Introduction to Compressive Sensing Aswin Sankaranarayanan system Is
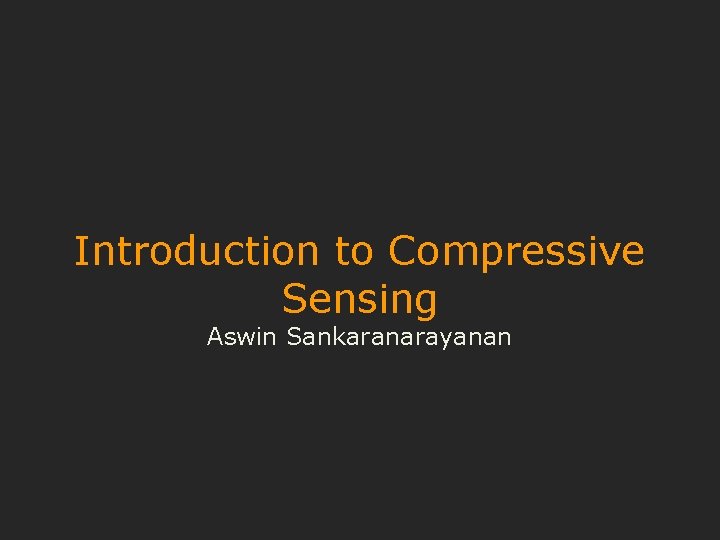
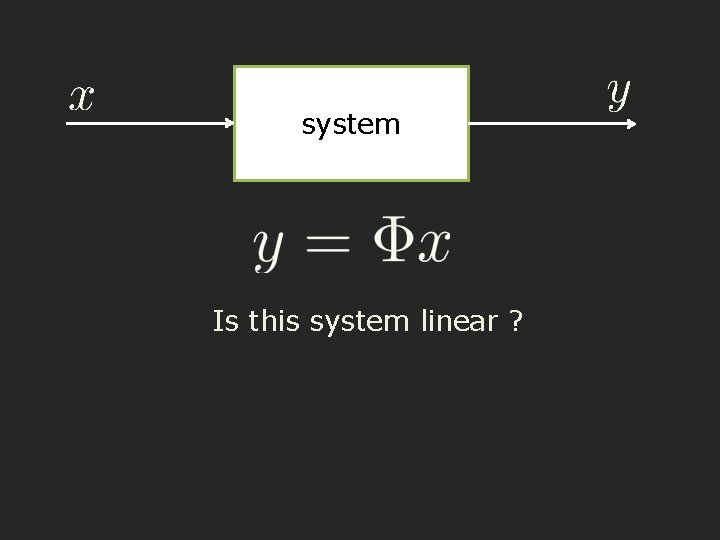
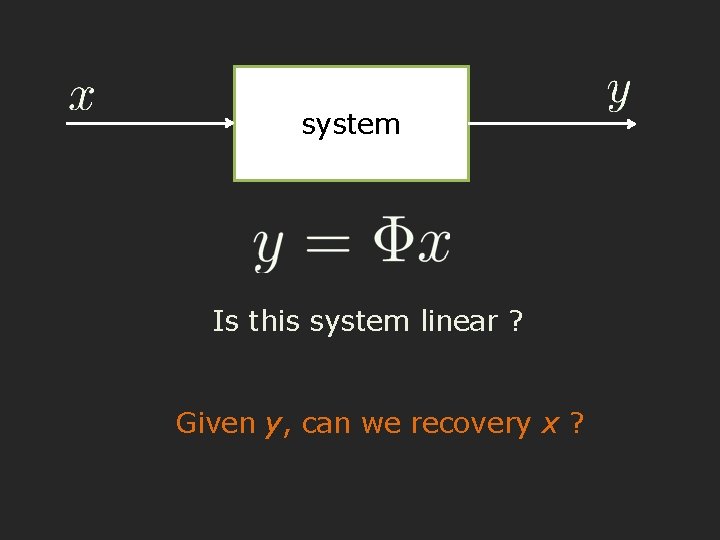
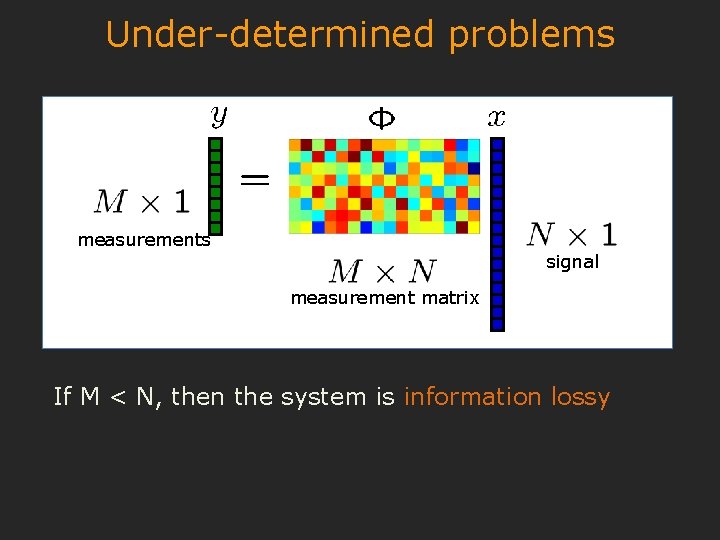
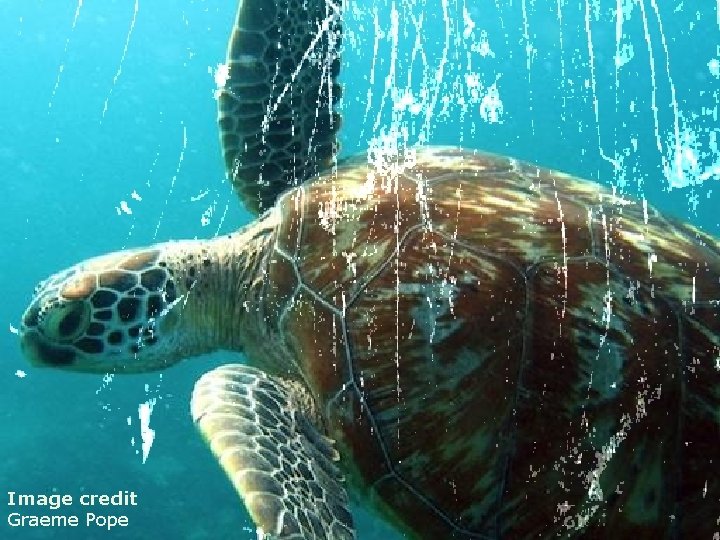
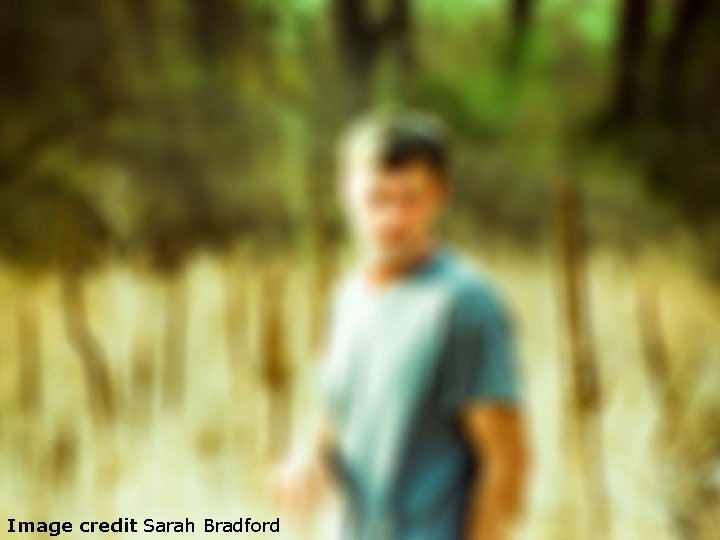
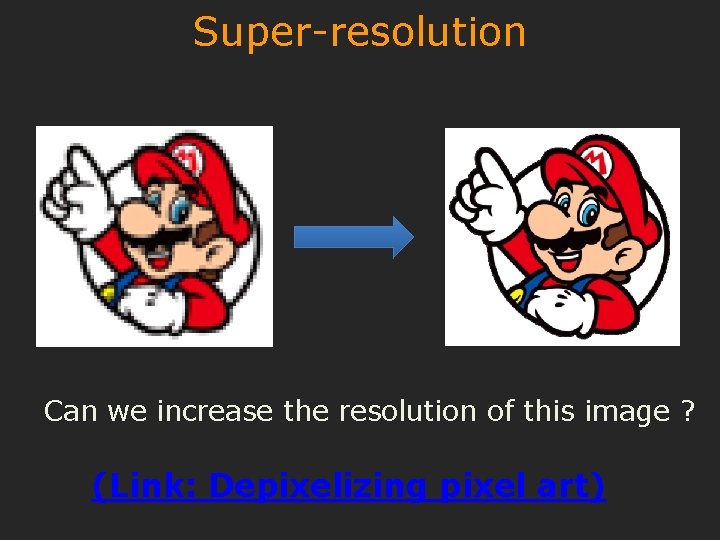
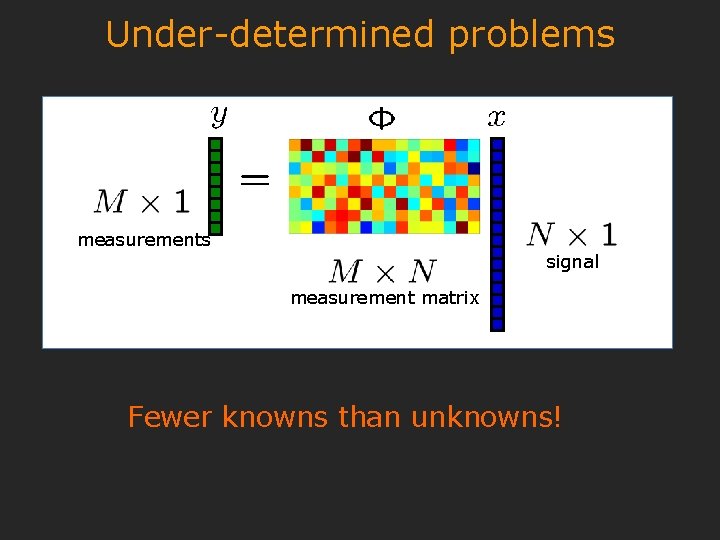
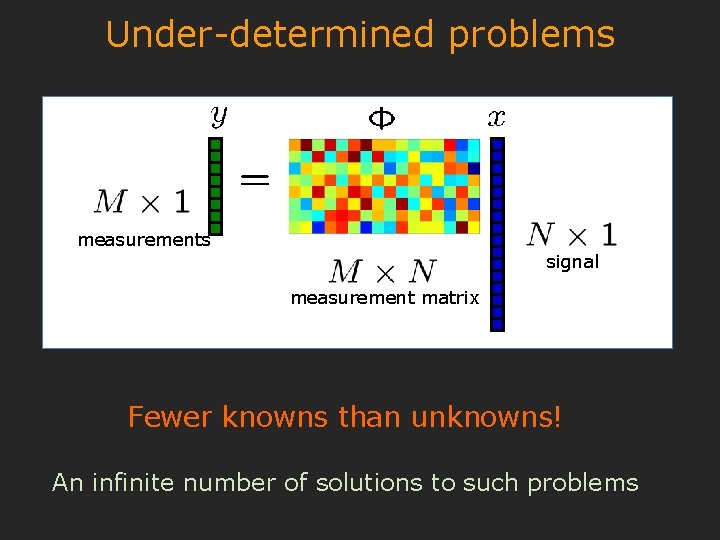
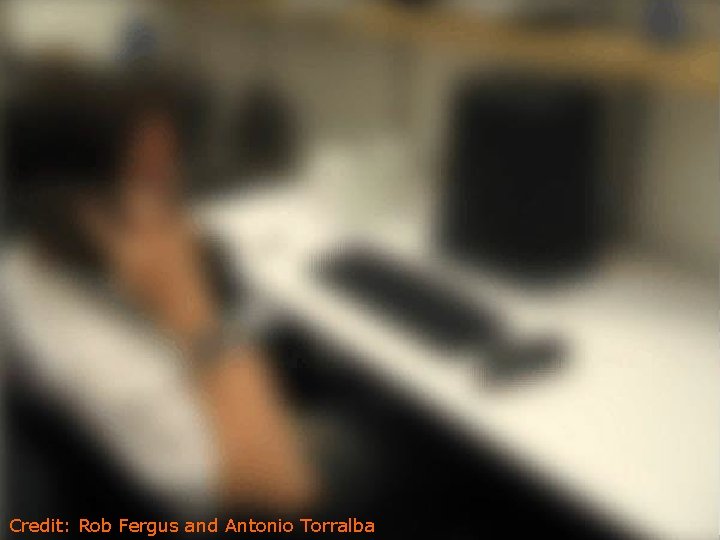
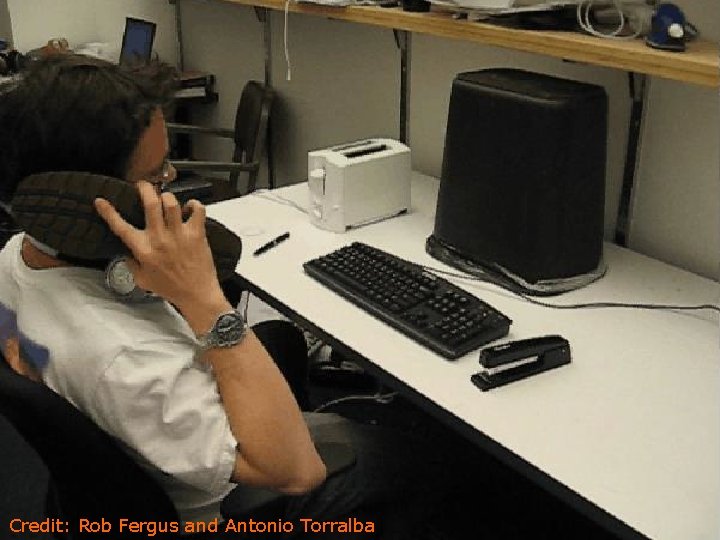
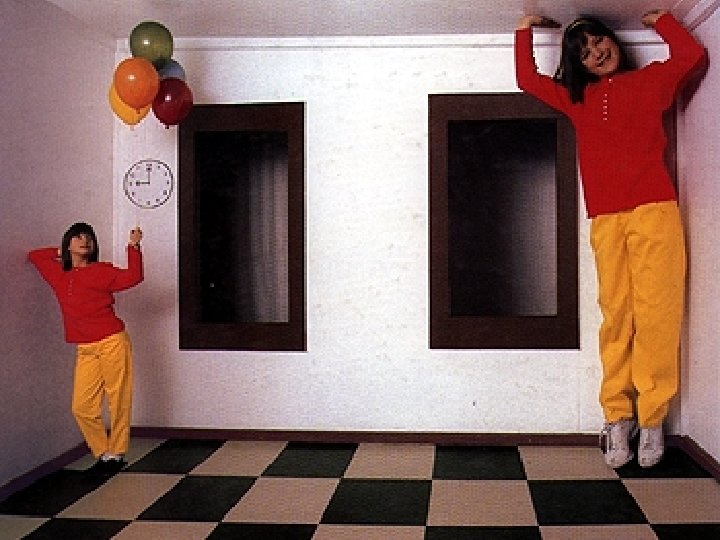
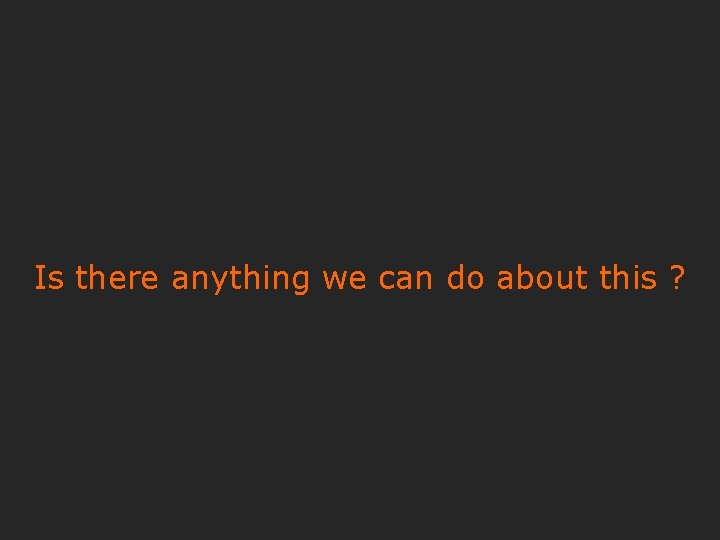
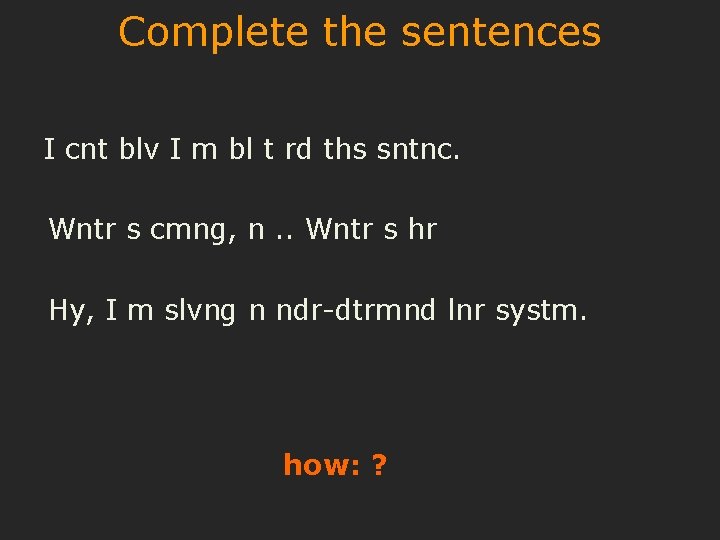
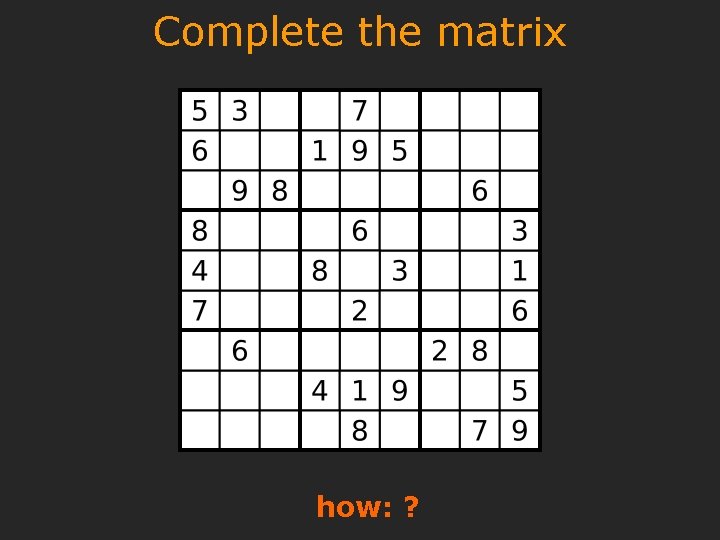
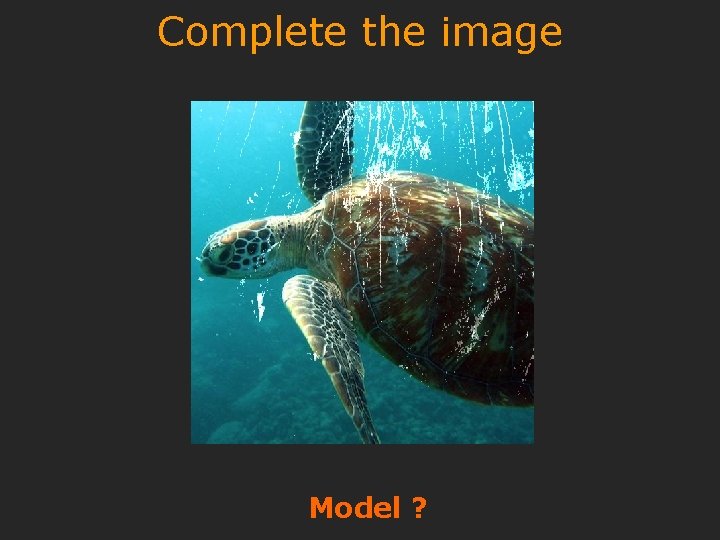
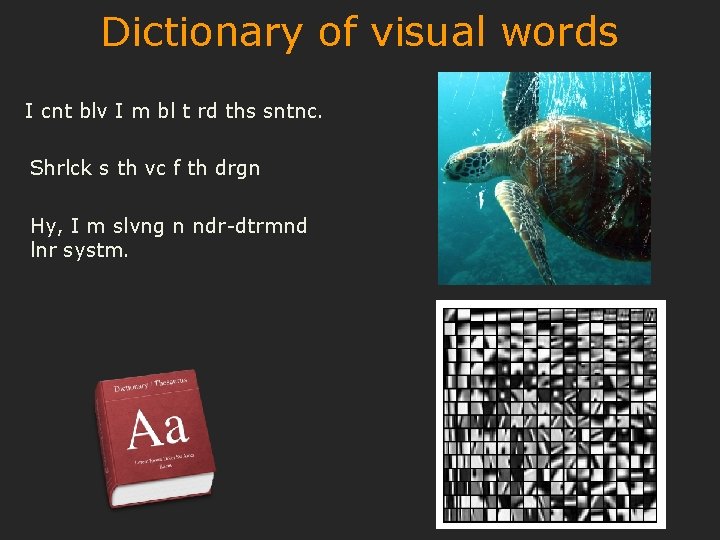
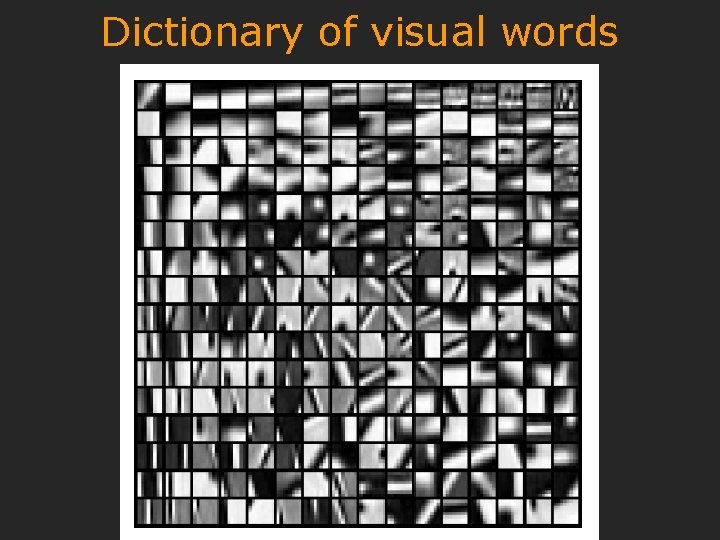
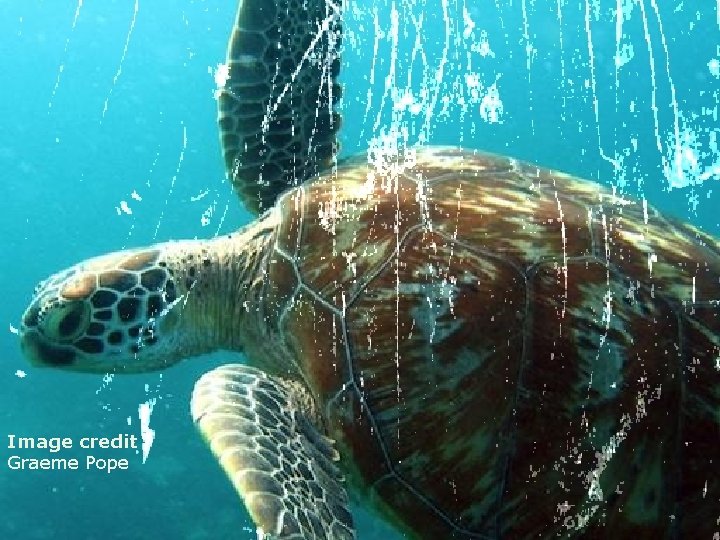
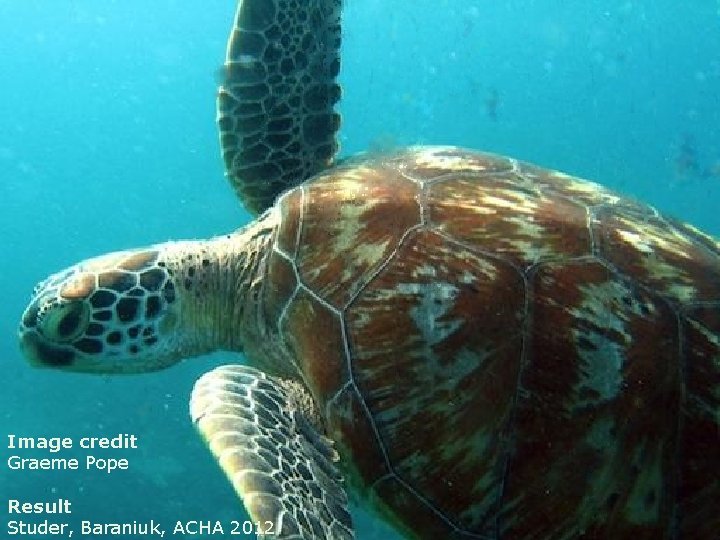
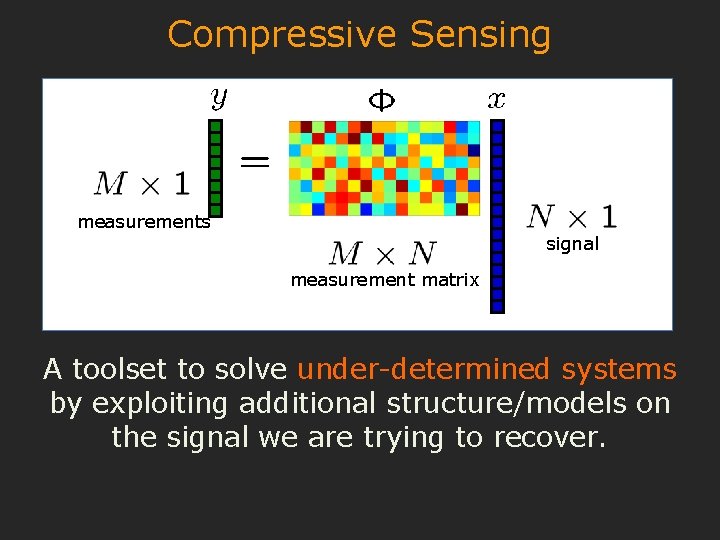
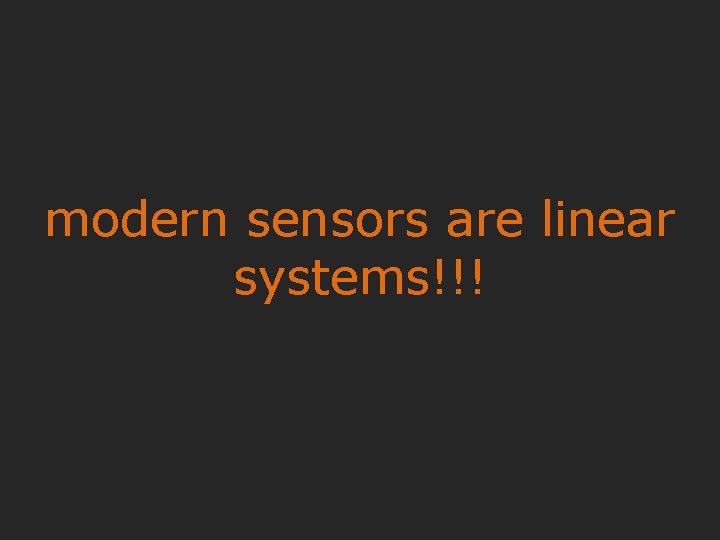
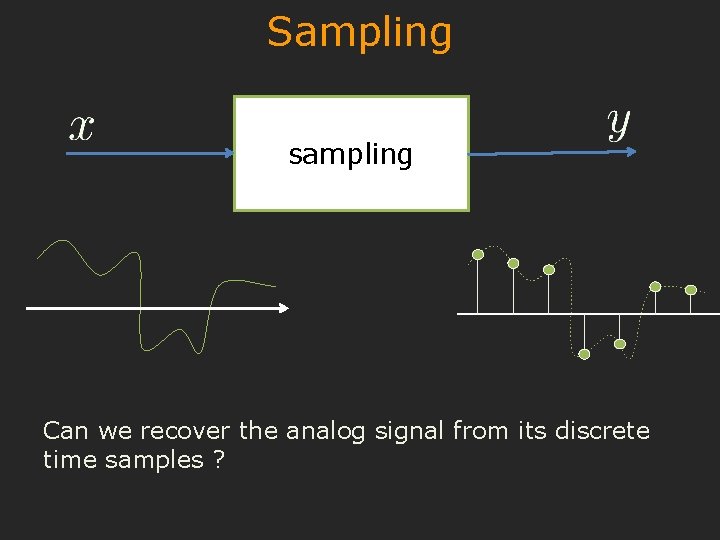
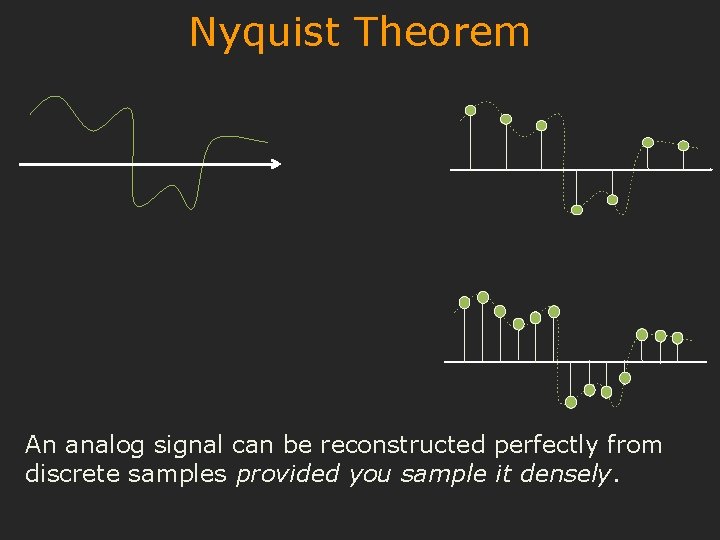
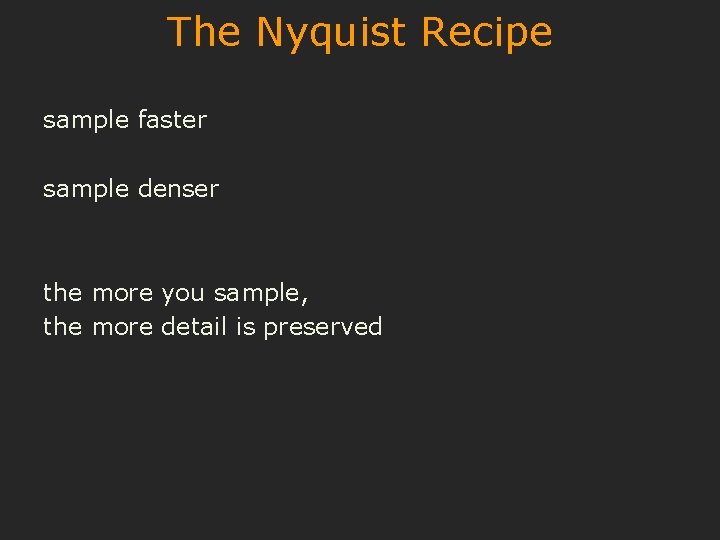
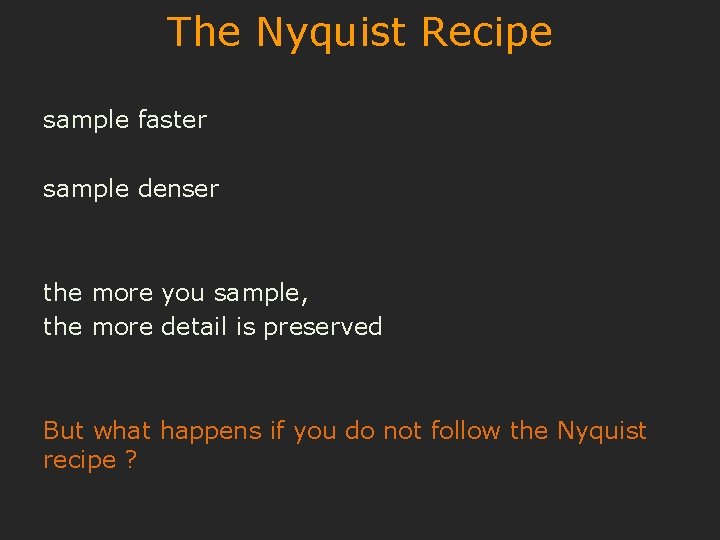
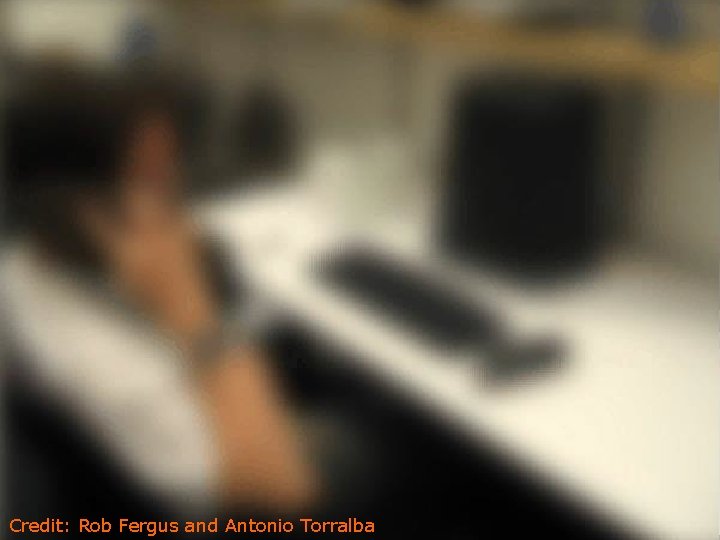
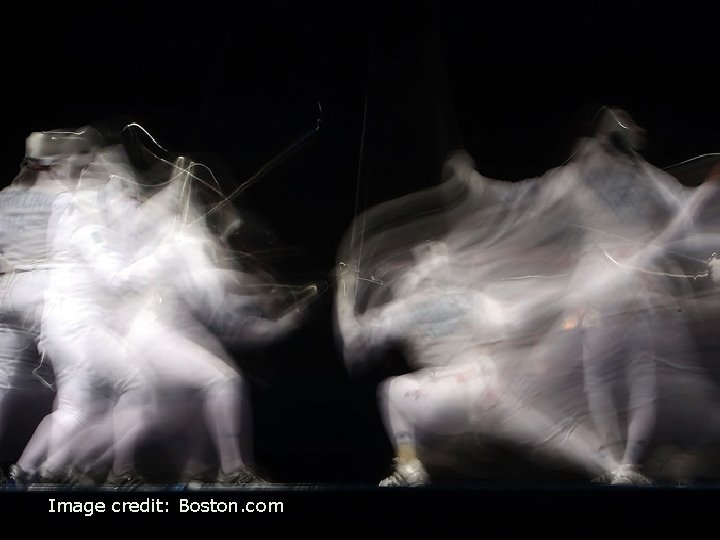
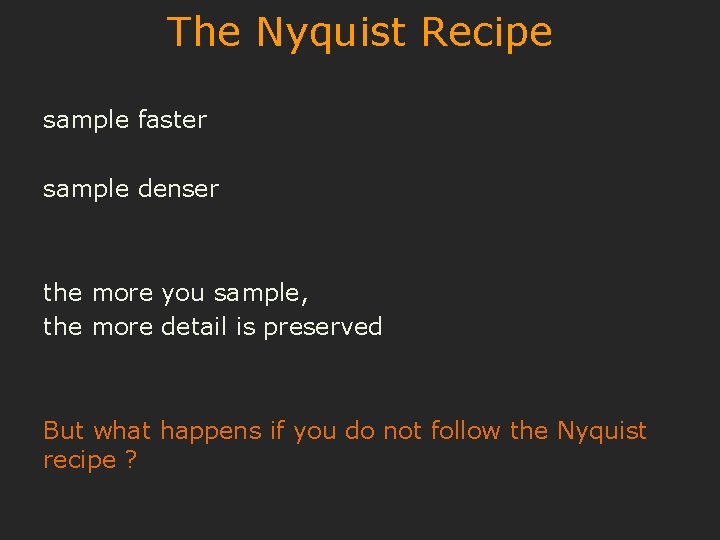
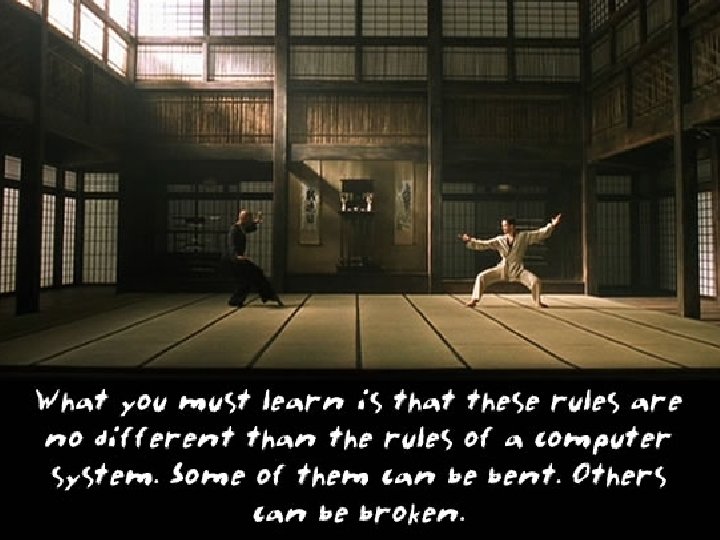
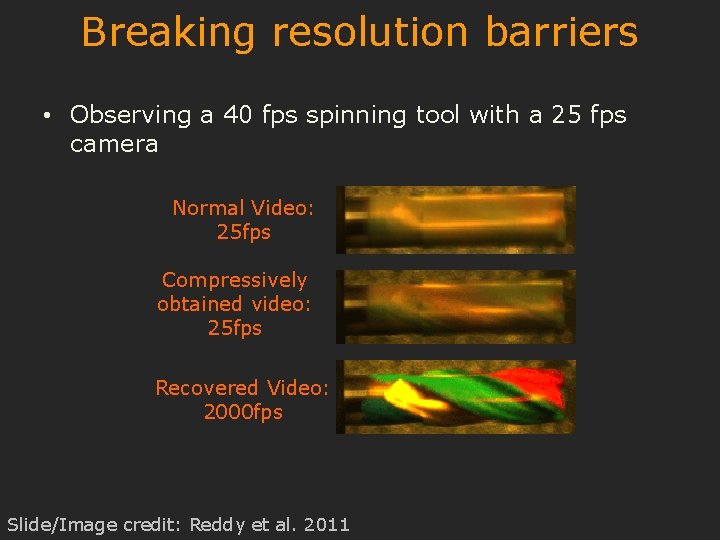
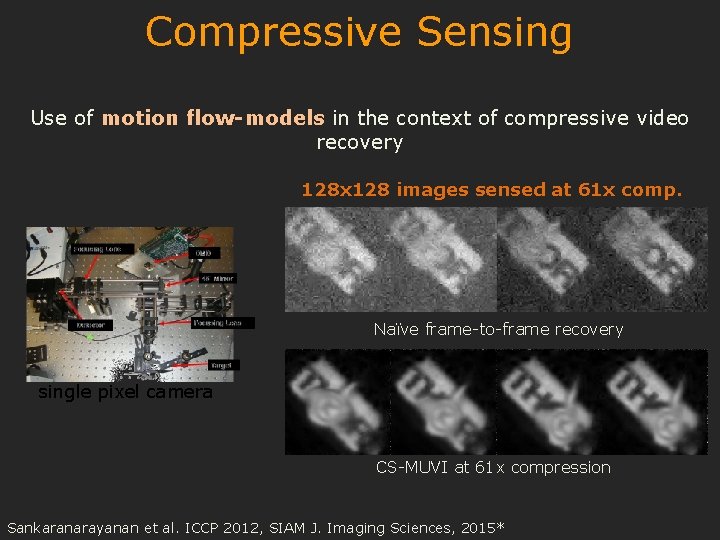
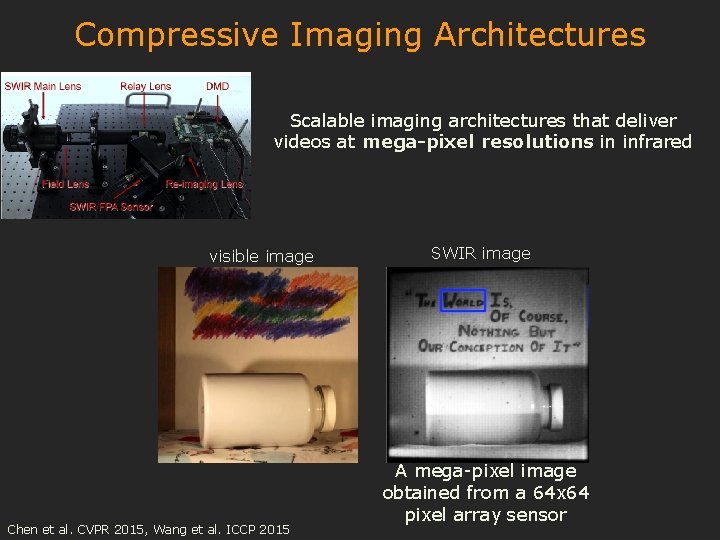
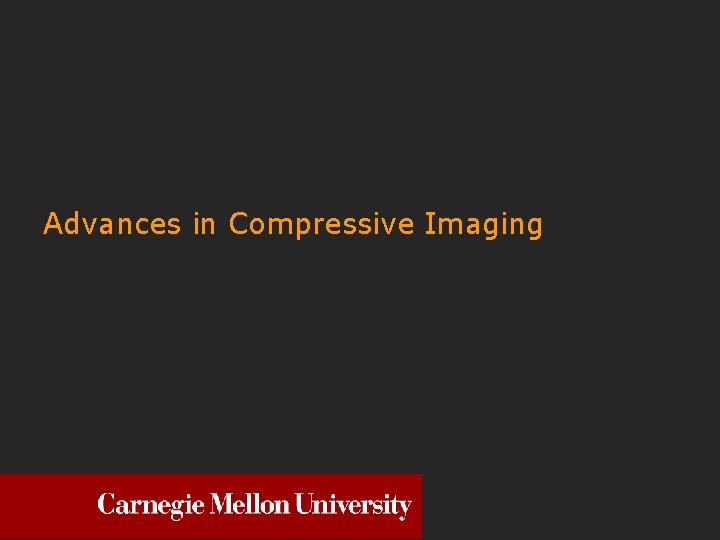
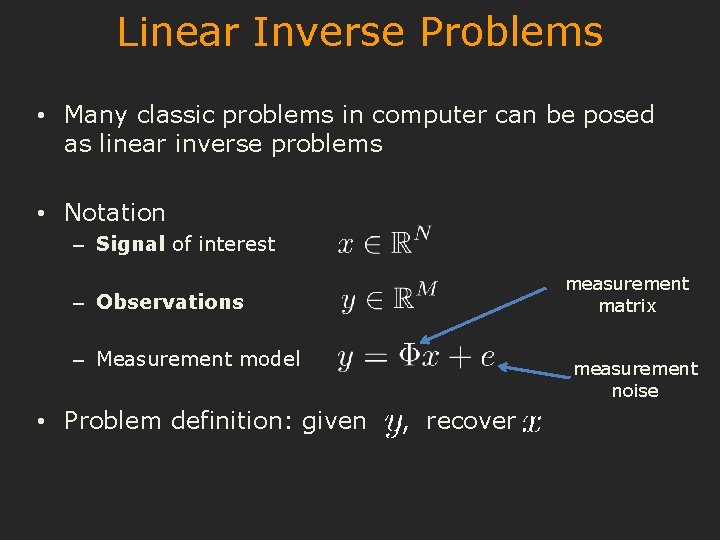
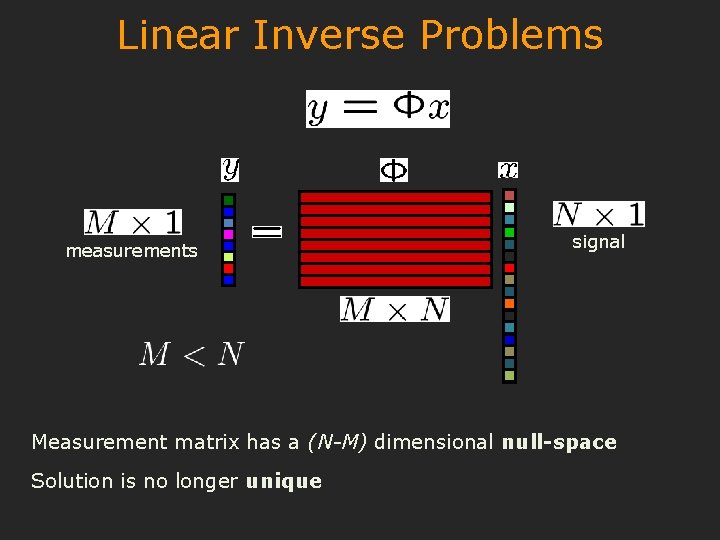
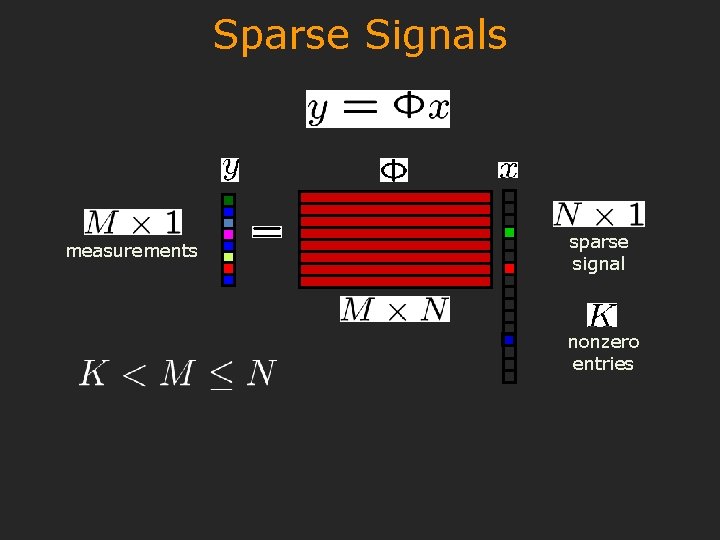
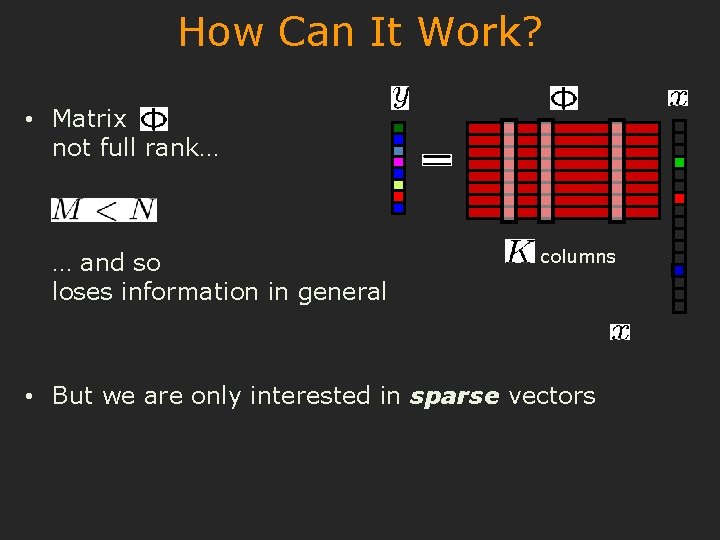
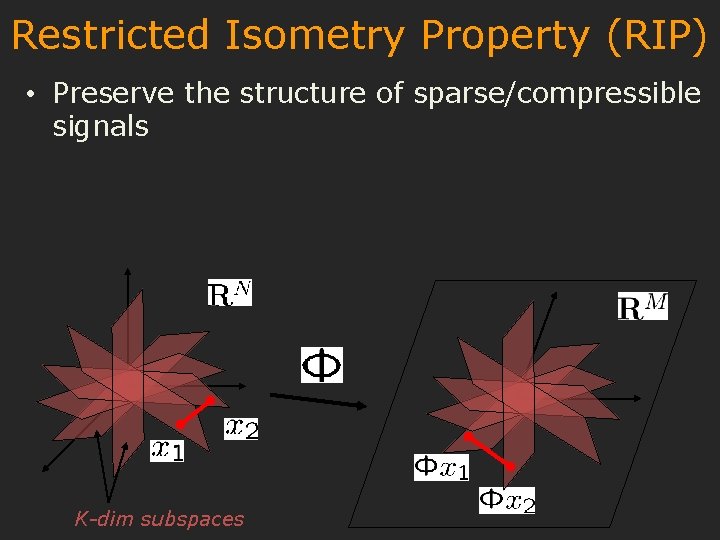
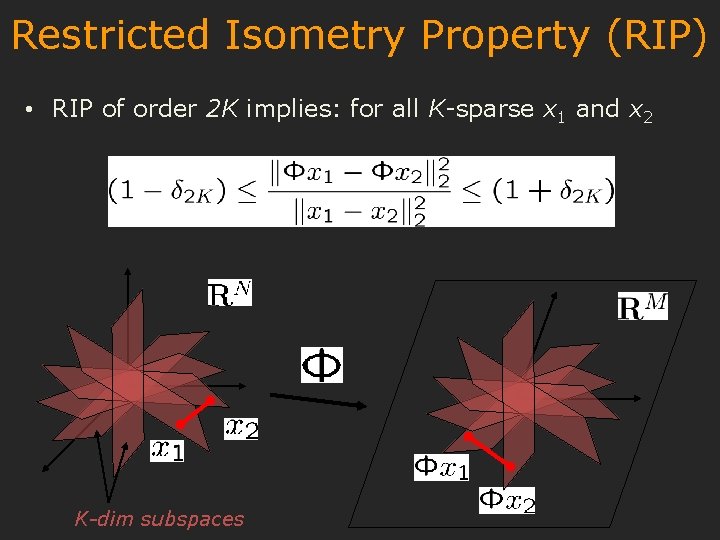
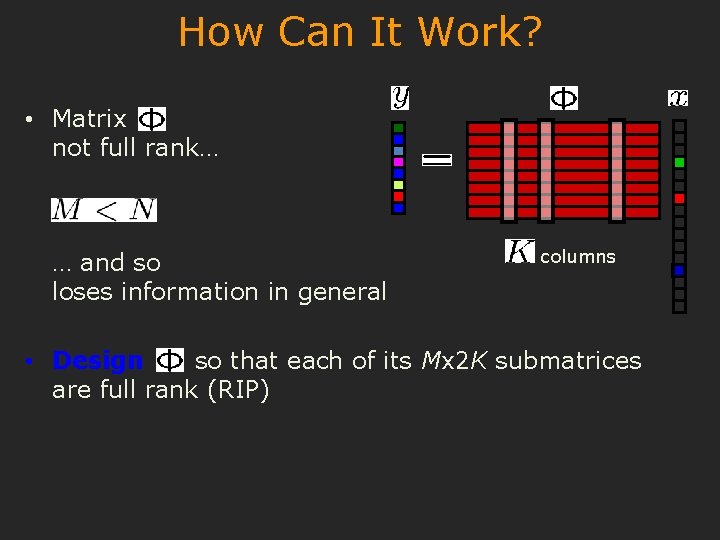
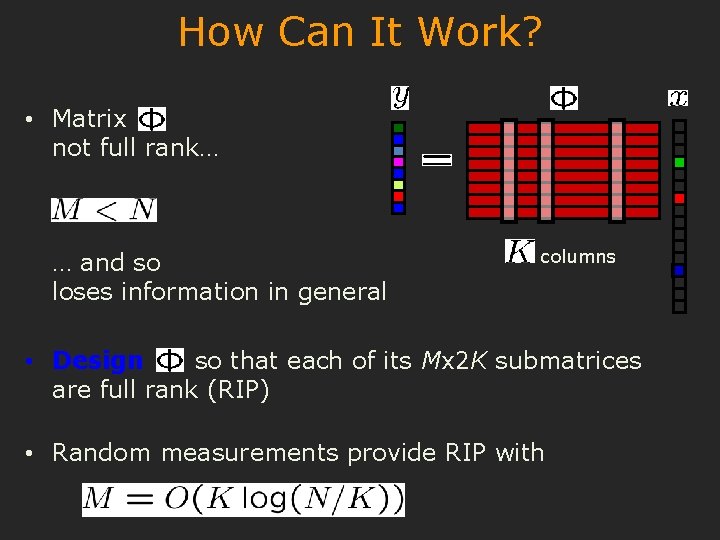
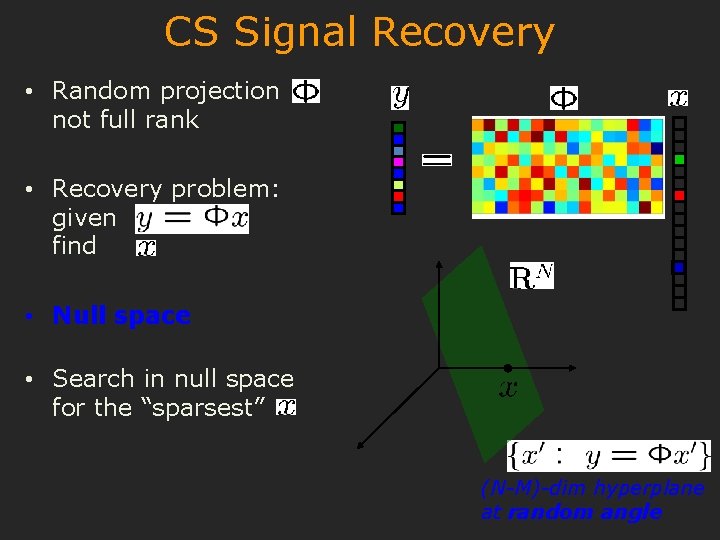
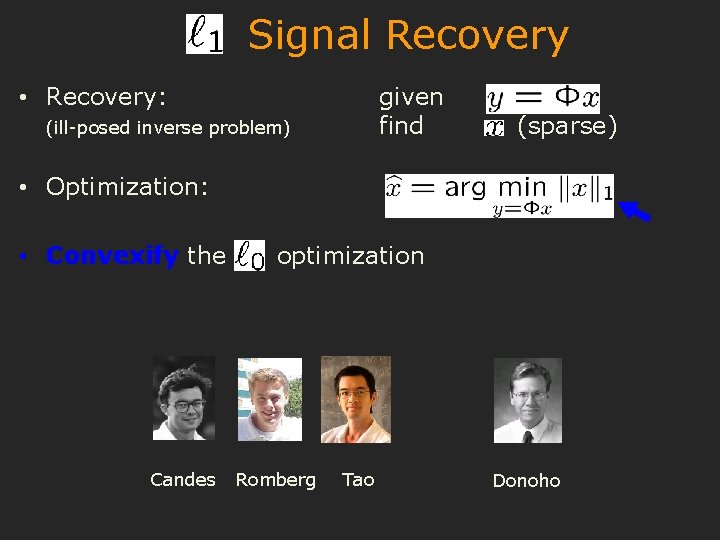
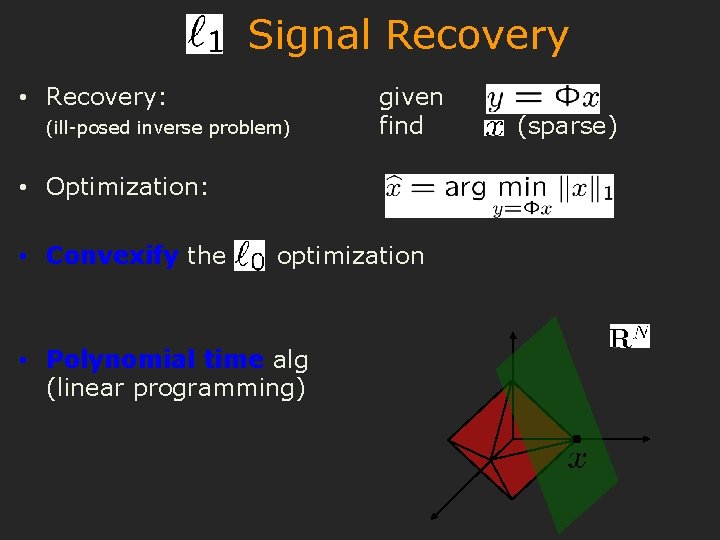
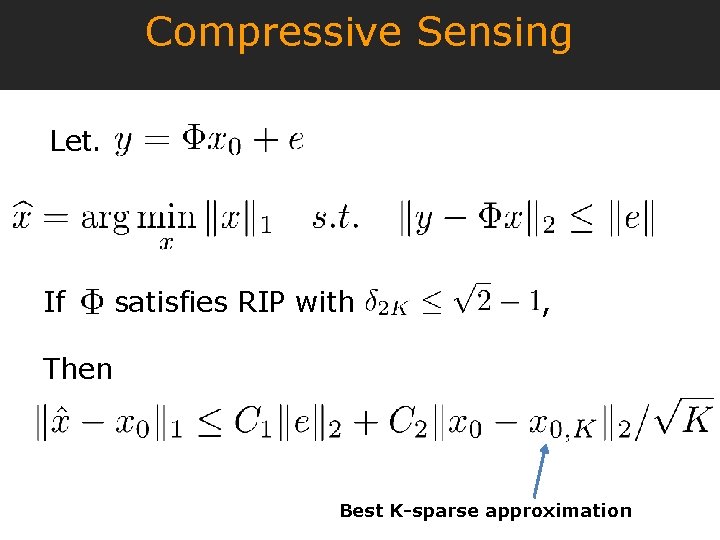
- Slides: 46
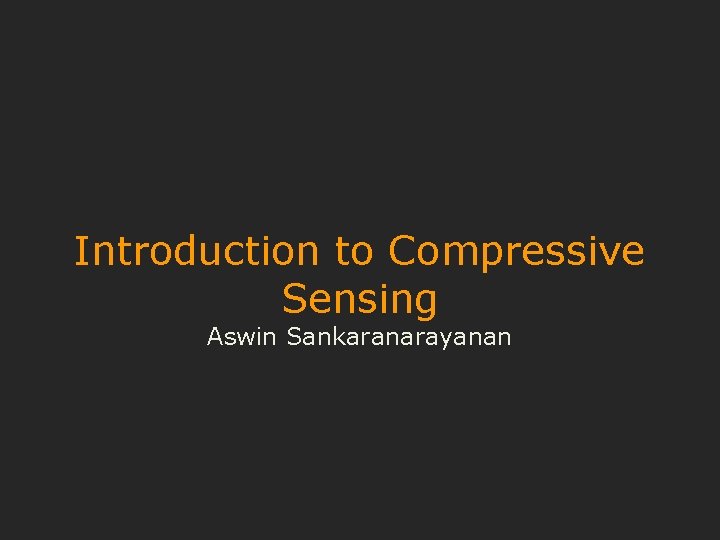
Introduction to Compressive Sensing Aswin Sankaranarayanan
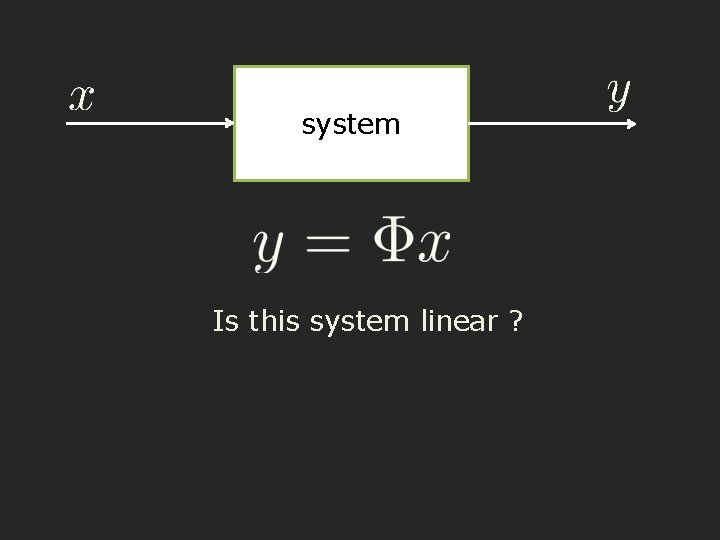
system Is this system linear ?
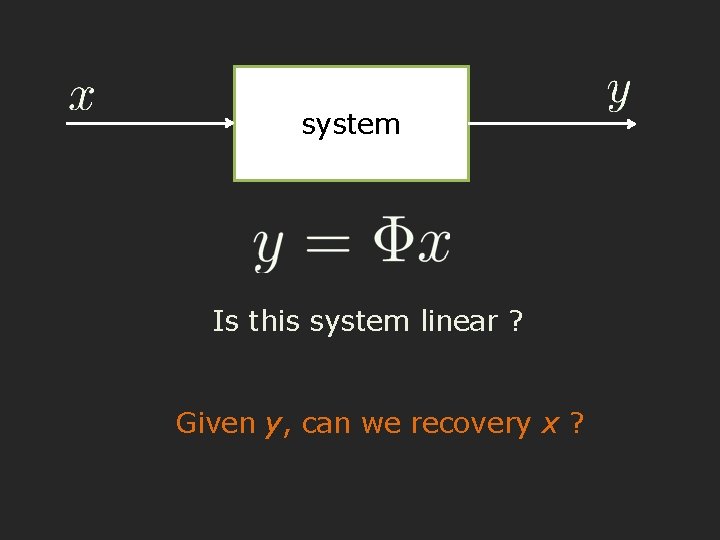
system Is this system linear ? Given y, can we recovery x ?
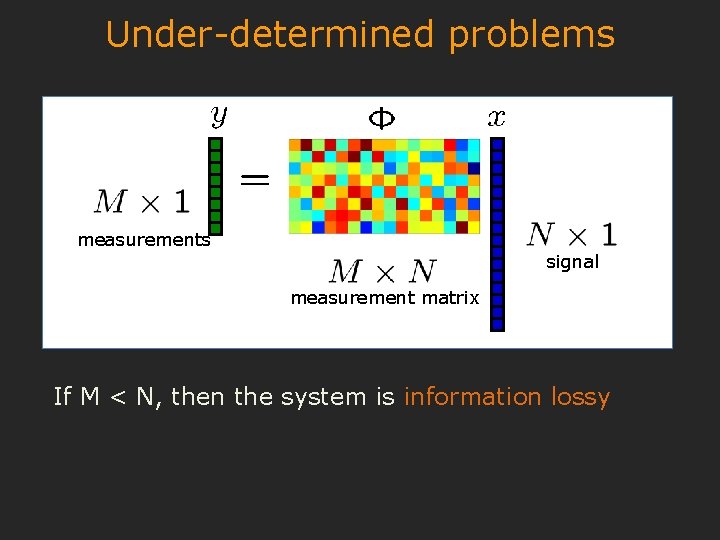
Under-determined problems measurements signal measurement matrix If M < N, then the system is information lossy
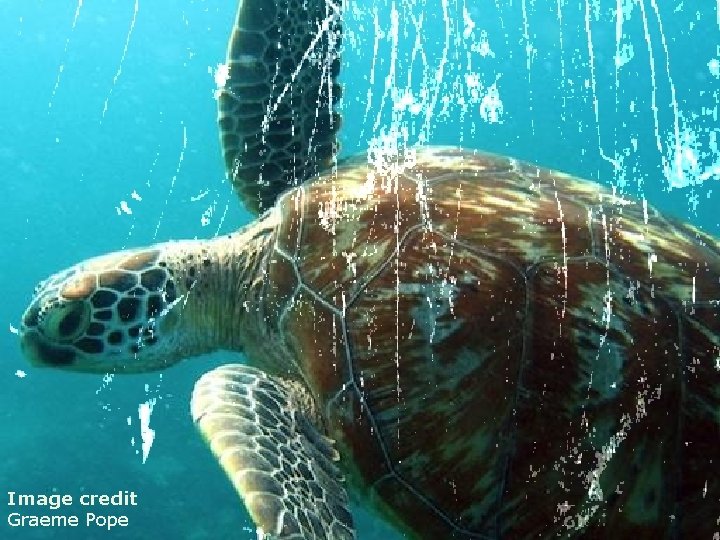
Image credit Graeme Pope
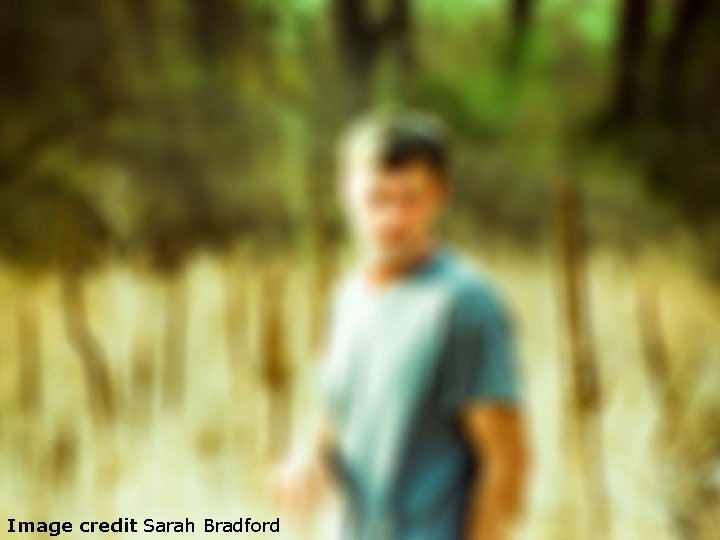
Image credit Sarah Bradford
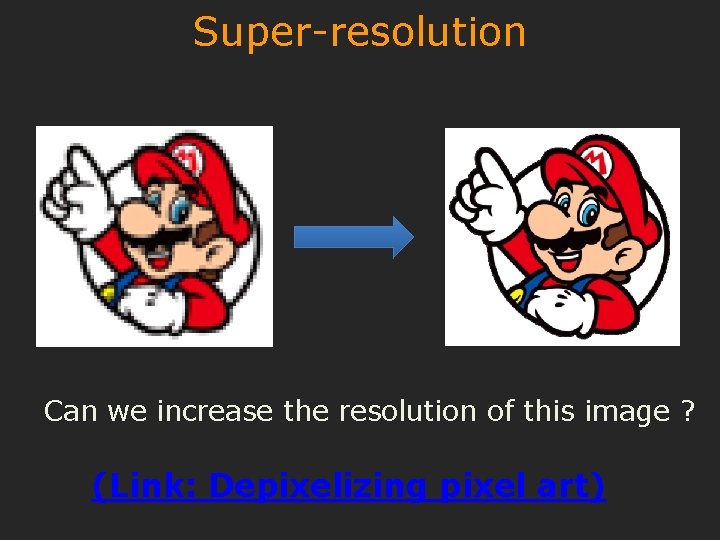
Super-resolution Can we increase the resolution of this image ? (Link: Depixelizing pixel art)
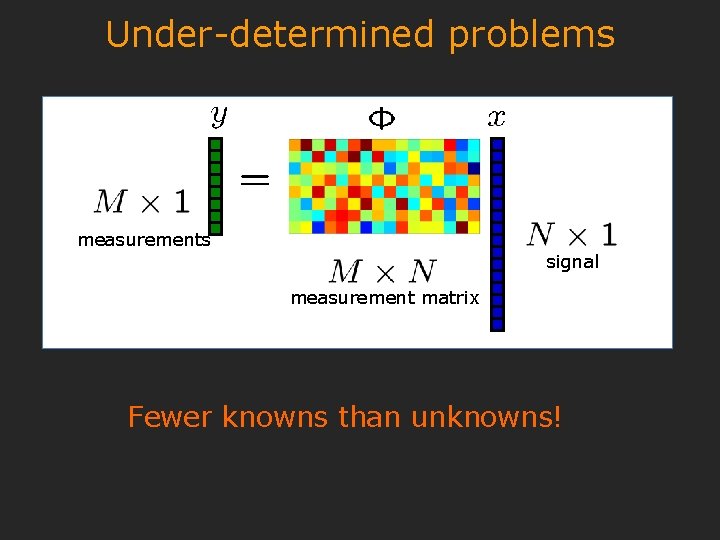
Under-determined problems measurements signal measurement matrix Fewer knowns than unknowns!
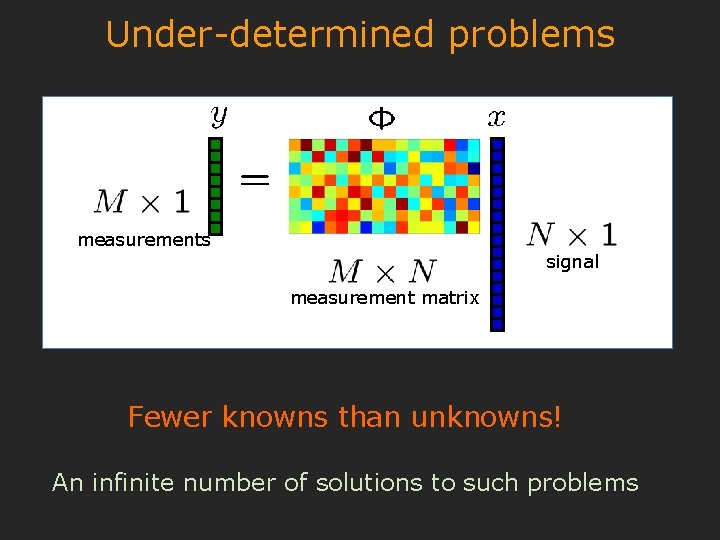
Under-determined problems measurements signal measurement matrix Fewer knowns than unknowns! An infinite number of solutions to such problems
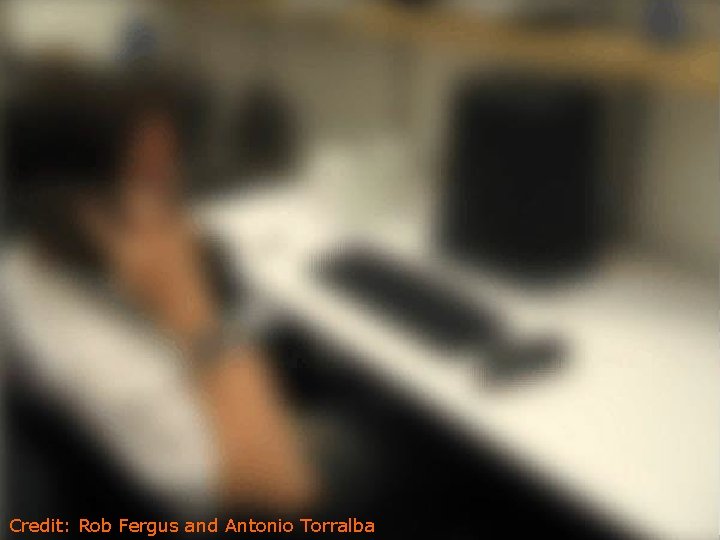
Credit: Rob Fergus and Antonio Torralba
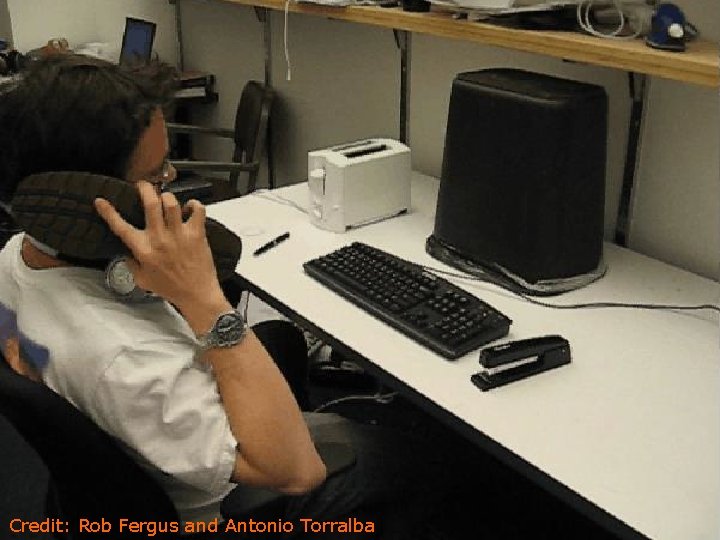
Credit: Rob Fergus and Antonio Torralba
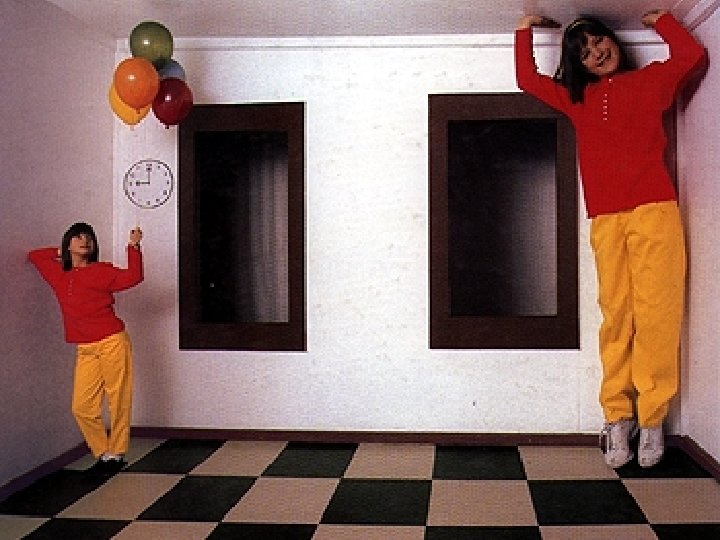
Ames Room
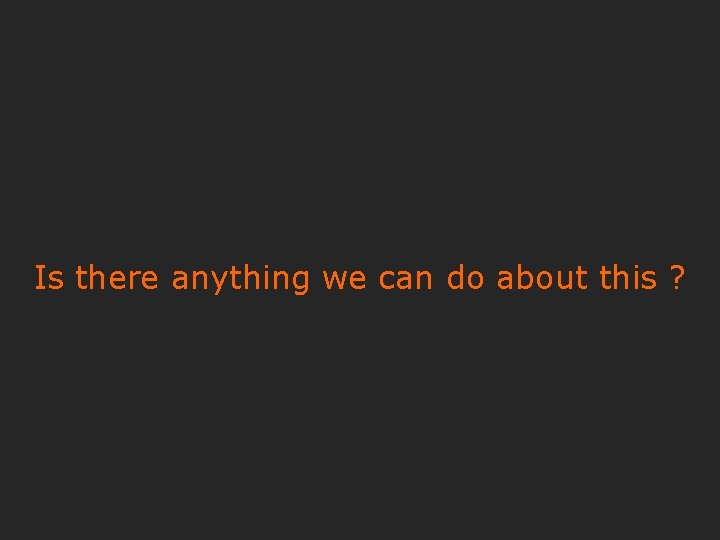
Is there anything we can do about this ?
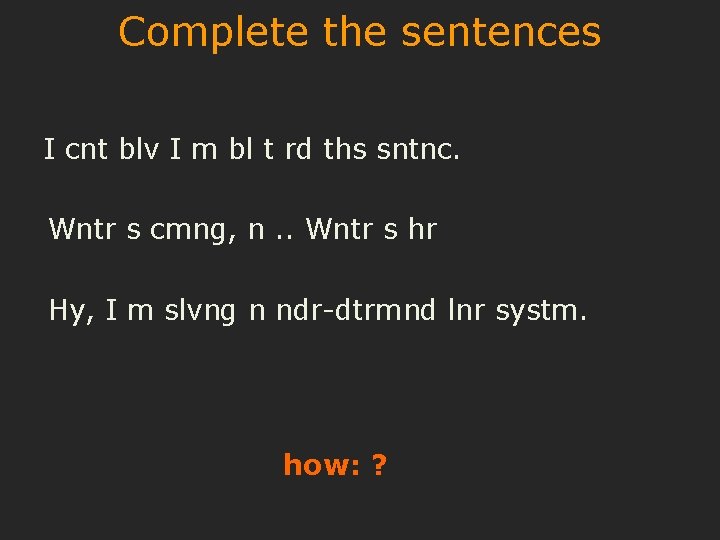
Complete the sentences I cnt blv I m bl t rd ths sntnc. Wntr s cmng, n. . Wntr s hr Hy, I m slvng n ndr-dtrmnd lnr systm. how: ?
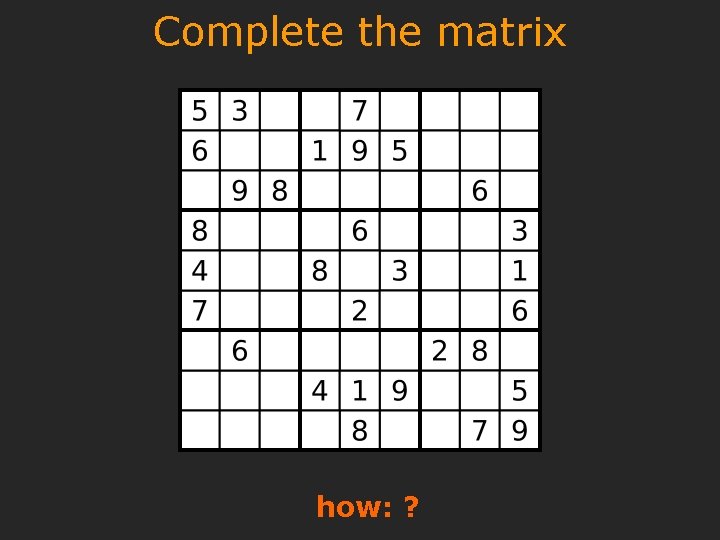
Complete the matrix how: ?
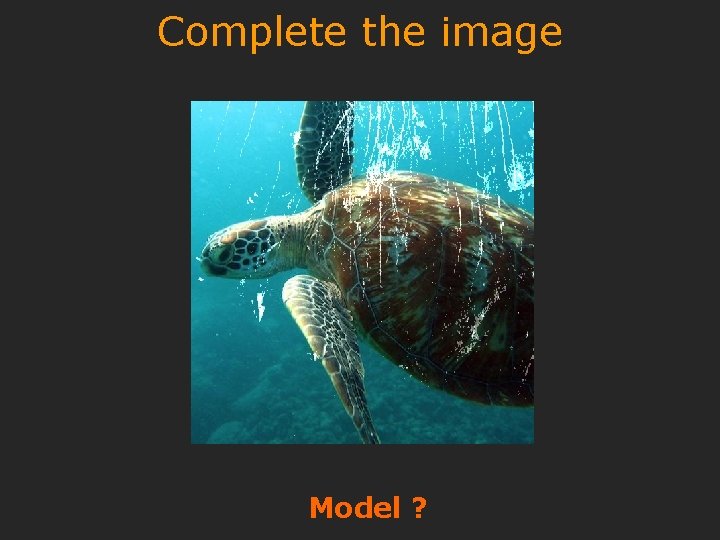
Complete the image Model ?
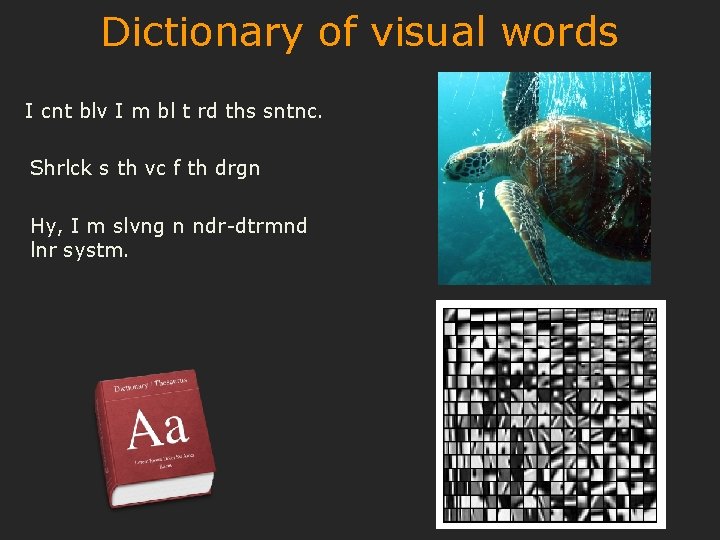
Dictionary of visual words I cnt blv I m bl t rd ths sntnc. Shrlck s th vc f th drgn Hy, I m slvng n ndr-dtrmnd lnr systm.
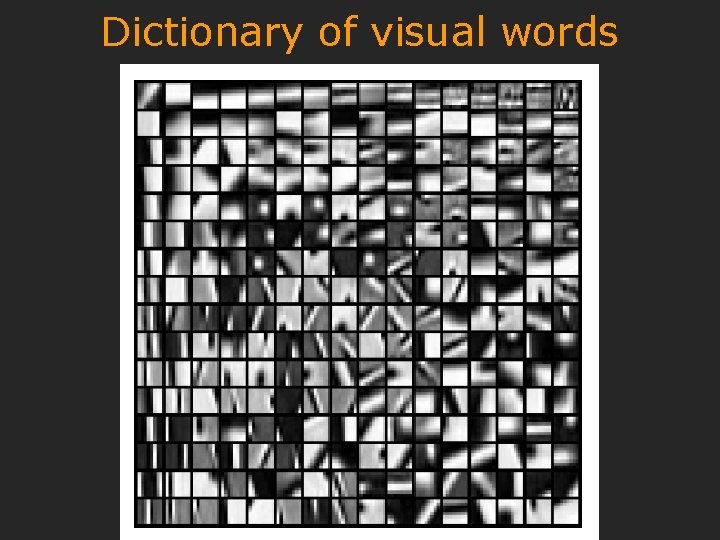
Dictionary of visual words
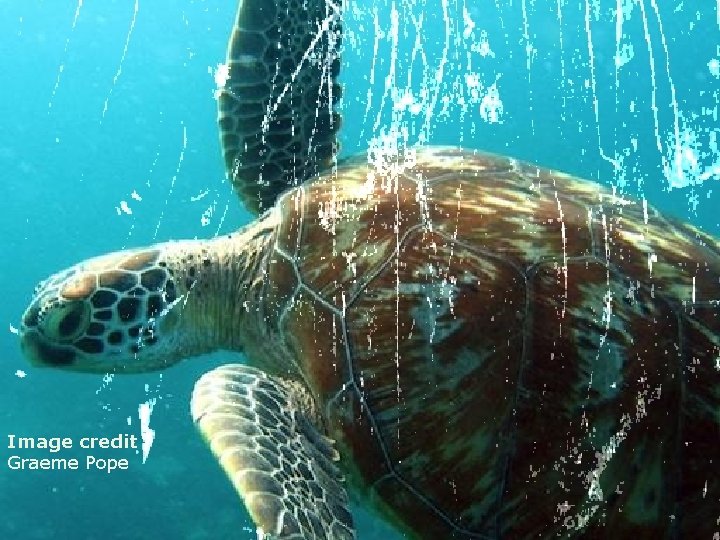
Image credit Graeme Pope
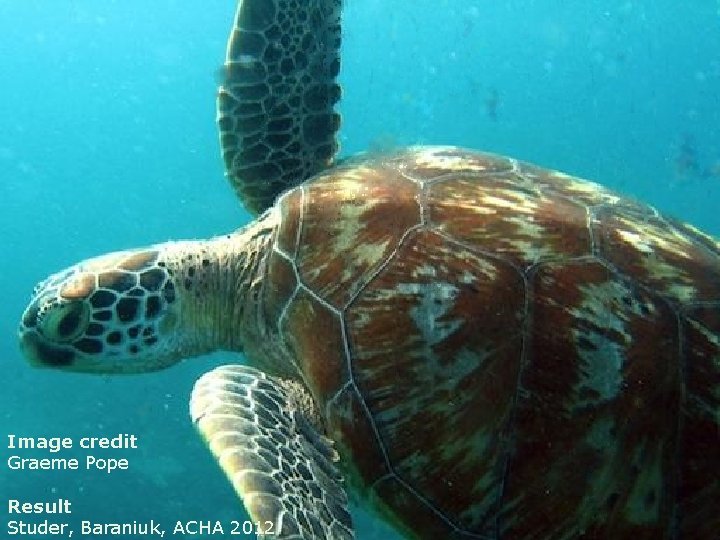
Image credit Graeme Pope Result Studer, Baraniuk, ACHA 2012
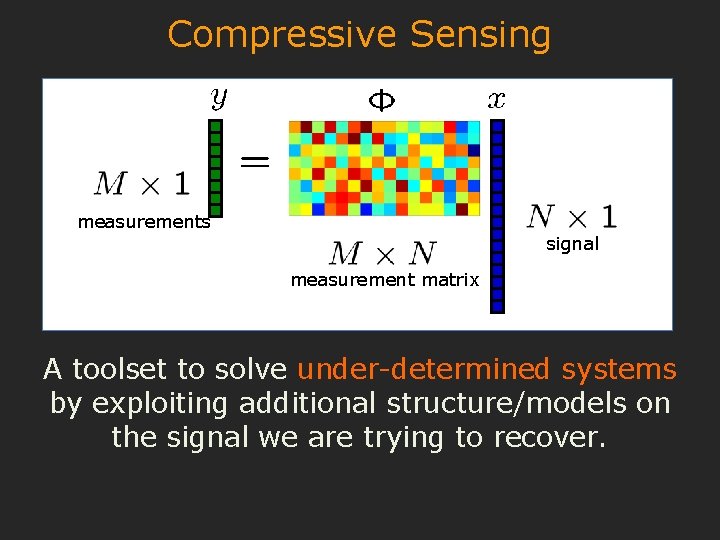
Compressive Sensing measurements signal measurement matrix A toolset to solve under-determined systems by exploiting additional structure/models on the signal we are trying to recover.
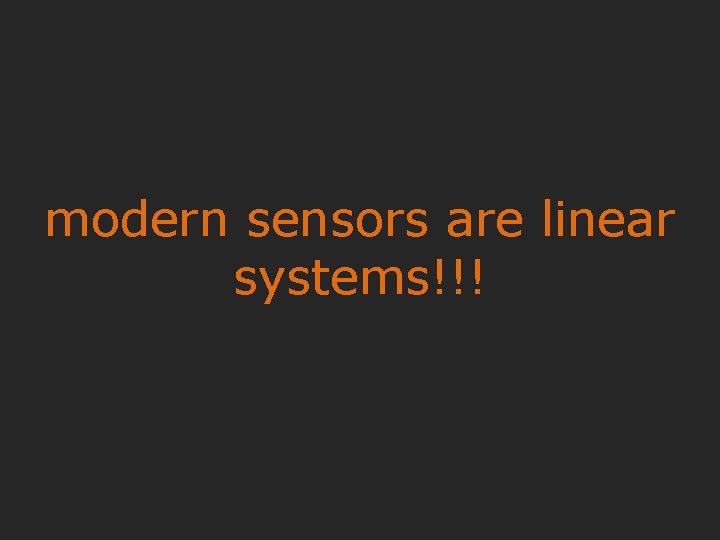
modern sensors are linear systems!!!
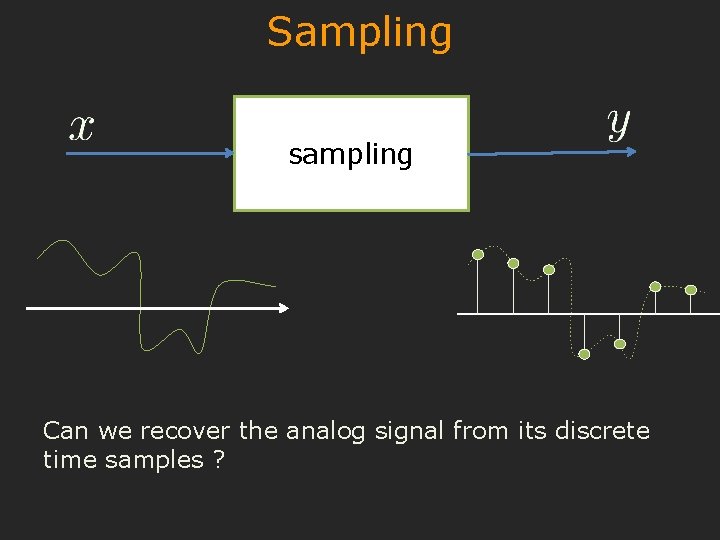
Sampling sampling Can we recover the analog signal from its discrete time samples ?
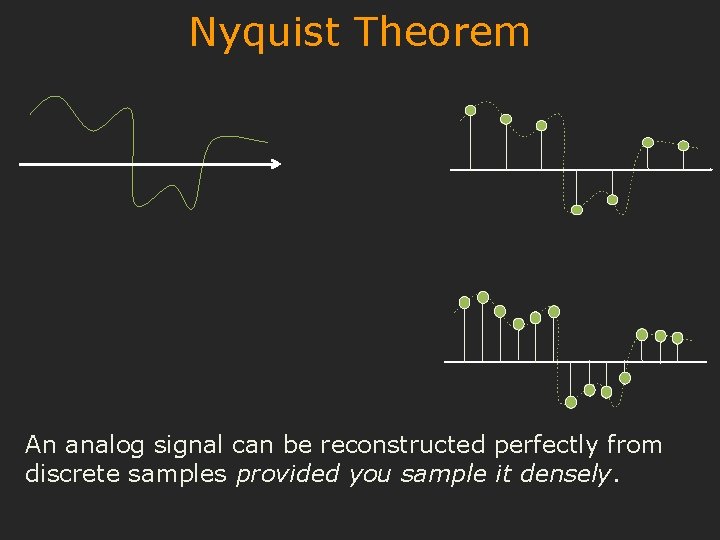
Nyquist Theorem An analog signal can be reconstructed perfectly from discrete samples provided you sample it densely.
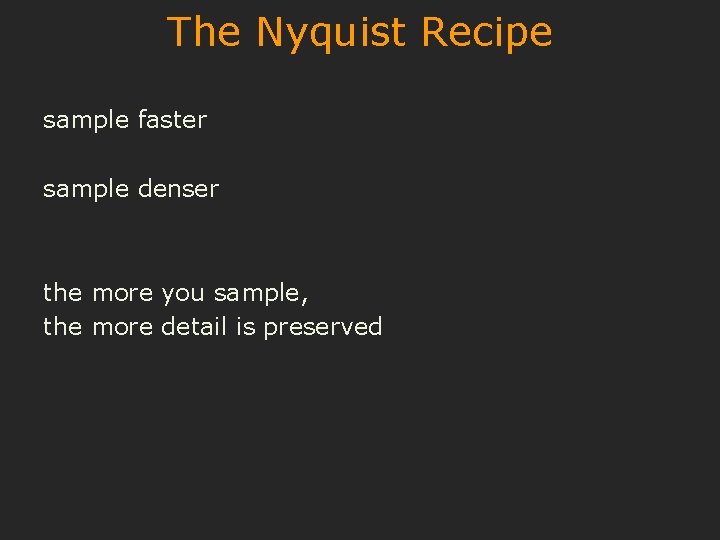
The Nyquist Recipe sample faster sample denser the more you sample, the more detail is preserved
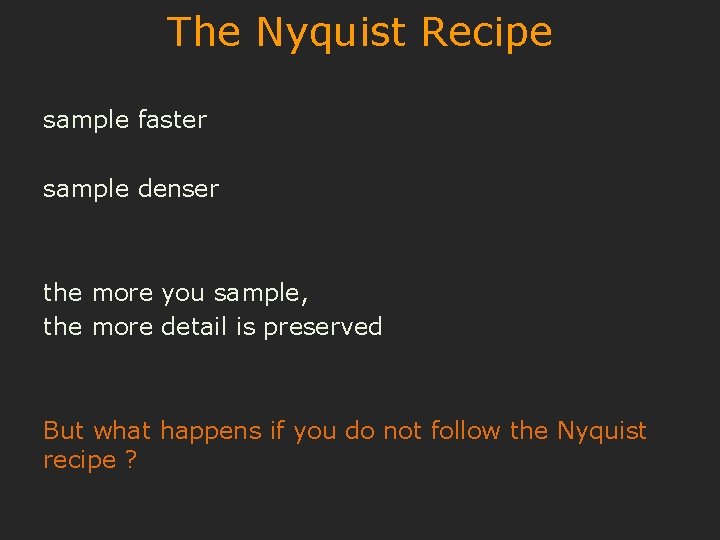
The Nyquist Recipe sample faster sample denser the more you sample, the more detail is preserved But what happens if you do not follow the Nyquist recipe ?
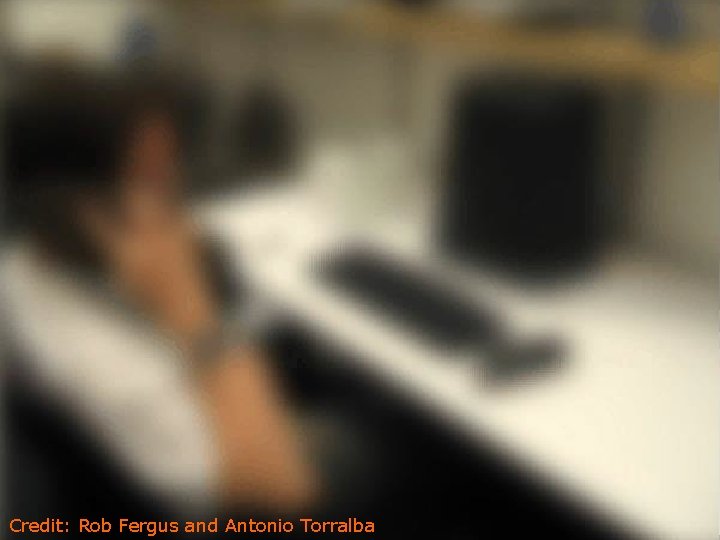
Credit: Rob Fergus and Antonio Torralba
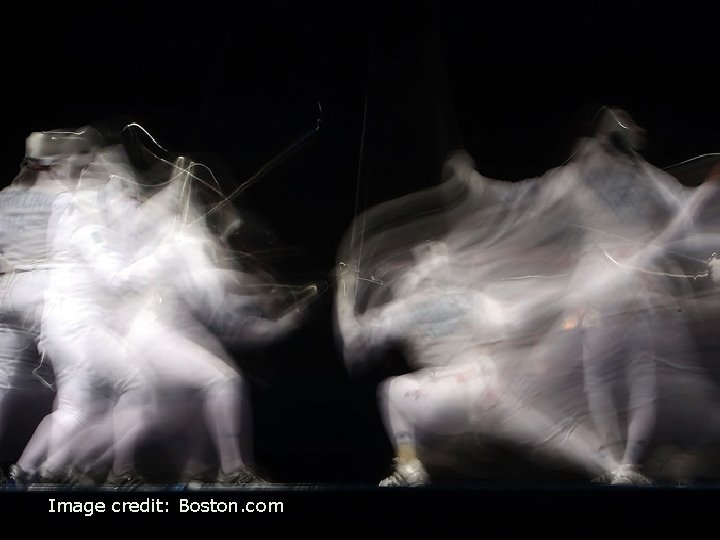
Image credit: Boston. com
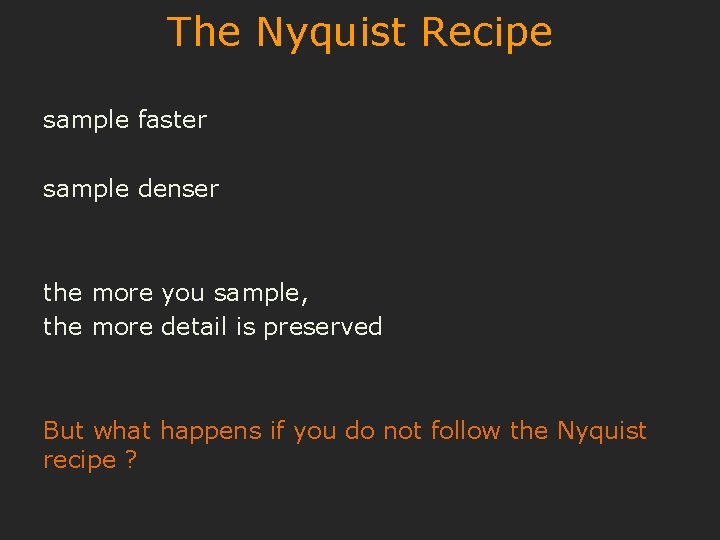
The Nyquist Recipe sample faster sample denser the more you sample, the more detail is preserved But what happens if you do not follow the Nyquist recipe ?
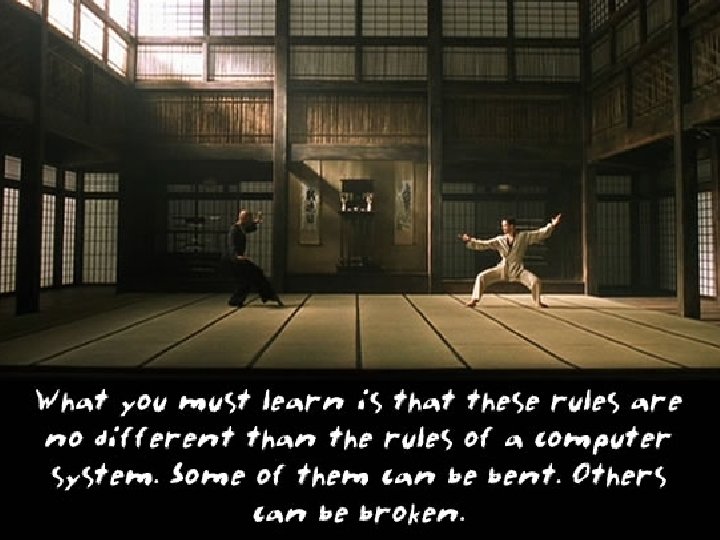
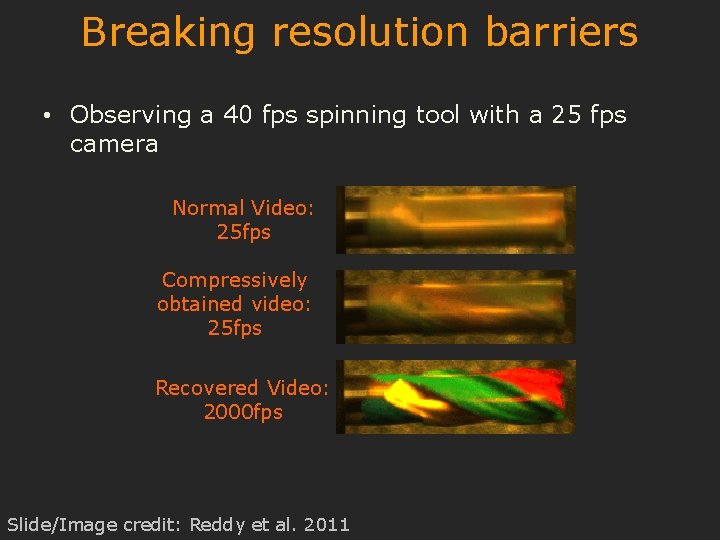
Breaking resolution barriers • Observing a 40 fps spinning tool with a 25 fps camera Normal Video: 25 fps Compressively obtained video: 25 fps Recovered Video: 2000 fps Slide/Image credit: Reddy et al. 2011
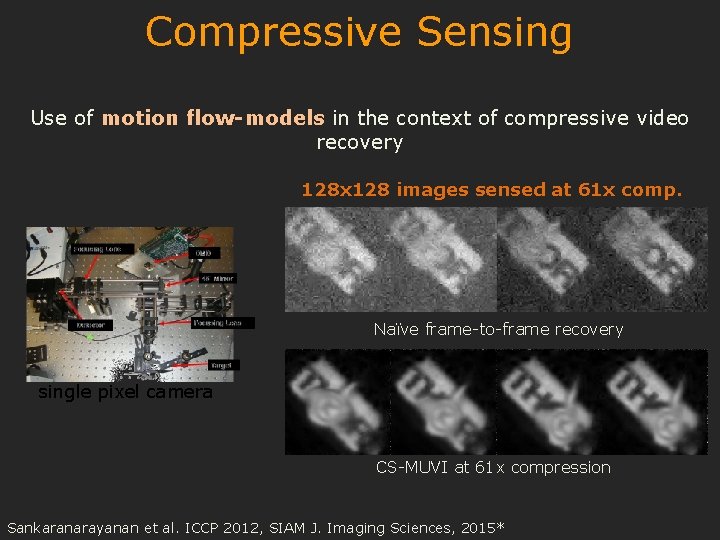
Compressive Sensing Use of motion flow-models in the context of compressive video recovery 128 x 128 images sensed at 61 x comp. Naïve frame-to-frame recovery single pixel camera CS-MUVI at 61 x compression Sankaranarayanan et al. ICCP 2012, SIAM J. Imaging Sciences, 2015*
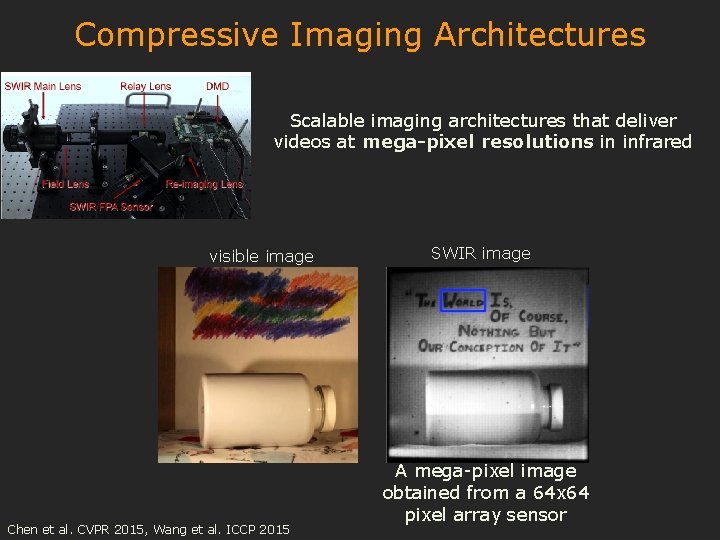
Compressive Imaging Architectures Scalable imaging architectures that deliver videos at mega-pixel resolutions in infrared visible image Chen et al. CVPR 2015, Wang et al. ICCP 2015 SWIR image A mega-pixel image obtained from a 64 x 64 pixel array sensor
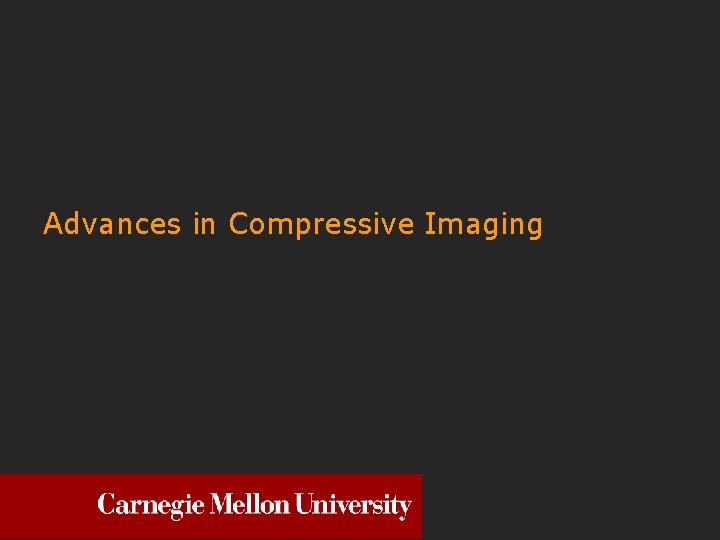
Advances in Compressive Imaging
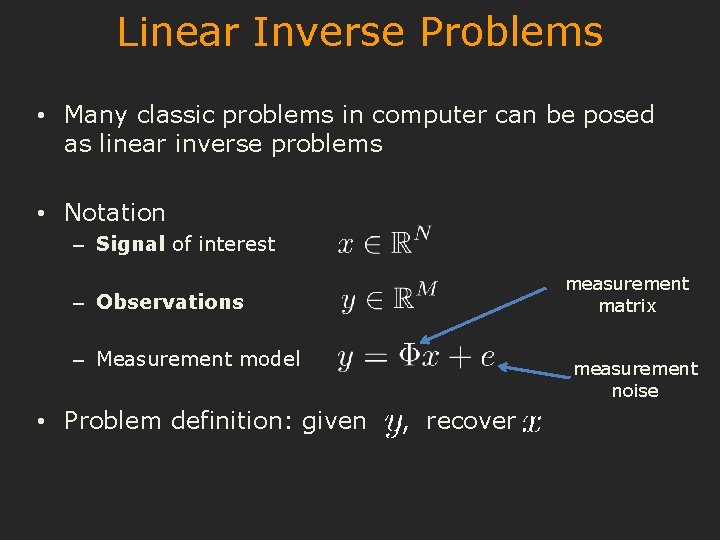
Linear Inverse Problems • Many classic problems in computer can be posed as linear inverse problems • Notation – Signal of interest measurement matrix – Observations – Measurement model • Problem definition: given measurement noise , recover
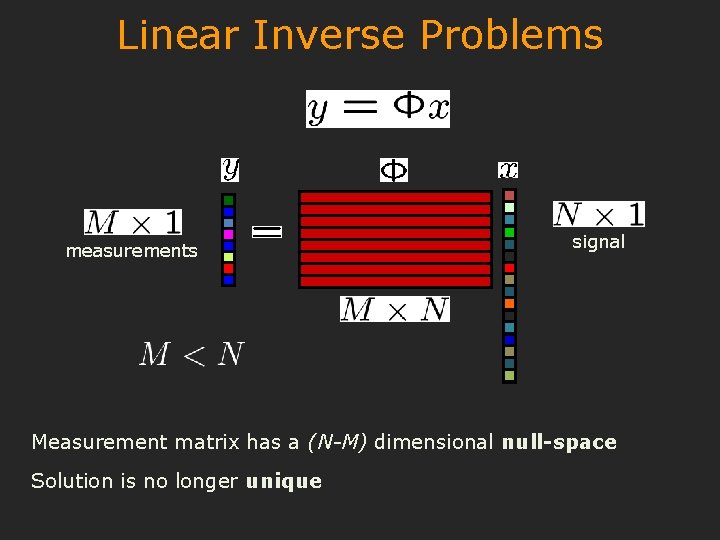
Linear Inverse Problems measurements signal Measurement matrix has a (N-M) dimensional null-space Solution is no longer unique
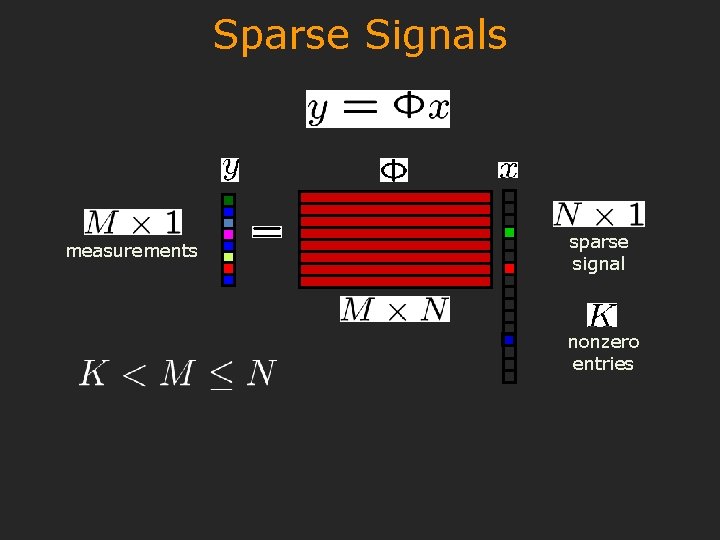
Sparse Signals measurements sparse signal nonzero entries
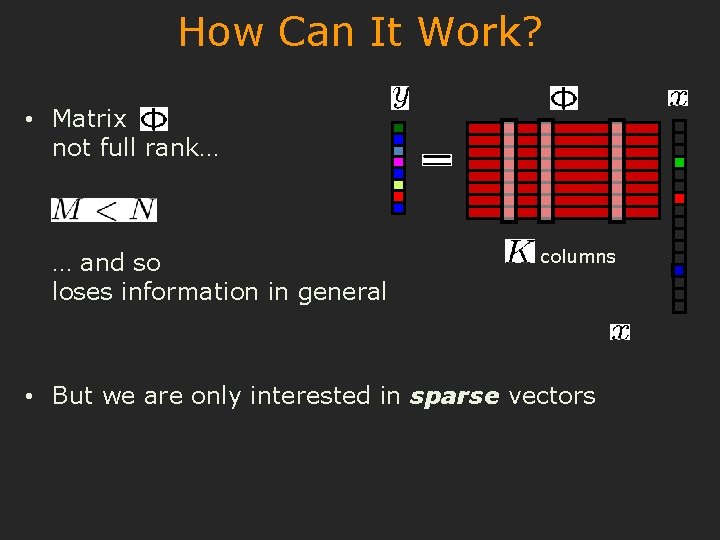
How Can It Work? • Matrix not full rank… … and so loses information in general columns • But we are only interested in sparse vectors
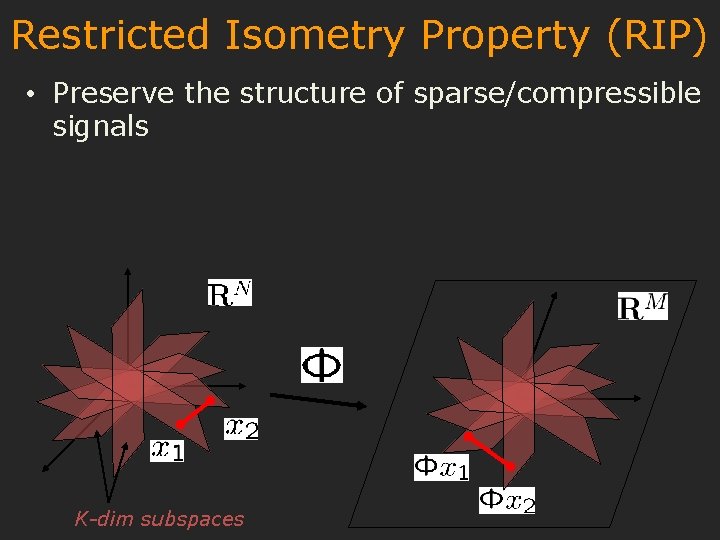
Restricted Isometry Property (RIP) • Preserve the structure of sparse/compressible signals K-dim subspaces
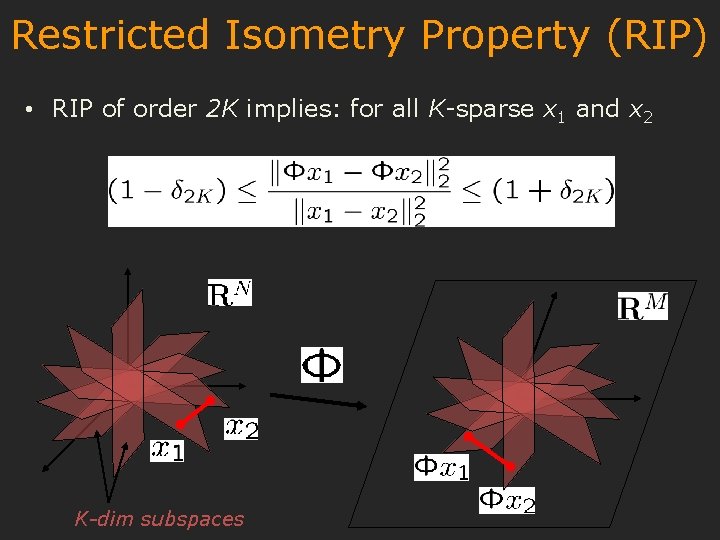
Restricted Isometry Property (RIP) • RIP of order 2 K implies: for all K-sparse x 1 and x 2 K-dim subspaces
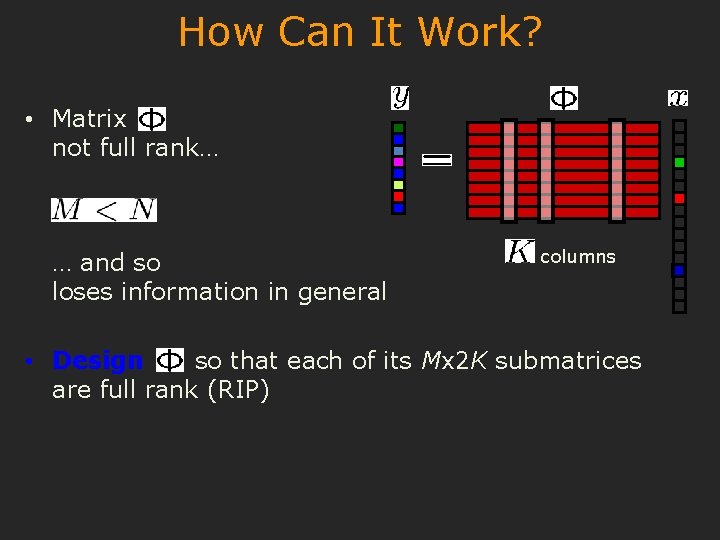
How Can It Work? • Matrix not full rank… … and so loses information in general columns • Design so that each of its Mx 2 K submatrices are full rank (RIP)
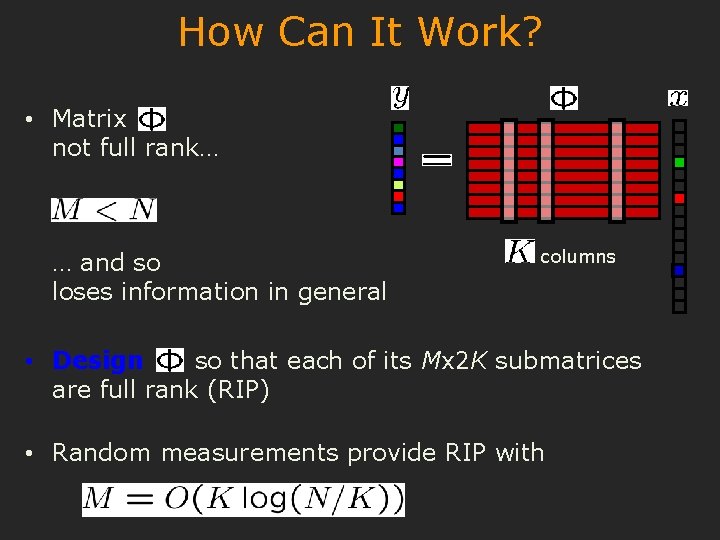
How Can It Work? • Matrix not full rank… … and so loses information in general columns • Design so that each of its Mx 2 K submatrices are full rank (RIP) • Random measurements provide RIP with
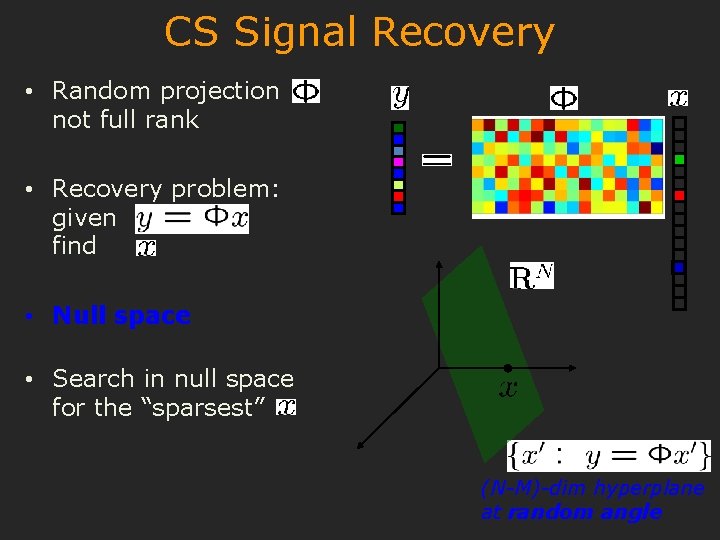
CS Signal Recovery • Random projection not full rank • Recovery problem: given find • Null space • Search in null space for the “sparsest” (N-M)-dim hyperplane at random angle
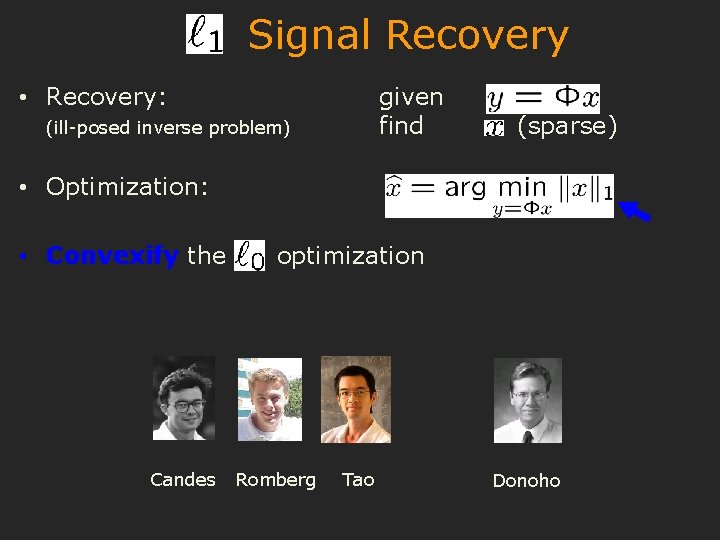
Signal Recovery • Recovery: given find (ill-posed inverse problem) (sparse) • Optimization: • Convexify the Candes optimization Romberg Tao Donoho
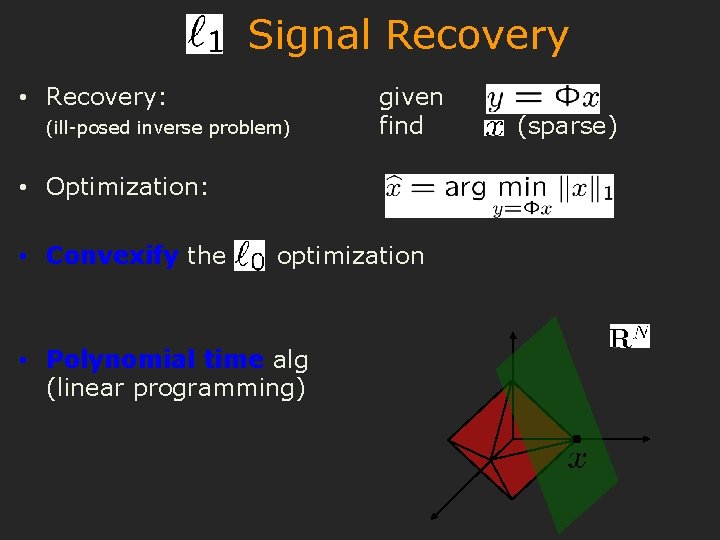
Signal Recovery • Recovery: (ill-posed inverse problem) given find • Optimization: • Convexify the optimization • Polynomial time alg (linear programming) (sparse)
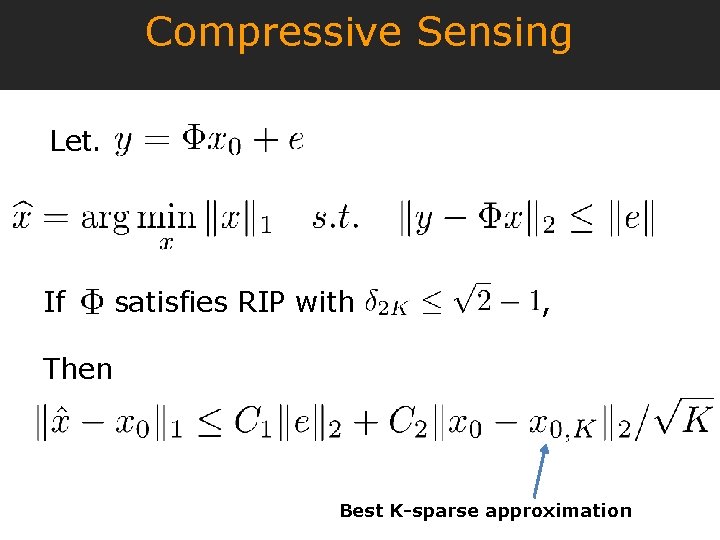
Compressive Sensing Let. If satisfies RIP with , Then Best K-sparse approximation
Aswin suharsono
Parasite that causes loeffler's syndrome
Aswin suharsono
Target strength of concrete
"usa spine care"
Which rock possesses very high compressive strength?
Introduction to microwave remote sensing
Ideal remote sensing system
Idealized remote sensing system
Limitations of remote sensing
Remote sensing platforms
Pressure sensing elements
Passive remote sensing
What is sensing
Carl jung intuition vs sensing
What is source of measurand
Digital number in remote sensing
Demand sensing and shaping
Limitations of remote sensing
Twiddler syndrome
Nbg pacemaker code
Aggregation definition ap human geography
Remote sensing ap human geo
Parallax in aerial photography
Strip camera in remote sensing
Nuvations
Remote sensing image
Ifov and fov in remote sensing
Elements of vertical photographs
Virtual carrier sensing
National authority for remote sensing and space sciences
The internship mbti
Fiber optic force sensing catheter
Market driven strategy
Automatic rain sensing wiper using arduino
Canadian centre for remote sensing
Geospatial data ap human geography
Remote sensing applications center
Remote sensing applications center
Remote sensing physics
Microwave remote sensing lecture notes
Remote sensing in precision agriculture
Aerial photography in remote sensing pdf
Remote sensing physics
Acquisition image
Tangential scale distortion
Cegeg