TRACE INITIATIVE Data Quality Hello I am xxx
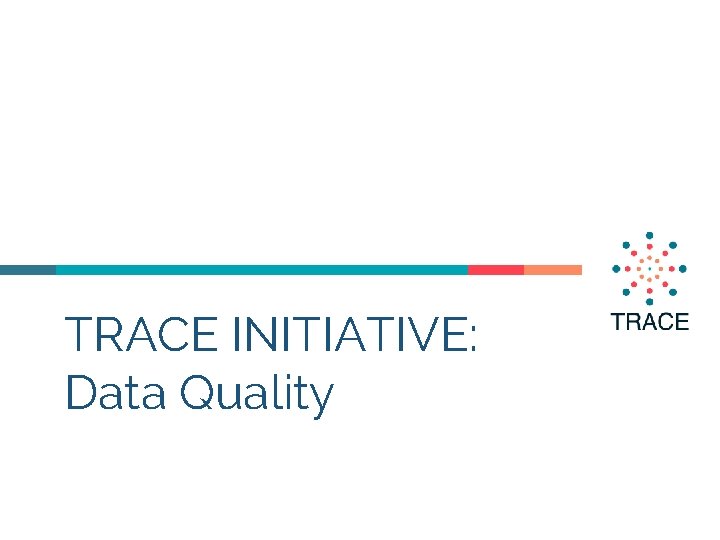
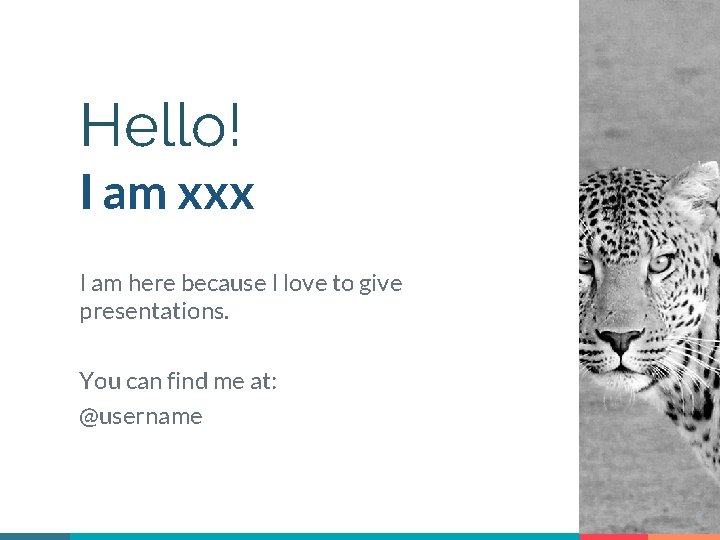
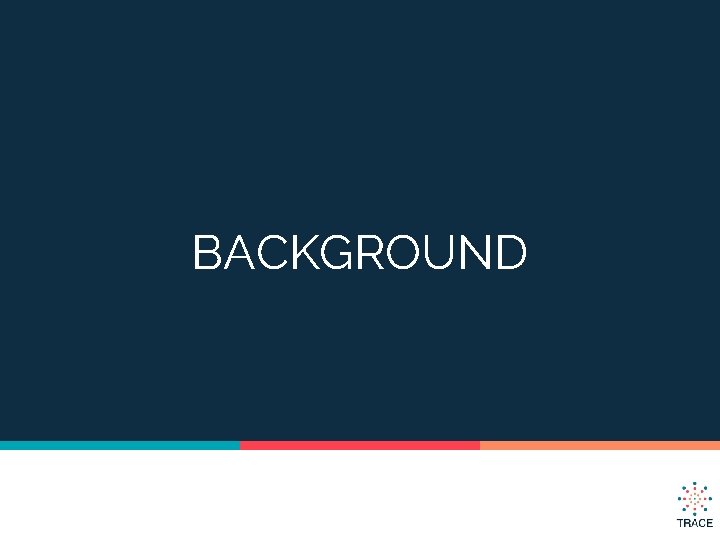
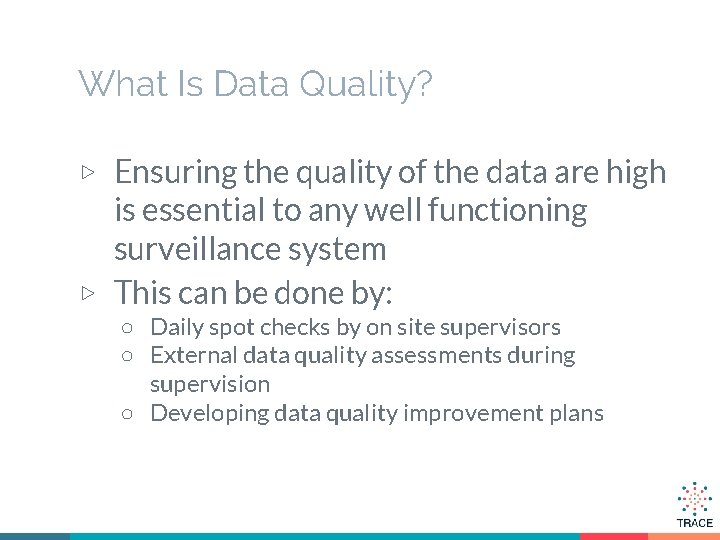
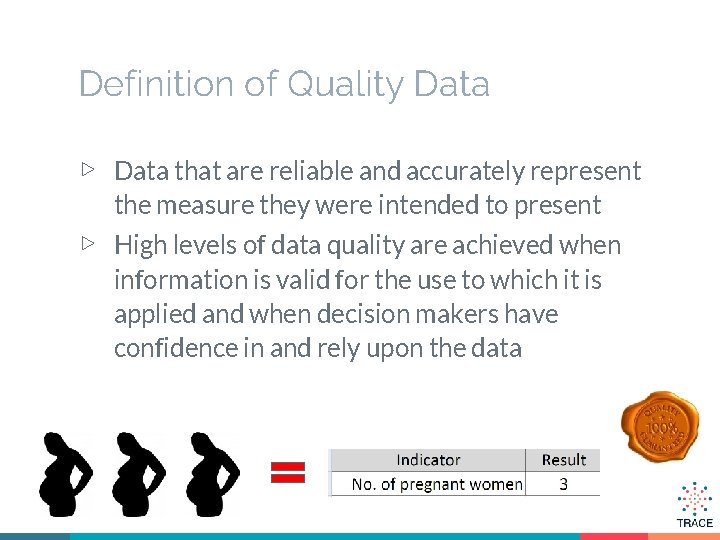
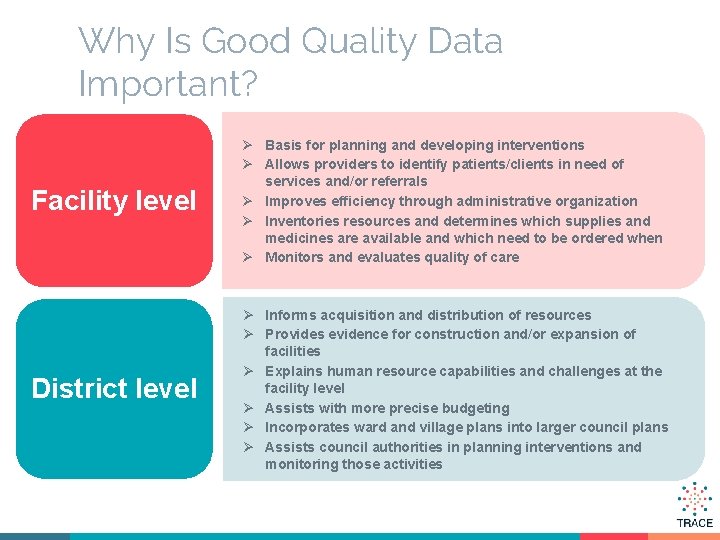
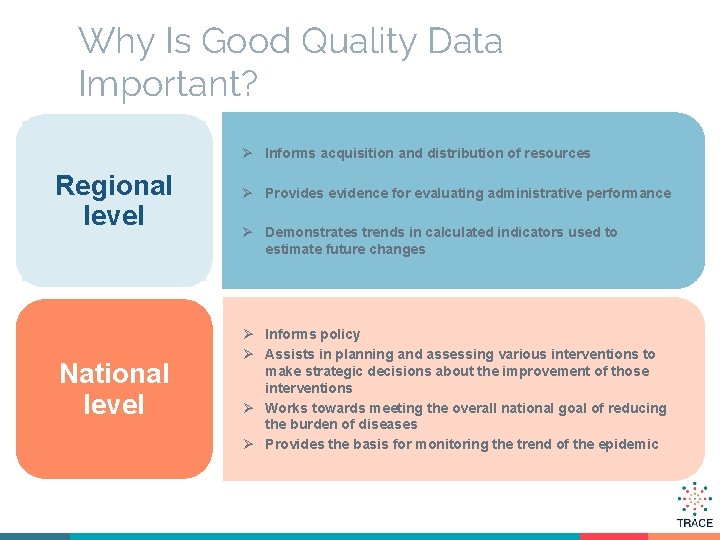
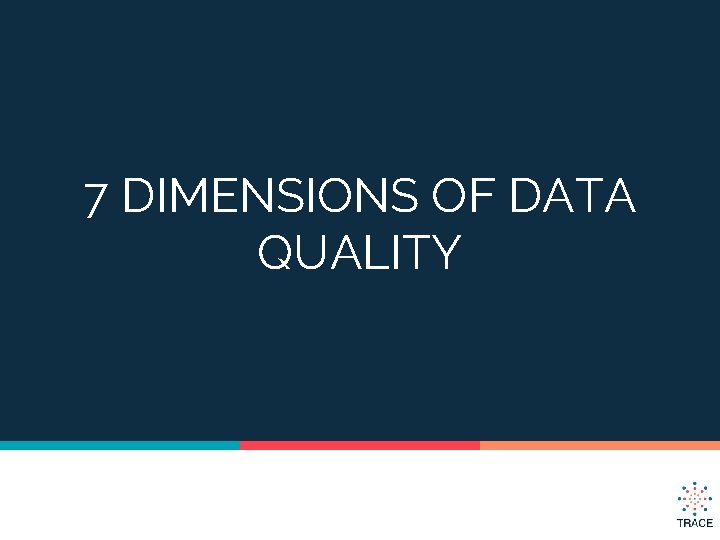
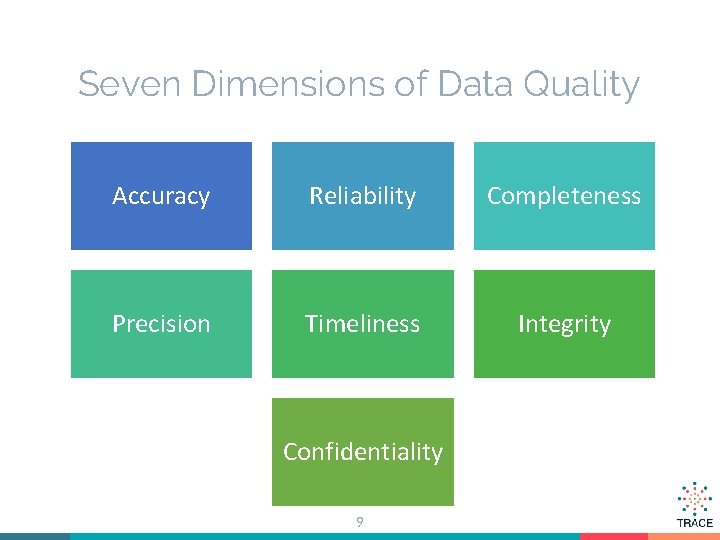
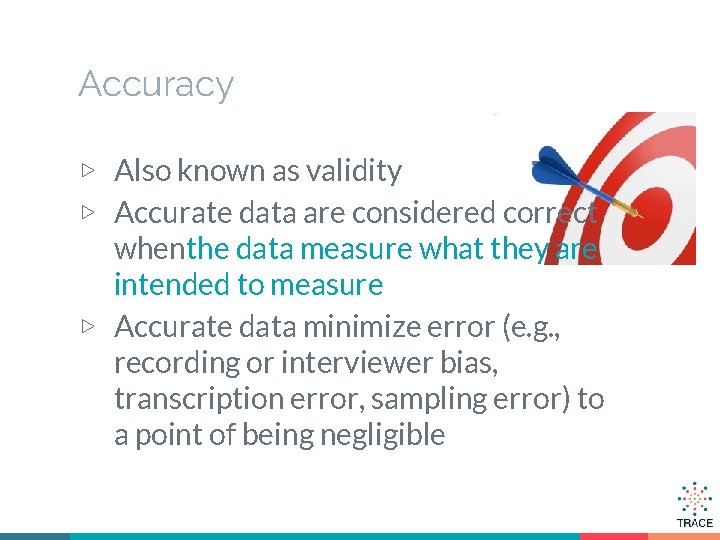
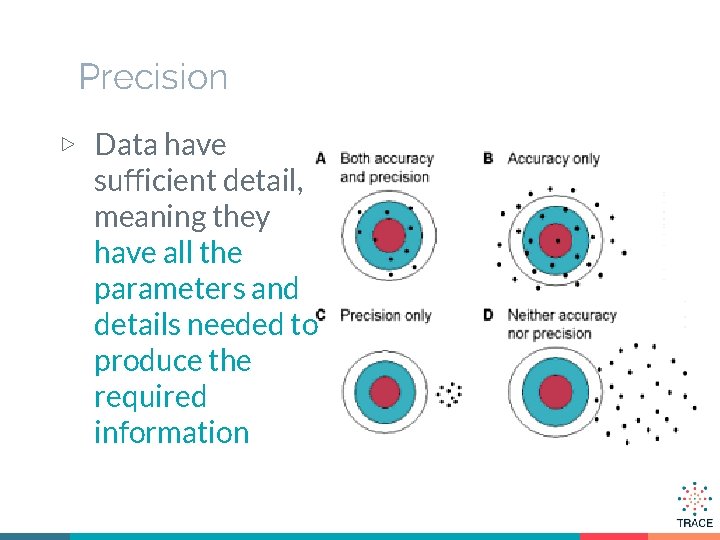
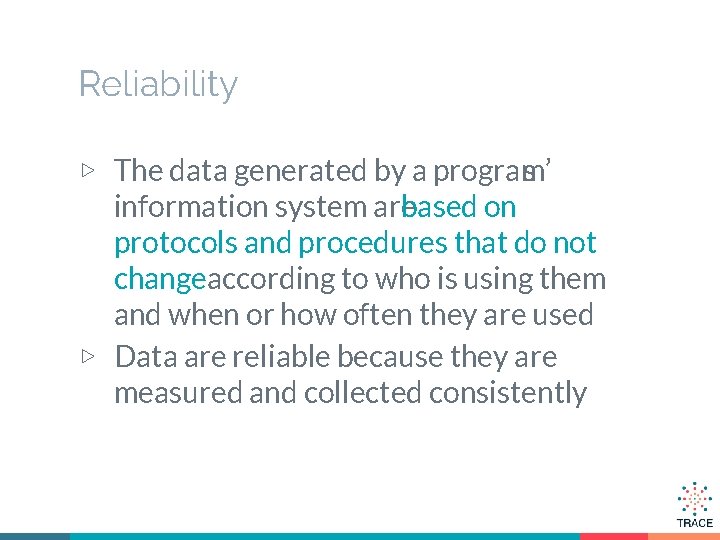
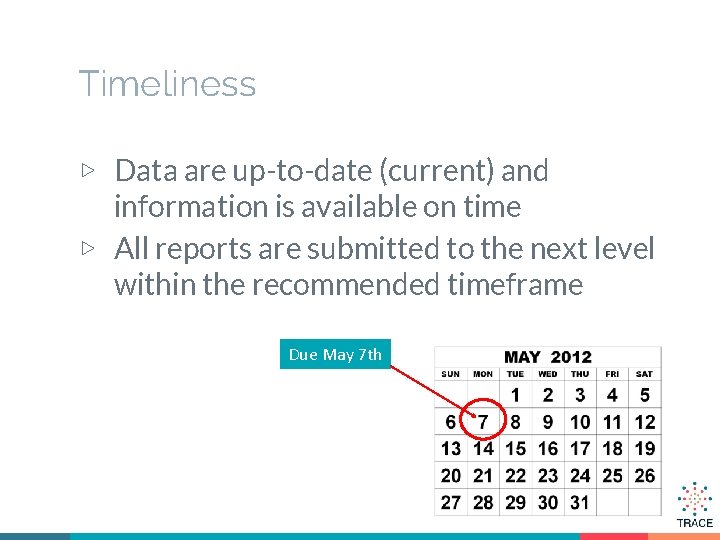
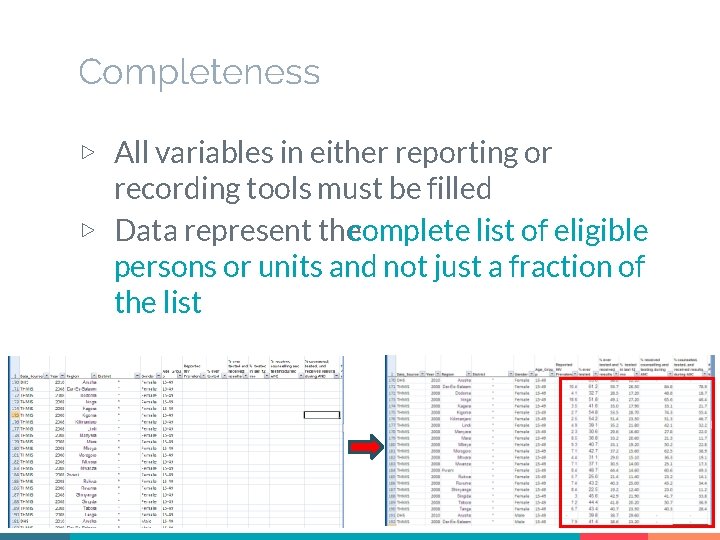
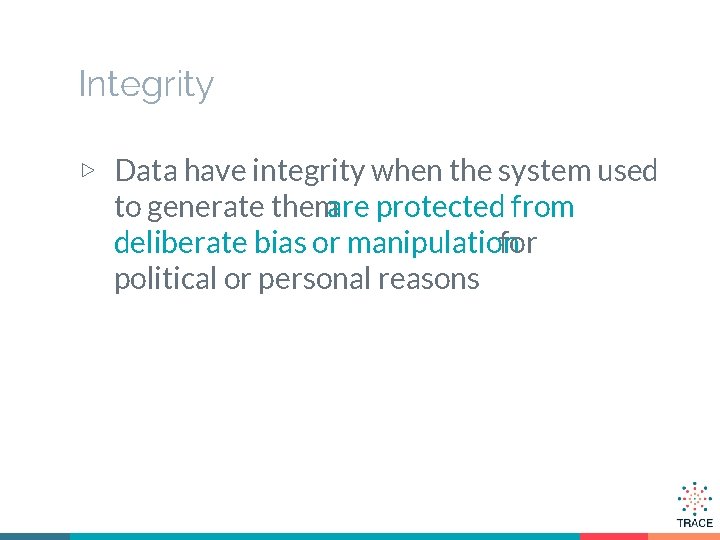
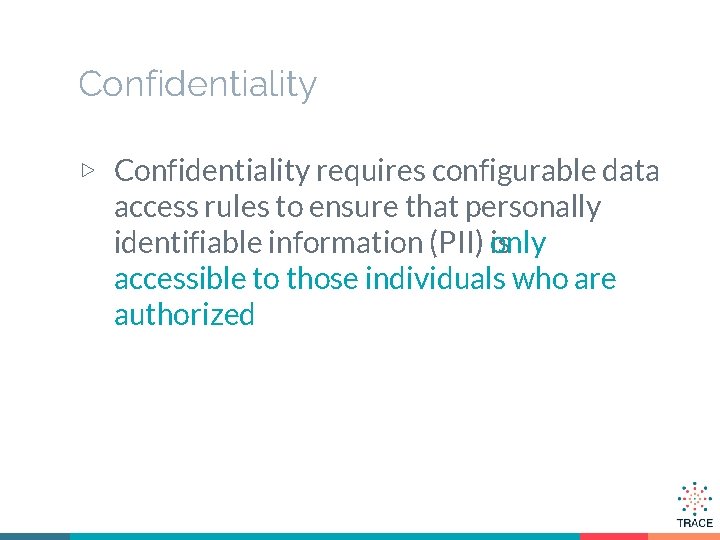
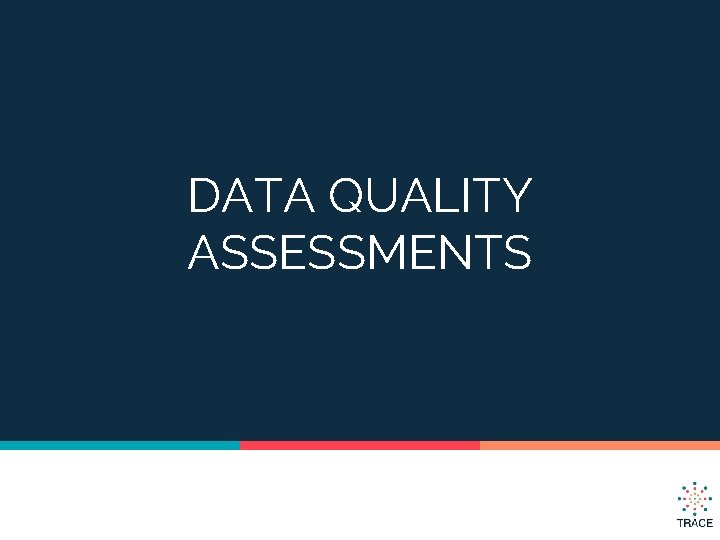
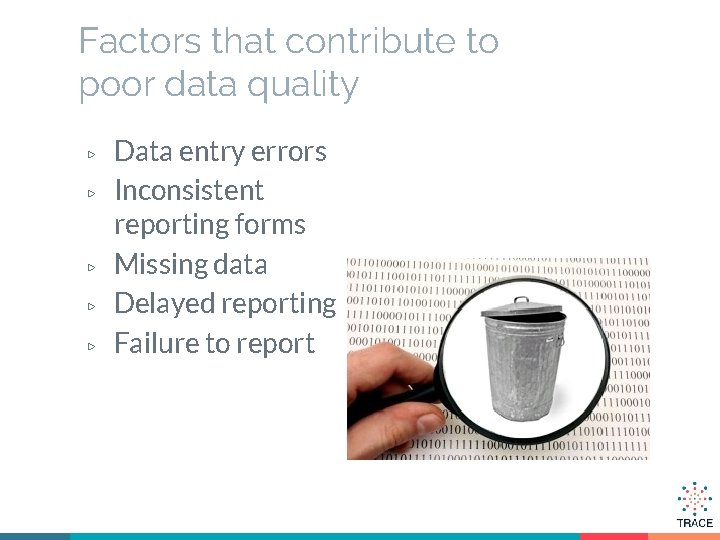
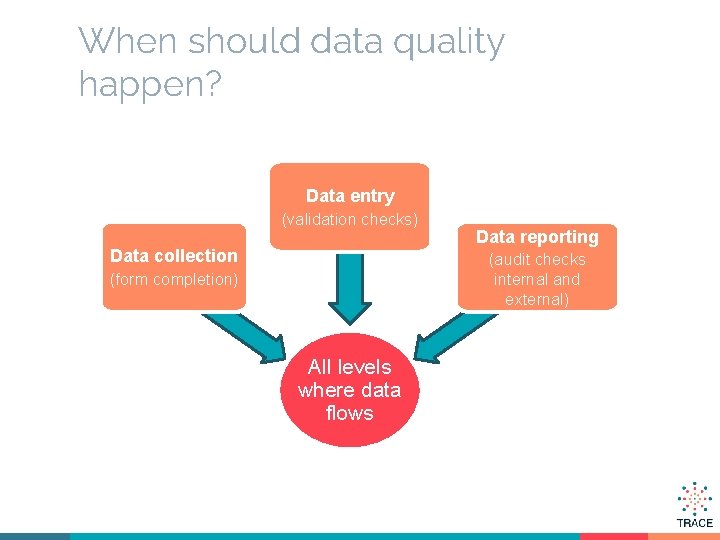
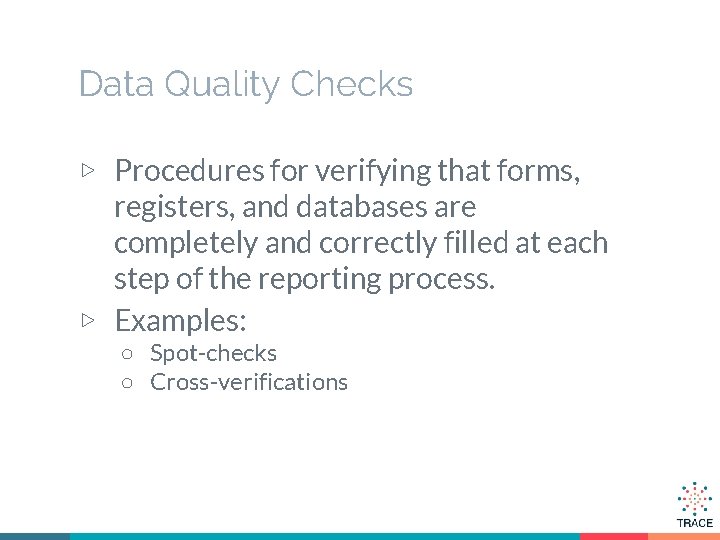
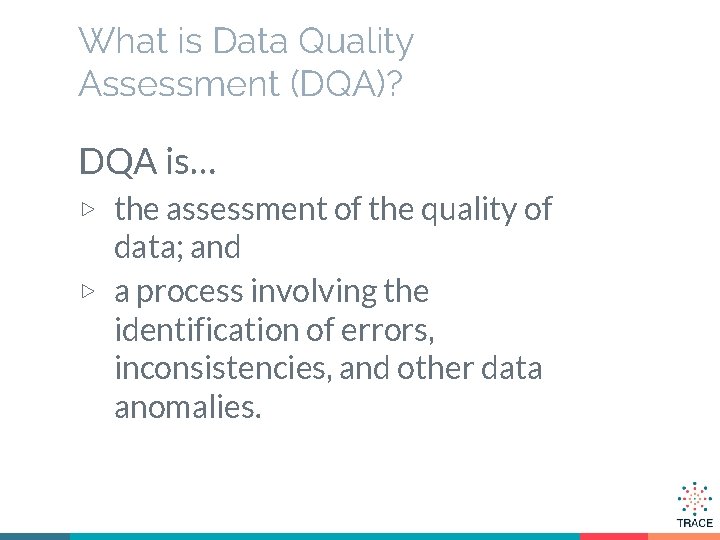
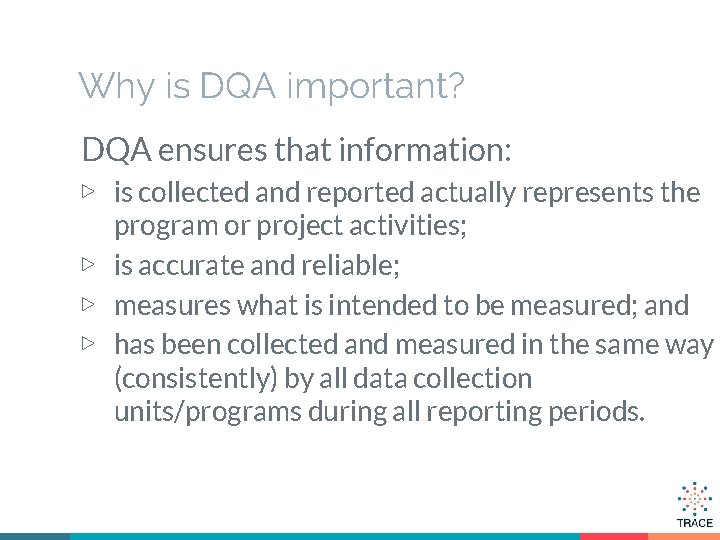
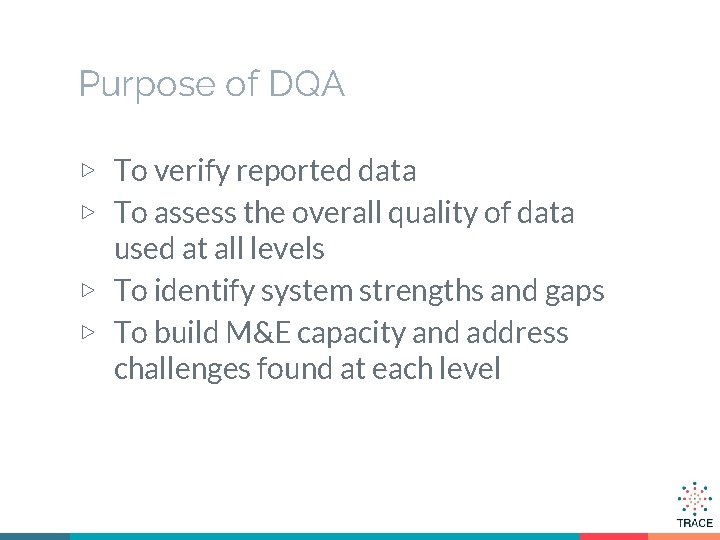
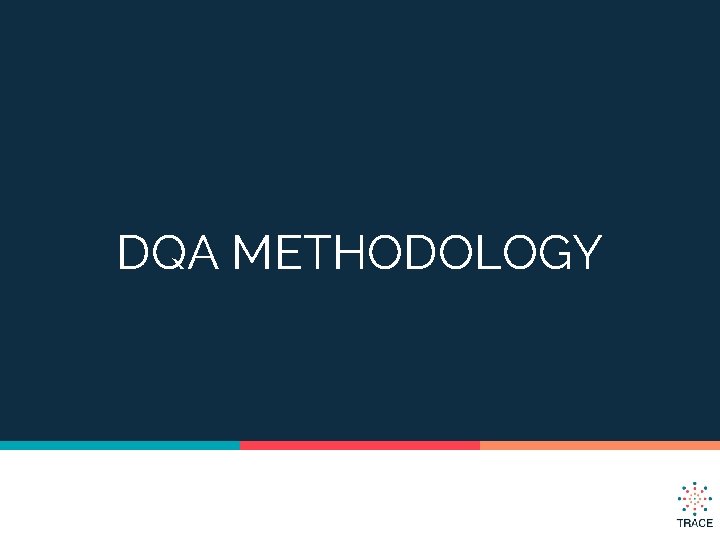
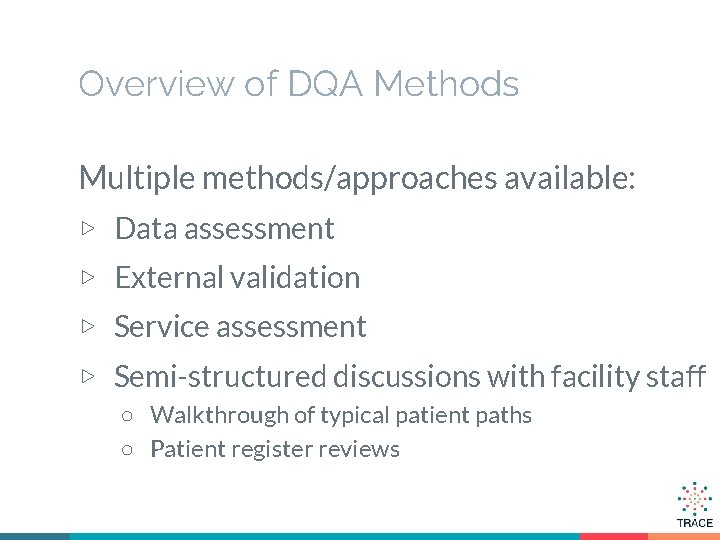
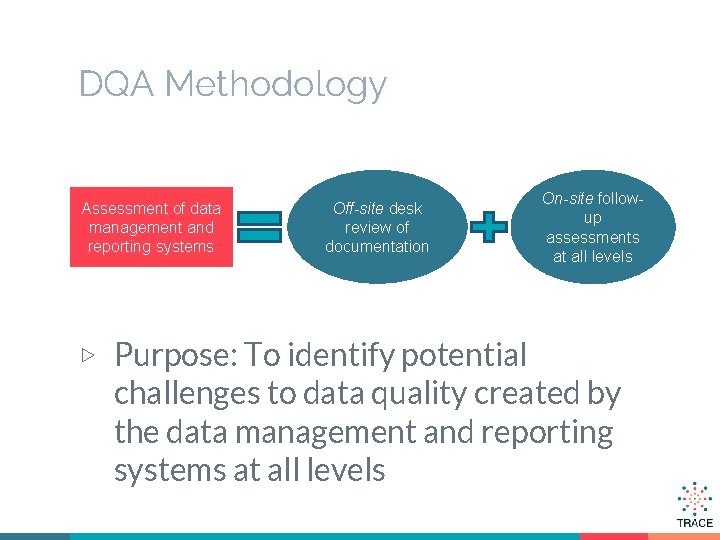
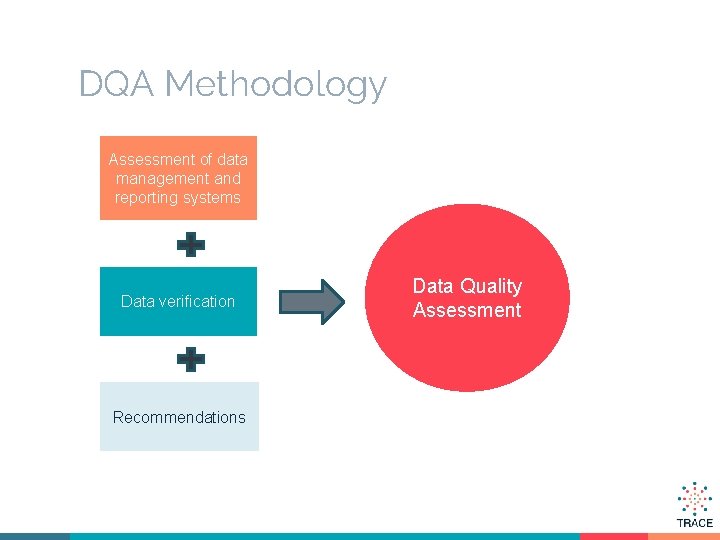
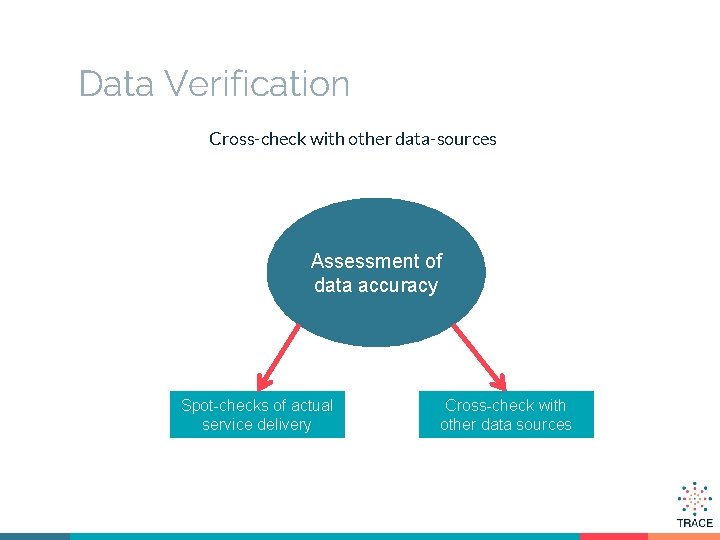
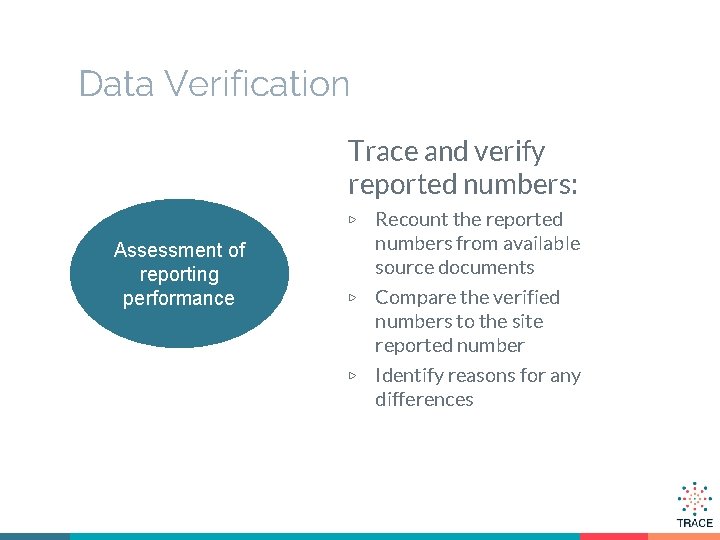
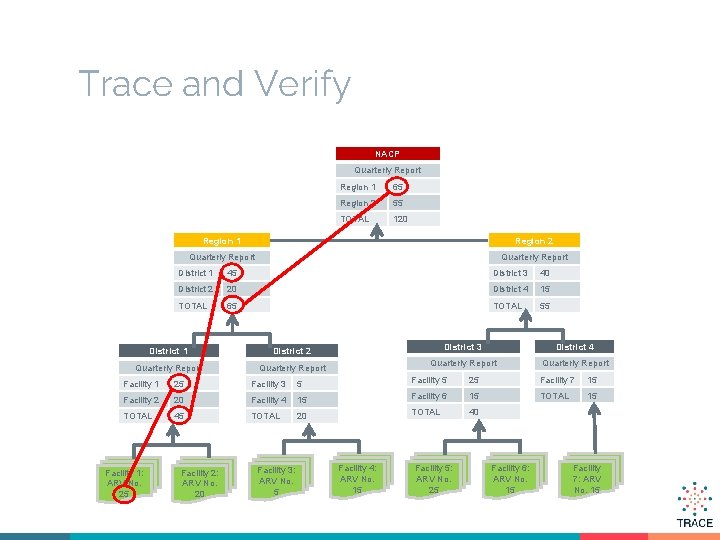
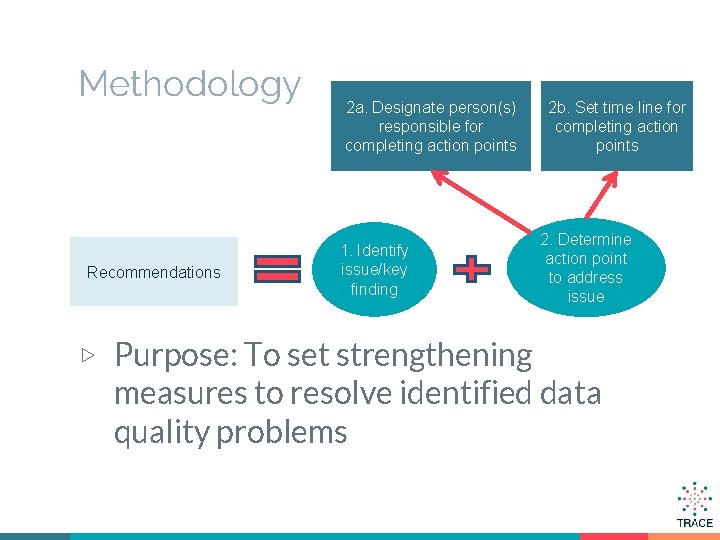
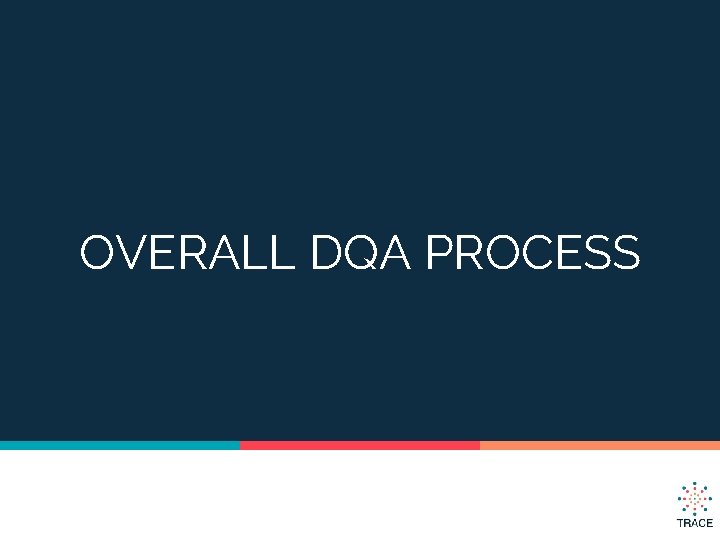
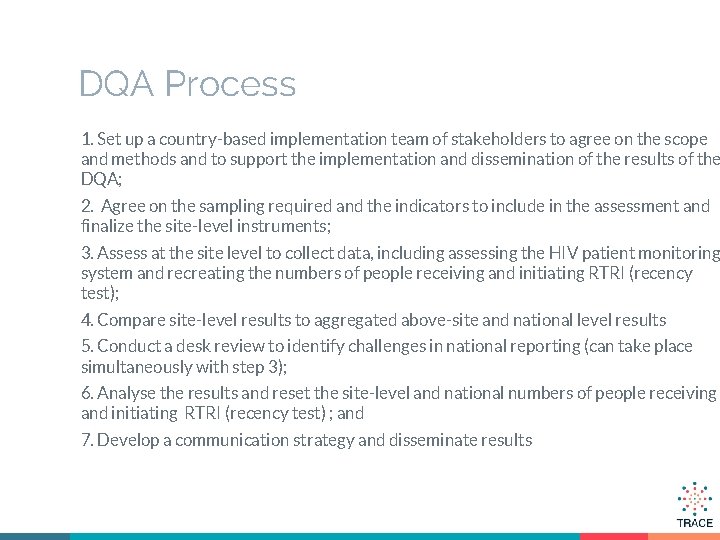
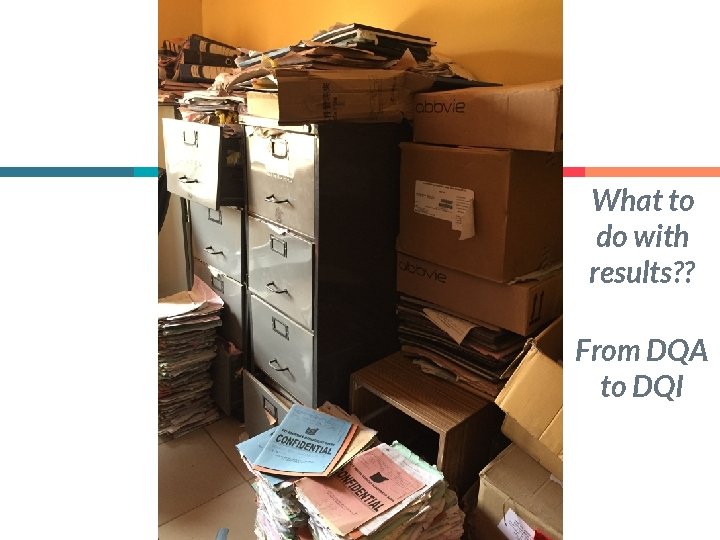
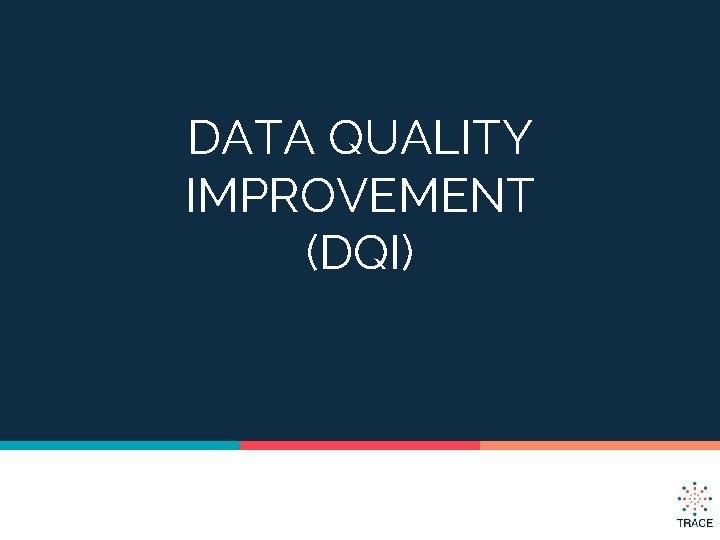
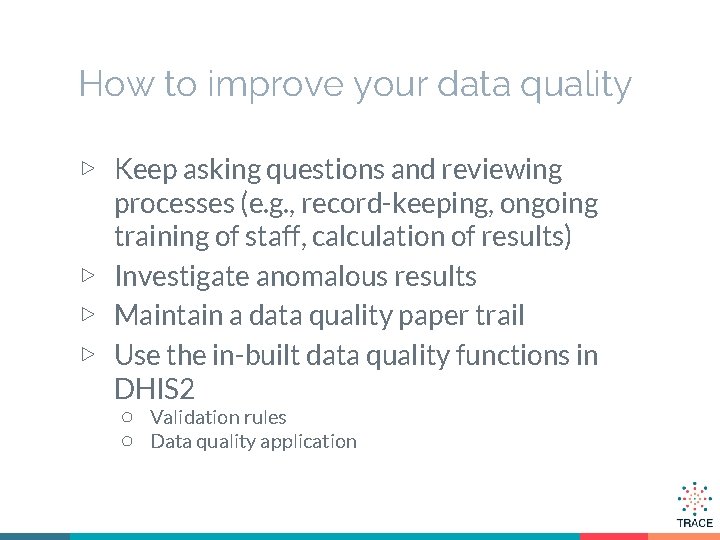
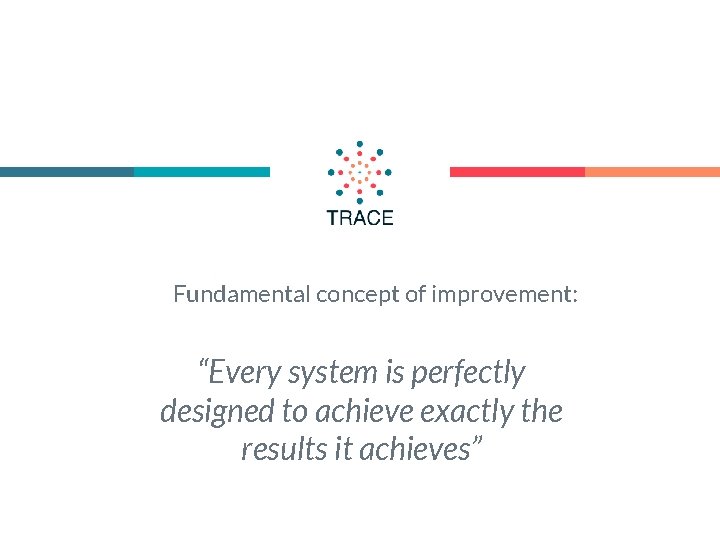
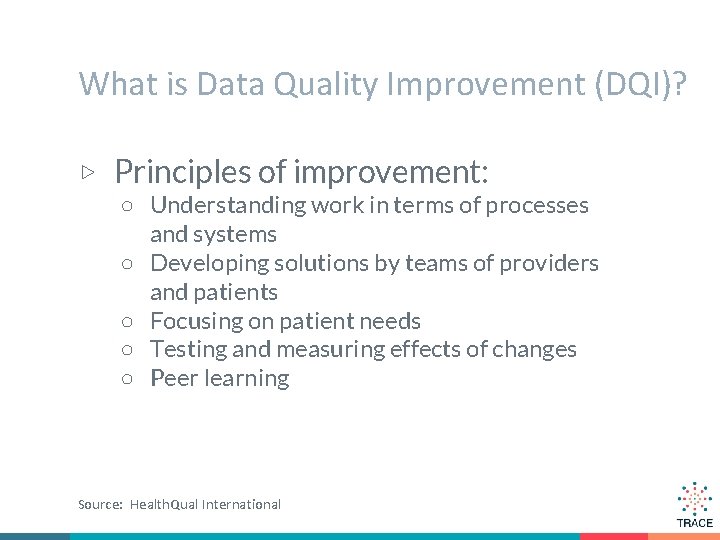
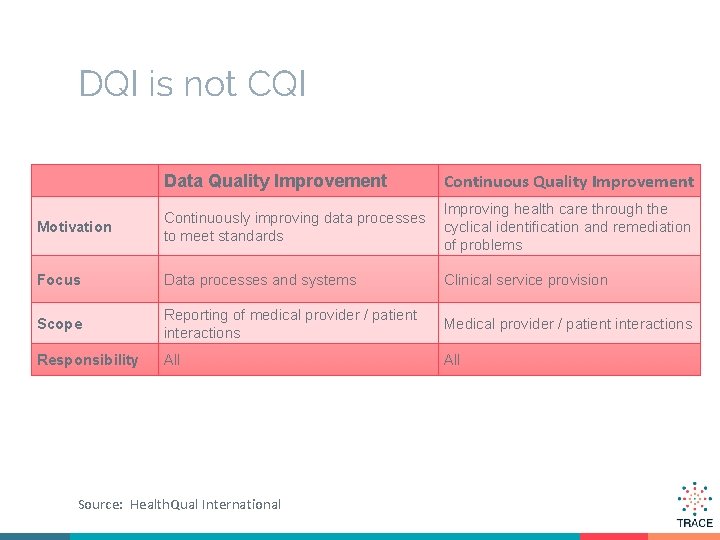
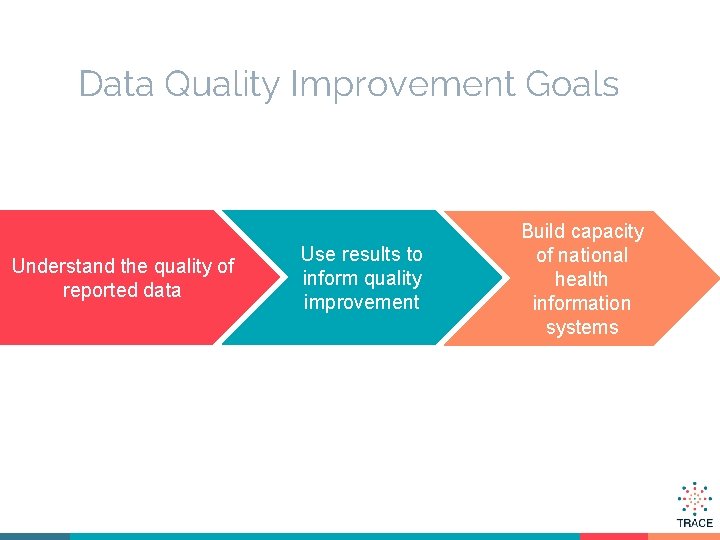
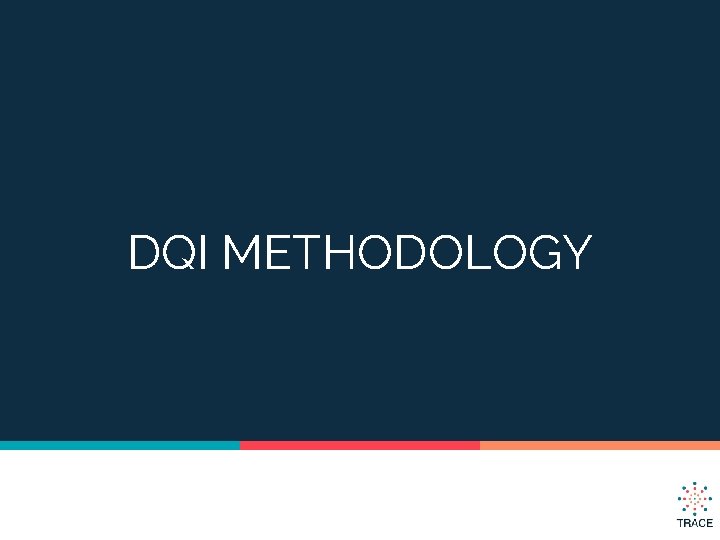
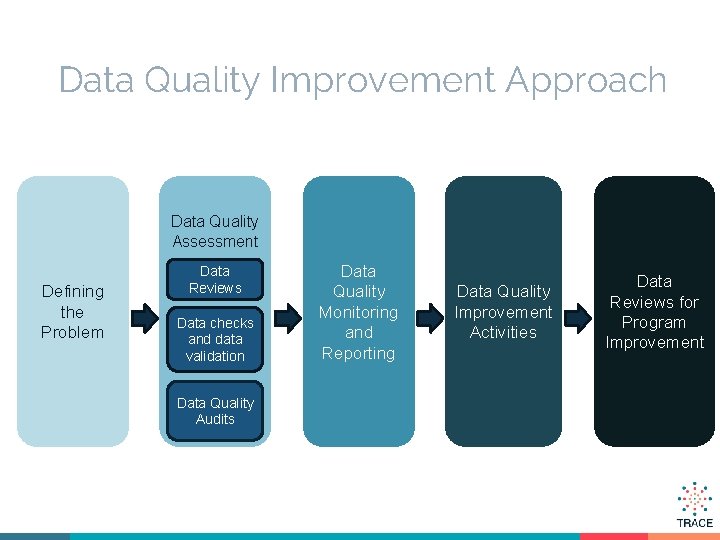
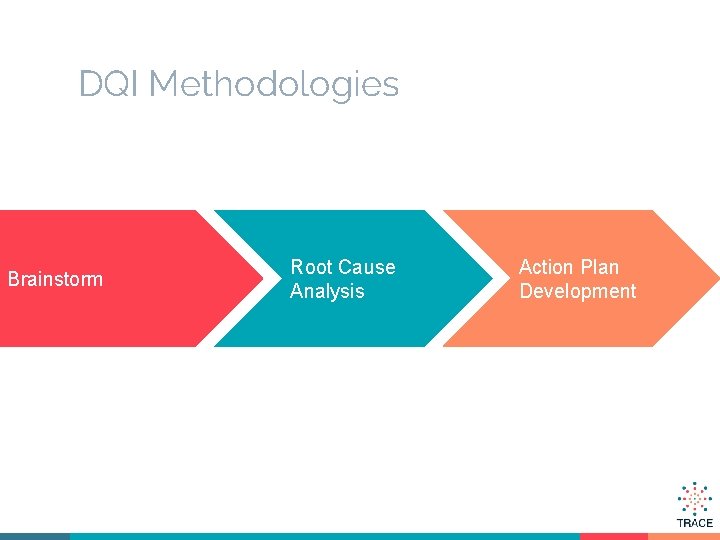
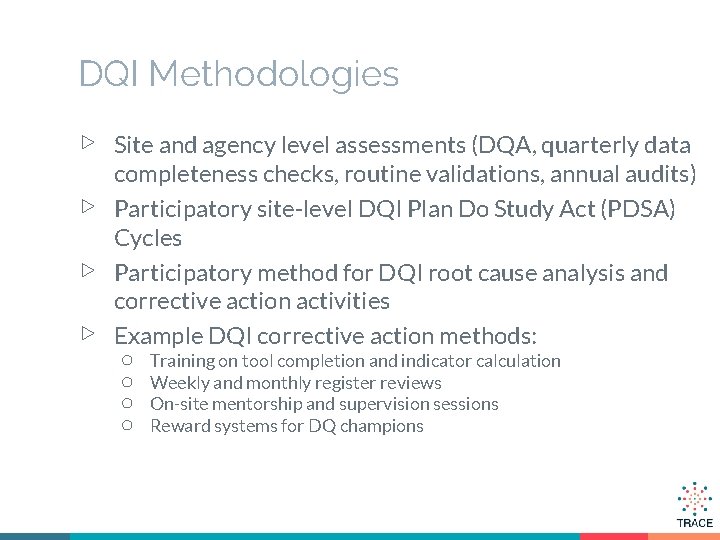
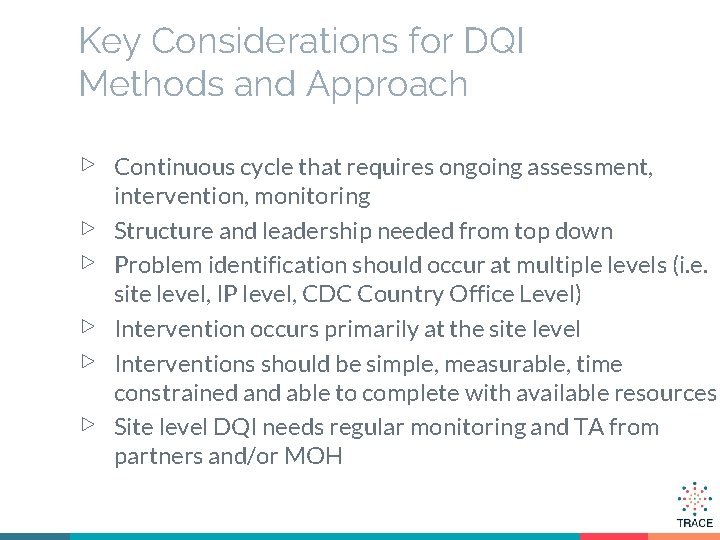
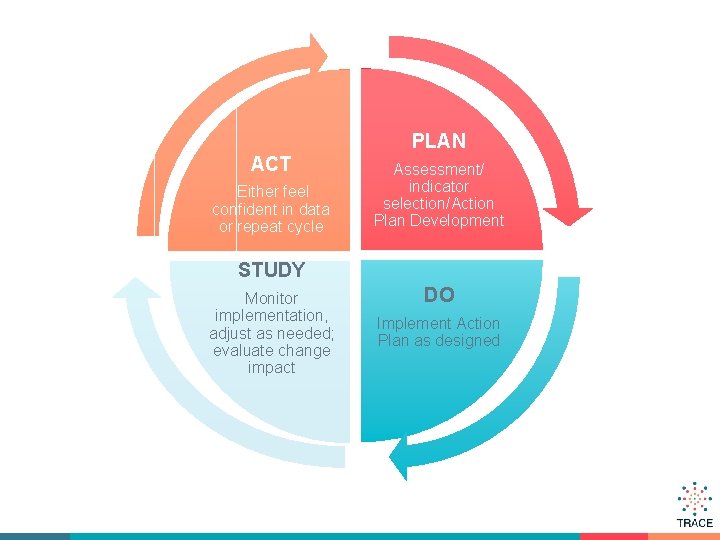
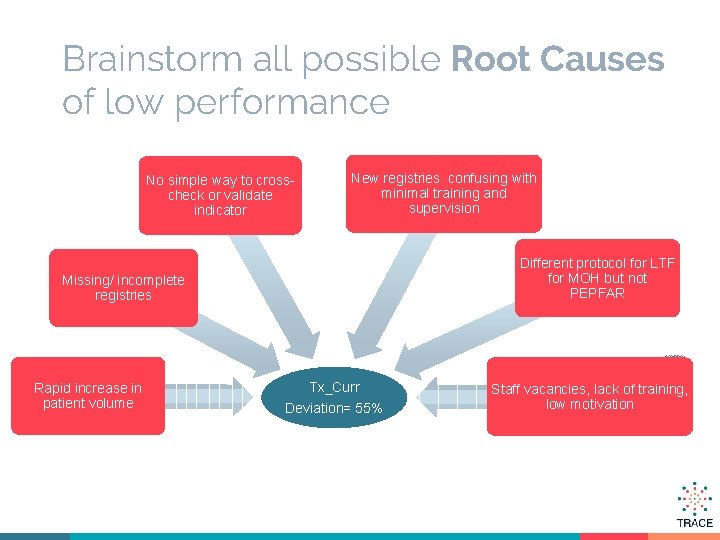
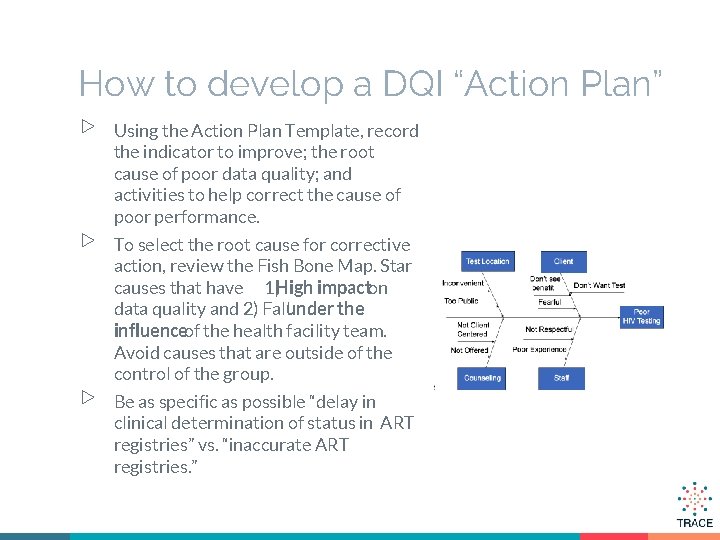
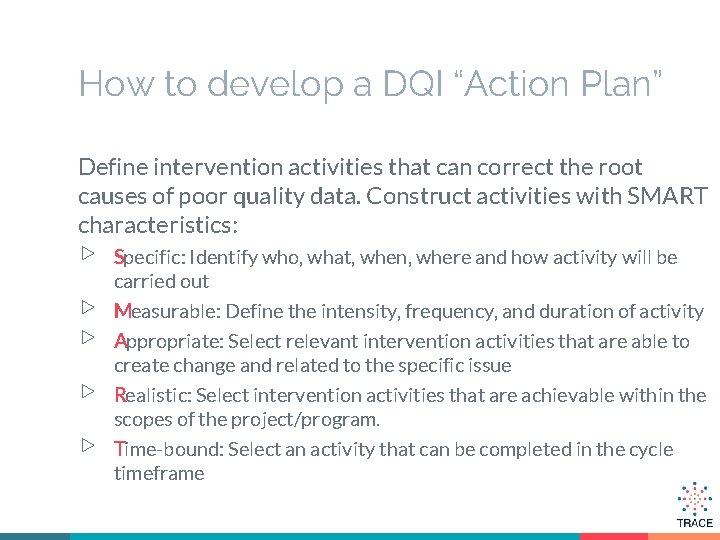
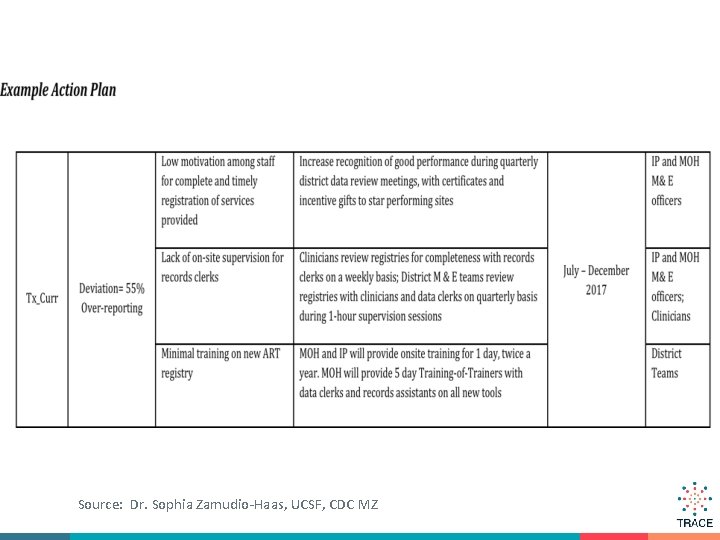
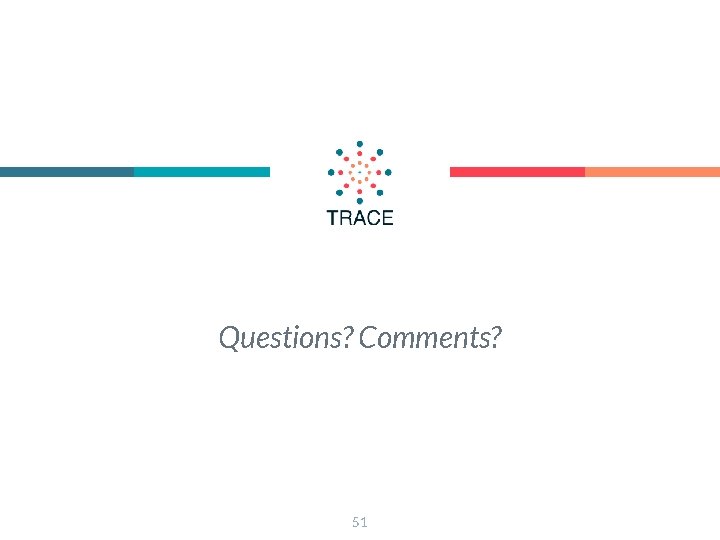
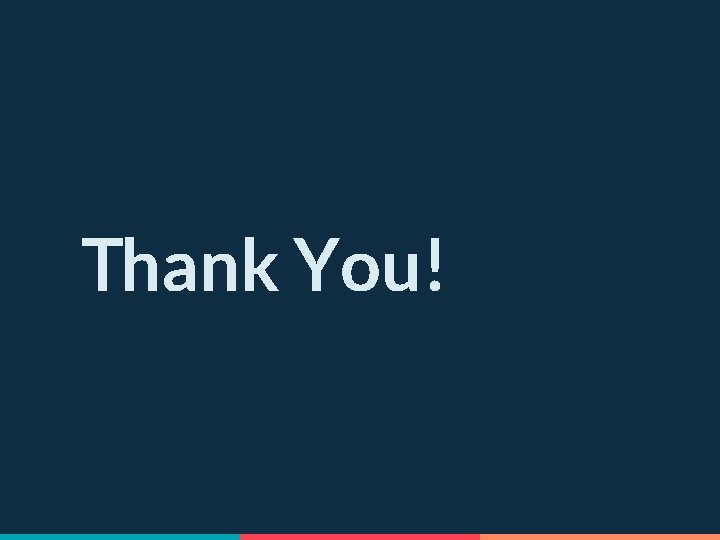
- Slides: 52
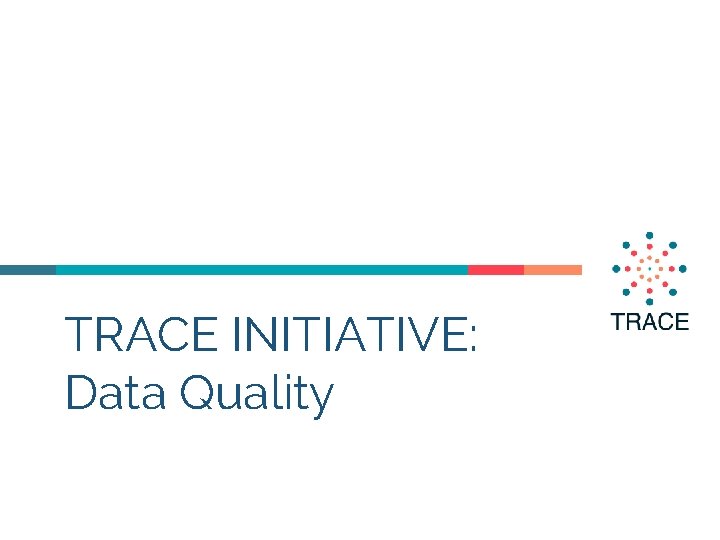
TRACE INITIATIVE: Data Quality
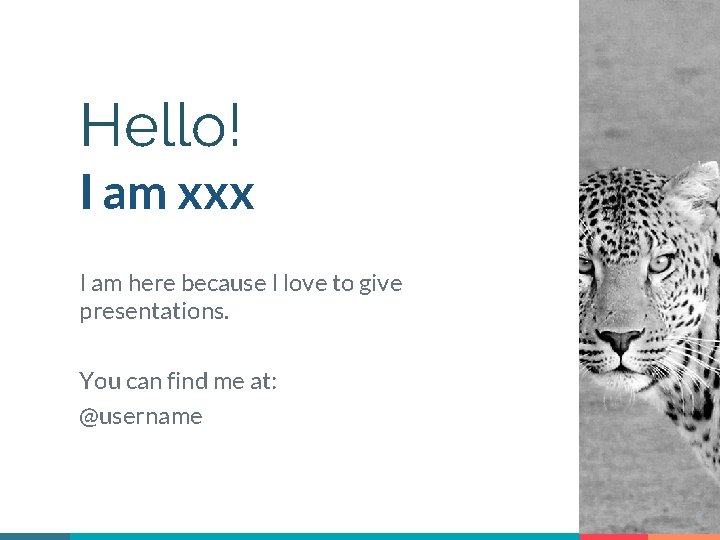
Hello! I am xxx I am here because I love to give presentations. You can find me at: @username 2
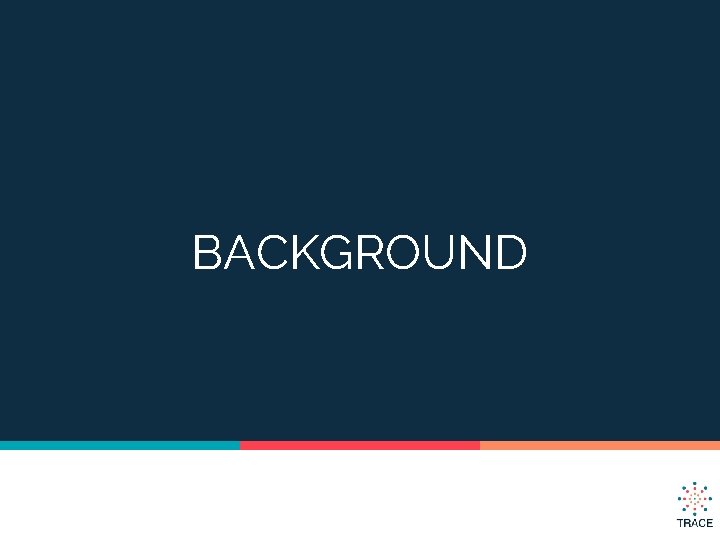
BACKGROUND
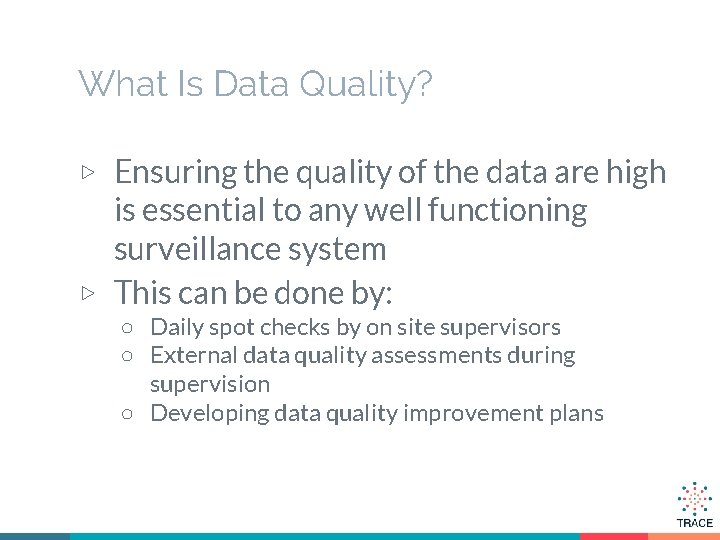
What Is Data Quality? ▷ Ensuring the quality of the data are high is essential to any well functioning surveillance system ▷ This can be done by: ○ Daily spot checks by on site supervisors ○ External data quality assessments during supervision ○ Developing data quality improvement plans
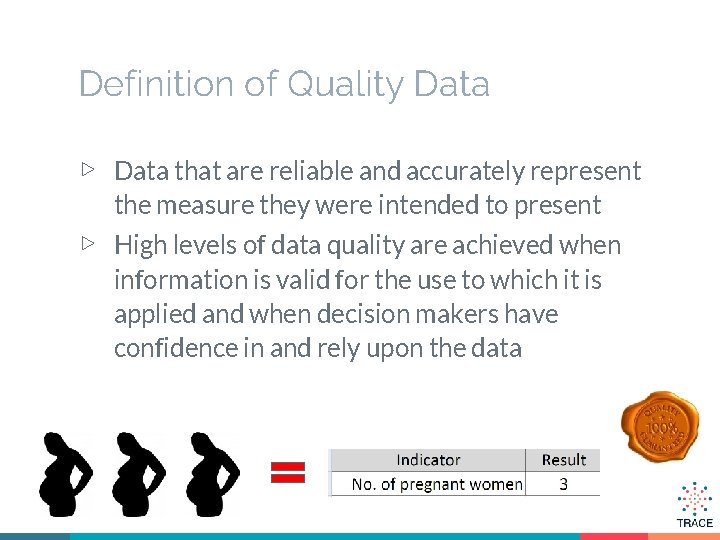
Definition of Quality Data ▷ Data that are reliable and accurately represent the measure they were intended to present ▷ High levels of data quality are achieved when information is valid for the use to which it is applied and when decision makers have confidence in and rely upon the data
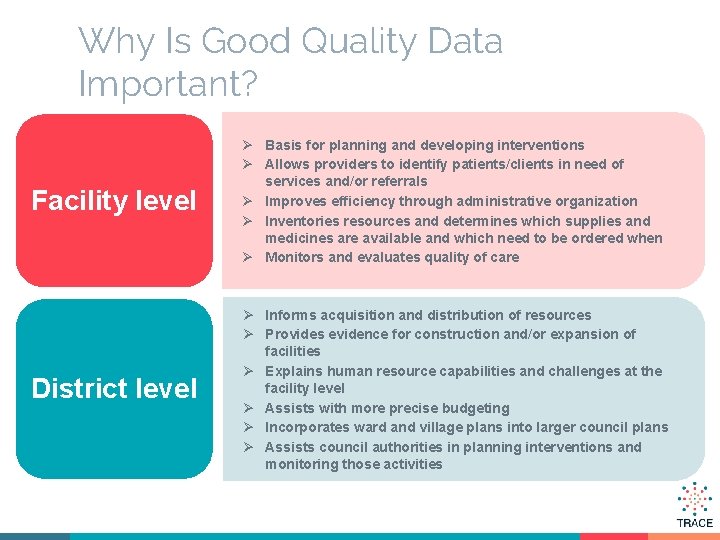
Why Is Good Quality Data Important? Facility level Ø Basis for planning and developing interventions Ø Allows providers to identify patients/clients in need of services and/or referrals Ø Improves efficiency through administrative organization Ø Inventories resources and determines which supplies and medicines are available and which need to be ordered when Ø Monitors and evaluates quality of care District level Ø Informs acquisition and distribution of resources Ø Provides evidence for construction and/or expansion of facilities Ø Explains human resource capabilities and challenges at the facility level Ø Assists with more precise budgeting Ø Incorporates ward and village plans into larger council plans Ø Assists council authorities in planning interventions and monitoring those activities
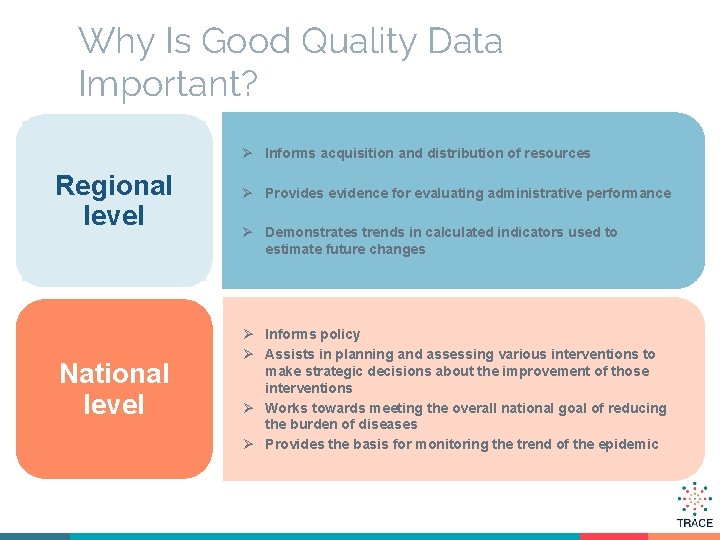
Why Is Good Quality Data Important? Ø Informs acquisition and distribution of resources Regional level National level Ø Provides evidence for evaluating administrative performance Ø Demonstrates trends in calculated indicators used to estimate future changes Ø Informs policy Ø Assists in planning and assessing various interventions to make strategic decisions about the improvement of those interventions Ø Works towards meeting the overall national goal of reducing the burden of diseases Ø Provides the basis for monitoring the trend of the epidemic
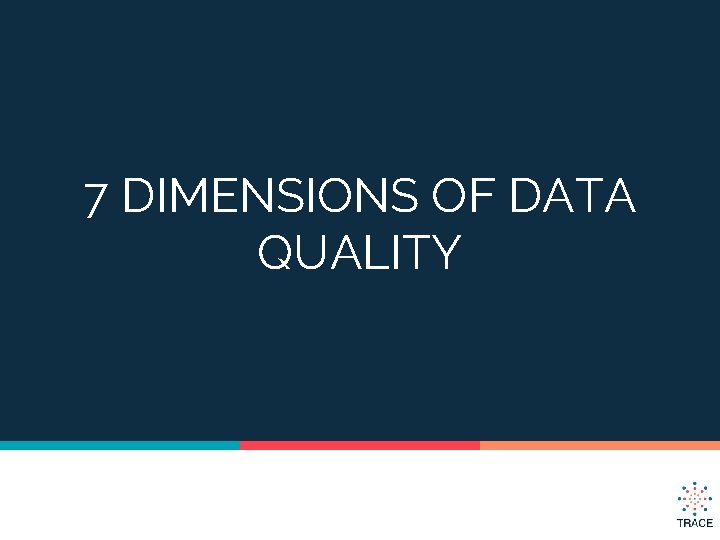
7 DIMENSIONS OF DATA QUALITY
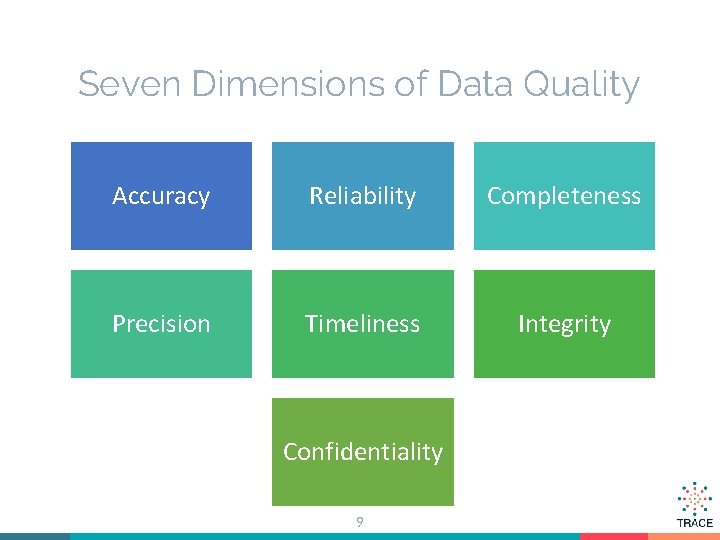
Seven Dimensions of Data Quality Accuracy Reliability Completeness Precision Timeliness Integrity Confidentiality 9
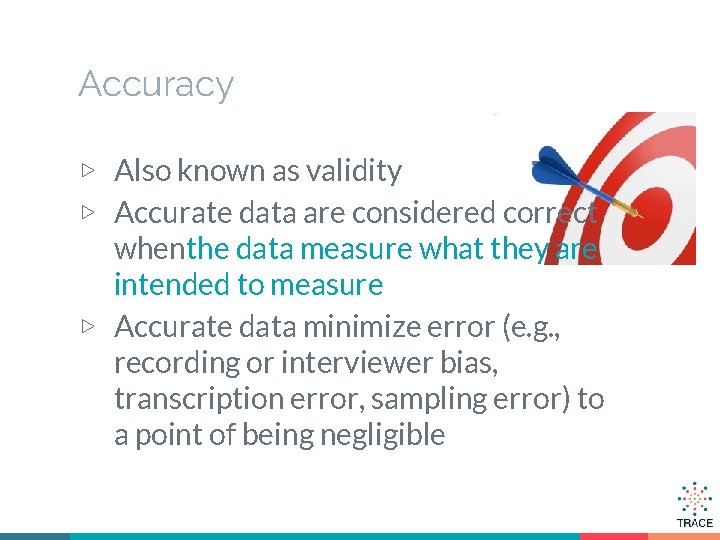
Accuracy ▷ Also known as validity ▷ Accurate data are considered correct whenthe data measure what they are intended to measure ▷ Accurate data minimize error (e. g. , recording or interviewer bias, transcription error, sampling error) to a point of being negligible
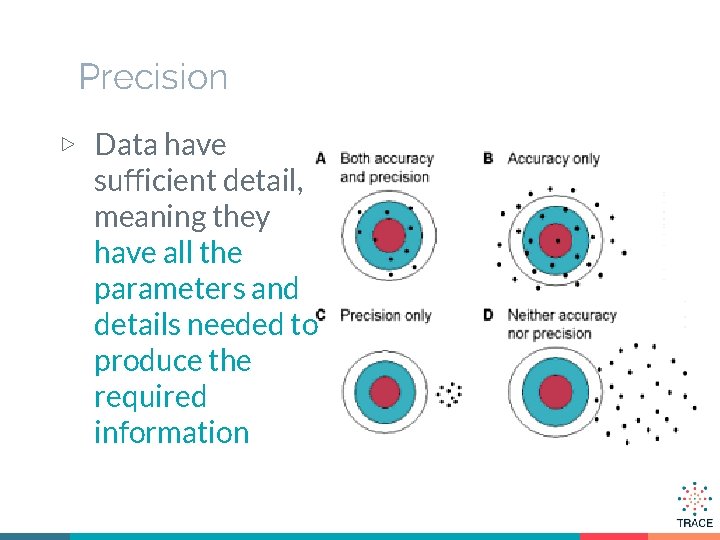
Precision ▷ Data have sufficient detail, meaning they have all the parameters and details needed to produce the required information
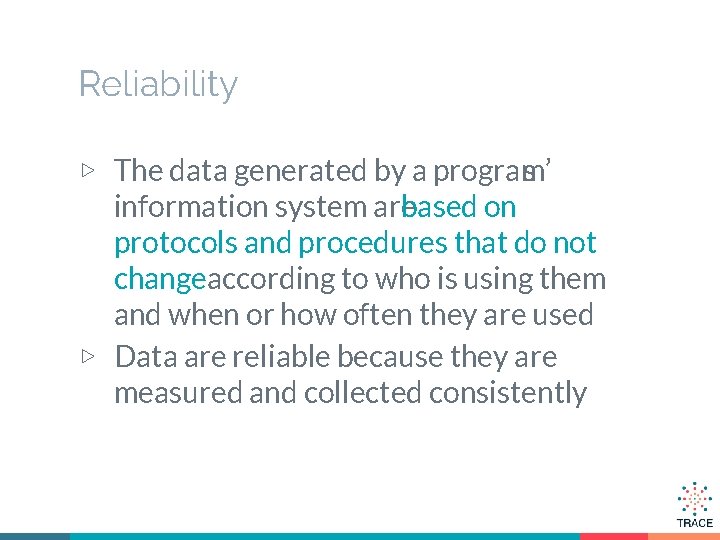
Reliability ▷ The data generated by a program’ s information system are based on protocols and procedures that do not changeaccording to who is using them and when or how often they are used ▷ Data are reliable because they are measured and collected consistently
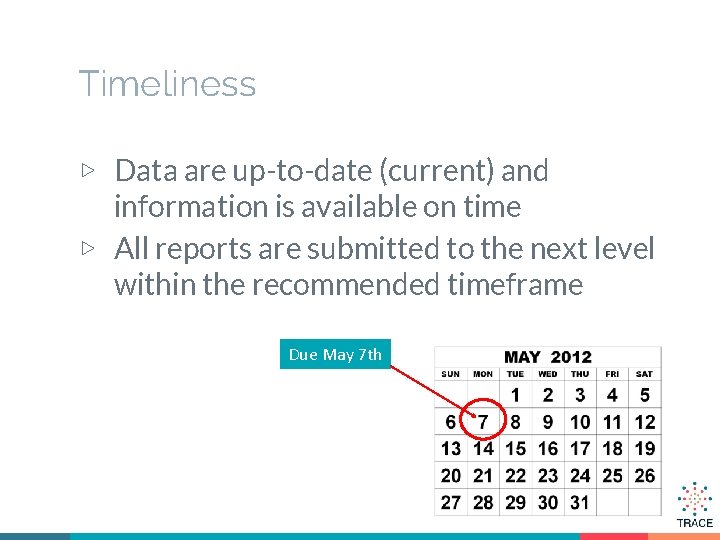
Timeliness ▷ Data are up-to-date (current) and information is available on time ▷ All reports are submitted to the next level within the recommended timeframe Due May 7 th
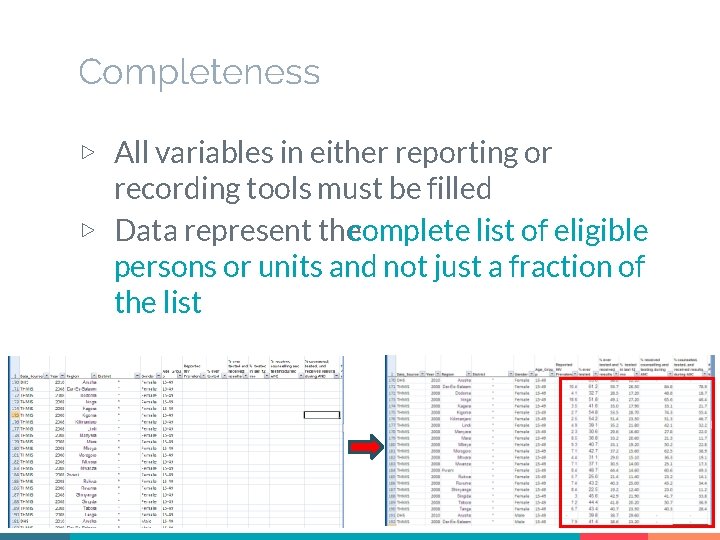
Completeness ▷ All variables in either reporting or recording tools must be filled ▷ Data represent thecomplete list of eligible persons or units and not just a fraction of the list
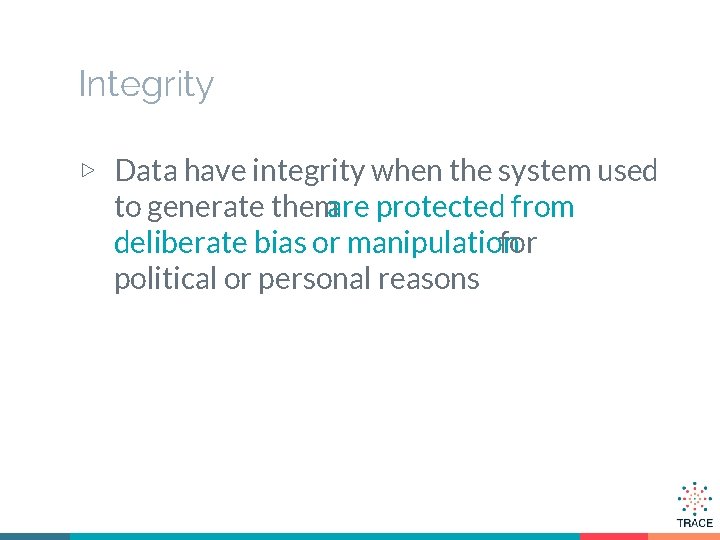
Integrity ▷ Data have integrity when the system used to generate themare protected from deliberate bias or manipulation for political or personal reasons
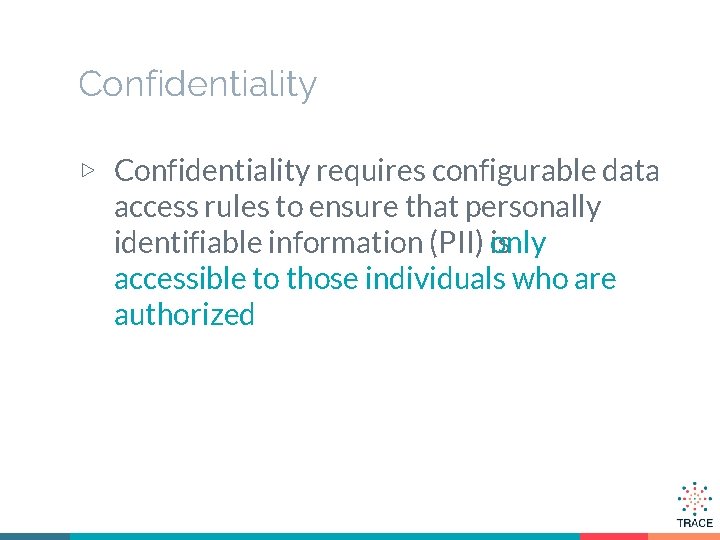
Confidentiality ▷ Confidentiality requires configurable data access rules to ensure that personally identifiable information (PII) only is accessible to those individuals who are authorized
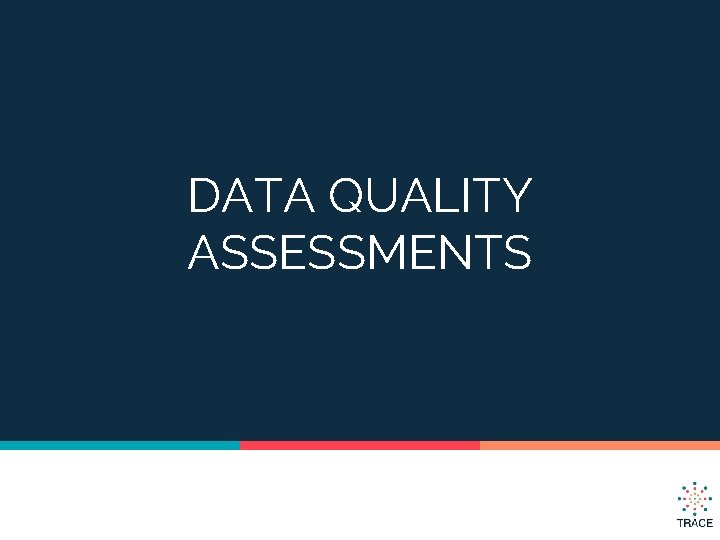
DATA QUALITY ASSESSMENTS
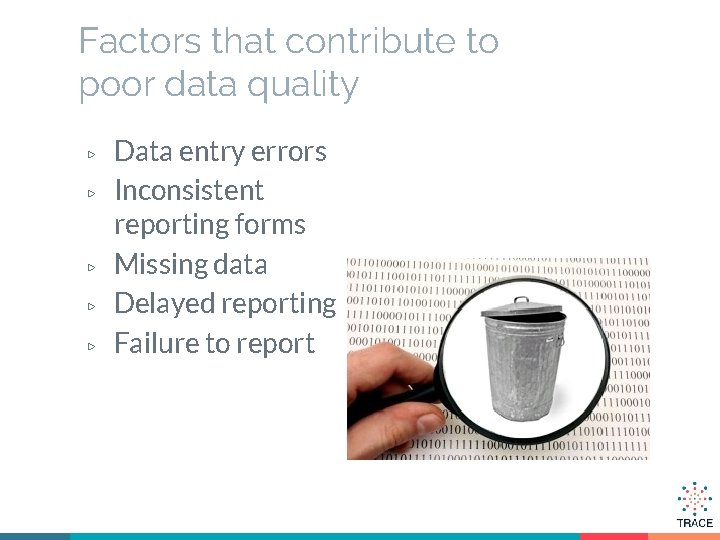
Factors that contribute to poor data quality ▷ ▷ ▷ Data entry errors Inconsistent reporting forms Missing data Delayed reporting Failure to report
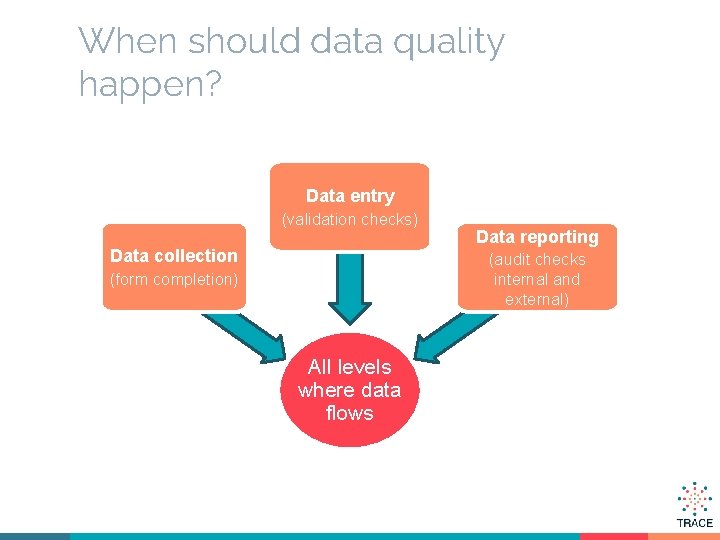
When should data quality happen? Data entry (validation checks) Data collection Data reporting (audit checks internal and external) (form completion) All levels where data flows
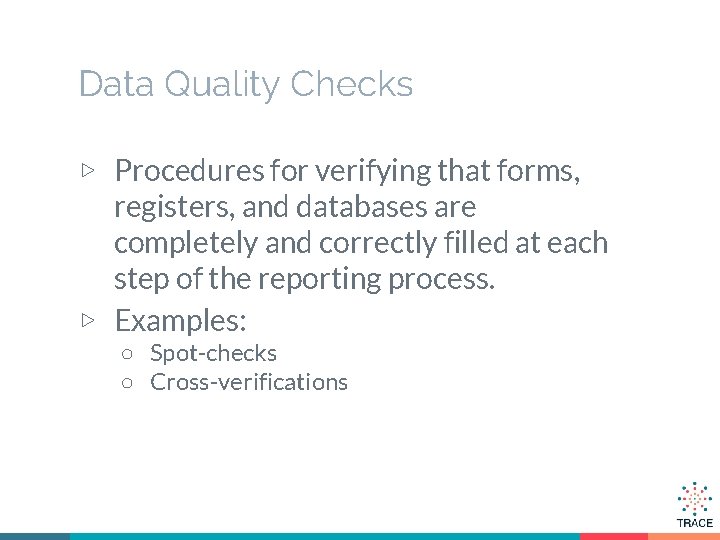
Data Quality Checks ▷ Procedures for verifying that forms, registers, and databases are completely and correctly filled at each step of the reporting process. ▷ Examples: ○ Spot-checks ○ Cross-verifications
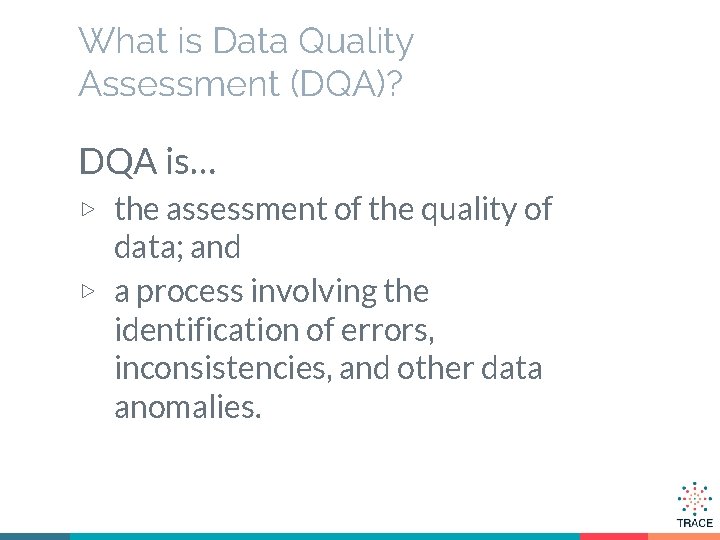
What is Data Quality Assessment (DQA)? DQA is… ▷ the assessment of the quality of data; and ▷ a process involving the identification of errors, inconsistencies, and other data anomalies.
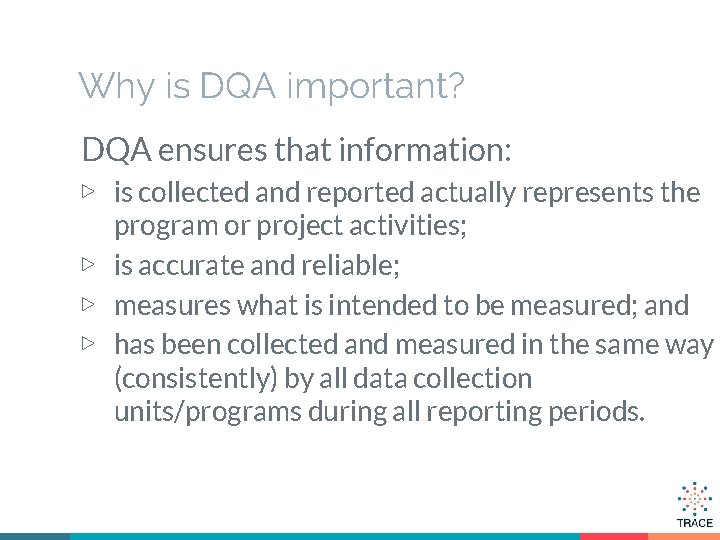
Why is DQA important? DQA ensures that information: ▷ is collected and reported actually represents the program or project activities; ▷ is accurate and reliable; ▷ measures what is intended to be measured; and ▷ has been collected and measured in the same way (consistently) by all data collection units/programs during all reporting periods.
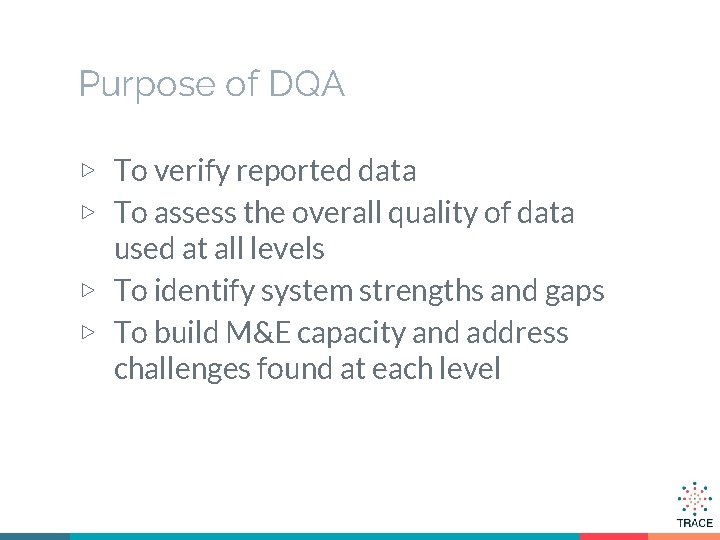
Purpose of DQA ▷ To verify reported data ▷ To assess the overall quality of data used at all levels ▷ To identify system strengths and gaps ▷ To build M&E capacity and address challenges found at each level
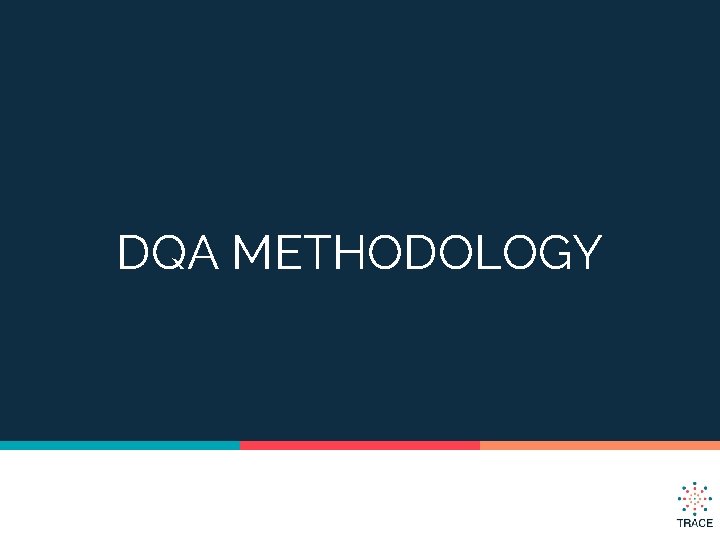
DQA METHODOLOGY
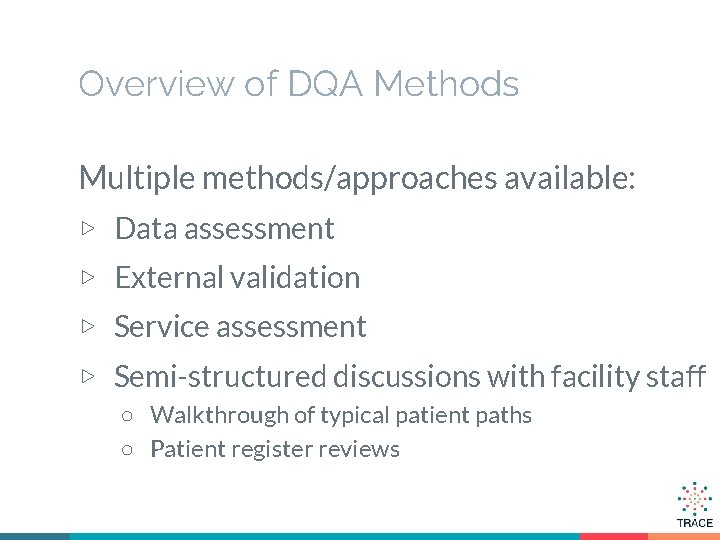
Overview of DQA Methods Multiple methods/approaches available: ▷ Data assessment ▷ External validation ▷ Service assessment ▷ Semi-structured discussions with facility staff ○ Walkthrough of typical patient paths ○ Patient register reviews
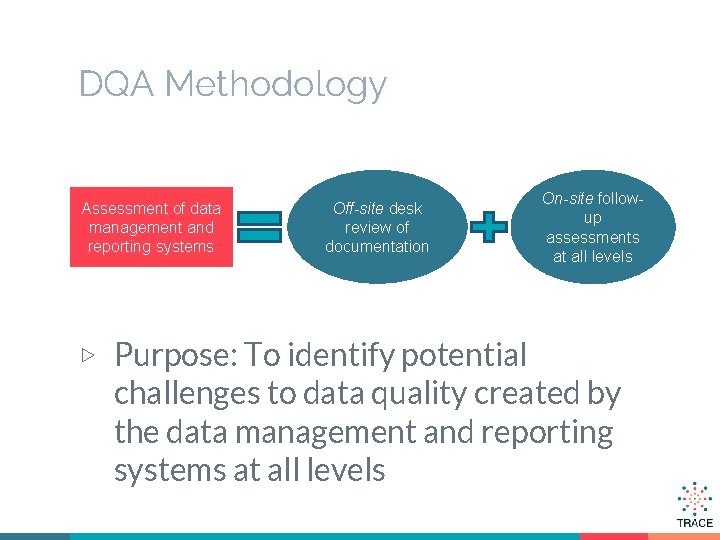
DQA Methodology Assessment of data management and reporting systems Off-site desk review of documentation ▷ Purpose: To identify potential On-site followup assessments at all levels challenges to data quality created by the data management and reporting systems at all levels
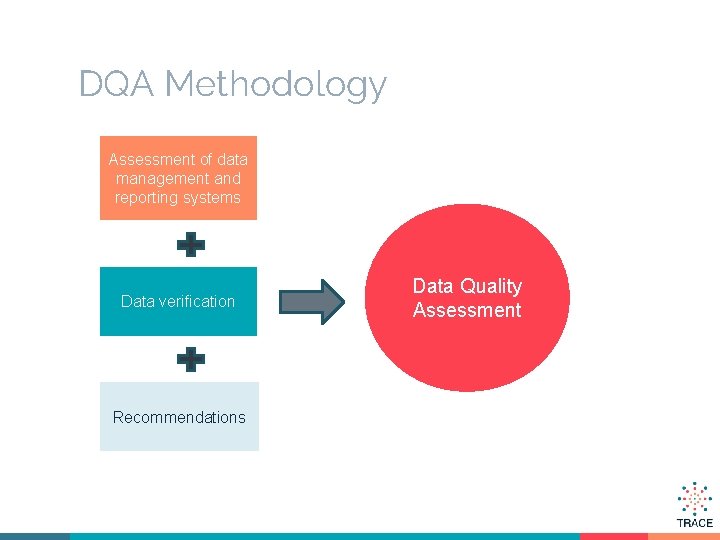
DQA Methodology Assessment of data management and reporting systems Data verification Recommendations Data Quality Assessment
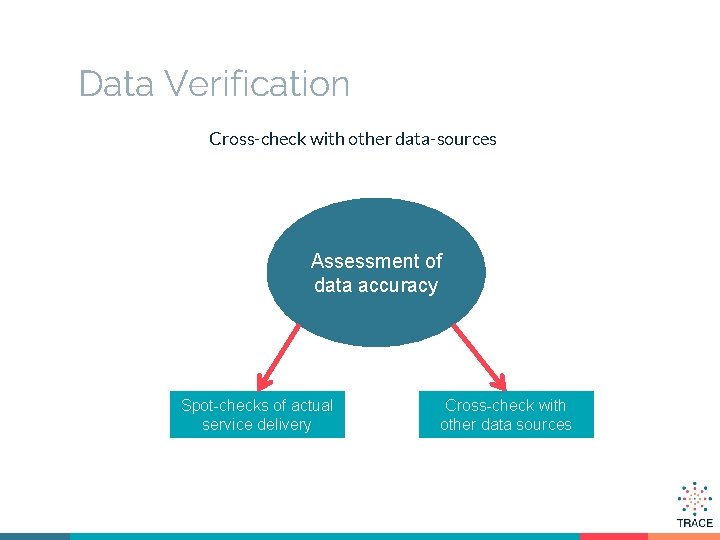
Data Verification Cross-check with other data-sources Assessment of data accuracy Spot-checks of actual service delivery Cross-check with other data sources
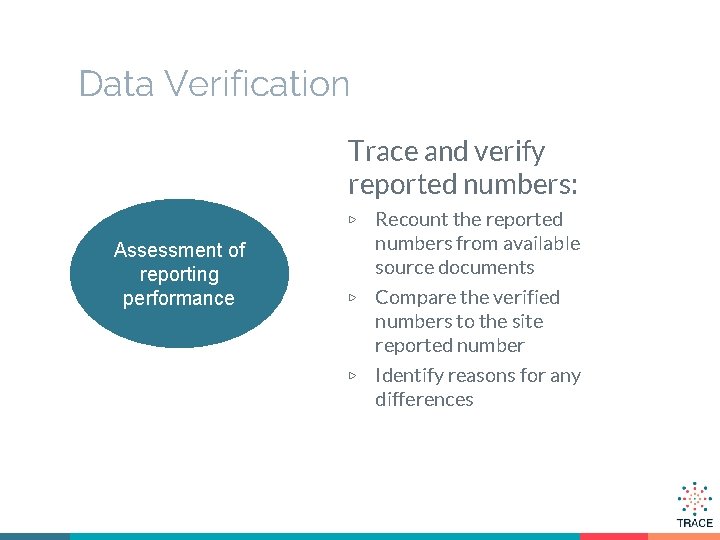
Data Verification Trace and verify reported numbers: Recount the reported numbers from available source documents ▷ Compare the verified numbers to the site reported number ▷ Identify reasons for any differences ▷ Assessment of reporting performance
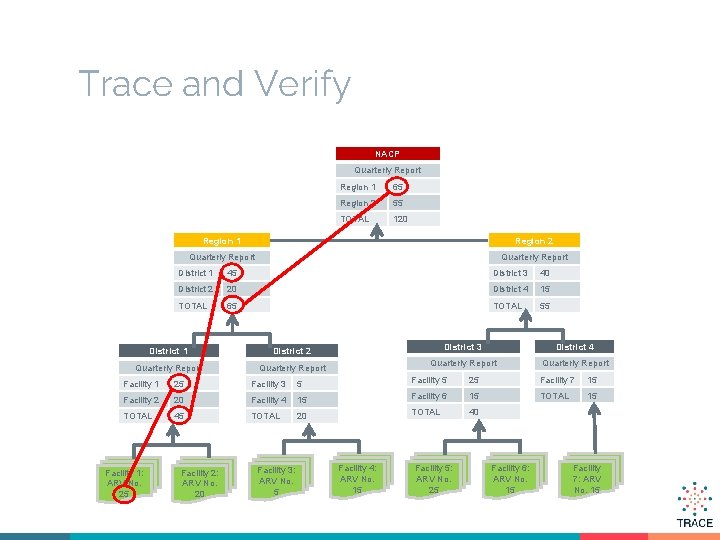
Trace and Verify NACP Quarterly Report Region 1 65 Region 2 55 TOTAL 120 Region 1 Region 2 Quarterly Report District 1 45 District 3 40 District 2 20 District 4 15 TOTAL 65 TOTAL 55 District 1 District 2 District 3 District 4 Quarterly Report Facility 1 25 Facility 3 5 Facility 5 25 Facility 7 15 Facility 2 20 Facility 4 15 Facility 6 15 TOTAL 45 TOTAL 20 TOTAL 40 Facility 1: ARV No. 25 Facility 2: ARV No. 20 Facility 3: ARV No. 5 Facility 4: ARV No. 15 Facility 5: ARV No. 25 Facility 6: ARV No. 15 Facility 7: ARV No. 15
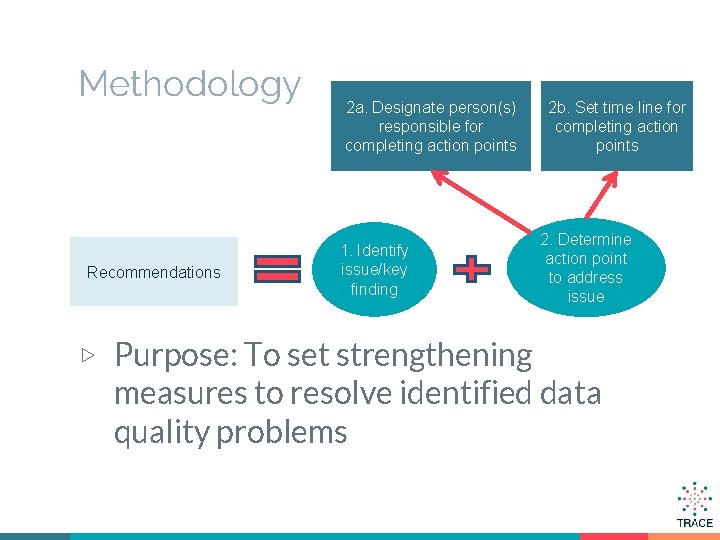
Methodology Recommendations 2 a. Designate person(s) responsible for completing action points 1. Identify issue/key finding ▷ Purpose: To set strengthening 2 b. Set time line for completing action points 2. Determine action point to address issue measures to resolve identified data quality problems
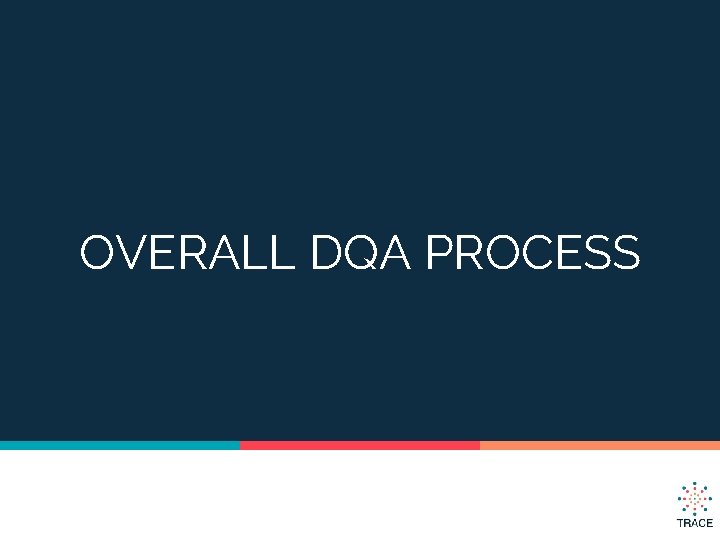
OVERALL DQA PROCESS
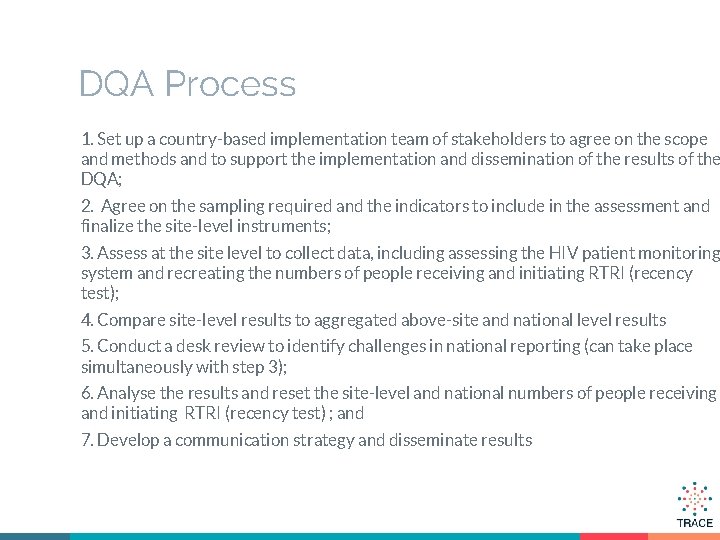
DQA Process 1. Set up a country-based implementation team of stakeholders to agree on the scope and methods and to support the implementation and dissemination of the results of the DQA; 2. Agree on the sampling required and the indicators to include in the assessment and finalize the site-level instruments; 3. Assess at the site level to collect data, including assessing the HIV patient monitoring system and recreating the numbers of people receiving and initiating RTRI (recency test); 4. Compare site-level results to aggregated above-site and national level results 5. Conduct a desk review to identify challenges in national reporting (can take place simultaneously with step 3); 6. Analyse the results and reset the site-level and national numbers of people receiving and initiating RTRI (recency test) ; and 7. Develop a communication strategy and disseminate results
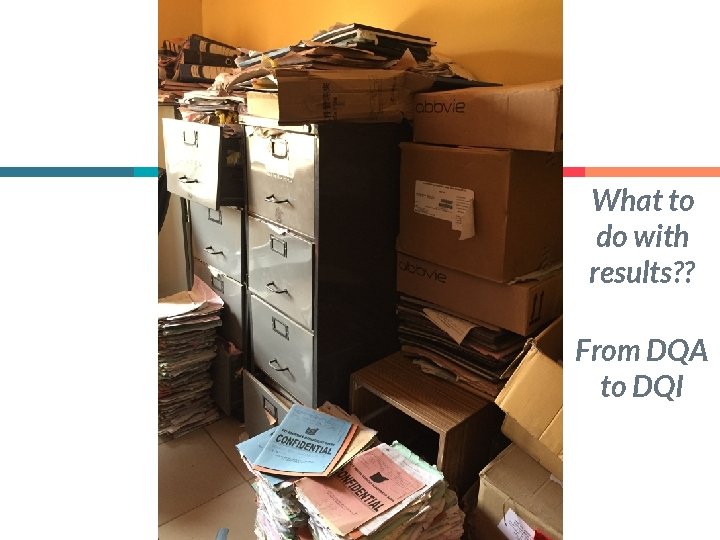
What to do with results? ? From DQA to DQI
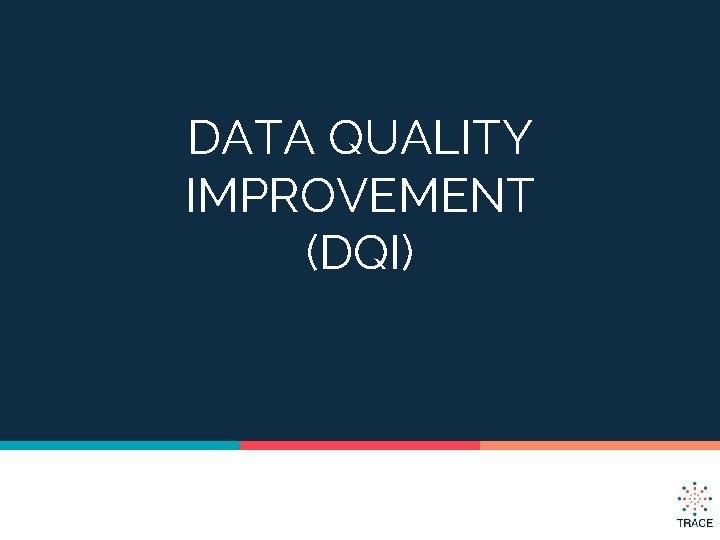
DATA QUALITY IMPROVEMENT (DQI)
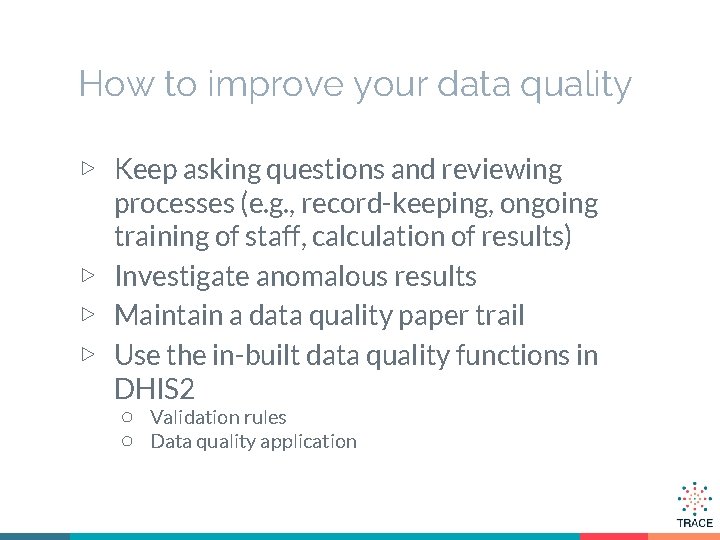
How to improve your data quality ▷ Keep asking questions and reviewing processes (e. g. , record-keeping, ongoing training of staff, calculation of results) ▷ Investigate anomalous results ▷ Maintain a data quality paper trail ▷ Use the in-built data quality functions in DHIS 2 ○ Validation rules ○ Data quality application
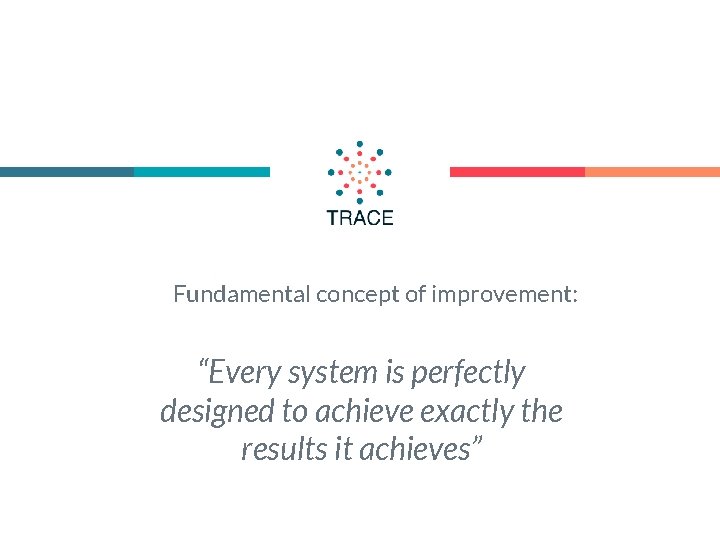
Fundamental concept of improvement: “Every system is perfectly designed to achieve exactly the results it achieves”
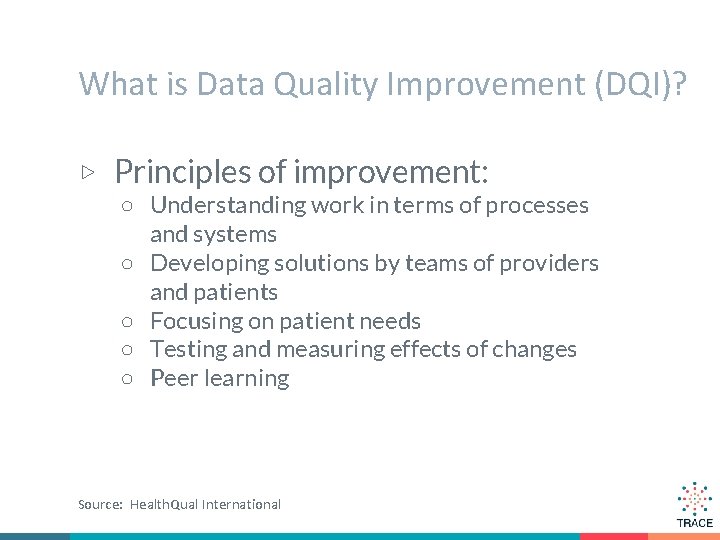
What is Data Quality Improvement (DQI)? ▷ Principles of improvement: ○ Understanding work in terms of processes and systems ○ Developing solutions by teams of providers and patients ○ Focusing on patient needs ○ Testing and measuring effects of changes ○ Peer learning Source: Health. Qual International
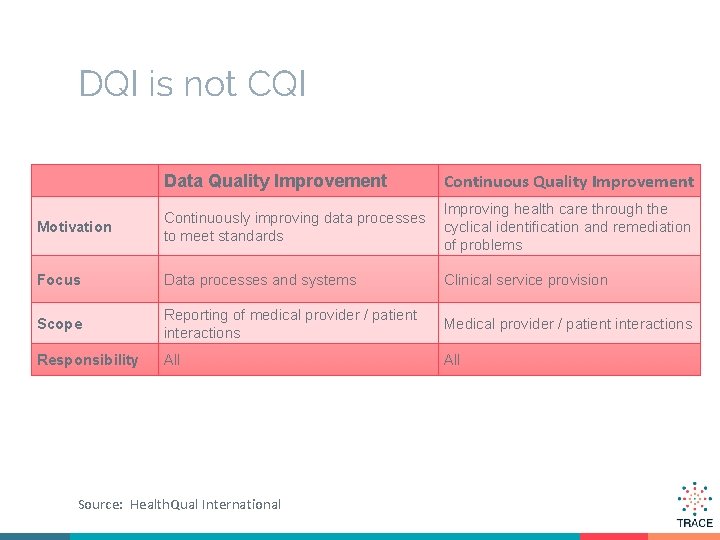
DQI is not CQI Data Quality Improvement Continuous Quality Improvement Motivation Continuously improving data processes to meet standards Improving health care through the cyclical identification and remediation of problems Focus Data processes and systems Clinical service provision Scope Reporting of medical provider / patient interactions Medical provider / patient interactions Responsibility All Source: Health. Qual International
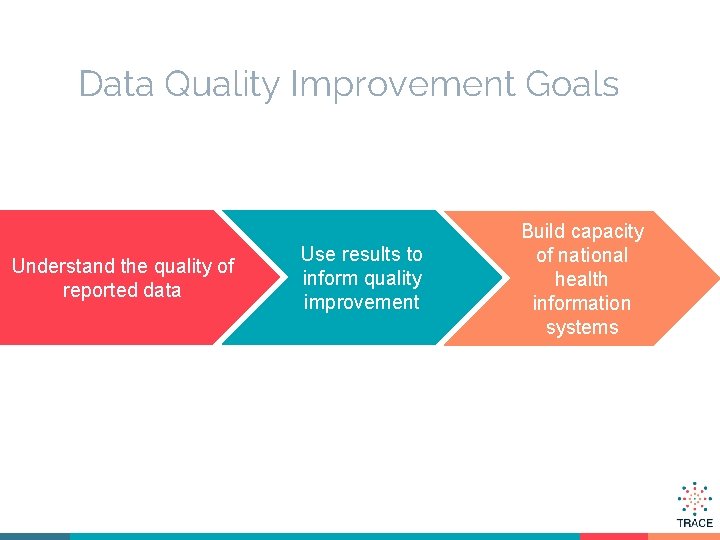
Data Quality Improvement Goals Understand the quality of reported data Use results to inform quality improvement Build capacity of national health information systems
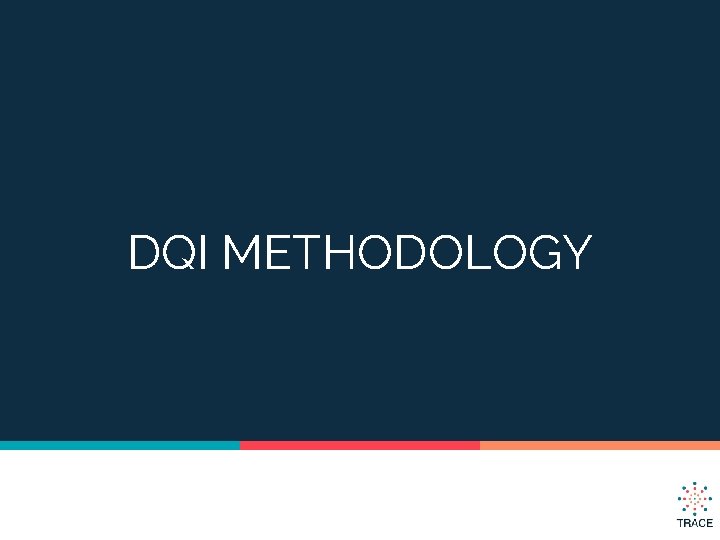
DQI METHODOLOGY
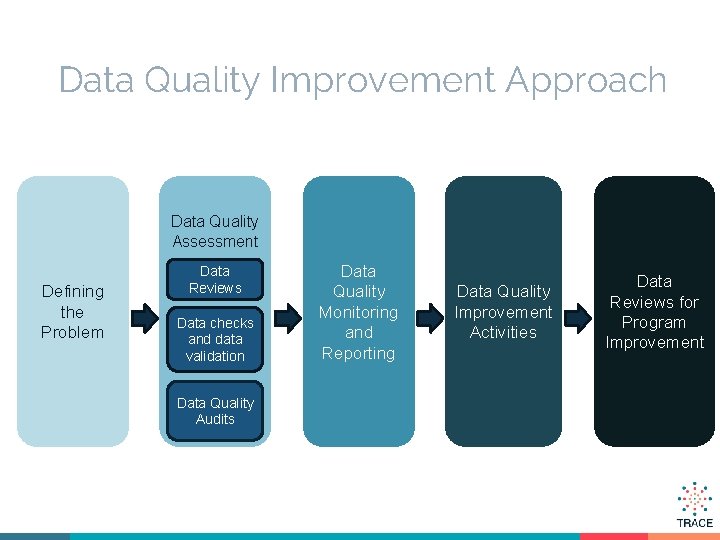
Data Quality Improvement Approach Data Quality Assessment Defining the Problem Data Reviews Data checks and data validation Data Quality Audits Data Quality Monitoring and Reporting Data Quality Improvement Activities Data Reviews for Program Improvement
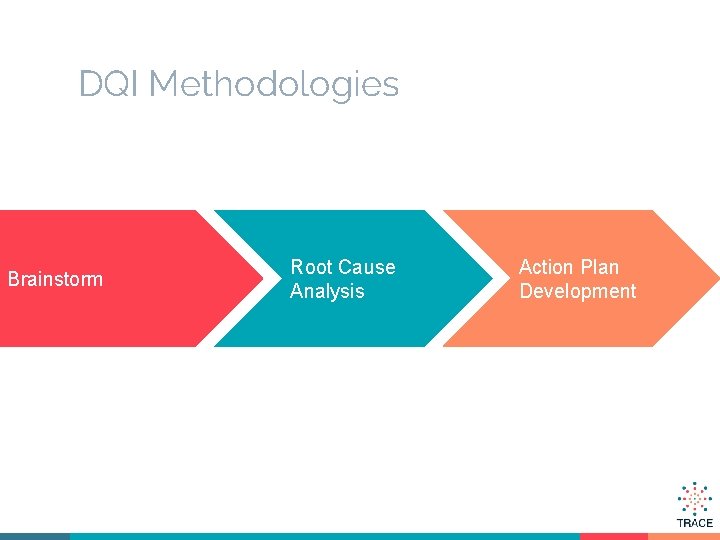
DQI Methodologies Brainstorm Root Cause Analysis Action Plan Development
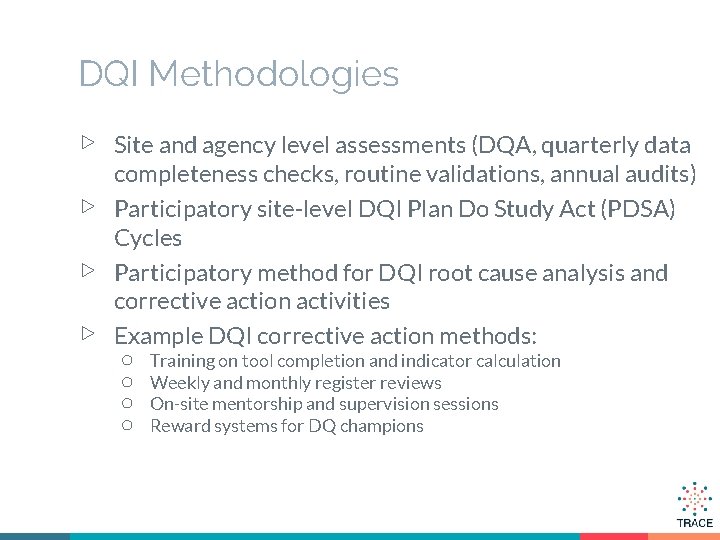
DQI Methodologies ▷ ▷ Site and agency level assessments (DQA, quarterly data completeness checks, routine validations, annual audits) Participatory site-level DQI Plan Do Study Act (PDSA) Cycles Participatory method for DQI root cause analysis and corrective action activities Example DQI corrective action methods: ○ Training on tool completion and indicator calculation ○ Weekly and monthly register reviews ○ On-site mentorship and supervision sessions ○ Reward systems for DQ champions
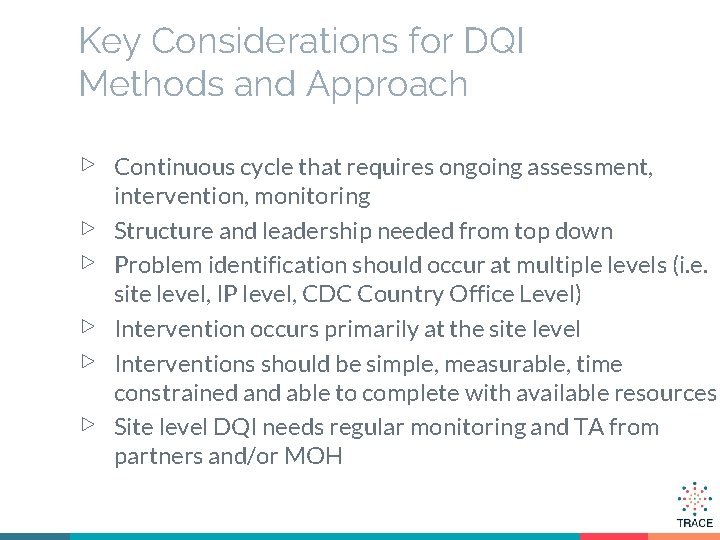
Key Considerations for DQI Methods and Approach ▷ ▷ ▷ Continuous cycle that requires ongoing assessment, intervention, monitoring Structure and leadership needed from top down Problem identification should occur at multiple levels (i. e. site level, IP level, CDC Country Office Level) Intervention occurs primarily at the site level Interventions should be simple, measurable, time constrained and able to complete with available resources Site level DQI needs regular monitoring and TA from partners and/or MOH
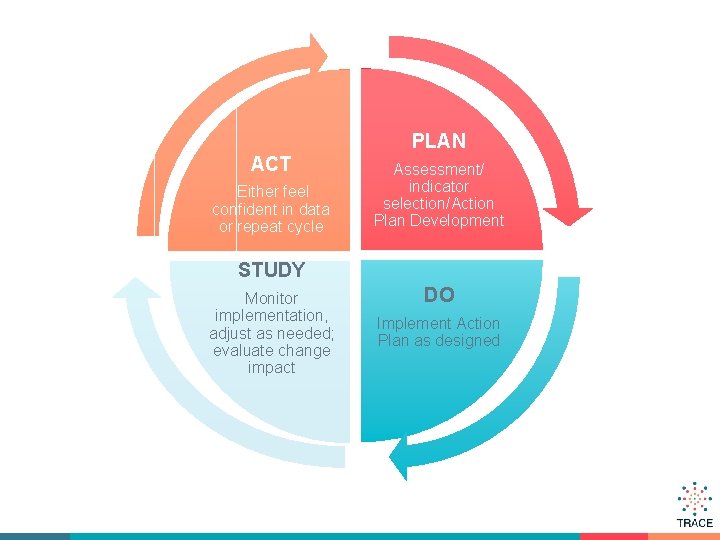
PLAN ACT Either feel confident in data or repeat cycle Assessment/ indicator selection/Action Plan Development STUDY Monitor implementation, adjust as needed; evaluate change impact DO Implement Action Plan as designed
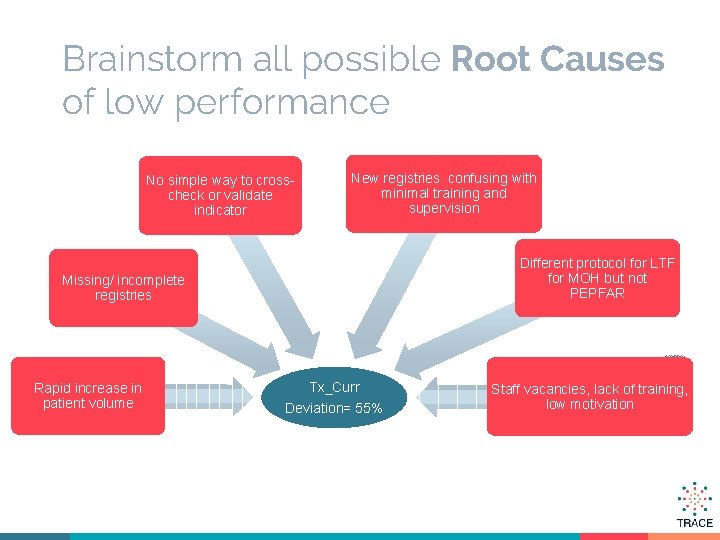
Brainstorm all possible Root Causes of low performance No simple way to crosscheck or validate indicator New registries confusing with minimal training and supervision Different protocol for LTF for MOH but not PEPFAR Missing/ incomplete registries 6/3/2021 Rapid increase in patient volume Tx_Curr Deviation= 55% Staff vacancies, lack of training, low motivation
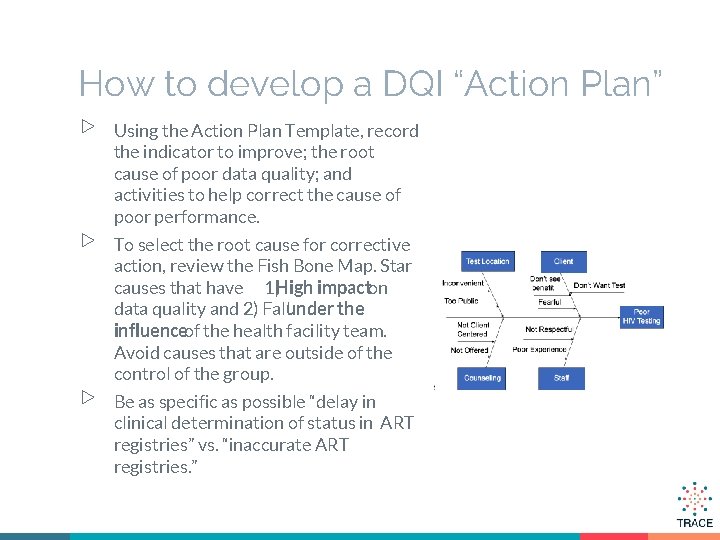
How to develop a DQI “Action Plan” ▷ ▷ ▷ Using the Action Plan Template, record the indicator to improve; the root cause of poor data quality; and activities to help correct the cause of poor performance. To select the root cause for corrective action, review the Fish Bone Map. Star causes that have 1)High impacton data quality and 2) Fallunder the influenceof the health facility team. Avoid causes that are outside of the control of the group. Be as specific as possible “delay in clinical determination of status in ART registries” vs. “inaccurate ART registries. ”
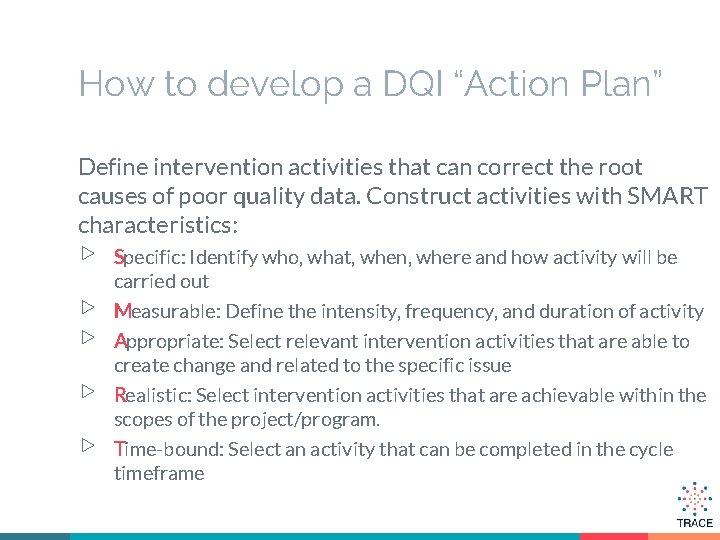
How to develop a DQI “Action Plan” Define intervention activities that can correct the root causes of poor quality data. Construct activities with SMART characteristics: ▷ ▷ ▷ Specific: Identify who, what, when, where and how activity will be carried out Measurable: Define the intensity, frequency, and duration of activity Appropriate: Select relevant intervention activities that are able to create change and related to the specific issue Realistic: Select intervention activities that are achievable within the scopes of the project/program. Time-bound: Select an activity that can be completed in the cycle timeframe
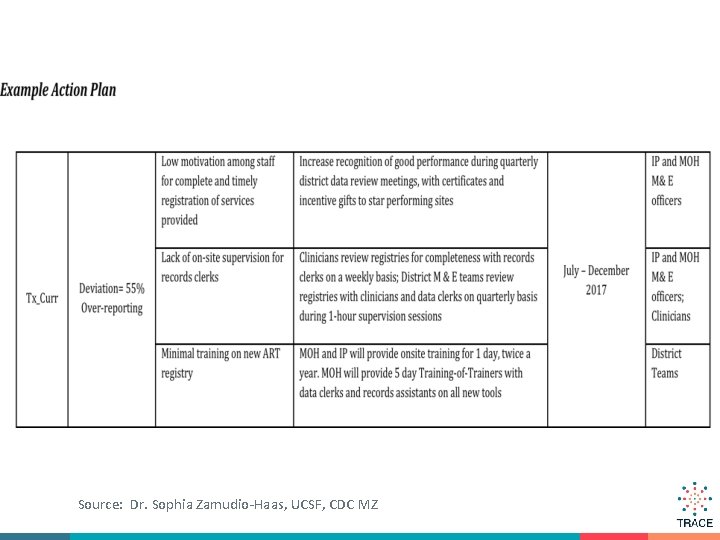
Source: Dr. Sophia Zamudio-Haas, UCSF, CDC MZ
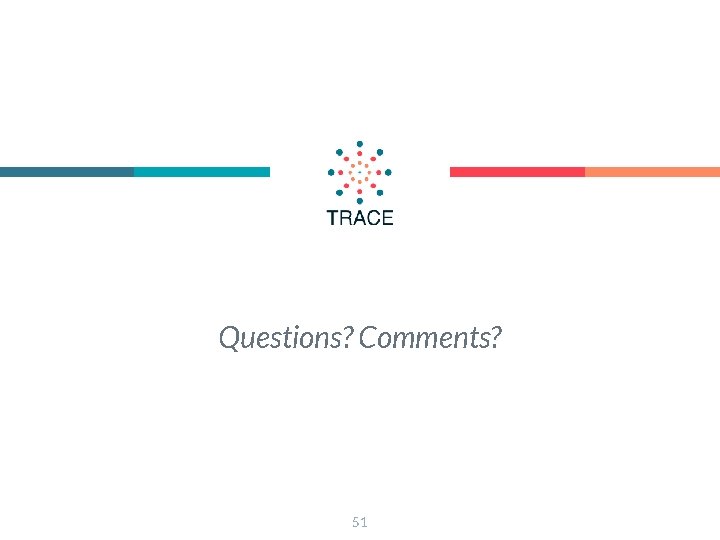
Questions? Comments? 51
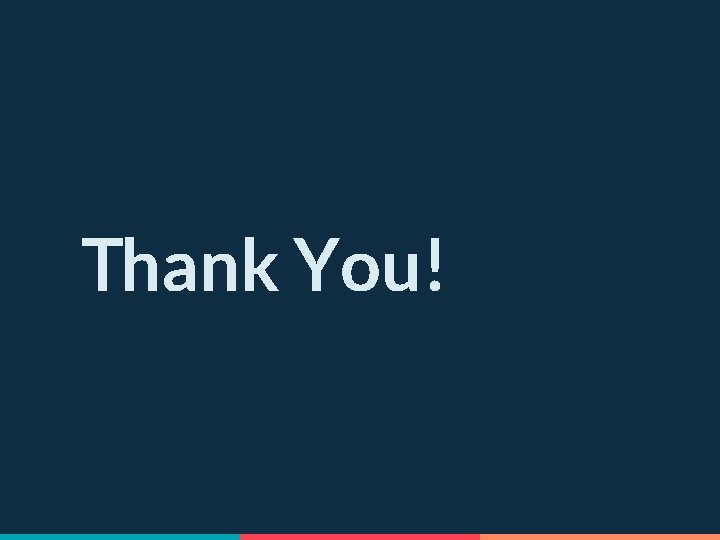
Thank You!
Good morning formal greetings
Xxxx v
Xxxx.xx
X xxx
Hello hello hello how are you
Hello what's your name jenny and you
Horizontal trace
Workforce data quality initiative
Mqii
Hello my friend
"good afternoon everyone"
Birchfield nursery
Hello the sun is shining
Good morning
Nanny shine has got
Hello hello to everyone it's english time
Index.php?page=
Hello i am muzzy
Hello hello will i slide
I am peter pan
Open data initiative
Data quality and data cleaning an overview
Data quality and data cleaning an overview
Content analysis is a type of secondary data analysis
Data quality and data cleaning an overview
Perform quality assurance
Pmp quality management
Pmp gold plating
Define seminar in nursing management
Compliance vs quality
Basic concepts of quality
Gurus of total quality management
Crosby's fourteen steps to quality improvement
Old quality vs new quality
Leave no trace canada
Tracer un arc surbaissé
Steam tracing in piping
Interrupted medulla
Tracing evidence examples
Trace evidence examples
Box trace recursion
Box trace recursion
Eugene hecht
X-trace: a pervasive network tracing framework
Outdoor code and leave no trace
Trainable ocr
Tracé du canal de provence
Cub scout leave no trace award
Trace scheduling
The accounting issue(s) in the crazy eddie case were:
Vouch vs trace
Vouch vs trace
Simplest sorting algorithm