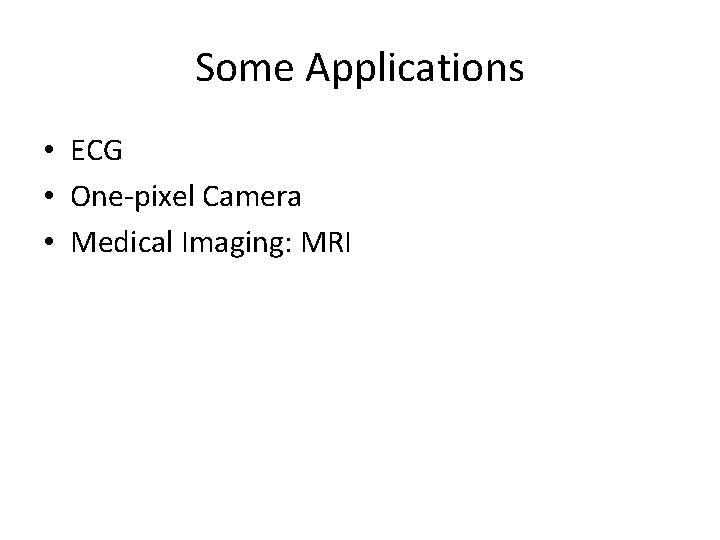
Some Applications • ECG • One-pixel Camera • Medical Imaging: MRI
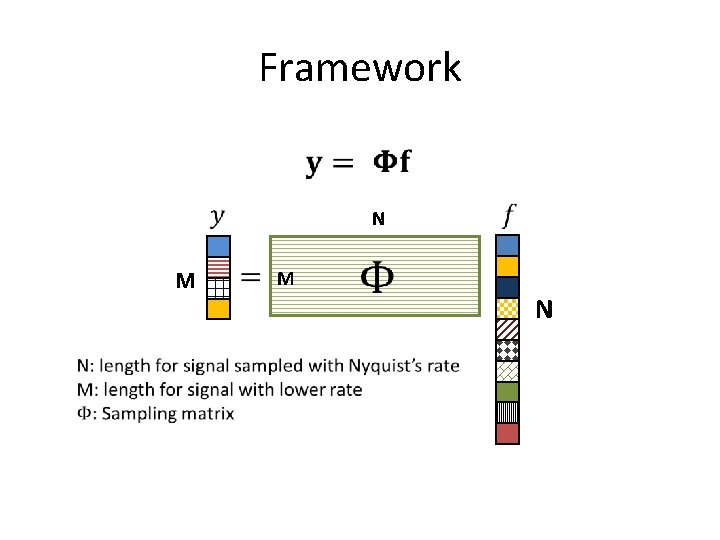
Framework N M M N
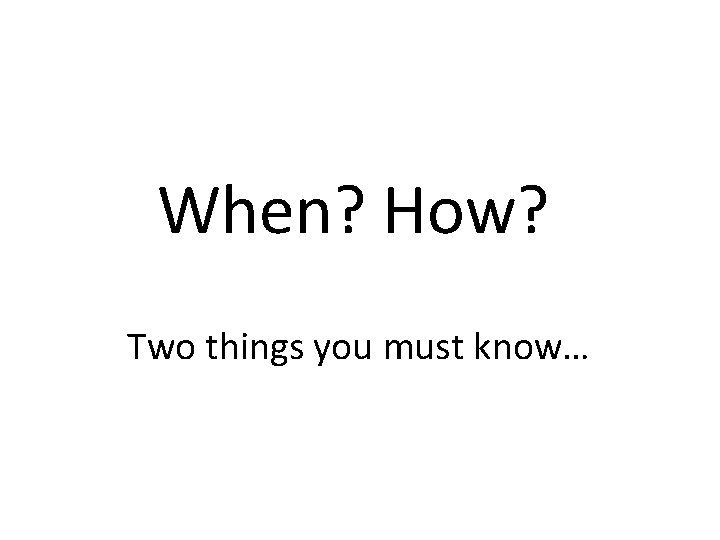
When? How? Two things you must know…
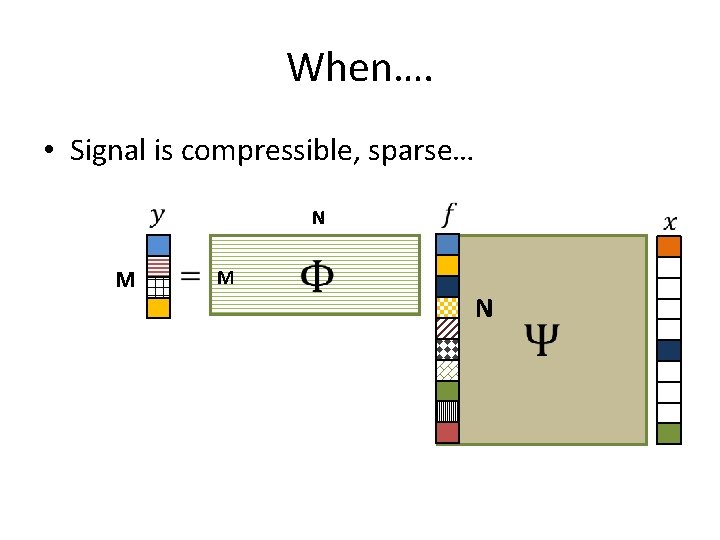
When…. • Signal is compressible, sparse… N M M N
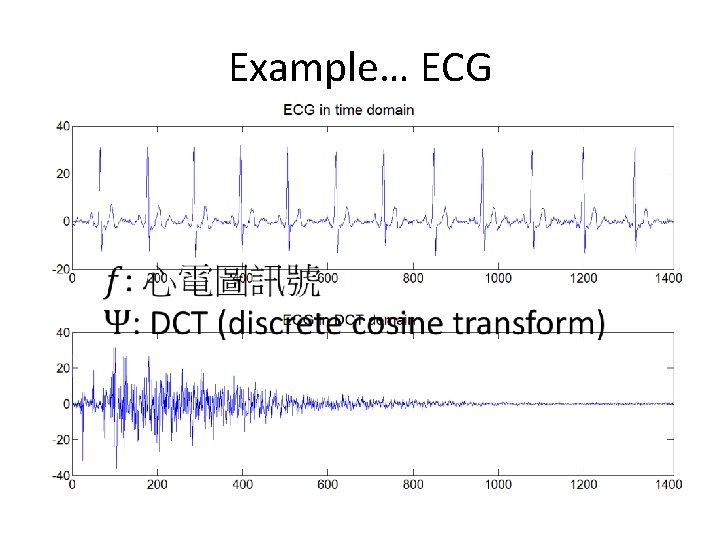
Example… ECG
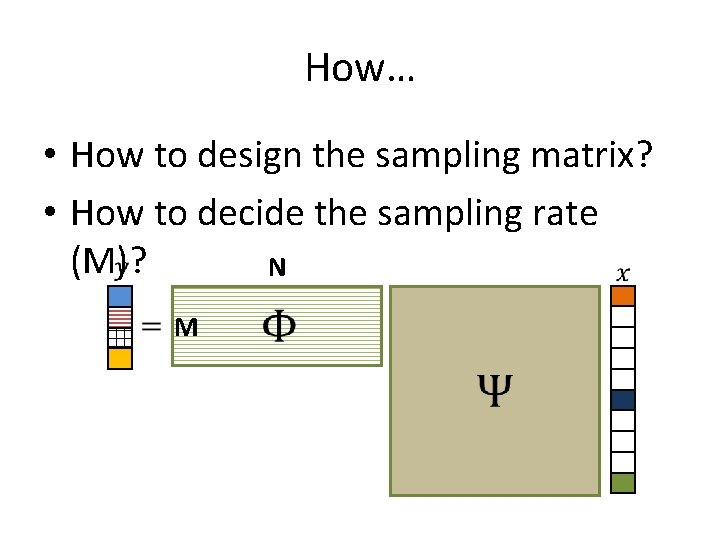
How… • How to design the sampling matrix? • How to decide the sampling rate (M)? N M
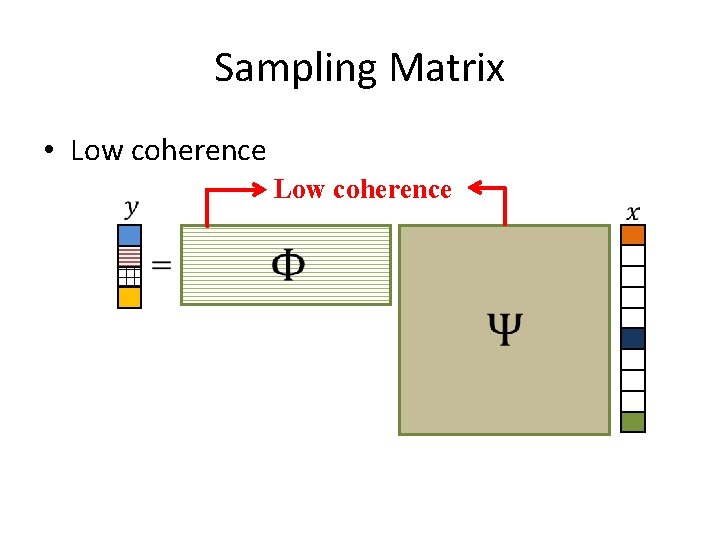
Sampling Matrix • Low coherence
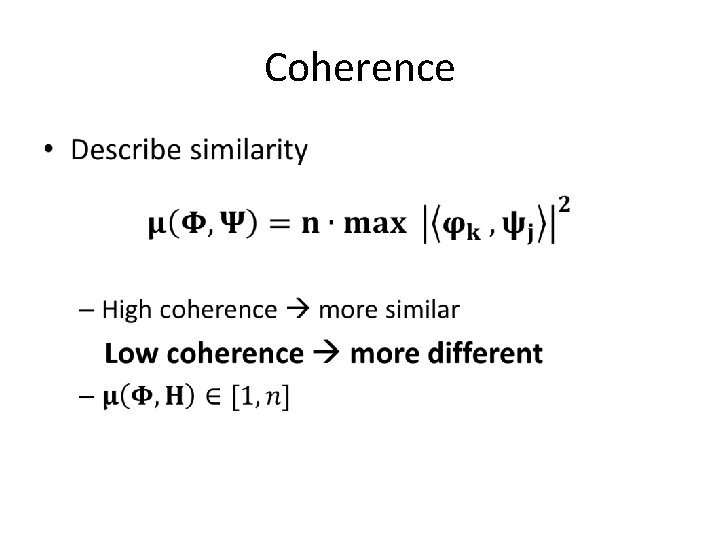
Coherence •
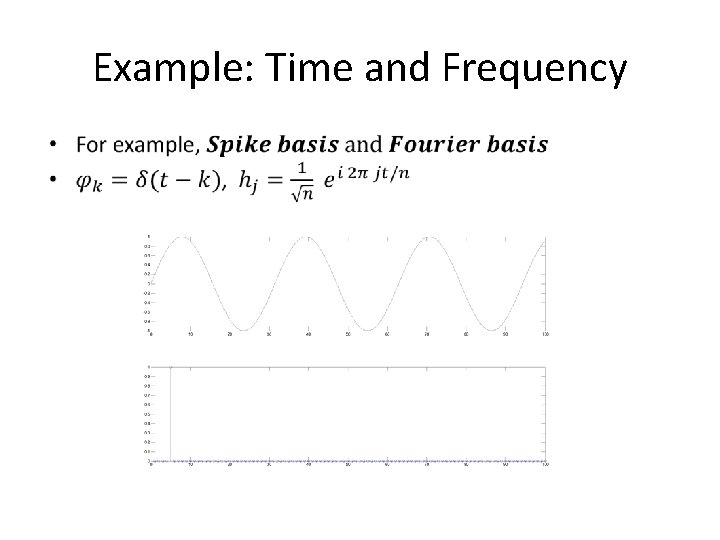
Example: Time and Frequency
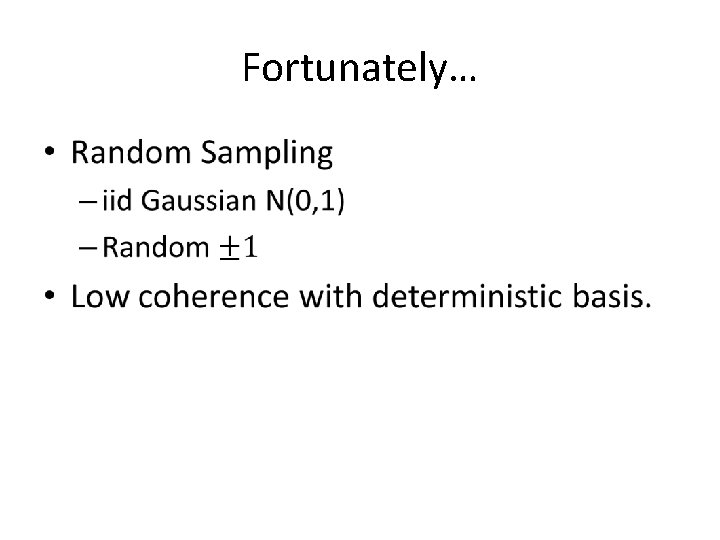
Fortunately… •
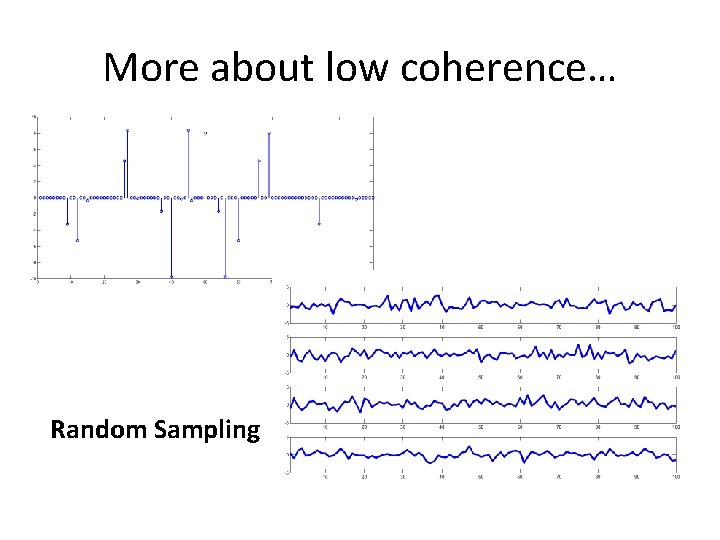
More about low coherence… Random Sampling
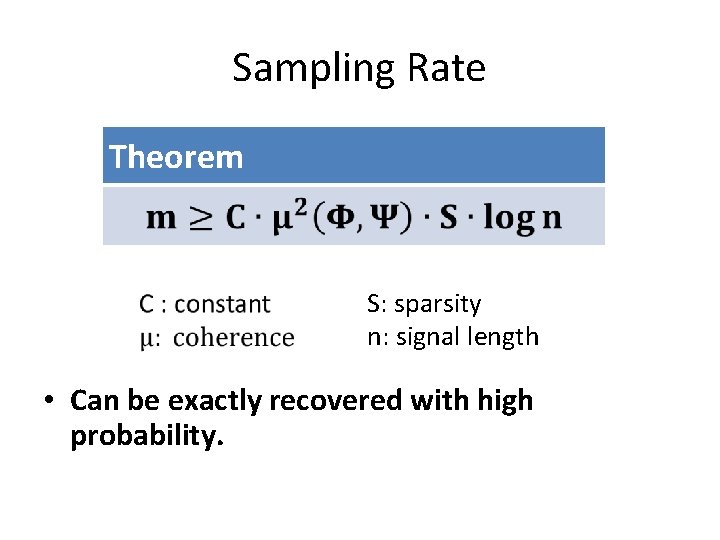
Sampling Rate Theorem S: sparsity n: signal length • Can be exactly recovered with high probability.
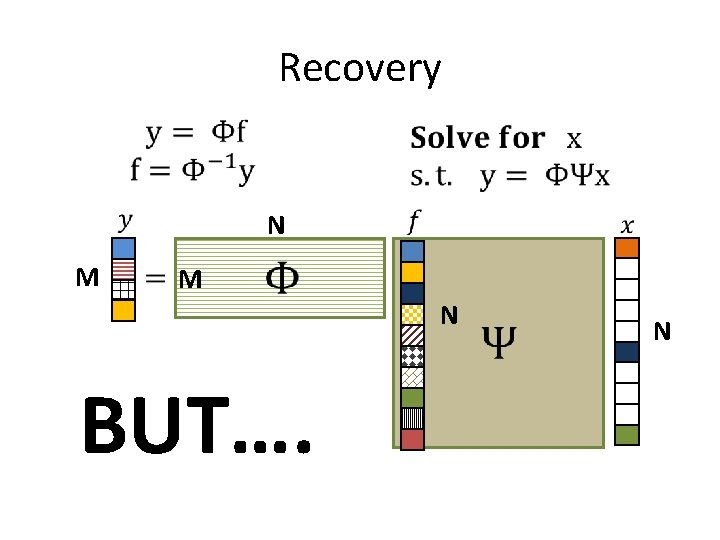
Recovery N M M N BUT…. N
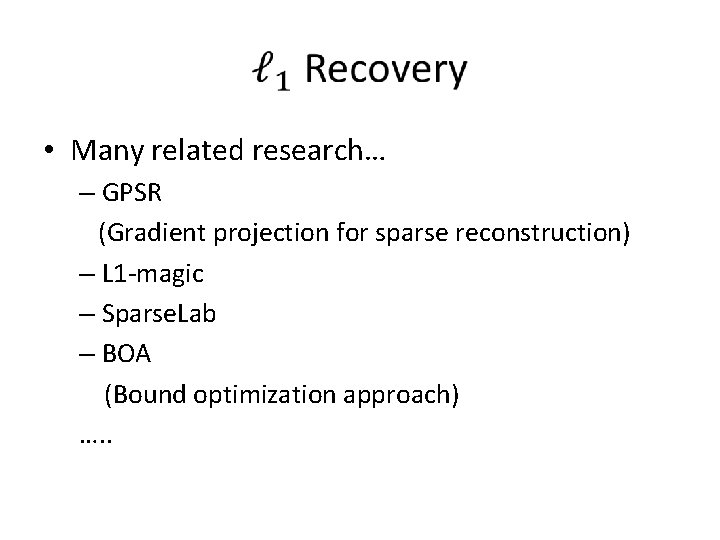
• Many related research… – GPSR (Gradient projection for sparse reconstruction) – L 1 -magic – Sparse. Lab – BOA (Bound optimization approach) …. .
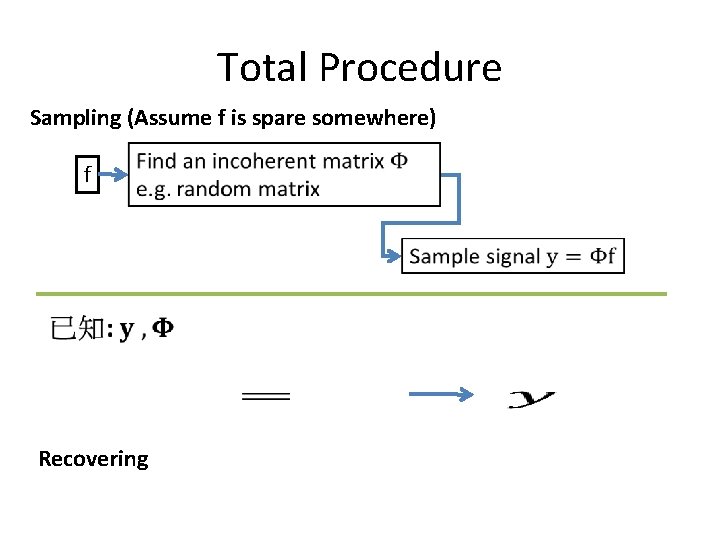
Total Procedure Sampling (Assume f is spare somewhere) f Recovering
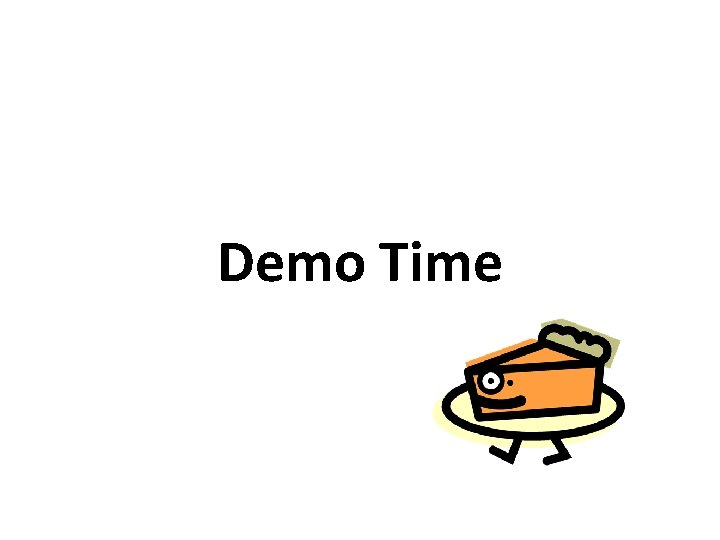
Demo Time
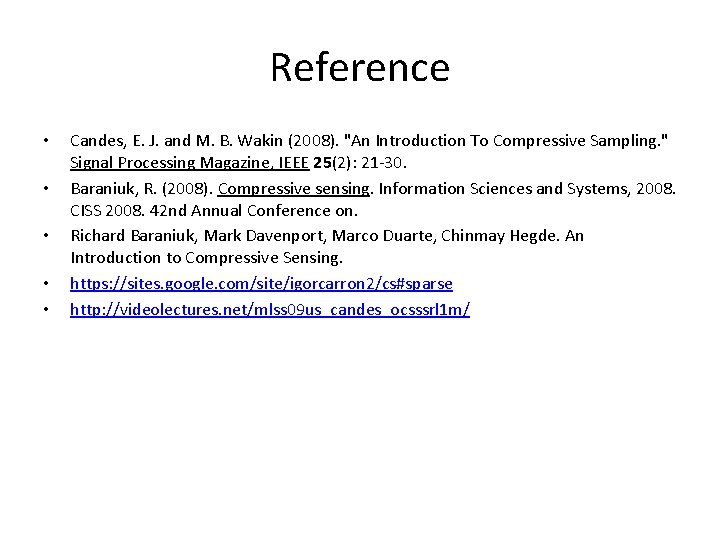
Reference • • • Candes, E. J. and M. B. Wakin (2008). "An Introduction To Compressive Sampling. " Signal Processing Magazine, IEEE 25(2): 21 -30. Baraniuk, R. (2008). Compressive sensing. Information Sciences and Systems, 2008. CISS 2008. 42 nd Annual Conference on. Richard Baraniuk, Mark Davenport, Marco Duarte, Chinmay Hegde. An Introduction to Compressive Sensing. https: //sites. google. com/site/igorcarron 2/cs#sparse http: //videolectures. net/mlss 09 us_candes_ocsssrl 1 m/
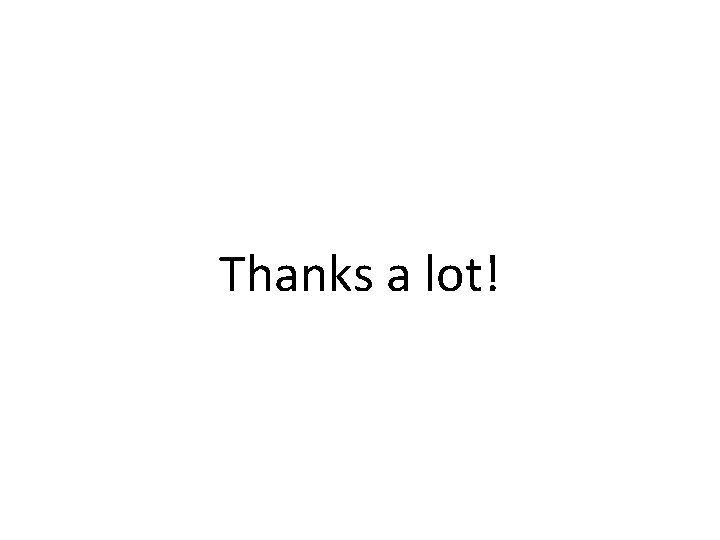
Thanks a lot!
Chen chen berlin
Admixture definition in civil engineering
Spinal cord spasticity
Which rock possesses very high compressive strength
Introduction to microwave remote sensing
Welcome address for webinar sample
N-rays
Remote sensing platforms
Pressure sensing elements
Active remote sensing
What is sensing
Sensing intuition feeling thinking
Advantages of remote sensing
What is source of measurand
Digital number remote sensing
Demand sensing and shaping
Limitations of remote sensing
Idealized remote sensing system
Twiddler syndrome
Pacemaker not sensing
Choropleth map example ap human geography
Thematic map meaning
Stereoscopic parallax
Strip camera in remote sensing
Nuvations
Remote sensing image
Ifov and fov in remote sensing
Geometry of aerial photography pdf