WORKSHOP Data Management Concepts and Data Profiling Getting
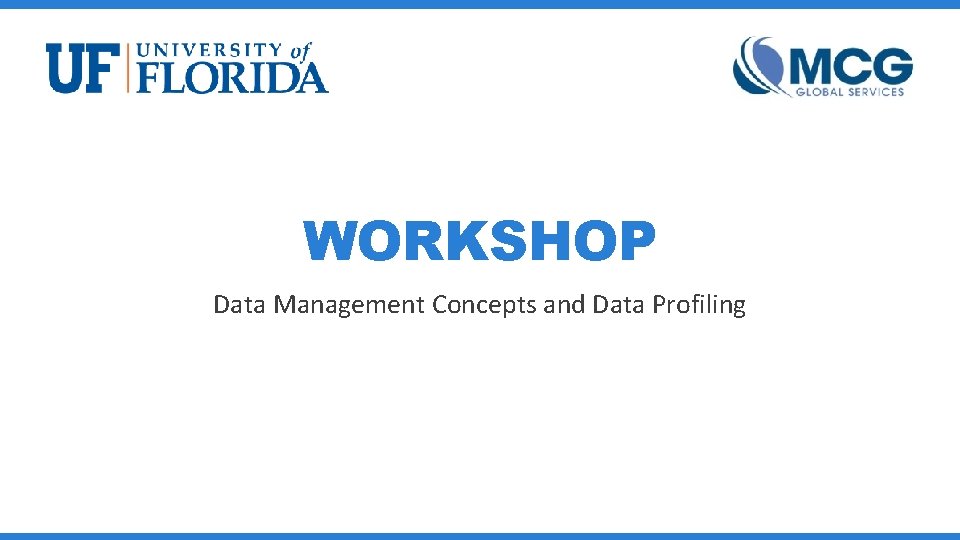
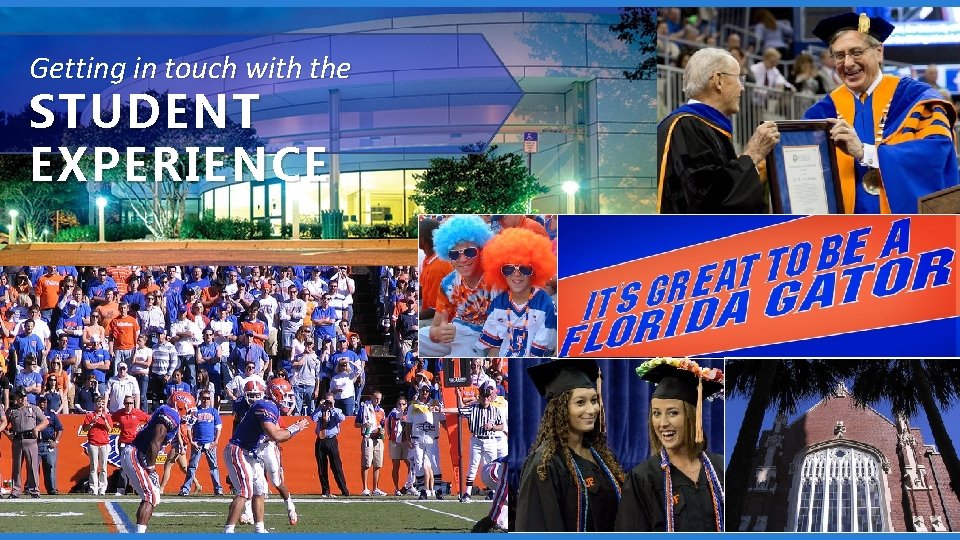
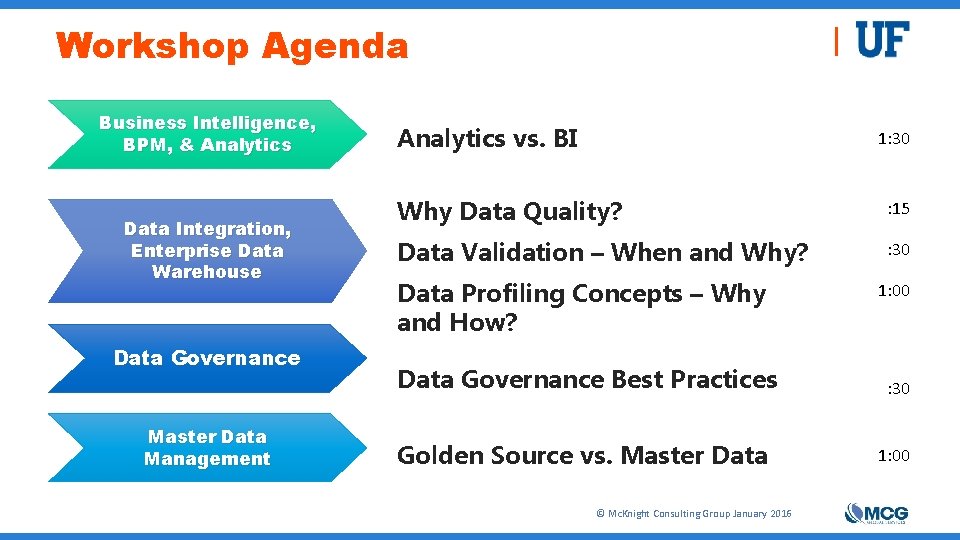
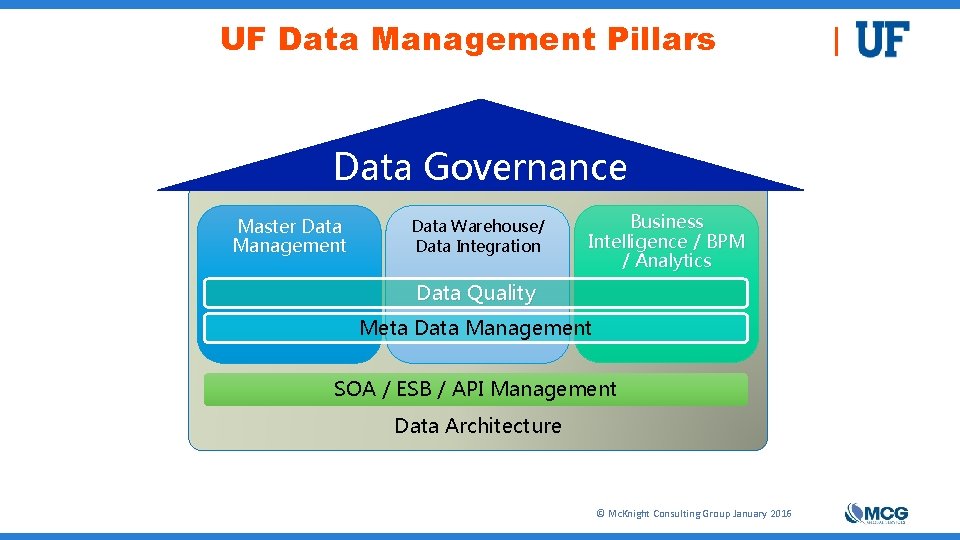
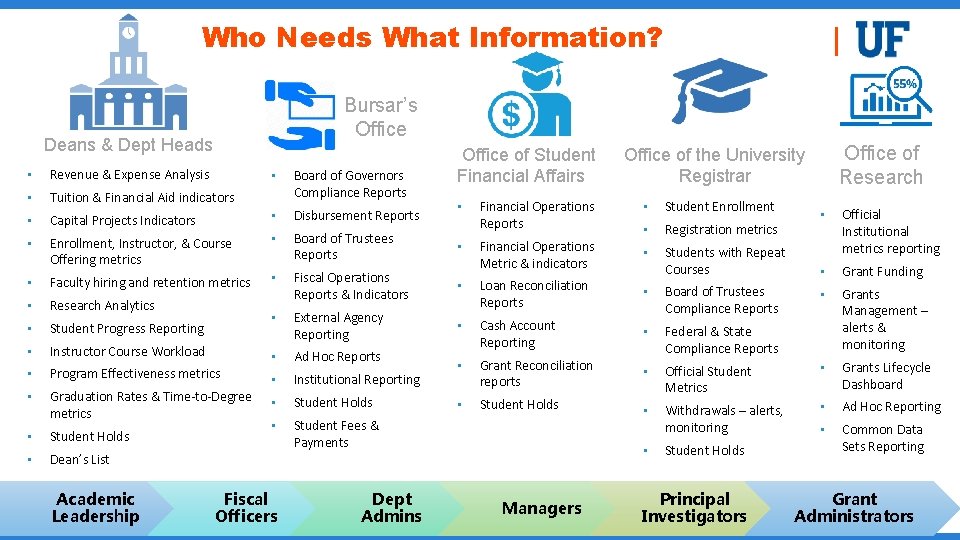
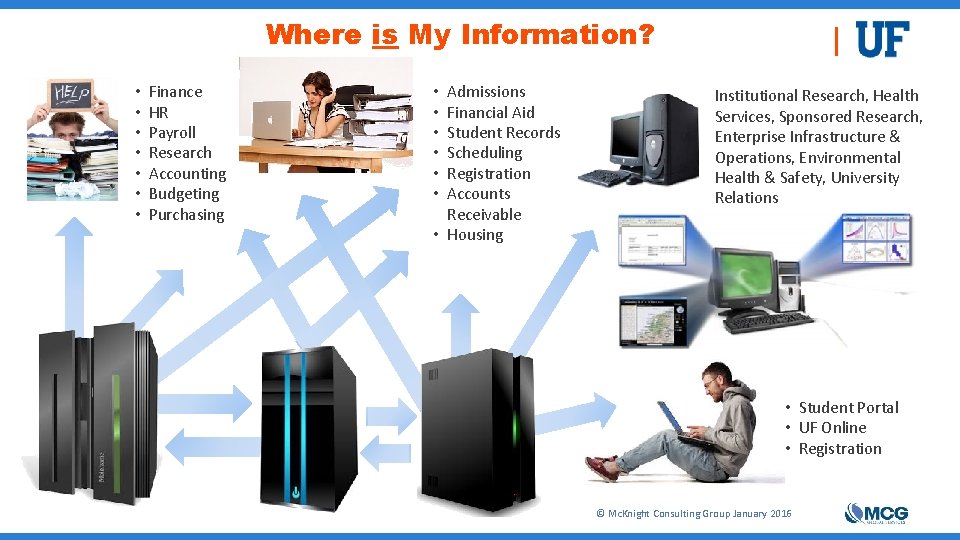
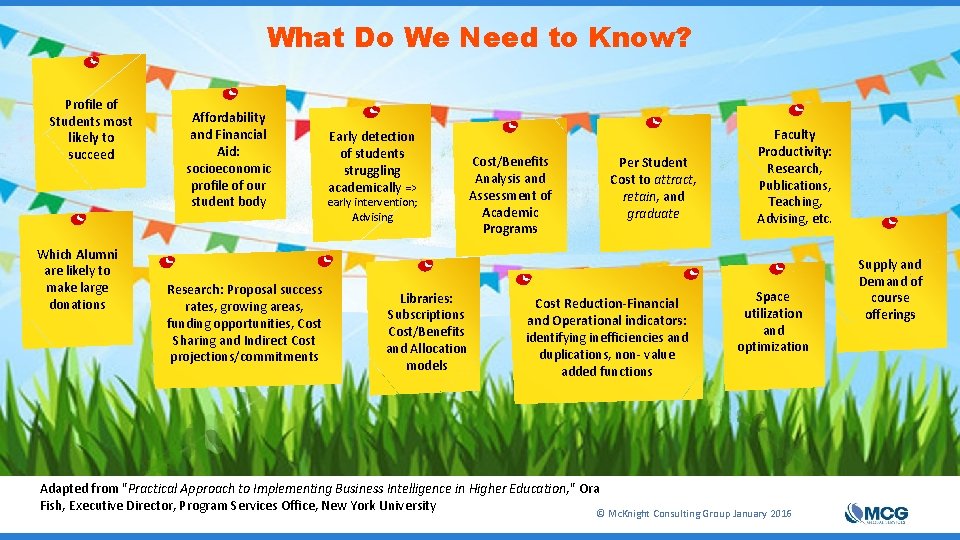
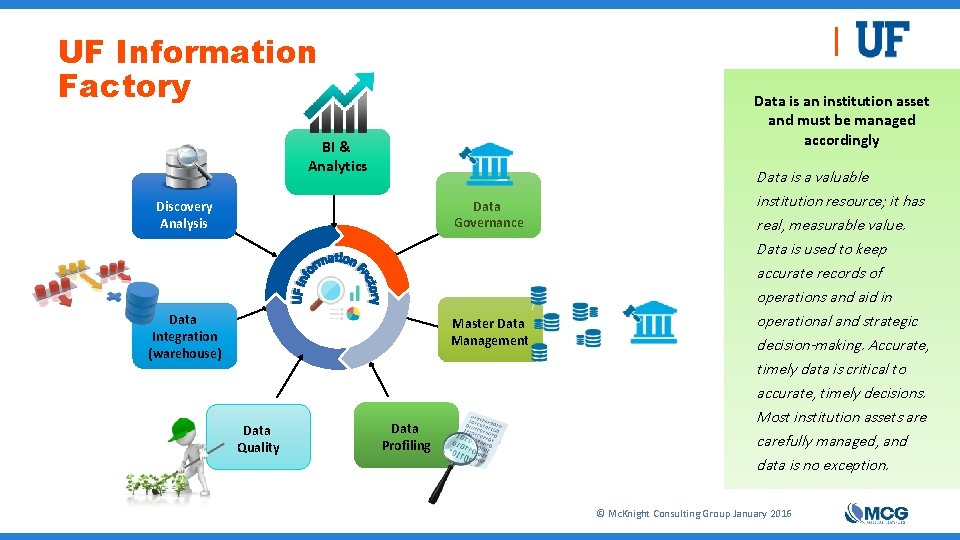
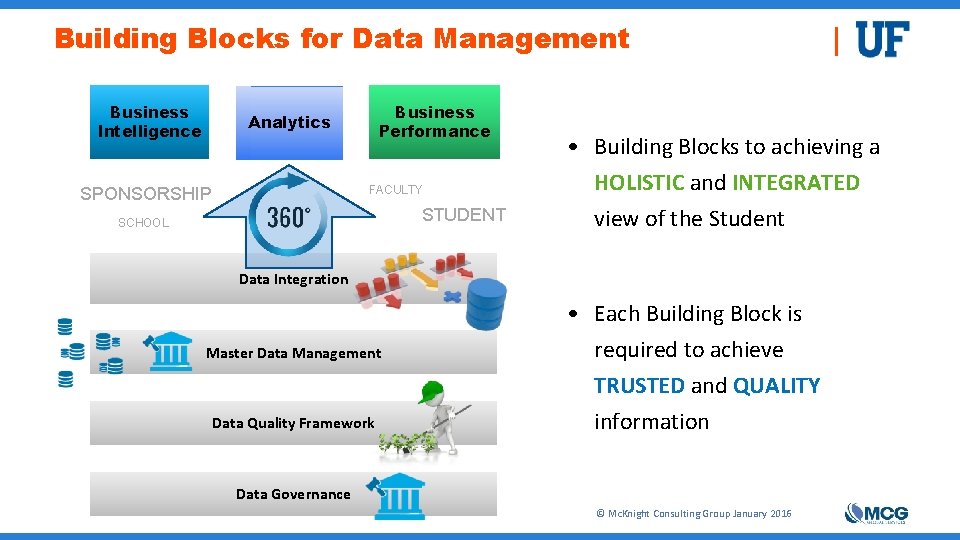
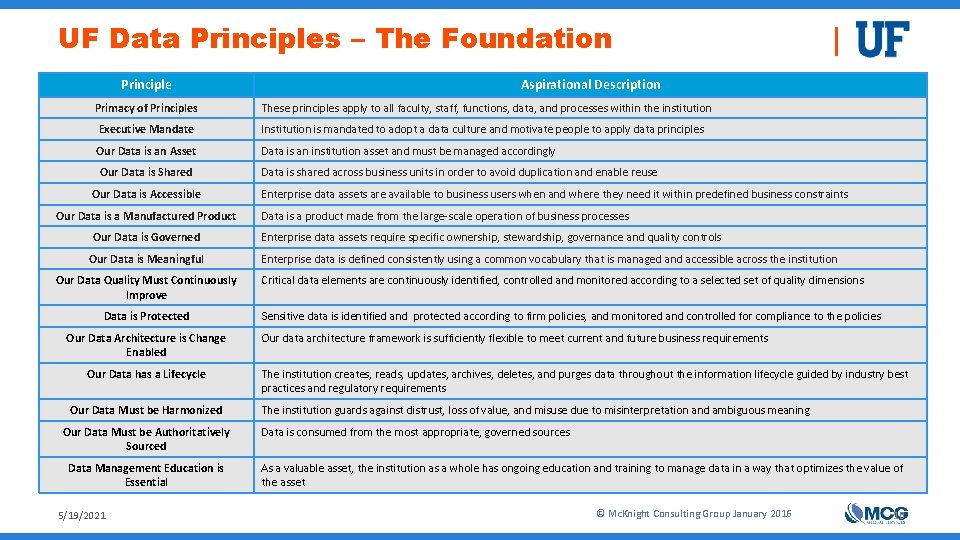
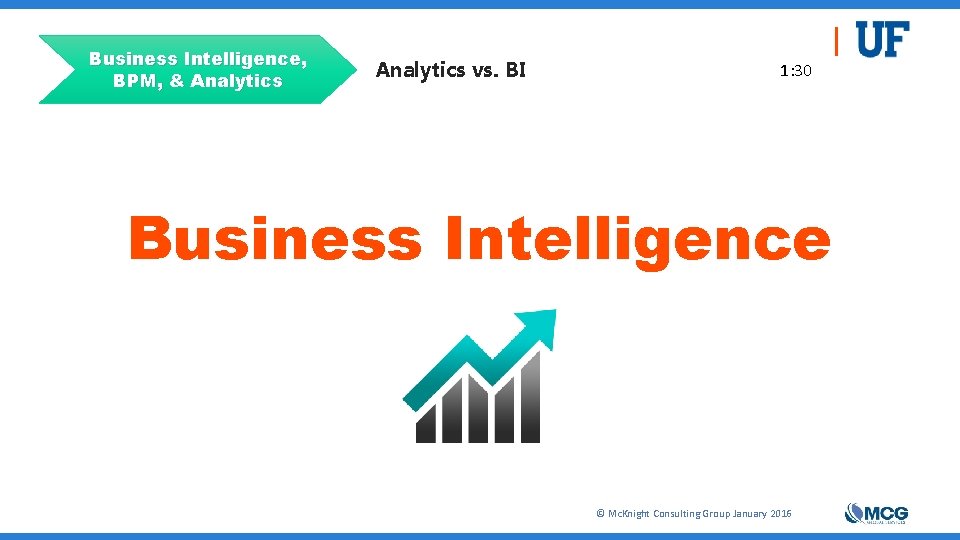
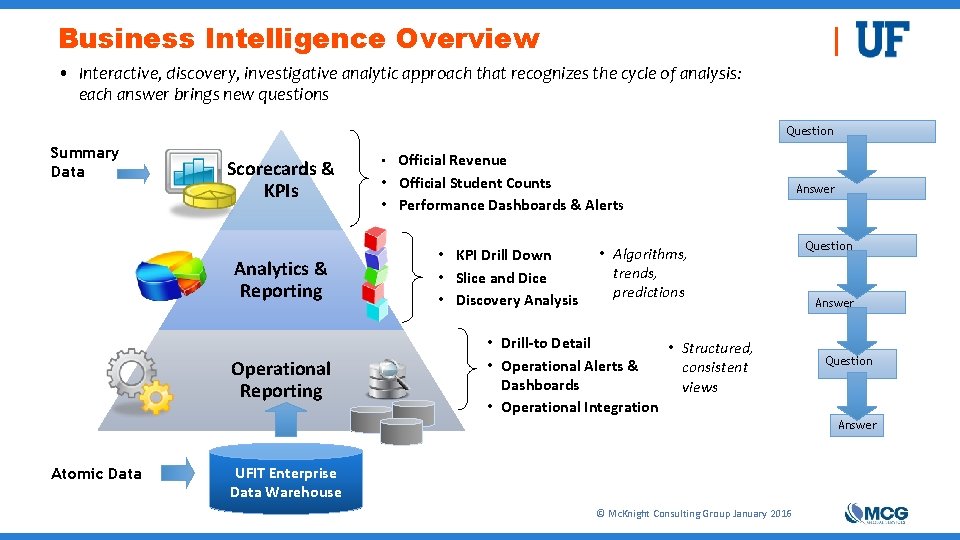
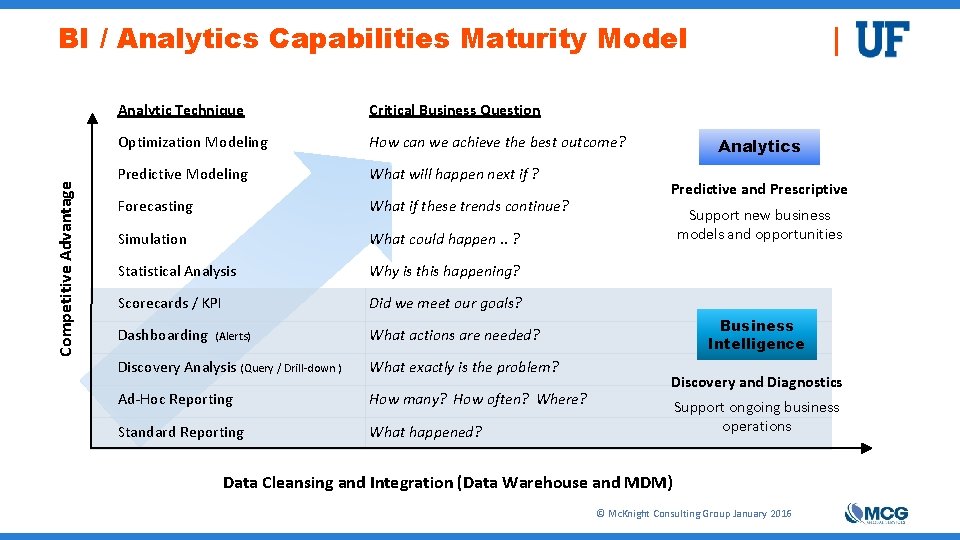
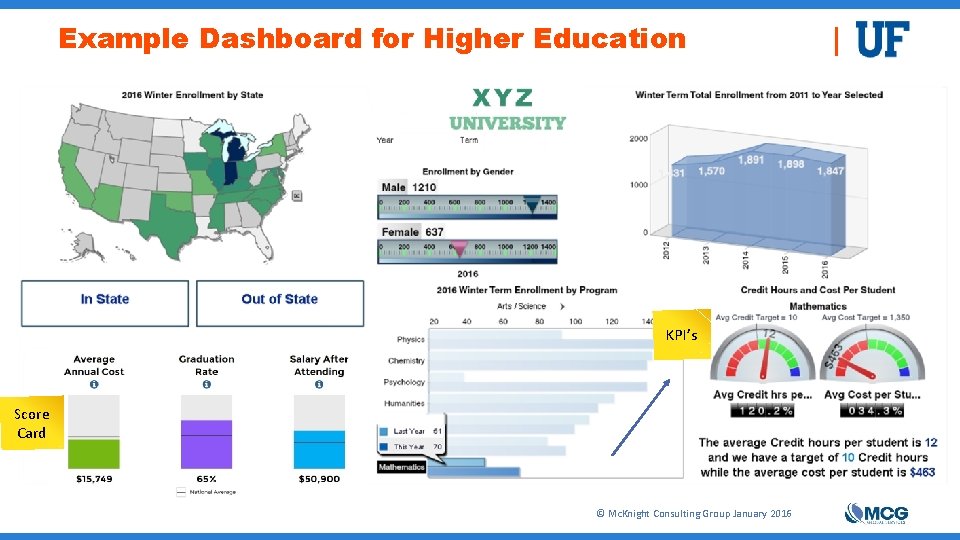
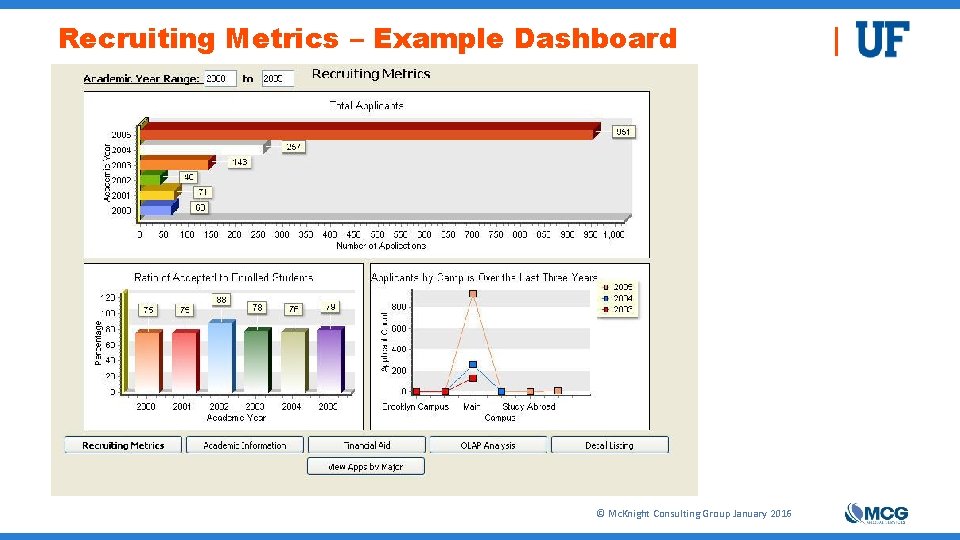
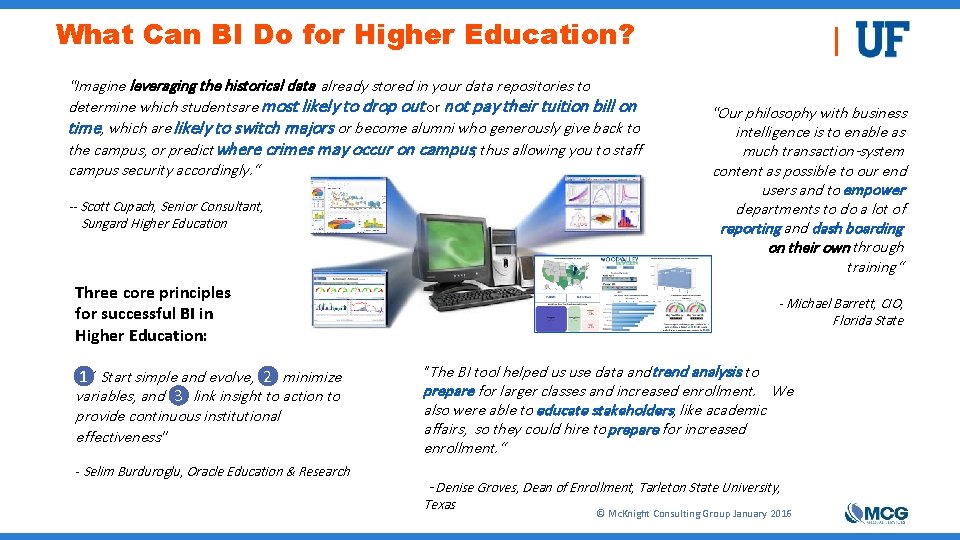
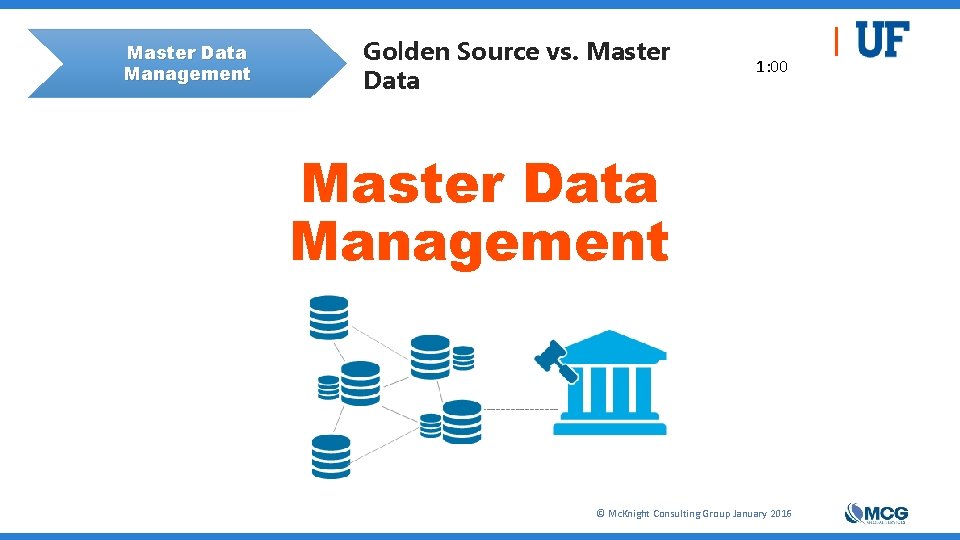
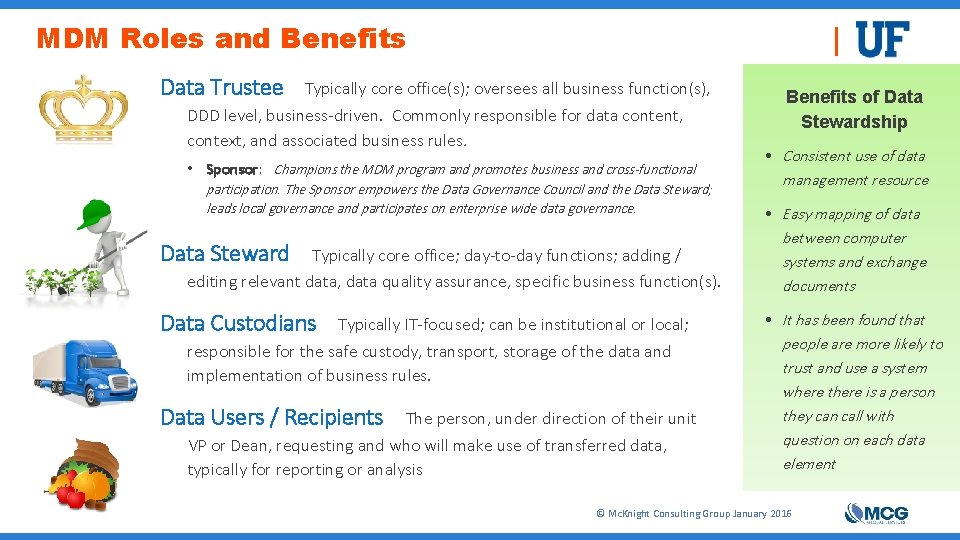
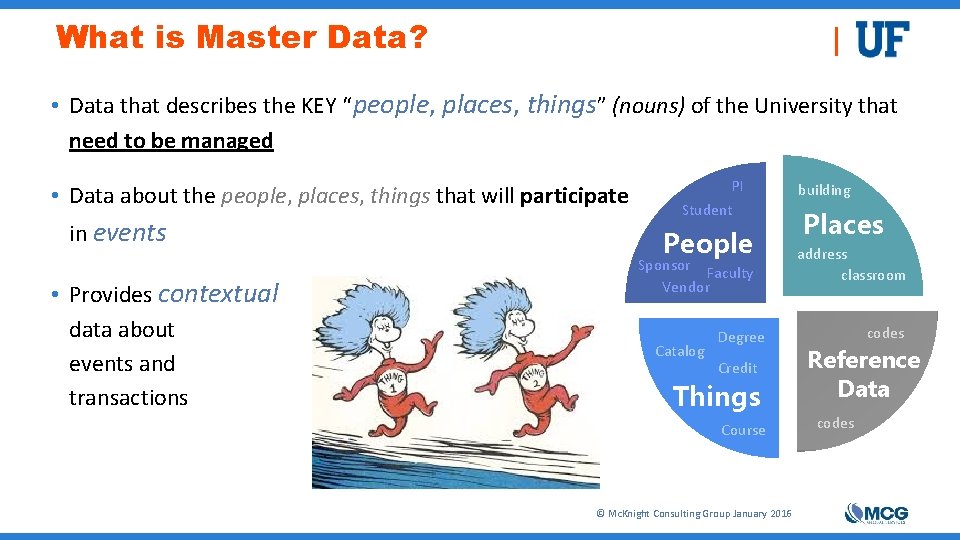
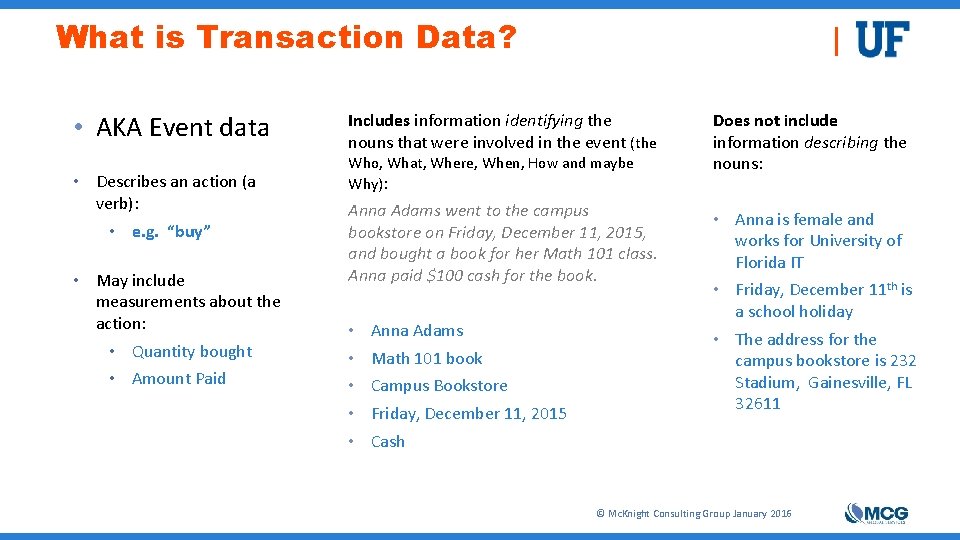
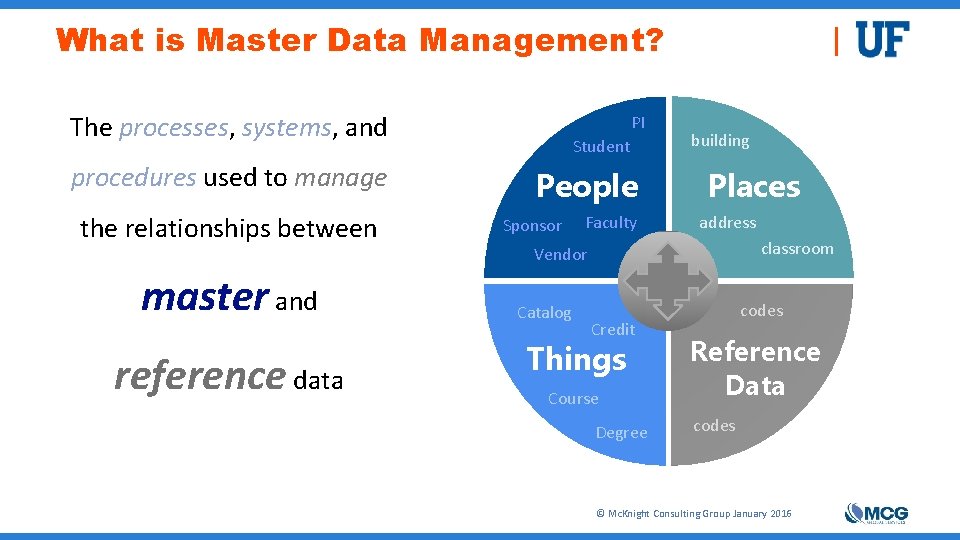
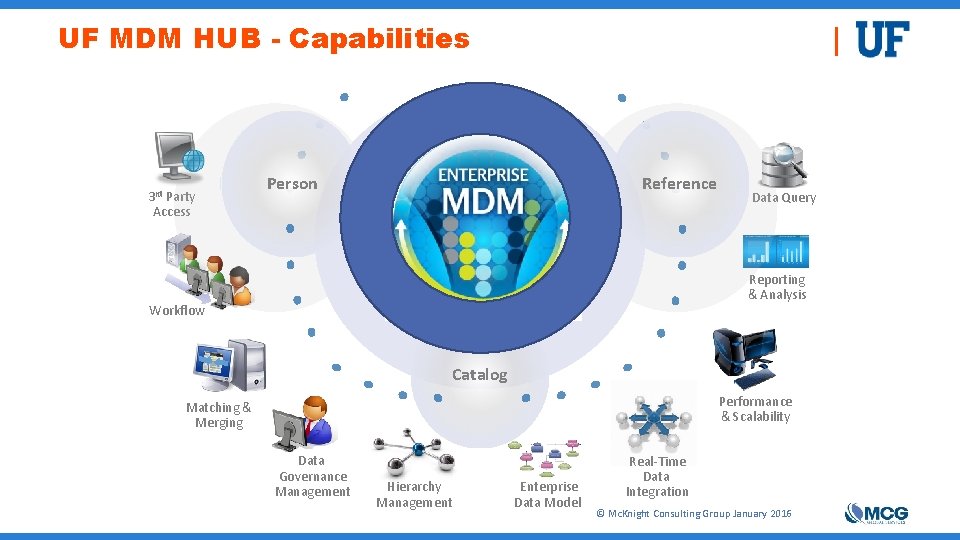
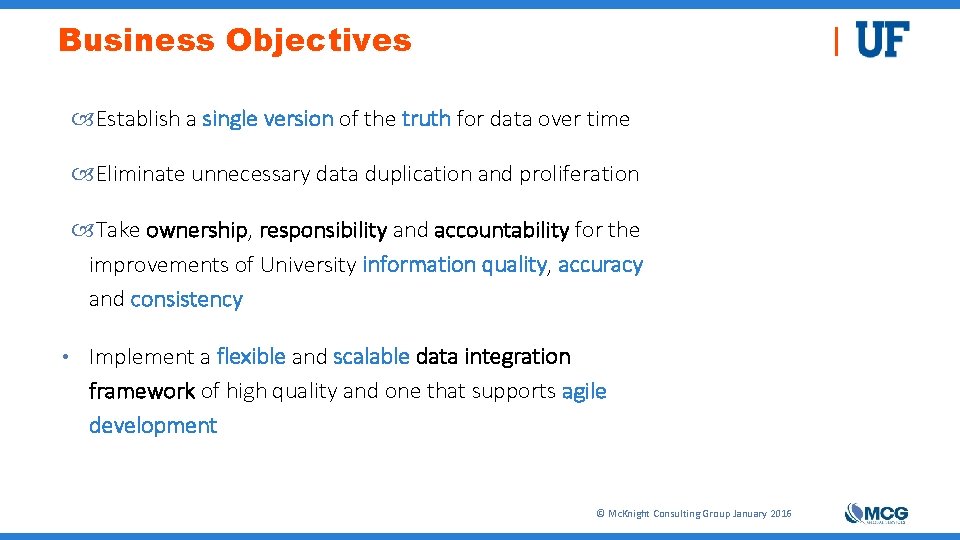
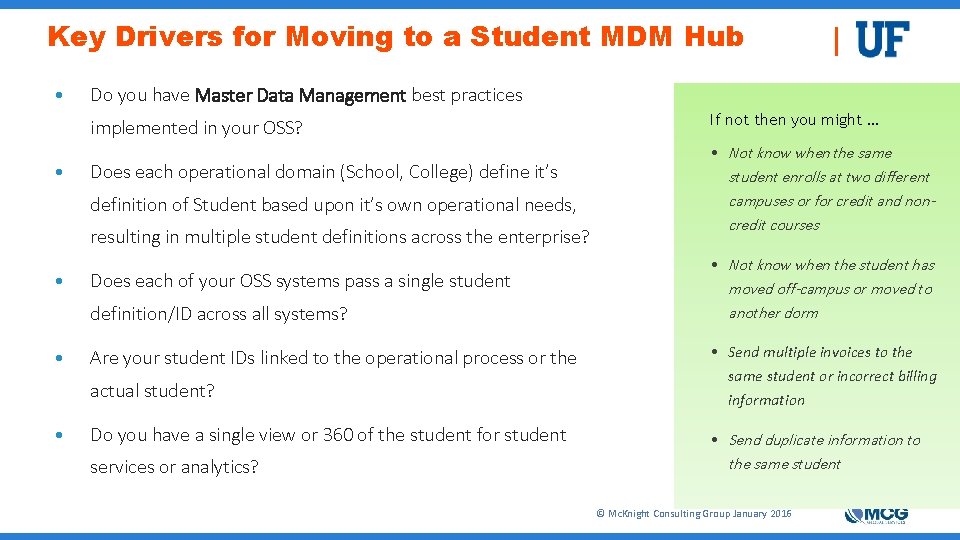
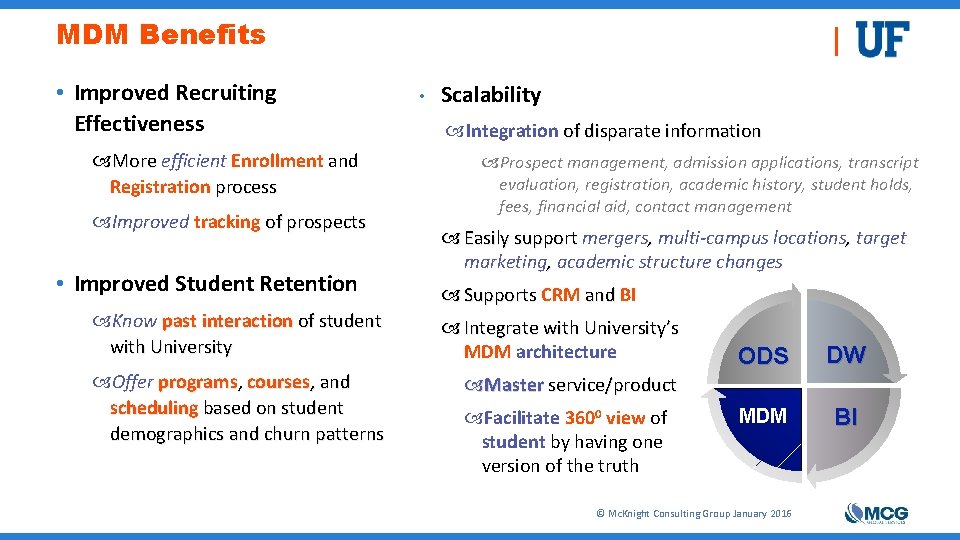
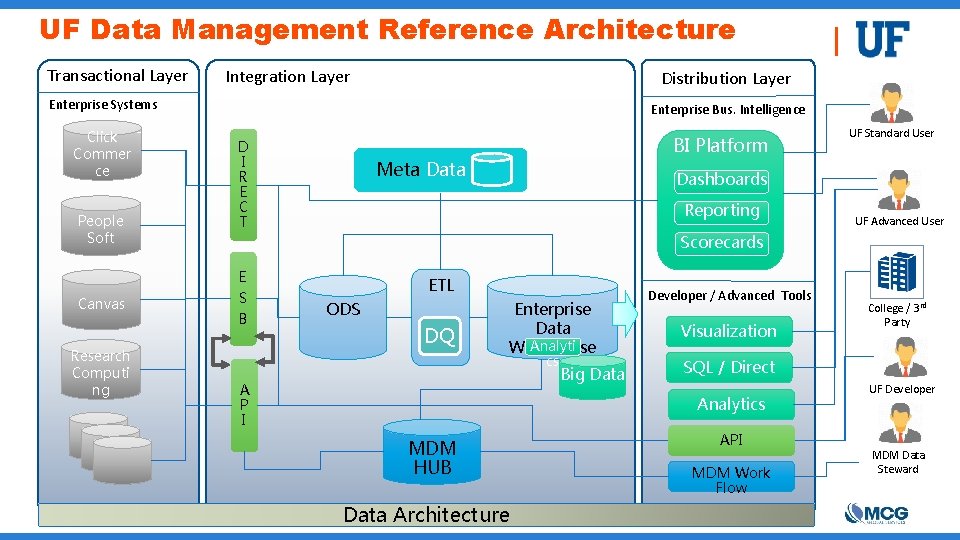
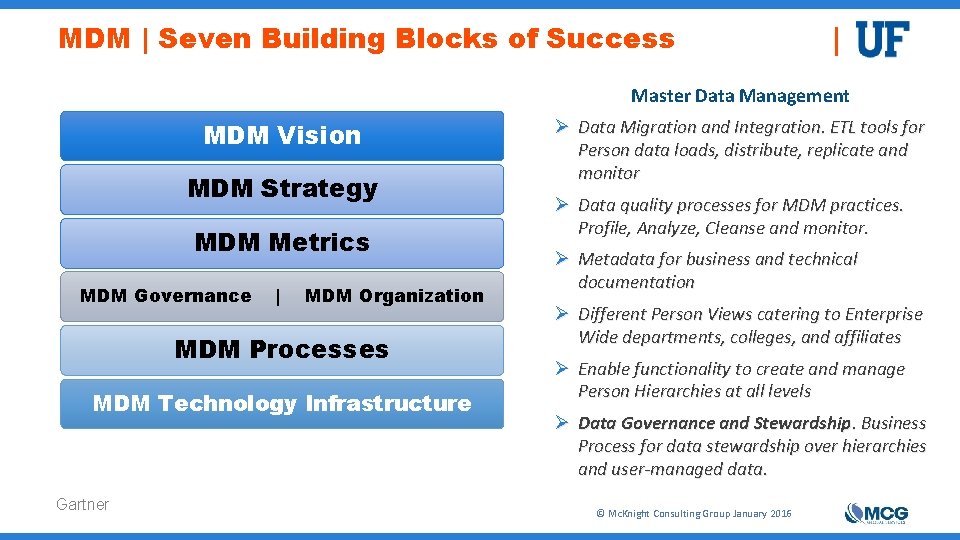
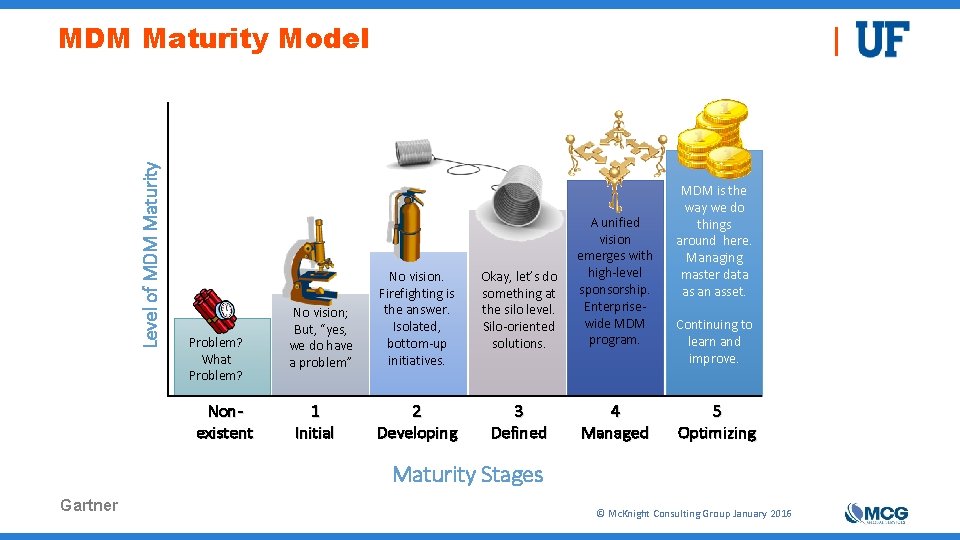
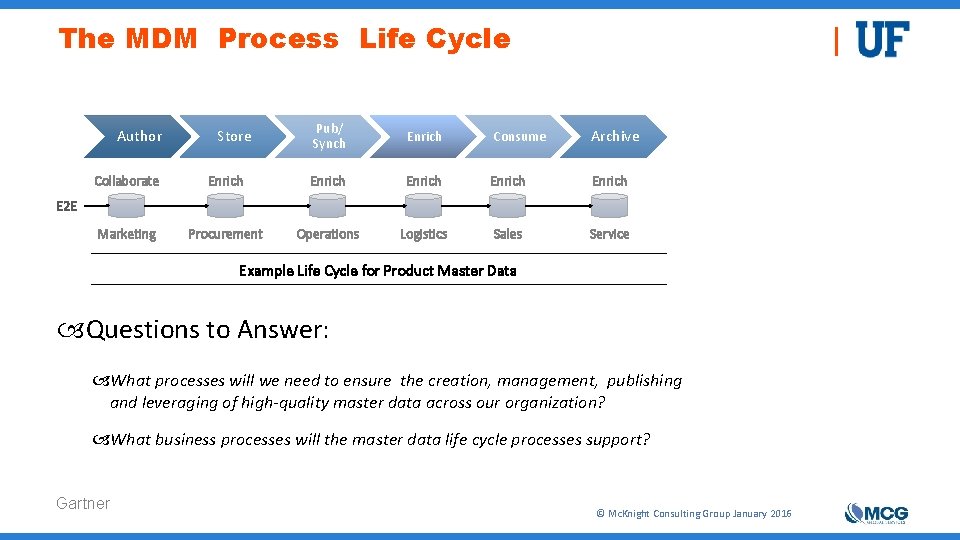
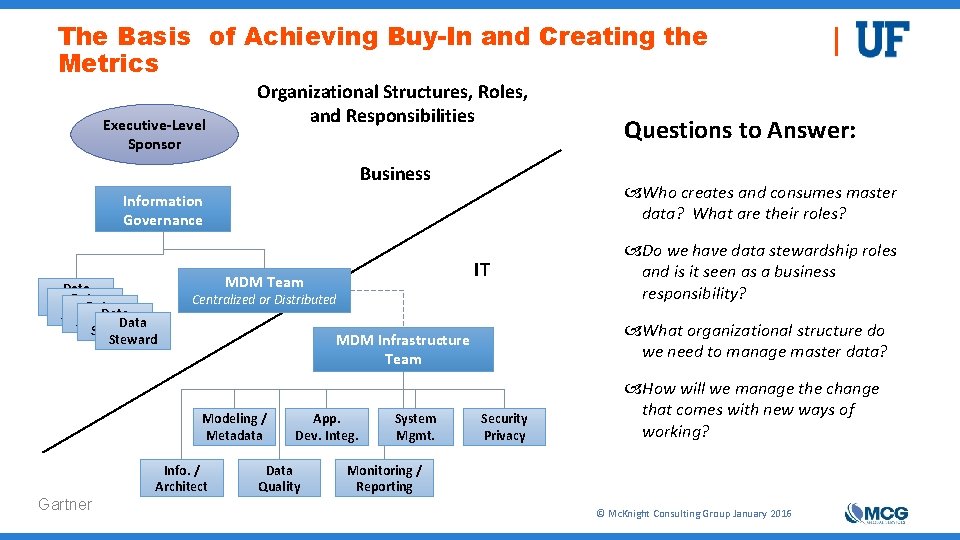
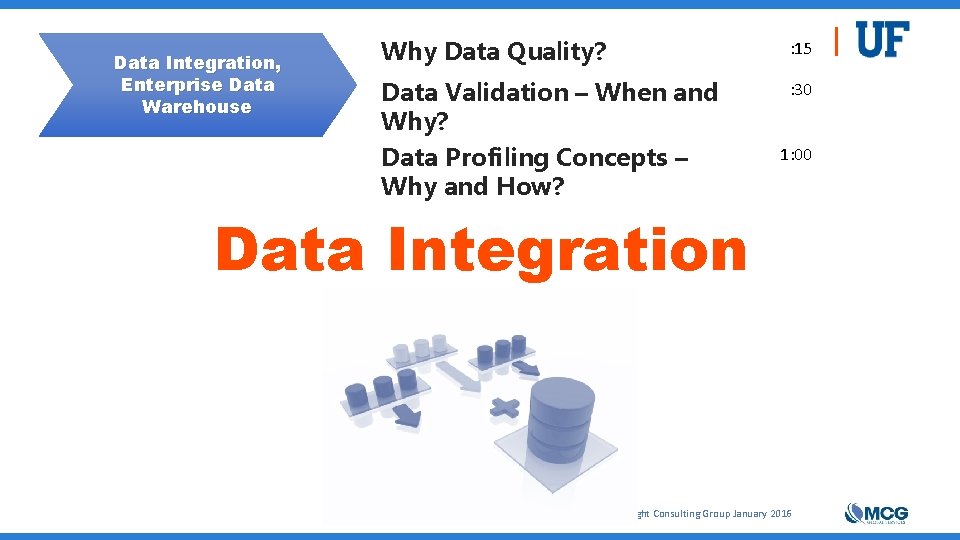
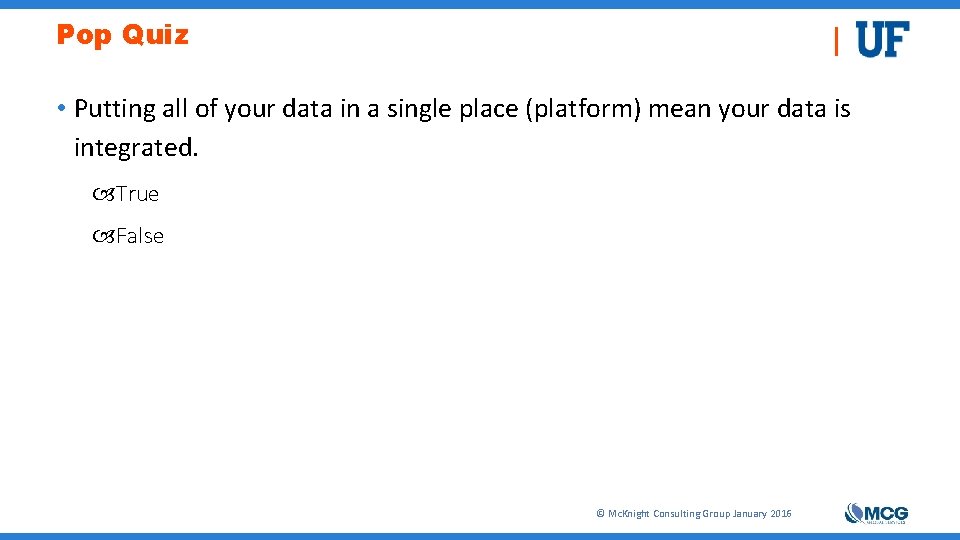
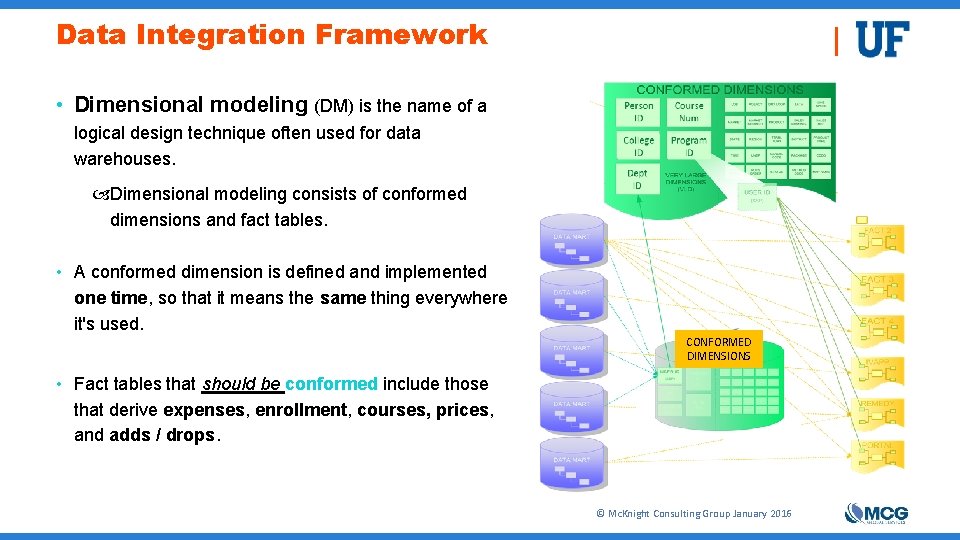
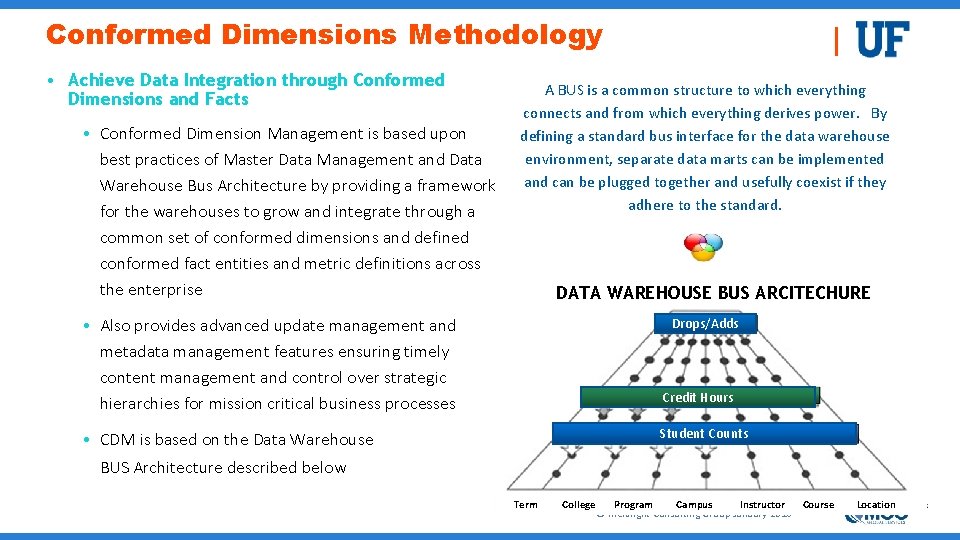
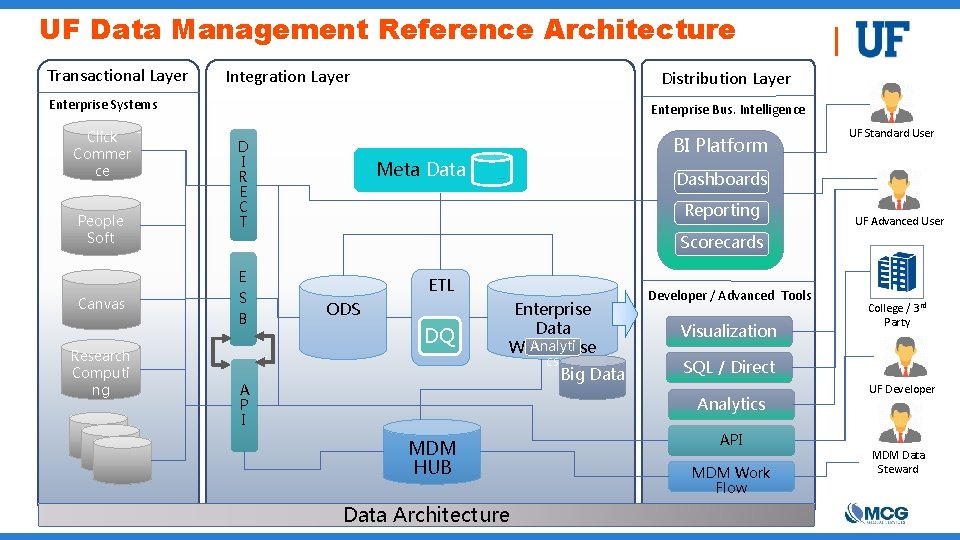
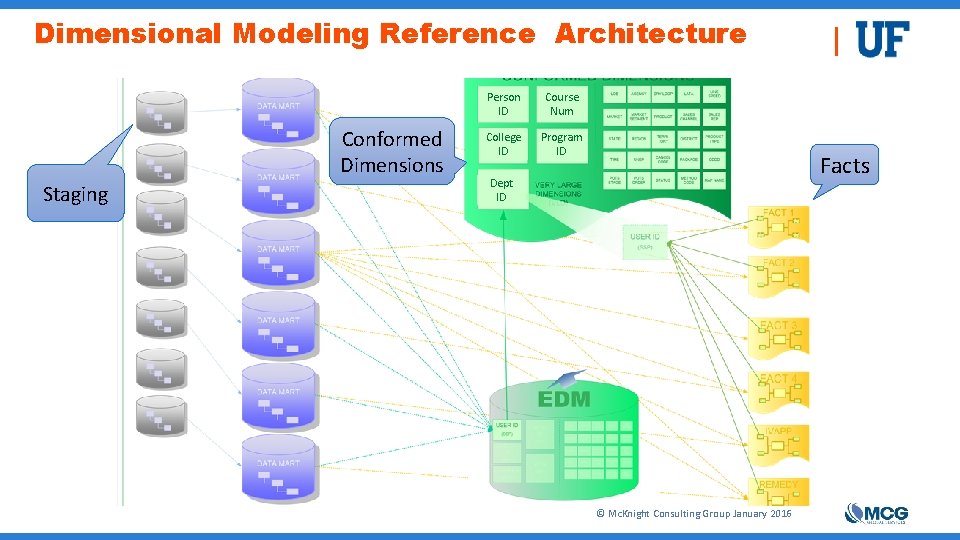
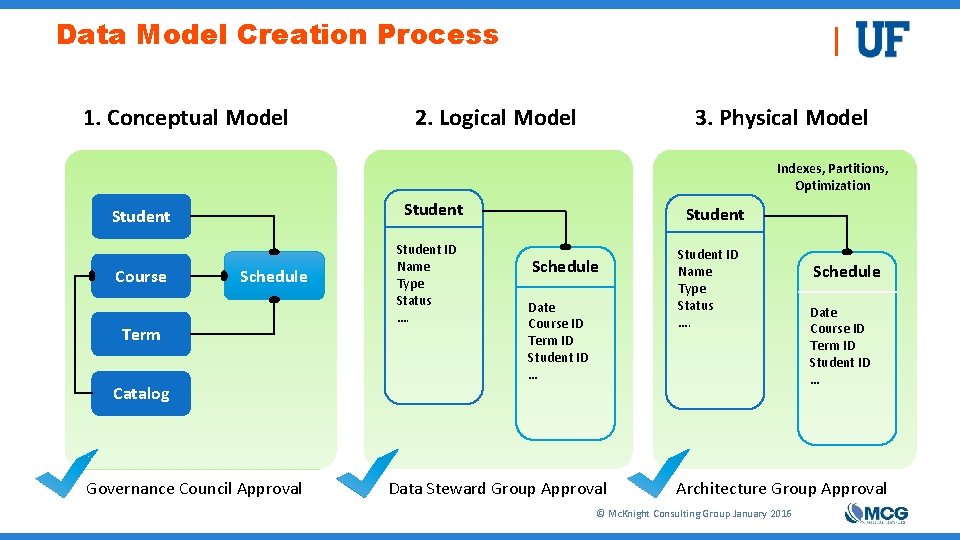
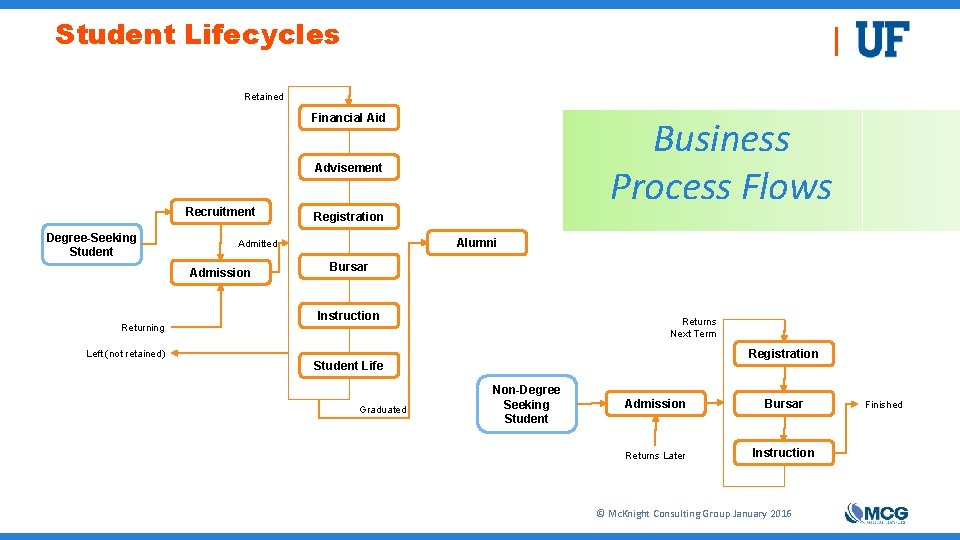
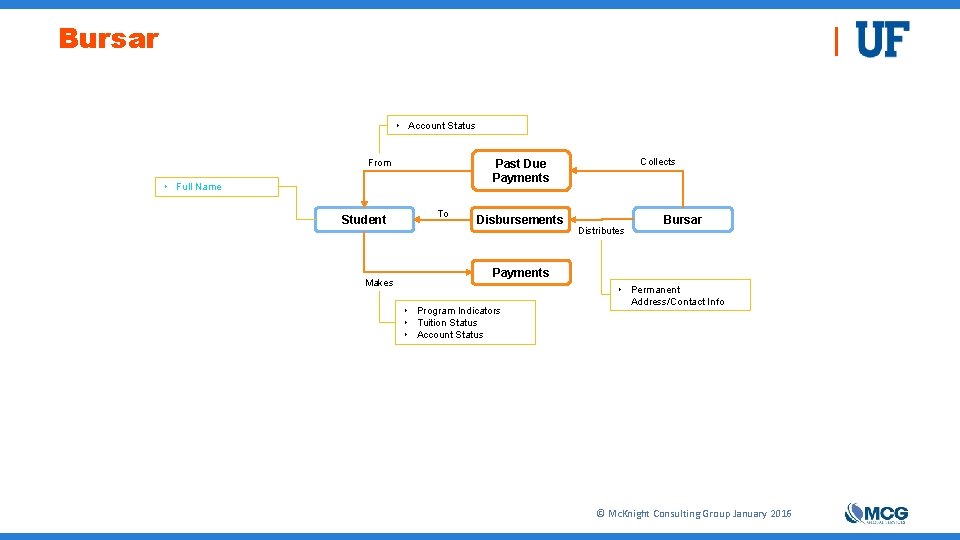
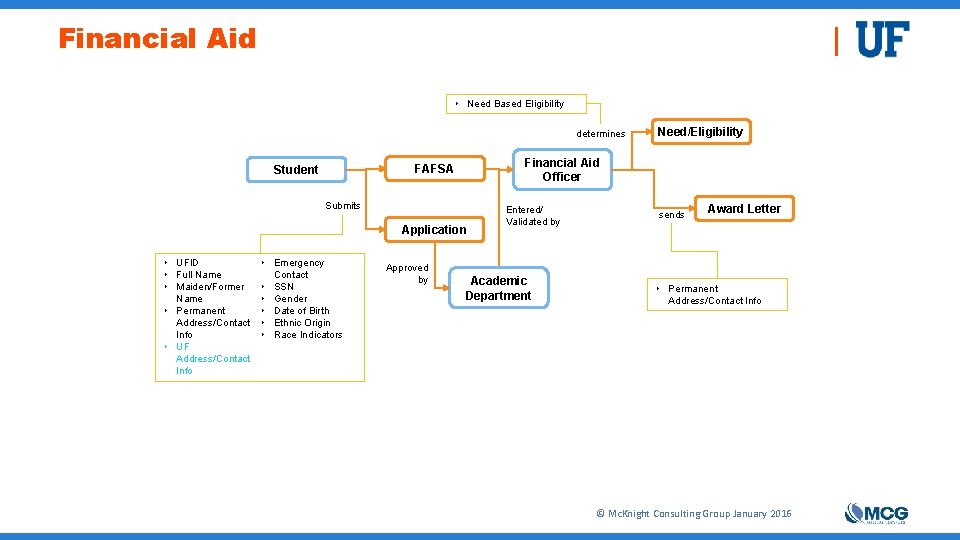
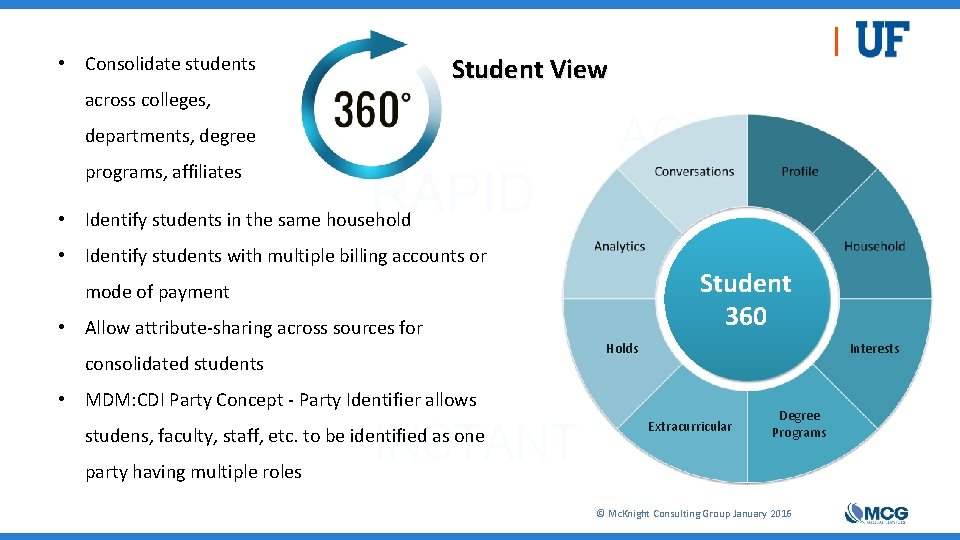
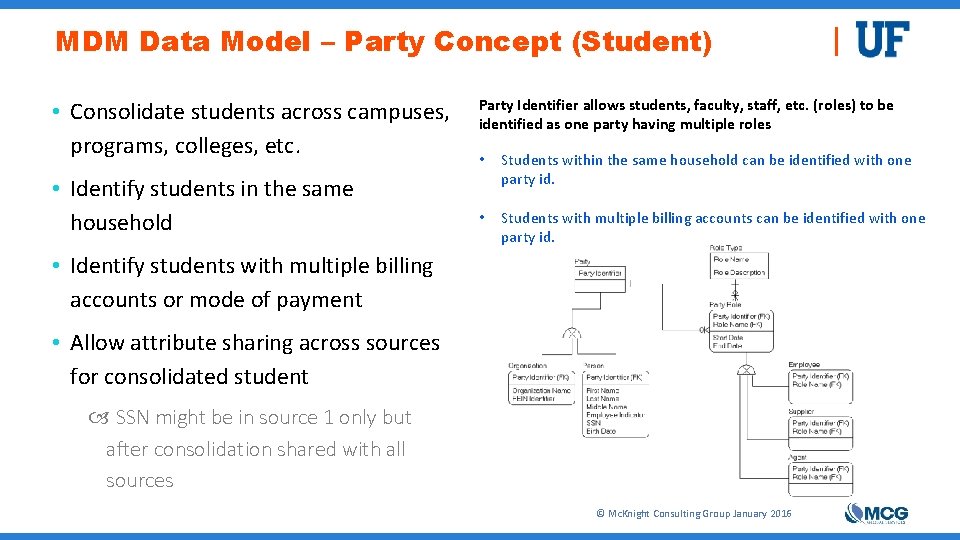
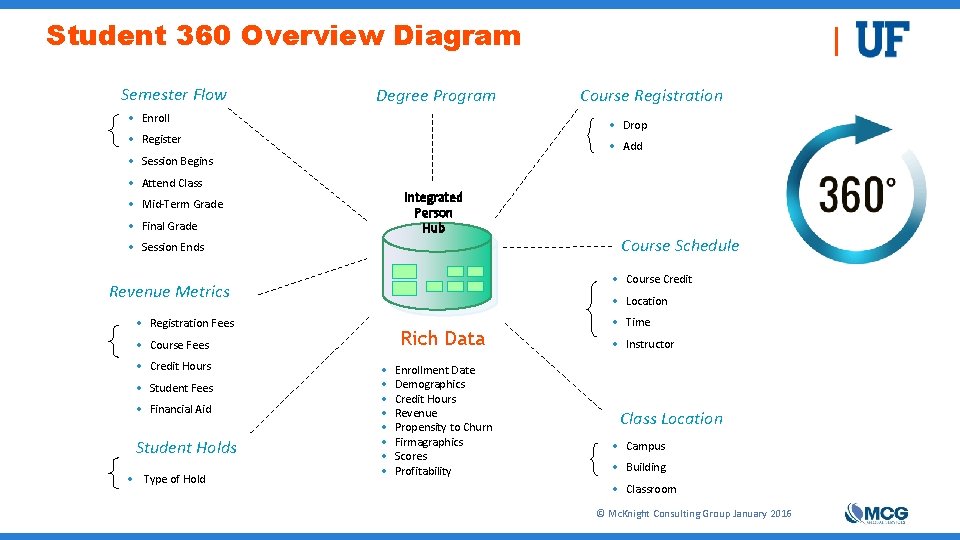
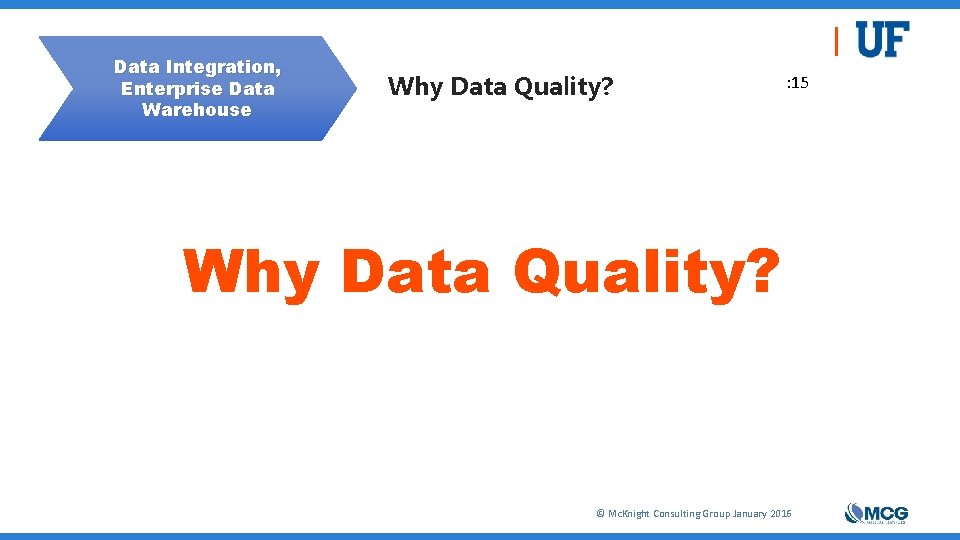
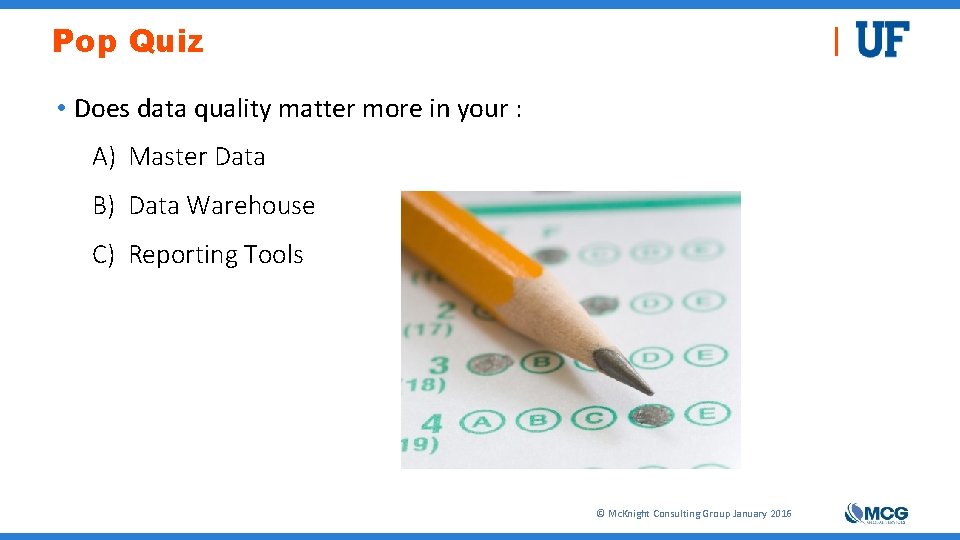
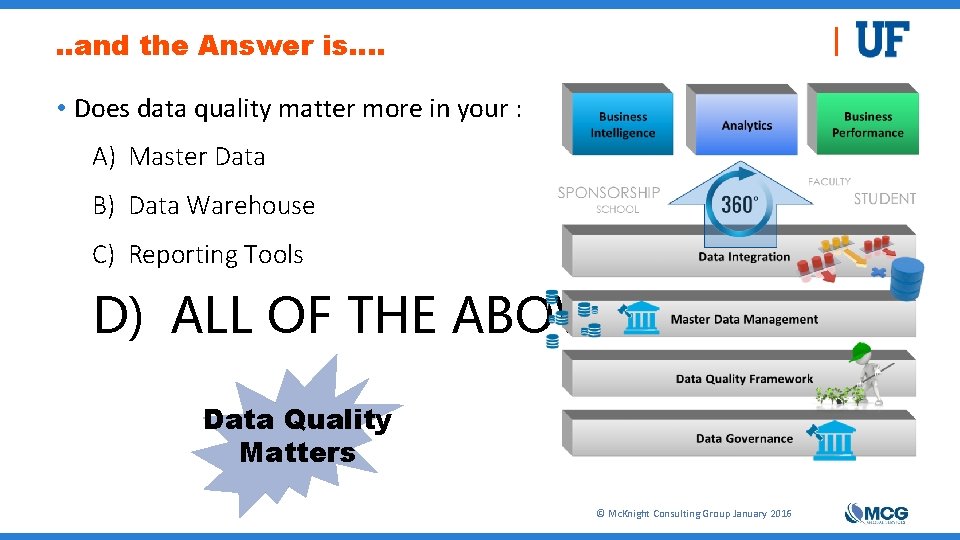
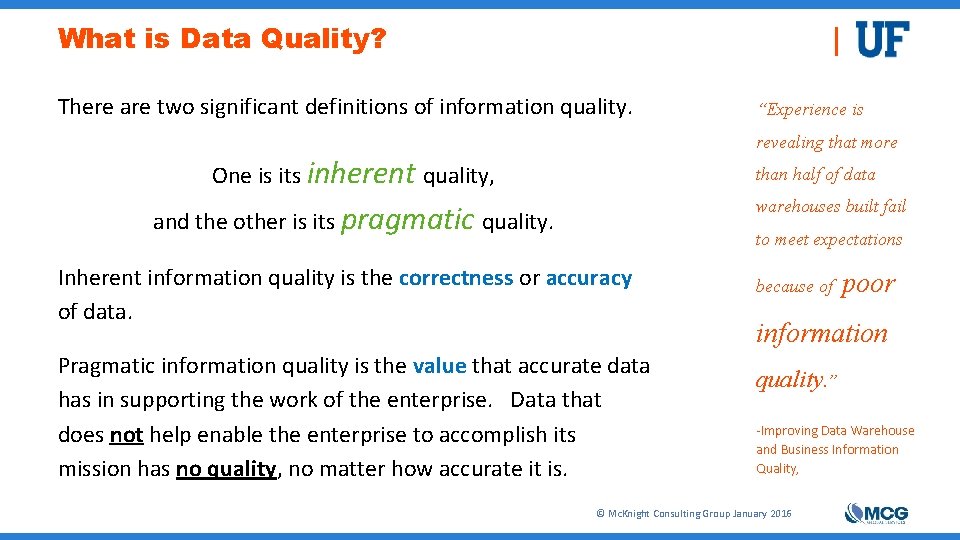
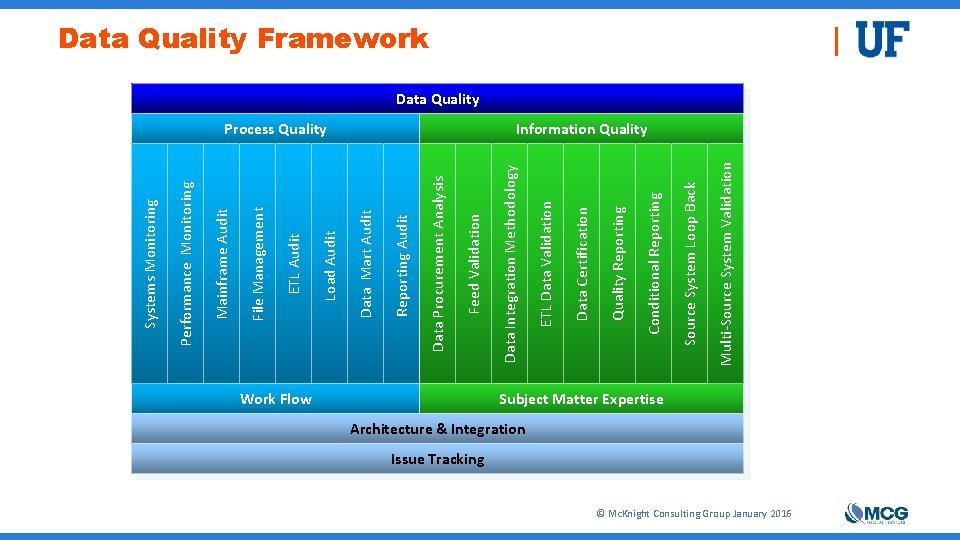
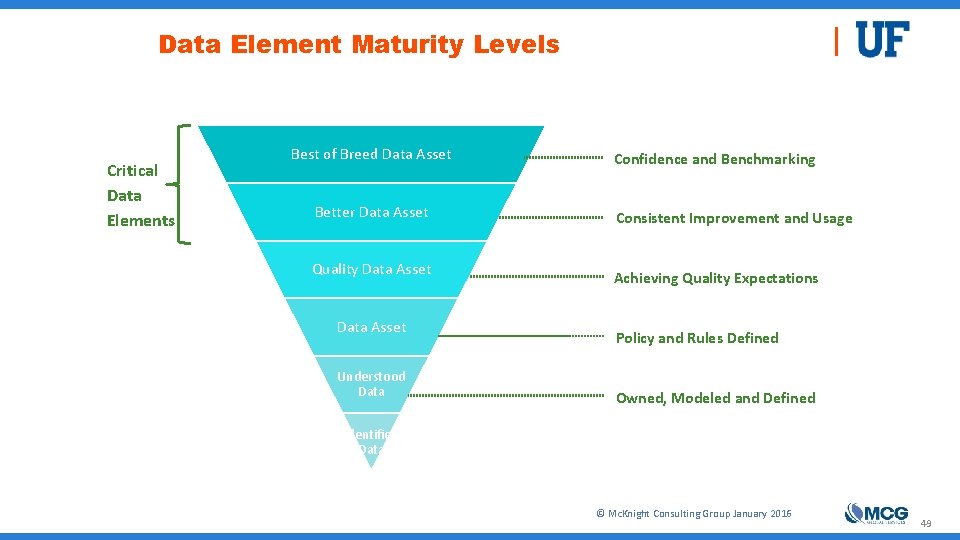
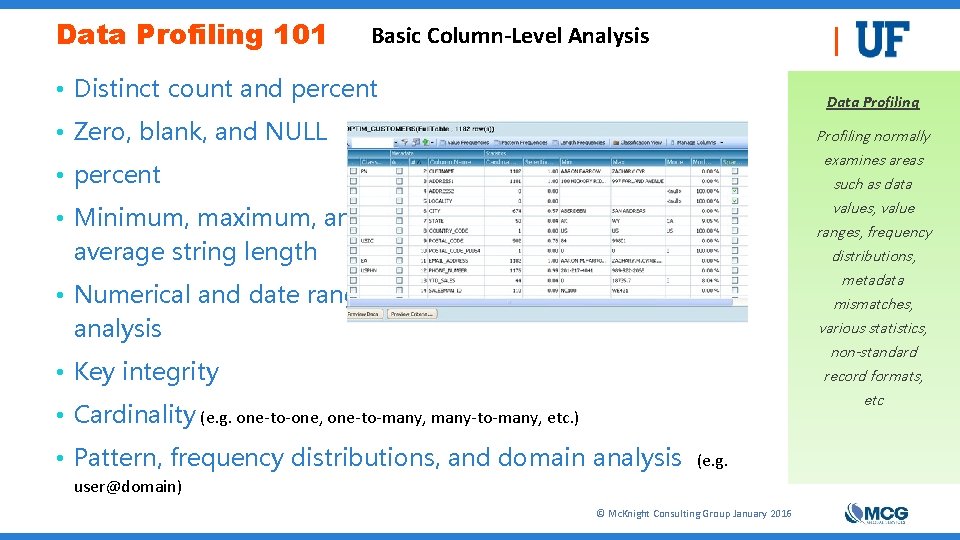
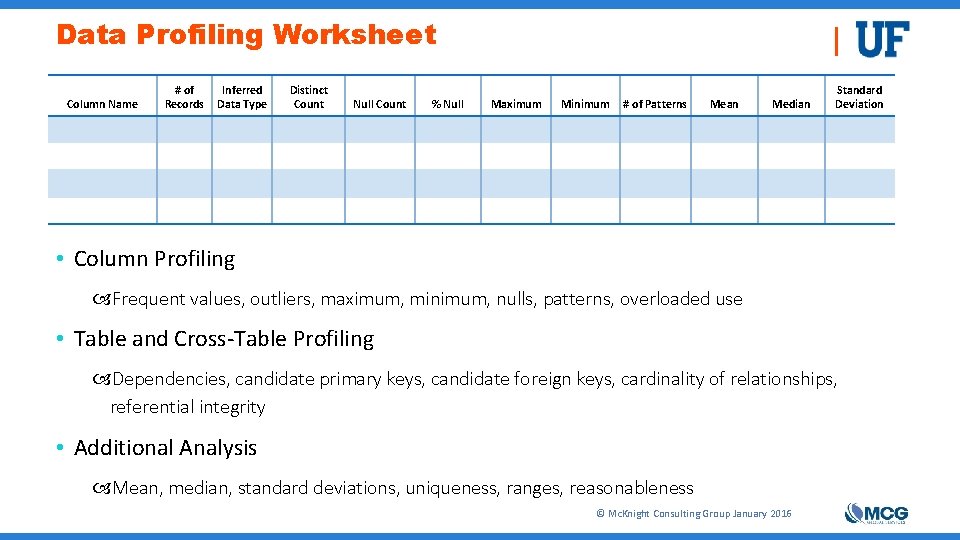
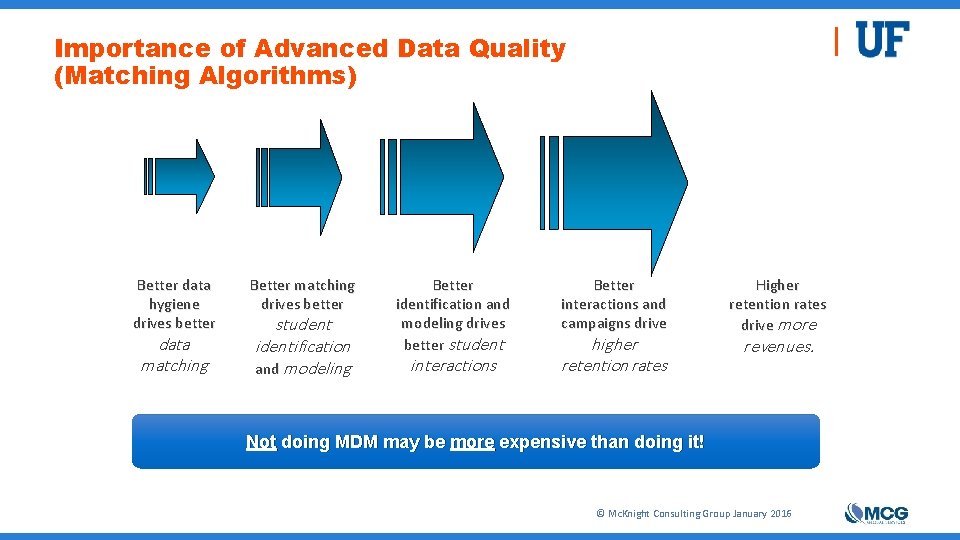
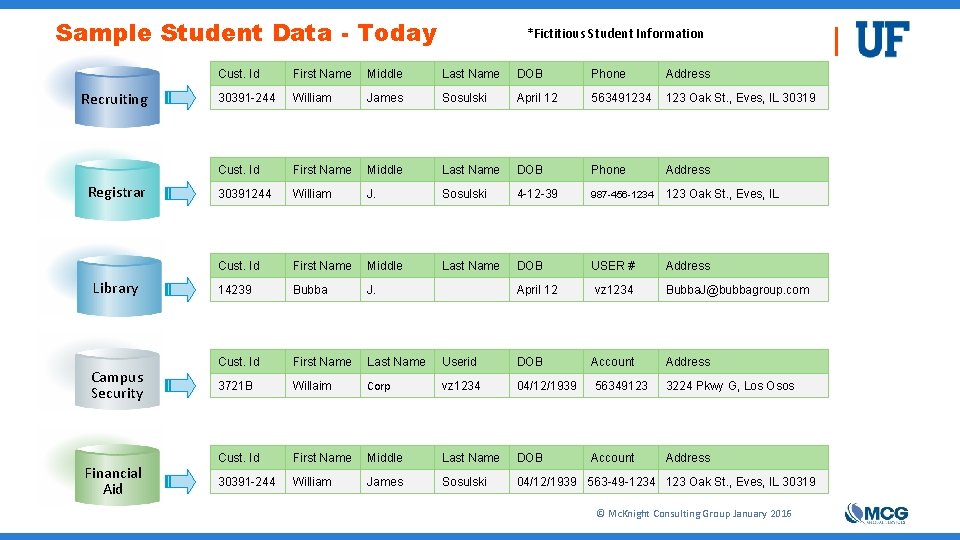
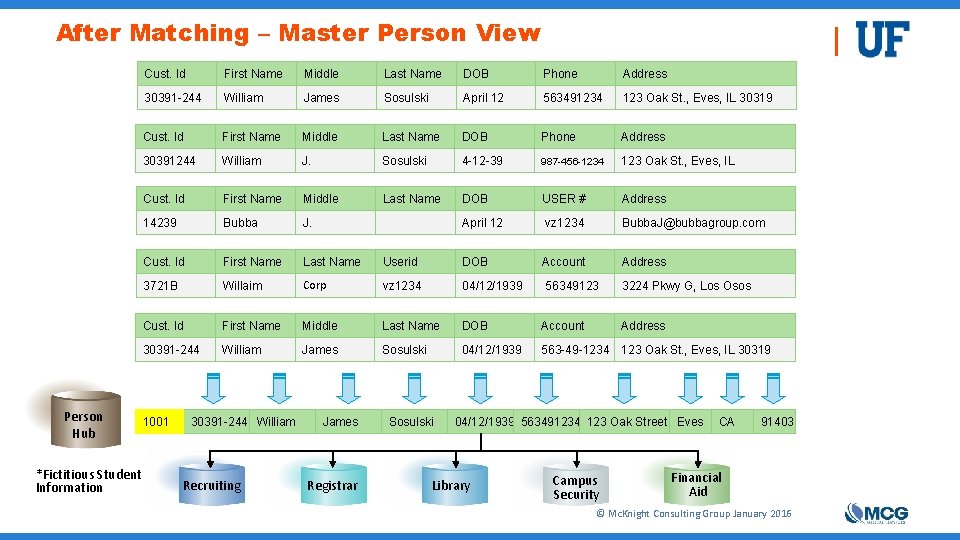
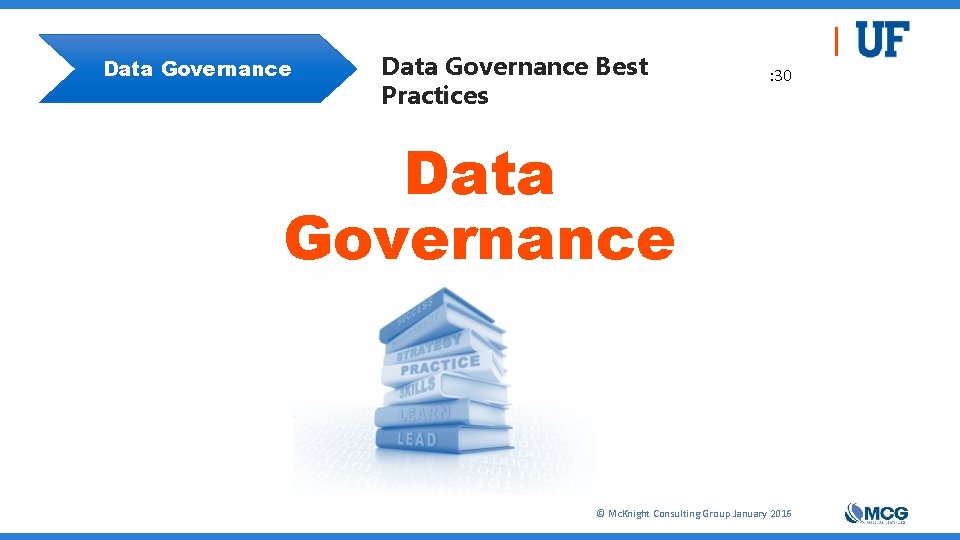
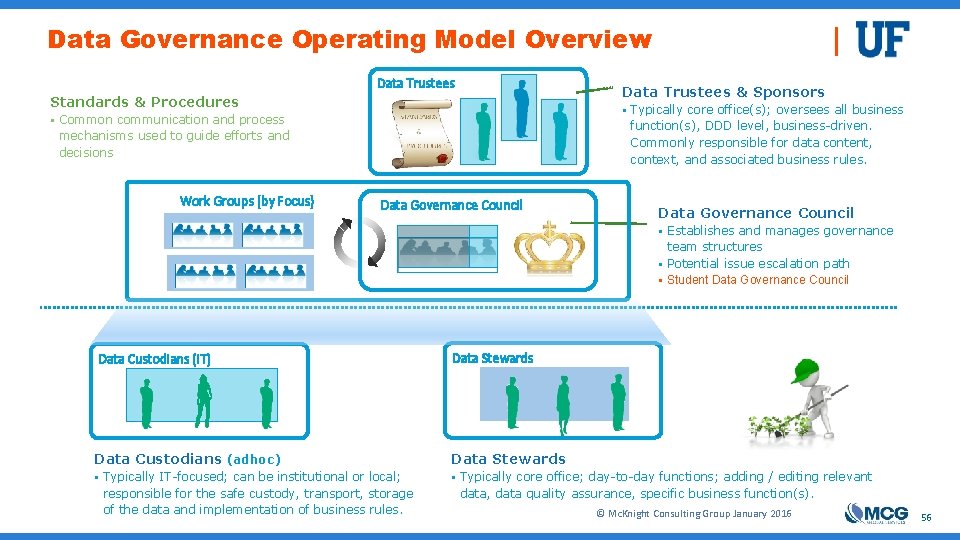
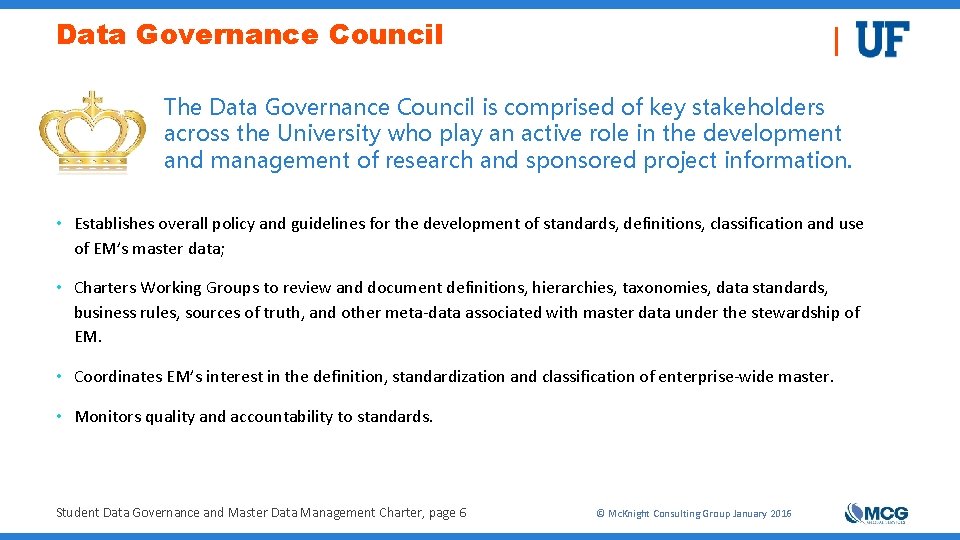
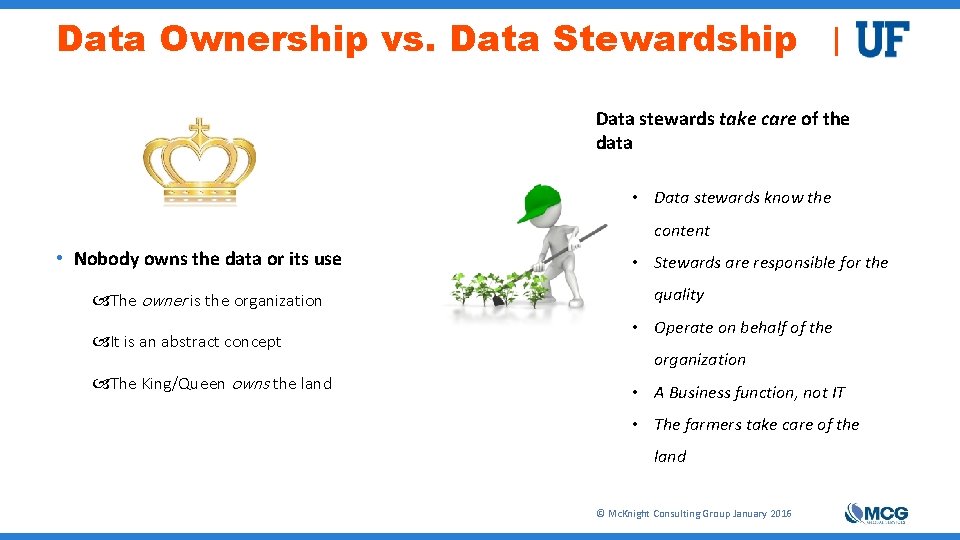
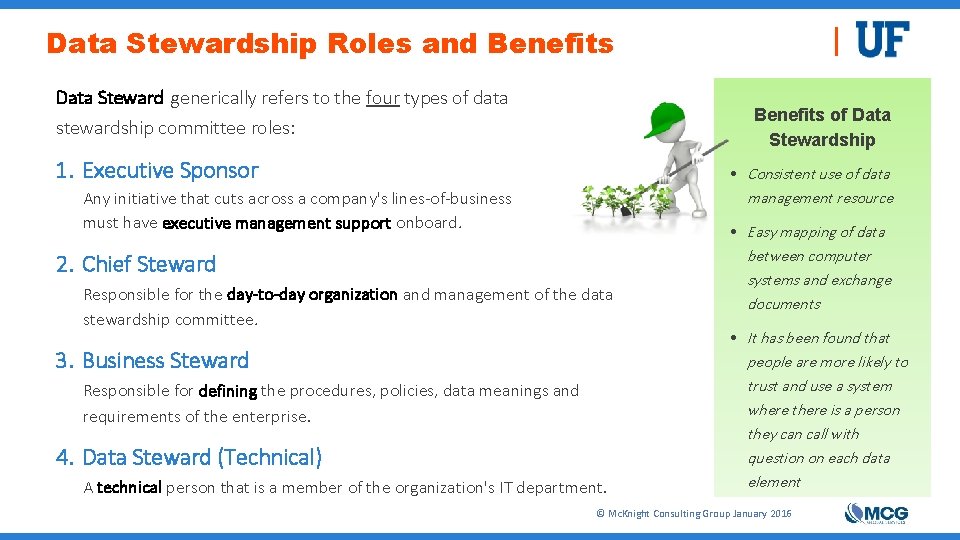
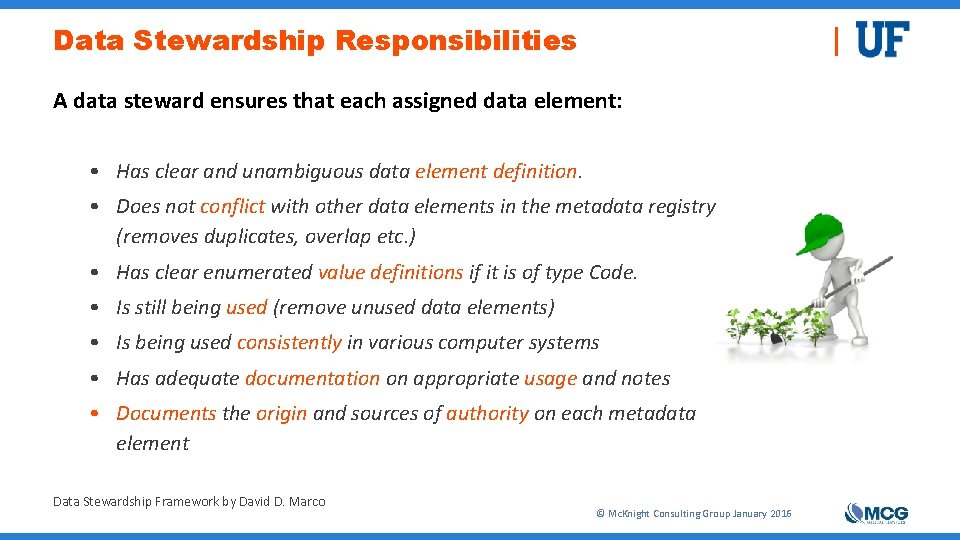
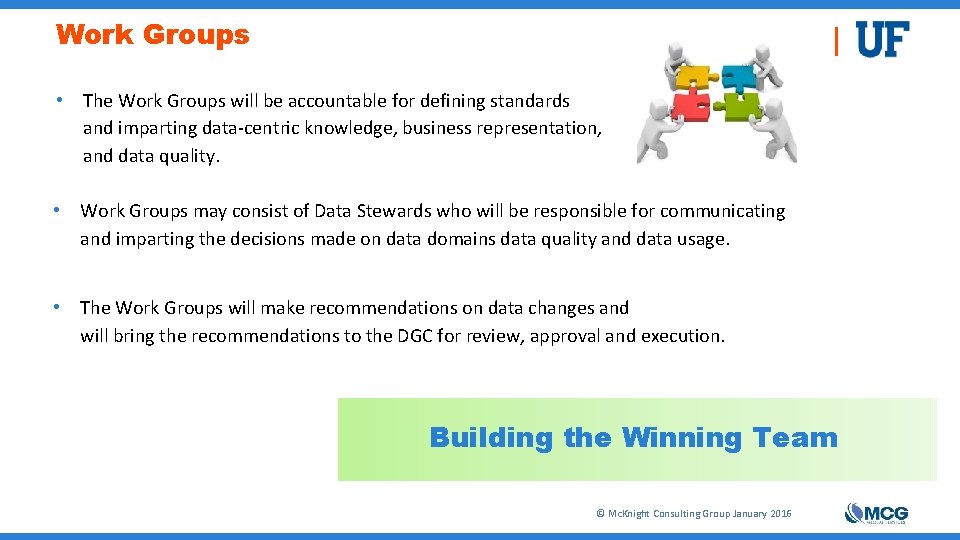
- Slides: 61
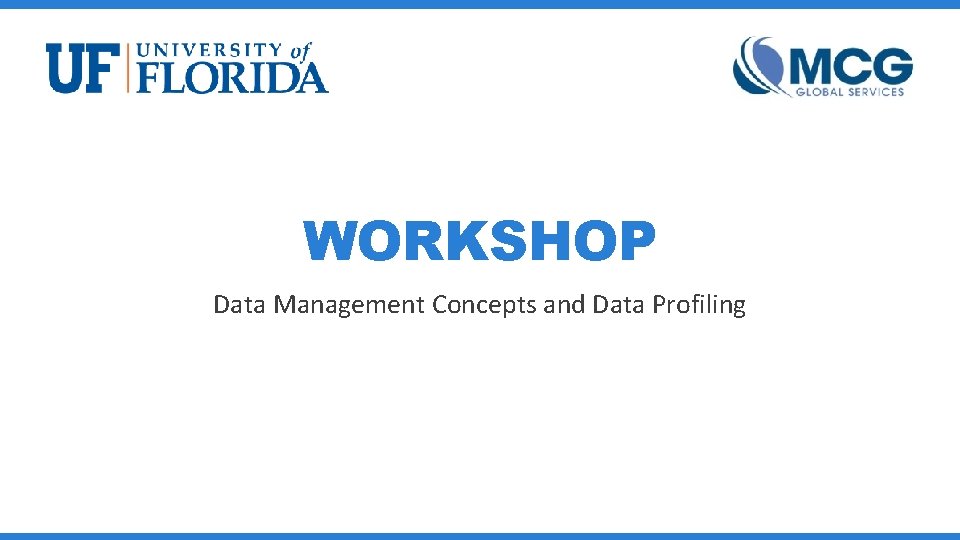
WORKSHOP Data Management Concepts and Data Profiling
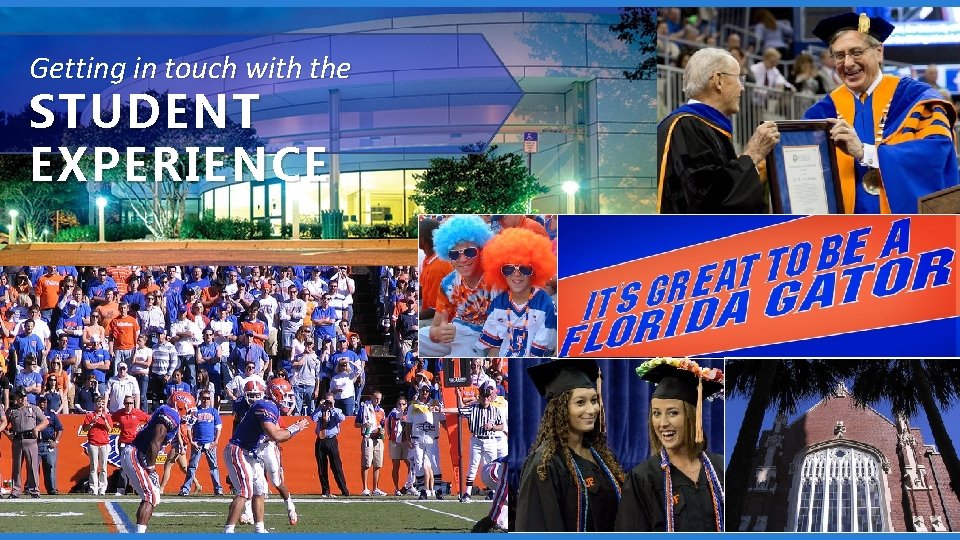
Getting in touch with the STUDENT EXPERIENCE © Mc. Knight Consulting Group January 2016
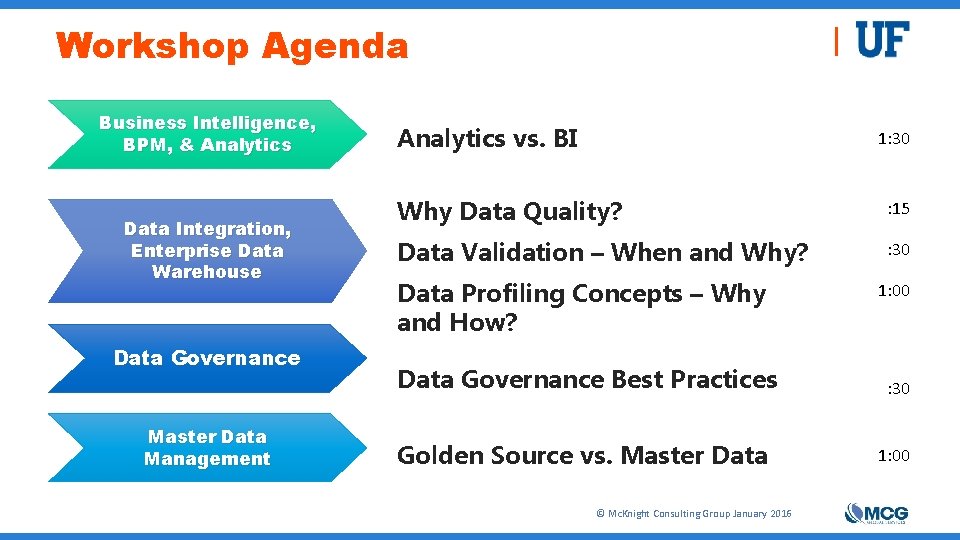
Workshop Agenda Business Intelligence, BPM, & Analytics Data Integration, Enterprise Data Warehouse Data Governance Master Data Management Analytics vs. BI 1: 30 Why Data Quality? : 15 Data Validation – When and Why? : 30 Data Profiling Concepts – Why and How? 1: 00 Data Governance Best Practices : 30 Golden Source vs. Master Data 1: 00 © Mc. Knight Consulting Group January 2016
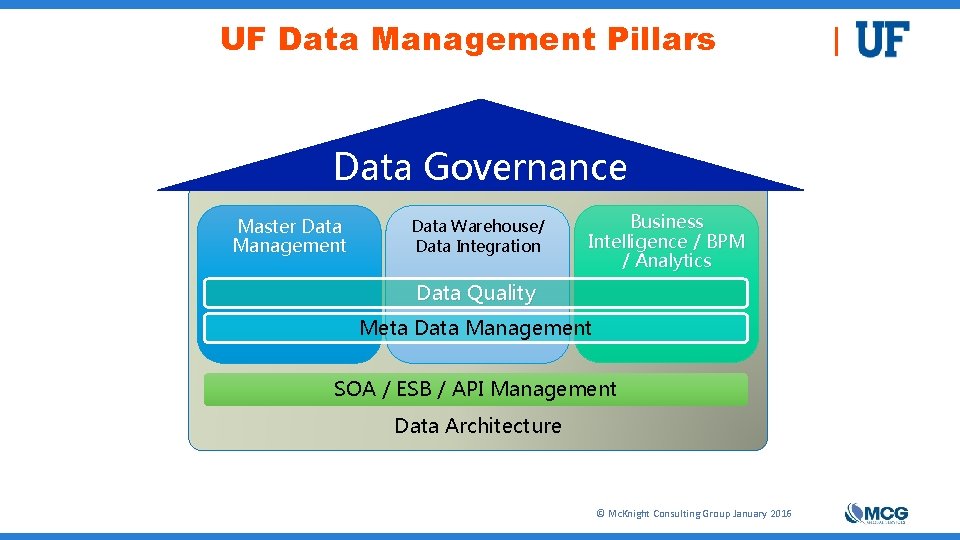
UF Data Management Pillars Data Governance Master Data Management Data Warehouse/ Data Integration Business Intelligence / BPM / Analytics Data Quality Meta Data Management SOA / ESB / API Management Data Architecture © Mc. Knight Consulting Group January 2016
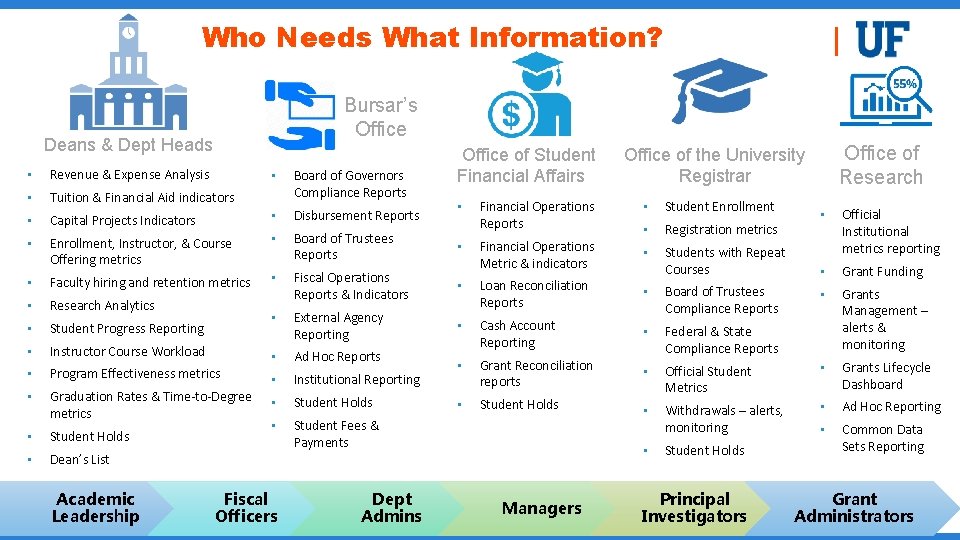
Who Needs What Information? Bursar’s Office Deans & Dept Heads • Revenue & Expense Analysis • Tuition & Financial Aid indicators • Board of Governors Compliance Reports Disbursement Reports Office of Student Financial Affairs • Financial Operations Reports • Student Enrollment • Financial Operations Metric & indicators Registration metrics • Students with Repeat Courses • Official Institutional metrics reporting • Grant Funding • Grants Management – alerts & monitoring Official Student Metrics • Grants Lifecycle Dashboard Withdrawals – alerts, monitoring • Ad Hoc Reporting • Common Data Sets Reporting • Capital Projects Indicators • • Enrollment, Instructor, & Course Offering metrics • Board of Trustees Reports • • Faculty hiring and retention metrics • • • Research Analytics Fiscal Operations Reports & Indicators • Student Progress Reporting • • • Grant Reconciliation reports • Graduation Rates & Time-to-Degree metrics Institutional Reporting • Federal & State Compliance Reports Program Effectiveness metrics Ad Hoc Reports Cash Account Reporting • Instructor Course Workload External Agency Reporting Board of Trustees Compliance Reports • • Loan Reconciliation Reports • Student Holds • • Dean’s List Student Fees & Payments • Academic Leadership Fiscal Officers Dept Admins • Managers Office of Research Office of the University Registrar Student Holds Principal Grant © Mc. Knight Consulting Group January 2016 Administrators Investigators
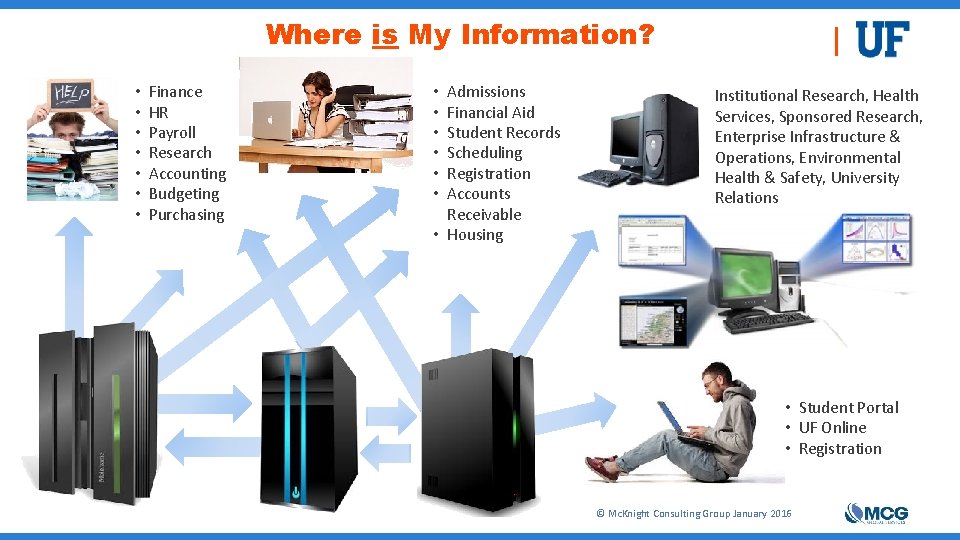
Where is My Information? • • Finance HR Payroll Research Accounting Budgeting Purchasing Admissions Financial Aid Student Records Scheduling Registration Accounts Receivable • Housing • • • Institutional Research, Health Services, Sponsored Research, Enterprise Infrastructure & Operations, Environmental Health & Safety, University Relations • Student Portal • UF Online • Registration © Mc. Knight Consulting Group January 2016
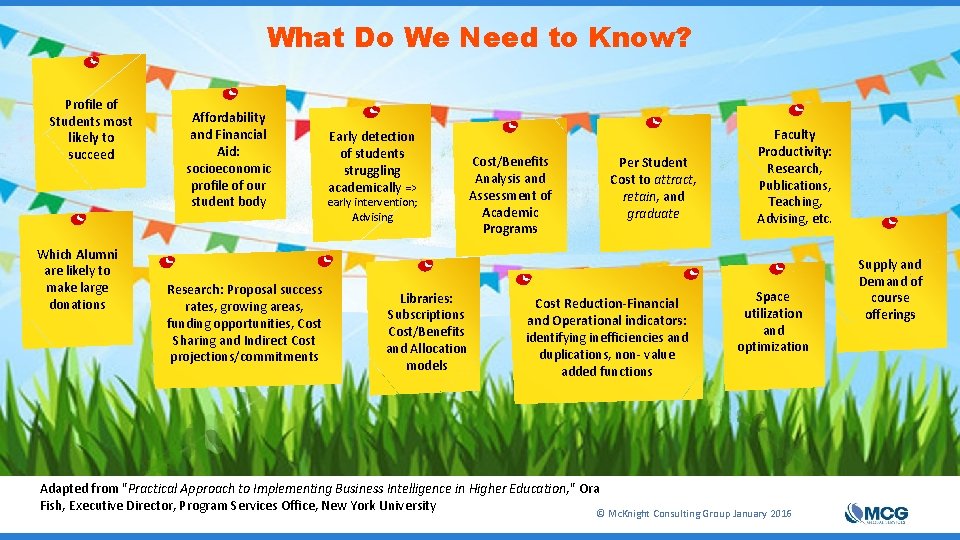
What Do We Need to Know? Profile of Students most likely to succeed Which Alumni are likely to make large donations Affordability and Financial Aid: socioeconomic profile of our student body Research: Proposal success rates, growing areas, funding opportunities, Cost Sharing and Indirect Cost projections/commitments Early detection of students struggling academically => early intervention; Advising Libraries: Subscriptions Cost/Benefits and Allocation models Cost/Benefits Analysis and Assessment of Academic Programs Per Student Cost to attract, retain, and graduate Cost Reduction-Financial and Operational indicators: identifying inefficiencies and duplications, non- value added functions Faculty Productivity: Research, Publications, Teaching, Advising, etc. Space utilization and optimization Adapted from "Practical Approach to Implementing Business Intelligence in Higher Education, " Ora Fish, Executive Director, Program Services Office, New York University © Mc. Knight Consulting Group January 2016 Supply and Demand of course offerings
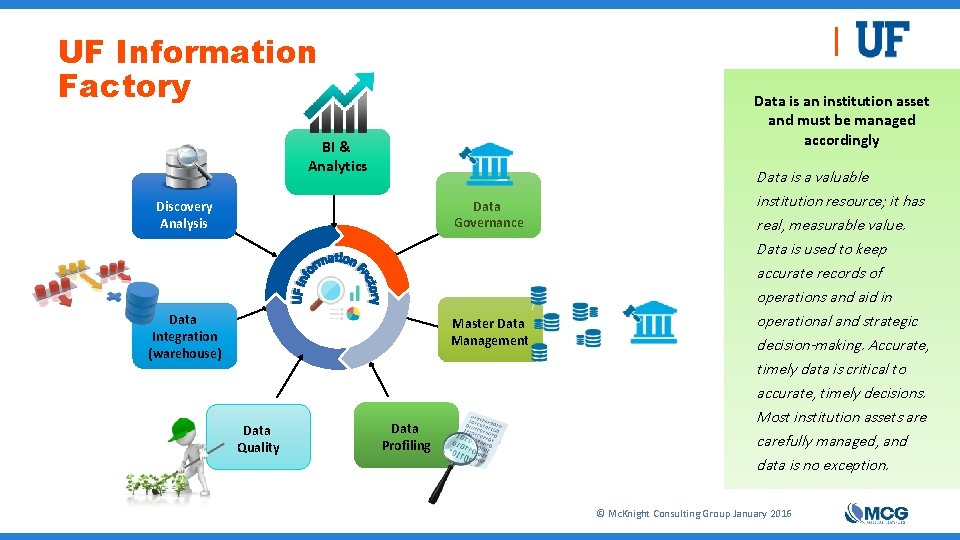
UF Information Factory Data is an institution asset and must be managed accordingly BI & Analytics Discovery Analysis Data Governance Data Integration (warehouse) Master Data Management Data Quality Data Profiling Data is a valuable institution resource; it has real, measurable value. Data is used to keep accurate records of operations and aid in operational and strategic decision-making. Accurate, timely data is critical to accurate, timely decisions. Most institution assets are carefully managed, and data is no exception. © Mc. Knight Consulting Group January 2016
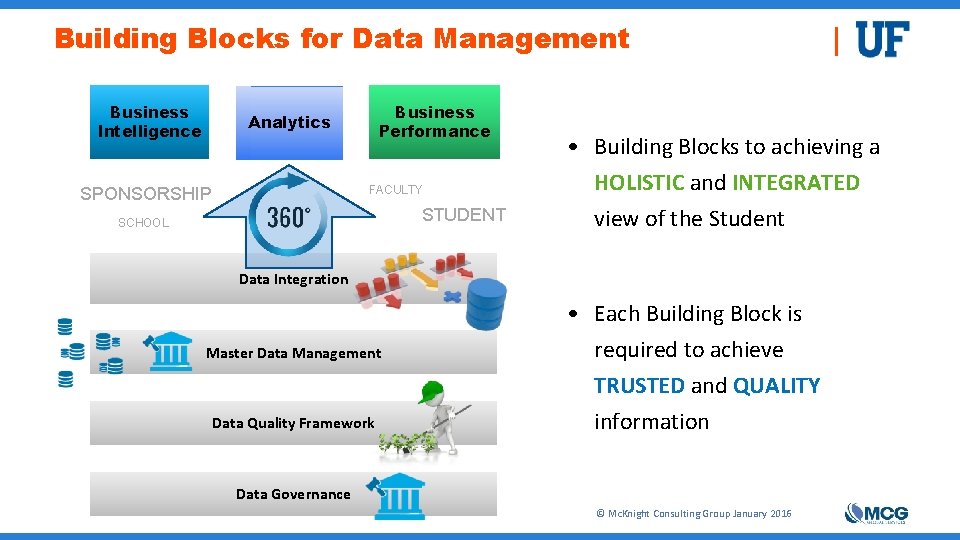
Building Blocks for Data Management Business Intelligence Business Performance Analytics FACULTY SPONSORSHIP STUDENT SCHOOL • Building Blocks to achieving a HOLISTIC and INTEGRATED view of the Student Data Integration Master Data Management Data Quality Framework • Each Building Block is required to achieve TRUSTED and QUALITY information Data Governance © Mc. Knight Consulting Group January 2016
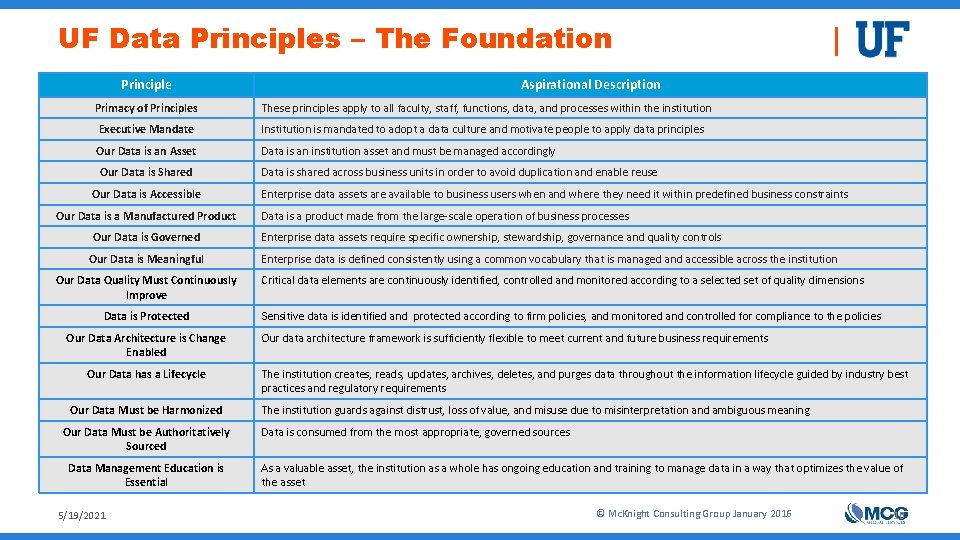
UF Data Principles – The Foundation Principle Primacy of Principles Aspirational Description These principles apply to all faculty, staff, functions, data, and processes within the institution Executive Mandate Institution is mandated to adopt a data culture and motivate people to apply data principles Our Data is an Asset Data is an institution asset and must be managed accordingly Our Data is Shared Our Data is Accessible Our Data is a Manufactured Product Our Data is Governed Our Data is Meaningful Our Data Quality Must Continuously Improve Data is Protected Our Data Architecture is Change Enabled Our Data has a Lifecycle Our Data Must be Harmonized Our Data Must be Authoritatively Sourced Data Management Education is Essential 5/19/2021 Data is shared across business units in order to avoid duplication and enable reuse Enterprise data assets are available to business users when and where they need it within predefined business constraints Data is a product made from the large-scale operation of business processes Enterprise data assets require specific ownership, stewardship, governance and quality controls Enterprise data is defined consistently using a common vocabulary that is managed and accessible across the institution Critical data elements are continuously identified, controlled and monitored according to a selected set of quality dimensions Sensitive data is identified and protected according to firm policies, and monitored and controlled for compliance to the policies Our data architecture framework is sufficiently flexible to meet current and future business requirements The institution creates, reads, updates, archives, deletes, and purges data throughout the information lifecycle guided by industry best practices and regulatory requirements The institution guards against distrust, loss of value, and misuse due to misinterpretation and ambiguous meaning Data is consumed from the most appropriate, governed sources As a valuable asset, the institution as a whole has ongoing education and training to manage data in a way that optimizes the value of the asset © Mc. Knight Consulting Group January 2016 10
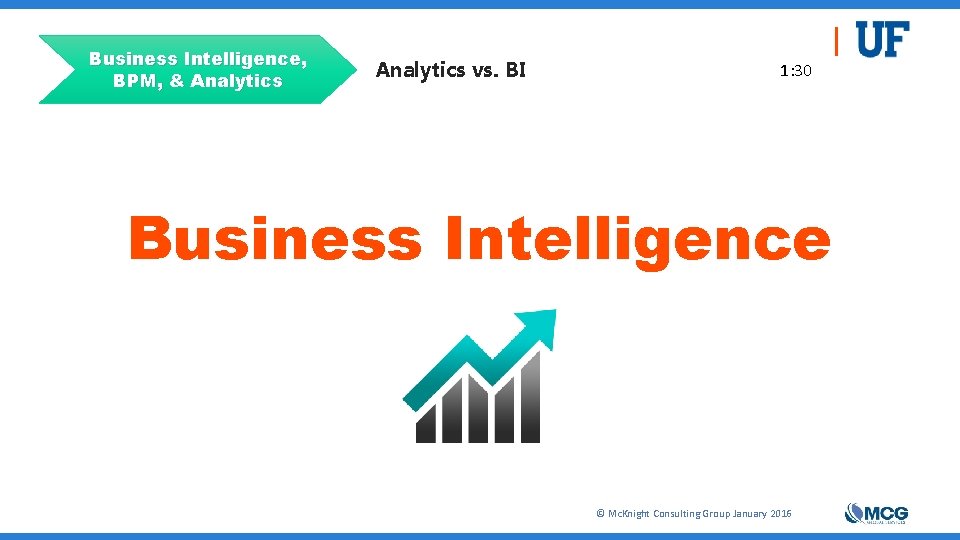
Business Intelligence, BPM, & Analytics vs. BI 1: 30 Business Intelligence © Mc. Knight Consulting Group January 2016
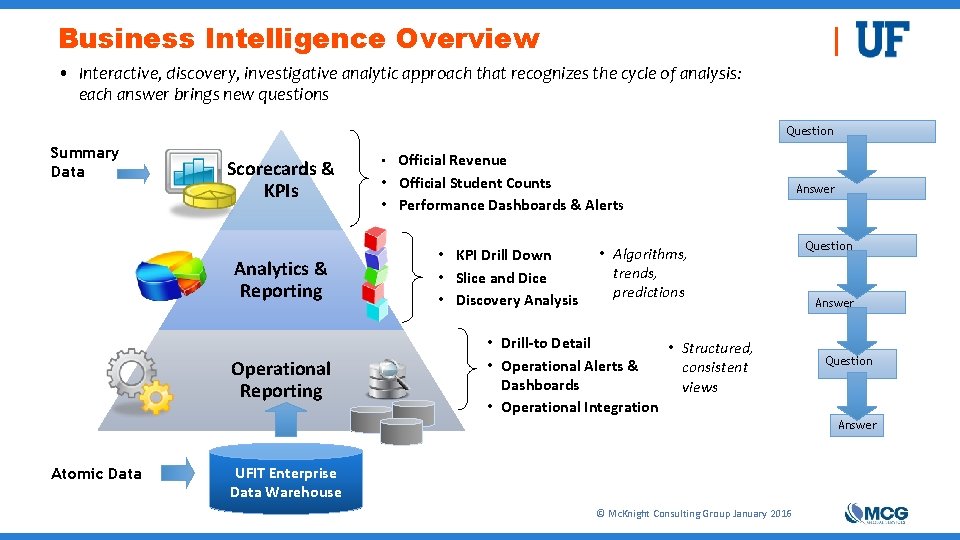
Business Intelligence Overview • Interactive, discovery, investigative analytic approach that recognizes the cycle of analysis: each answer brings new questions Question Summary Data Scorecards & KPIs Analytics & Reporting Operational Reporting • Official Revenue • Official Student Counts • Performance Dashboards & Alerts • KPI Drill Down • Slice and Dice • Discovery Analysis • Algorithms, trends, predictions • Drill-to Detail • Structured, • Operational Alerts & consistent Dashboards views • Operational Integration Answer Question Answer Atomic Data UFIT Enterprise Data Warehouse © Mc. Knight Consulting Group January 2016
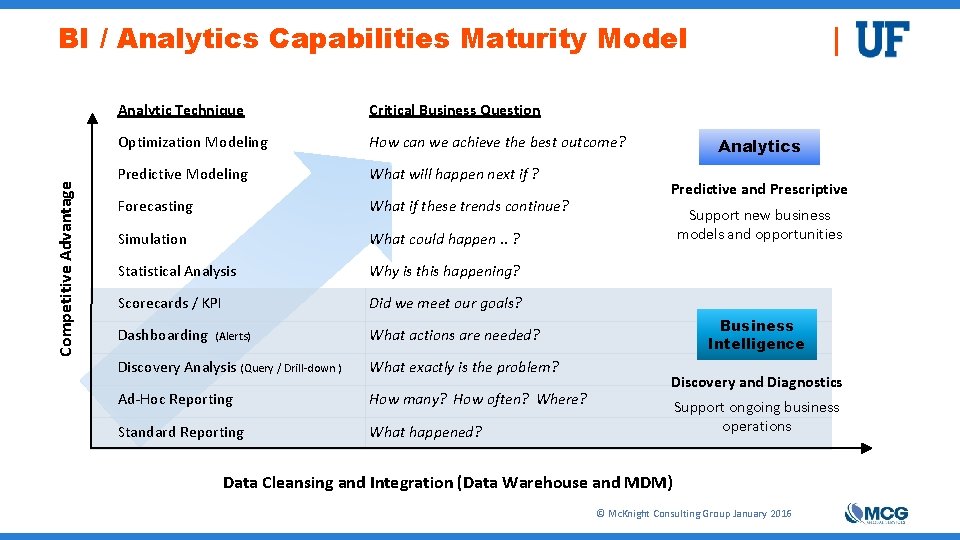
Competitive Advantage BI / Analytics Capabilities Maturity Model Analytic Technique Critical Business Question Optimization Modeling How can we achieve the best outcome? Predictive Modeling What will happen next if ? Forecasting What if these trends continue? Simulation What could happen. . ? Statistical Analysis Why is this happening? Scorecards / KPI Did we meet our goals? Dashboarding What actions are needed? (Alerts) Discovery Analysis (Query / Drill-down ) What exactly is the problem? Ad-Hoc Reporting How many? How often? Where? Standard Reporting What happened? Analytics Predictive and Prescriptive Support new business models and opportunities Business Intelligence Discovery and Diagnostics Support ongoing business operations Data Cleansing and Integration (Data Warehouse and MDM) © Mc. Knight Consulting Group January 2016
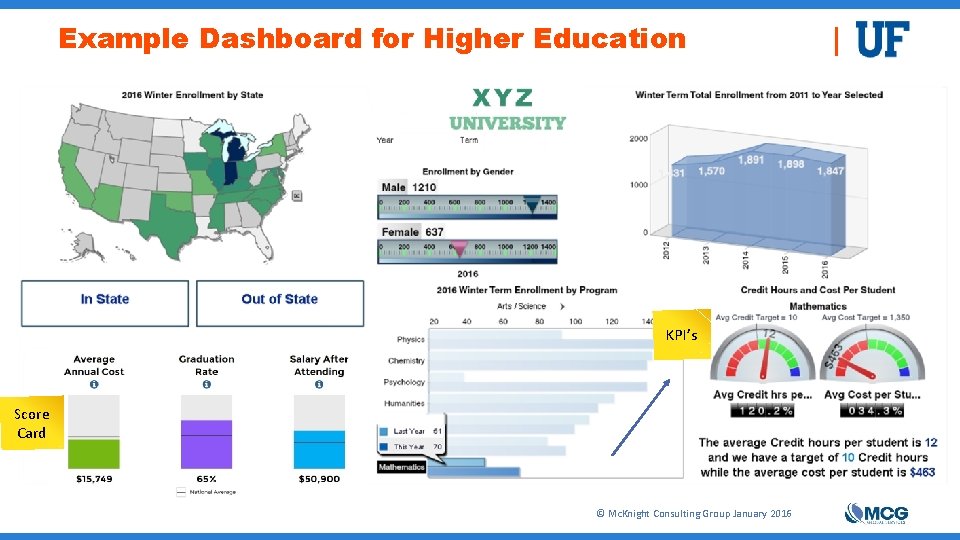
Example Dashboard for Higher Education KPI’s Score Card © Mc. Knight Consulting Group January 2016
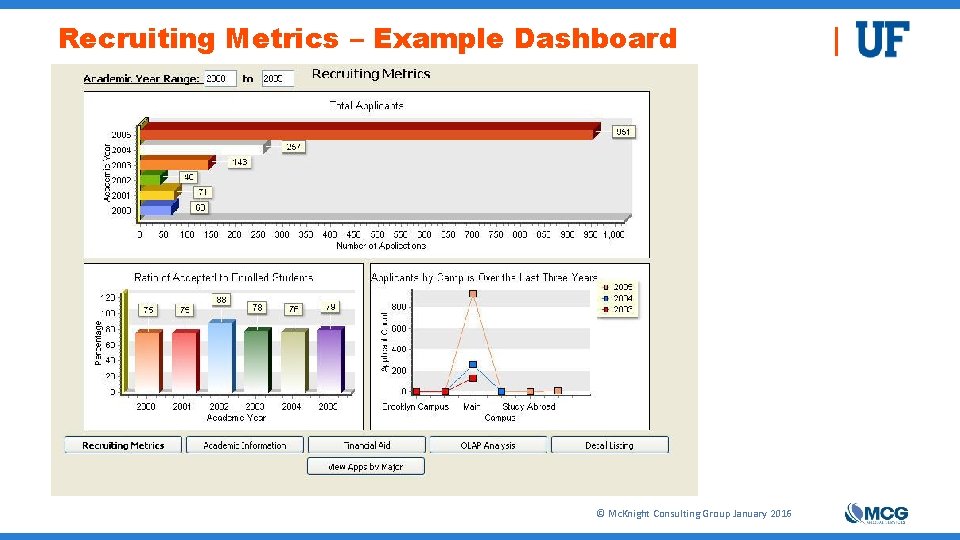
Recruiting Metrics – Example Dashboard © Mc. Knight Consulting Group January 2016
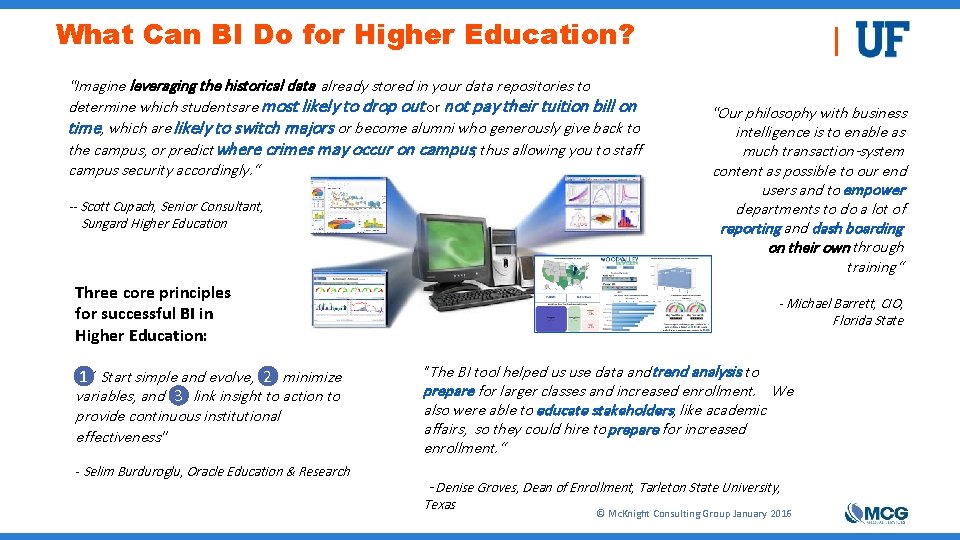
What Can BI Do for Higher Education? "Imagine leveraging the historical data already stored in your data repositories to determine which studentsare most likely to drop out or not pay their tuition bill on time, which are likely to switch majors or become alumni who generously give back to the campus, or predict where crimes may occur on campus, thus allowing you to staff campus security accordingly. ” -- Scott Cupach, Senior Consultant, Sungard Higher Education "Our philosophy with business intelligence is to enable as much transaction-system content as possible to our end users and to empower departments to do a lot of reporting and dash boarding on their own through training” Three core principles for successful BI in Higher Education: 1“ Start simple and evolve, 2 minimize variables, and 3 link insight to action to provide continuous institutional effectiveness. ” - Selim Burduroglu, Oracle Education & Research - Michael Barrett, CIO, Florida State "The BI tool helped us use data and trend analysis to prepare for larger classes and increased enrollment. We also were able to educate stakeholders, like academic affairs, so they could hire to prepare for increased enrollment. ” - Denise Groves, Dean of Enrollment, Tarleton State University, Texas © Mc. Knight Consulting Group January 2016
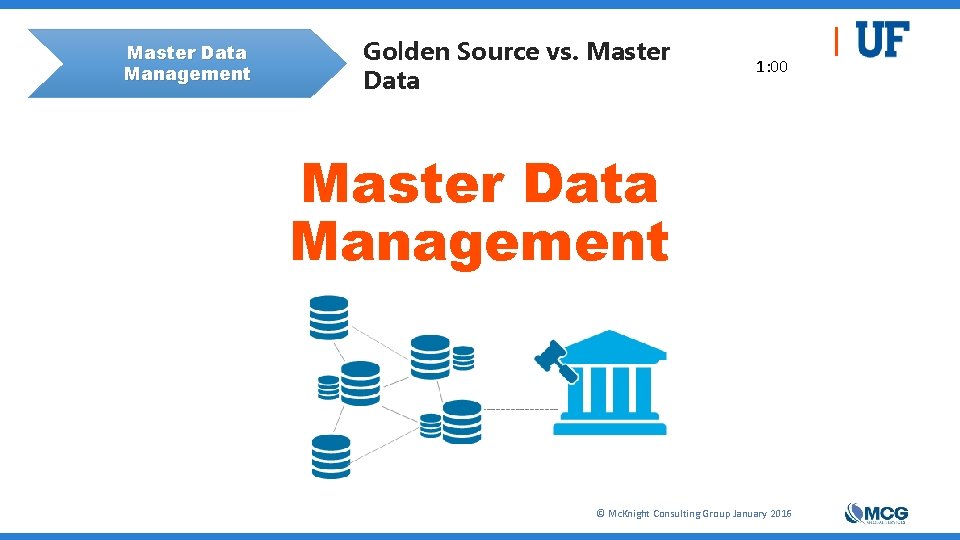
Master Data Management Golden Source vs. Master Data 1: 00 Master Data Management © Mc. Knight Consulting Group January 2016
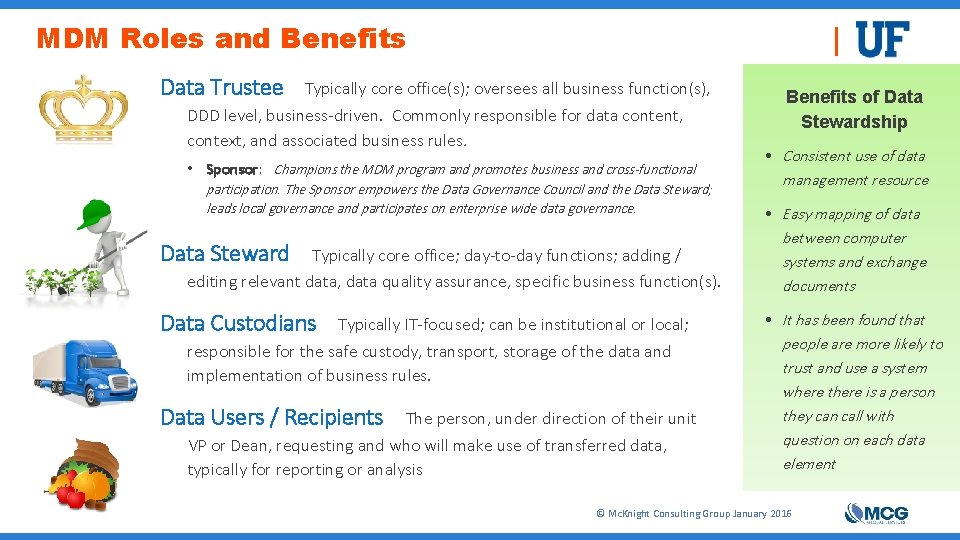
MDM Roles and Benefits Data Trustee Typically core office(s); oversees all business function(s), DDD level, business-driven. Commonly responsible for data content, context, and associated business rules. • Sponsor: Champions the MDM program and promotes business and cross-functional participation. The Sponsor empowers the Data Governance Council and the Data Steward; leads local governance and participates on enterprise wide data governance. Data Steward Typically core office; day-to-day functions; adding / editing relevant data, data quality assurance, specific business function(s). Data Custodians Typically IT-focused; can be institutional or local; responsible for the safe custody, transport, storage of the data and implementation of business rules. Data Users / Recipients The person, under direction of their unit VP or Dean, requesting and who will make use of transferred data, typically for reporting or analysis Benefits of Data Stewardship • Consistent use of data management resource • Easy mapping of data between computer systems and exchange documents • It has been found that people are more likely to trust and use a system where there is a person they can call with question on each data element © Mc. Knight Consulting Group January 2016
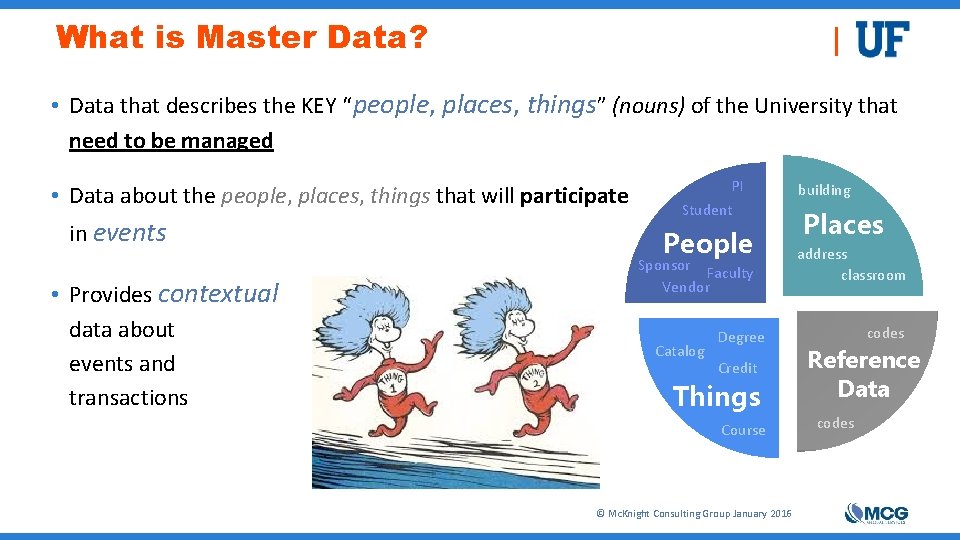
What is Master Data? • Data that describes the KEY “people, places, things” (nouns) of the University that need to be managed • Data about the people, places, things that will participate in events • Provides contextual data about events and transactions PI Student People Sponsor Faculty Vendor Catalog Degree Credit Things Course © Mc. Knight Consulting Group January 2016 building Places address classroom codes Reference Data codes
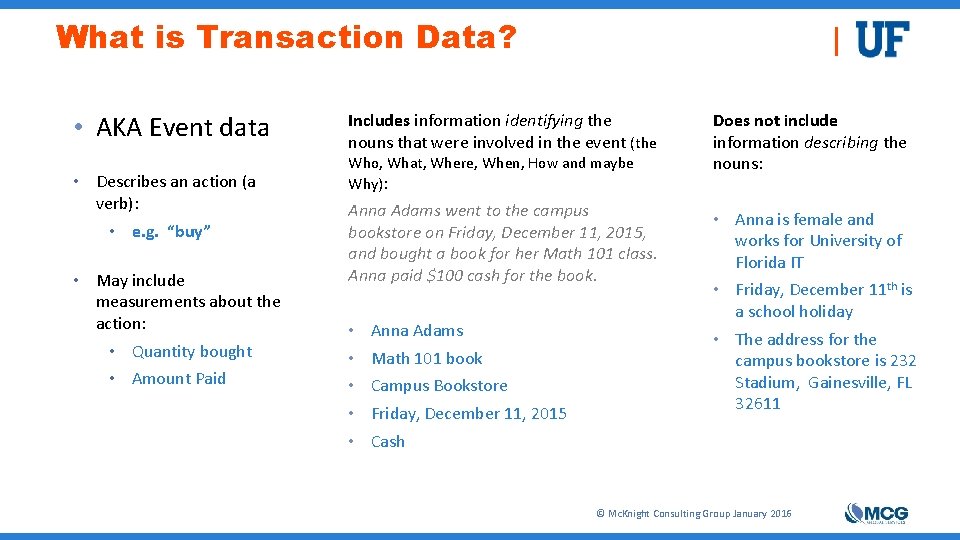
What is Transaction Data? • AKA Event data • Describes an action (a verb): • e. g. “buy” • May include measurements about the action: • Quantity bought • Amount Paid Includes information identifying the nouns that were involved in the event (the Does not include information describing the nouns: Anna Adams went to the campus bookstore on Friday, December 11, 2015, and bought a book for her Math 101 class. Anna paid $100 cash for the book. • Anna is female and works for University of Florida IT • Friday, December 11 th is a school holiday • The address for the campus bookstore is 232 Stadium, Gainesville, FL 32611 Who, What, Where, When, How and maybe Why): • • • Anna Adams Math 101 book Campus Bookstore Friday, December 11, 2015 Cash © Mc. Knight Consulting Group January 2016
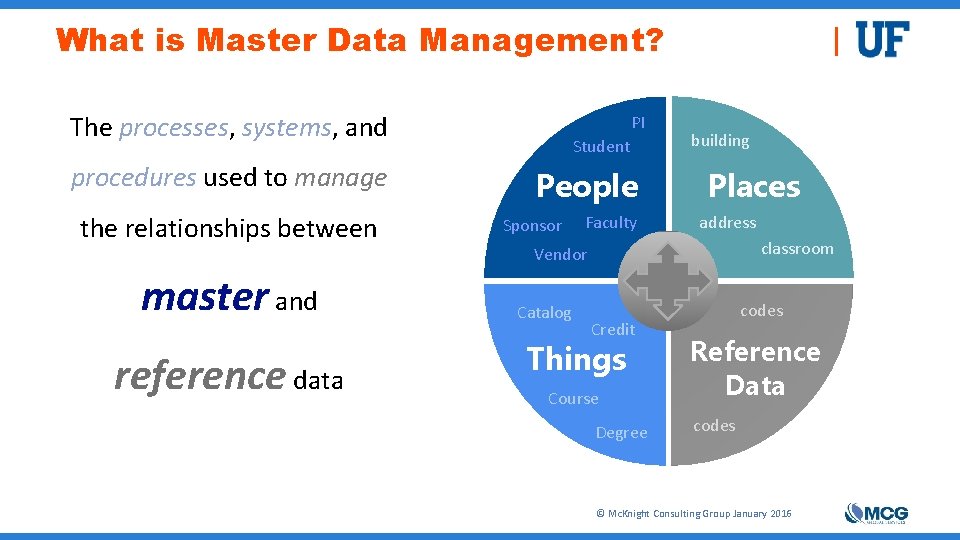
What is Master Data Management? The processes, systems, and procedures used to manage the relationships between master and reference data PI Student People Sponsor Faculty building Places address classroom Vendor Catalog Credit Things Course Degree codes Reference Data codes © Mc. Knight Consulting Group January 2016
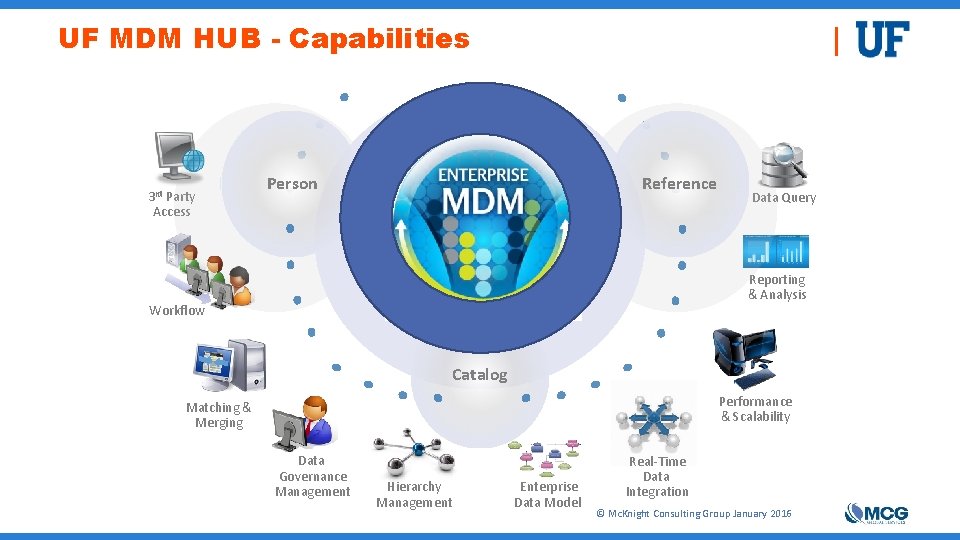
UF MDM HUB - Capabilities 3 rd Party Access Reference Person Data Query Reporting & Analysis Workflow Catalog Performance & Scalability Matching & Merging Data Governance Management Hierarchy Management Enterprise Data Model Real-Time Data Integration © Mc. Knight Consulting Group January 2016
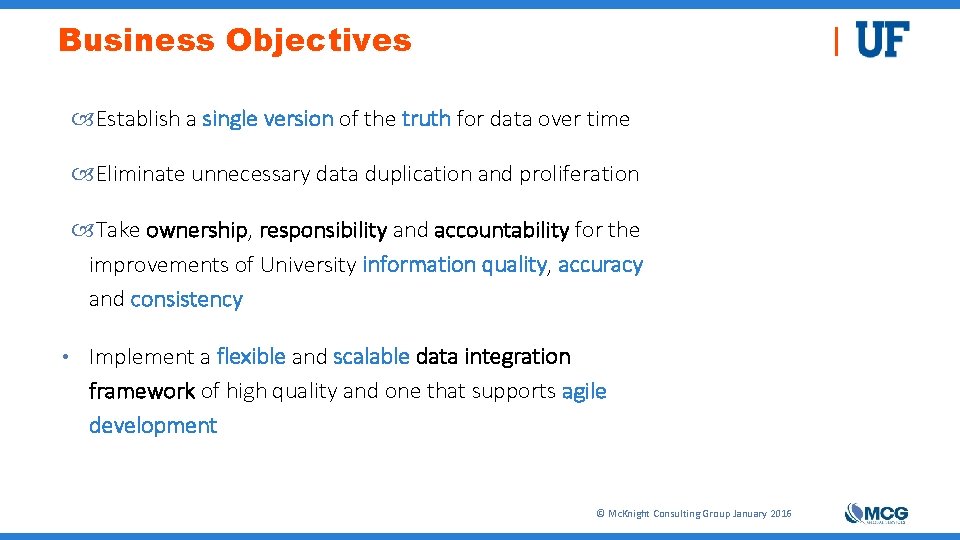
Business Objectives Establish a single version of the truth for data over time Eliminate unnecessary data duplication and proliferation Take ownership, responsibility and accountability for the improvements of University information quality, accuracy and consistency • Implement a flexible and scalable data integration framework of high quality and one that supports agile development © Mc. Knight Consulting Group January 2016
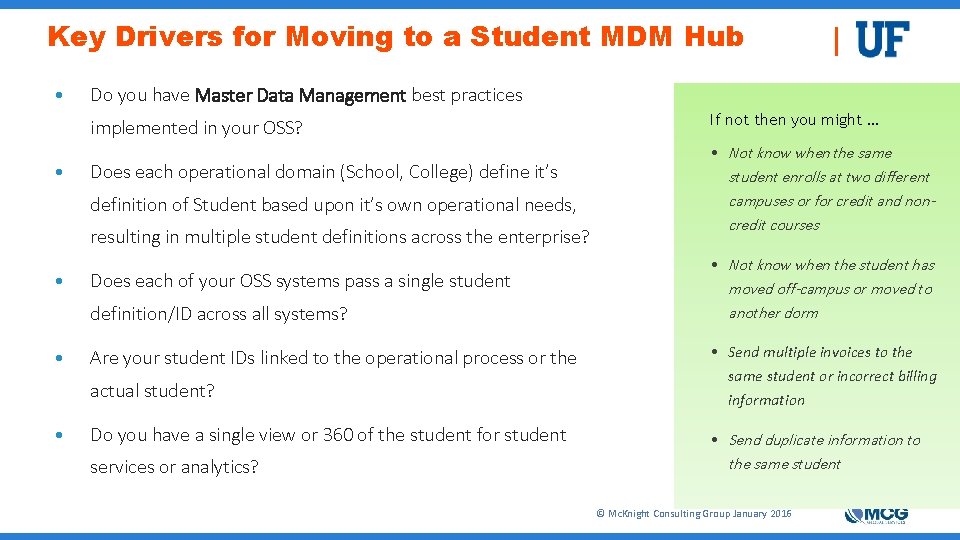
Key Drivers for Moving to a Student MDM Hub • Do you have Master Data Management best practices implemented in your OSS? • Does each operational domain (School, College) define it’s definition of Student based upon it’s own operational needs, resulting in multiple student definitions across the enterprise? • Does each of your OSS systems pass a single student definition/ID across all systems? • Are your student IDs linked to the operational process or the actual student? • Do you have a single view or 360 of the student for student services or analytics? If not then you might … • Not know when the same student enrolls at two different campuses or for credit and noncredit courses • Not know when the student has moved off-campus or moved to another dorm • Send multiple invoices to the same student or incorrect billing information • Send duplicate information to the same student © Mc. Knight Consulting Group January 2016
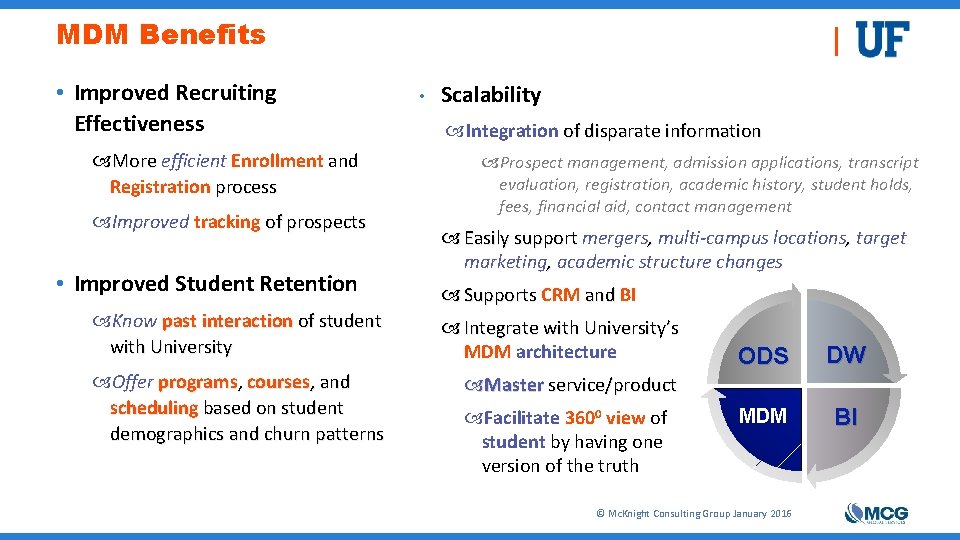
MDM Benefits • Improved Recruiting Effectiveness More efficient Enrollment and Registration process Improved tracking of prospects • Improved Student Retention • Scalability Integration of disparate information Prospect management, admission applications, transcript evaluation, registration, academic history, student holds, fees, financial aid, contact management Easily support mergers, multi-campus locations, target marketing, academic structure changes Supports CRM and BI Know past interaction of student with University Integrate with University’s MDM architecture Offer programs, courses, and scheduling based on student demographics and churn patterns Master service/product Facilitate 3600 view of student by having one version of the truth ODS DW MDM BI © Mc. Knight Consulting Group January 2016
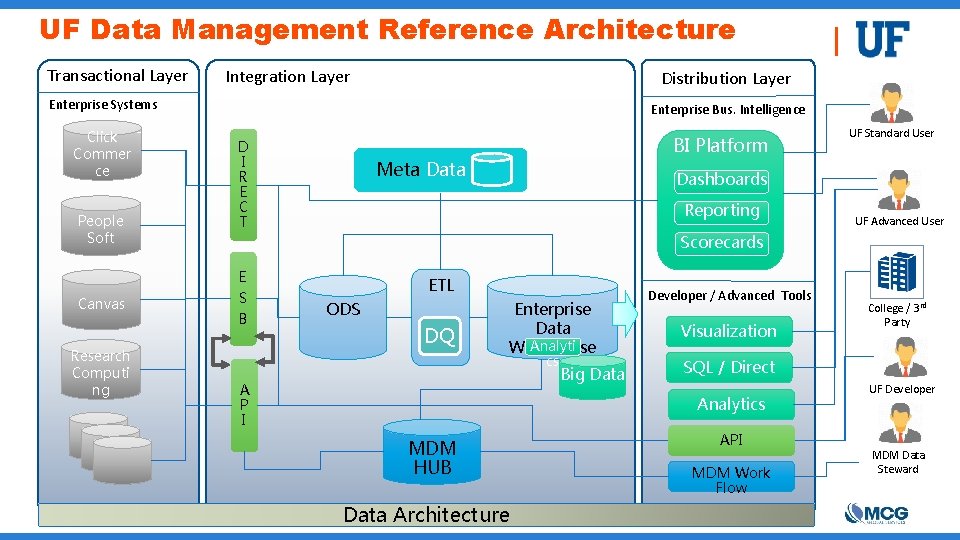
UF Data Management Reference Architecture Transactional Layer Integration Layer Distribution Layer Enterprise Systems Click Commer ce People Soft Canvas Research Computi ng Enterprise Bus. Intelligence D I R E C T E S B Meta Data BI Platform _ UF Standard User Dashboards Reporting UF Advanced User Scorecards ETL ODS DQ Enterprise Data Analyti Warehouse cs A P I Big Data Developer / Advanced Tools Visualization SQL / Direct Analytics MDM HUB Data Architecture College / 3 rd Party API MDM Work Flow © Mc. Knight Consulting Group January 2016 UF Developer MDM Data Steward
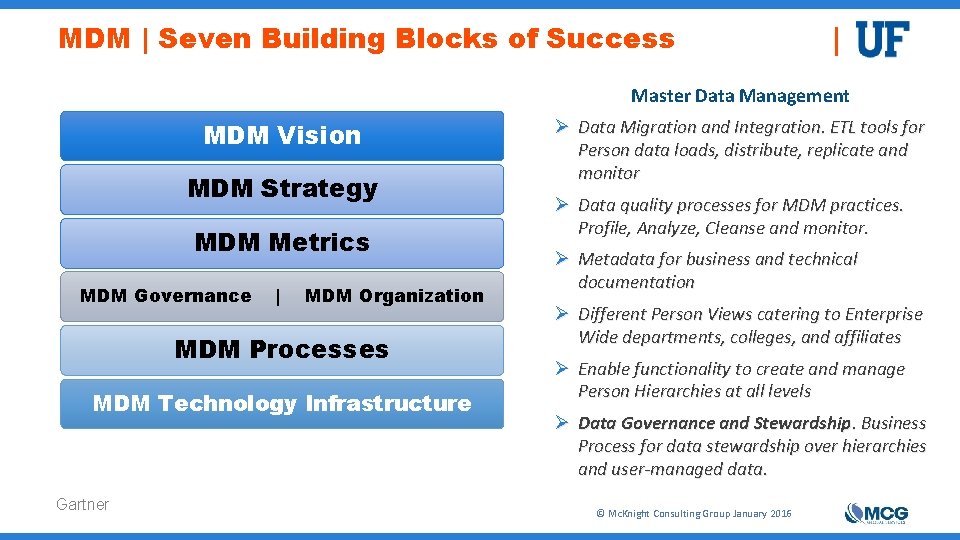
MDM | Seven Building Blocks of Success Master Data Management MDM Vision MDM Strategy MDM Metrics MDM Governance | MDM Organization MDM Processes MDM Technology Infrastructure Gartner Ø Data Migration and Integration. ETL tools for Person data loads, distribute, replicate and monitor Ø Data quality processes for MDM practices. Profile, Analyze, Cleanse and monitor. Ø Metadata for business and technical documentation Ø Different Person Views catering to Enterprise Wide departments, colleges, and affiliates Ø Enable functionality to create and manage Person Hierarchies at all levels Ø Data Governance and Stewardship. Business Process for data stewardship over hierarchies and user-managed data. © Mc. Knight Consulting Group January 2016
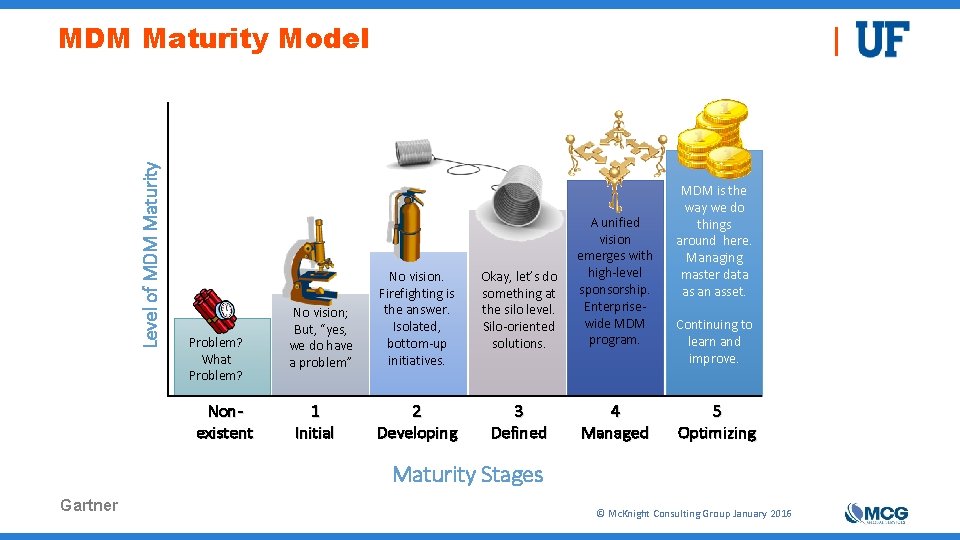
Level of MDM Maturity Model Problem? What Problem? Nonexistent No vision; But, “yes, we do have a problem” 1 Initial No vision. Firefighting is the answer. Isolated, bottom-up initiatives. Okay, let’s do something at the silo level. Silo-oriented solutions. A unified vision emerges with high-level sponsorship. Enterprisewide MDM program. 2 Developing 3 Defined 4 Managed MDM is the way we do things around here. Managing master data as an asset. Continuing to learn and improve. 5 Optimizing Maturity Stages Gartner © Mc. Knight Consulting Group January 2016
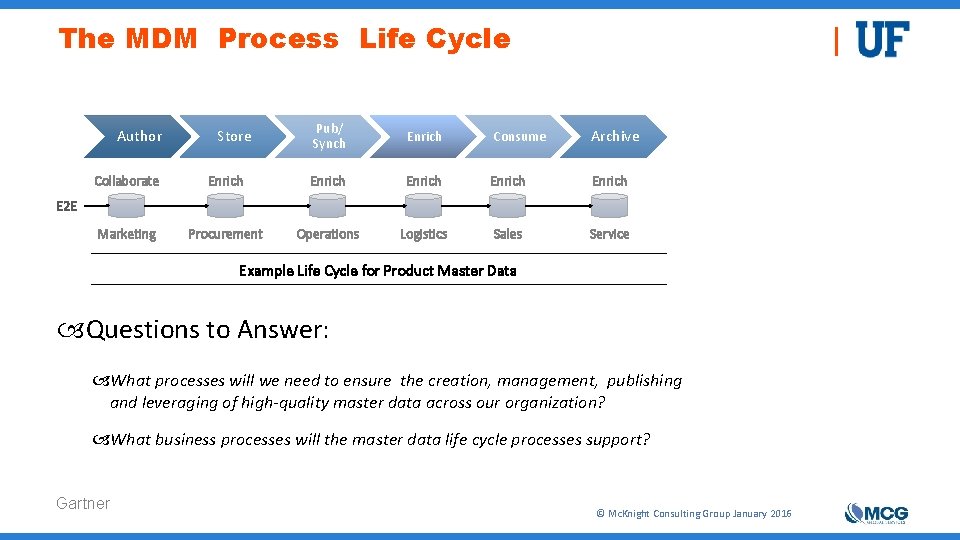
The MDM Process Life Cycle Author Store Pub/ Synch Enrich Consume Archive Collaborate Enrich Enrich Marketing Procurement Operations Logistics Sales Service E 2 E Example Life Cycle for Product Master Data Questions to Answer: What processes will we need to ensure the creation, management, publishing and leveraging of high-quality master data across our organization? What business processes will the master data life cycle processes support? Gartner © Mc. Knight Consulting Group January 2016
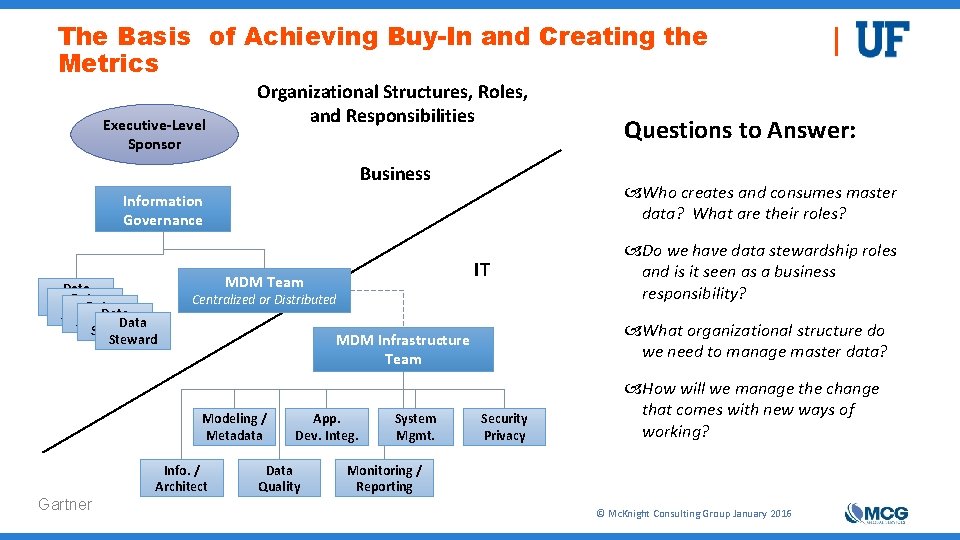
The Basis of Achieving Buy-In and Creating the Metrics Executive-Level Sponsor Organizational Structures, Roles, and Responsibilities Business Who creates and consumes master data? What are their roles? Information Governance Board Data Steward Centralized or Distributed Info. / Architect App. Dev. Integ. Data Quality System Mgmt. Do we have data stewardship roles and is it seen as a business responsibility? What organizational structure do we need to manage master data? MDM Infrastructure Team Modeling / Metadata Gartner IT MDM Team Questions to Answer: Security Privacy How will we manage the change that comes with new ways of working? Monitoring / Reporting © Mc. Knight Consulting Group January 2016
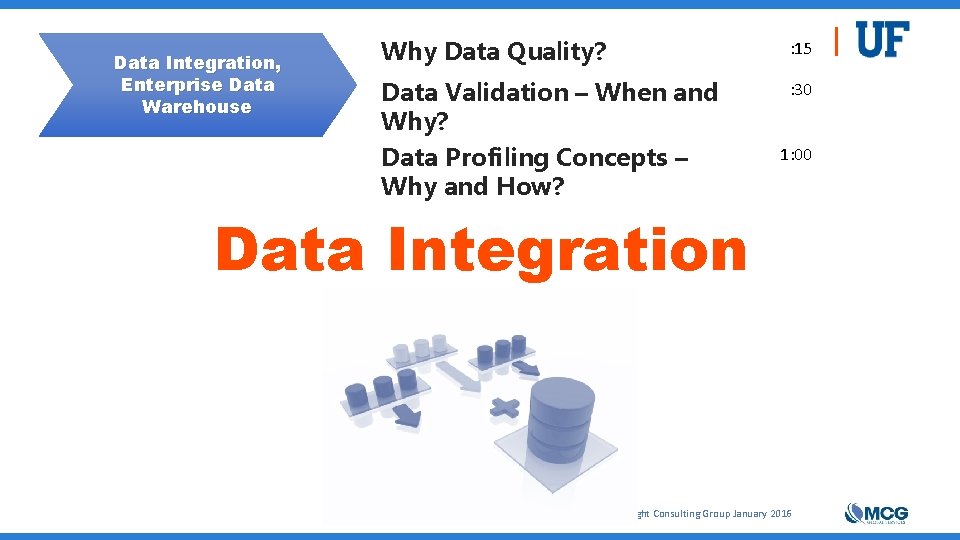
Data Integration, Enterprise Data Warehouse Why Data Quality? : 15 Data Validation – When and Why? Data Profiling Concepts – Why and How? : 30 1: 00 Data Integration © Mc. Knight Consulting Group January 2016
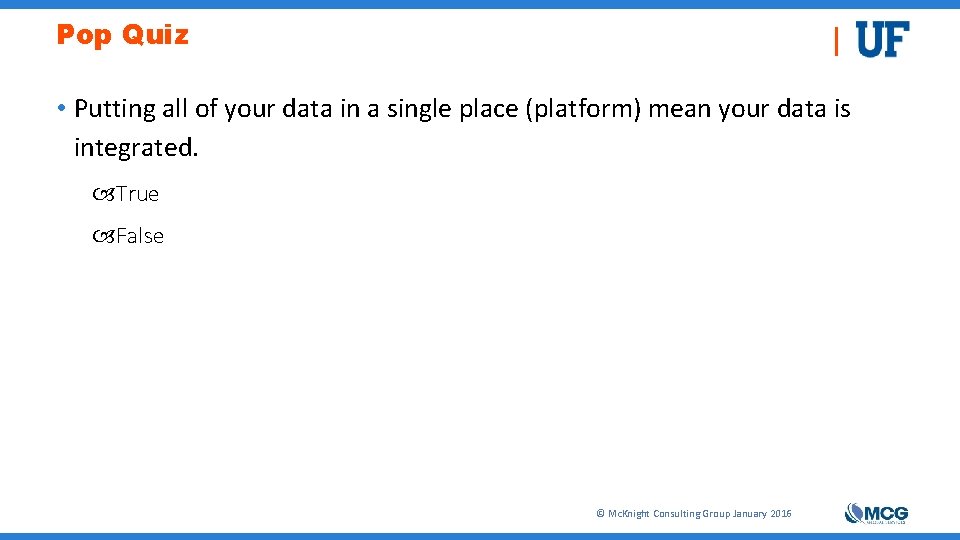
Pop Quiz • Putting all of your data in a single place (platform) mean your data is integrated. True False © Mc. Knight Consulting Group January 2016
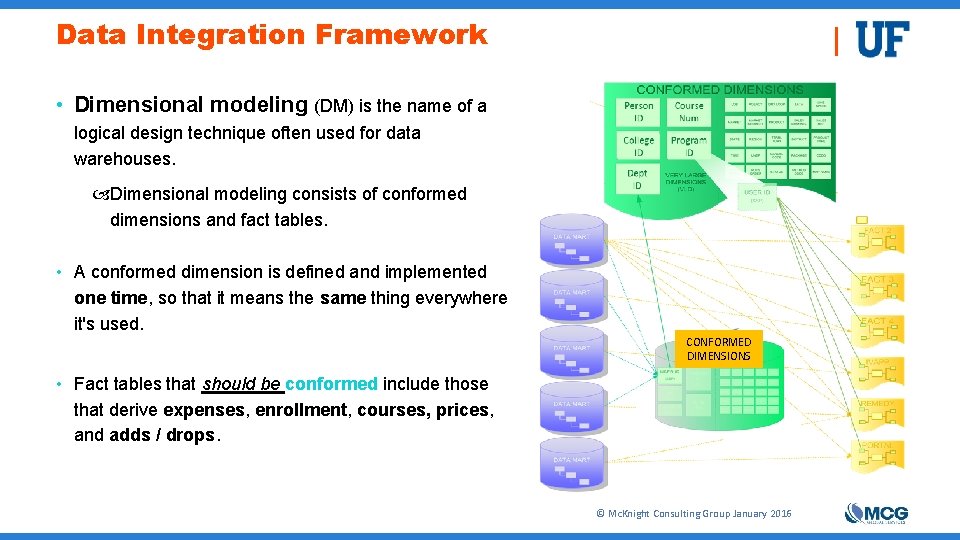
Data Integration Framework • Dimensional modeling (DM) is the name of a logical design technique often used for data warehouses. Dimensional modeling consists of conformed dimensions and fact tables. • A conformed dimension is defined and implemented one time, so that it means the same thing everywhere it's used. CONFORMED DIMENSIONS • Fact tables that should be conformed include those that derive expenses, enrollment, courses, prices, and adds / drops. © Mc. Knight Consulting Group January 2016
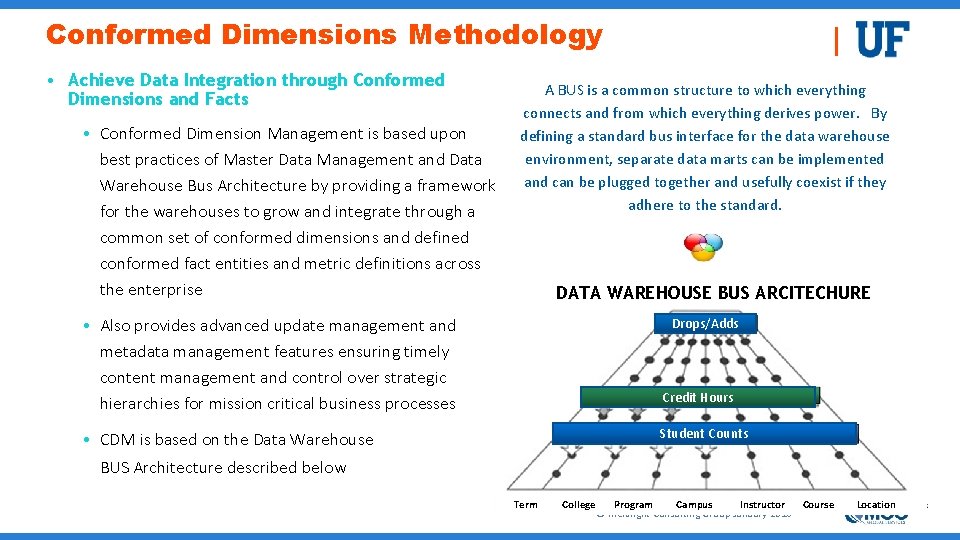
Conformed Dimensions Methodology • Achieve Data Integration through Conformed Dimensions and Facts • Conformed Dimension Management is based upon best practices of Master Data Management and Data Warehouse Bus Architecture by providing a framework for the warehouses to grow and integrate through a common set of conformed dimensions and defined conformed fact entities and metric definitions across the enterprise A BUS is a common structure to which everything connects and from which everything derives power. By defining a standard bus interface for the data warehouse environment, separate data marts can be implemented and can be plugged together and usefully coexist if they adhere to the standard. DATA WAREHOUSE BUS ARCITECHURE • Also provides advanced update management and metadata management features ensuring timely content management and control over strategic hierarchies for mission critical business processes Drops/Adds Credit Hours Student Counts • CDM is based on the Data Warehouse BUS Architecture described below Term College Program Instructor Course Campus © Mc. Knight Consulting Group January 2016 Location
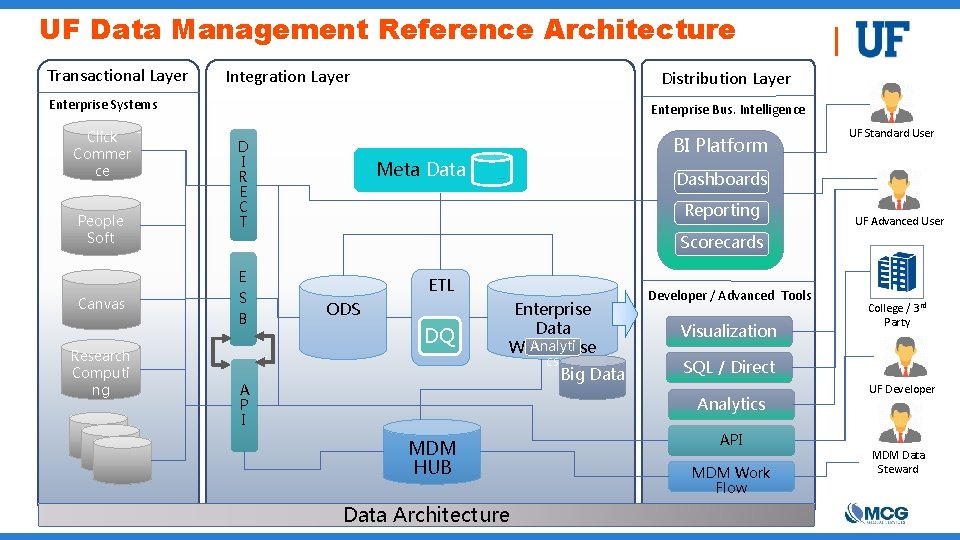
UF Data Management Reference Architecture Transactional Layer Integration Layer Distribution Layer Enterprise Systems Click Commer ce People Soft Canvas Research Computi ng Enterprise Bus. Intelligence D I R E C T E S B Meta Data BI Platform _ UF Standard User Dashboards Reporting UF Advanced User Scorecards ETL ODS DQ Enterprise Data Analyti Warehouse cs A P I Big Data Developer / Advanced Tools Visualization SQL / Direct Analytics MDM HUB Data Architecture College / 3 rd Party API MDM Work Flow © Mc. Knight Consulting Group January 2016 UF Developer MDM Data Steward
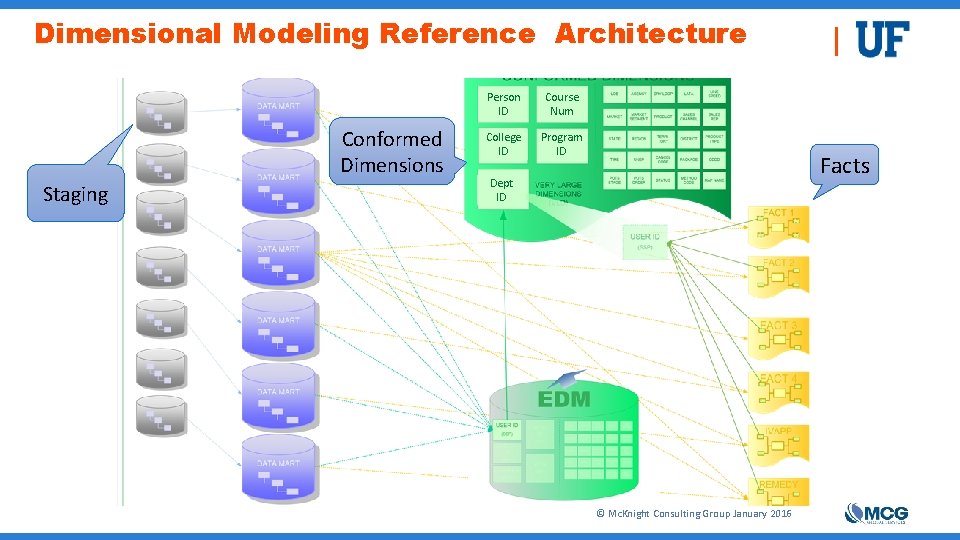
Dimensional Modeling Reference Architecture Conformed Dimensions Staging Person ID Course Num College ID Program ID Facts Dept ID © Mc. Knight Consulting Group January 2016
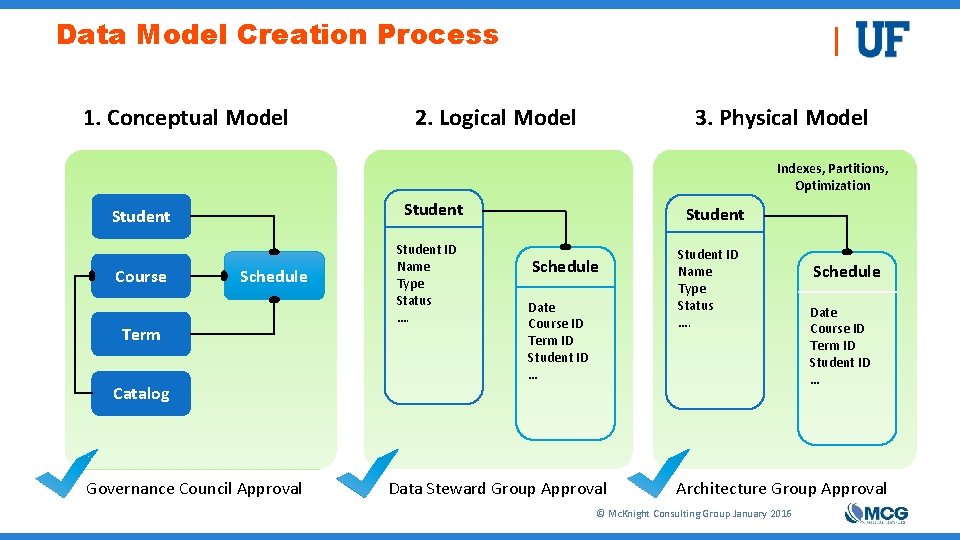
Data Model Creation Process 1. Conceptual Model 2. Logical Model 3. Physical Model Indexes, Partitions, Optimization Student Course Schedule Term Catalog Governance Council Approval Student ID Name Type Status …. Student Schedule Date Course ID Term ID Student ID … Data Steward Group Approval Student ID Name Type Status …. Schedule Date Course ID Term ID Student ID … Architecture Group Approval © Mc. Knight Consulting Group January 2016
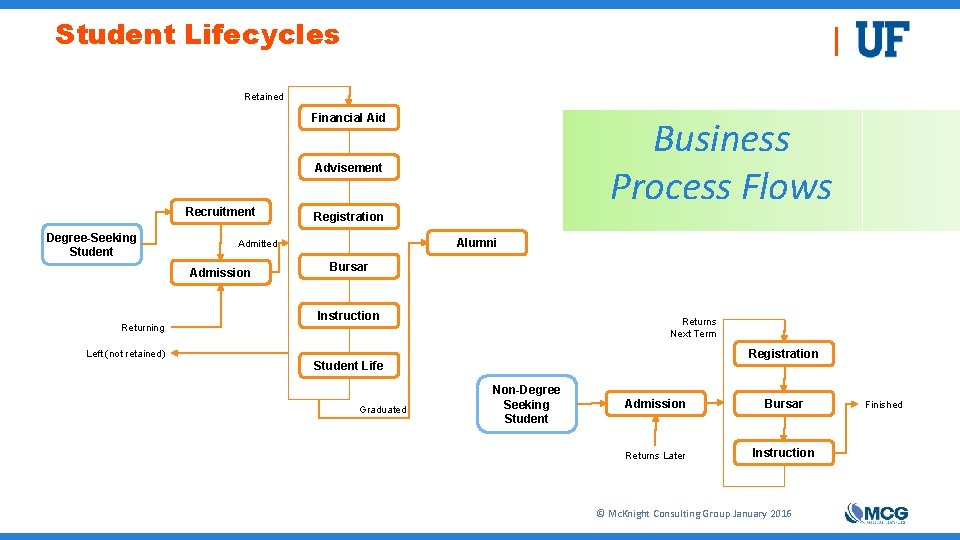
Student Lifecycles Retained Financial Aid Business Process Flows Advisement Recruitment Degree-Seeking Student Left (not retained) Alumni Admitted Admission Returning Registration Bursar Instruction Returns Next Term Registration Student Life Graduated Non-Degree Seeking Student Admission Bursar Returns Later Instruction © Mc. Knight Consulting Group January 2016 Finished
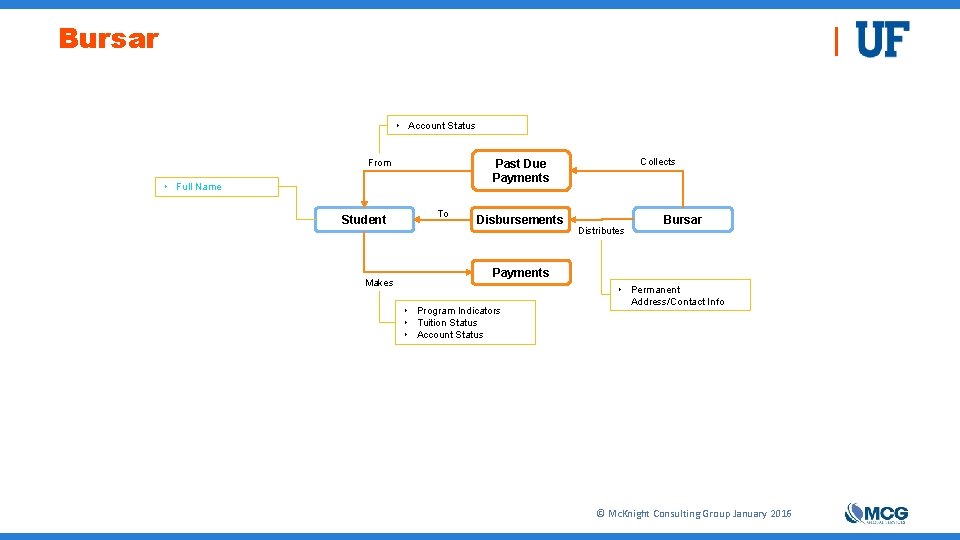
Bursar ‣ Account Status From ‣ Full Name Student Makes Collects Past Due Payments To Disbursements Distributes Bursar Payments ‣ Program Indicators ‣ Tuition Status ‣ Account Status ‣ Permanent Address/Contact Info © Mc. Knight Consulting Group January 2016
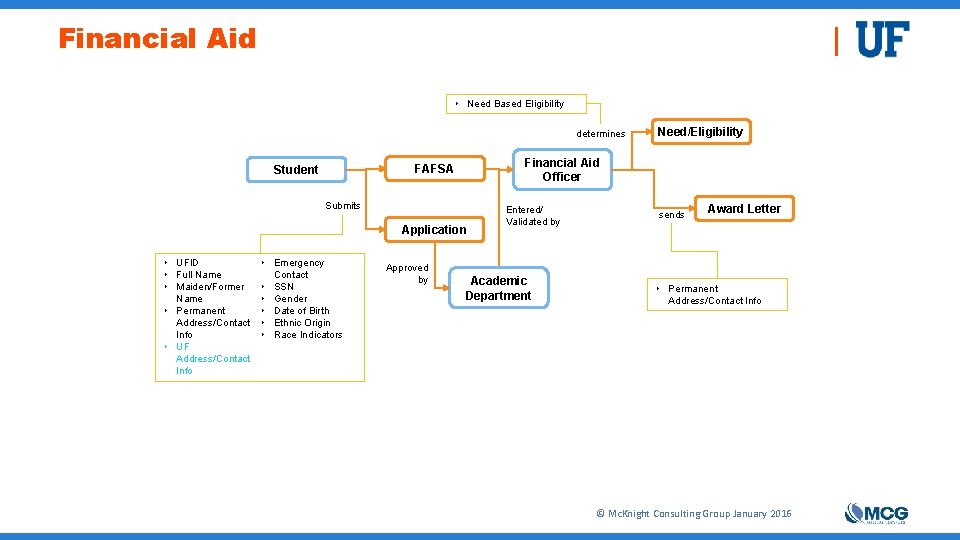
Financial Aid ‣ Need Based Eligibility determines Financial Aid Officer FAFSA Student Submits Application ‣ UFID ‣ Full Name ‣ Maiden/Former Name ‣ Permanent Address/Contact Info ‣ UF Address/Contact Info ‣ Emergency Contact ‣ SSN ‣ Gender ‣ Date of Birth ‣ Ethnic Origin ‣ Race Indicators Approved by Need/Eligibility Entered/ Validated by Academic Department sends Award Letter ‣ Permanent Address/Contact Info © Mc. Knight Consulting Group January 2016
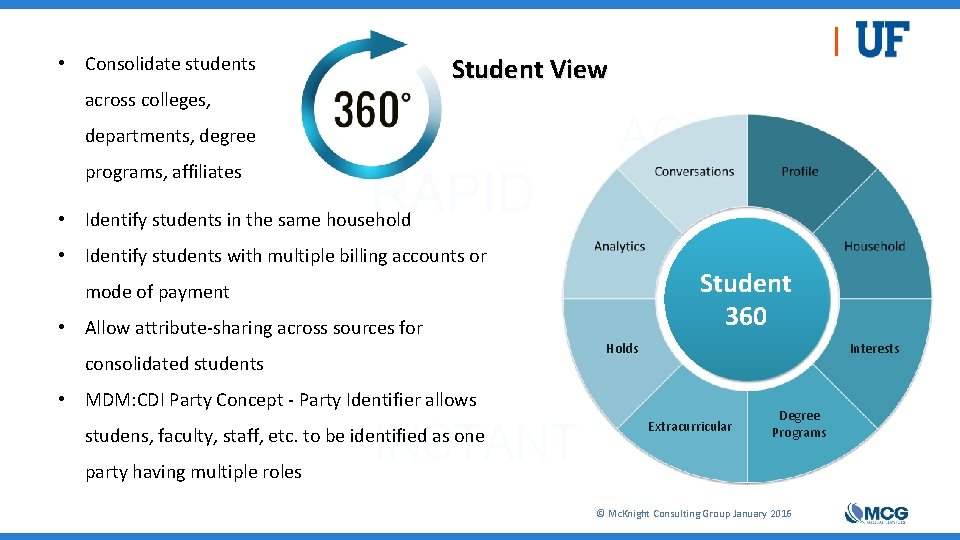
• Consolidate students Student View across colleges, ACCESS departments, degree programs, affiliates RAPID • Identify students in the same household • Identify students with multiple billing accounts or Student 360 mode of payment • Allow attribute-sharing across sources for Holds consolidated students Interests • MDM: CDI Party Concept - Party Identifier allows INSTANT studens, faculty, staff, etc. to be identified as one party having multiple roles Extracurricular Degree Programs © Mc. Knight Consulting Group January 2016
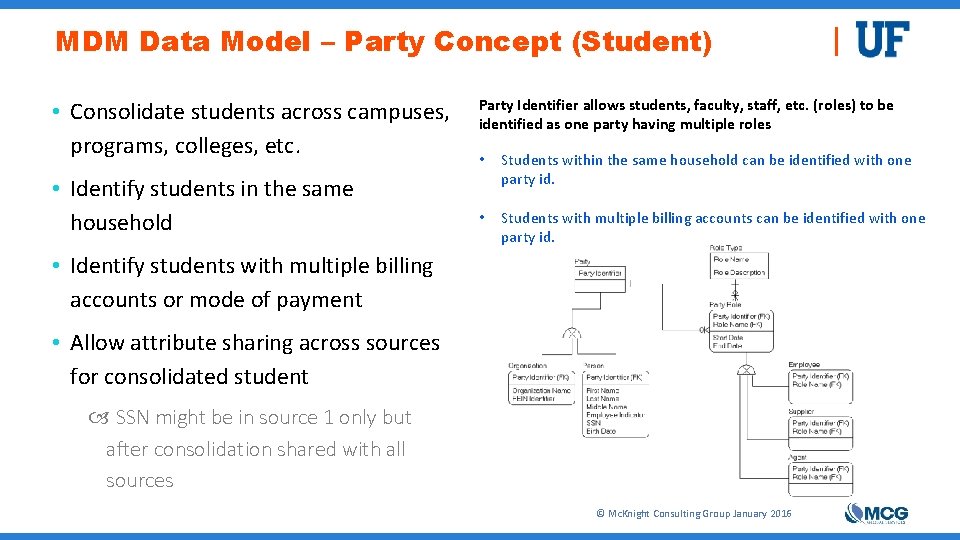
MDM Data Model – Party Concept (Student) • Consolidate students across campuses, programs, colleges, etc. • Identify students in the same household Party Identifier allows students, faculty, staff, etc. (roles) to be identified as one party having multiple roles • Students within the same household can be identified with one party id. • Students with multiple billing accounts can be identified with one party id. • Identify students with multiple billing accounts or mode of payment • Allow attribute sharing across sources for consolidated student SSN might be in source 1 only but after consolidation shared with all sources © Mc. Knight Consulting Group January 2016
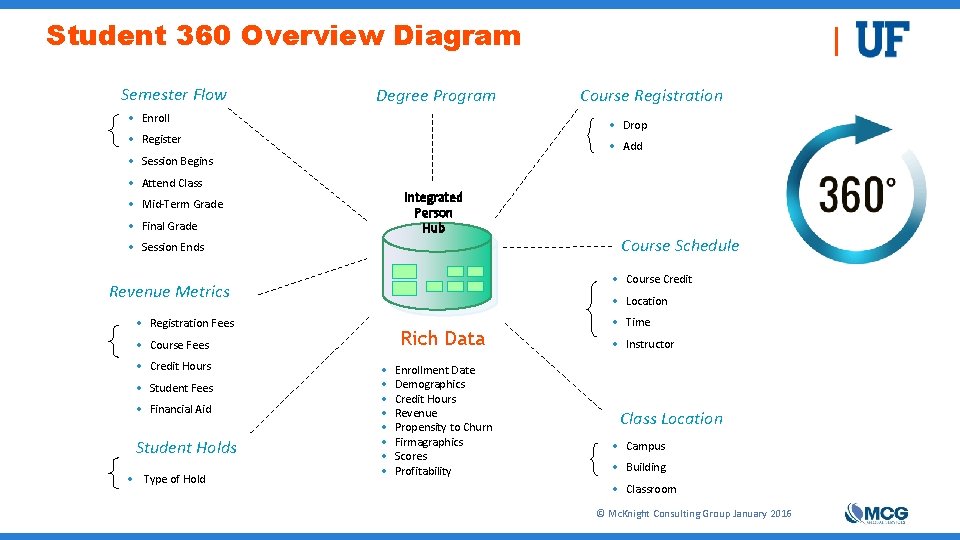
Student 360 Overview Diagram Semester Flow Degree Program • Enroll • Drop • Register • Add • Session Begins • Attend Class Integrated Person Hub • Mid-Term Grade • Final Grade • Session Ends • Location • Registration Fees Rich Data • Course Fees • Student Fees • Financial Aid Student Holds • Type of Hold Course Schedule • Course Credit Revenue Metrics • Credit Hours Course Registration • • Enrollment Date Demographics Credit Hours Revenue Propensity to Churn Firmagraphics Scores Profitability • Time • Instructor Class Location • Campus • Building • Classroom © Mc. Knight Consulting Group January 2016
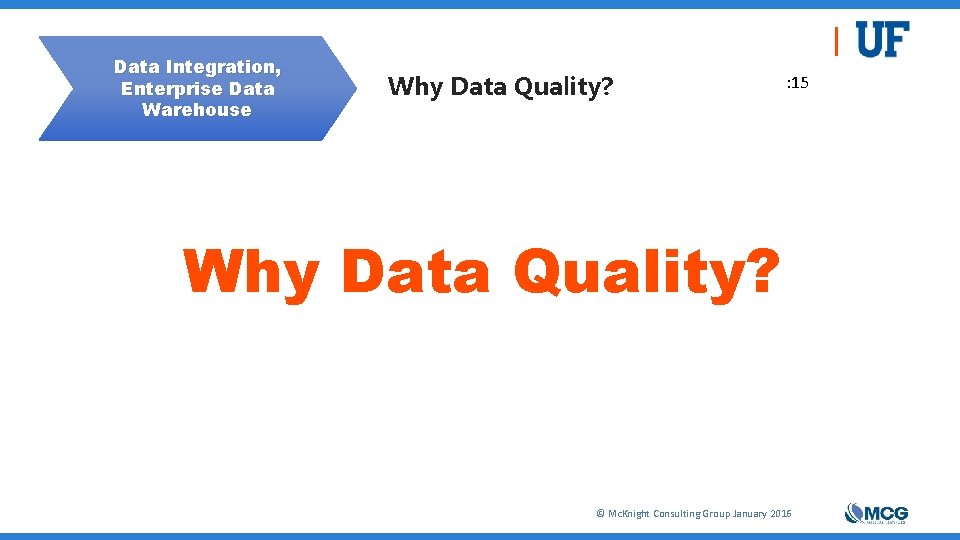
Data Integration, Enterprise Data Warehouse Why Data Quality? : 15 Why Data Quality? © Mc. Knight Consulting Group January 2016
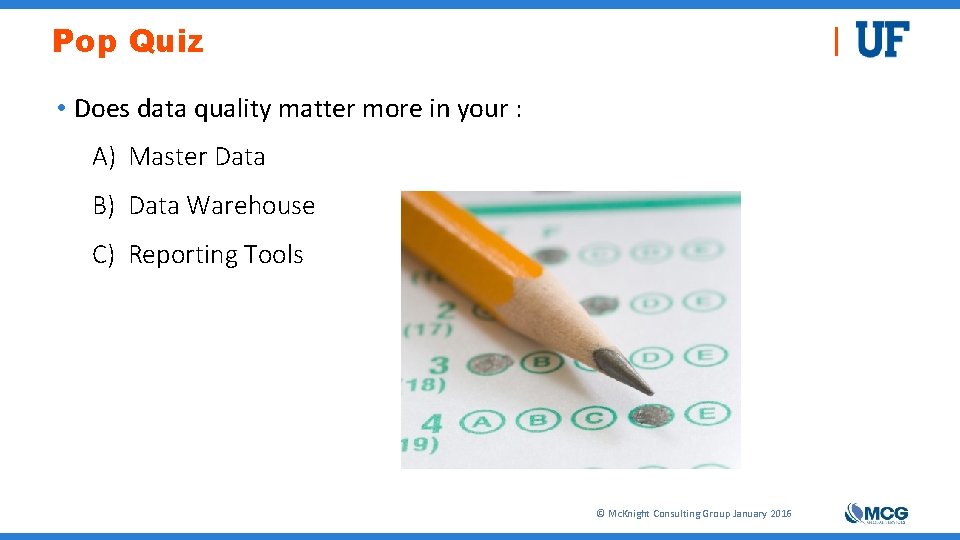
Pop Quiz • Does data quality matter more in your : A) Master Data B) Data Warehouse C) Reporting Tools © Mc. Knight Consulting Group January 2016
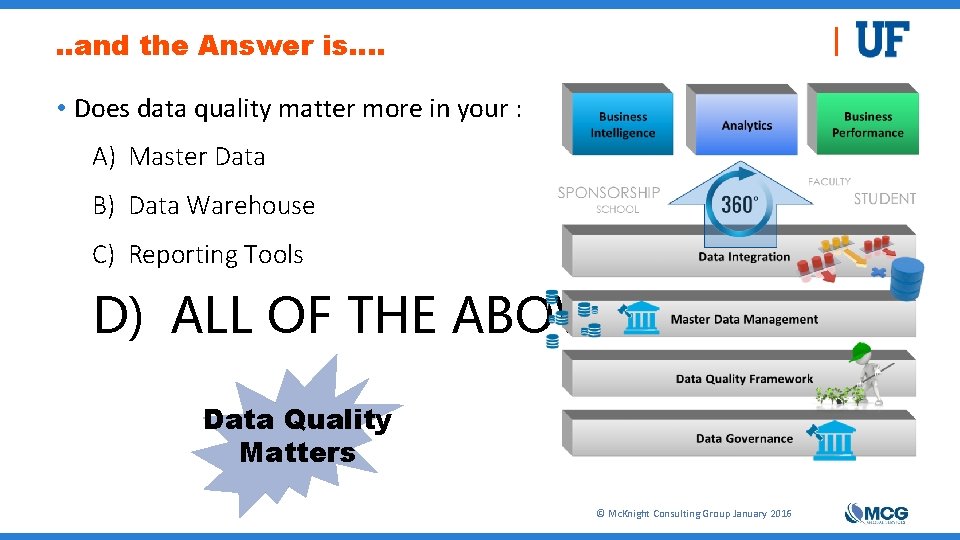
. . and the Answer is…. • Does data quality matter more in your : A) Master Data B) Data Warehouse C) Reporting Tools D) ALL OF THE ABOVE Data Quality Matters © Mc. Knight Consulting Group January 2016
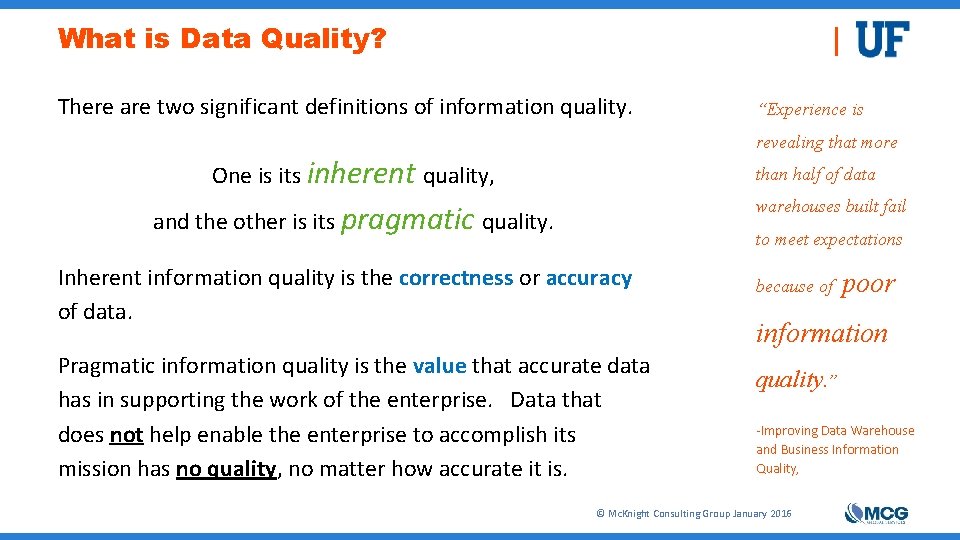
What is Data Quality? There are two significant definitions of information quality. “Experience is revealing that more One is its inherent quality, than half of data warehouses built fail and the other is its pragmatic quality. to meet expectations Inherent information quality is the correctness or accuracy of data. Pragmatic information quality is the value that accurate data has in supporting the work of the enterprise. Data that does not help enable the enterprise to accomplish its mission has no quality, no matter how accurate it is. because of poor information quality. ” -Improving Data Warehouse and Business Information Quality, © Mc. Knight Consulting Group January 2016
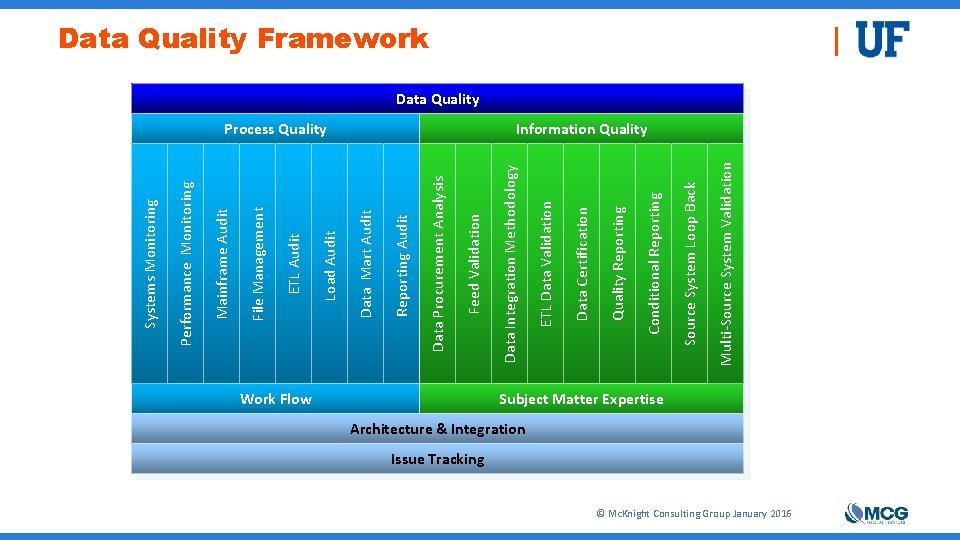
Work Flow Multi-Source System Validation Source System Loop Back Conditional Reporting Quality Reporting Data Certification ETL Data Validation Process Quality Data Integration Methodology Feed Validation Data Procurement Analysis Reporting Audit Data Mart Audit Load Audit ETL Audit File Management Mainframe Audit Performance Monitoring Systems Monitoring Data Quality Framework Data Quality Information Quality Subject Matter Expertise Architecture & Integration Issue Tracking © Mc. Knight Consulting Group January 2016
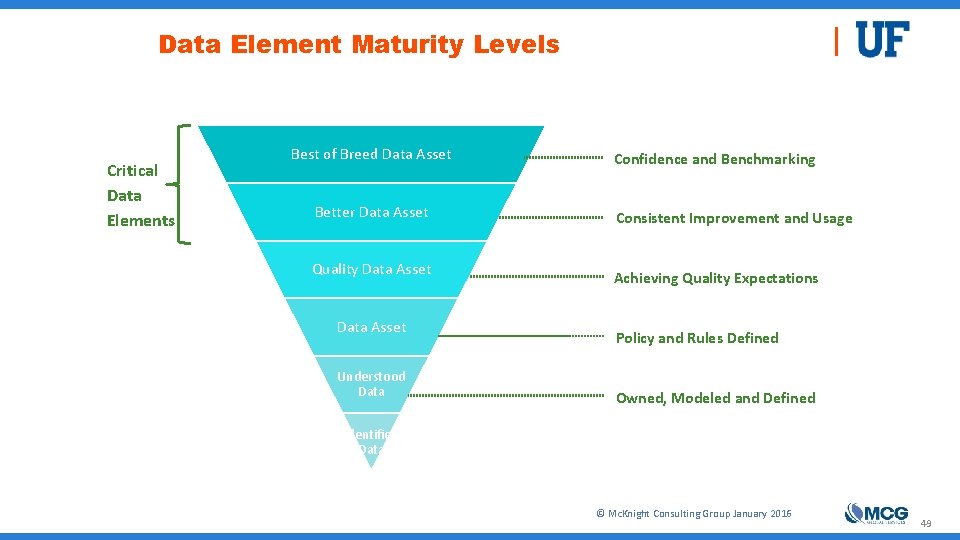
Data Element Maturity Levels Critical Data Elements Best of Breed Data Asset Better Data Asset Quality Data Asset Understood Data Confidence and Benchmarking Consistent Improvement and Usage Achieving Quality Expectations Policy and Rules Defined Owned, Modeled and Defined Identified Data © Mc. Knight Consulting Group January 2016 49
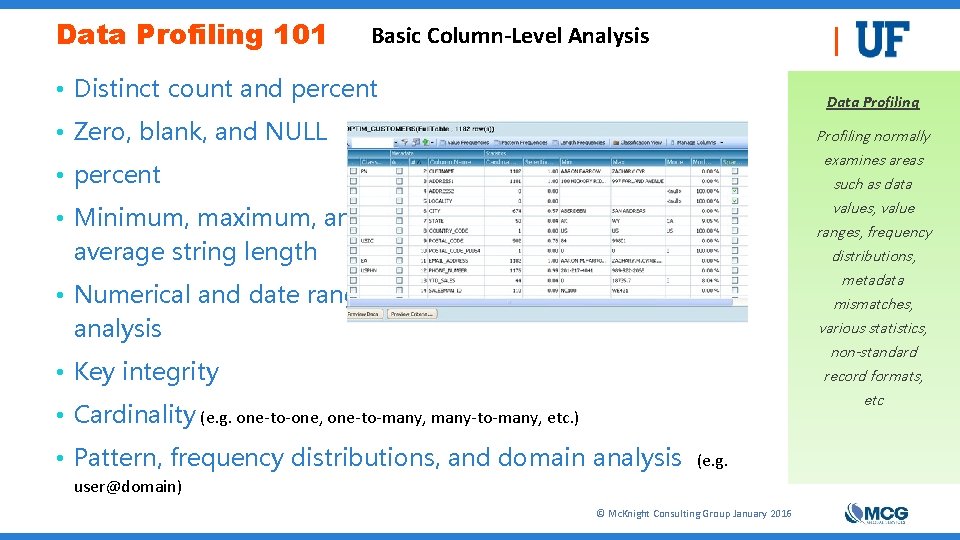
Data Profiling 101 Basic Column-Level Analysis • Distinct count and percent Data Profiling • Zero, blank, and NULL Profiling normally examines areas such as data values, value ranges, frequency distributions, metadata mismatches, various statistics, non-standard record formats, etc • percent • Minimum, maximum, and average string length • Numerical and date range analysis • Key integrity • Cardinality (e. g. one-to-one, one-to-many, many-to-many, etc. ) • Pattern, frequency distributions, and domain analysis (e. g. user@domain) © Mc. Knight Consulting Group January 2016
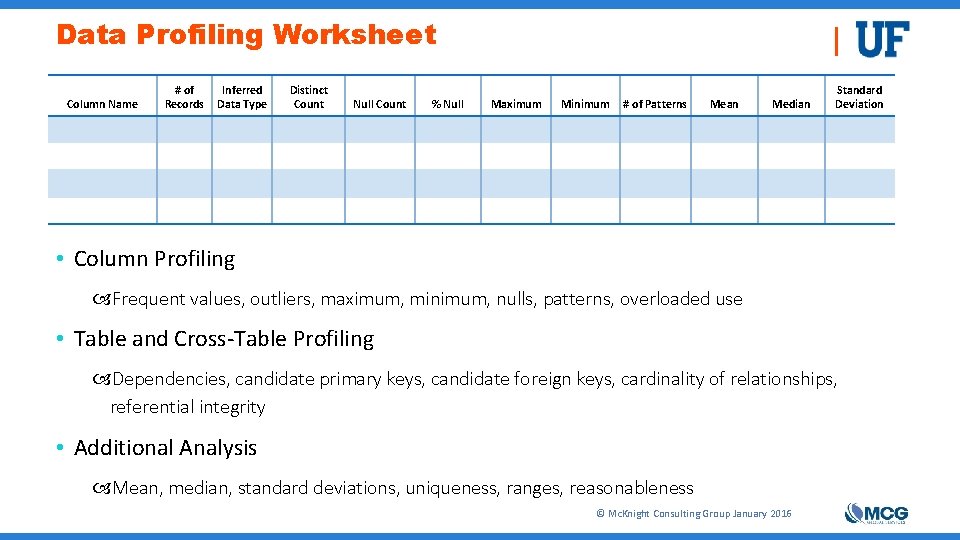
Data Profiling Worksheet Column Name # of Records Inferred Data Type Distinct Count Null Count % Null Maximum Minimum # of Patterns Mean Median Standard Deviation • Column Profiling Frequent values, outliers, maximum, minimum, nulls, patterns, overloaded use • Table and Cross-Table Profiling Dependencies, candidate primary keys, candidate foreign keys, cardinality of relationships, referential integrity • Additional Analysis Mean, median, standard deviations, uniqueness, ranges, reasonableness © Mc. Knight Consulting Group January 2016
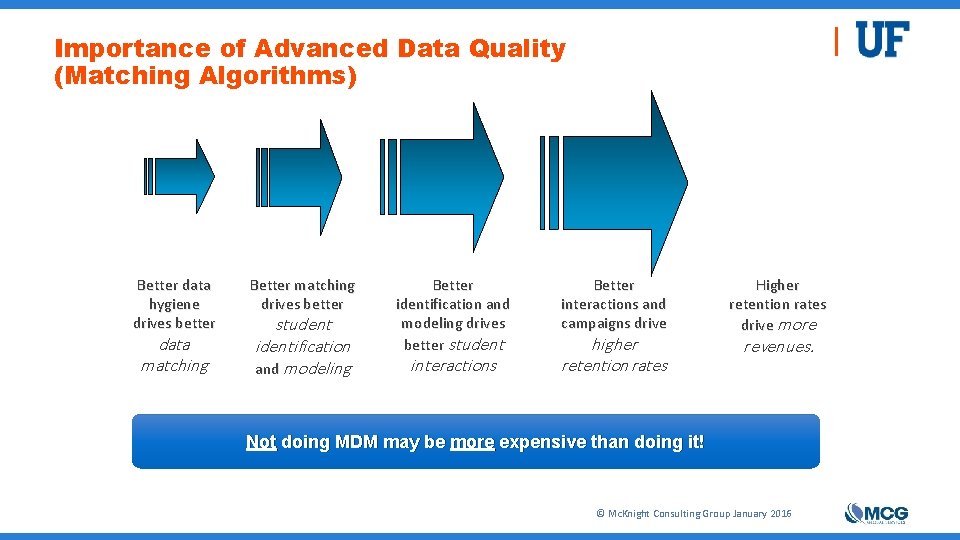
Importance of Advanced Data Quality (Matching Algorithms) Better data hygiene drives better data matching Better matching drives better student identification and modeling Better identification and modeling drives better student interactions Better interactions and campaigns drive higher retention rates Higher retention rates drive more revenues. Not doing MDM may be more expensive than doing it! © Mc. Knight Consulting Group January 2016
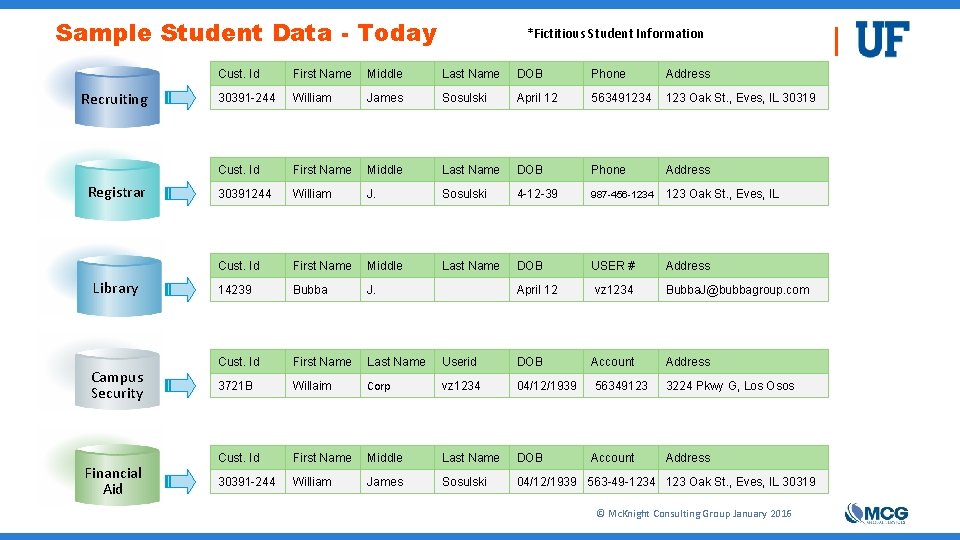
Sample Student Data - Today Recruiting Registrar Library Campus Security Financial Aid *Fictitious Student Information Cust. Id First Name Middle Last Name DOB Phone Address 30391 -244 William James Sosulski April 12 563491234 123 Oak St. , Eves, IL 30319 Cust. Id First Name Middle Last Name DOB Phone Address 30391244 William J. Sosulski 4 -12 -39 987 -456 -1234 123 Oak St. , Eves, IL Cust. Id First Name Middle Last Name DOB USER # Address 14239 Bubba J. April 12 vz 1234 Bubba. J@bubbagroup. com Cust. Id First Name Last Name Userid DOB Account Address 3721 B Willaim Corp vz 1234 04/12/1939 56349123 3224 Pkwy G, Los Osos Cust. Id First Name Middle Last Name DOB Account Address 30391 -244 William James Sosulski 04/12/1939 563 -49 -1234 123 Oak St. , Eves, IL 30319 © Mc. Knight Consulting Group January 2016
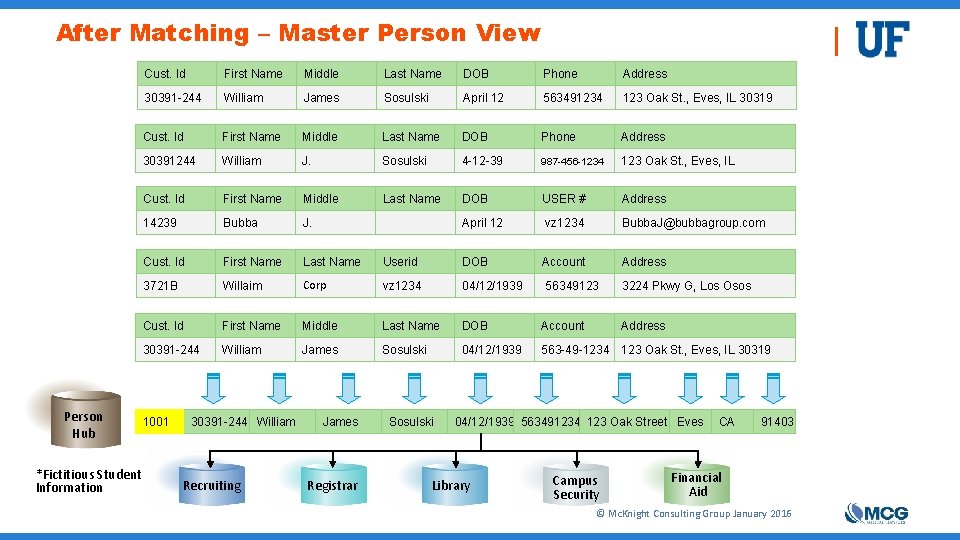
After Matching – Master Person View Person Hub *Fictitious Student Information Cust. Id First Name Middle Last Name DOB Phone Address 30391 -244 William James Sosulski April 12 563491234 123 Oak St. , Eves, IL 30319 Cust. Id First Name Middle Last Name DOB Phone Address 30391244 William J. Sosulski 4 -12 -39 987 -456 -1234 123 Oak St. , Eves, IL Cust. Id First Name Middle Last Name DOB USER # Address 14239 Bubba J. April 12 vz 1234 Bubba. J@bubbagroup. com Cust. Id First Name Last Name Userid DOB Account Address 3721 B Willaim Corp vz 1234 04/12/1939 56349123 3224 Pkwy G, Los Osos Cust. Id First Name Middle Last Name DOB Account 30391 -244 William James Sosulski 04/12/1939 563 -49 -1234 123 Oak St. , Eves, IL 30319 1001 30391 -244 William Recruiting James Registrar Sosulski Address 04/12/1939 563491234 123 Oak Street Eves Library Campus Security CA 91403 Financial Aid © Mc. Knight Consulting Group January 2016
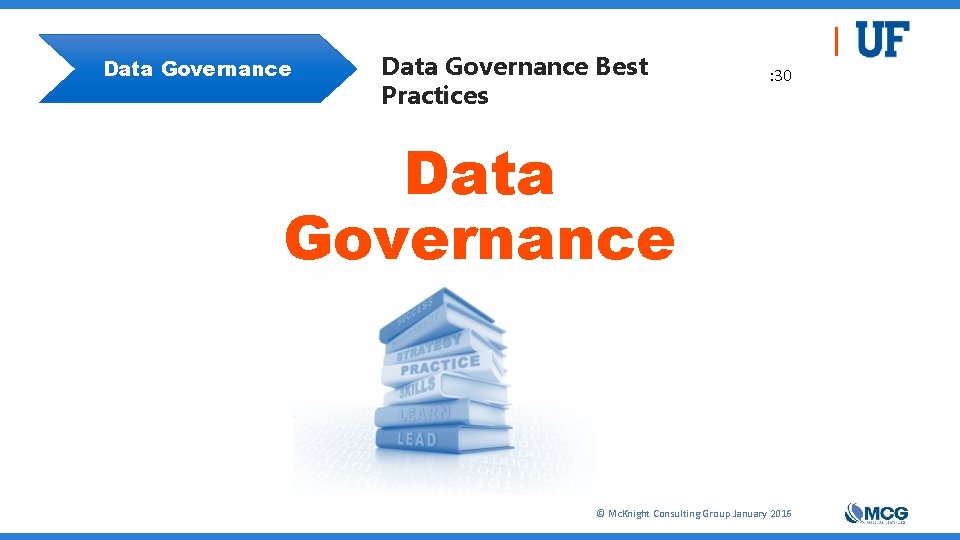
Data Governance Best Practices : 30 Data Governance © Mc. Knight Consulting Group January 2016
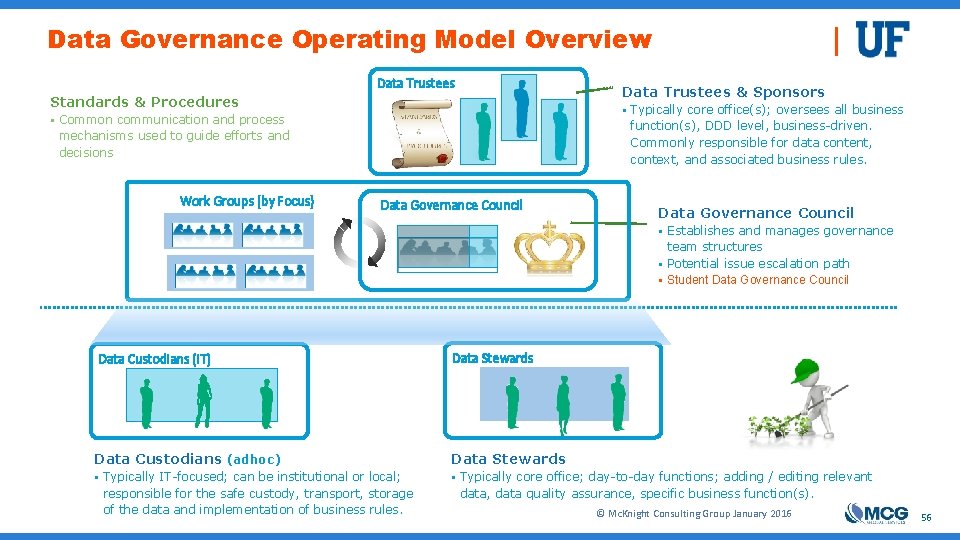
Data Governance Operating Model Overview Data Trustees & Sponsors Standards & Procedures § Typically core office(s); oversees all business § Common communication and process function(s), DDD level, business-driven. Commonly responsible for data content, context, and associated business rules. mechanisms used to guide efforts and decisions Work Groups (by Focus) Data Governance Council § Establishes and manages governance team structures § Potential issue escalation path § Student Data Governance Council Data Custodians (IT) Data Stewards Data Custodians (adhoc) Data Stewards § Typically IT-focused; can be institutional or local; § Typically core office; day-to-day functions; adding / editing relevant responsible for the safe custody, transport, storage of the data and implementation of business rules. data, data quality assurance, specific business function(s). © Mc. Knight Consulting Group January 2016 56
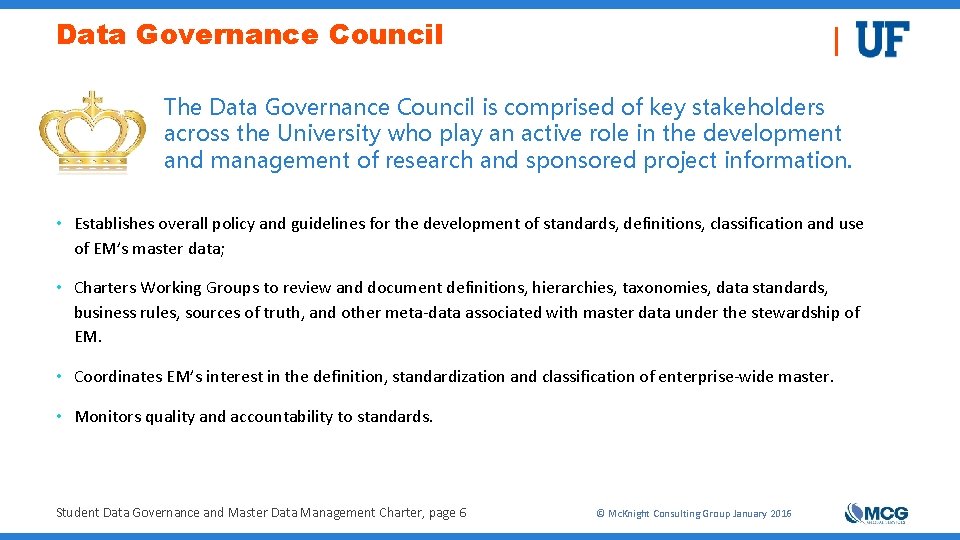
Data Governance Council The Data Governance Council is comprised of key stakeholders across the University who play an active role in the development and management of research and sponsored project information. • Establishes overall policy and guidelines for the development of standards, definitions, classification and use of EM’s master data; • Charters Working Groups to review and document definitions, hierarchies, taxonomies, data standards, business rules, sources of truth, and other meta-data associated with master data under the stewardship of EM. • Coordinates EM’s interest in the definition, standardization and classification of enterprise-wide master. • Monitors quality and accountability to standards. Student Data Governance and Master Data Management Charter, page 6 © Mc. Knight Consulting Group January 2016
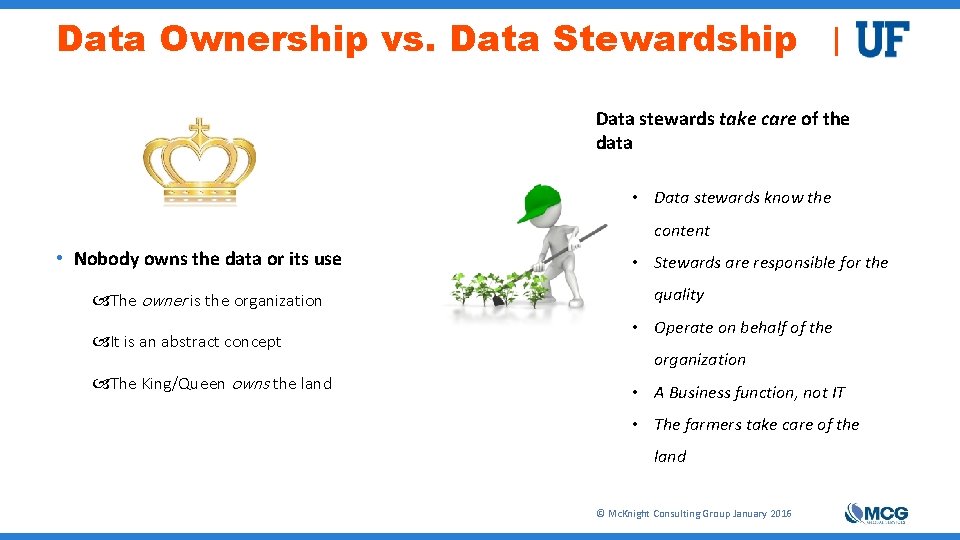
Data Ownership vs. Data Stewardship Data stewards take care of the data • Data stewards know the content • Nobody owns the data or its use The owner is the organization It is an abstract concept The King/Queen owns the land • Stewards are responsible for the quality • Operate on behalf of the organization • A Business function, not IT • The farmers take care of the land © Mc. Knight Consulting Group January 2016
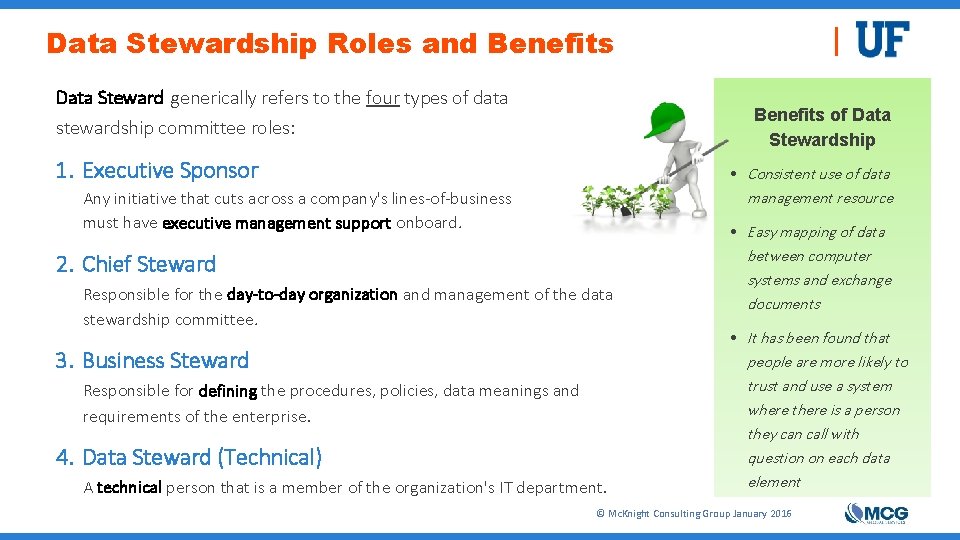
Data Stewardship Roles and Benefits Data Steward generically refers to the four types of data stewardship committee roles: Benefits of Data Stewardship 1. Executive Sponsor • Consistent use of data management resource Any initiative that cuts across a company's lines-of-business must have executive management support onboard. 2. Chief Steward Responsible for the day-to-day organization and management of the data stewardship committee. 3. Business Steward Responsible for defining the procedures, policies, data meanings and requirements of the enterprise. 4. Data Steward (Technical) A technical person that is a member of the organization's IT department. • Easy mapping of data between computer systems and exchange documents • It has been found that people are more likely to trust and use a system where there is a person they can call with question on each data element © Mc. Knight Consulting Group January 2016
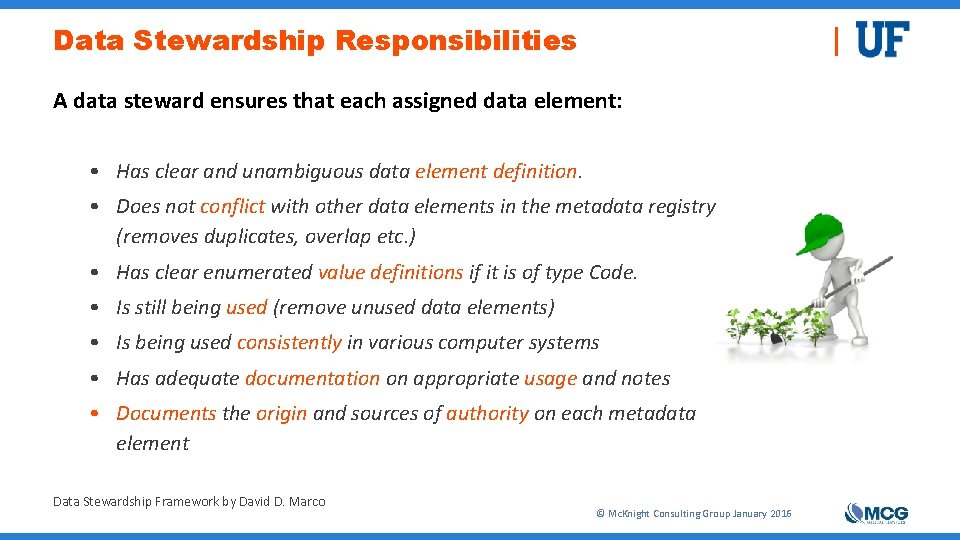
Data Stewardship Responsibilities A data steward ensures that each assigned data element: • Has clear and unambiguous data element definition. • Does not conflict with other data elements in the metadata registry (removes duplicates, overlap etc. ) • Has clear enumerated value definitions if it is of type Code. • Is still being used (remove unused data elements) • Is being used consistently in various computer systems • Has adequate documentation on appropriate usage and notes • Documents the origin and sources of authority on each metadata element Data Stewardship Framework by David D. Marco © Mc. Knight Consulting Group January 2016
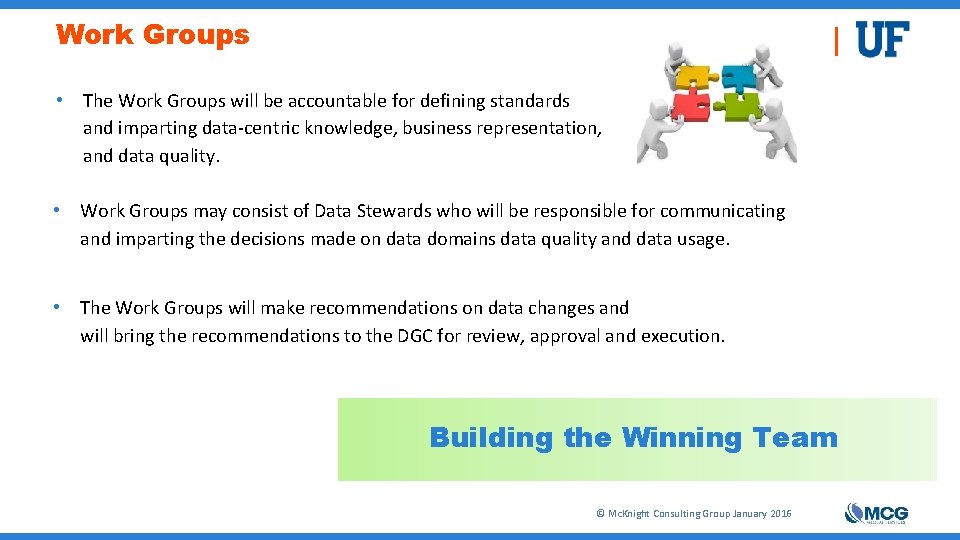
Work Groups • The Work Groups will be accountable for defining standards and imparting data-centric knowledge, business representation, and data quality. • Work Groups may consist of Data Stewards who will be responsible for communicating and imparting the decisions made on data domains data quality and data usage. • The Work Groups will make recommendations on data changes and will bring the recommendations to the DGC for review, approval and execution. Building the Winning Team © Mc. Knight Consulting Group January 2016
The secret to getting ahead is getting started
Management is an art of getting things done through people
Data management concepts
Warehouse profiling
Limitation as a student
Spokesperson profiling
E profilling
Typological profiling
Partner profiling
Google wide profiling
Nilai jabatan
Leadership profiling tools
David carpenter serial killer
Gather approach in counselling
Competency profiling
Ase profiling
What is bottom up offender profiling
Top down approach to offender profiling
Qnx memory usage
Brianmac scat test
Duplex fixture
What is character profiling
Performance profiling process
Compucentric
Ribosome profiling
Farmer profiling
Risk response matrix
Skus
Geographic profiling
Lighthouse profiling
Spontaneous generation in data flow diagram
Dfd chapter 5
Getting to si, ja, oui, hai, and da
Seal getting on and falling out
Getting on and falling out
My name means colored bodies
Marta and berta are getting ready for school
Eep mobile al
Value management workshop agenda
Data mining concepts and techniques slides
Data mining concepts and techniques
ñ
Data transmission concepts
Data mining concepts and techniques slides
Data mining concepts and techniques slides
Data strategy workshop
Data capturing workshop
Dmmsm
Time management concepts
Core concepts of management
Relational database management system concepts
Construction management concepts
Conversion cost formula
Business ethics and
Basic concepts of strategic management
Basic concepts of strategic management
Basic concept of strategic management
Middle level management
Basic concept of strategic management
Political management in strategic triangle
Financial management basic concepts
Why is not advisable to pursue too many strategies at once