The ATLAS Trigger HighLevel Trigger Commissioning and Operation
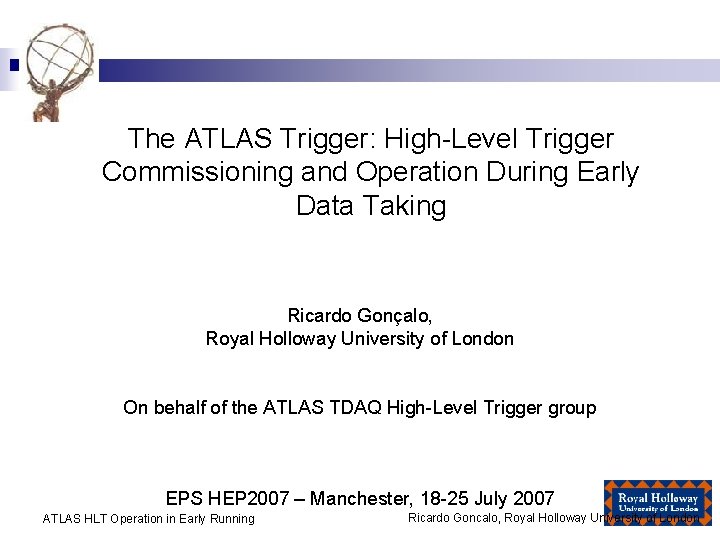
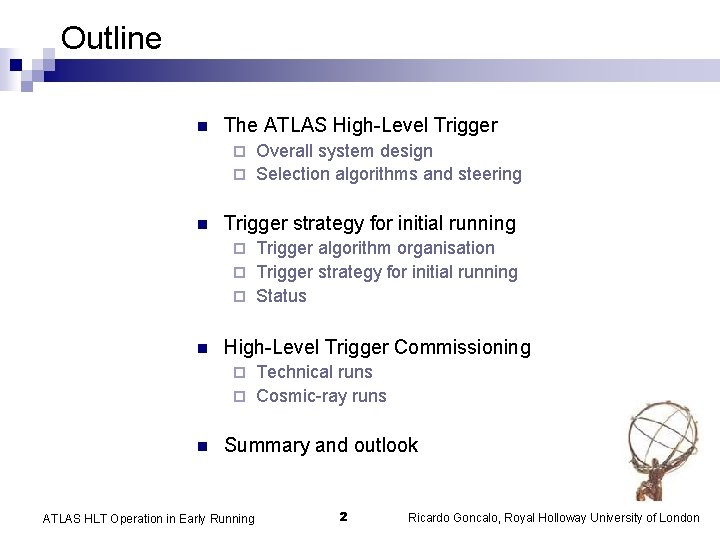
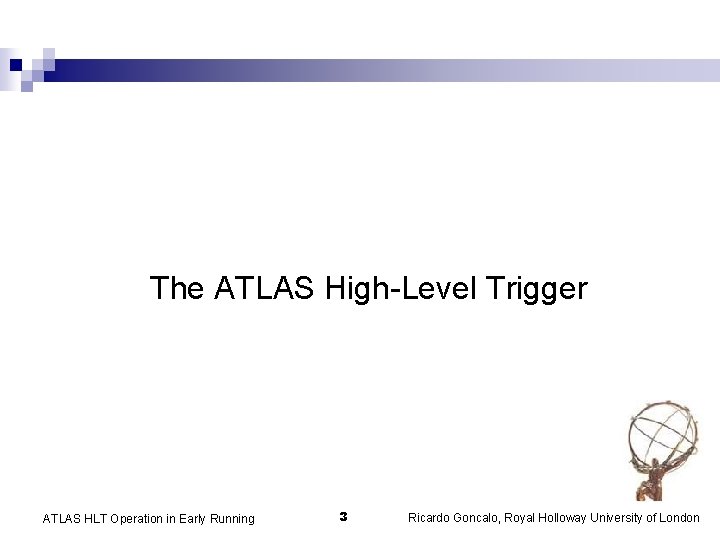
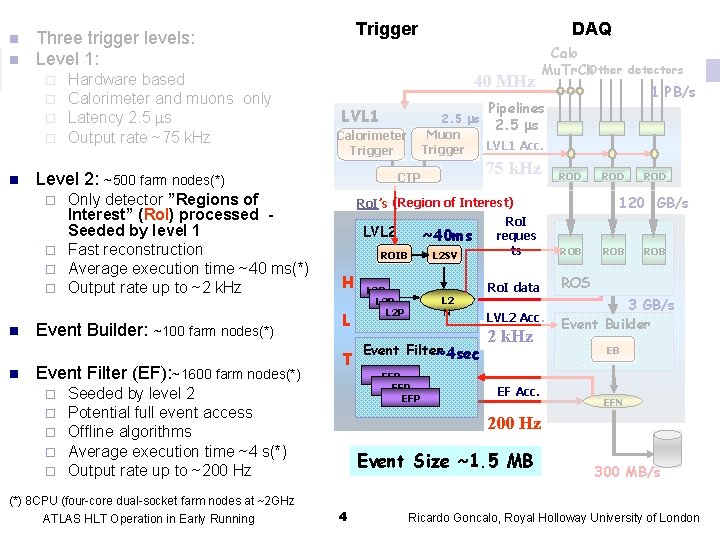
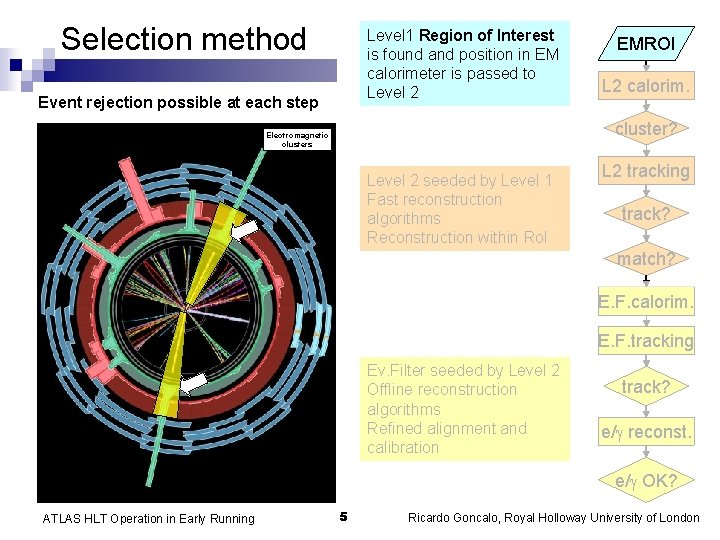
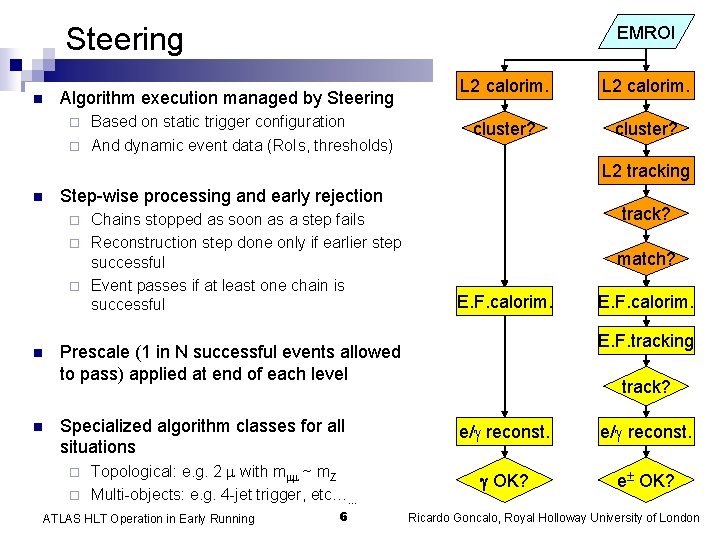
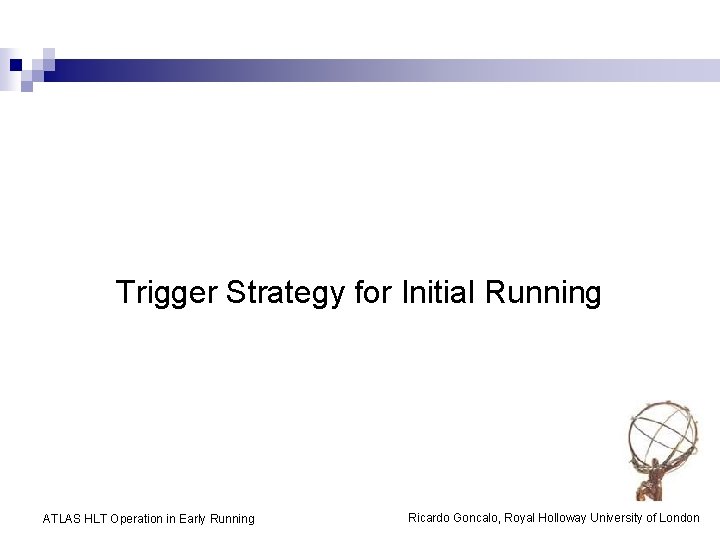
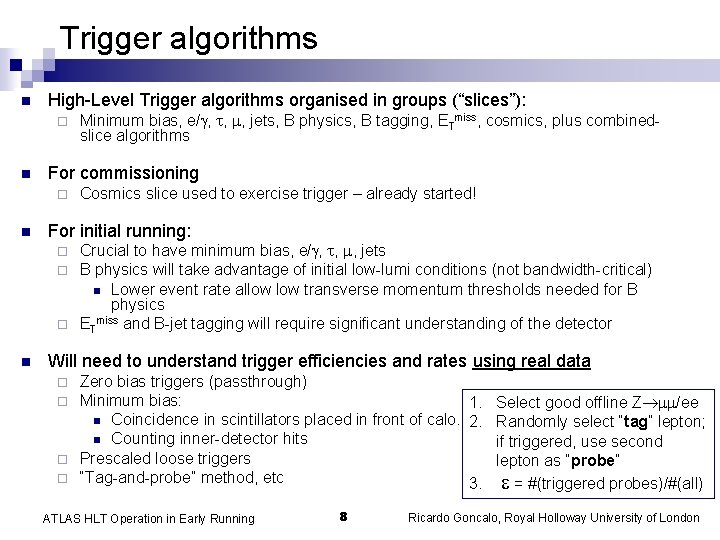
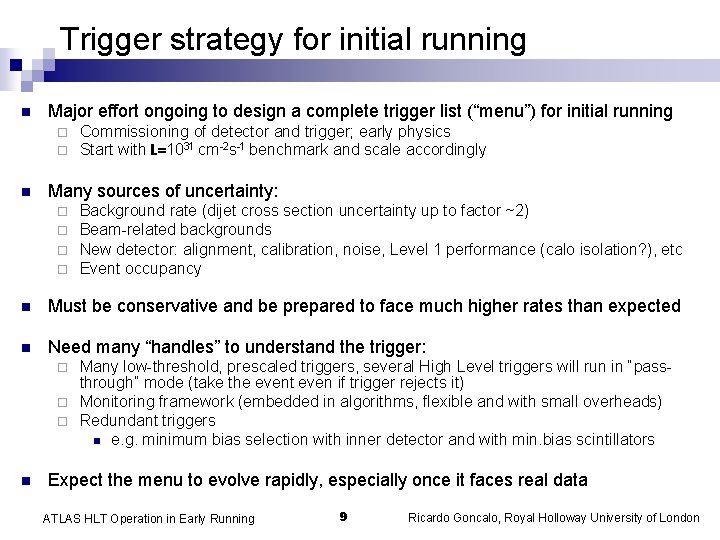
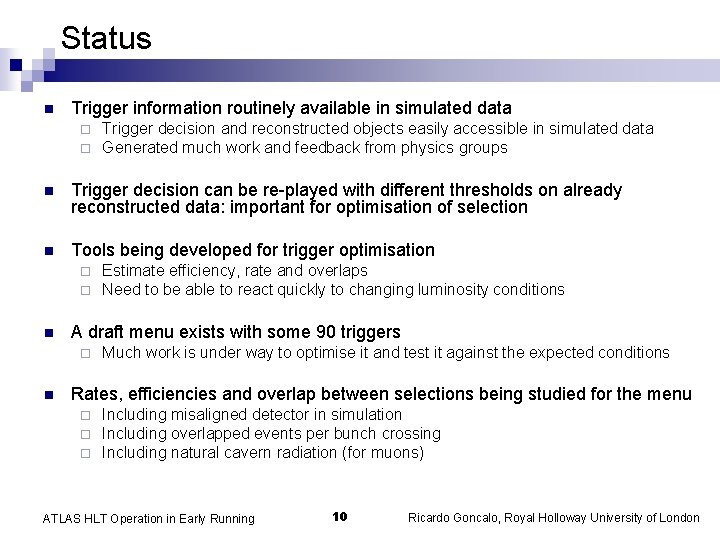
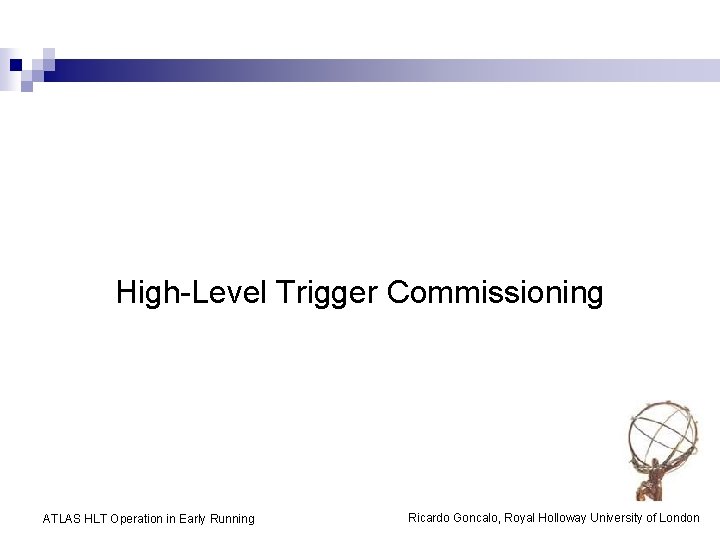
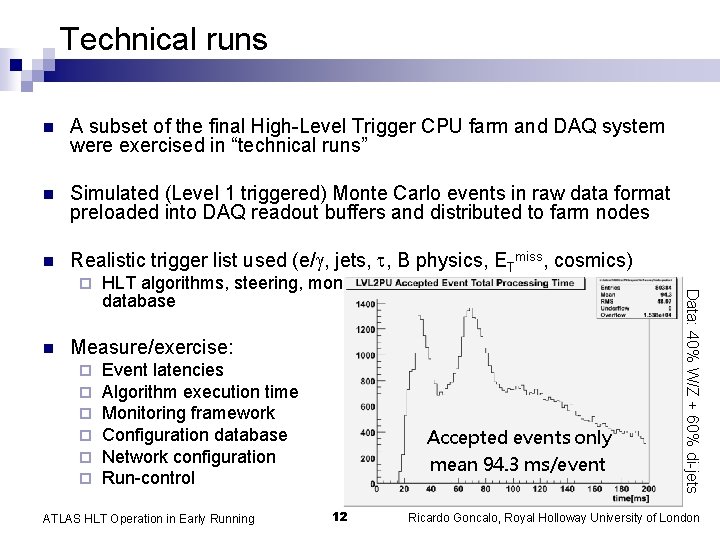
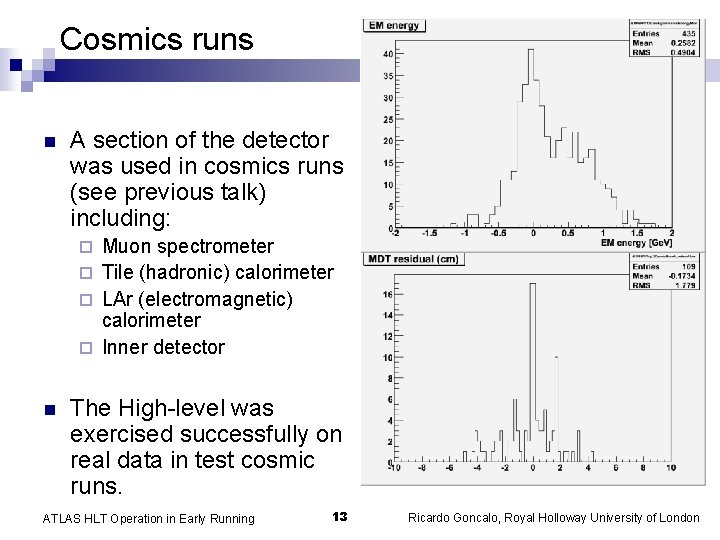
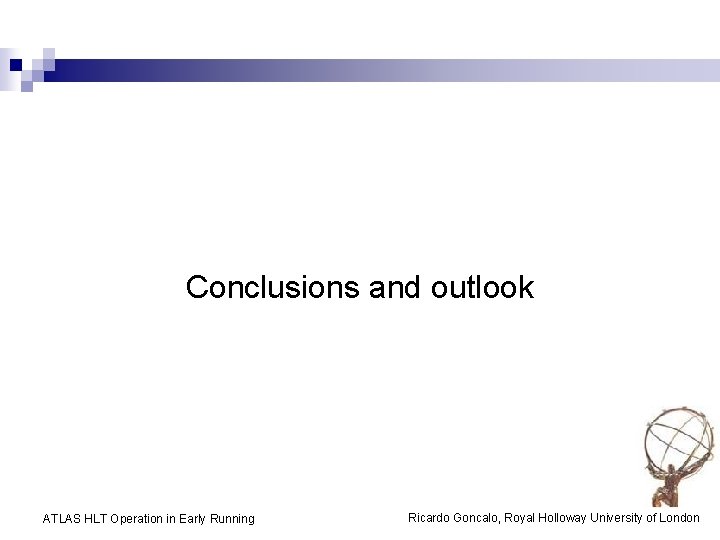
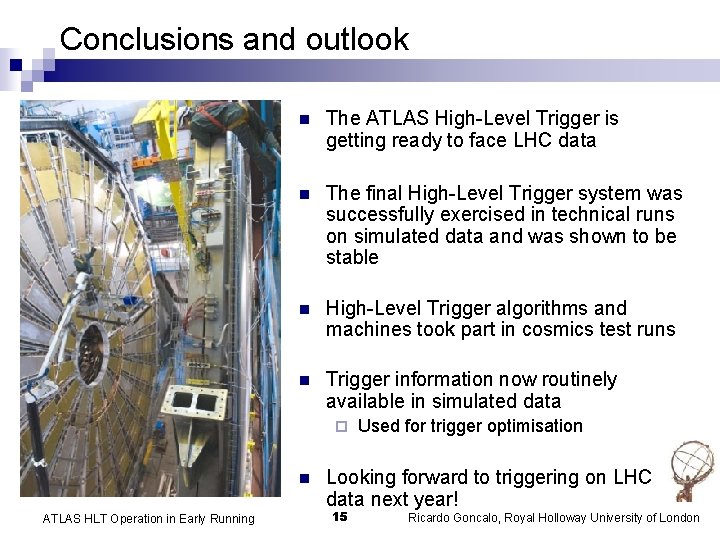
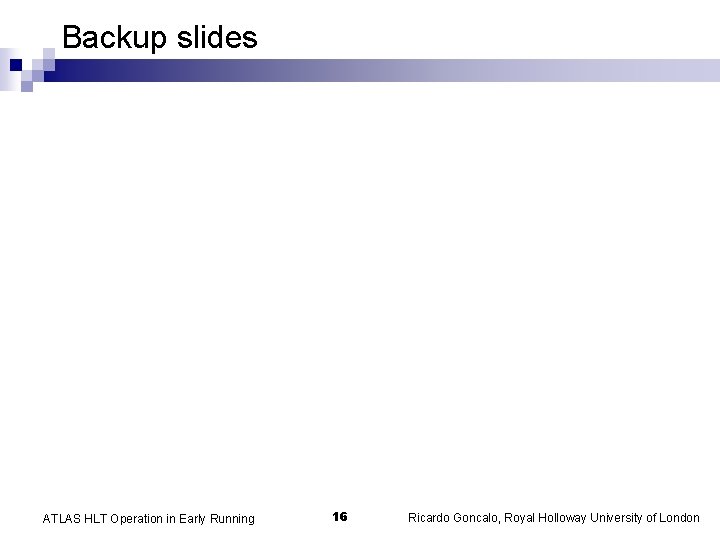
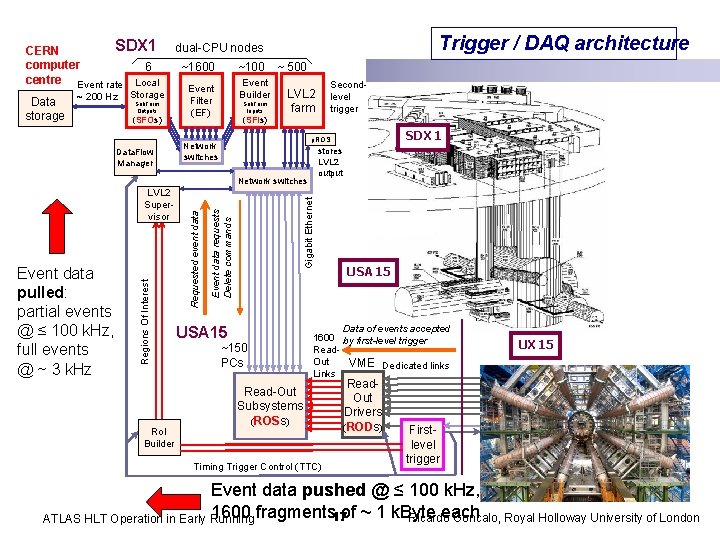
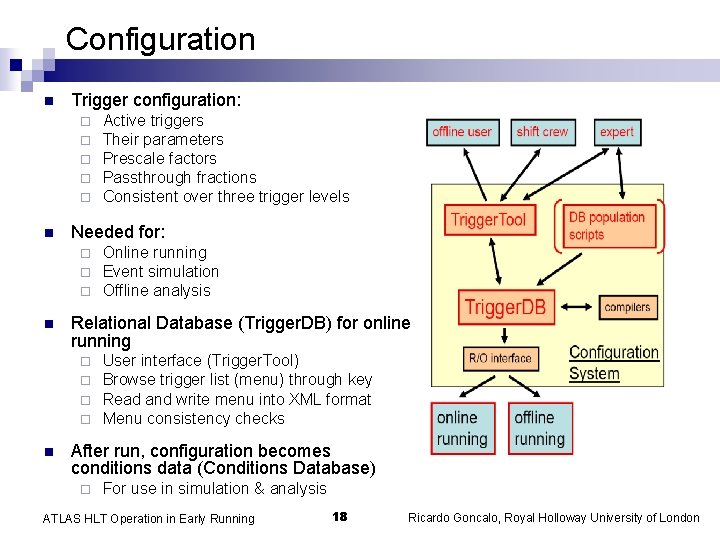
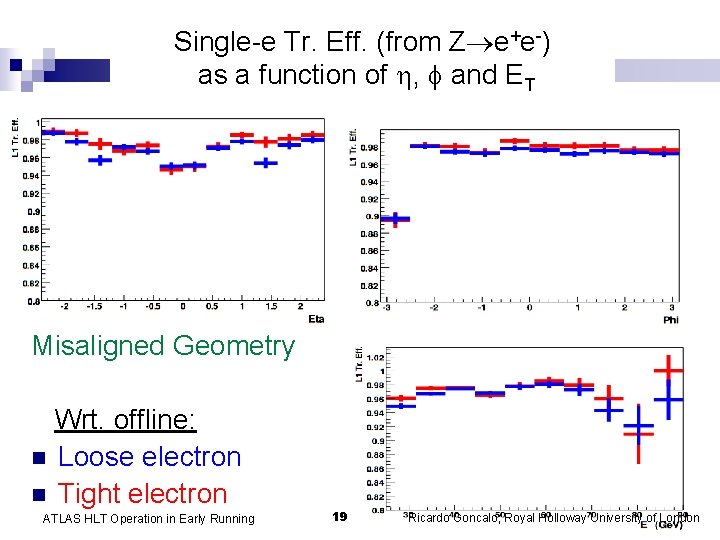
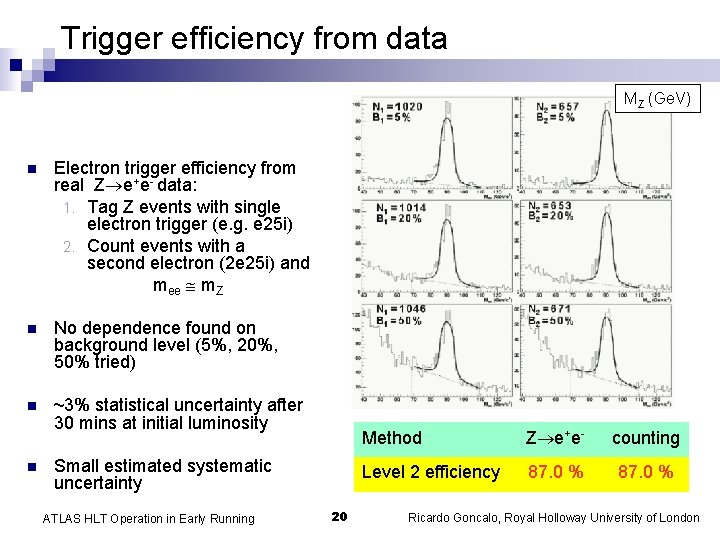
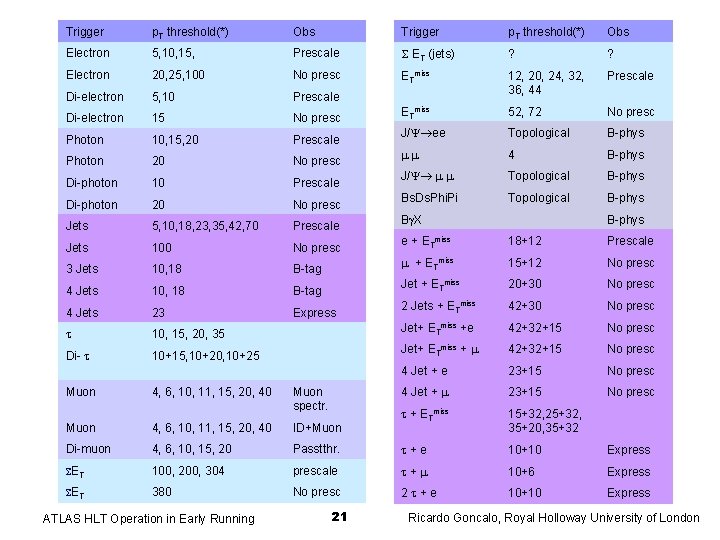
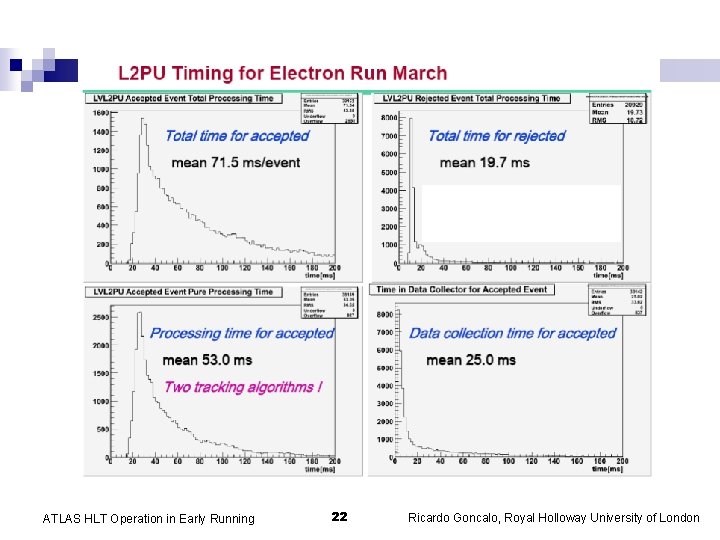
- Slides: 22
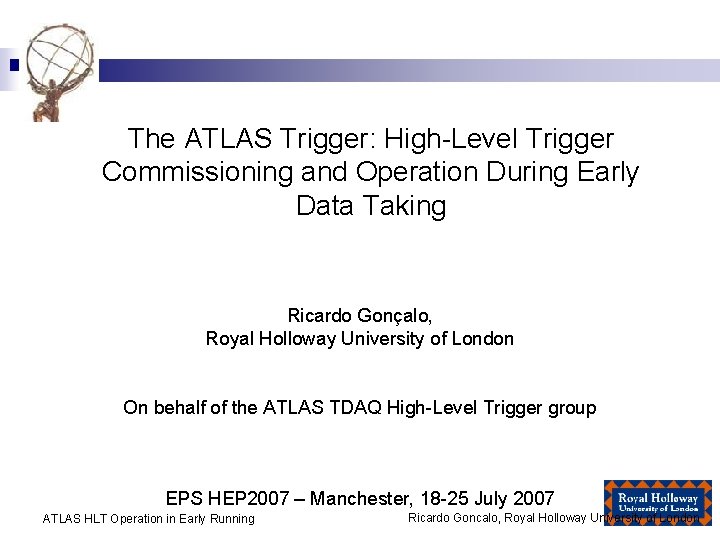
The ATLAS Trigger: High-Level Trigger Commissioning and Operation During Early Data Taking Ricardo Gonçalo, Royal Holloway University of London On behalf of the ATLAS TDAQ High-Level Trigger group EPS HEP 2007 – Manchester, 18 -25 July 2007 ATLAS HLT Operation in Early Running Ricardo Goncalo, Royal Holloway University of London
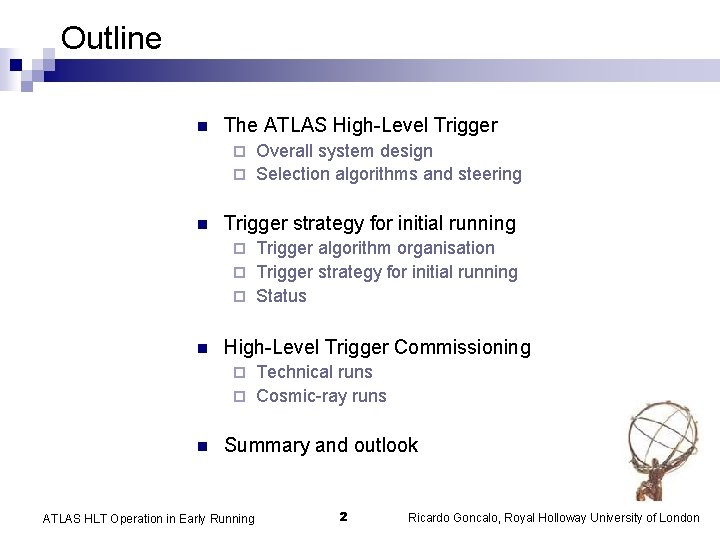
Outline n The ATLAS High-Level Trigger Overall system design ¨ Selection algorithms and steering ¨ n Trigger strategy for initial running Trigger algorithm organisation ¨ Trigger strategy for initial running ¨ Status ¨ n High-Level Trigger Commissioning Technical runs ¨ Cosmic-ray runs ¨ n Summary and outlook ATLAS HLT Operation in Early Running 2 Ricardo Goncalo, Royal Holloway University of London
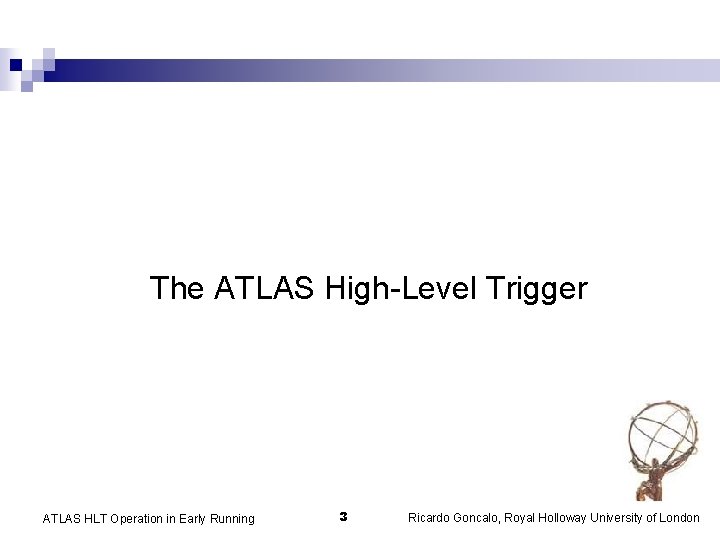
The ATLAS High-Level Trigger ATLAS HLT Operation in Early Running 3 Ricardo Goncalo, Royal Holloway University of London
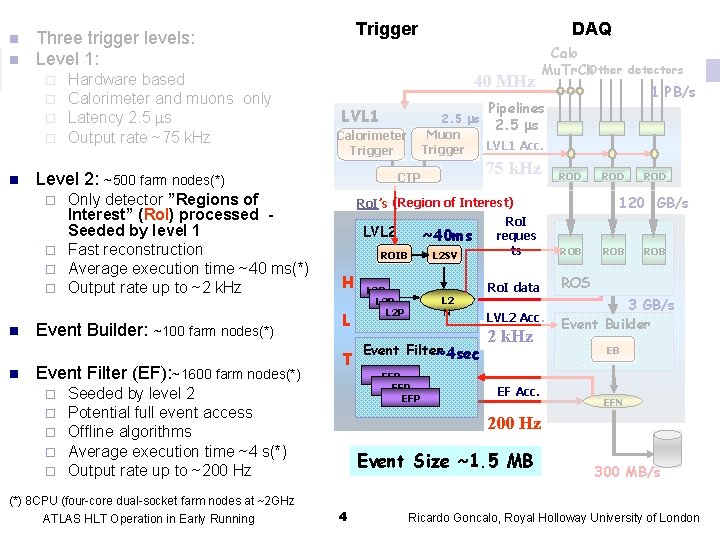
n n ¨ ¨ n Trigger Three trigger levels: Level 1: Hardware based Calorimeter and muons only Latency 2. 5 s Output rate ~75 k. Hz 40 MHz n n Event Builder: ~100 farm nodes(*) Event Filter (EF): ~1600 farm nodes(*) ¨ ¨ ¨ Ro. I’s (Region of Interest) Ro. I LVL 2 ~40 ms reques ts ROIB L 2 SV L T L 2 P L 2 N Event Filter ~4 sec EFP EFP Seeded by level 2 Potential full event access Offline algorithms Average execution time ~4 s(*) Output rate up to ~200 Hz (*) 8 CPU (four-core dual-socket farm nodes at ~2 GHz ATLAS HLT Operation in Early Running 75 k. Hz CTP H 1 PB/s 2. 5 ms Muon LVL 1 Acc. Trigger Calorimeter Trigger ¨ Calo Mu. Tr. Ch. Other detectors Pipelines LVL 1 Level 2: ~500 farm nodes(*) Only detector ”Regions of Interest” (Ro. I) processed Seeded by level 1 ¨ Fast reconstruction ¨ Average execution time ~40 ms(*) ¨ Output rate up to ~2 k. Hz DAQ Ro. I data LVL 2 Acc. 2 k. Hz EF Acc. ROD ROD 120 GB/s ROB ROB ROS 3 GB/s Event Builder EB EFN 200 Hz Event Size ~1. 5 MB 4 300 MB/s Ricardo Goncalo, Royal Holloway University of London
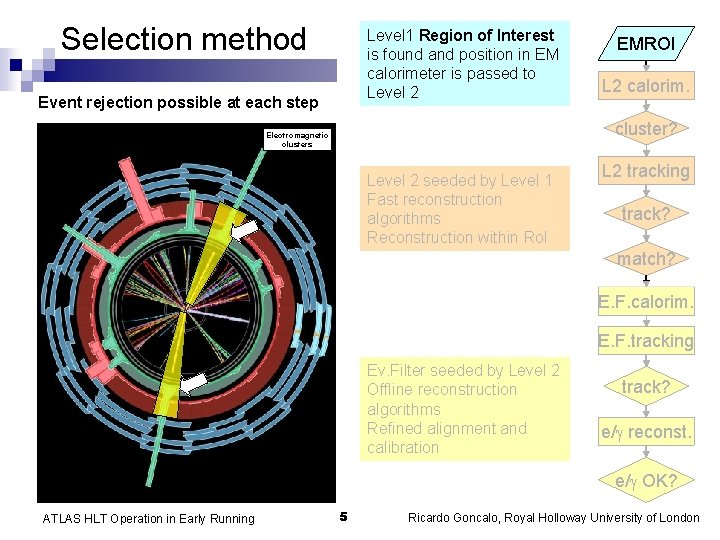
Selection method Level 1 Region of Interest is found and position in EM calorimeter is passed to Level 2 Event rejection possible at each step EMROI L 2 calorim. cluster? Electromagnetic clusters Level 2 seeded by Level 1 Fast reconstruction algorithms Reconstruction within Ro. I L 2 tracking track? match? E. F. calorim. E. F. tracking Ev. Filter seeded by Level 2 Offline reconstruction algorithms Refined alignment and calibration track? e/ reconst. e/ OK? ATLAS HLT Operation in Early Running 5 Ricardo Goncalo, Royal Holloway University of London
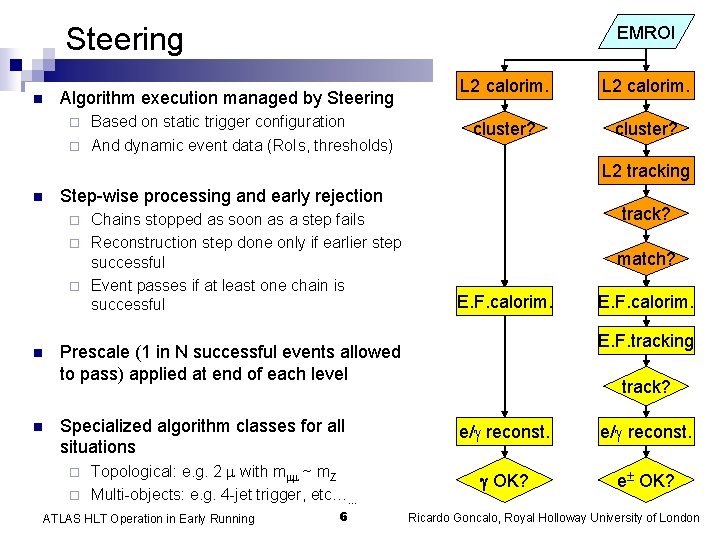
Steering n EMROI Algorithm execution managed by Steering Based on static trigger configuration ¨ And dynamic event data (Ro. Is, thresholds) ¨ L 2 calorim. cluster? L 2 tracking n Step-wise processing and early rejection Chains stopped as soon as a step fails ¨ Reconstruction step done only if earlier step successful ¨ Event passes if at least one chain is successful track? ¨ n n match? E. F. calorim. E. F. tracking Prescale (1 in N successful events allowed to pass) applied at end of each level Specialized algorithm classes for all situations Topological: e. g. 2 with m ~ m. Z ¨ Multi-objects: e. g. 4 -jet trigger, etc…… ¨ ATLAS HLT Operation in Early Running 6 E. F. calorim. track? e/ reconst. OK? e OK? Ricardo Goncalo, Royal Holloway University of London
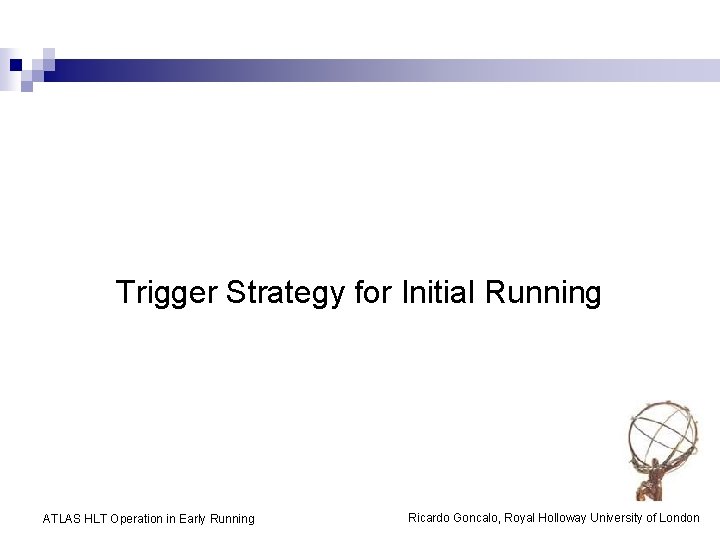
Trigger Strategy for Initial Running ATLAS HLT Operation in Early Running Ricardo Goncalo, Royal Holloway University of London
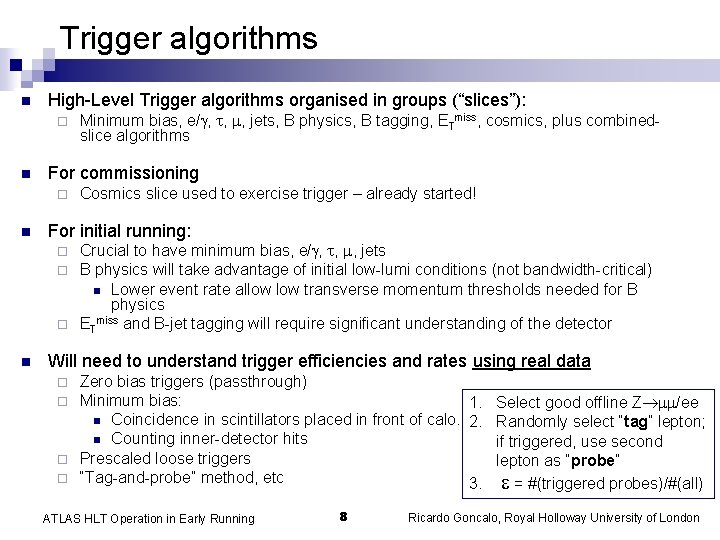
Trigger algorithms n High-Level Trigger algorithms organised in groups (“slices”): ¨ n For commissioning ¨ n Minimum bias, e/ , , , jets, B physics, B tagging, ETmiss, cosmics, plus combinedslice algorithms Cosmics slice used to exercise trigger – already started! For initial running: Crucial to have minimum bias, e/ , , , jets B physics will take advantage of initial low-lumi conditions (not bandwidth-critical) n Lower event rate allow transverse momentum thresholds needed for B physics ¨ ETmiss and B-jet tagging will require significant understanding of the detector ¨ ¨ n Will need to understand trigger efficiencies and rates using real data Zero bias triggers (passthrough) Minimum bias: 1. Select good offline Z /ee n Coincidence in scintillators placed in front of calo. 2. Randomly select “tag” lepton; n Counting inner-detector hits if triggered, use second ¨ Prescaled loose triggers lepton as “probe” ¨ “Tag-and-probe” method, etc 3. = #(triggered probes)/#(all) ¨ ¨ ATLAS HLT Operation in Early Running 8 Ricardo Goncalo, Royal Holloway University of London
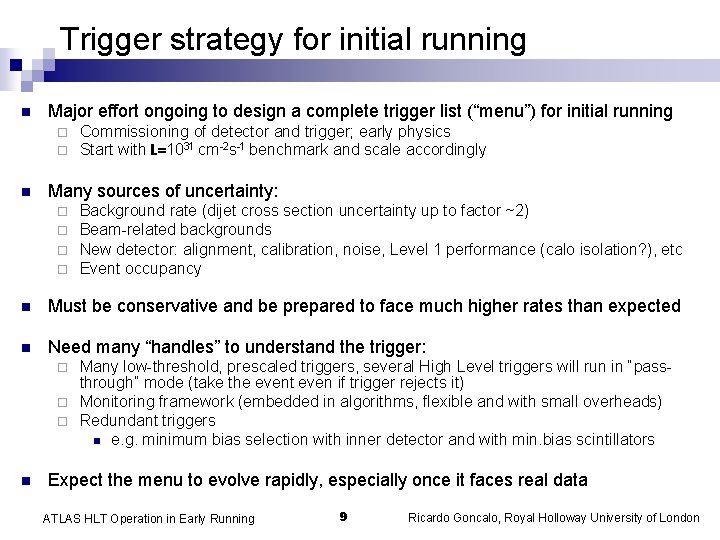
Trigger strategy for initial running n Major effort ongoing to design a complete trigger list (“menu”) for initial running ¨ ¨ n Commissioning of detector and trigger; early physics Start with L=1031 cm-2 s-1 benchmark and scale accordingly Many sources of uncertainty: ¨ ¨ Background rate (dijet cross section uncertainty up to factor ~2) Beam-related backgrounds New detector: alignment, calibration, noise, Level 1 performance (calo isolation? ), etc Event occupancy n Must be conservative and be prepared to face much higher rates than expected n Need many “handles” to understand the trigger: Many low-threshold, prescaled triggers, several High Level triggers will run in “passthrough” mode (take the event even if trigger rejects it) ¨ Monitoring framework (embedded in algorithms, flexible and with small overheads) ¨ Redundant triggers n e. g. minimum bias selection with inner detector and with min. bias scintillators ¨ n Expect the menu to evolve rapidly, especially once it faces real data ATLAS HLT Operation in Early Running 9 Ricardo Goncalo, Royal Holloway University of London
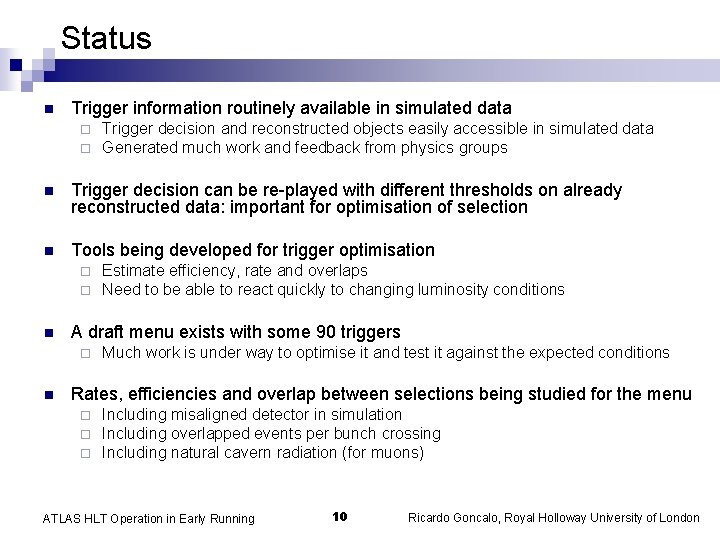
Status n Trigger information routinely available in simulated data ¨ ¨ Trigger decision and reconstructed objects easily accessible in simulated data Generated much work and feedback from physics groups n Trigger decision can be re-played with different thresholds on already reconstructed data: important for optimisation of selection n Tools being developed for trigger optimisation ¨ ¨ n A draft menu exists with some 90 triggers ¨ n Estimate efficiency, rate and overlaps Need to be able to react quickly to changing luminosity conditions Much work is under way to optimise it and test it against the expected conditions Rates, efficiencies and overlap between selections being studied for the menu ¨ ¨ ¨ Including misaligned detector in simulation Including overlapped events per bunch crossing Including natural cavern radiation (for muons) ATLAS HLT Operation in Early Running 10 Ricardo Goncalo, Royal Holloway University of London
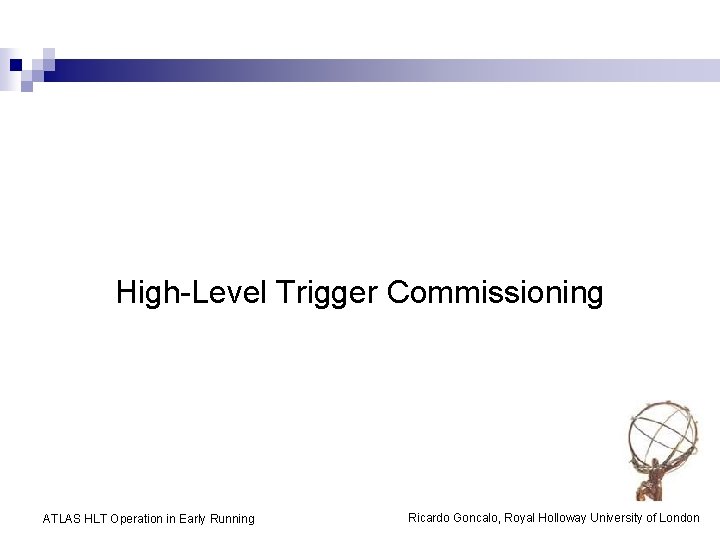
High-Level Trigger Commissioning ATLAS HLT Operation in Early Running Ricardo Goncalo, Royal Holloway University of London
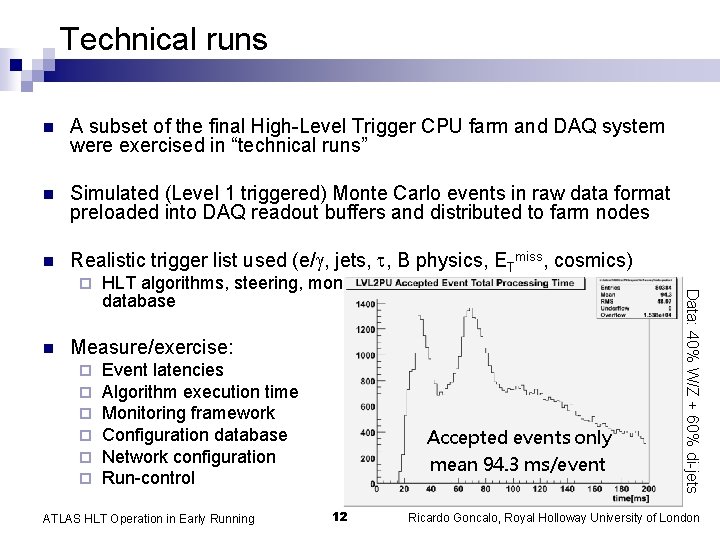
Technical runs n A subset of the final High-Level Trigger CPU farm and DAQ system were exercised in “technical runs” n Simulated (Level 1 triggered) Monte Carlo events in raw data format preloaded into DAQ readout buffers and distributed to farm nodes n Realistic trigger list used (e/ , jets, , B physics, ETmiss, cosmics) n HLT algorithms, steering, monitoring infrastructure, configuration database • Mean 31. 5 ms/event • 98% rejection @ L 2 • Average 40 ms/ev available Measure/exercise: ¨ ¨ ¨ Event latencies Algorithm execution time Monitoring framework Configuration database Network configuration Run-control ATLAS HLT Operation in Early Running Accepted events only mean 94. 3 ms/event 12 Data: 40% W/Z + 60% di-jets ¨ Ricardo Goncalo, Royal Holloway University of London
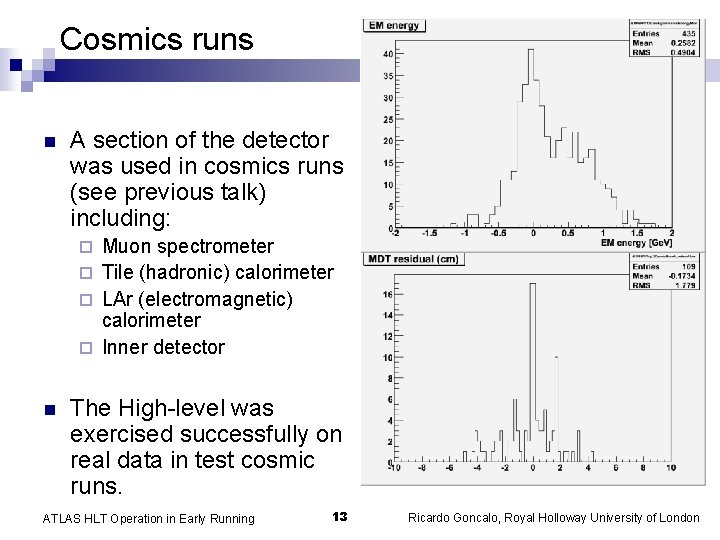
Cosmics runs n A section of the detector was used in cosmics runs (see previous talk) including: Muon spectrometer ¨ Tile (hadronic) calorimeter ¨ LAr (electromagnetic) calorimeter ¨ Inner detector ¨ n The High-level was exercised successfully on real data in test cosmic runs. ATLAS HLT Operation in Early Running 13 Ricardo Goncalo, Royal Holloway University of London
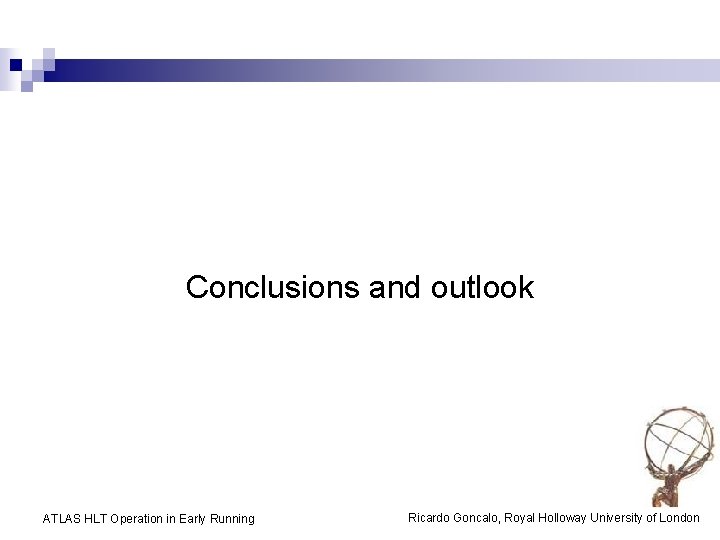
Conclusions and outlook ATLAS HLT Operation in Early Running Ricardo Goncalo, Royal Holloway University of London
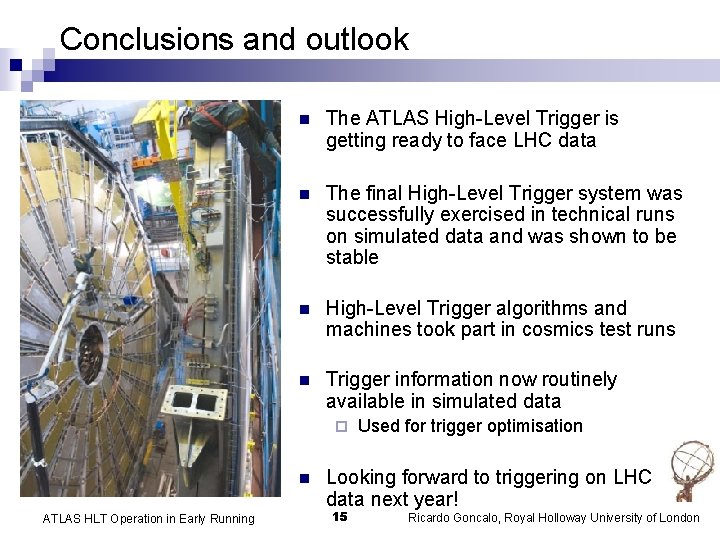
Conclusions and outlook n The ATLAS High-Level Trigger is getting ready to face LHC data n The final High-Level Trigger system was successfully exercised in technical runs on simulated data and was shown to be stable n High-Level Trigger algorithms and machines took part in cosmics test runs n Trigger information now routinely available in simulated data ¨ n ATLAS HLT Operation in Early Running Used for trigger optimisation Looking forward to triggering on LHC data next year! 15 Ricardo Goncalo, Royal Holloway University of London
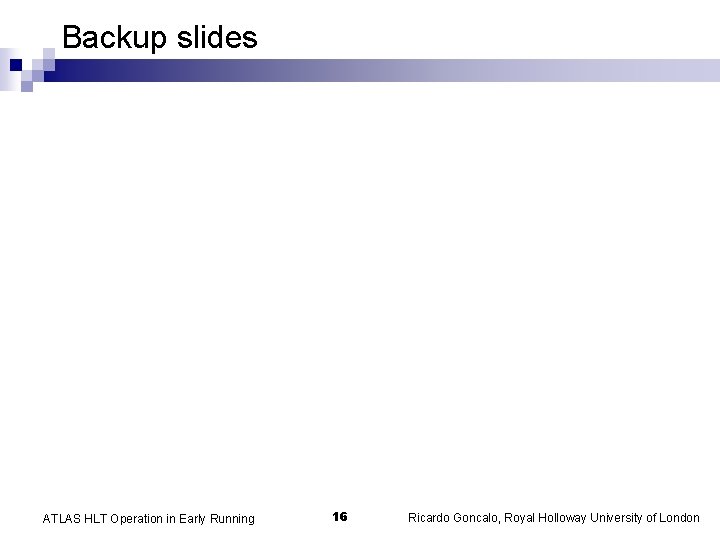
Backup slides ATLAS HLT Operation in Early Running 16 Ricardo Goncalo, Royal Holloway University of London
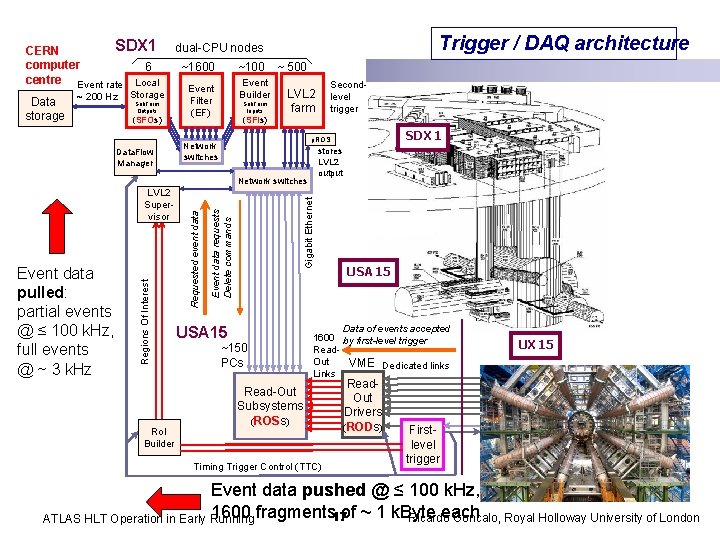
SDX 1 CERN computer 6 centre Event rate Local Data storage ~ 200 Hz Storage Sub. Farm Outputs (SFOs) Data. Flow Manager Trigger / DAQ architecture dual-CPU nodes ~1600 ~100 ~ 500 Event Builder Event Filter (EF) Sub. Farm Inputs (SFIs) Ro. I Builder stores LVL 2 output Gigabit Ethernet Event data requests Delete commands Requested event data Regions Of Interest Event data pulled: partial events @ ≤ 100 k. Hz, full events @ ~ 3 k. Hz SDX 1 p. ROS Network switches LVL 2 Supervisor Secondlevel trigger LVL 2 farm USA 15 ~150 PCs USA 15 Data of events accepted 1600 by first-level trigger Read. Out VME Dedicated links Links Read-Out Subsystems (ROSs) Timing Trigger Control (TTC) Read. Out Drivers (RODs) UX 15 Firstlevel trigger Event data pushed @ ≤ 100 k. Hz, 1600 fragments 17 of ~ 1 k. Byte each Ricardo Goncalo, Royal Holloway University of London ATLAS HLT Operation in Early Running
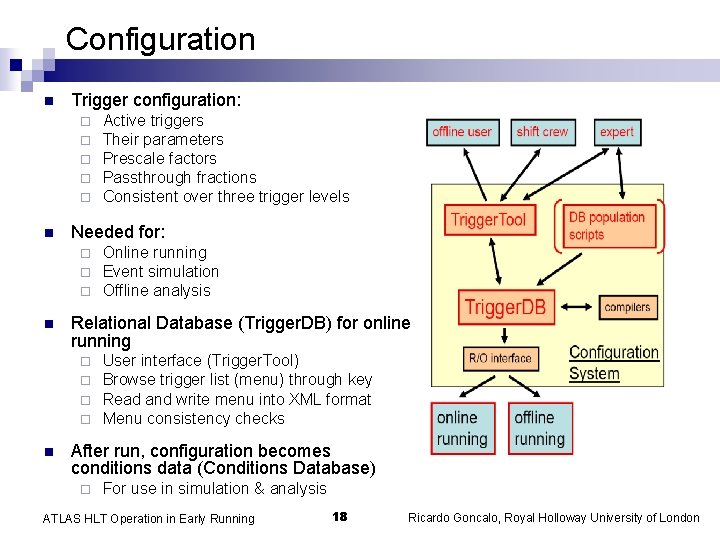
Configuration n Trigger configuration: ¨ ¨ ¨ n Needed for: ¨ ¨ ¨ n Online running Event simulation Offline analysis Relational Database (Trigger. DB) for online running ¨ ¨ n Active triggers Their parameters Prescale factors Passthrough fractions Consistent over three trigger levels User interface (Trigger. Tool) Browse trigger list (menu) through key Read and write menu into XML format Menu consistency checks After run, configuration becomes conditions data (Conditions Database) ¨ For use in simulation & analysis ATLAS HLT Operation in Early Running 18 Ricardo Goncalo, Royal Holloway University of London
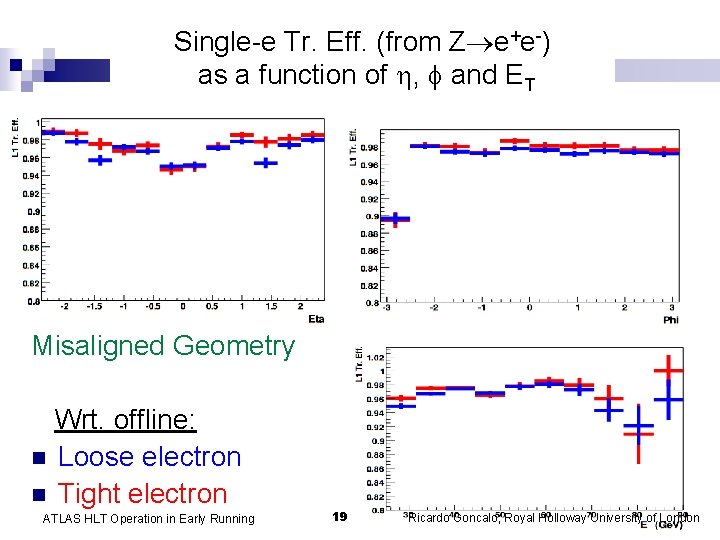
Single-e Tr. Eff. (from Z e+e-) as a function of , and ET Misaligned Geometry Wrt. offline: n Loose electron n Tight electron ATLAS HLT Operation in Early Running 19 Ricardo Goncalo, Royal Holloway University of London
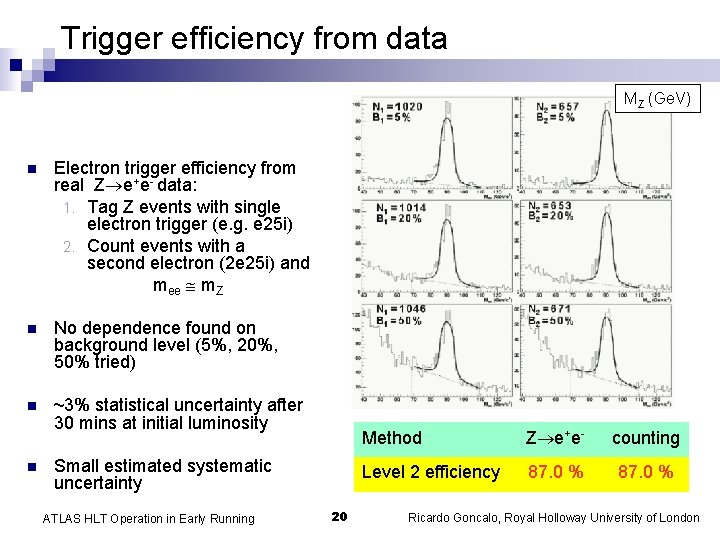
Trigger efficiency from data MZ (Ge. V) n Electron trigger efficiency from real Z e+e- data: 1. Tag Z events with single electron trigger (e. g. e 25 i) 2. Count events with a second electron (2 e 25 i) and mee m. Z n No dependence found on background level (5%, 20%, 50% tried) n ~3% statistical uncertainty after 30 mins at initial luminosity n Small estimated systematic uncertainty ATLAS HLT Operation in Early Running 20 Method Z e+e- counting Level 2 efficiency 87. 0 % Ricardo Goncalo, Royal Holloway University of London
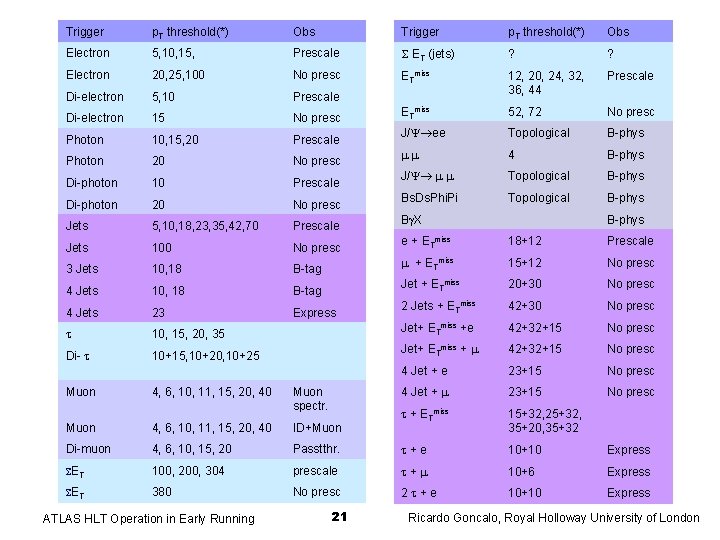
Trigger p. T threshold(*) Obs Electron 5, 10, 15, Prescale ET (jets) ? ? Electron 20, 25, 100 No presc ETmiss Prescale Di-electron 5, 10 Prescale 12, 20, 24, 32, 36, 44 Di-electron 15 No presc ETmiss 52, 72 No presc Photon 10, 15, 20 Prescale J/ ee Topological B-phys Photon 20 No presc 4 B-phys Di-photon 10 Prescale J/ Topological B-phys Di-photon 20 No presc Bs. Ds. Phi. Pi Topological B-phys Jets 5, 10, 18, 23, 35, 42, 70 Prescale Jets 100 No presc e + ETmiss 18+12 Prescale 3 Jets 10, 18 B-tag + ETmiss 15+12 No presc 4 Jets 10, 18 B-tag Jet + ETmiss 20+30 No presc 4 Jets 23 Express 2 Jets + ETmiss 42+30 No presc 10, 15, 20, 35 Jet+ ETmiss +e 42+32+15 No presc Di- 10+15, 10+20, 10+25 Jet+ ETmiss + 42+32+15 No presc 4 Jet + e 23+15 No presc 4 Jet + 23+15 No presc + ETmiss 15+32, 25+32, 35+20, 35+32 Muon 4, 6, 10, 11, 15, 20, 40 Muon spectr. B X B-phys Muon 4, 6, 10, 11, 15, 20, 40 ID+Muon Di-muon 4, 6, 10, 15, 20 Passtthr. +e 10+10 Express ET 100, 200, 304 prescale + 10+6 Express ET 380 No presc 2 +e 10+10 Express ATLAS HLT Operation in Early Running 21 Ricardo Goncalo, Royal Holloway University of London
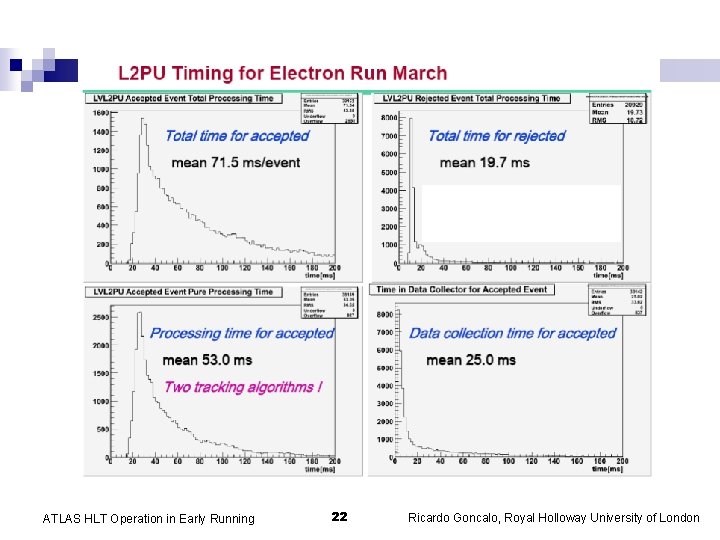
ATLAS HLT Operation in Early Running 22 Ricardo Goncalo, Royal Holloway University of London
Highlevel language
Highlevel programming language
Highlevel language
Highlevel language
Integrated commissioning and progress system
Commissioning cycle
Aecp requirements
Commissioning cycle
Ecs commissioning
Fortis furnace commissioning sheet
Timeline jwst
Human and physical features
C3sc
Value based commissioning
Vacuum systems commissioning
Power system commissioning
Nihr central commissioning facility
Dominik sikora
North west london ccg
Commissioning
Aabc commissioning group
Lidl stores with ev charging
Croydon clinical commissioning group