Zhao Zhou Zhang Chen PNAS 2016 Mutual information
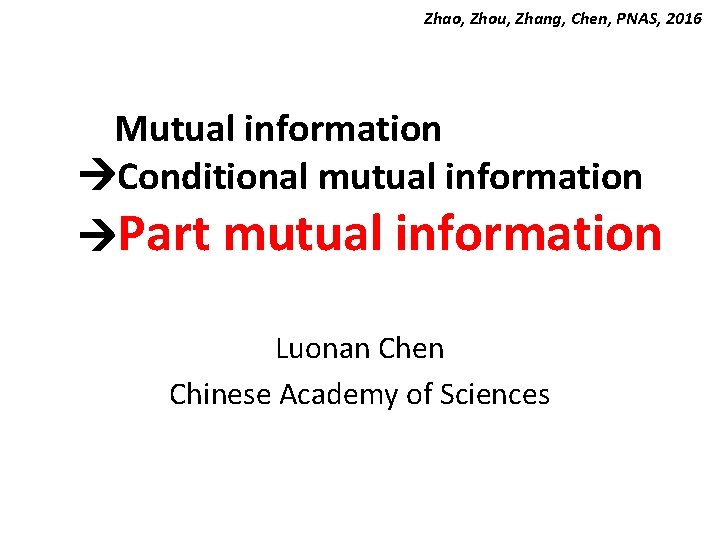
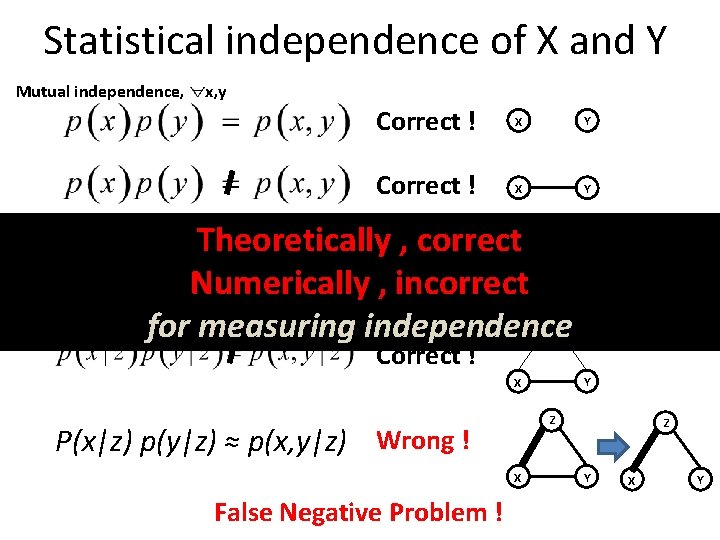
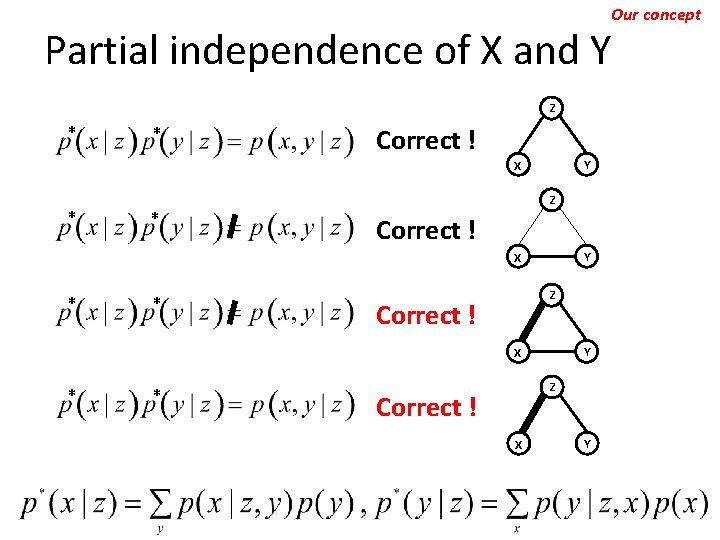
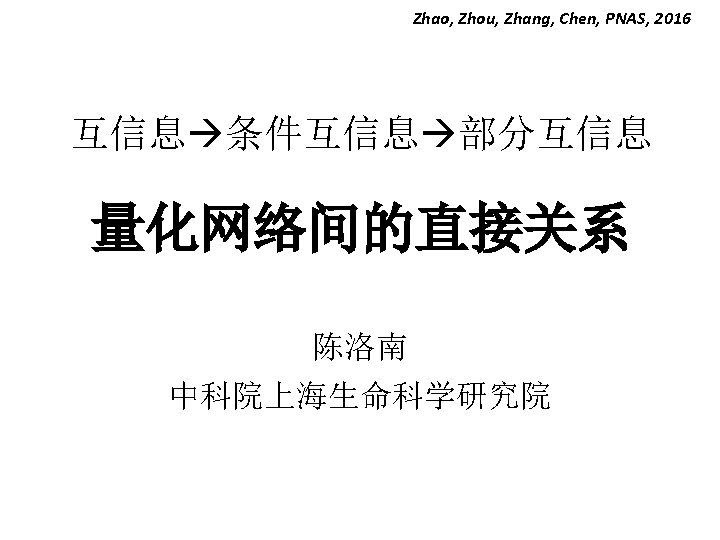
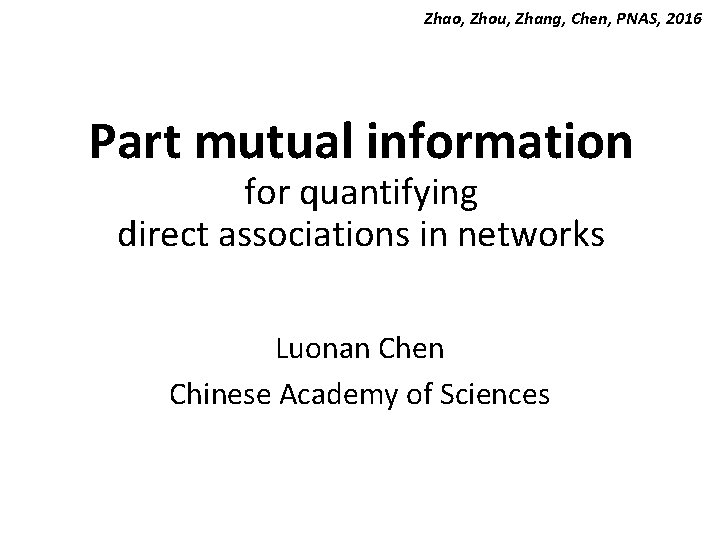
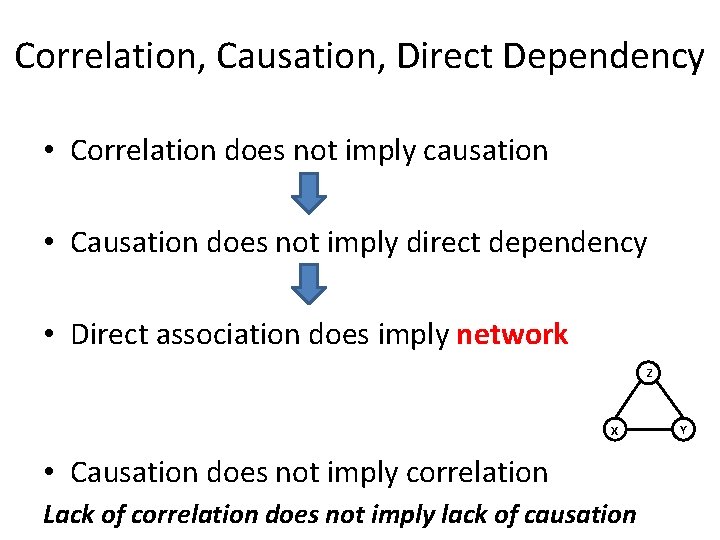
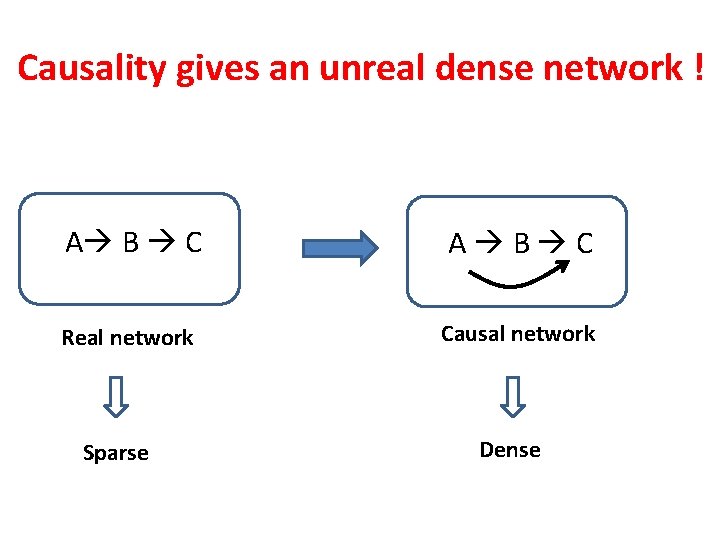
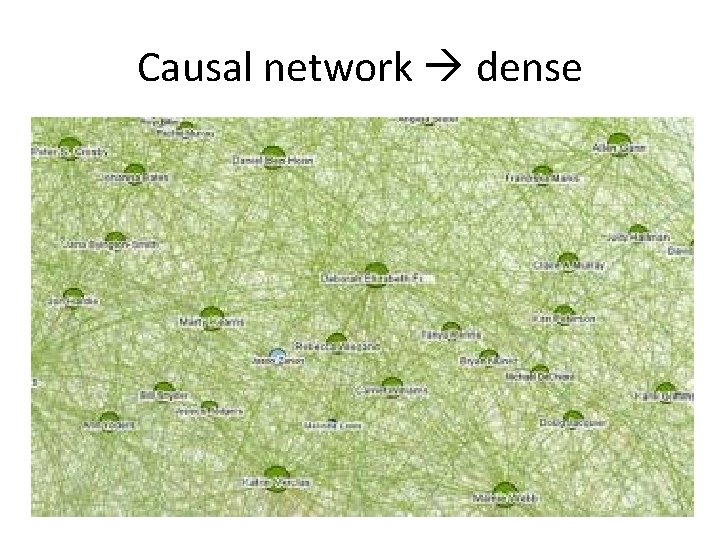
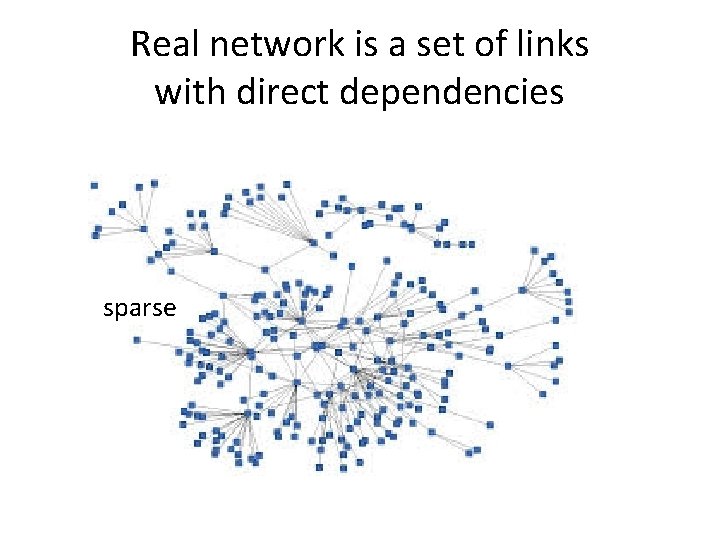
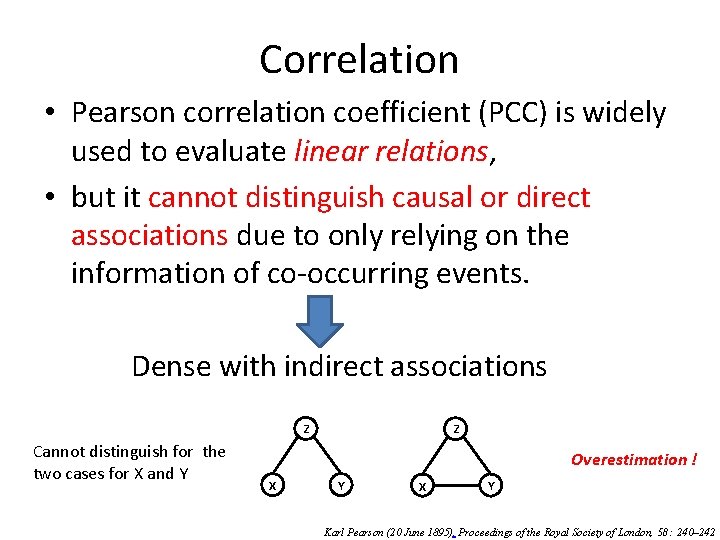
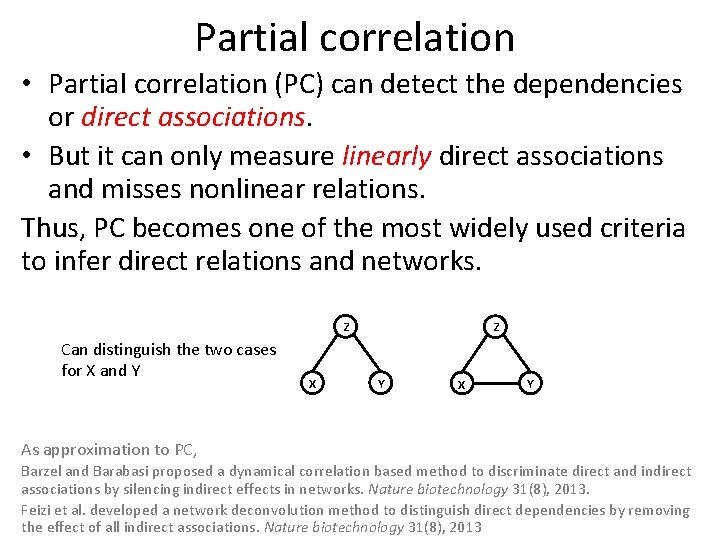
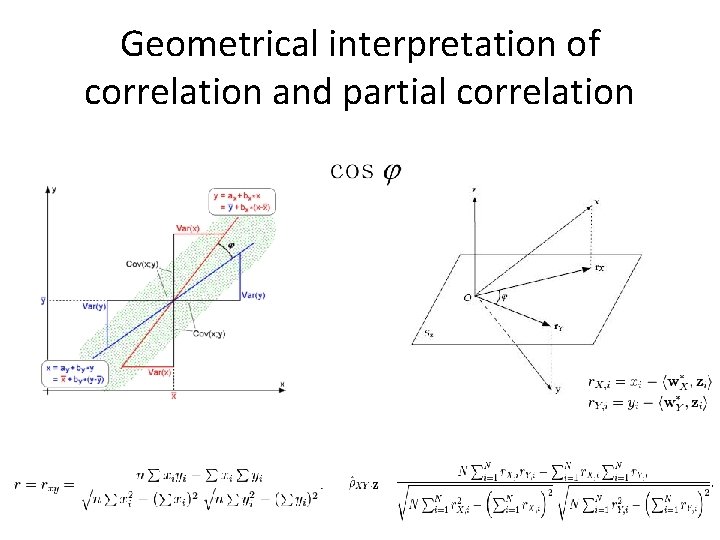
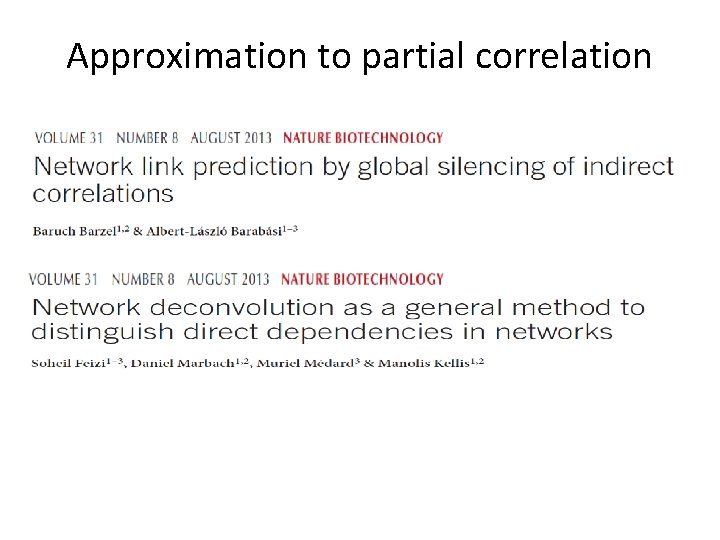
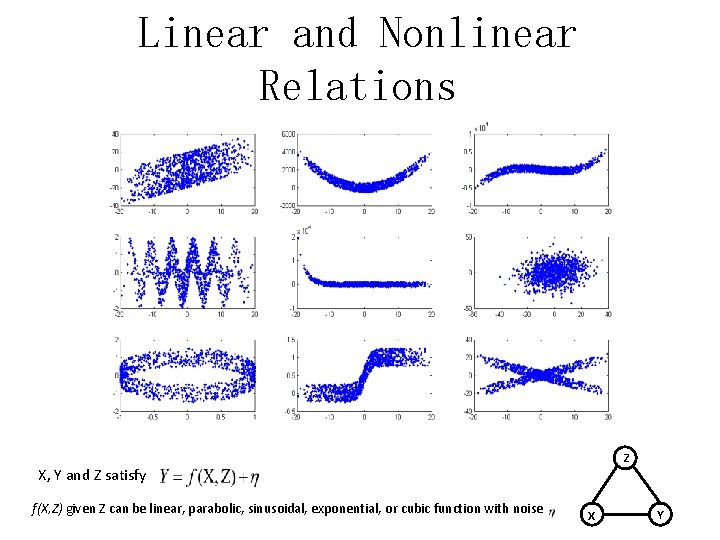
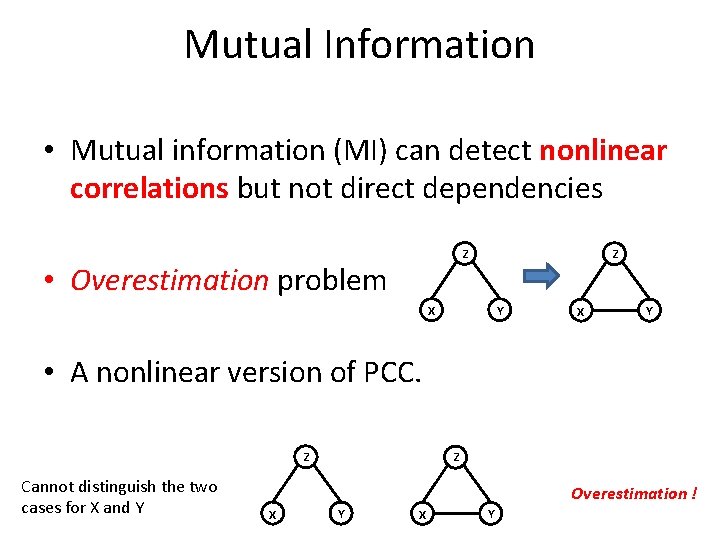
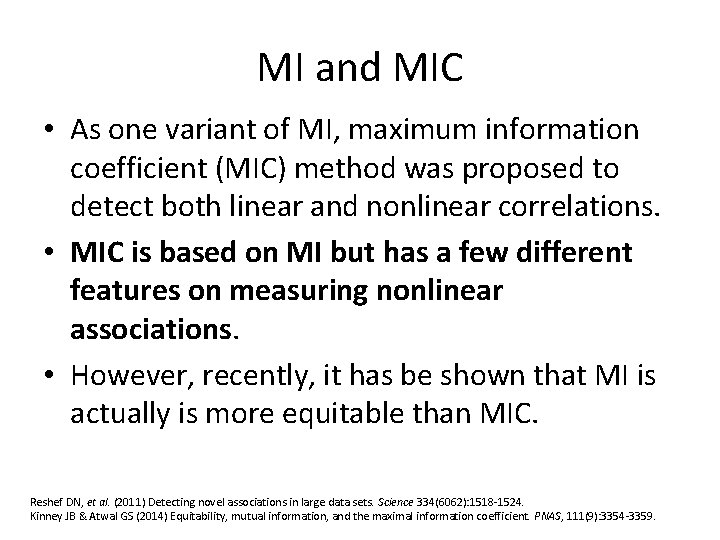
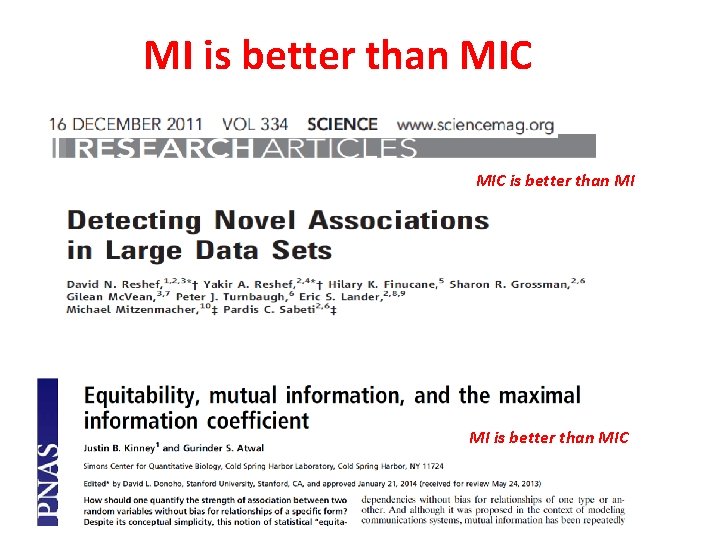
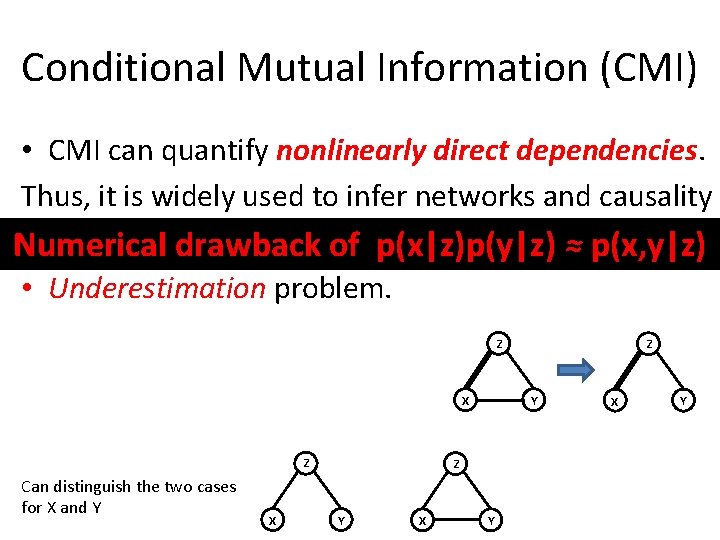
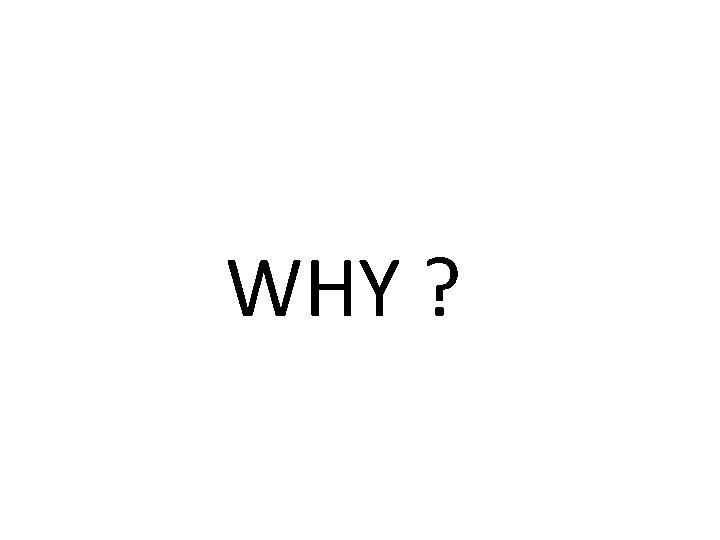
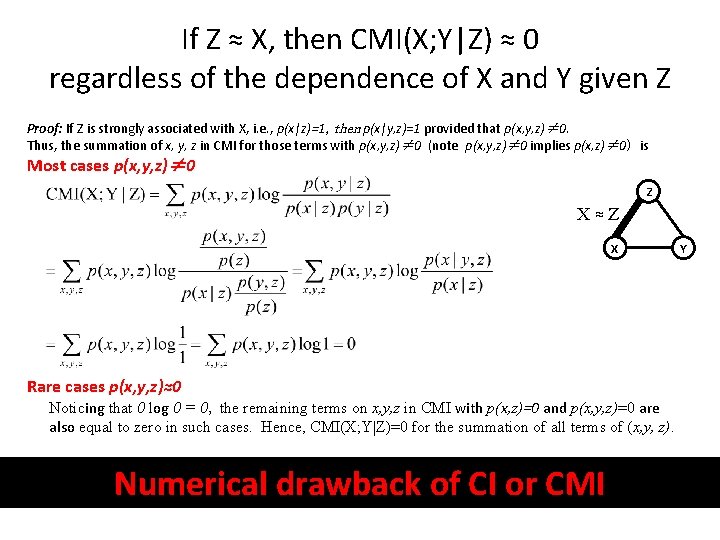
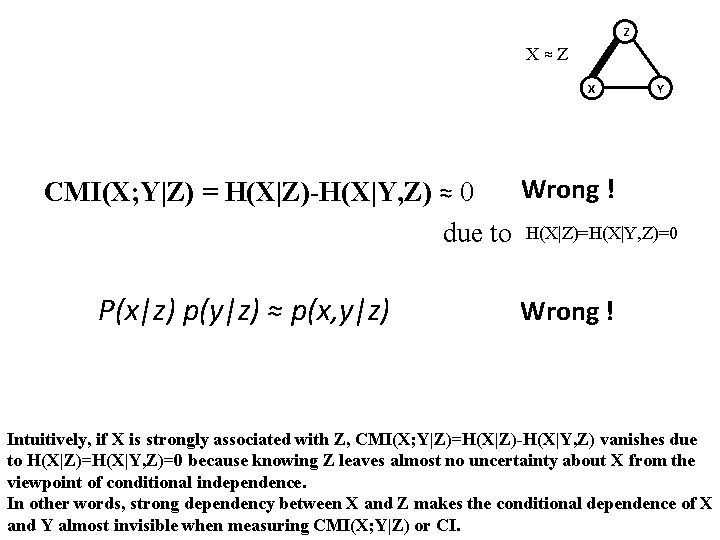
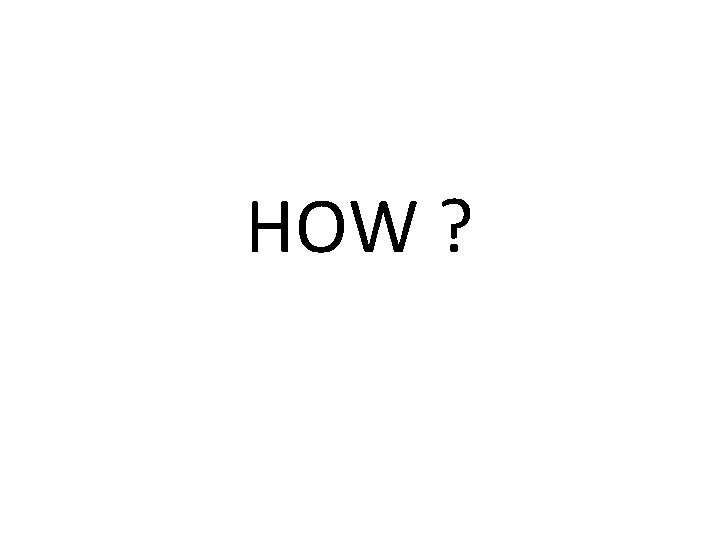
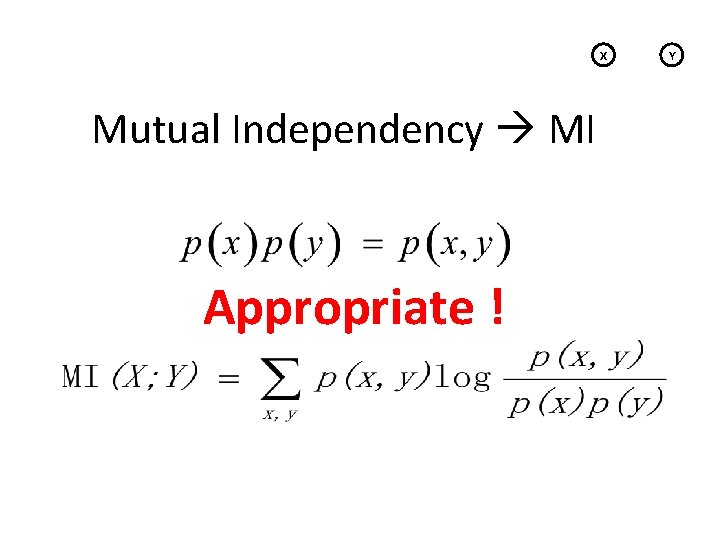
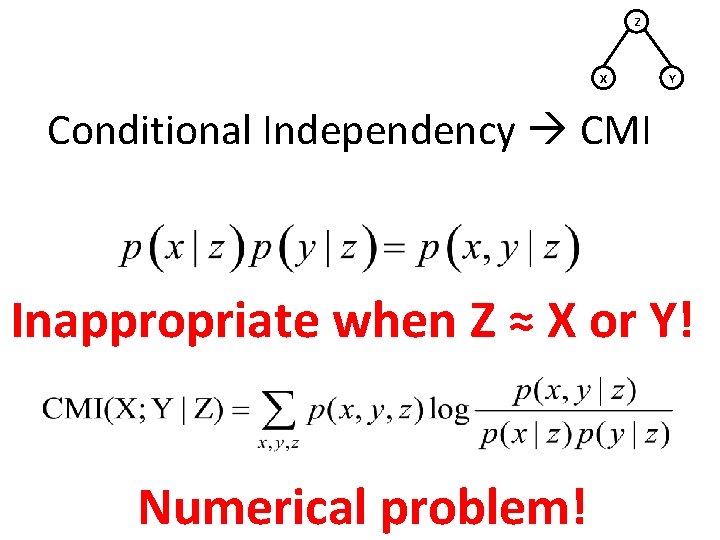
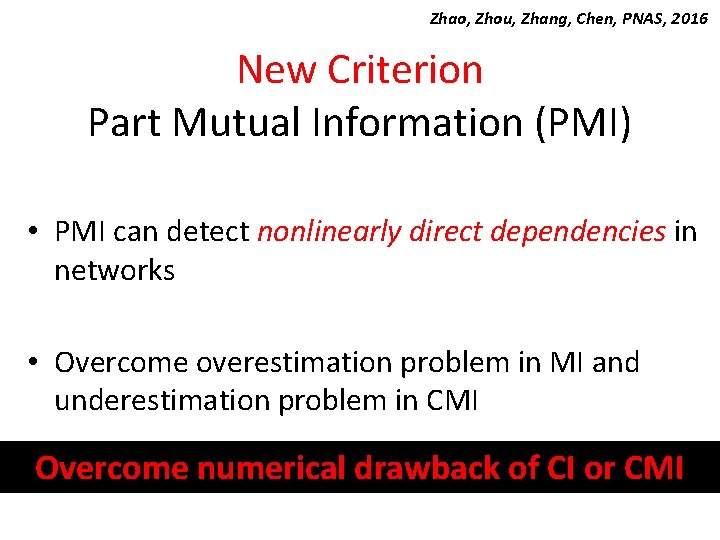
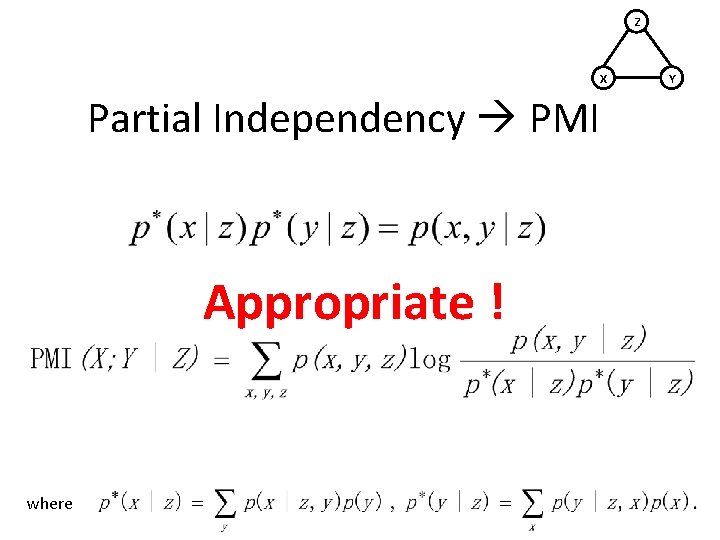
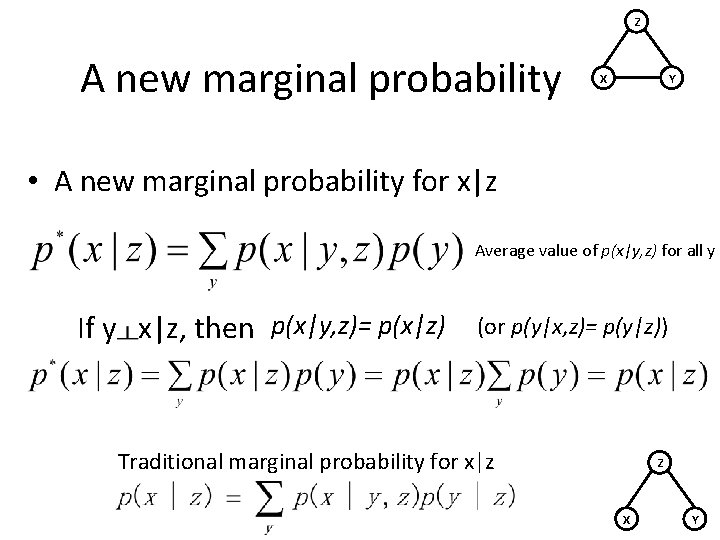
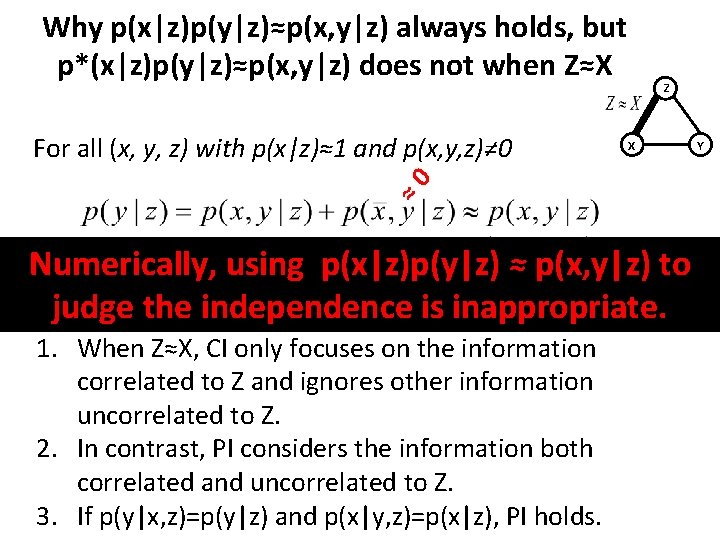
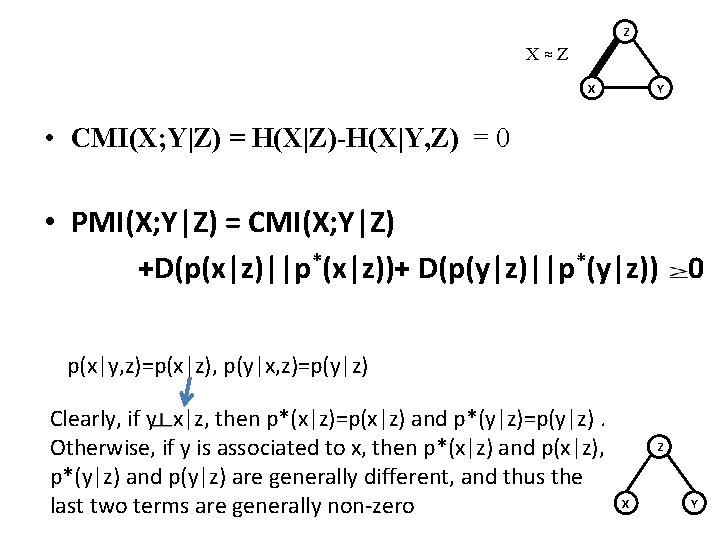
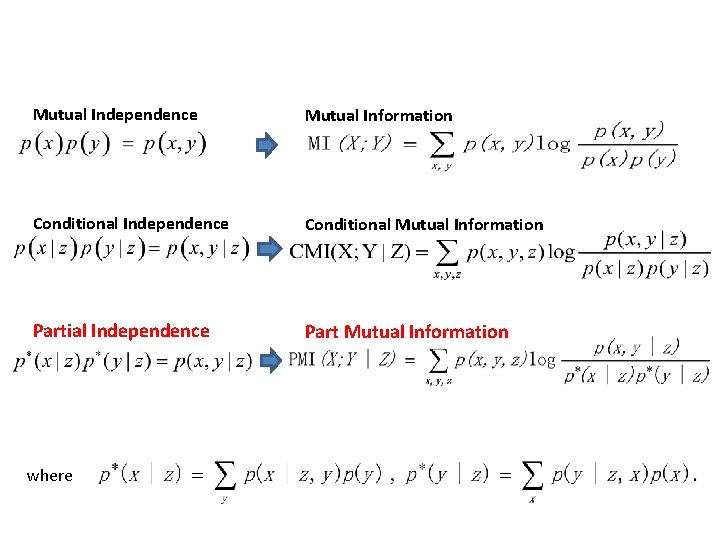
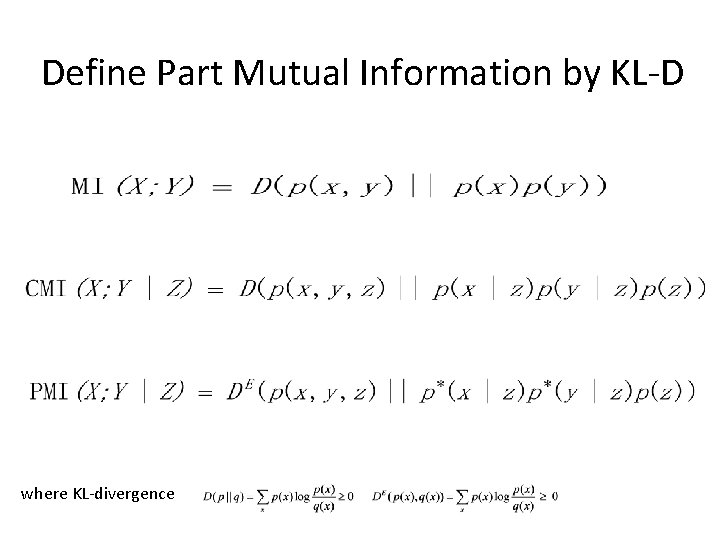
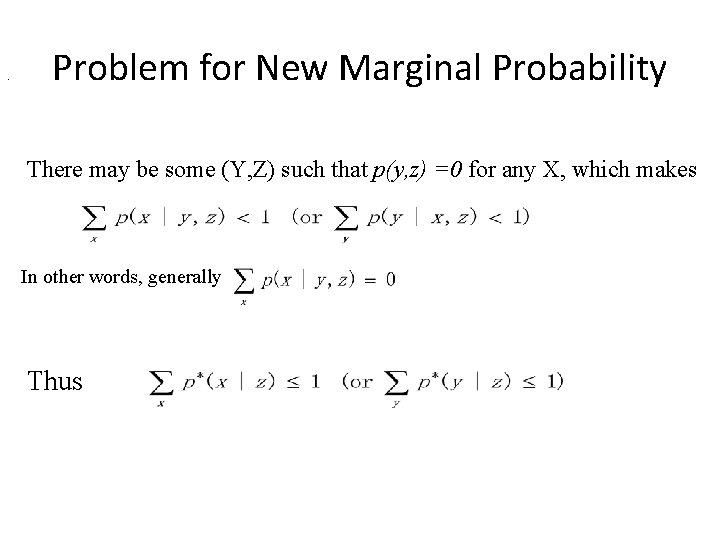
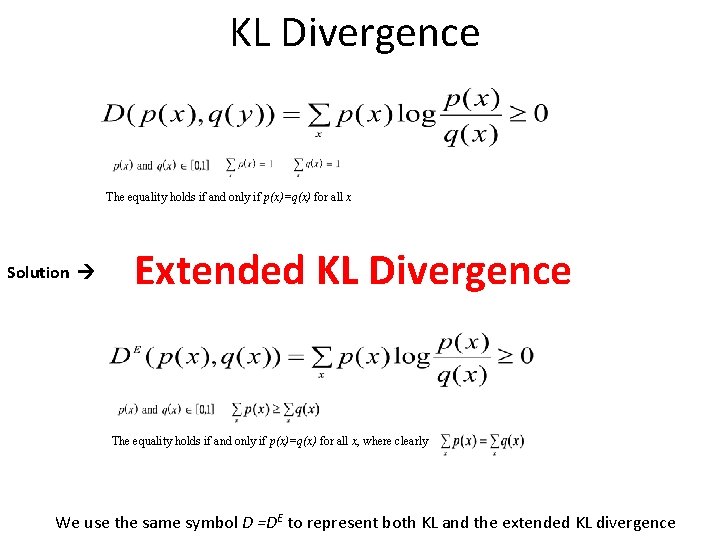
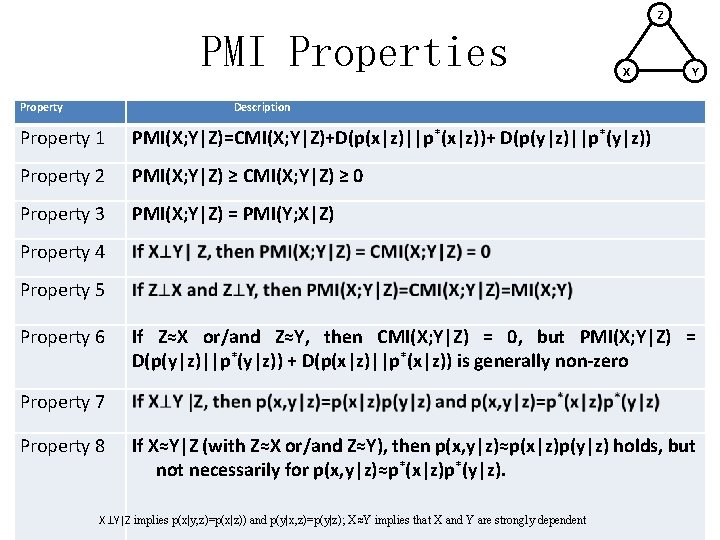
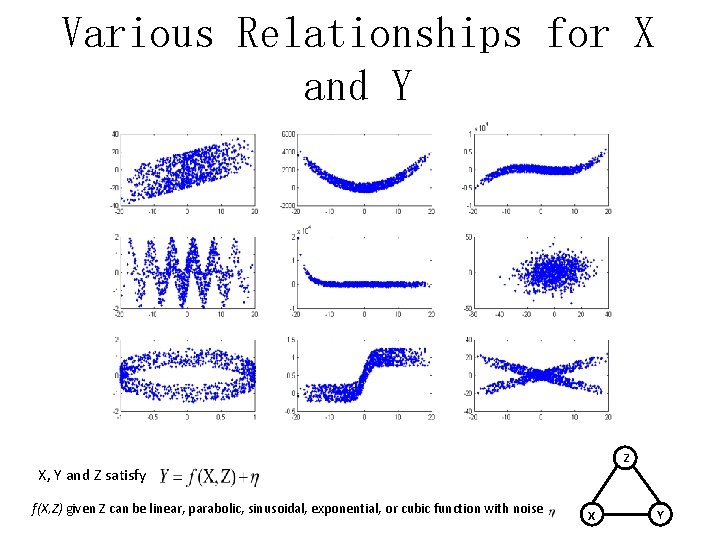
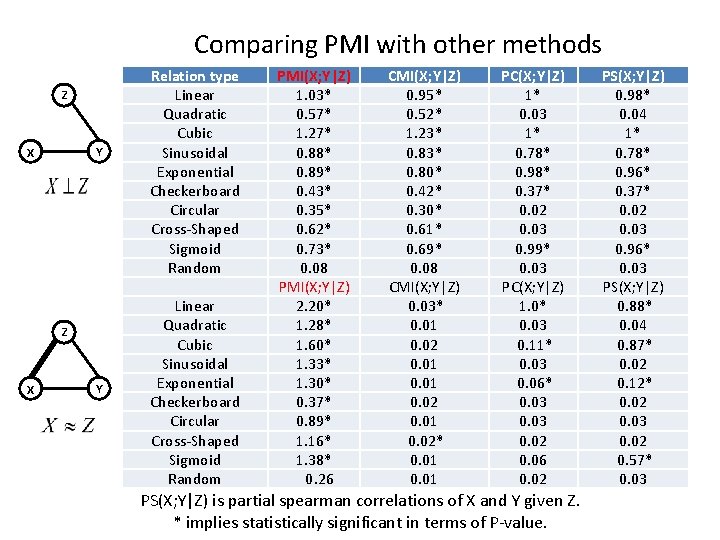
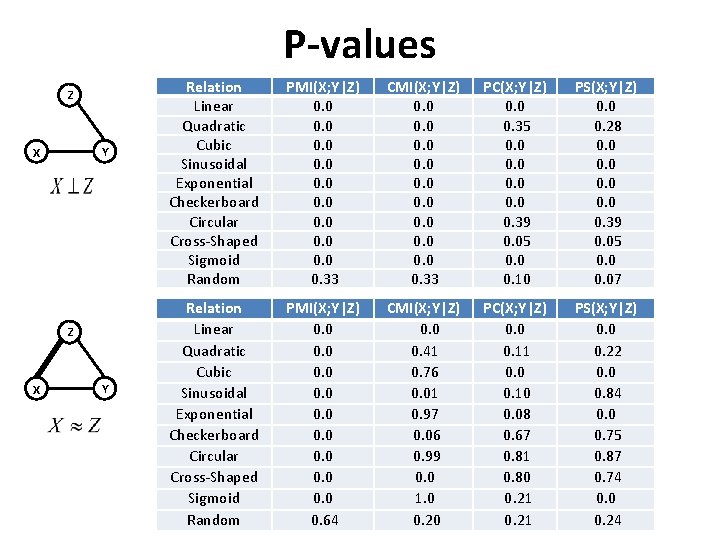
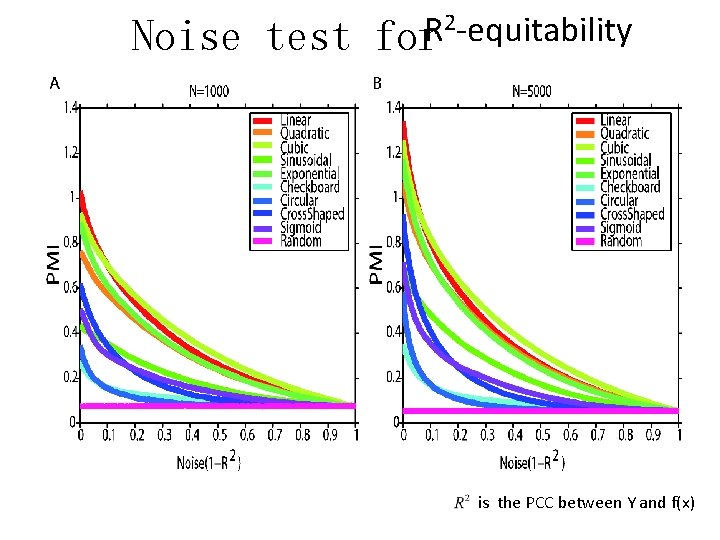
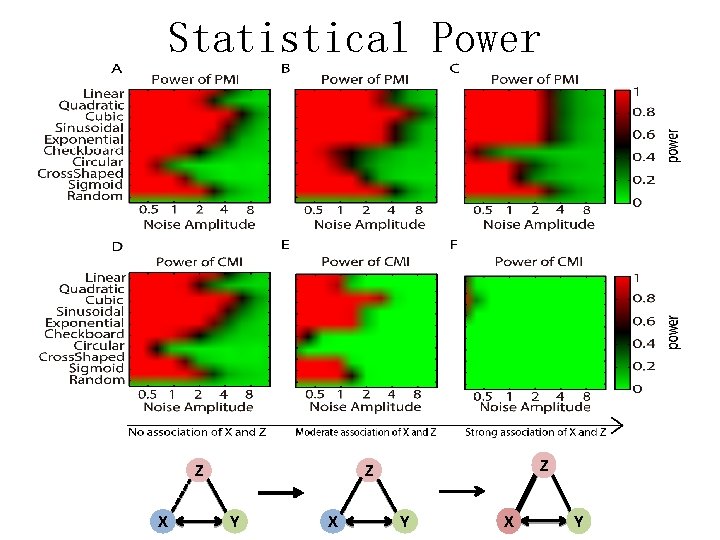
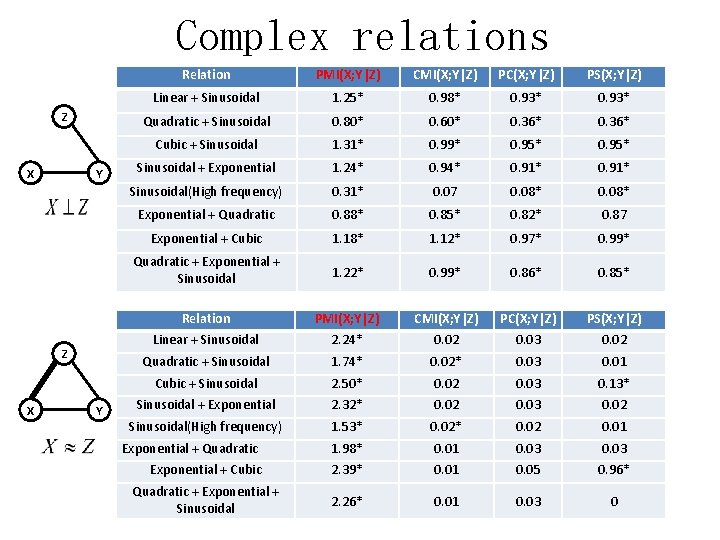
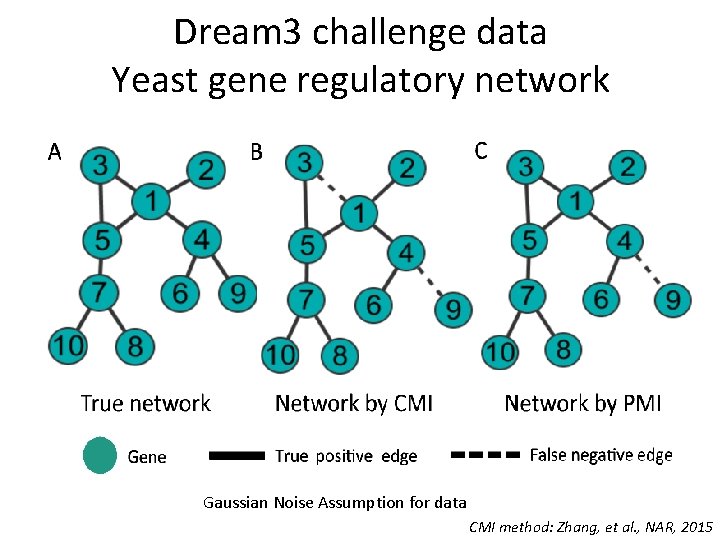
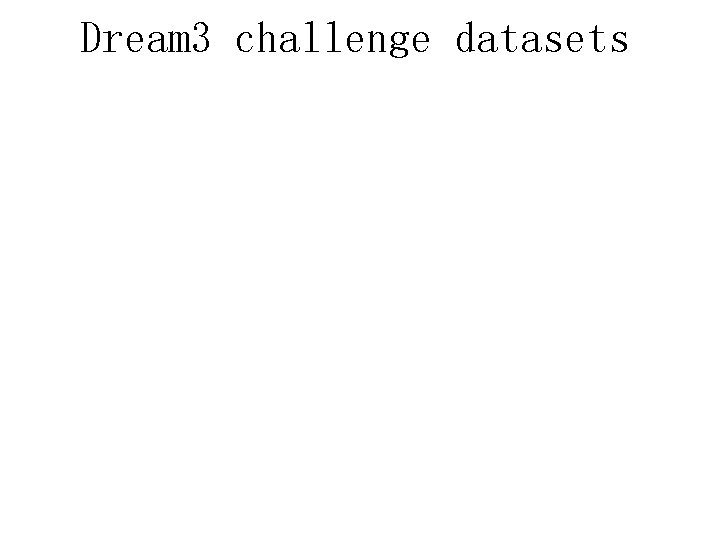
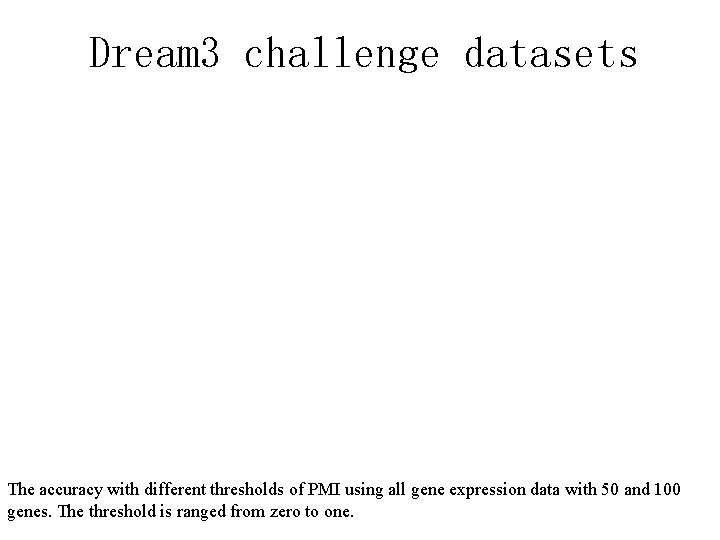
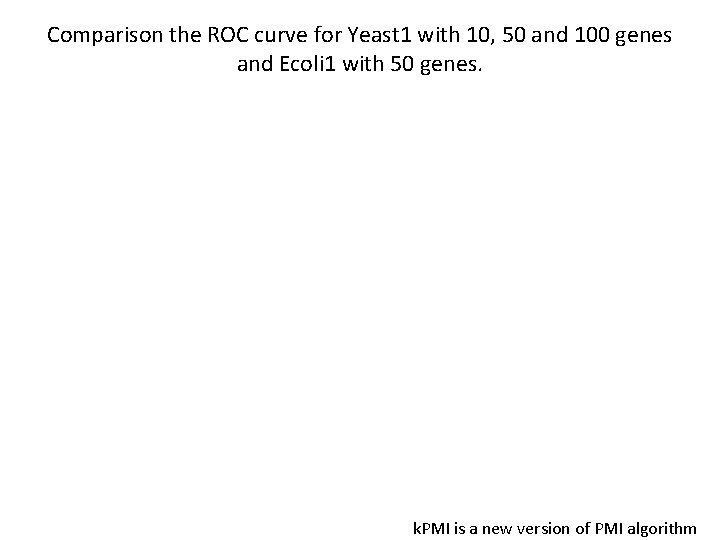
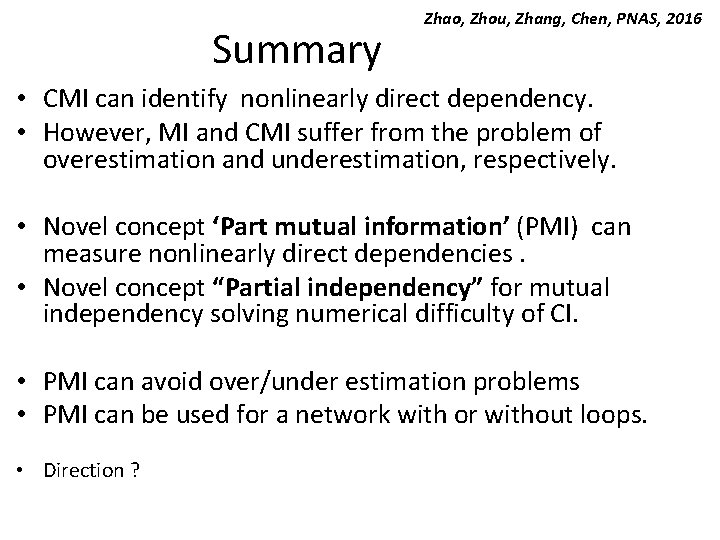
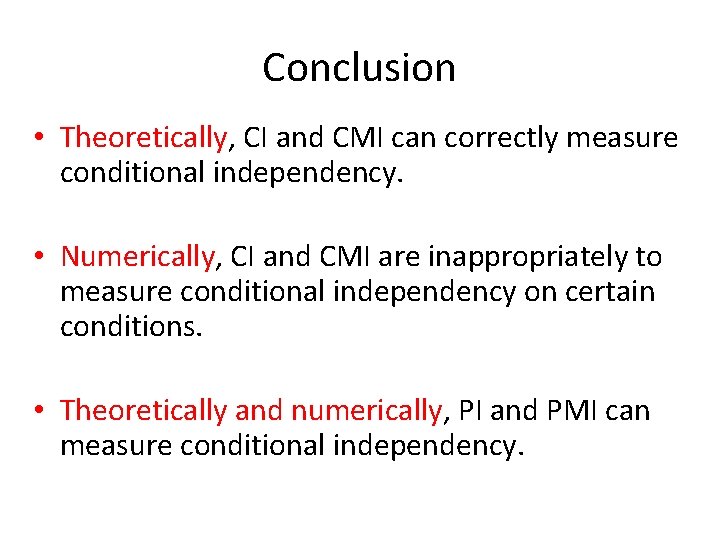
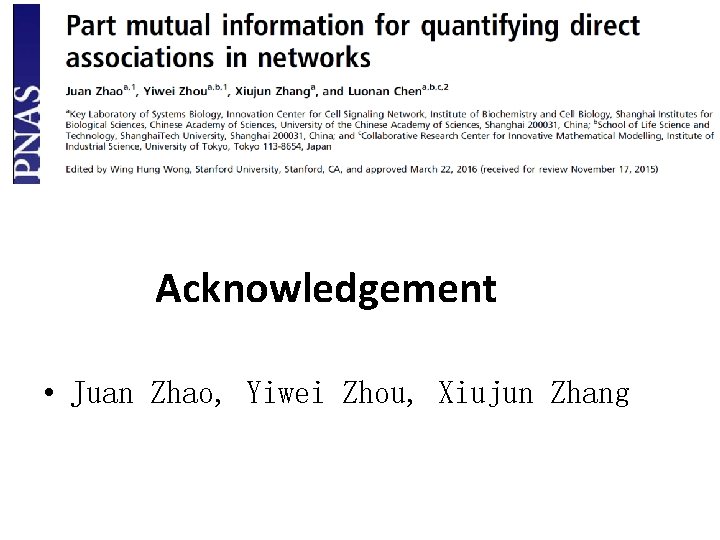
- Slides: 47
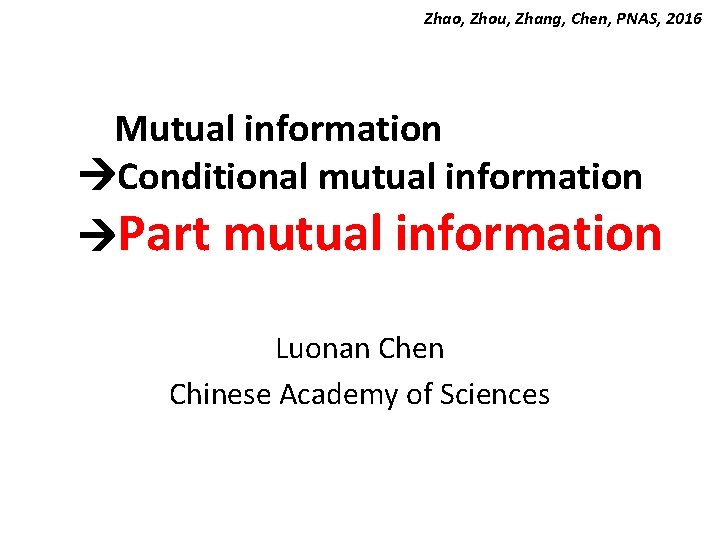
Zhao, Zhou, Zhang, Chen, PNAS, 2016 Mutual information Conditional mutual information Part mutual information Luonan Chen Chinese Academy of Sciences
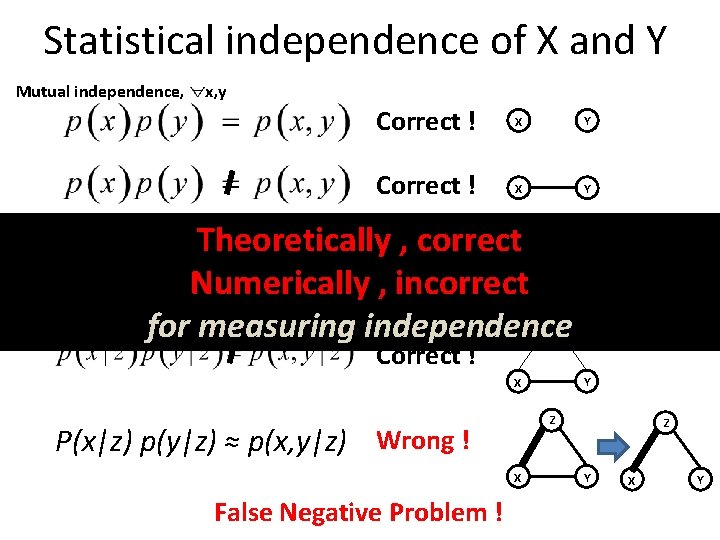
Statistical independence of X and Y Mutual independence, x, y Correct ! X Y Theoretically , correct Correct ! Numerically , incorrect for measuring independence Z Conditional independence, x, y | z X Y Z Correct ! Y X Z P(x|z) p(y|z) ≈ p(x, y|z) Wrong ! X False Negative Problem ! Z Y X Y
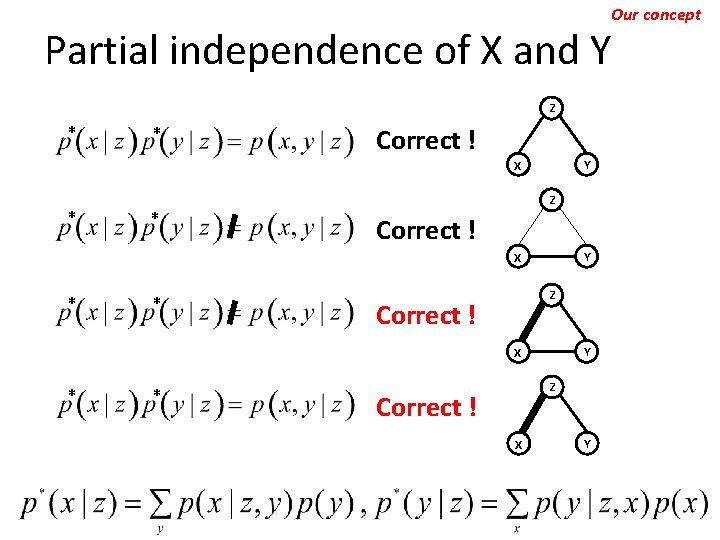
Our concept Partial independence of X and Y Z * * Correct ! Y X * Z * Correct ! Y X * * Z Correct ! X Y
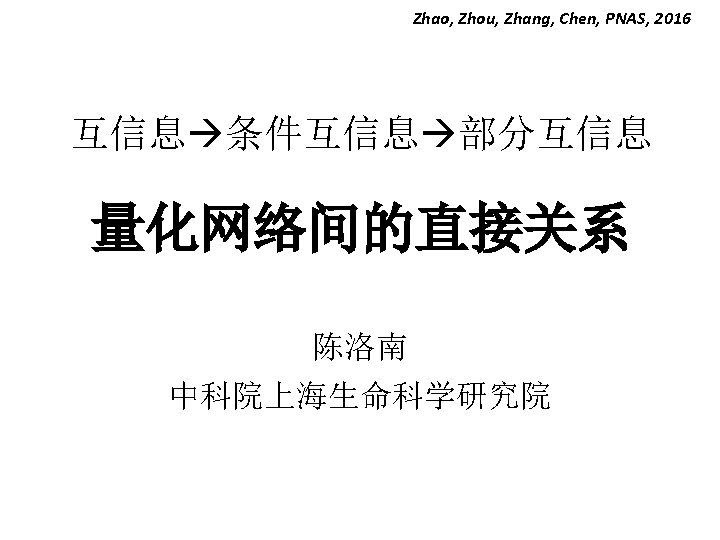
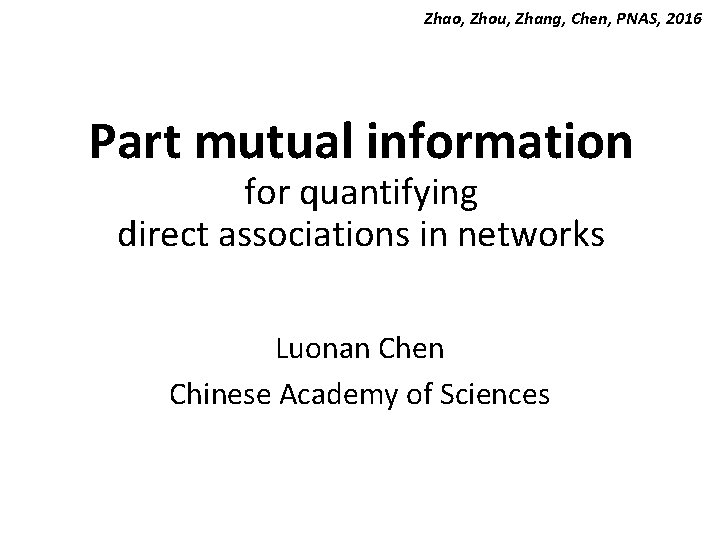
Zhao, Zhou, Zhang, Chen, PNAS, 2016 Part mutual information for quantifying direct associations in networks Luonan Chen Chinese Academy of Sciences
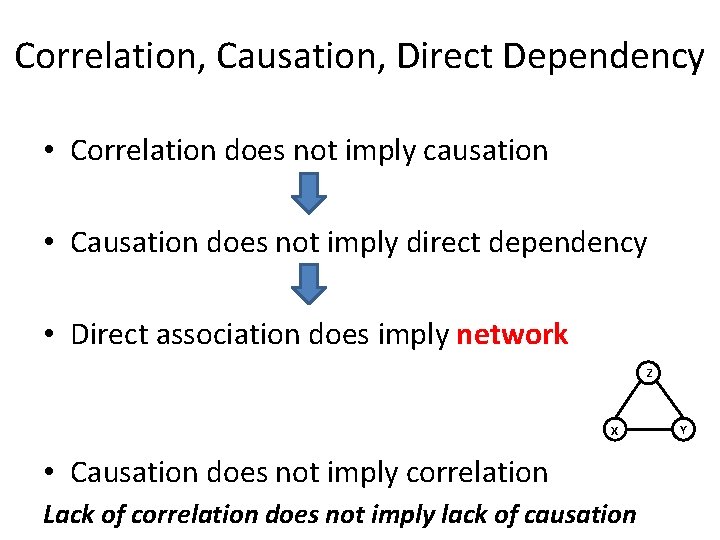
Correlation, Causation, Direct Dependency • Correlation does not imply causation • Causation does not imply direct dependency • Direct association does imply network Z X • Causation does not imply correlation Lack of correlation does not imply lack of causation Y
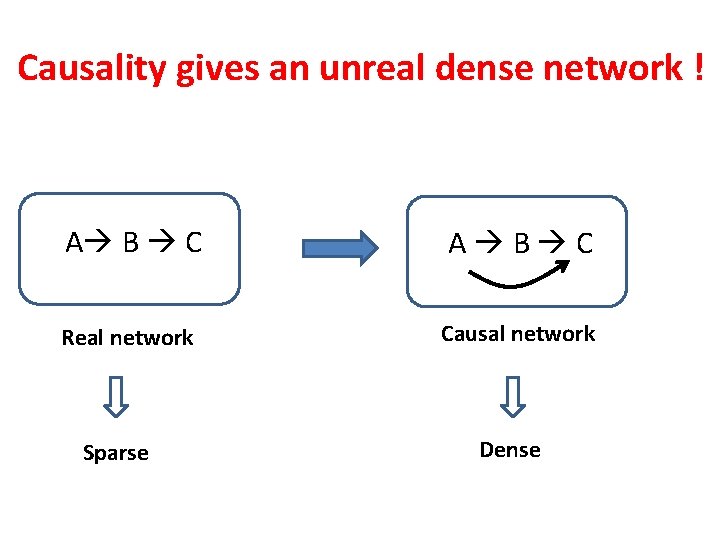
Causality gives an unreal dense network ! A B C A B C Real network Causal network Sparse Dense
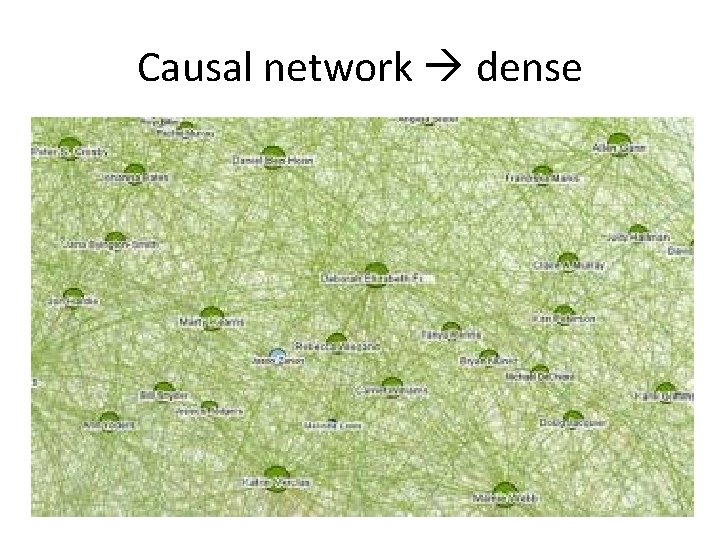
Causal network dense
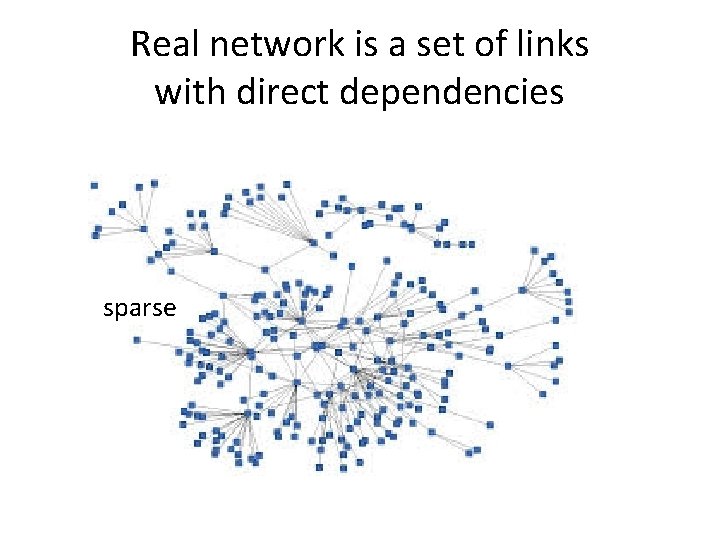
Real network is a set of links with direct dependencies sparse
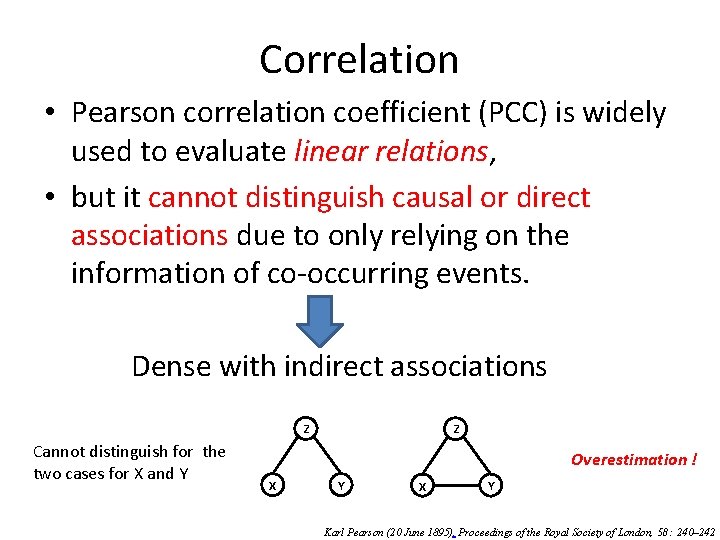
Correlation • Pearson correlation coefficient (PCC) is widely used to evaluate linear relations, • but it cannot distinguish causal or direct associations due to only relying on the information of co-occurring events. : Dense with indirect associations Z Cannot distinguish for the two cases for X and Y Z Overestimation ! X Y Karl Pearson (20 June 1895), Proceedings of the Royal Society of London, 58 : 240– 242
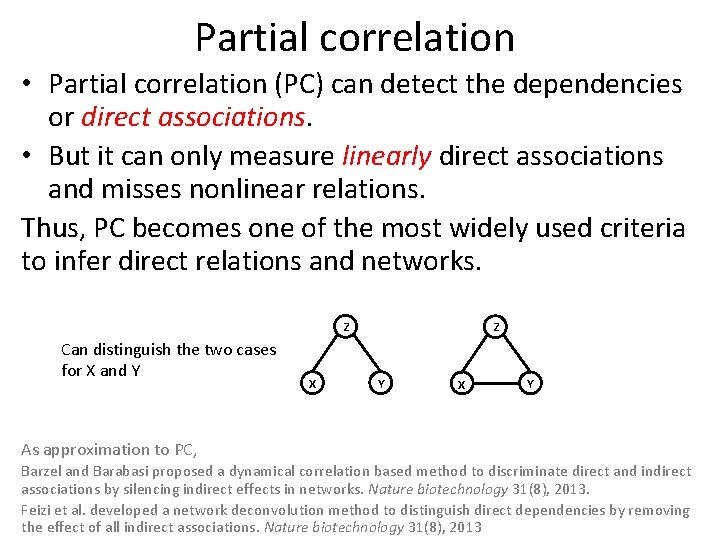
Partial correlation • Partial correlation (PC) can detect the dependencies or direct associations. • But it can only measure linearly direct associations and misses nonlinear relations. Thus, PC becomes one of the most widely used criteria to infer direct relations and networks. Z Can distinguish the two cases for X and Y X Z Y X Y As approximation to PC, Barzel and Barabasi proposed a dynamical correlation based method to discriminate direct and indirect associations by silencing indirect effects in networks. Nature biotechnology 31(8), 2013. Feizi et al. developed a network deconvolution method to distinguish direct dependencies by removing the effect of all indirect associations. Nature biotechnology 31(8), 2013
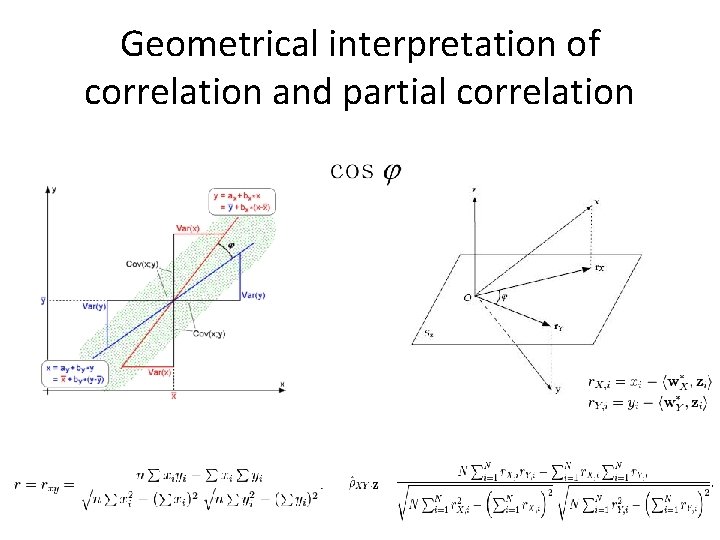
Geometrical interpretation of correlation and partial correlation
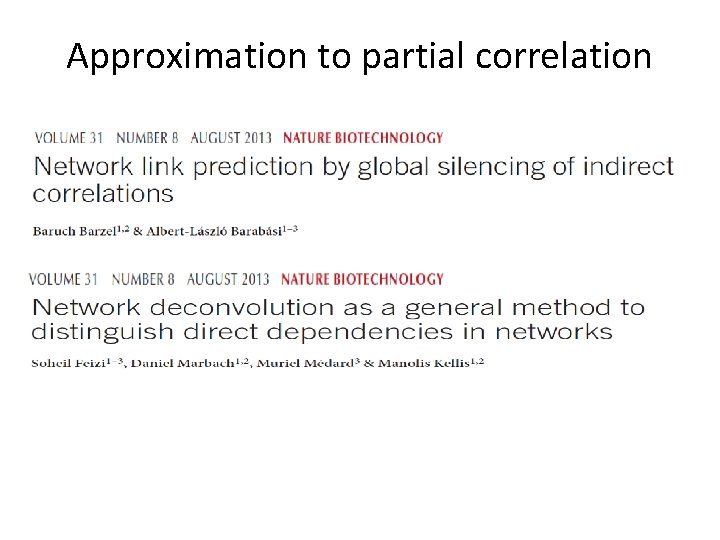
Approximation to partial correlation
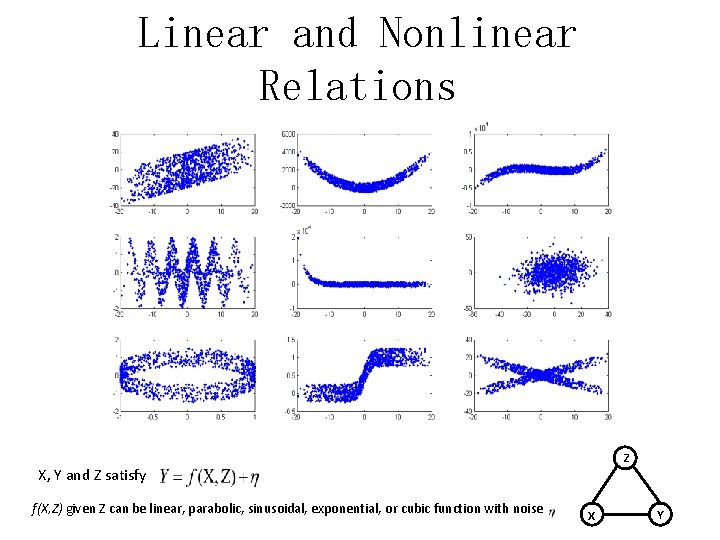
Linear and Nonlinear Relations Z X, Y and Z satisfy f(X, Z) given Z can be linear, parabolic, sinusoidal, exponential, or cubic function with noise X Y
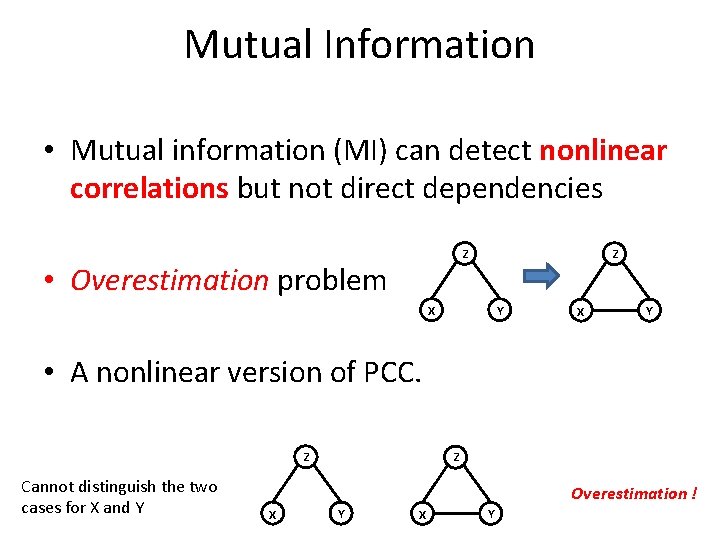
Mutual Information • Mutual information (MI) can detect nonlinear correlations but not direct dependencies Z • Overestimation problem Z Y X X Y • A nonlinear version of PCC. Z Cannot distinguish the two cases for X and Y Z Overestimation ! X Y
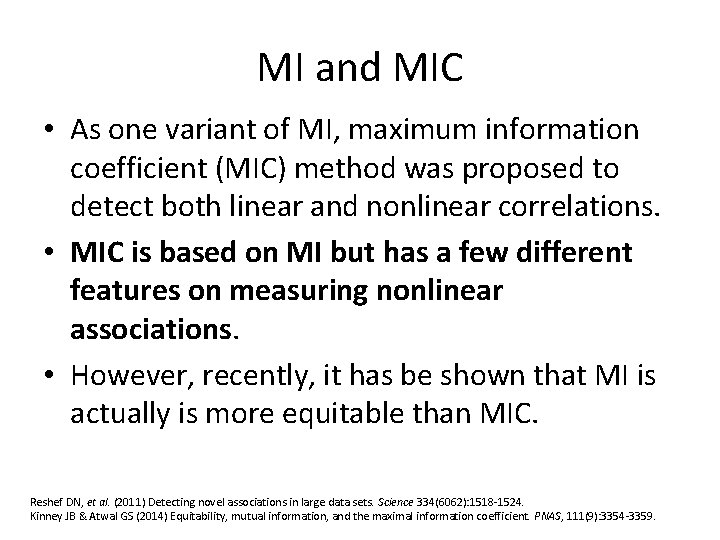
MI and MIC • As one variant of MI, maximum information coefficient (MIC) method was proposed to detect both linear and nonlinear correlations. • MIC is based on MI but has a few different features on measuring nonlinear associations. • However, recently, it has be shown that MI is actually is more equitable than MIC. Reshef DN, et al. (2011) Detecting novel associations in large data sets. Science 334(6062): 1518 -1524. Kinney JB & Atwal GS (2014) Equitability, mutual information, and the maximal information coefficient. PNAS, 111(9): 3354 -3359.
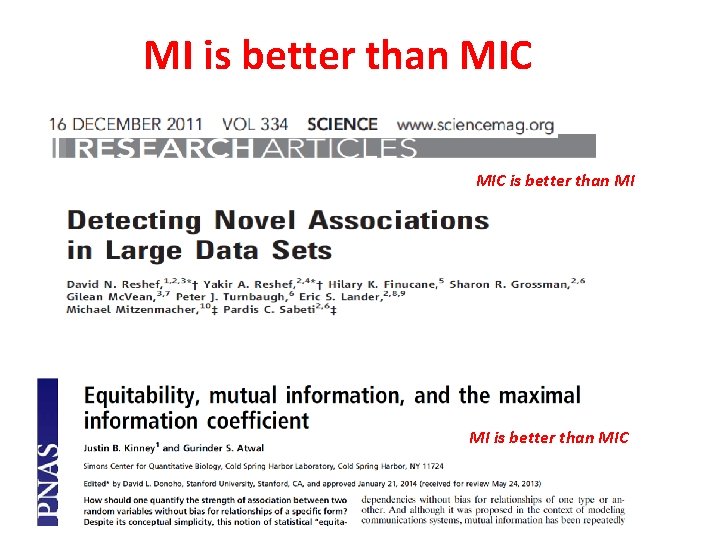
MI is better than MIC is better than MI MI is better than MIC
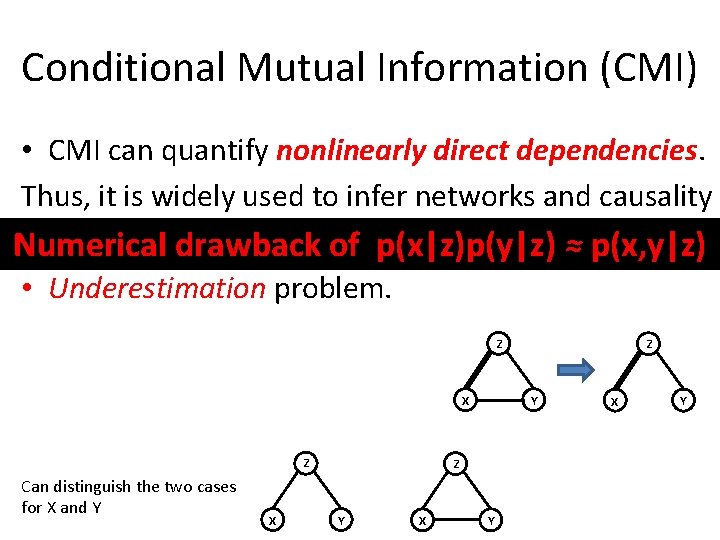
Conditional Mutual Information (CMI) • CMI can quantify nonlinearly direct dependencies. Thus, it is widely used to infer networks and causality Numerical drawback of p(x|z)p(y|z) ≈ p(x, y|z) • Underestimation problem. Z Y X Z Can distinguish the two cases for X and Y X Z Y X Y
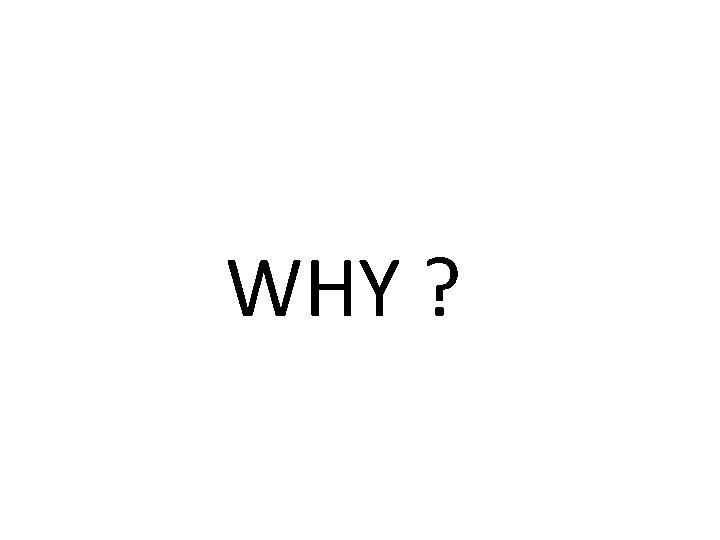
WHY ?
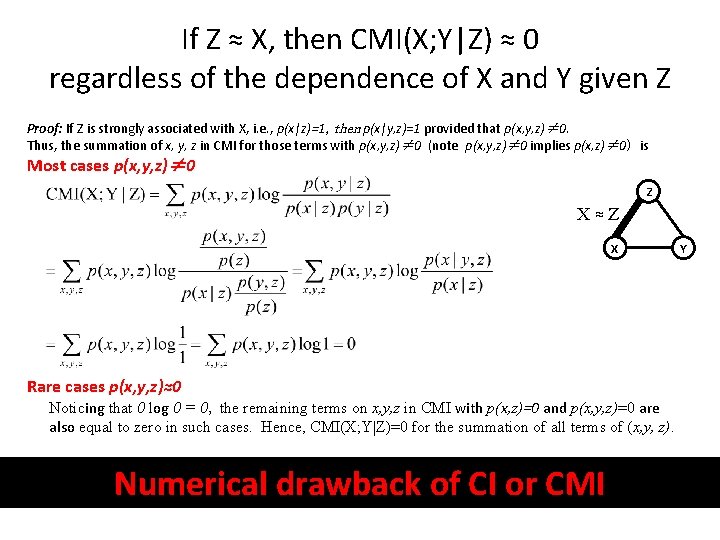
If Z ≈ X, then CMI(X; Y|Z) ≈ 0 regardless of the dependence of X and Y given Z Proof: If Z is strongly associated with X, i. e. , p(x|z)=1, then p(x|y, z)=1 provided that p(x, y, z)≠ 0. Thus, the summation of x, y, z in CMI for those terms with p(x, y, z)≠ 0 (note p(x, y, z)≠ 0 implies p(x, z)≠ 0) is Most cases p(x, y, z)≠ 0 Z X≈Z X Rare cases p(x, y, z)≈0 Noticing that 0 log 0 = 0, the remaining terms on x, y, z in CMI with p(x, z)=0 and p(x, y, z)=0 are also equal to zero in such cases. Hence, CMI(X; Y|Z)=0 for the summation of all terms of (x, y, z). Numerical drawback of CI or CMI Y
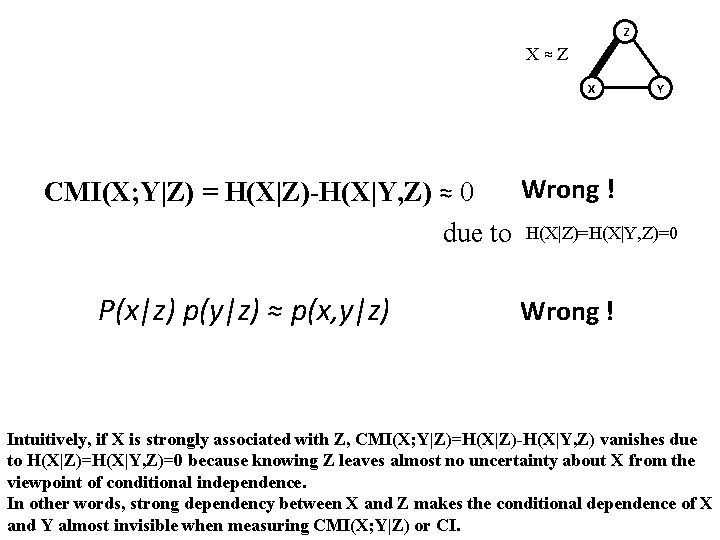
Z X≈Z X Y Wrong ! CMI(X; Y|Z) = H(X|Z)-H(X|Y, Z) ≈ 0 due to H(X|Z)=H(X|Y, Z)=0 P(x|z) p(y|z) ≈ p(x, y|z) Wrong ! Intuitively, if X is strongly associated with Z, CMI(X; Y|Z)=H(X|Z)-H(X|Y, Z) vanishes due to H(X|Z)=H(X|Y, Z)=0 because knowing Z leaves almost no uncertainty about X from the viewpoint of conditional independence. In other words, strong dependency between X and Z makes the conditional dependence of X and Y almost invisible when measuring CMI(X; Y|Z) or CI.
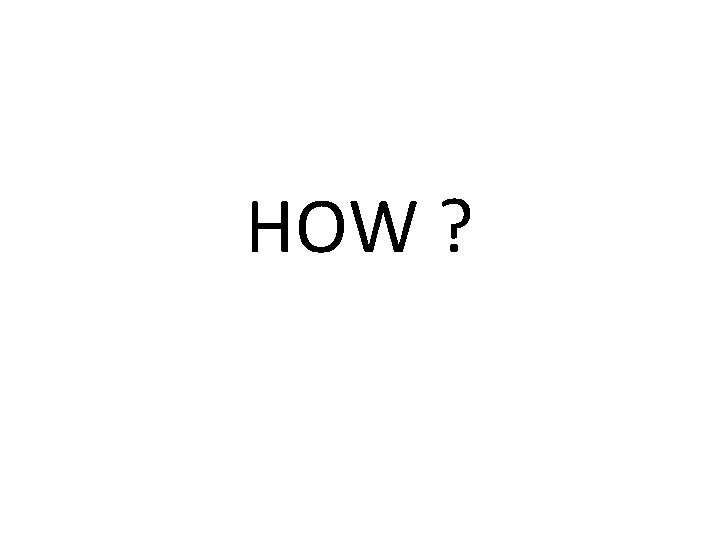
HOW ?
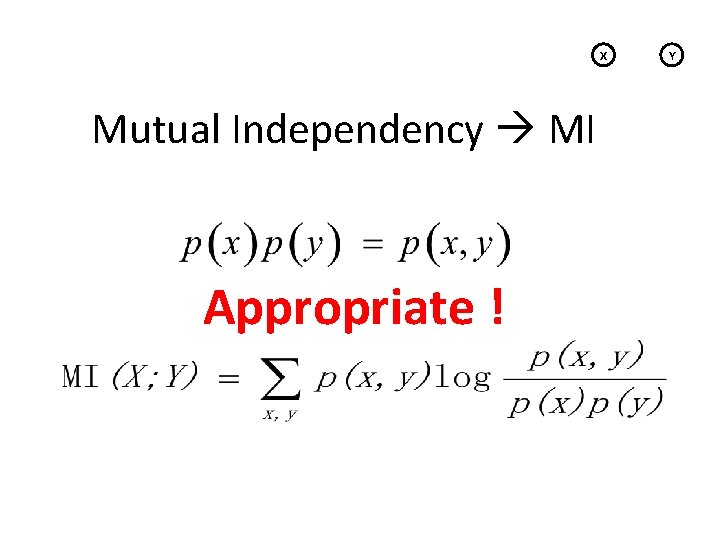
X Mutual Independency MI Appropriate ! Y
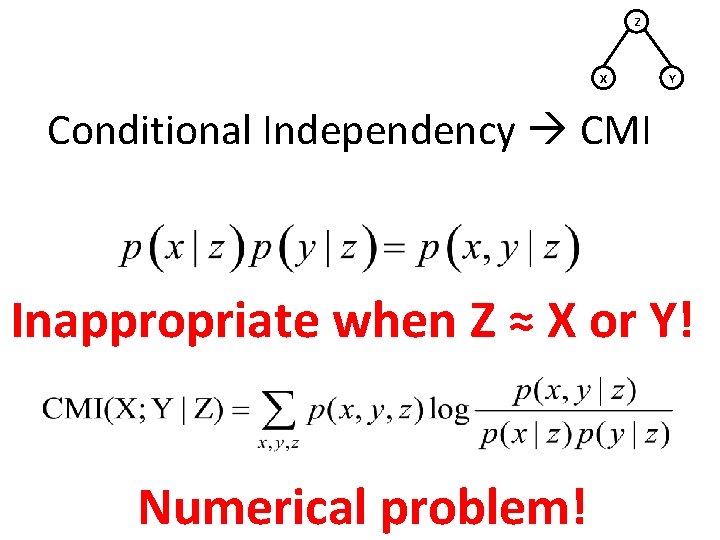
Z X Y Conditional Independency CMI Inappropriate when Z ≈ X or Y! Numerical problem!
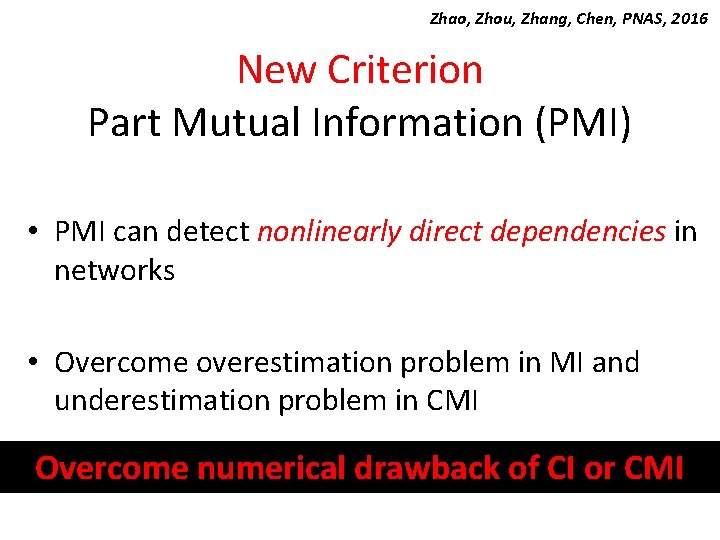
Zhao, Zhou, Zhang, Chen, PNAS, 2016 New Criterion Part Mutual Information (PMI) • PMI can detect nonlinearly direct dependencies in networks • Overcome overestimation problem in MI and underestimation problem in CMI Overcome numerical drawback of CI or CMI
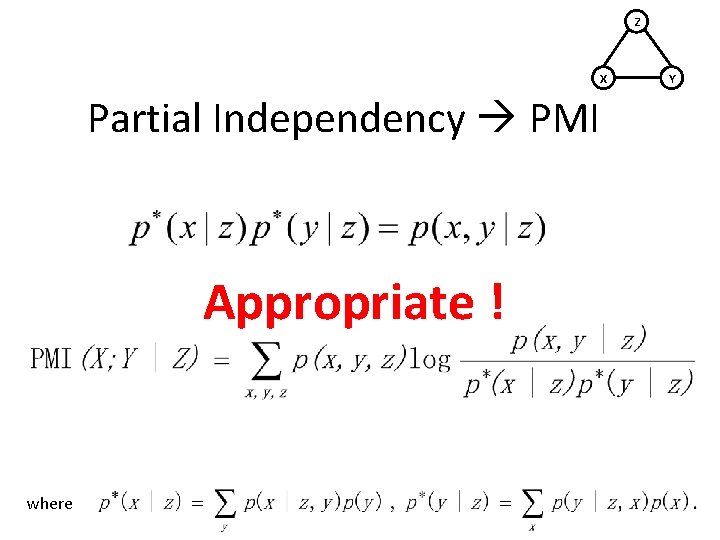
Z X Partial Independency PMI Appropriate ! where Y
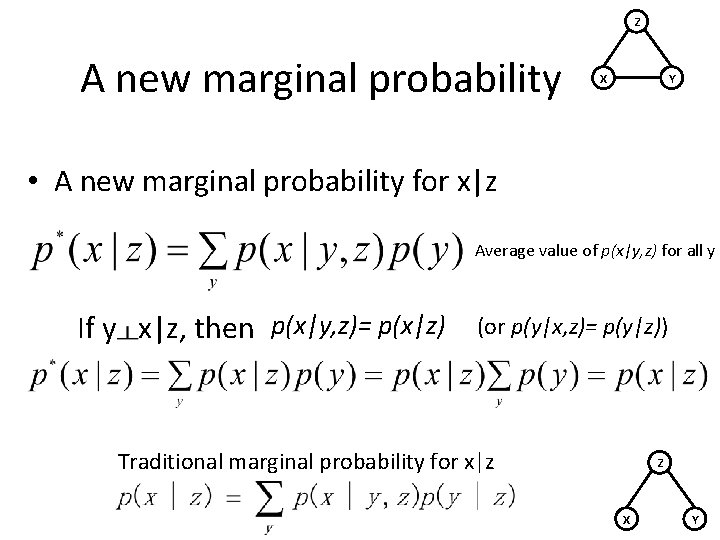
Z A new marginal probability Y X • A new marginal probability for x|z Average value of p(x|y, z) for all y If y x|z, then p(x|y, z)= p(x|z) (or p(y|x, z)= p(y|z)) Traditional marginal probability for x|z Z X Y
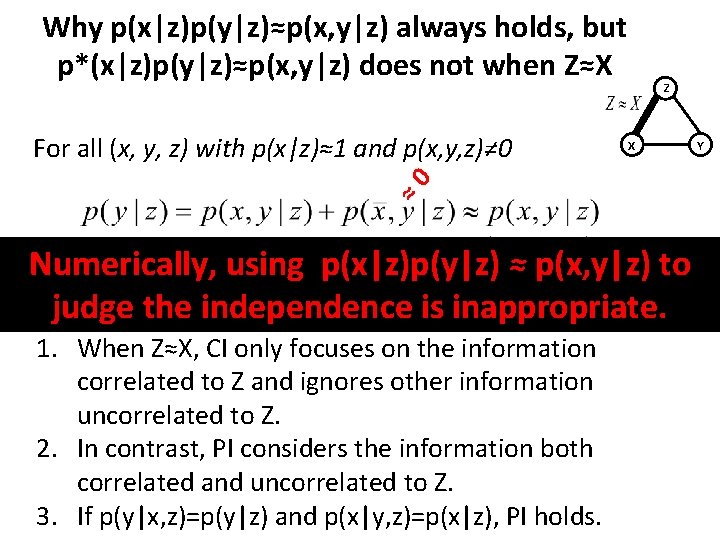
Why p(x|z)p(y|z)≈p(x, y|z) always holds, but p*(x|z)p(y|z)≈p(x, y|z) does not when Z≈X For all (x, y, z) with p(x|z)≈1 and p(x, y, z)≠ 0 Z ≈0 X ≠ 0 Numerically, using p(x|z)p(y|z) ≈ p(x, y|z) to judge the independence is inappropriate. 1. When Z≈X, CI only focuses on the information correlated to Z and ignores other information uncorrelated to Z. 2. In contrast, PI considers the information both correlated and uncorrelated to Z. 3. If p(y|x, z)=p(y|z) and p(x|y, z)=p(x|z), PI holds. Y
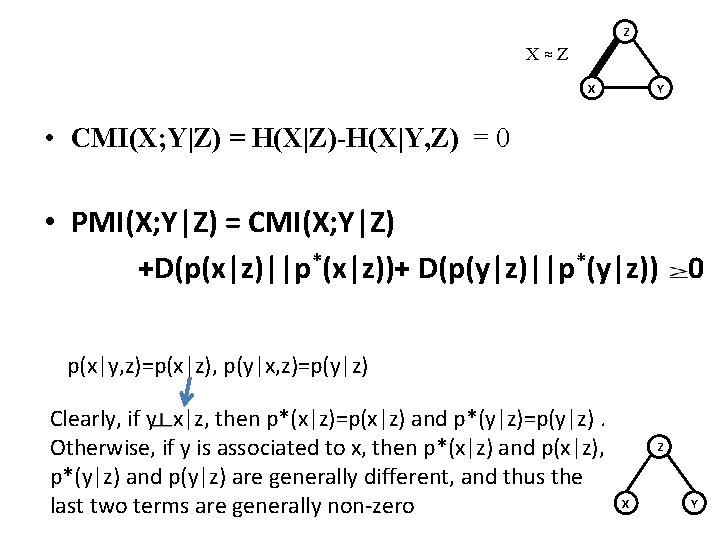
Z X≈Z Y X • CMI(X; Y|Z) = H(X|Z)-H(X|Y, Z) = 0 • PMI(X; Y|Z) = CMI(X; Y|Z) +D(p(x|z)||p*(x|z))+ D(p(y|z)||p*(y|z)) 0 p(x|y, z)=p(x|z), p(y|x, z)=p(y|z) Clearly, if y x|z, then p*(x|z)=p(x|z) and p*(y|z)=p(y|z). Otherwise, if y is associated to x, then p*(x|z) and p(x|z), p*(y|z) and p(y|z) are generally different, and thus the last two terms are generally non-zero Z X Y
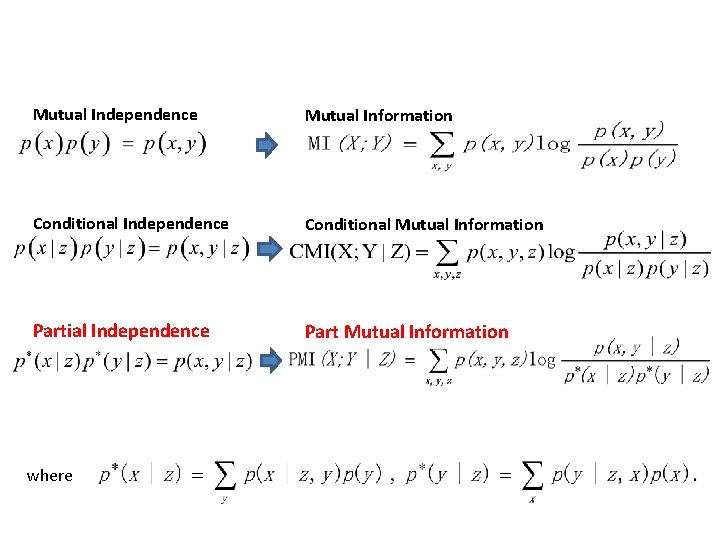
Mutual Independence Mutual Information Conditional Independence Conditional Mutual Information Partial Independence Part Mutual Information where
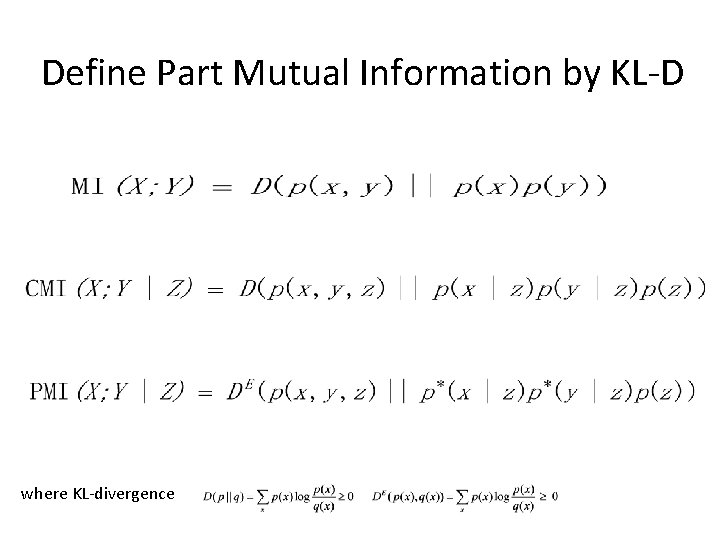
Define Part Mutual Information by KL-D where KL-divergence
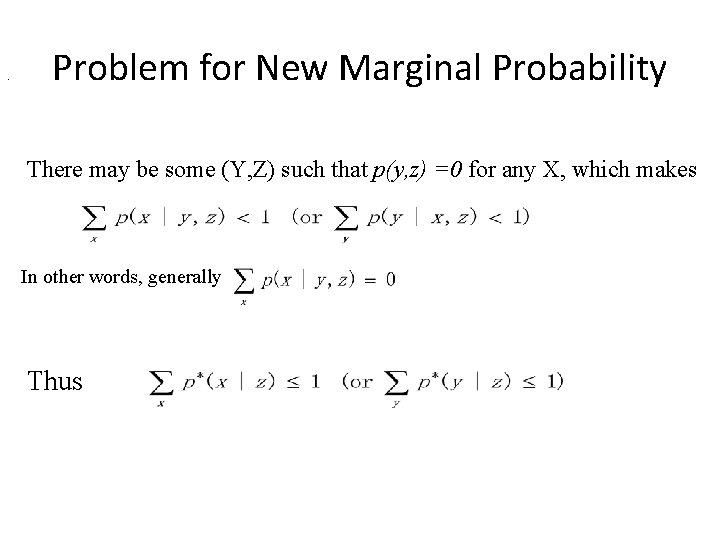
. Problem for New Marginal Probability There may be some (Y, Z) such that p(y, z) =0 for any X, which makes In other words, generally Thus
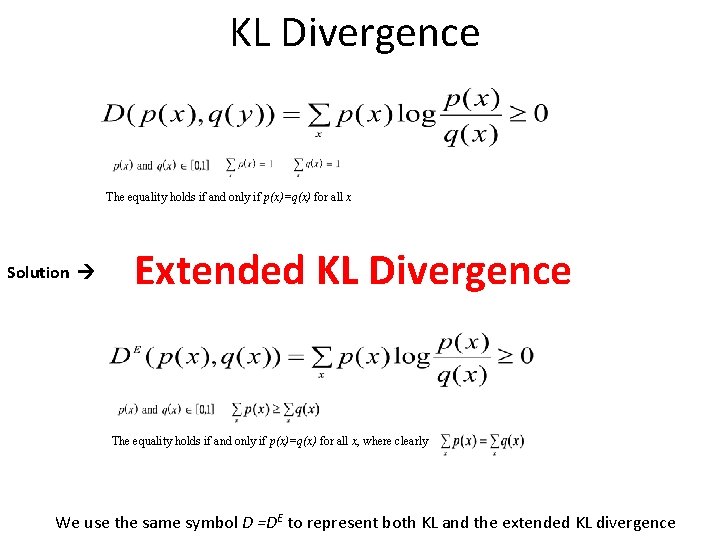
KL Divergence The equality holds if and only if p(x)=q(x) for all x Solution Extended KL Divergence The. equality holds if and only if p(x)=q(x) for all x, where clearly We use the same symbol D =DE to represent both KL and the extended KL divergence
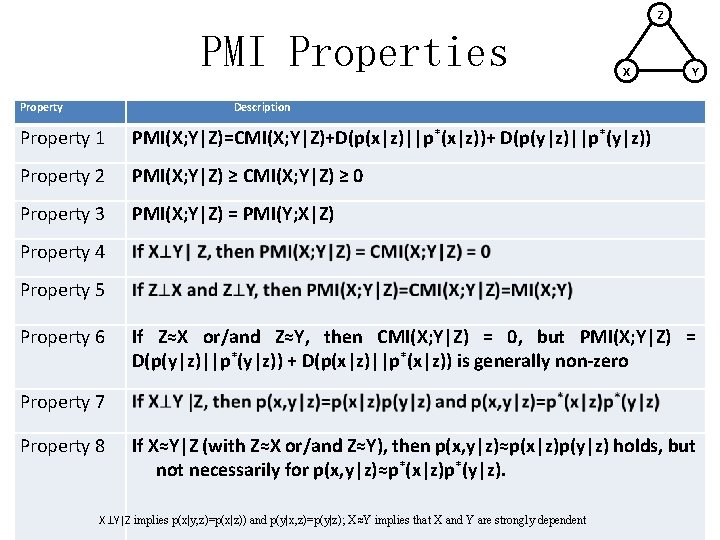
Z PMI Properties Property X Y Description Property 1 PMI(X; Y|Z)=CMI(X; Y|Z)+D(p(x|z)||p*(x|z))+ D(p(y|z)||p*(y|z)) Property 2 PMI(X; Y|Z) ≥ CMI(X; Y|Z) ≥ 0 Property 3 PMI(X; Y|Z) = PMI(Y; X|Z) Property 4 Property 5 Property 6 If Z≈X or/and Z≈Y, then CMI(X; Y|Z) = 0, but PMI(X; Y|Z) = D(p(y|z)||p*(y|z)) + D(p(x|z)||p*(x|z)) is generally non-zero Property 7 Property 8 If X≈Y|Z (with Z≈X or/and Z≈Y), then p(x, y|z)≈p(x|z)p(y|z) holds, but not necessarily for p(x, y|z)≈p*(x|z)p*(y|z). X⊥Y|Z implies p(x|y, z)=p(x|z)) and p(y|x, z)=p(y|z); X≈Y implies that X and Y are strongly dependent
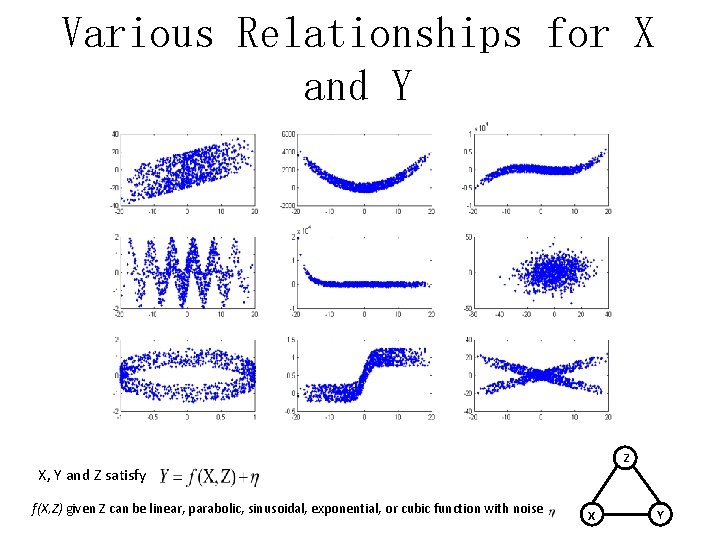
Various Relationships for X and Y Z X, Y and Z satisfy f(X, Z) given Z can be linear, parabolic, sinusoidal, exponential, or cubic function with noise X Y
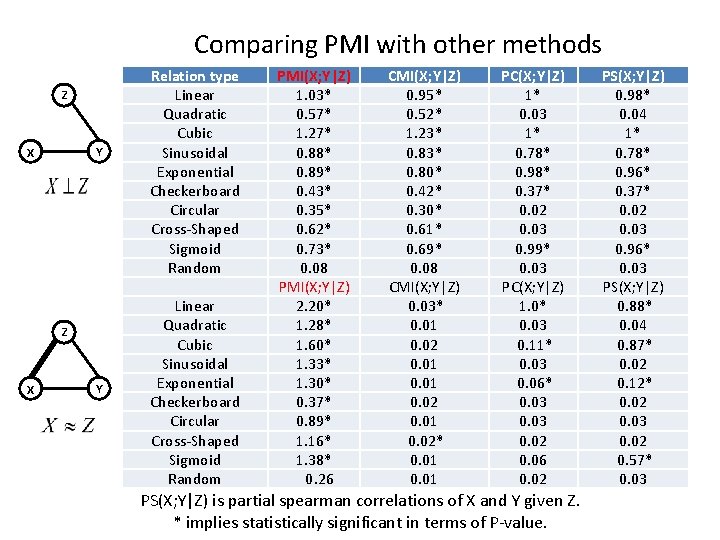
Comparing PMI with other methods Z Y X Z X Y Relation type Linear Quadratic Cubic Sinusoidal Exponential Checkerboard Circular Cross-Shaped Sigmoid Random PMI(X; Y|Z) 1. 03* 0. 57* 1. 27* 0. 88* 0. 89* 0. 43* 0. 35* 0. 62* 0. 73* 0. 08 PMI(X; Y|Z) 2. 20* 1. 28* 1. 60* 1. 33* 1. 30* 0. 37* 0. 89* 1. 16* 1. 38* 0. 26 CMI(X; Y|Z) 0. 95* 0. 52* 1. 23* 0. 80* 0. 42* 0. 30* 0. 61* 0. 69* 0. 08 CMI(X; Y|Z) 0. 03* 0. 01 0. 02* 0. 01 PC(X; Y|Z) 1* 0. 03 1* 0. 78* 0. 98* 0. 37* 0. 02 0. 03 0. 99* 0. 03 PC(X; Y|Z) 1. 0* 0. 03 0. 11* 0. 03 0. 06* 0. 03 0. 02 0. 06 0. 02 PS(X; Y|Z) is partial spearman correlations of X and Y given Z. * implies statistically significant in terms of P-value. PS(X; Y|Z) 0. 98* 0. 04 1* 0. 78* 0. 96* 0. 37* 0. 02 0. 03 0. 96* 0. 03 PS(X; Y|Z) 0. 88* 0. 04 0. 87* 0. 02 0. 12* 0. 02 0. 03 0. 02 0. 57* 0. 03
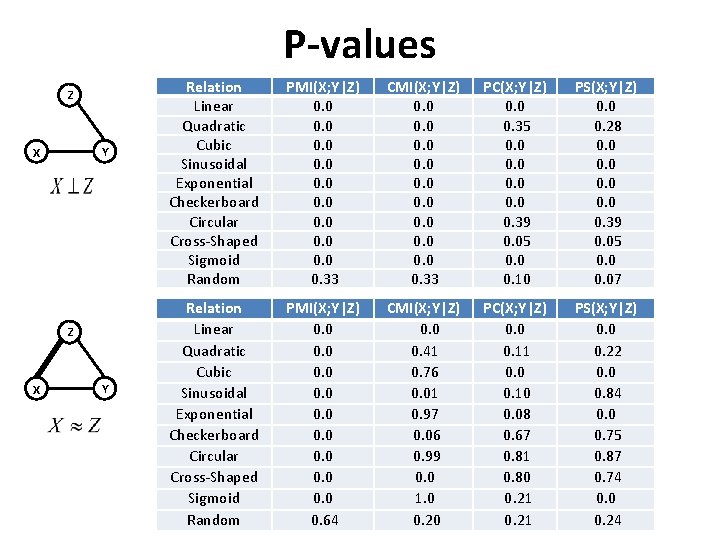
P-values Z Y X Z X Y Relation Linear Quadratic Cubic Sinusoidal Exponential Checkerboard Circular Cross-Shaped Sigmoid Random PMI(X; Y|Z) 0. 0 0. 33 CMI(X; Y|Z) 0. 0 0. 33 PC(X; Y|Z) 0. 0 0. 35 0. 0 0. 39 0. 05 0. 0 0. 10 PS(X; Y|Z) 0. 0 0. 28 0. 0 0. 39 0. 05 0. 07 Relation Linear Quadratic Cubic Sinusoidal Exponential Checkerboard Circular Cross-Shaped Sigmoid Random PMI(X; Y|Z) 0. 0 0. 64 CMI(X; Y|Z) 0. 0 0. 41 0. 76 0. 01 0. 97 0. 06 0. 99 0. 0 1. 0 0. 20 PC(X; Y|Z) 0. 0 0. 11 0. 0 0. 10 0. 08 0. 67 0. 81 0. 80 0. 21 PS(X; Y|Z) 0. 0 0. 22 0. 0 0. 84 0. 0 0. 75 0. 87 0. 74 0. 0 0. 24
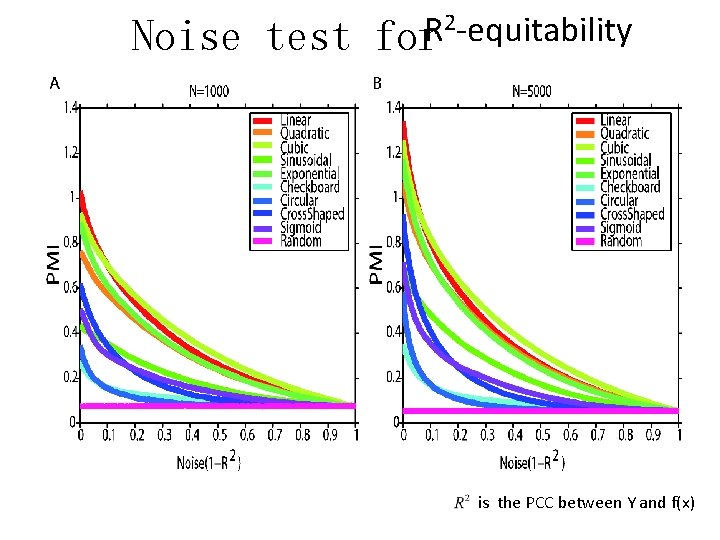
Noise test 2 -equitability R for is the PCC between Y and f(x)
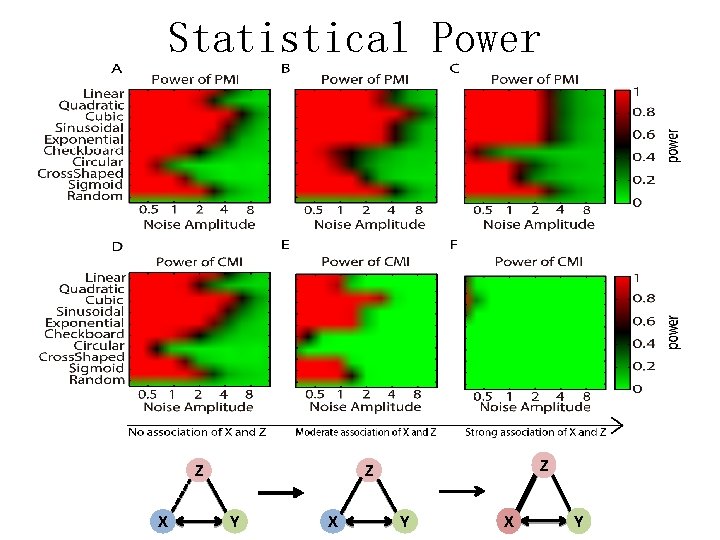
Statistical Power Z X Z Z Y X Y
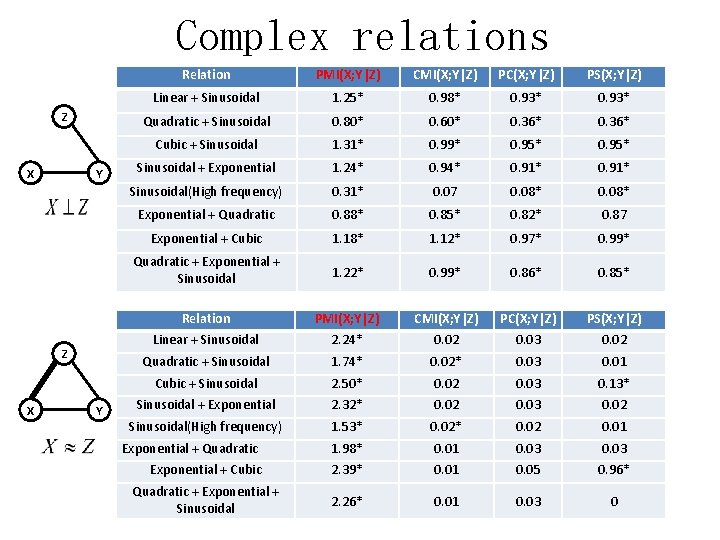
Complex relations Z Y X Z X Y Relation PMI(X; Y|Z) CMI(X; Y|Z) PC(X; Y|Z) PS(X; Y|Z) Linear + Sinusoidal 1. 25* 0. 98* 0. 93* Quadratic + Sinusoidal 0. 80* 0. 60* 0. 36* Cubic + Sinusoidal 1. 31* 0. 99* 0. 95* Sinusoidal + Exponential 1. 24* 0. 91* Sinusoidal(High frequency) 0. 31* 0. 07 0. 08* Exponential + Quadratic 0. 88* 0. 85* 0. 82* 0. 87 Exponential + Cubic 1. 18* 1. 12* 0. 97* 0. 99* Quadratic + Exponential + Sinusoidal 1. 22* 0. 99* 0. 86* 0. 85* Relation PMI(X; Y|Z) CMI(X; Y|Z) PC(X; Y|Z) PS(X; Y|Z) Linear + Sinusoidal 2. 24* 0. 02 0. 03 0. 02 Quadratic + Sinusoidal 1. 74* 0. 02* 0. 03 0. 01 Cubic + Sinusoidal 2. 50* 0. 02 0. 03 0. 13* Sinusoidal + Exponential 2. 32* 0. 02 0. 03 0. 02 Sinusoidal(High frequency) 1. 53* 0. 02 0. 01 Exponential + Quadratic 1. 98* 0. 01 0. 03 Exponential + Cubic 2. 39* 0. 01 0. 05 0. 96* Quadratic + Exponential + Sinusoidal 2. 26* 0. 01 0. 03 0
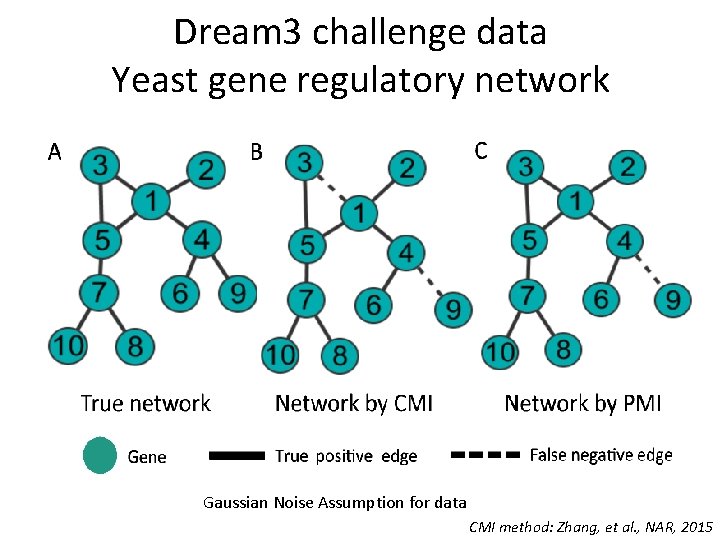
Dream 3 challenge data Yeast gene regulatory network Gaussian Noise Assumption for data CMI method: Zhang, et al. , NAR, 2015
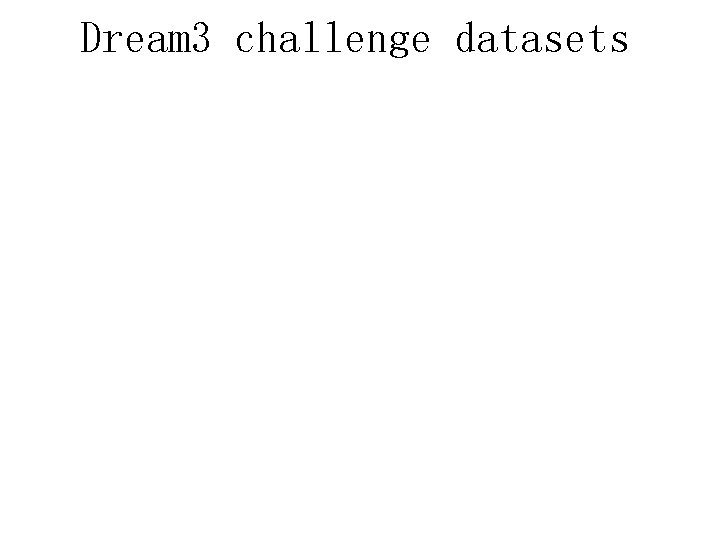
Dream 3 challenge datasets
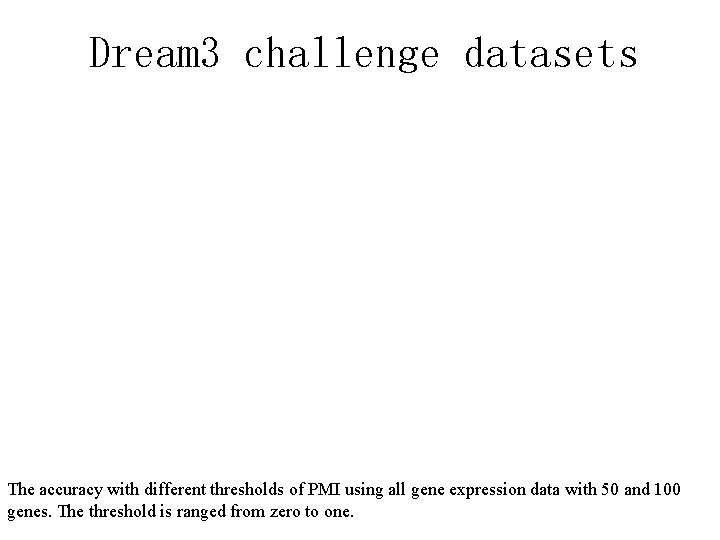
Dream 3 challenge datasets The accuracy with different thresholds of PMI using all gene expression data with 50 and 100 genes. The threshold is ranged from zero to one.
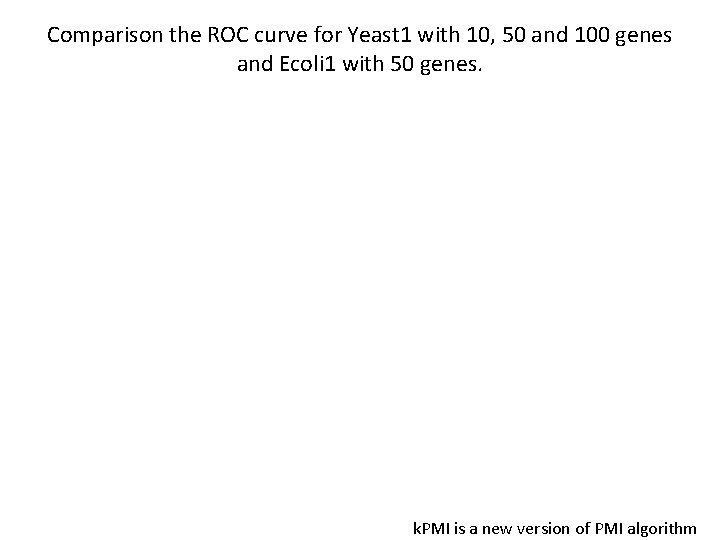
Comparison the ROC curve for Yeast 1 with 10, 50 and 100 genes and Ecoli 1 with 50 genes. k. PMI is a new version of PMI algorithm
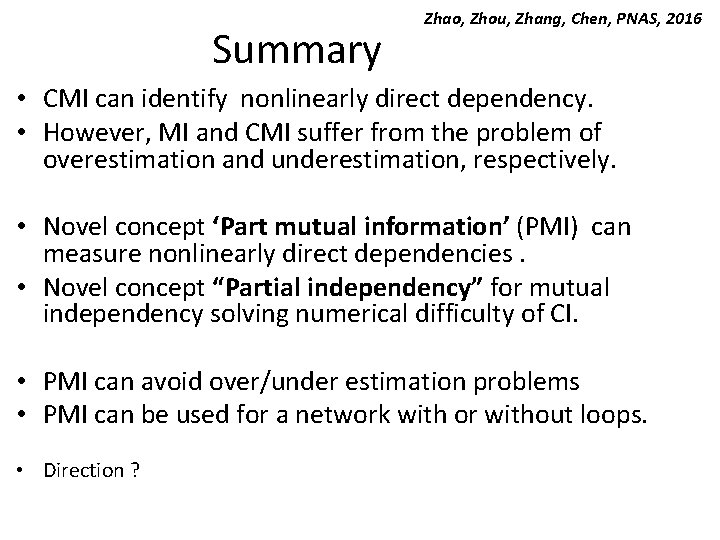
Summary Zhao, Zhou, Zhang, Chen, PNAS, 2016 • CMI can identify nonlinearly direct dependency. • However, MI and CMI suffer from the problem of overestimation and underestimation, respectively. • Novel concept ‘Part mutual information’ (PMI) can measure nonlinearly direct dependencies. • Novel concept “Partial independency” for mutual independency solving numerical difficulty of CI. • PMI can avoid over/under estimation problems • PMI can be used for a network with or without loops. • Direction ?
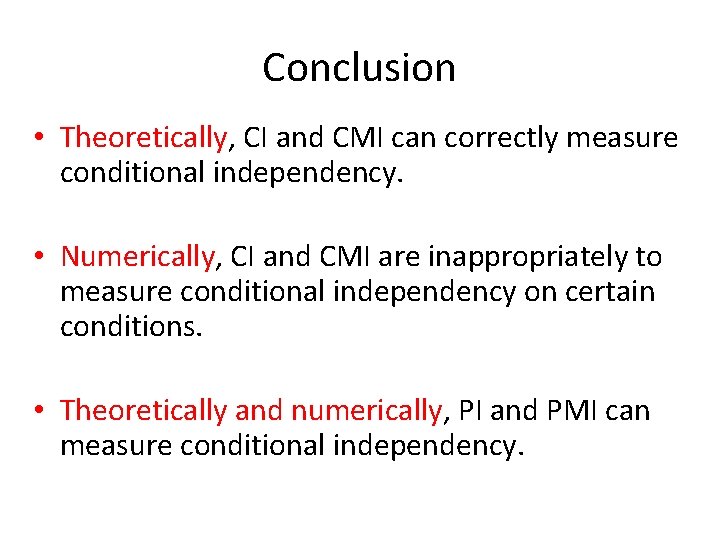
Conclusion • Theoretically, CI and CMI can correctly measure conditional independency. • Numerically, CI and CMI are inappropriately to measure conditional independency on certain conditions. • Theoretically and numerically, PI and PMI can measure conditional independency.
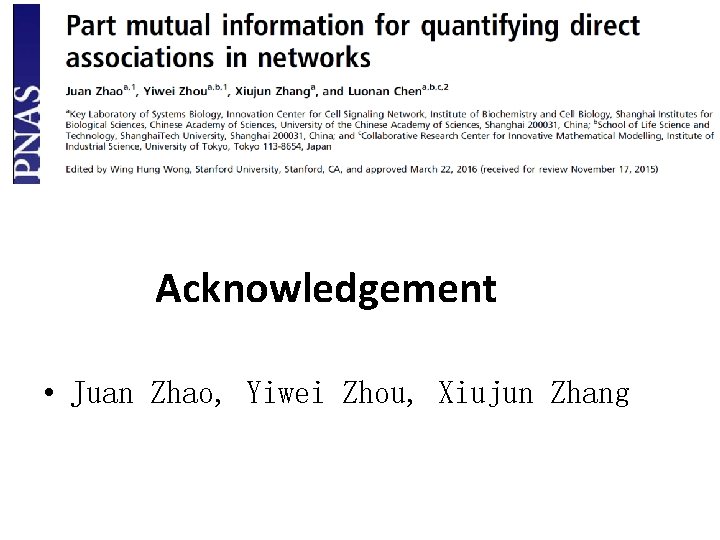
Acknowledgement • Juan Zhao, Yiwei Zhou, Xiujun Zhang
Guttman effect
Pnas presubmission inquiry
Chen chen berlin
Mutual correlation
Palabras con d y r
Hui zhao unlv
Dr jennifer a. baima
Zhao chun
Irene zhao dao
Irene zhao dao
Jeremy zhao
Ni zhao
David zhao md
Zhuoyue zhao
David zhao md
Zhao qian malaysia
Shangqing zhao
Mingda zhao
Princess pingyang
Zhao tingyang
Xin zhao challenge
Zhao cuiwei
"tcm"
Amanda zhao
Amanda zhao
Zhengji zhao
Mingmin zhao mit
Daniel propson
Ben y zhao
Wenbing zhao
Wenbing zhao
Mandate of heaven ancient china
Zhou dynasty facts
Pulse strecher
Wenchao zhou
Shuheng zhou
Huang ho river valley civilization
Huping zhou md
Zhou dynasty facts
Chinese dynasties in order song
Shang zhou qin han
River dynasties in china chapter 2 section 4
Zhou dynasty achievements
Runfang zhou
King cheng of zhou
Ancient china han
Feudalism zhou dynasty
Giulio zhou