Twitter is Faster Timeaware Video Recommendation from Twitter
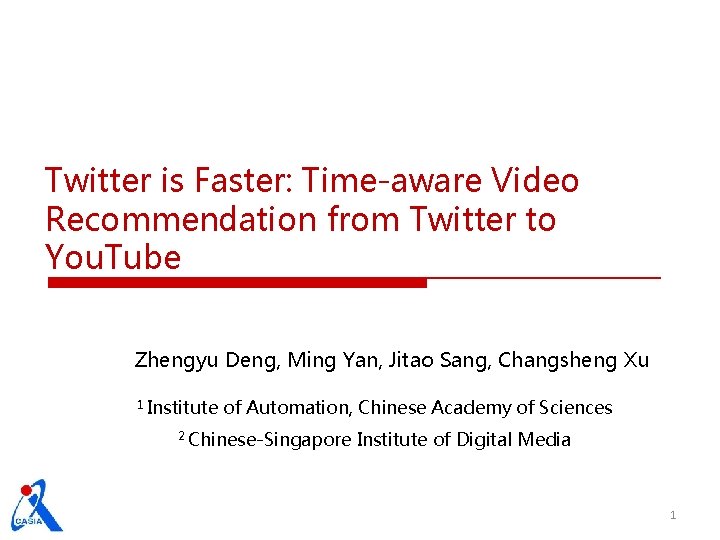
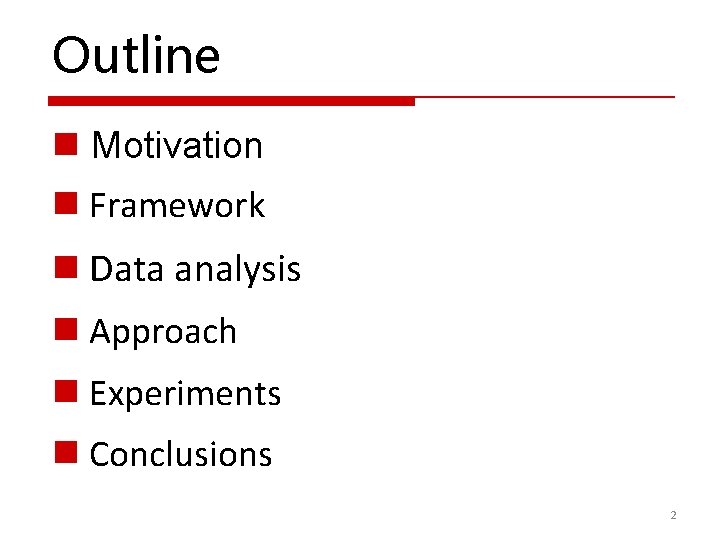
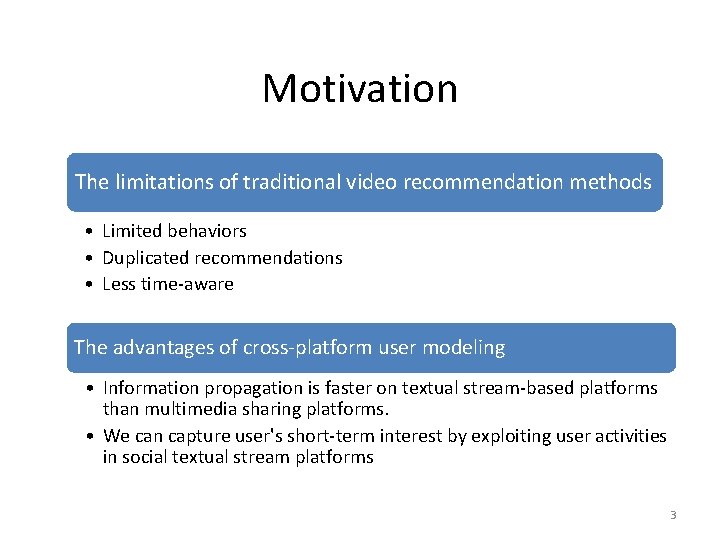
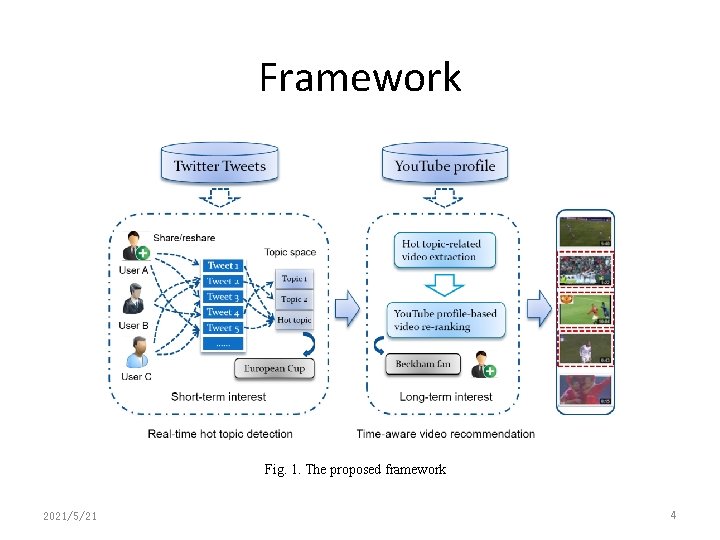
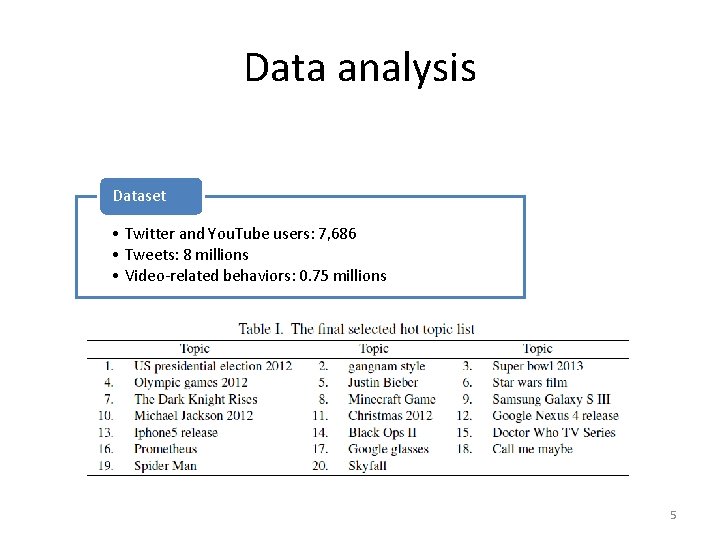
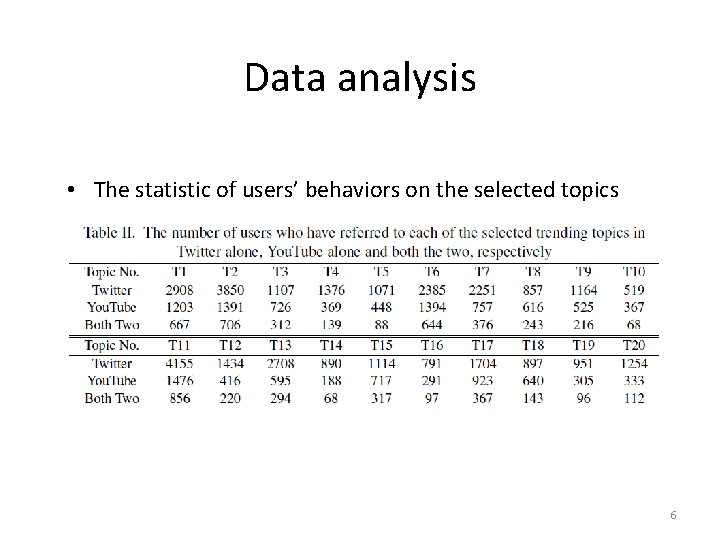
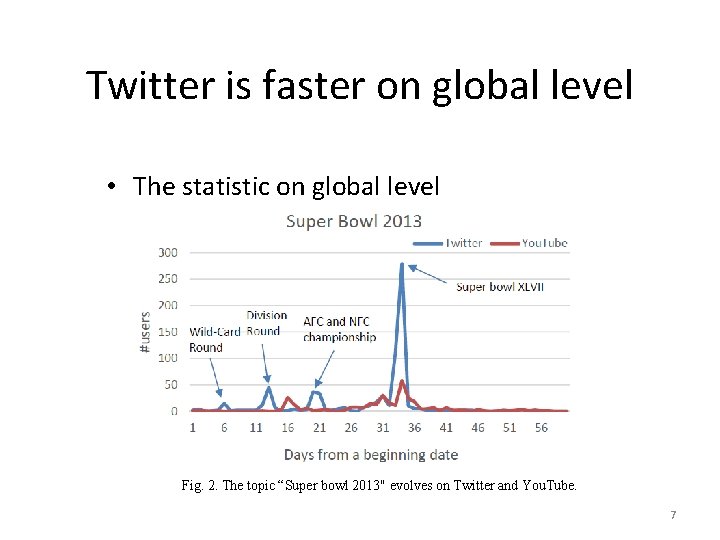
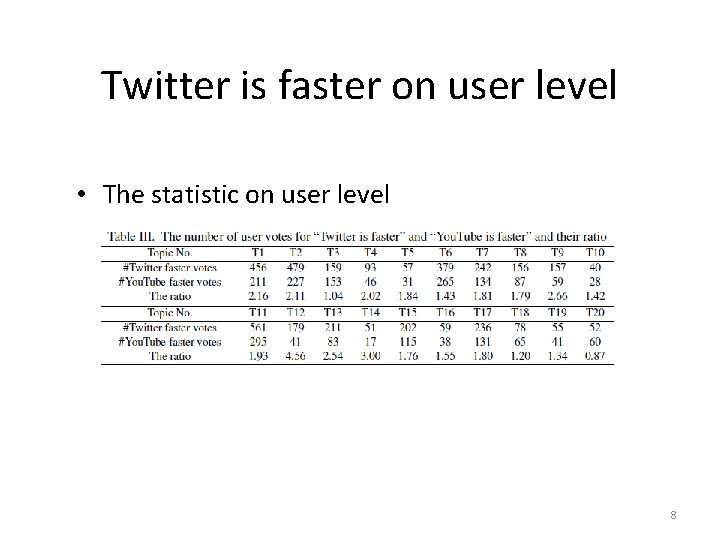
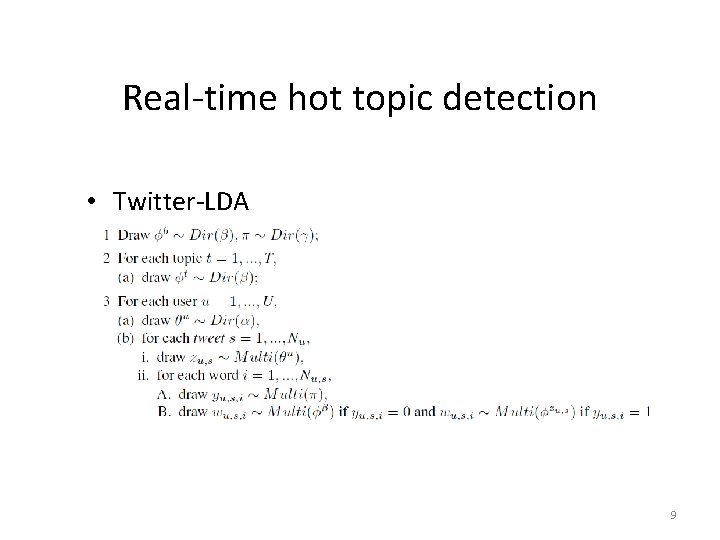
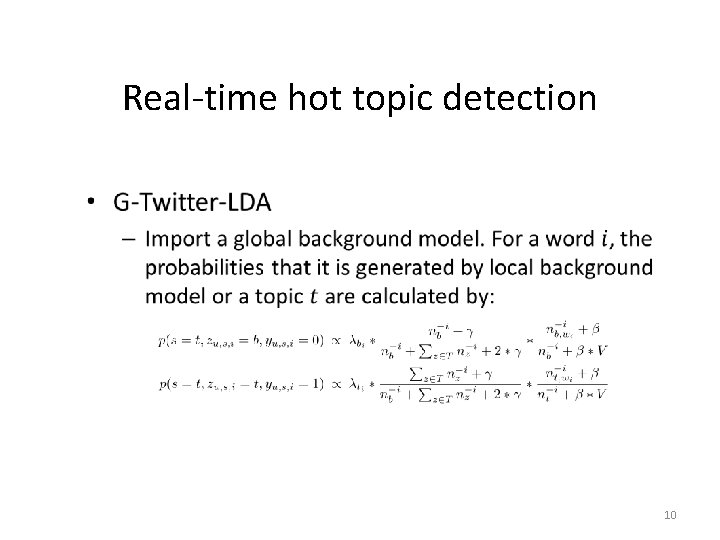
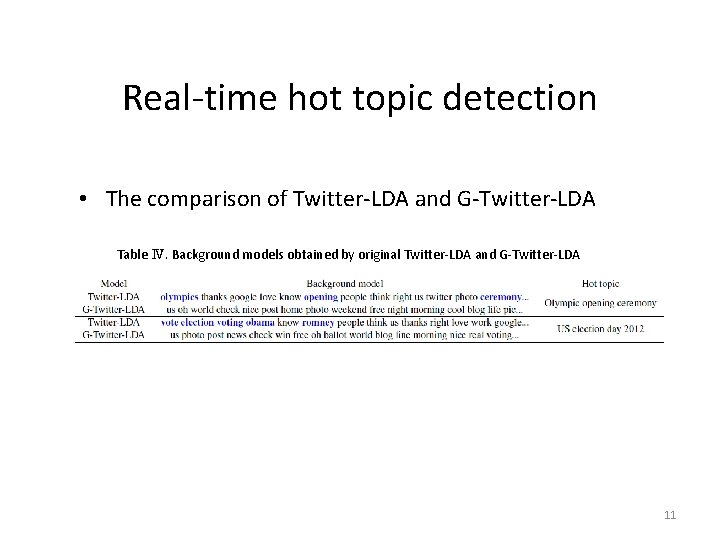
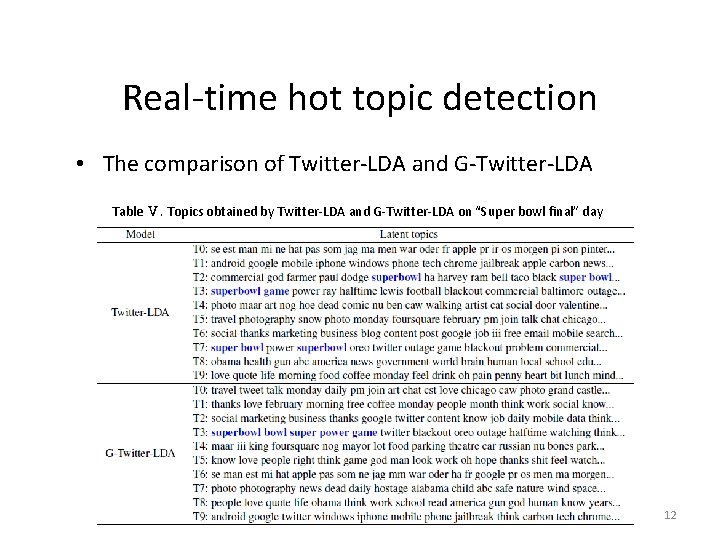
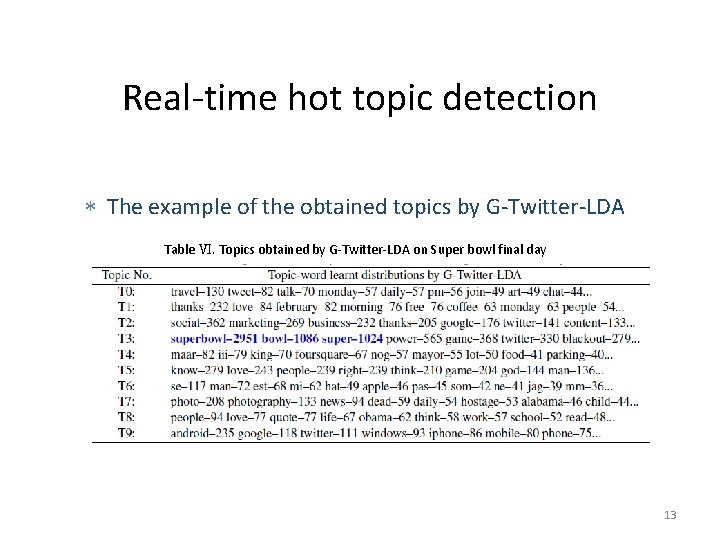
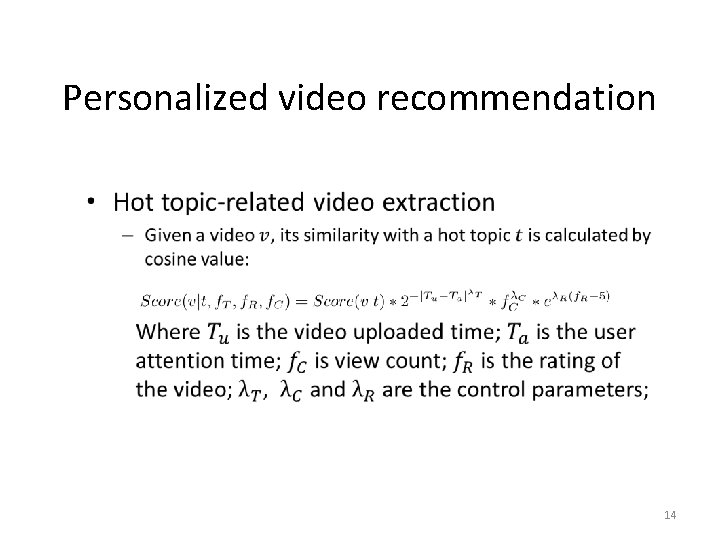
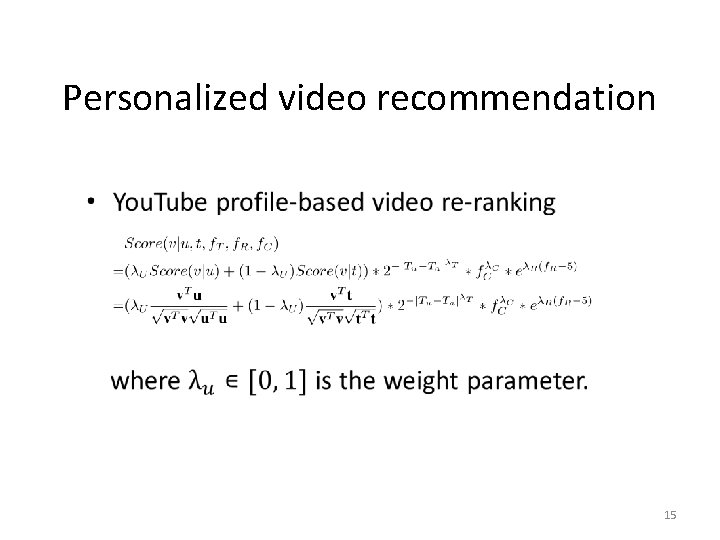
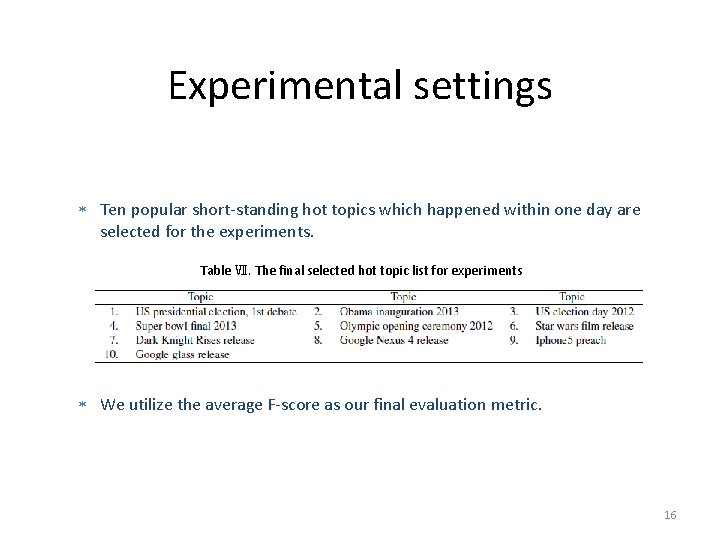
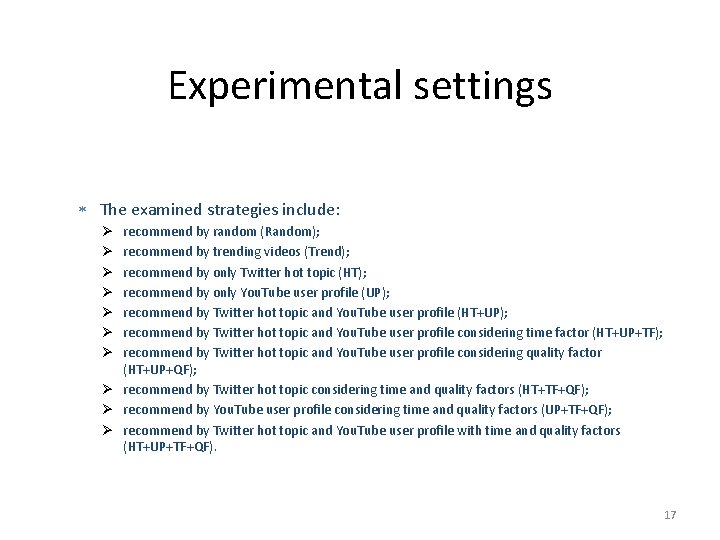
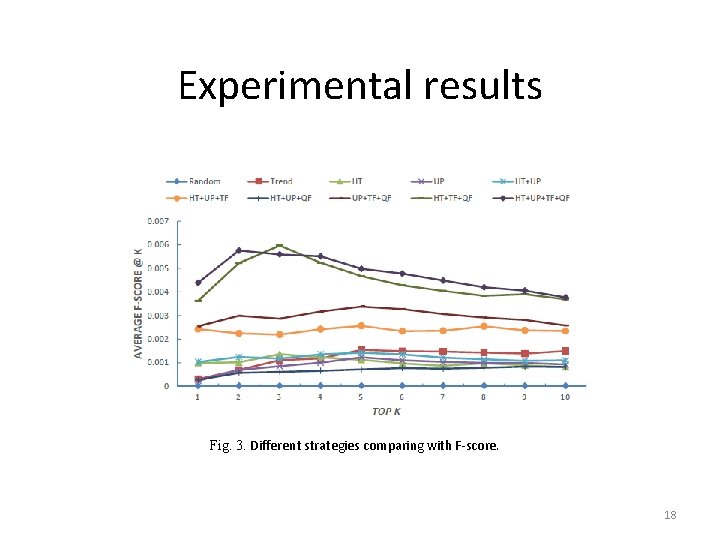
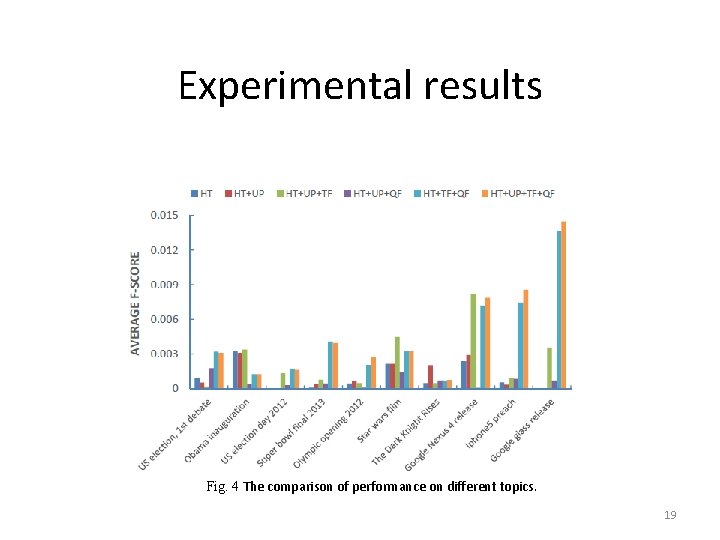
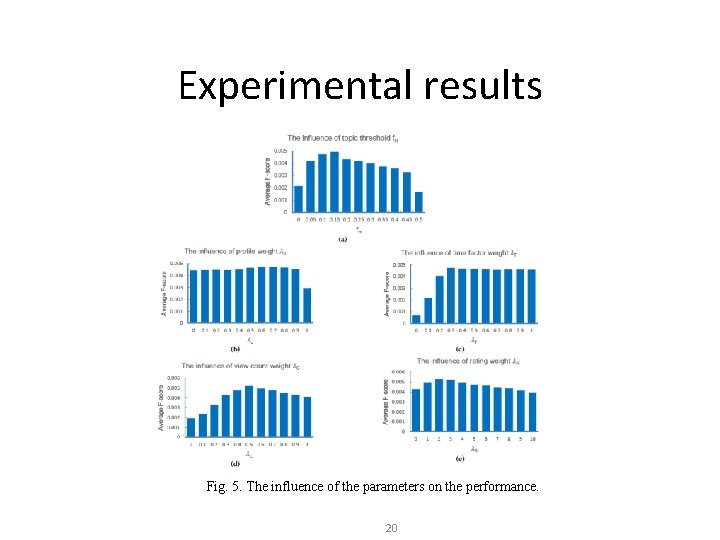
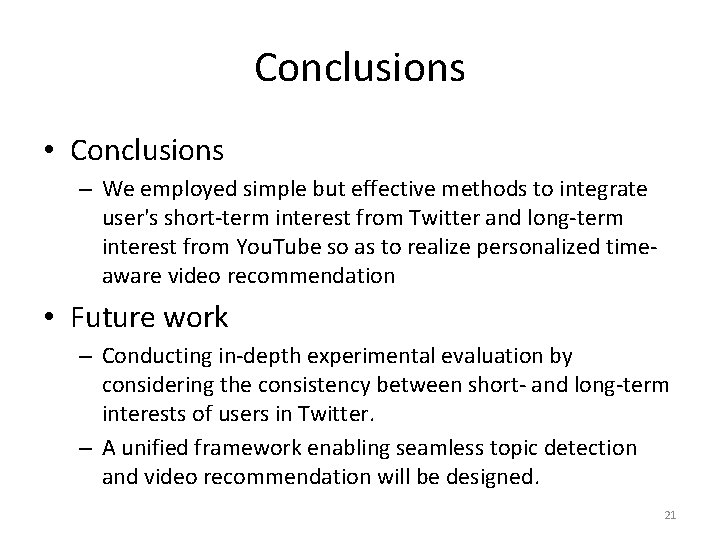
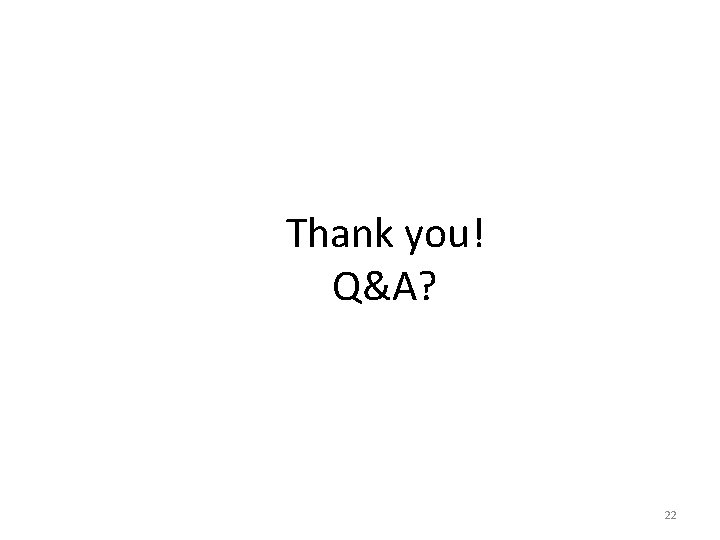
- Slides: 22
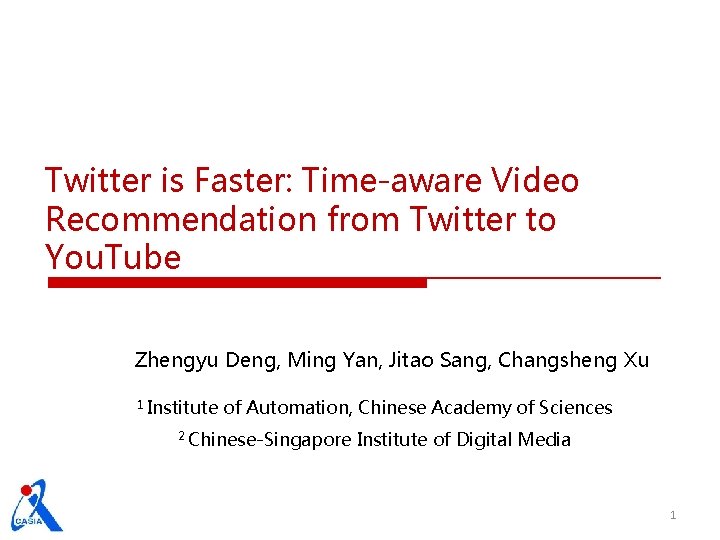
Twitter is Faster: Time-aware Video Recommendation from Twitter to You. Tube Zhengyu Deng, Ming Yan, Jitao Sang, Changsheng Xu 1 Institute of Automation, Chinese Academy of Sciences 2 Chinese-Singapore Institute of Digital Media 1
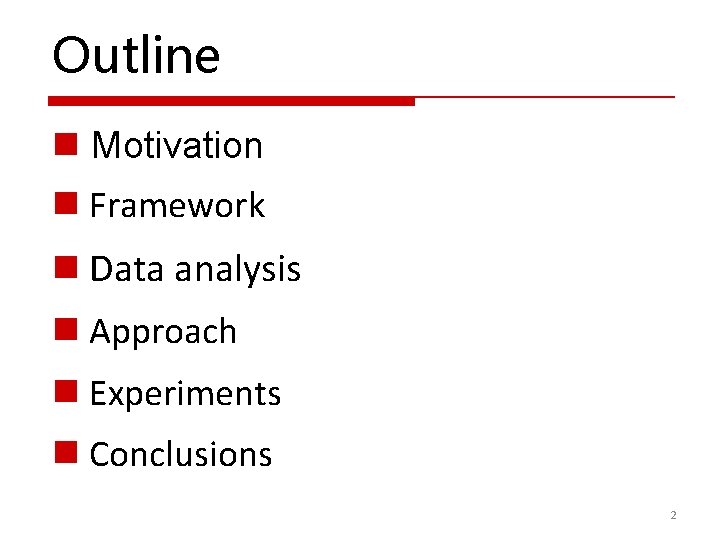
Outline n Motivation n Framework n Data analysis n Approach n Experiments n Conclusions 2
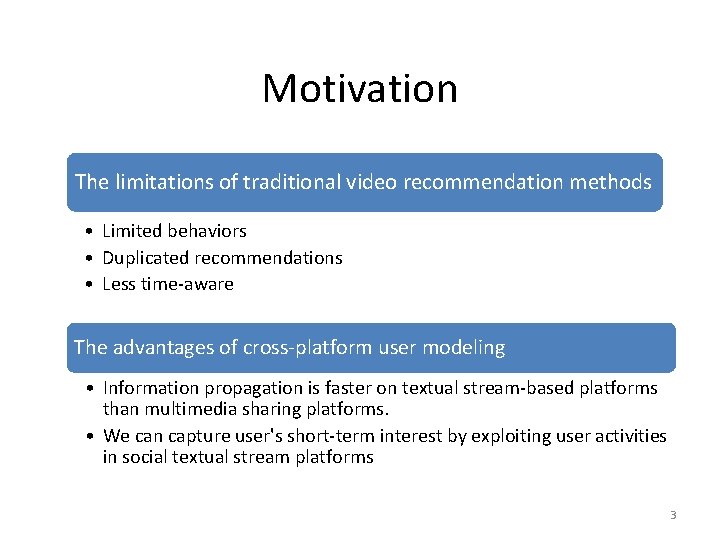
Motivation The limitations of traditional video recommendation methods • Limited behaviors • Duplicated recommendations • Less time-aware The advantages of cross-platform user modeling • Information propagation is faster on textual stream-based platforms than multimedia sharing platforms. • We can capture user's short-term interest by exploiting user activities in social textual stream platforms The limitations of traditional recommendation methods 3
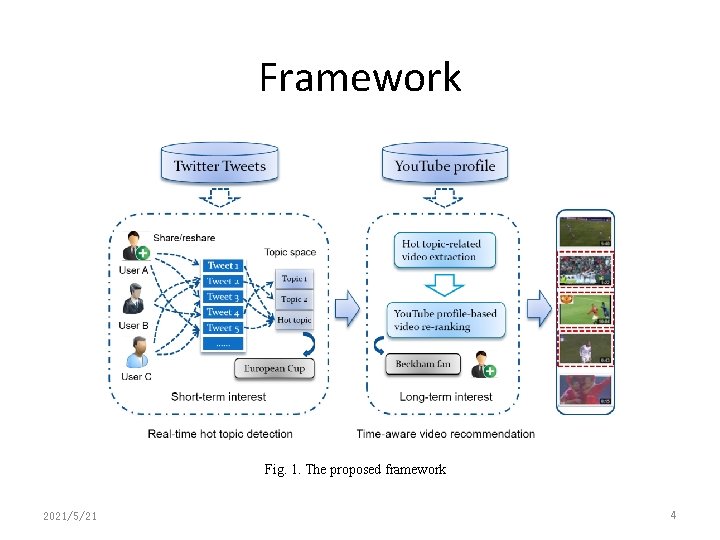
Framework Fig. 1. The proposed framework 2021/5/21 4
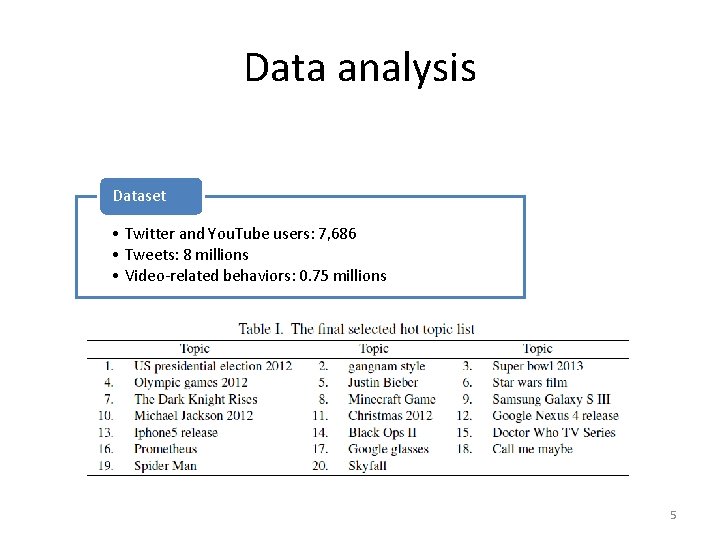
Data analysis Dataset • Twitter and You. Tube users: 7, 686 • Tweets: 8 millions • Video-related behaviors: 0. 75 millions 5
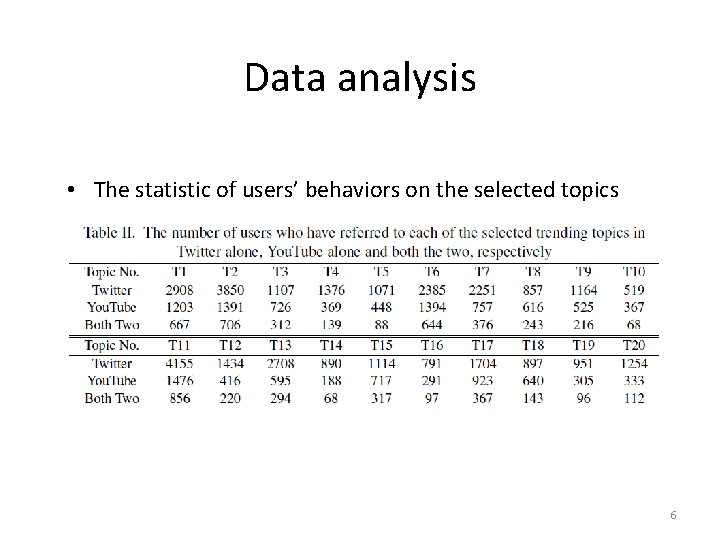
Data analysis • The statistic of users’ behaviors on the selected topics 6
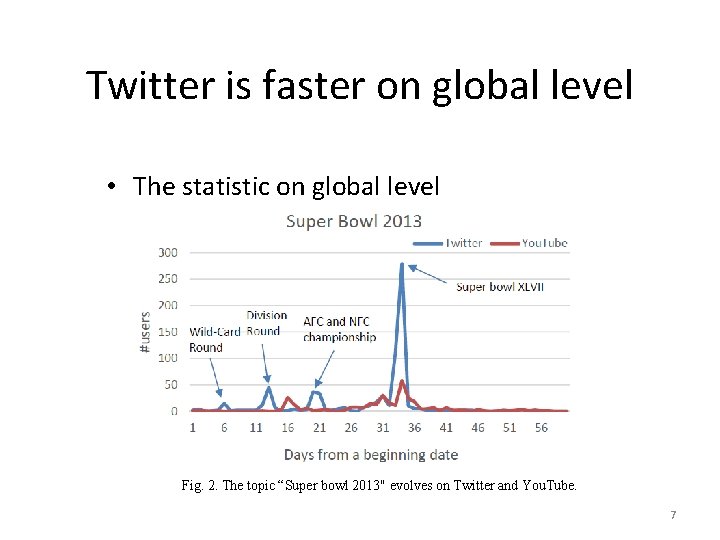
Data analysis Twitter is faster on global level • The statistic on global level Fig. 2. The topic “Super bowl 2013" evolves on Twitter and You. Tube. 7
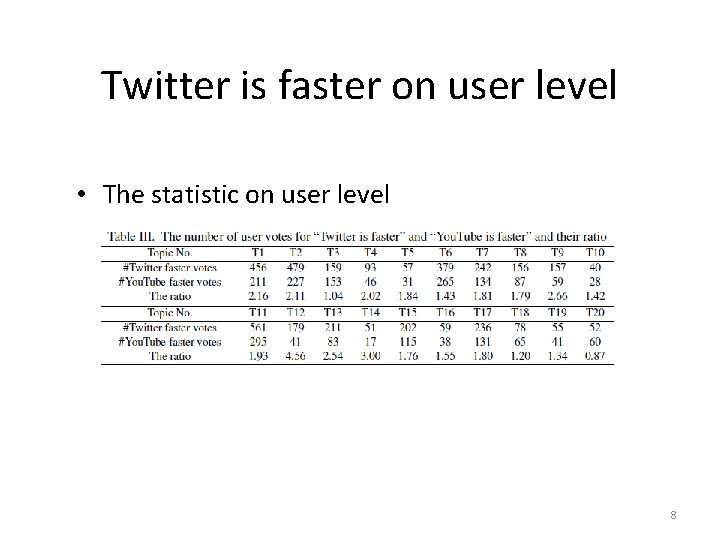
Data analysis Twitter is faster on user level • The statistic on user level 8
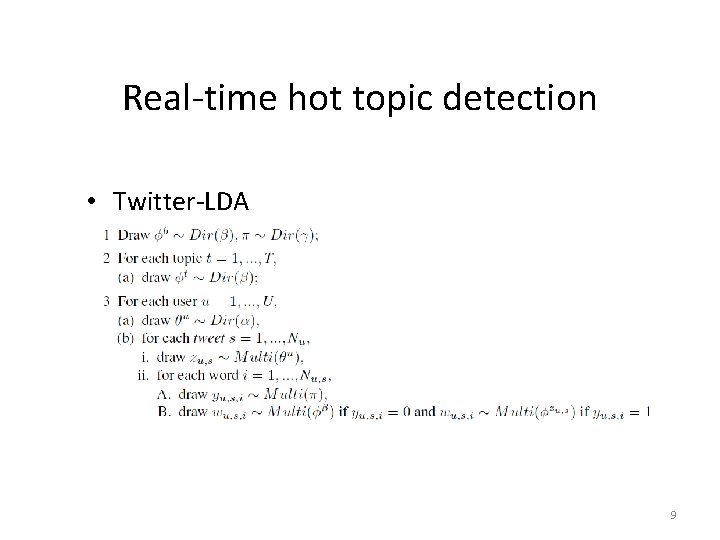
Approach Real-time hot topic detection • Twitter-LDA 9
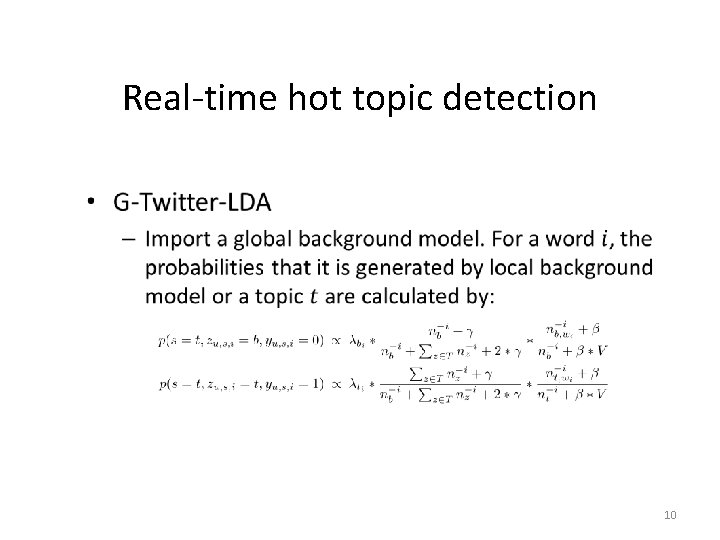
Approach Real-time hot topic detection • 10
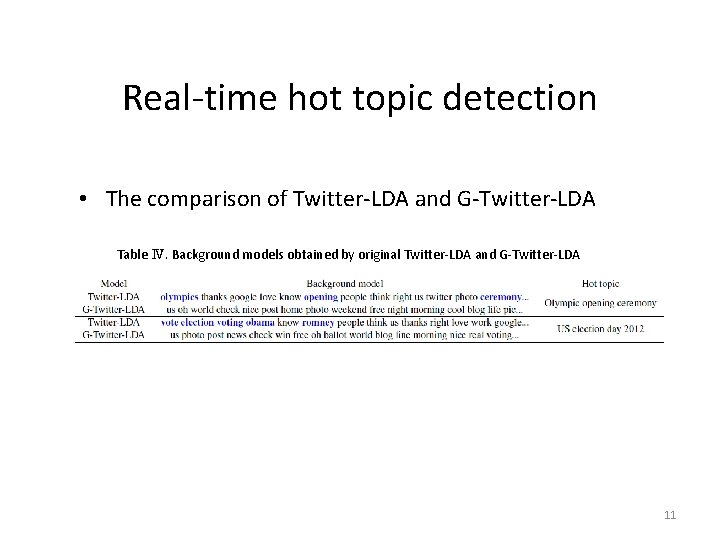
Approach Real-time hot topic detection • The comparison of Twitter-LDA and G-Twitter-LDA Table Ⅳ. Background models obtained by original Twitter-LDA and G-Twitter-LDA 11
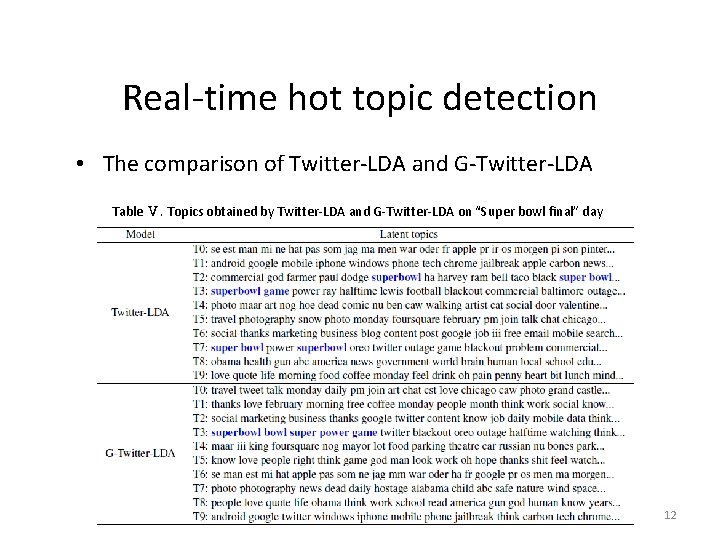
Approach Real-time hot topic detection • The comparison of Twitter-LDA and G-Twitter-LDA Table Ⅴ. Topics obtained by Twitter-LDA and G-Twitter-LDA on “Super bowl final” day 12
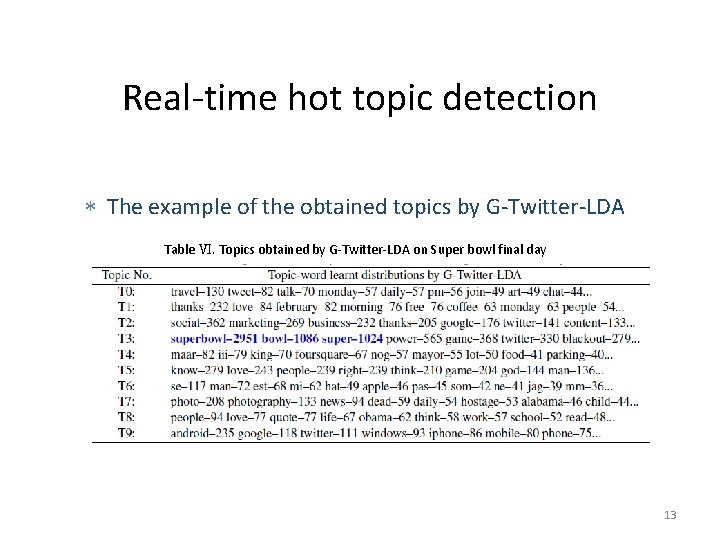
Approach Real-time hot topic detection The example of the obtained topics by G-Twitter-LDA Table Ⅵ. Topics obtained by G-Twitter-LDA on Super bowl final day 13
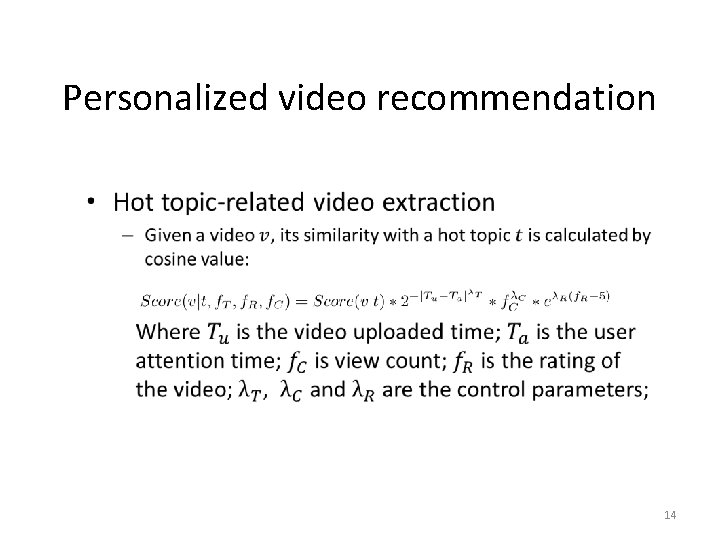
Approach Personalized video recommendation • 14
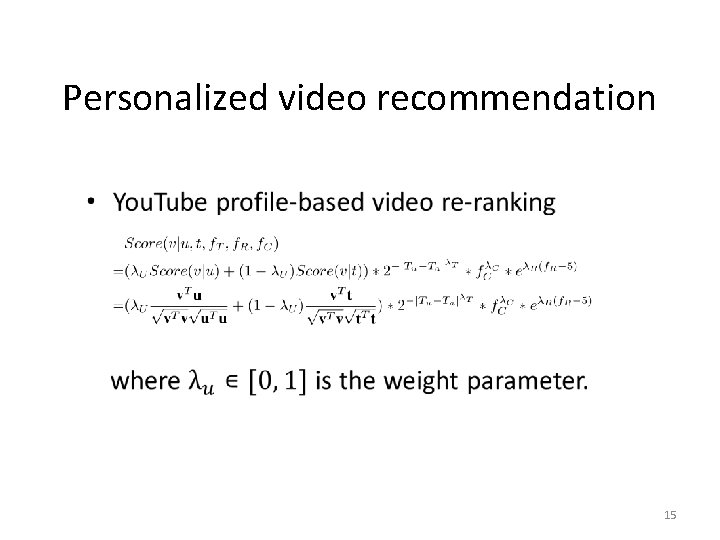
Approach Personalized video recommendation • 15
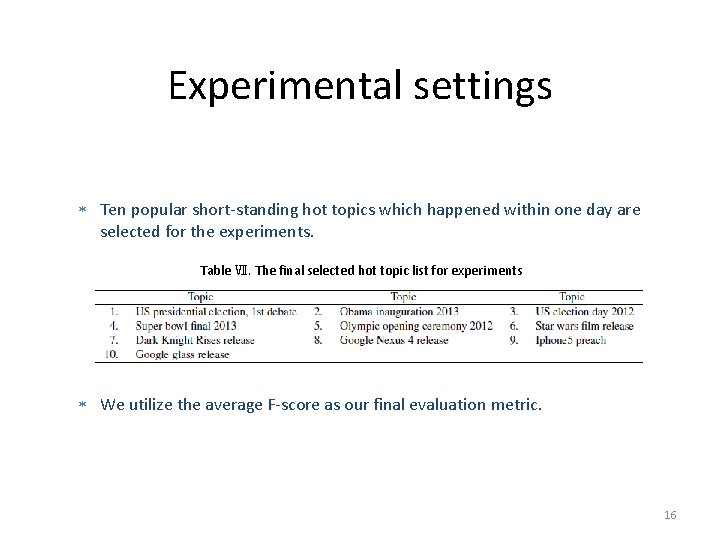
Experiments Experimental settings Ten popular short-standing hot topics which happened within one day are selected for the experiments. Table Ⅶ. The final selected hot topic list for experiments We utilize the average F-score as our final evaluation metric. 16
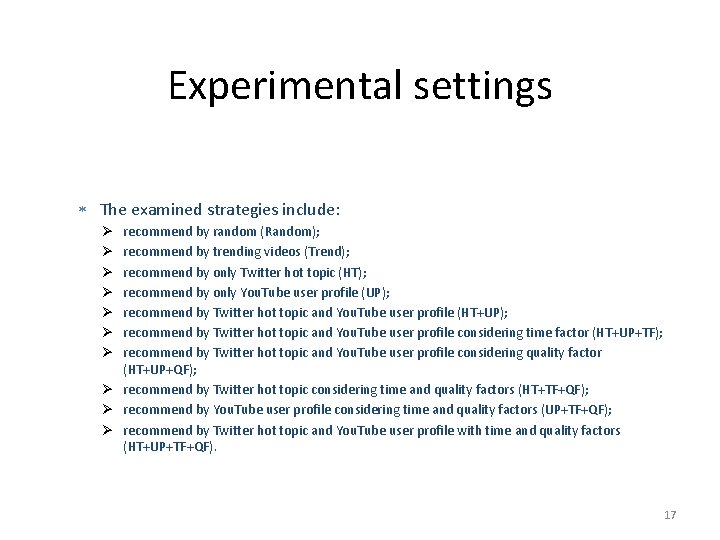
Experiments Experimental settings The examined strategies include: recommend by random (Random); recommend by trending videos (Trend); recommend by only Twitter hot topic (HT); recommend by only You. Tube user profile (UP); recommend by Twitter hot topic and You. Tube user profile (HT+UP); recommend by Twitter hot topic and You. Tube user profile considering time factor (HT+UP+TF); recommend by Twitter hot topic and You. Tube user profile considering quality factor (HT+UP+QF); Ø recommend by Twitter hot topic considering time and quality factors (HT+TF+QF); Ø recommend by You. Tube user profile considering time and quality factors (UP+TF+QF); Ø recommend by Twitter hot topic and You. Tube user profile with time and quality factors (HT+UP+TF+QF). Ø Ø Ø Ø 17
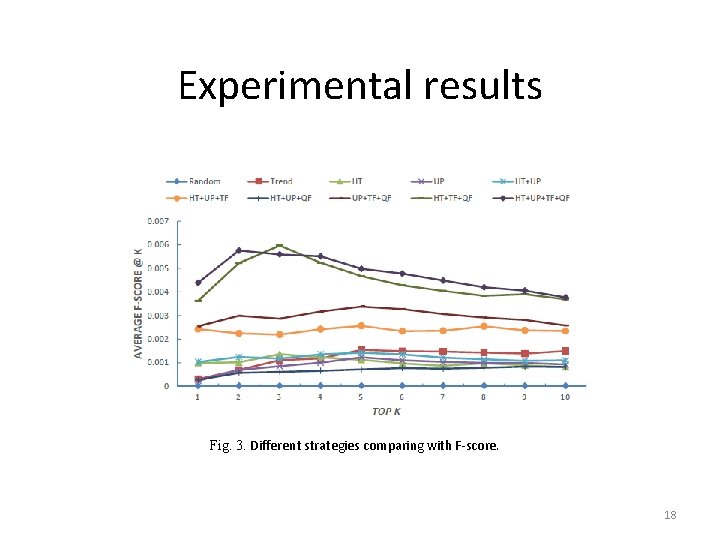
Experiments Experimental results Fig. 3. Different strategies comparing with F-score. 18
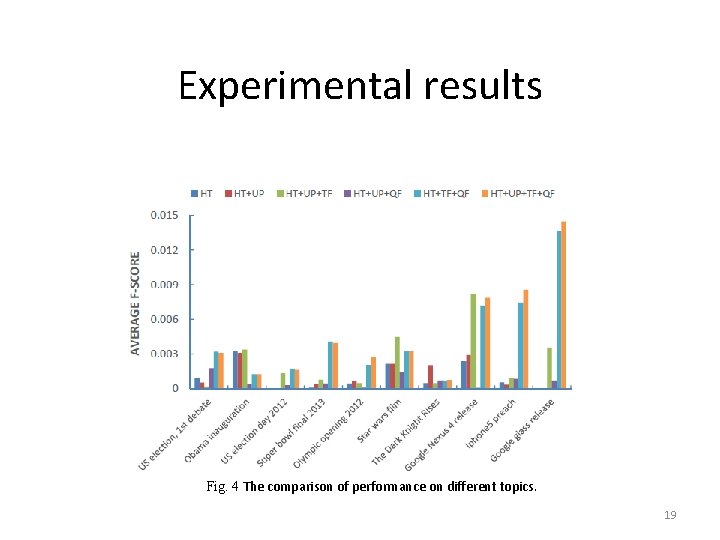
Experiments Experimental results Fig. 4 The comparison of performance on different topics. 19
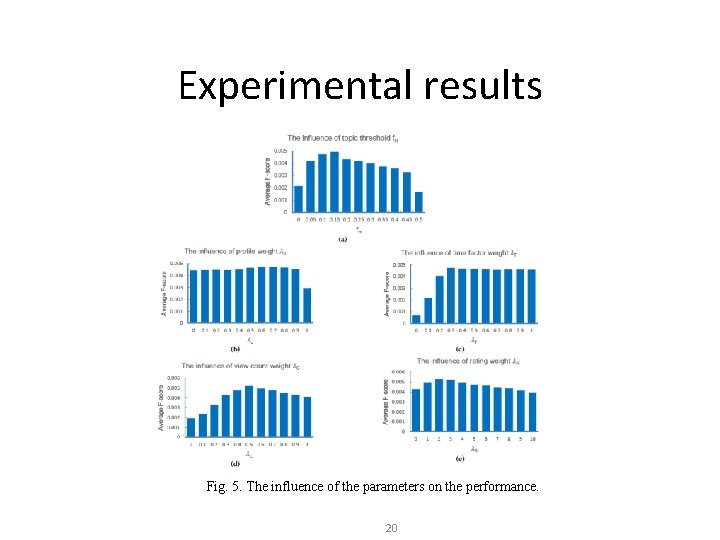
Experiments Experimental results Fig. 5. The influence of the parameters on the performance. 20
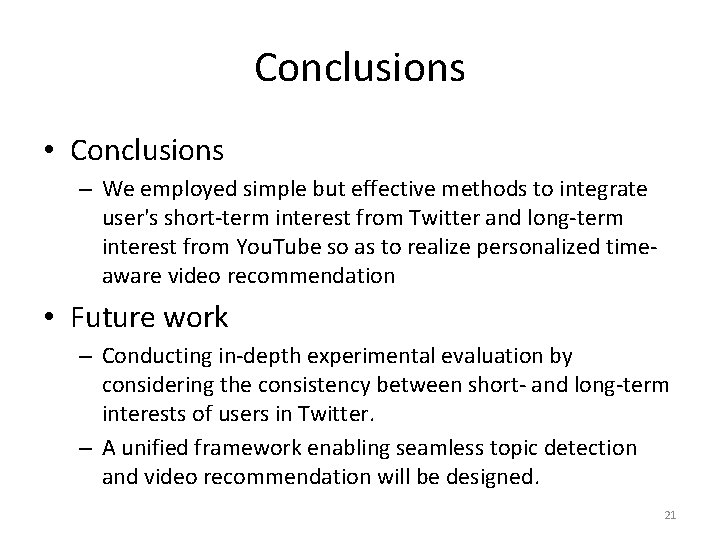
Conclusions • Conclusions – We employed simple but effective methods to integrate user's short-term interest from Twitter and long-term interest from You. Tube so as to realize personalized timeaware video recommendation • Future work – Conducting in-depth experimental evaluation by considering the consistency between short- and long-term interests of users in Twitter. – A unified framework enabling seamless topic detection and video recommendation will be designed. 21
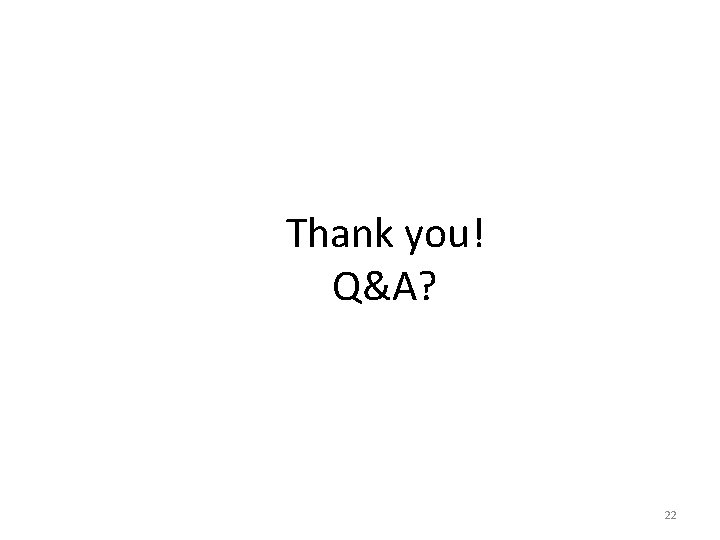
Thank you! Q&A? 22
Jo14 gameplay twitter
Digital media primer
Yandex ru video search text
Video.search.yahoo.com search video
Video search yahoo
Mara runs faster than gail
Free fall
The universe faster than should be
The superlative of hot
Faster than fairies
Which one is faster
Do heavier things fall faster
Searle
Gel electrophoresis why do smaller fragments move faster
The lower the activation energy the faster the reaction
28 day faster diagnosis standard
Driving faster can cause disaster
Faster than fairies
The faster should
If we had more rain our crops would grow faster
They've always been faster
Allthingshairy.com grow hair faster
Painted stations whistle by