1 Logistic Regression Political Analysis 2 Week 8
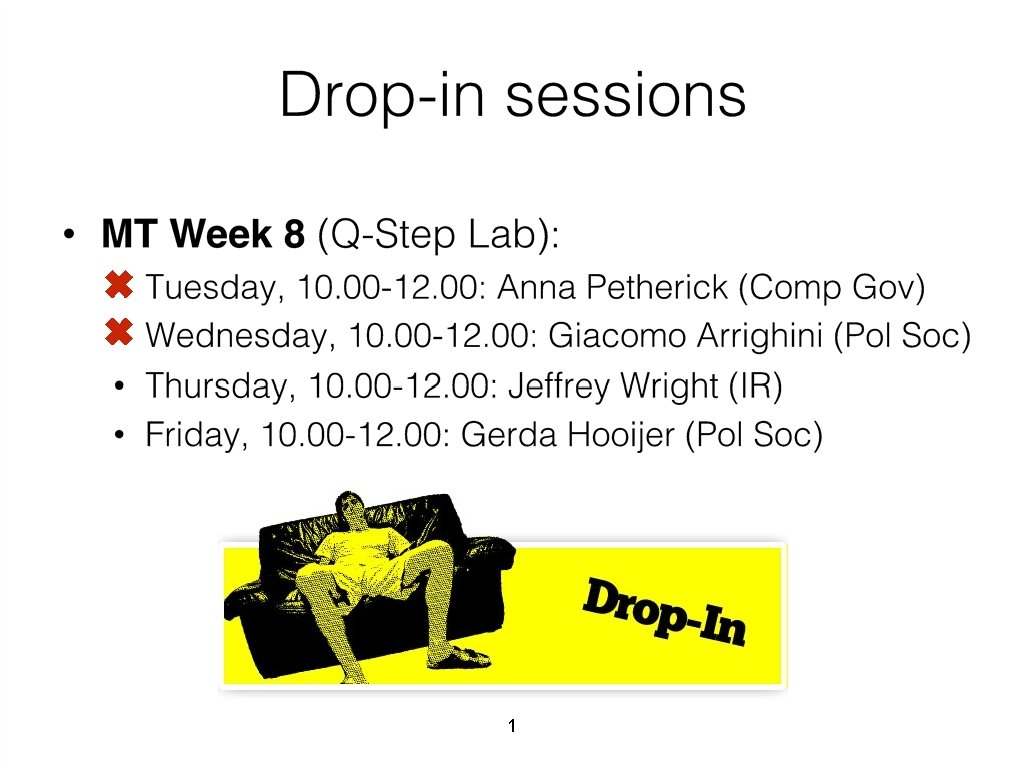
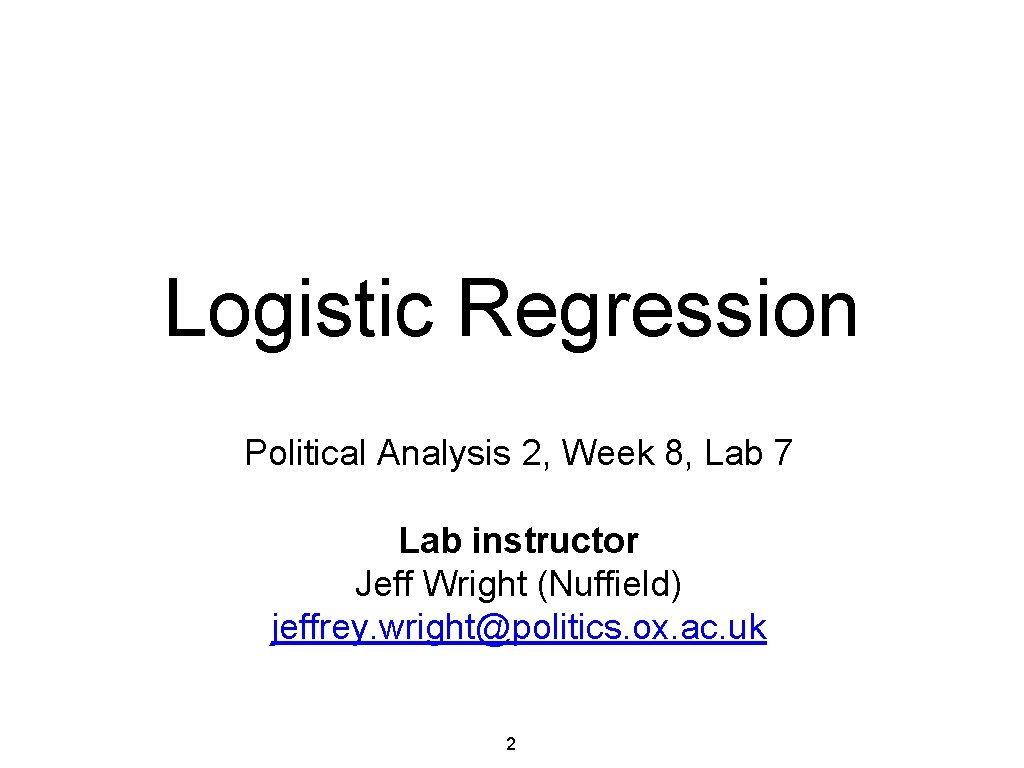
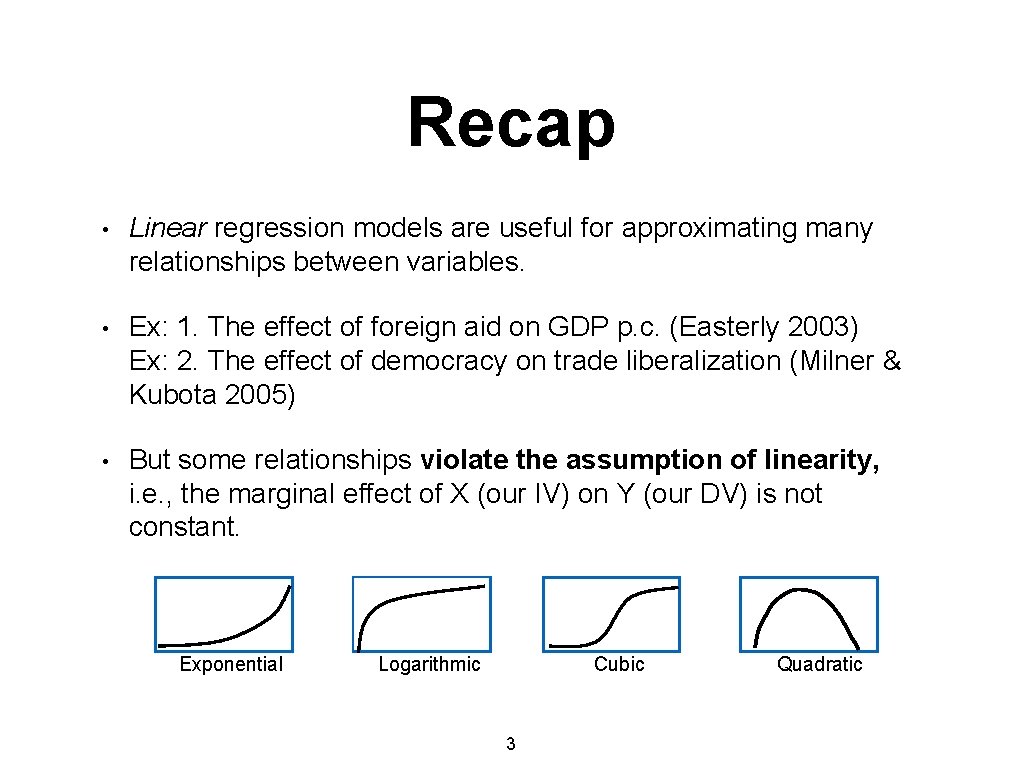
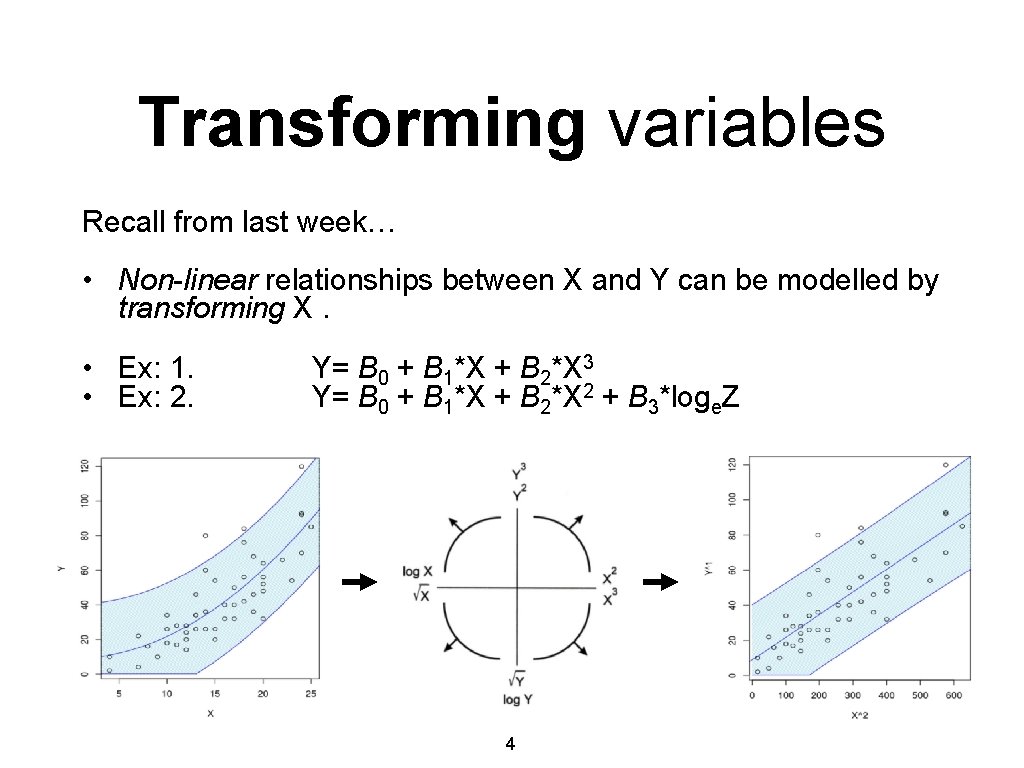
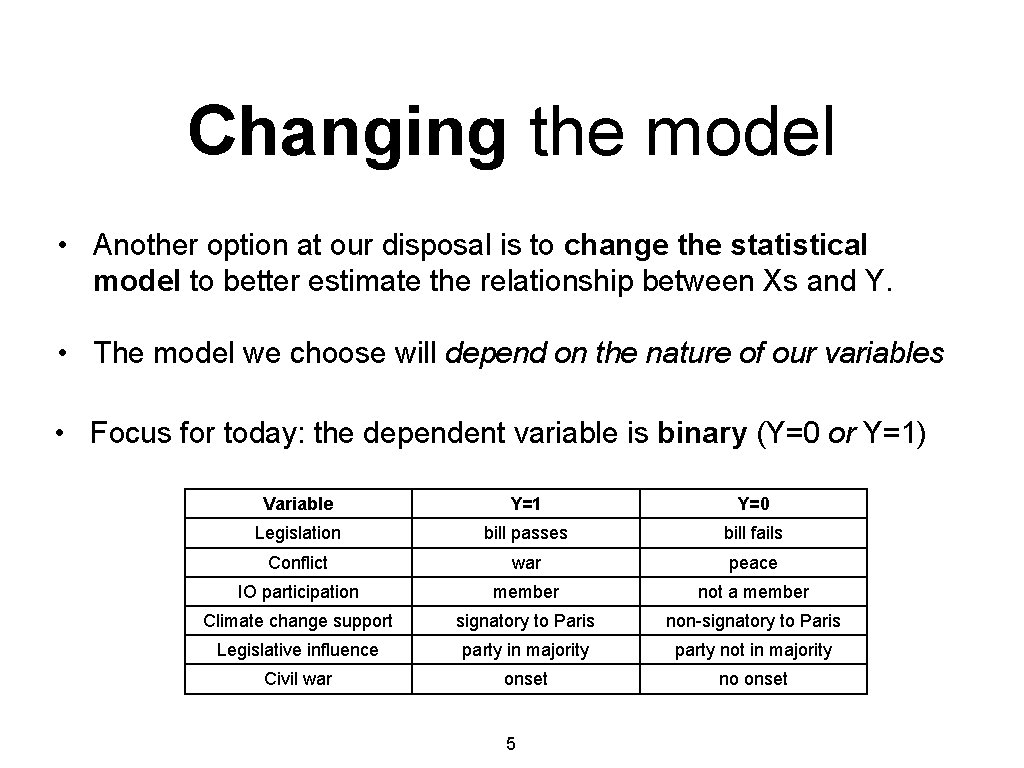
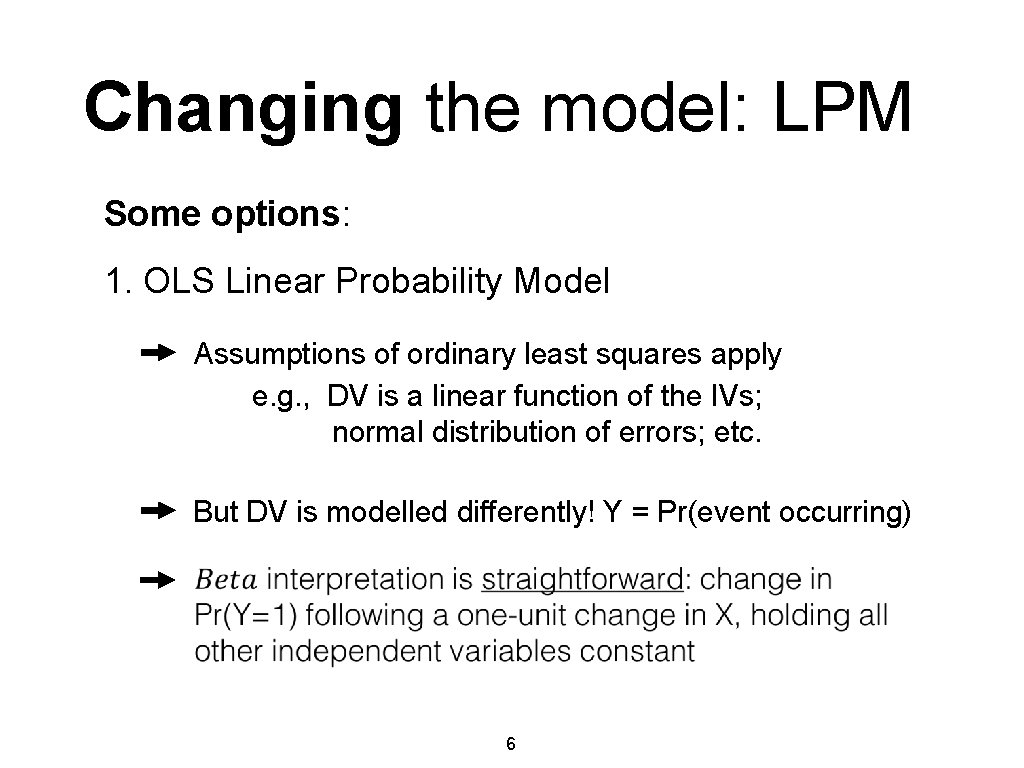
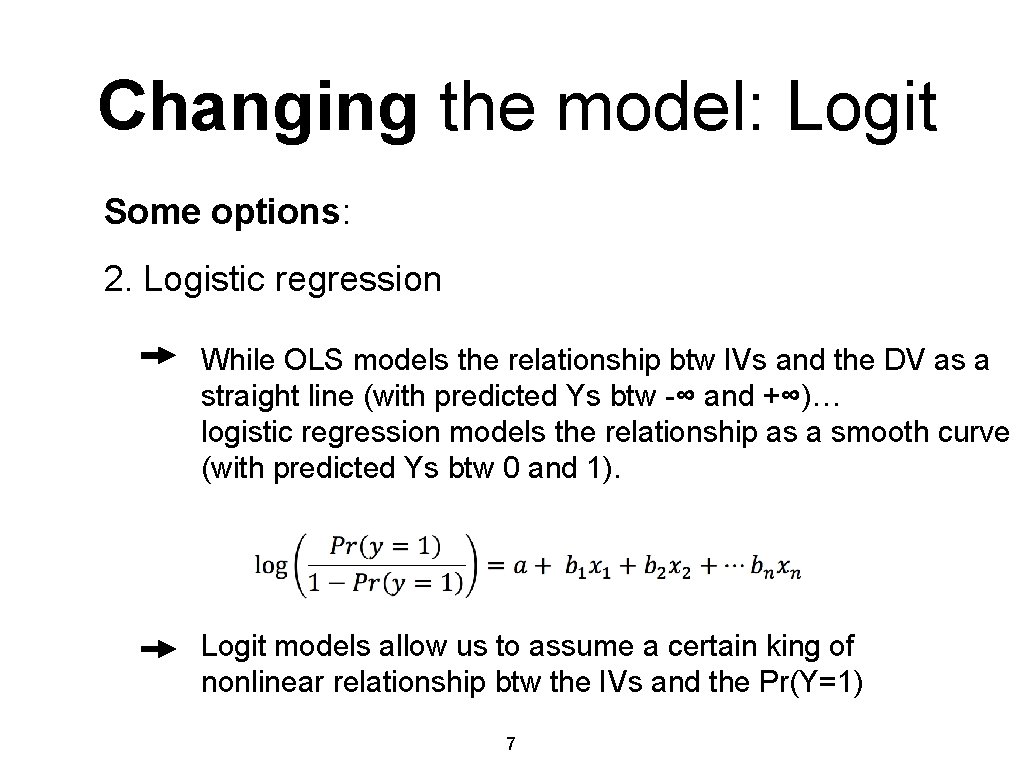
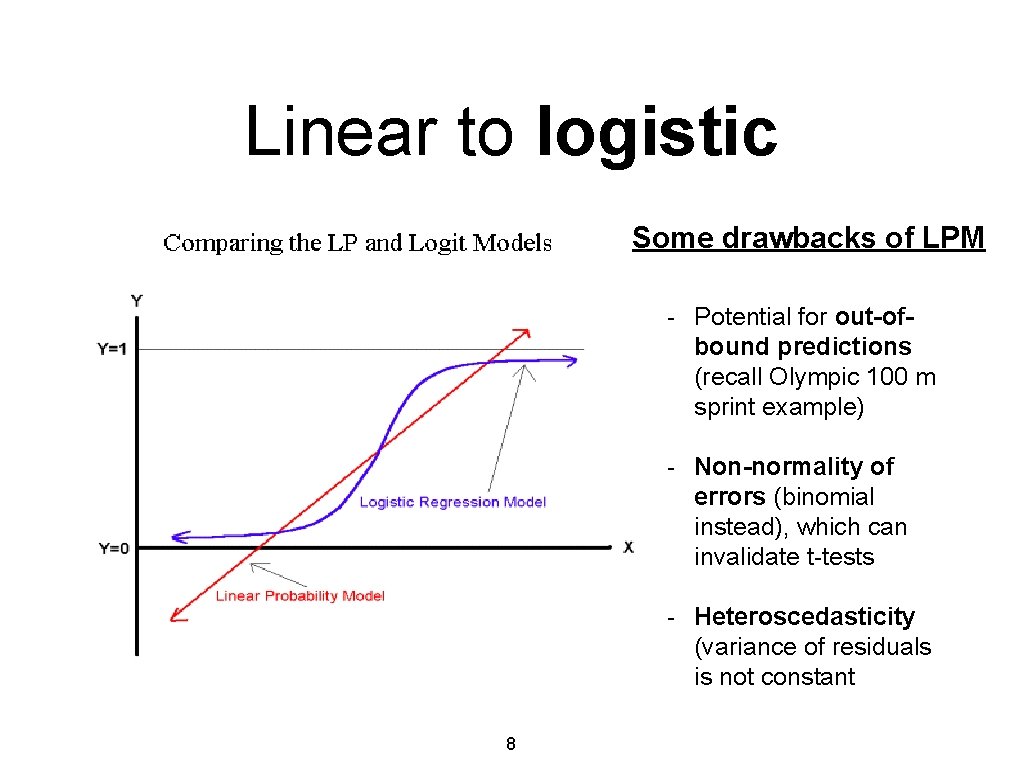
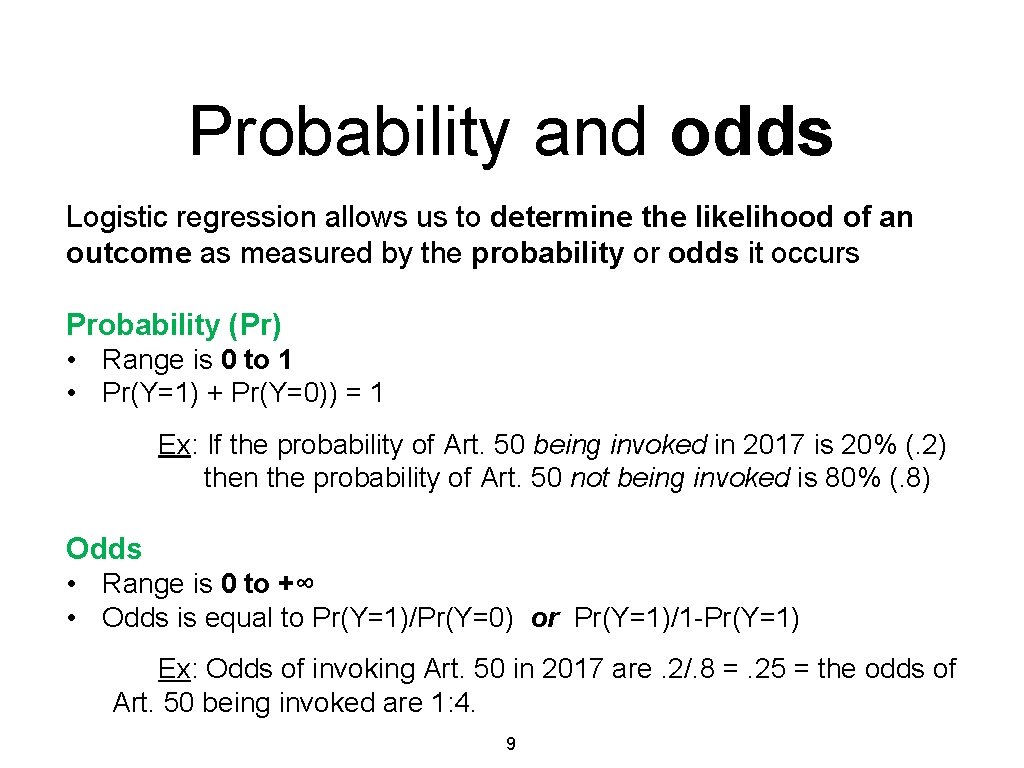
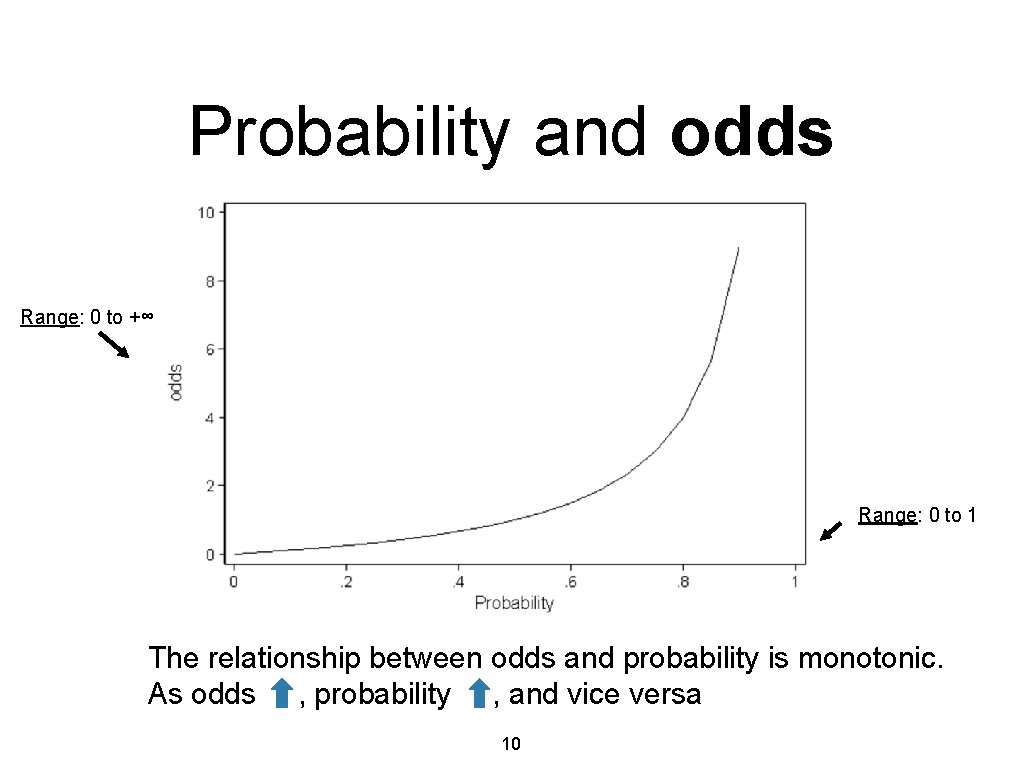
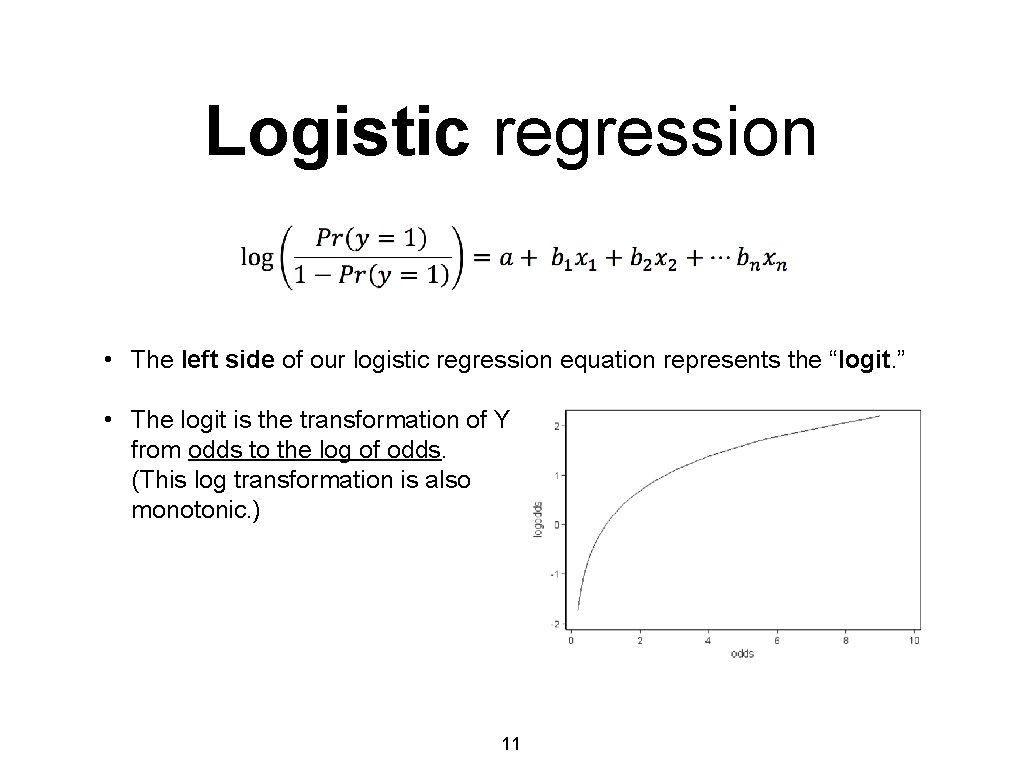
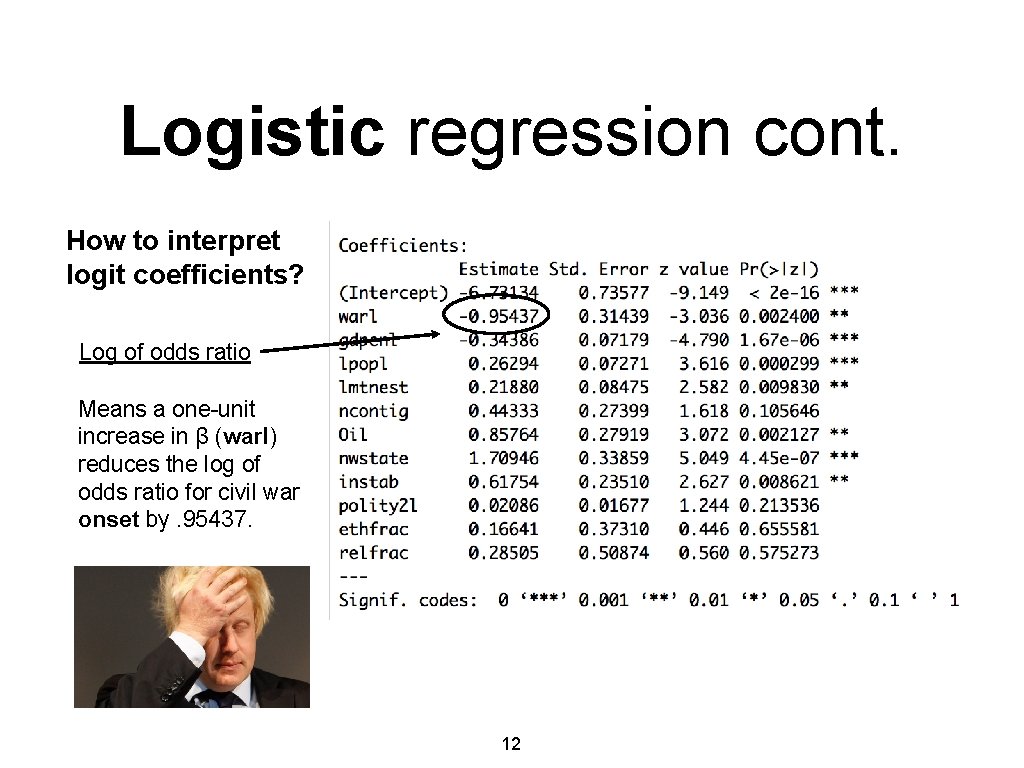
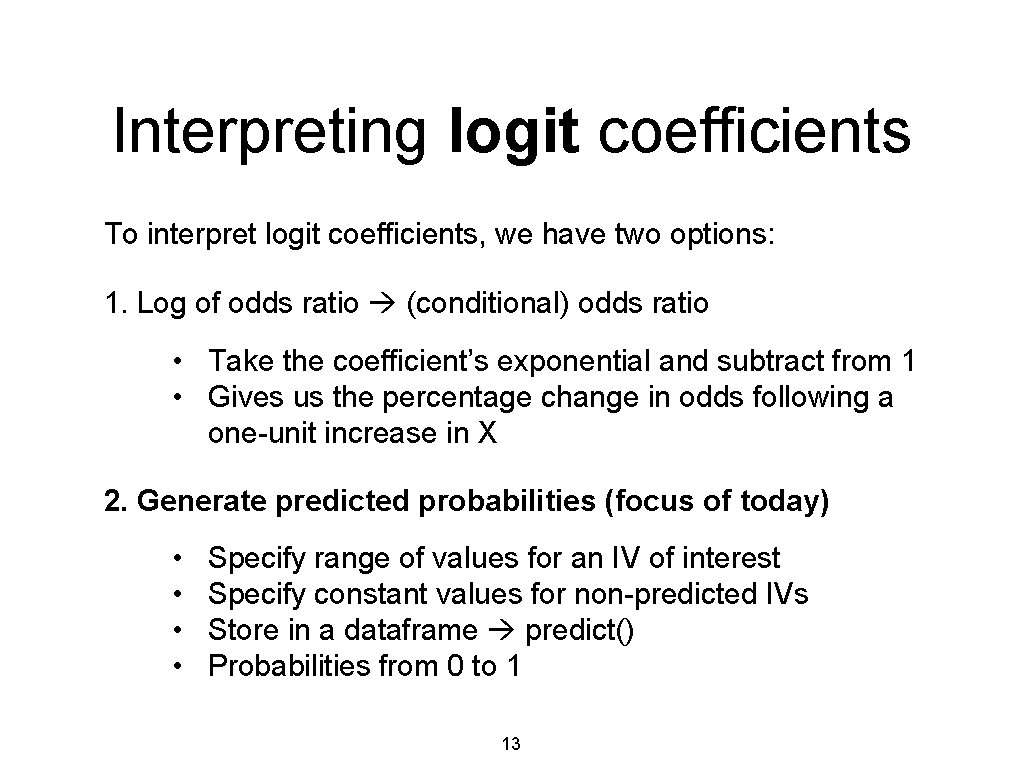
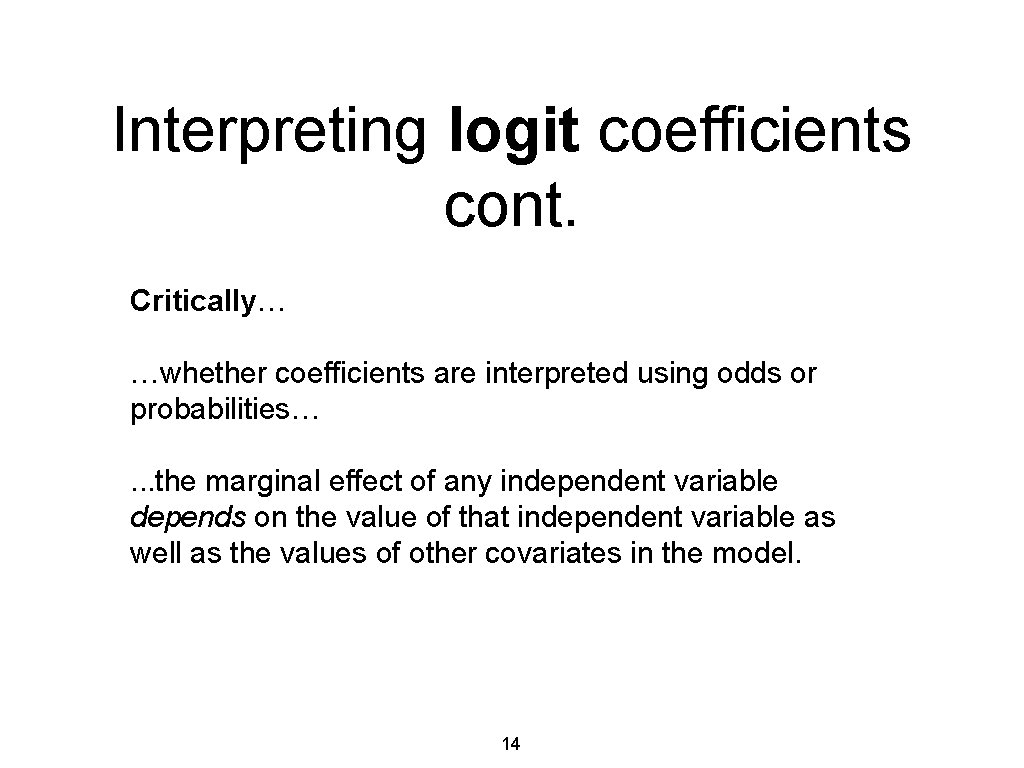
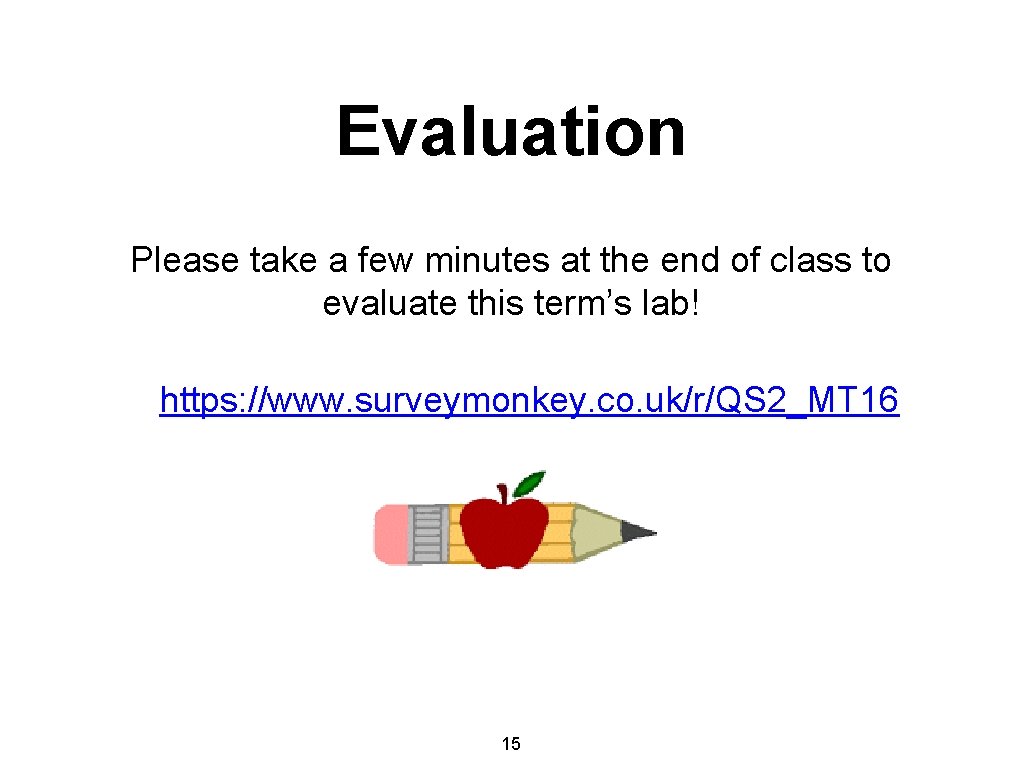
- Slides: 15
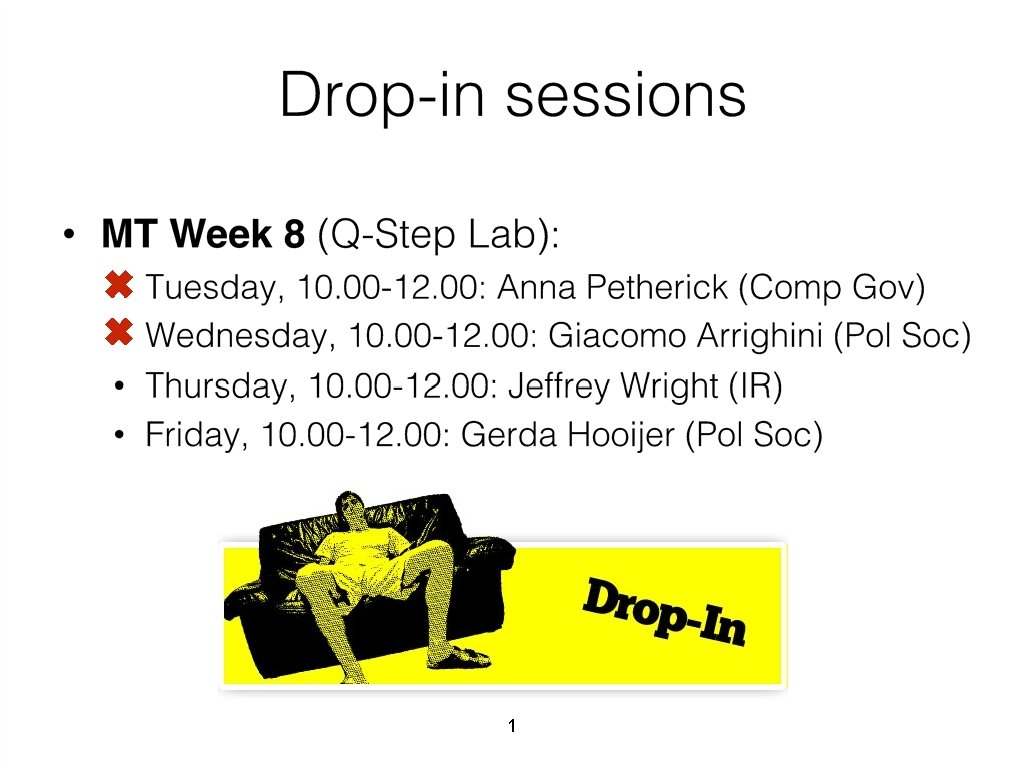
1
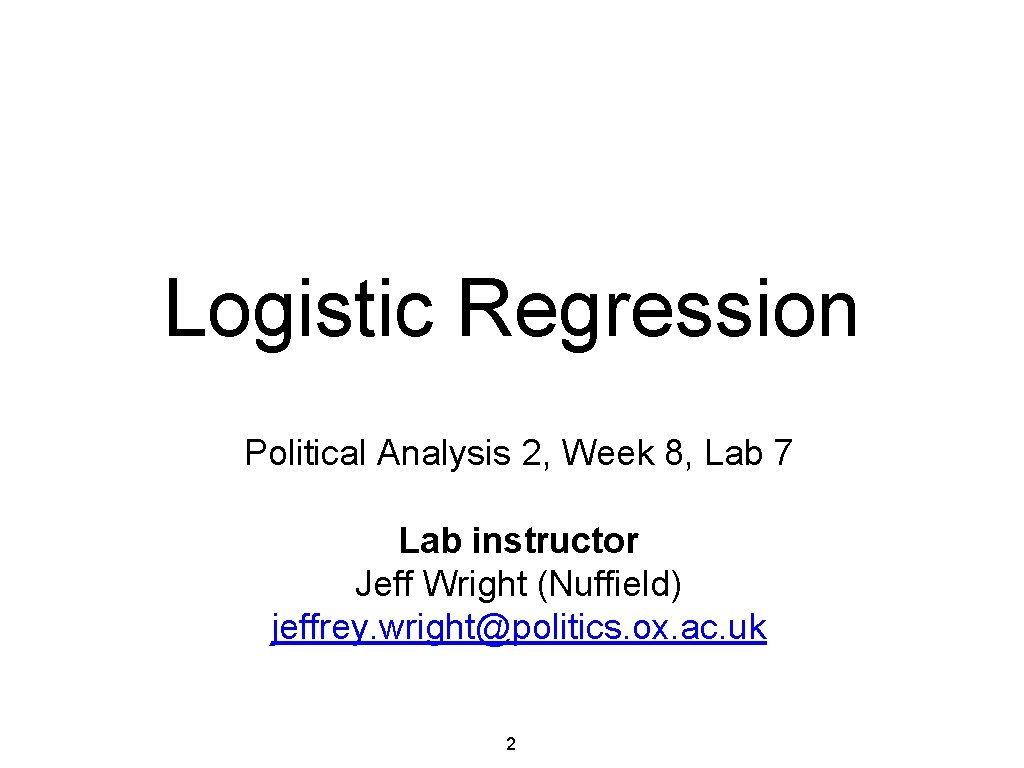
Logistic Regression Political Analysis 2, Week 8, Lab 7 Lab instructor Jeff Wright (Nuffield) jeffrey. wright@politics. ox. ac. uk 2
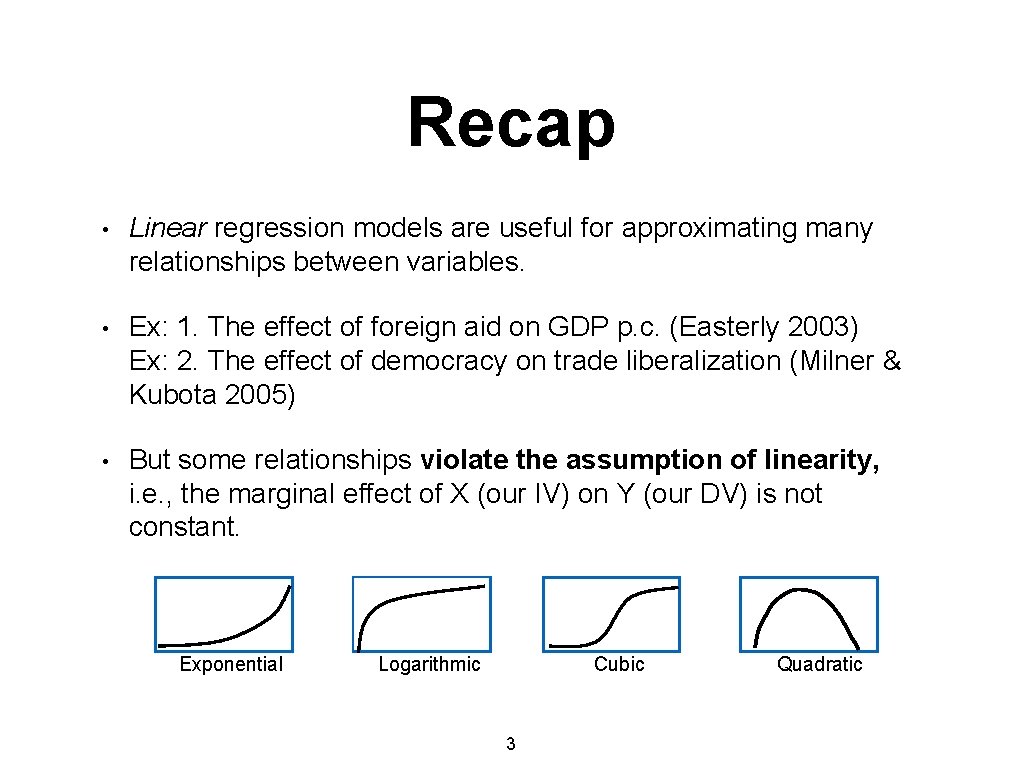
Recap • Linear regression models are useful for approximating many relationships between variables. • Ex: 1. The effect of foreign aid on GDP p. c. (Easterly 2003) Ex: 2. The effect of democracy on trade liberalization (Milner & Kubota 2005) • But some relationships violate the assumption of linearity, i. e. , the marginal effect of X (our IV) on Y (our DV) is not constant. Exponential Logarithmic Cubic 3 Quadratic
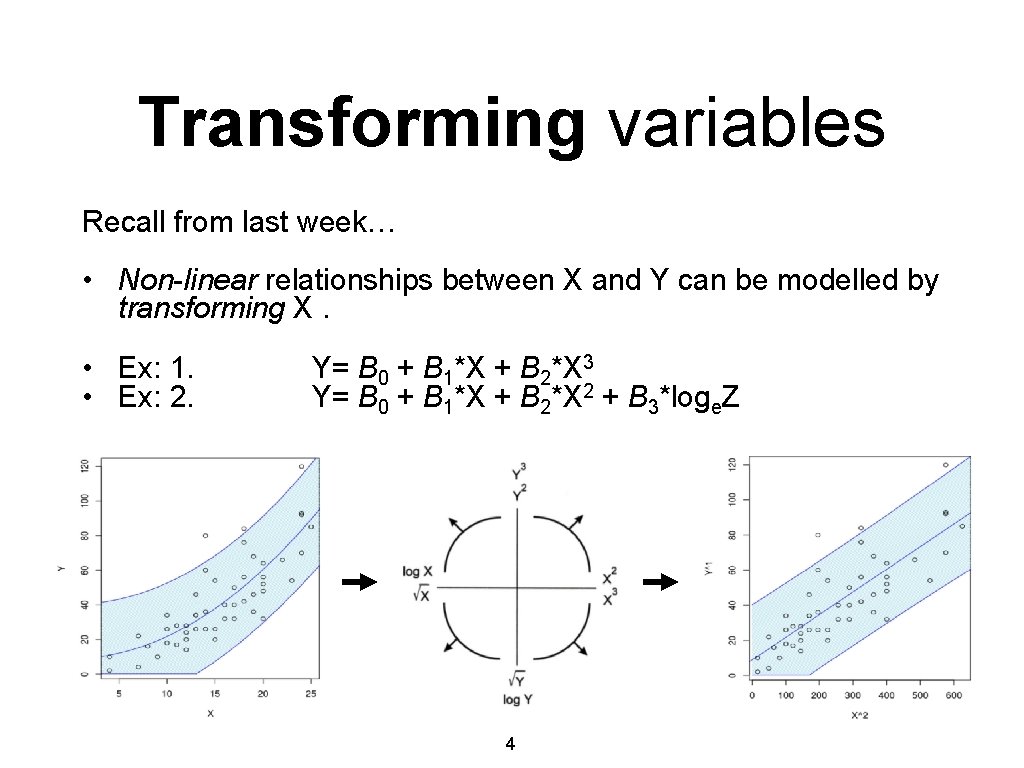
Transforming variables Recall from last week… • Non-linear relationships between X and Y can be modelled by transforming X. • Ex: 1. • Ex: 2. Y= B 0 + B 1*X + B 2*X 3 Y= B 0 + B 1*X + B 2*X 2 + B 3*loge. Z 4
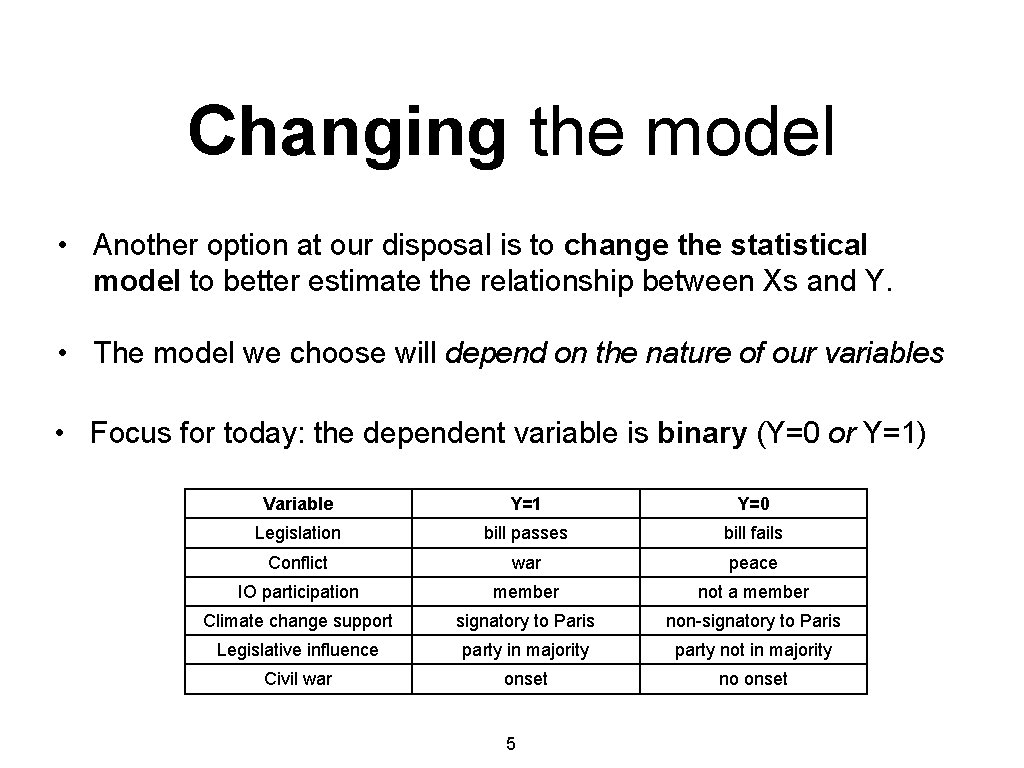
Changing the model • Another option at our disposal is to change the statistical model to better estimate the relationship between Xs and Y. • The model we choose will depend on the nature of our variables • Focus for today: the dependent variable is binary (Y=0 or Y=1) Variable Y=1 Y=0 Legislation bill passes bill fails Conflict war peace IO participation member not a member Climate change support signatory to Paris non-signatory to Paris Legislative influence party in majority party not in majority Civil war onset no onset 5
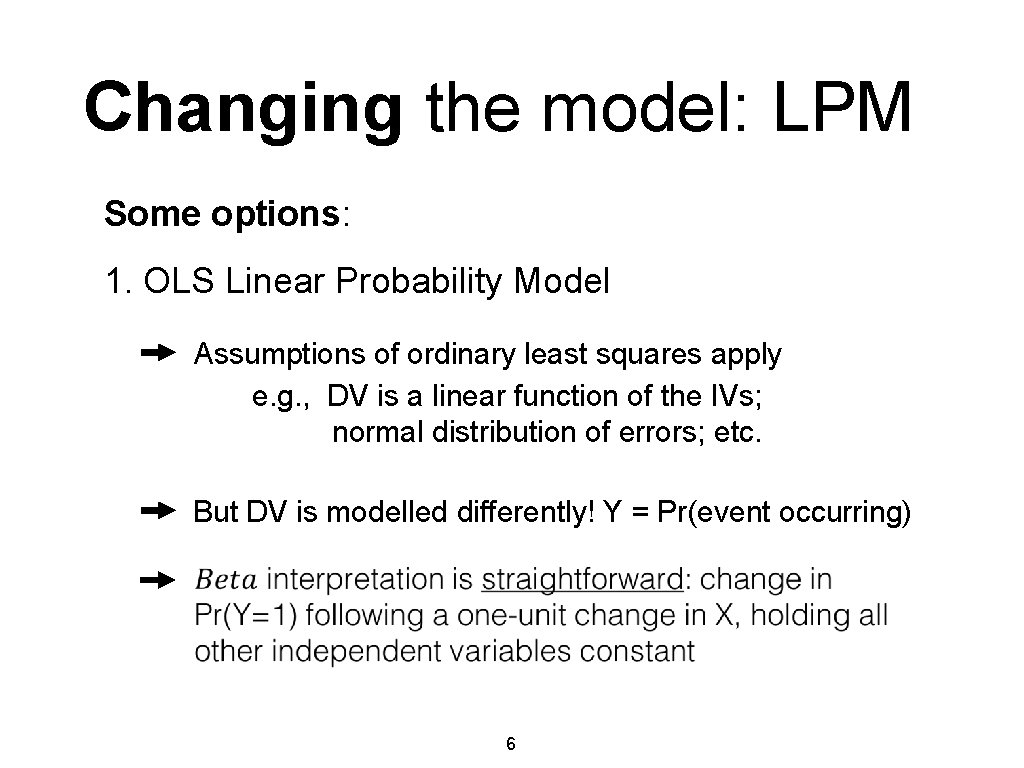
Changing the model: LPM Some options: 1. OLS Linear Probability Model Assumptions of ordinary least squares apply e. g. , DV is a linear function of the IVs; normal distribution of errors; etc. But DV is modelled differently! Y = Pr(event occurring) 6
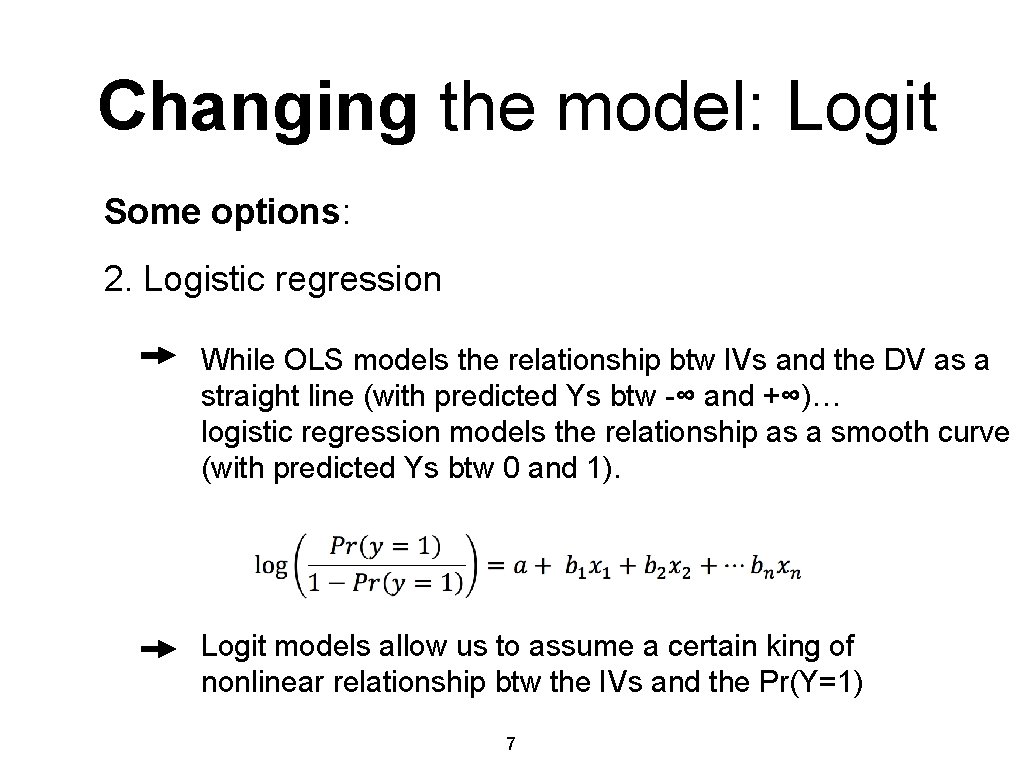
Changing the model: Logit Some options: 2. Logistic regression While OLS models the relationship btw IVs and the DV as a straight line (with predicted Ys btw -∞ and +∞)… logistic regression models the relationship as a smooth curve (with predicted Ys btw 0 and 1). Logit models allow us to assume a certain king of nonlinear relationship btw the IVs and the Pr(Y=1) 7
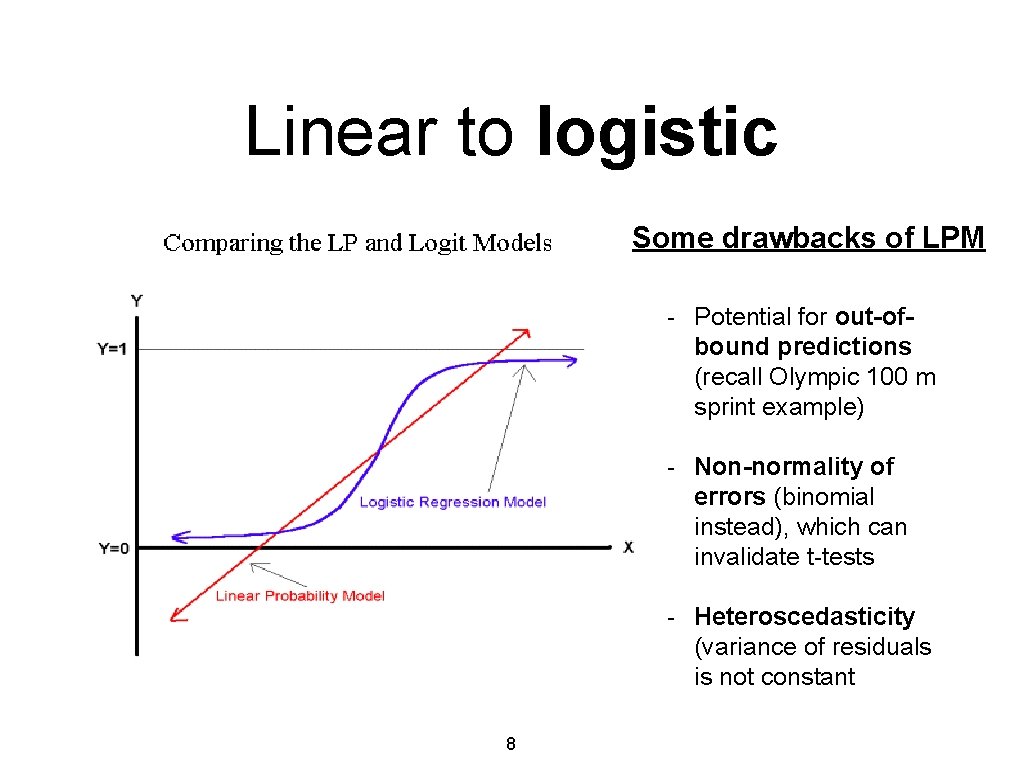
Linear to logistic Some drawbacks of LPM - Potential for out-ofbound predictions (recall Olympic 100 m sprint example) - Non-normality of errors (binomial instead), which can invalidate t-tests - Heteroscedasticity (variance of residuals is not constant 8
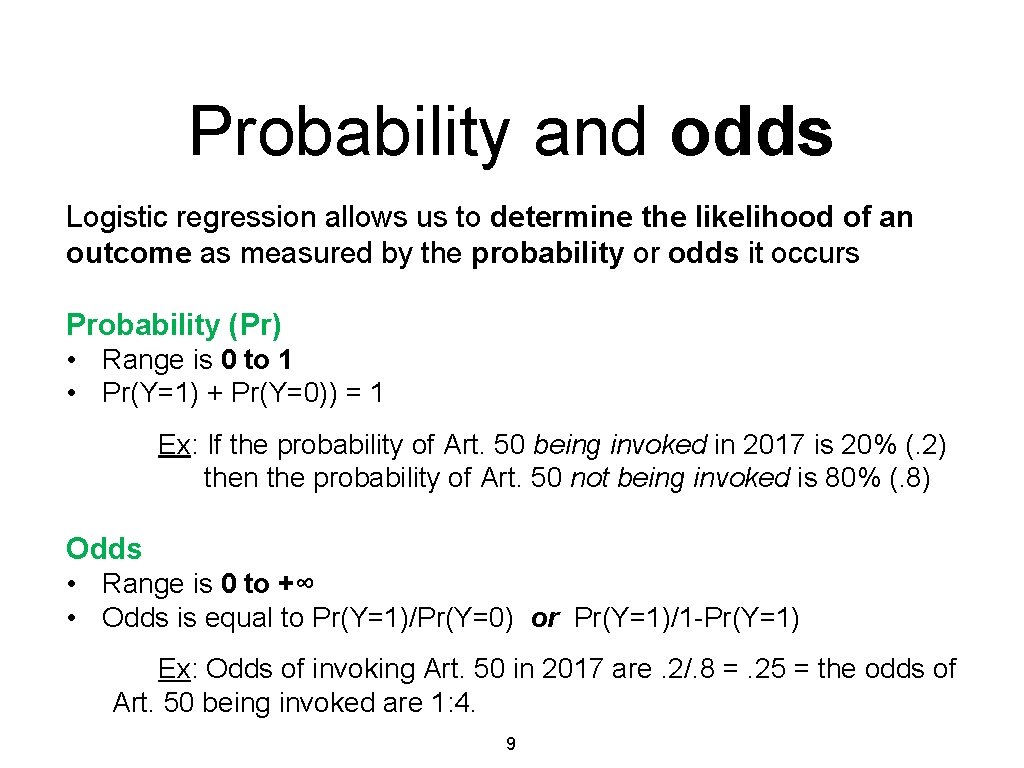
Probability and odds Logistic regression allows us to determine the likelihood of an outcome as measured by the probability or odds it occurs Probability (Pr) • Range is 0 to 1 • Pr(Y=1) + Pr(Y=0)) = 1 Ex: If the probability of Art. 50 being invoked in 2017 is 20% (. 2) then the probability of Art. 50 not being invoked is 80% (. 8) Odds • Range is 0 to +∞ • Odds is equal to Pr(Y=1)/Pr(Y=0) or Pr(Y=1)/1 -Pr(Y=1) Ex: Odds of invoking Art. 50 in 2017 are. 2/. 8 =. 25 = the odds of Art. 50 being invoked are 1: 4. 9
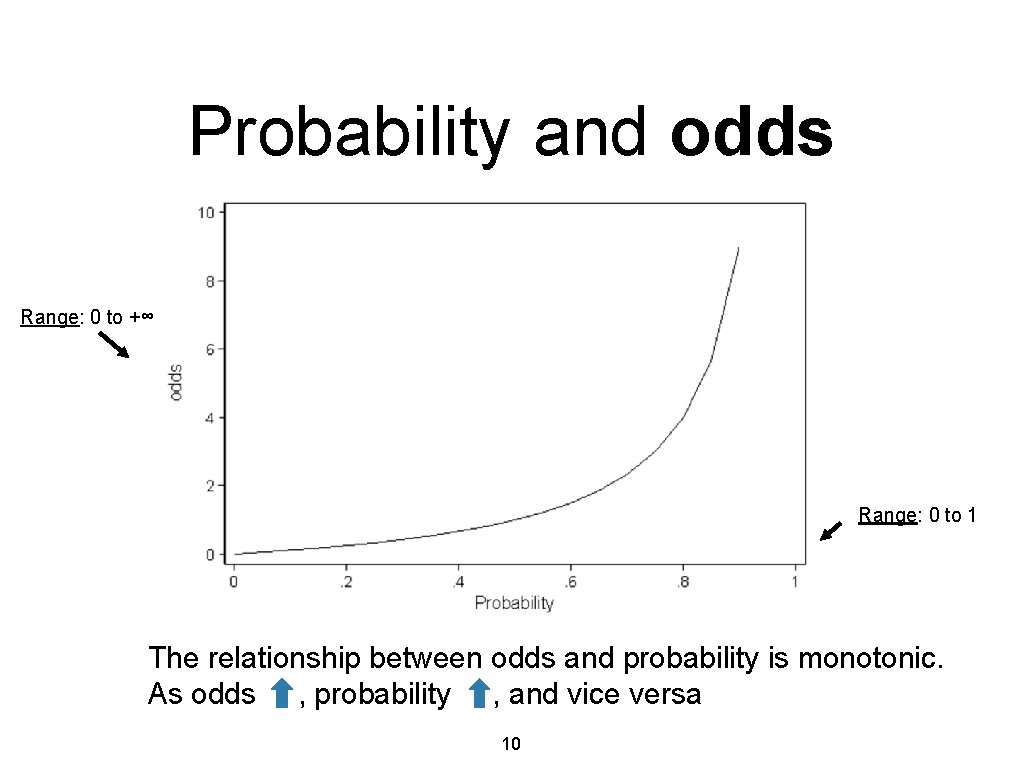
Probability and odds Range: 0 to +∞ Range: 0 to 1 The relationship between odds and probability is monotonic. As odds , probability , and vice versa 10
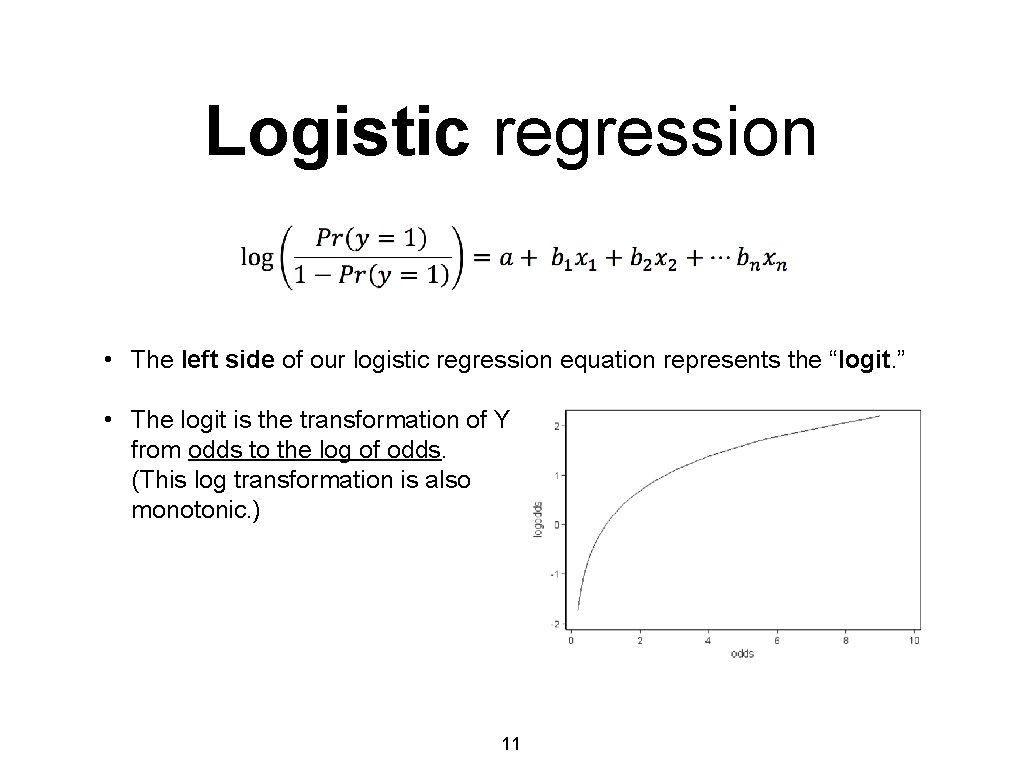
Logistic regression • The left side of our logistic regression equation represents the “logit. ” • The logit is the transformation of Y from odds to the log of odds. (This log transformation is also monotonic. ) 11
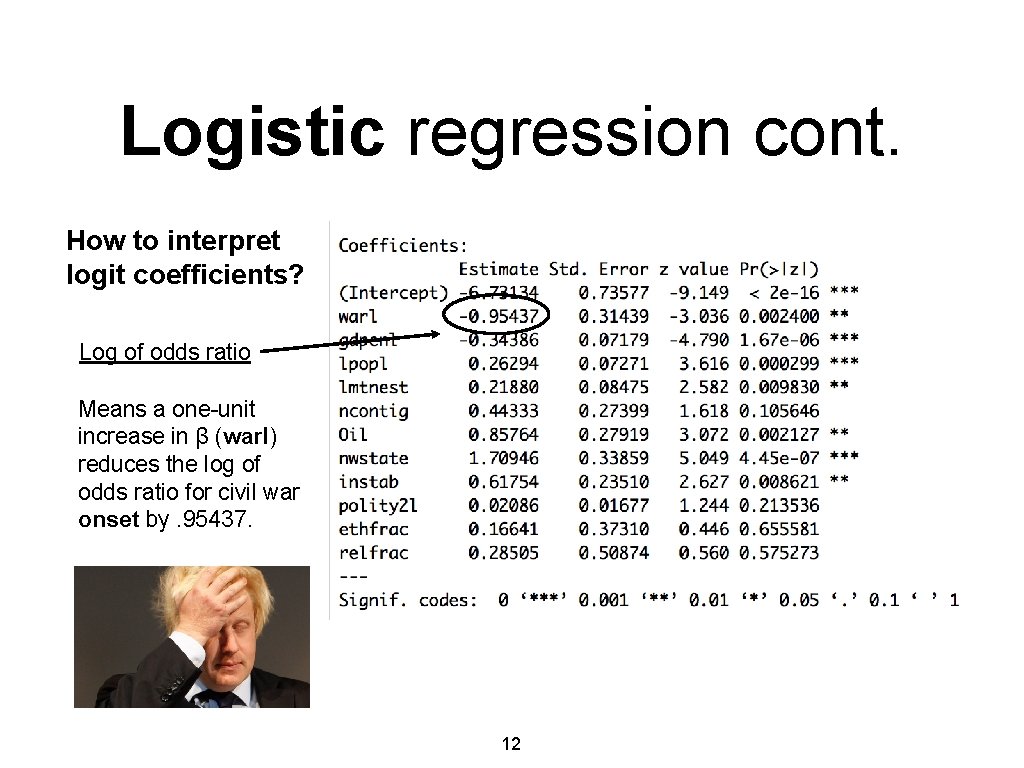
Logistic regression cont. How to interpret logit coefficients? Log of odds ratio Means a one-unit increase in β (warl) reduces the log of odds ratio for civil war onset by. 95437. 12
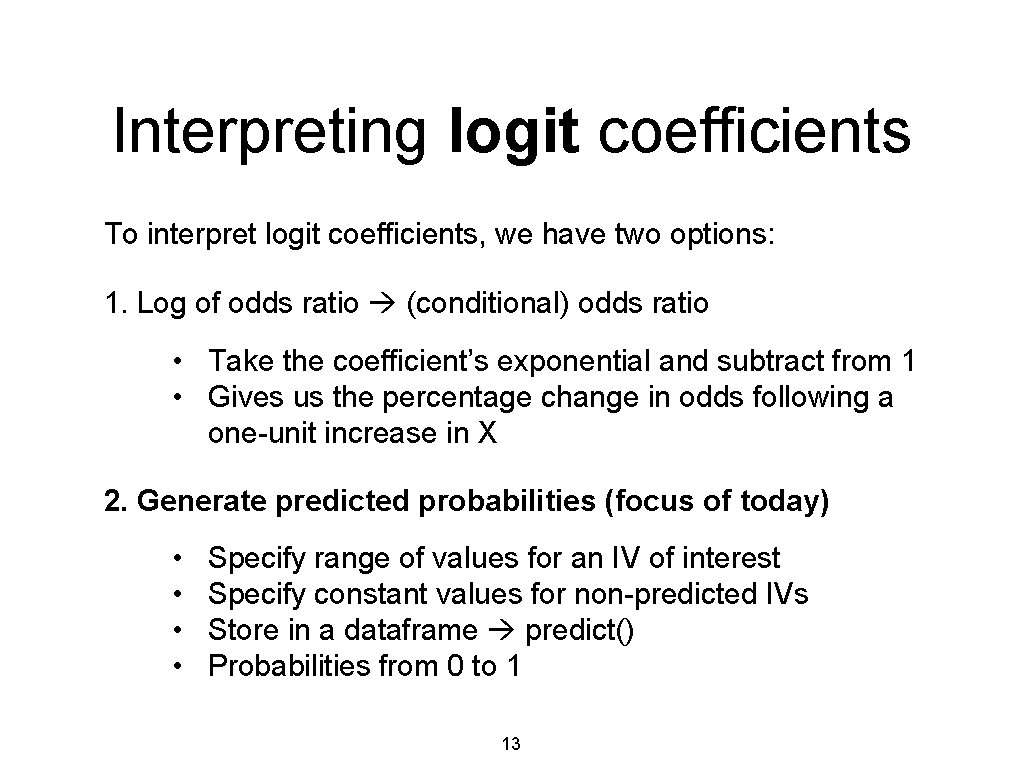
Interpreting logit coefficients To interpret logit coefficients, we have two options: 1. Log of odds ratio (conditional) odds ratio • Take the coefficient’s exponential and subtract from 1 • Gives us the percentage change in odds following a one-unit increase in X 2. Generate predicted probabilities (focus of today) • • Specify range of values for an IV of interest Specify constant values for non-predicted IVs Store in a dataframe predict() Probabilities from 0 to 1 13
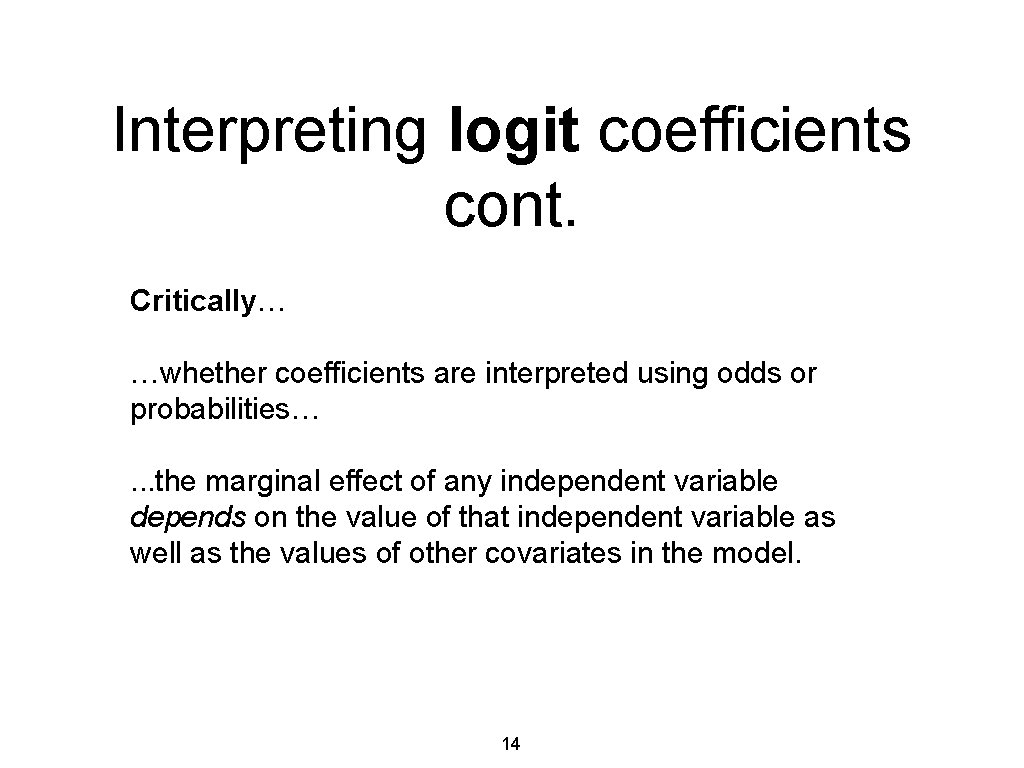
Interpreting logit coefficients cont. Critically… …whether coefficients are interpreted using odds or probabilities…. . . the marginal effect of any independent variable depends on the value of that independent variable as well as the values of other covariates in the model. 14
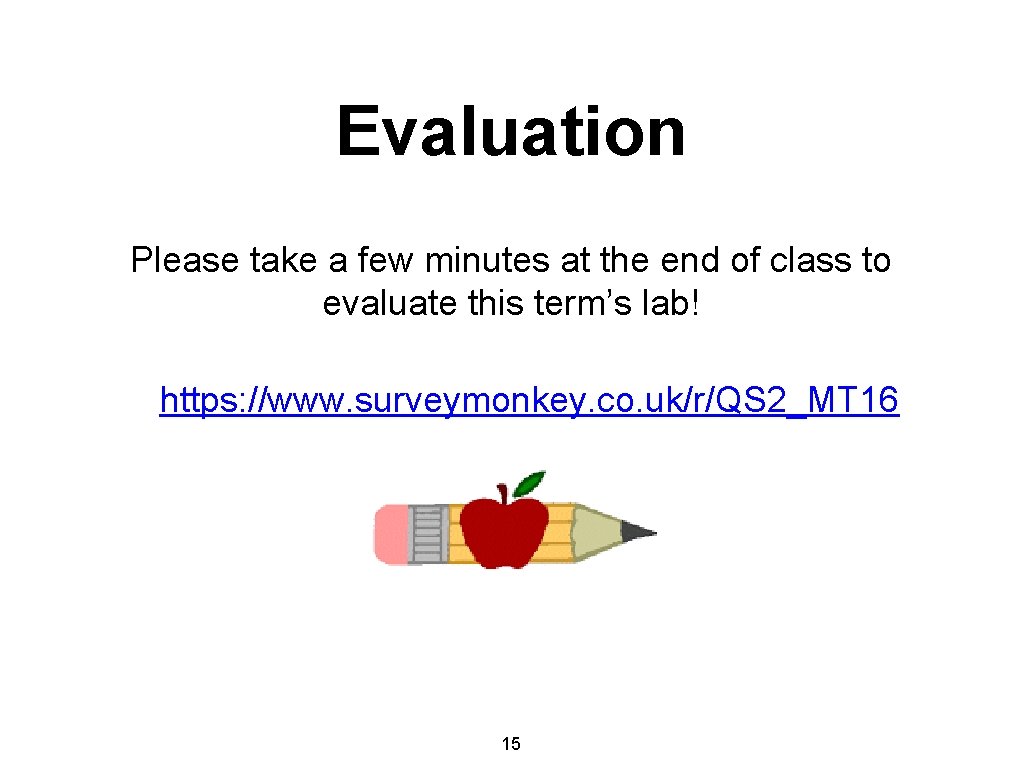
Evaluation Please take a few minutes at the end of class to evaluate this term’s lab! https: //www. surveymonkey. co. uk/r/QS 2_MT 16 15
Logistic regression vs linear regression
Logistic regression vs linear regression
Logistic regression and discriminant analysis
Logistic regression residual deviance
Sequential logistic regression
Random forest vs logistic regression
Perceptron logistic regression
Multinomial logistic regression
Cost function logistic regression
Andy field logistic regression
Logistic regression
Multinomial logistic regression
Logistic regression epidemiology
Hans burgerhof
Karl wuensch
Analisis regresi logistik berganda