Stat 155 Section 2 Last Time Continuous Random
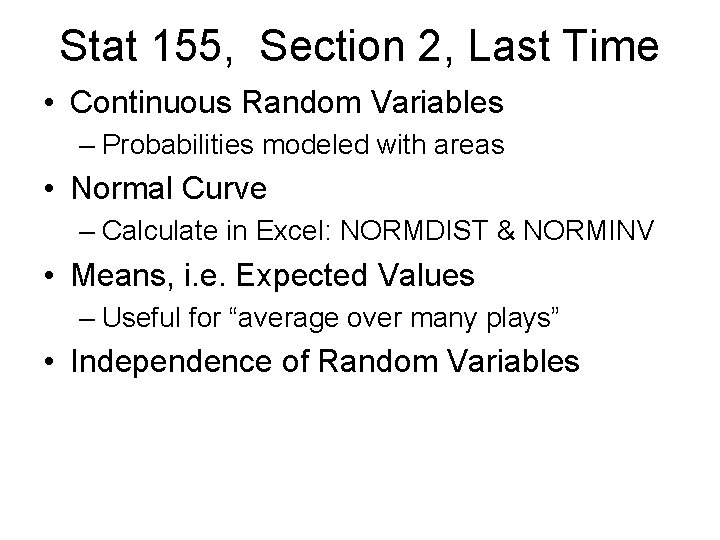
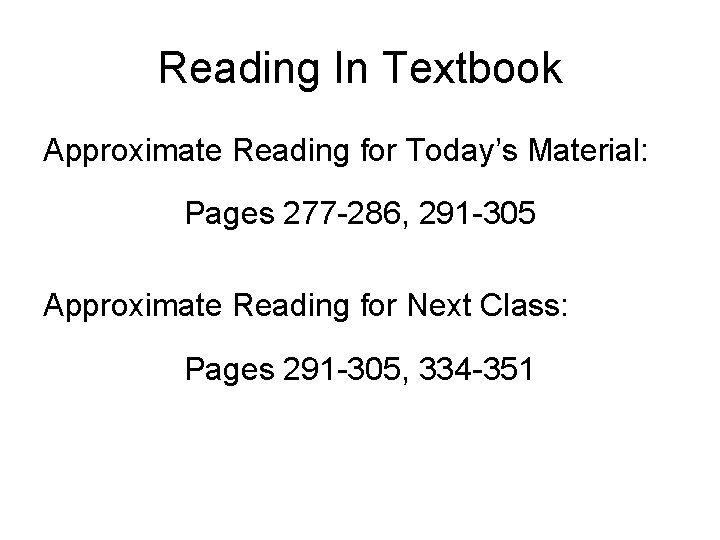
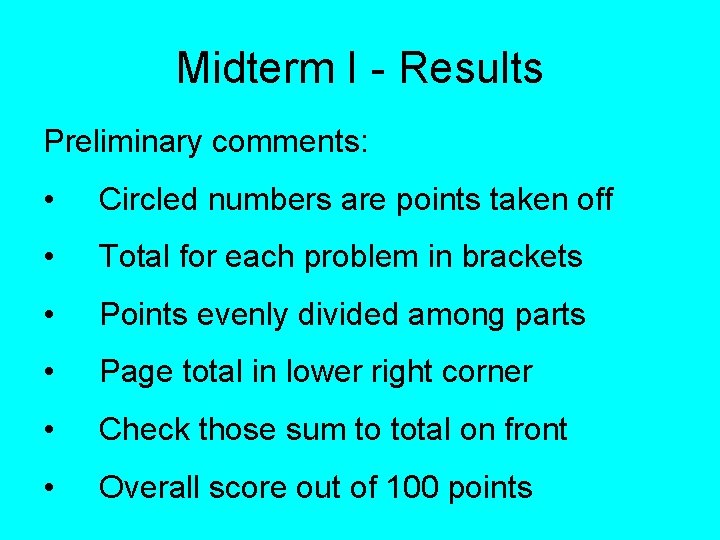
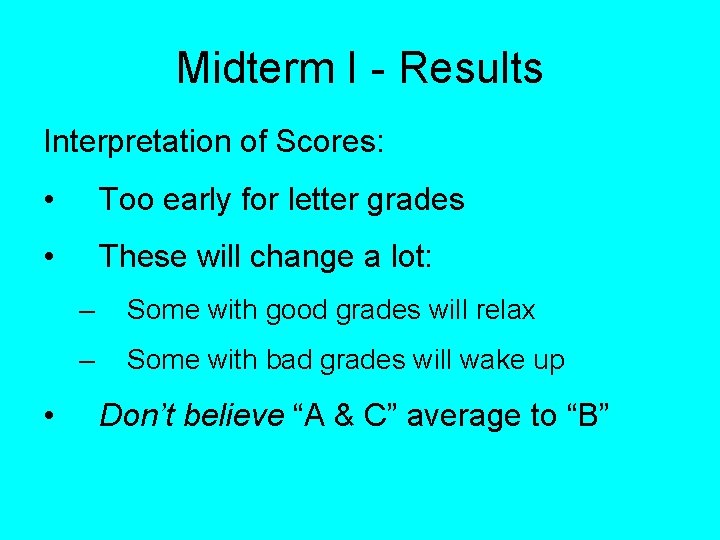
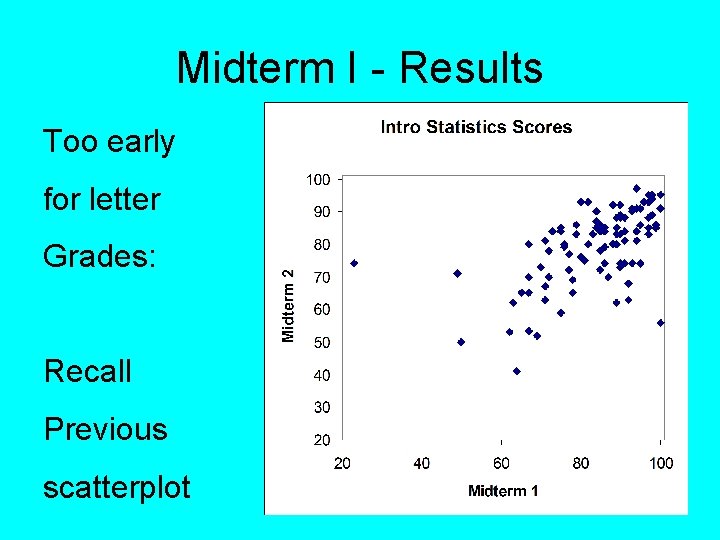
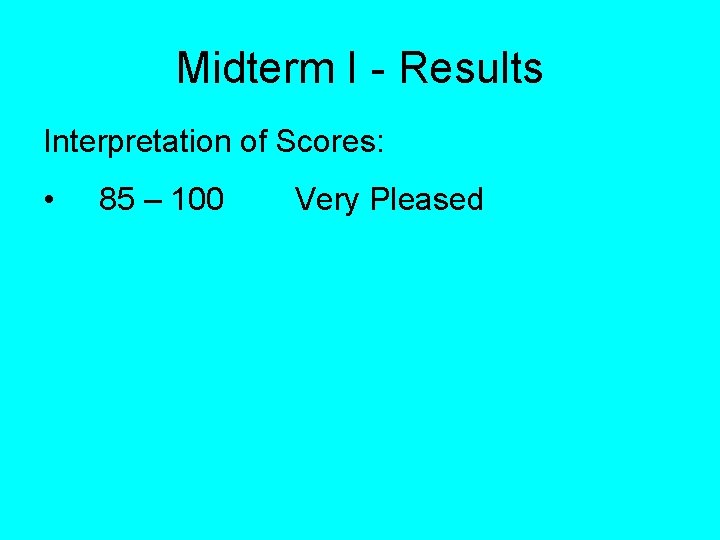
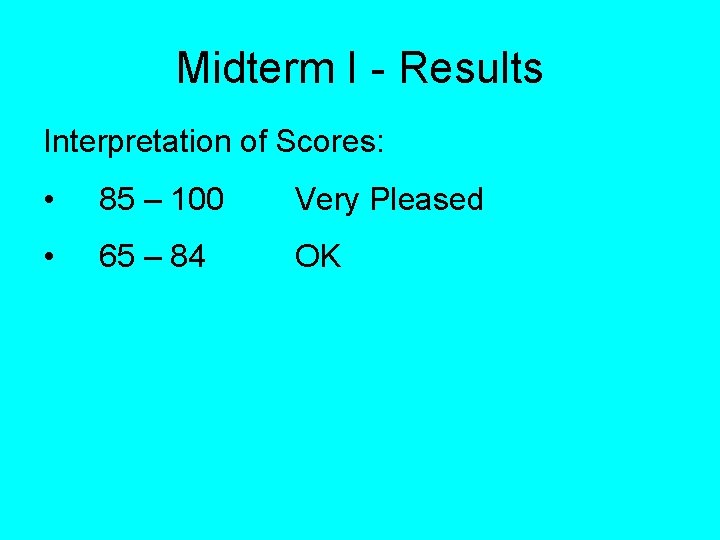
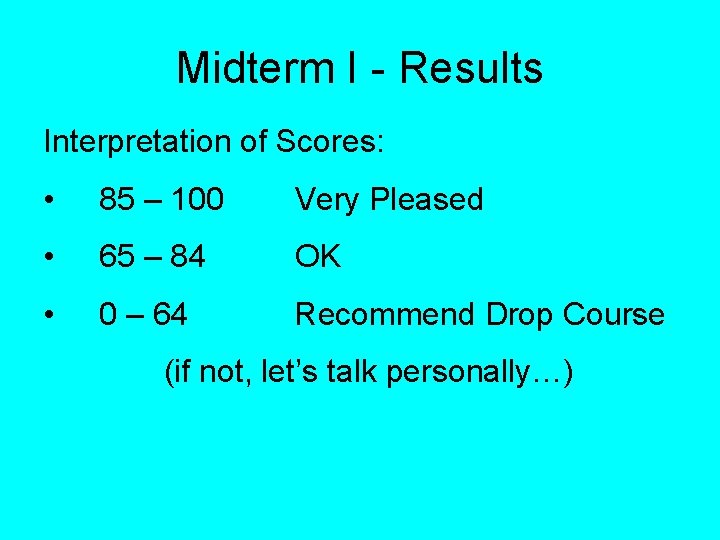
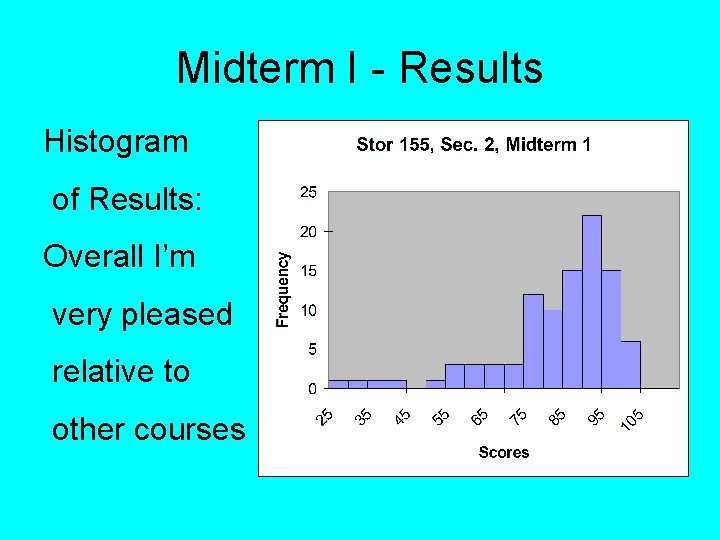
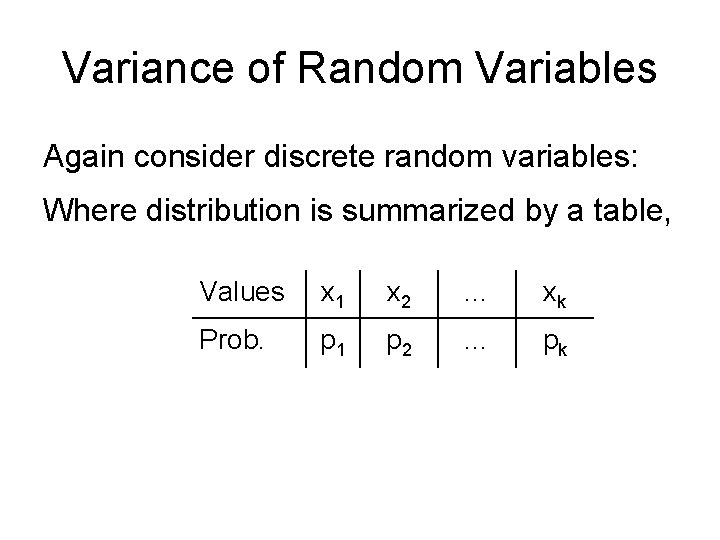
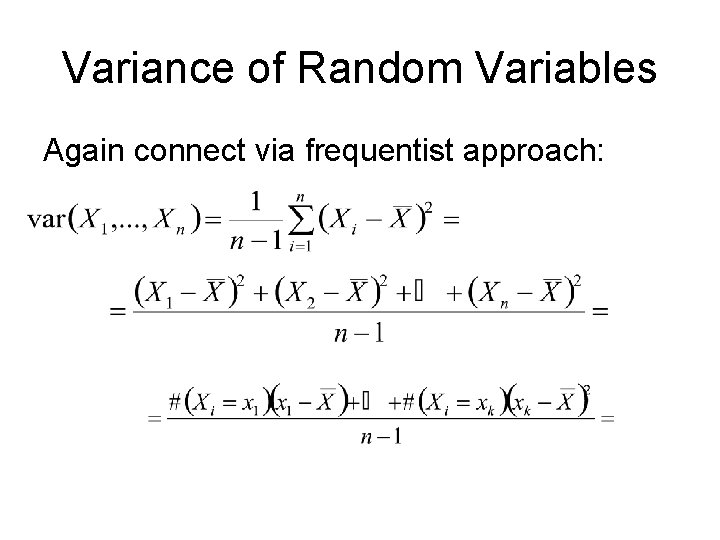
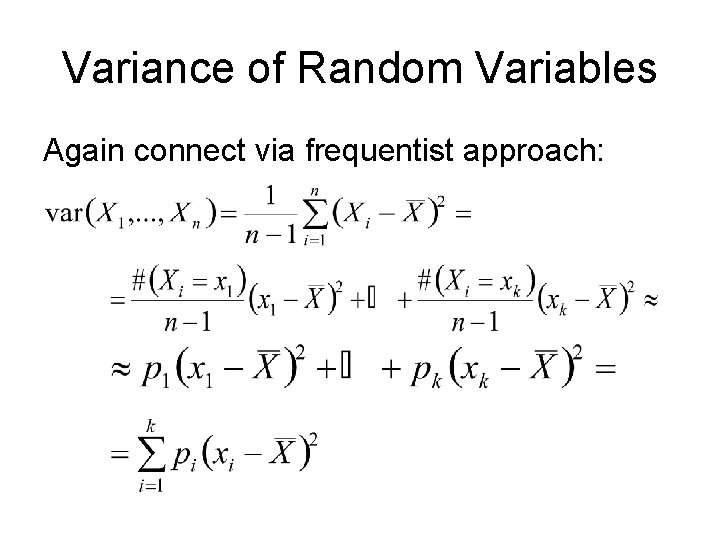
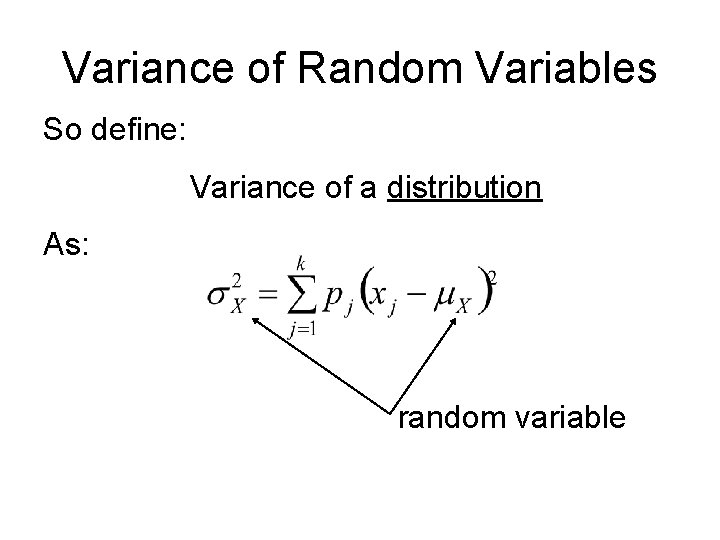
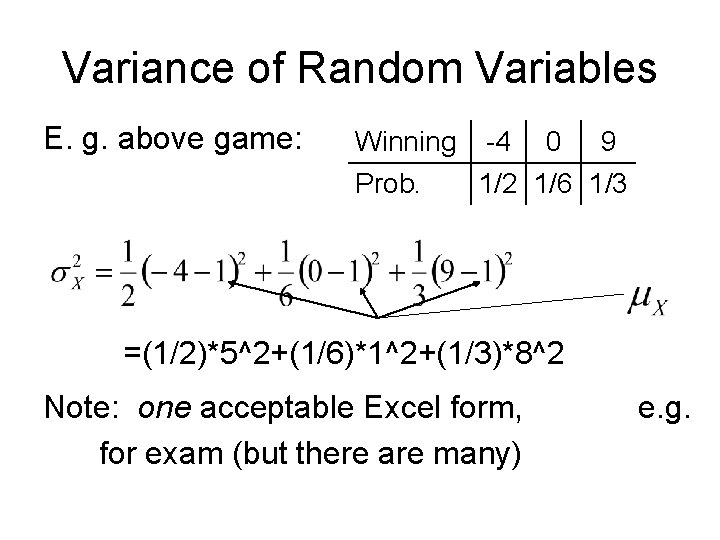
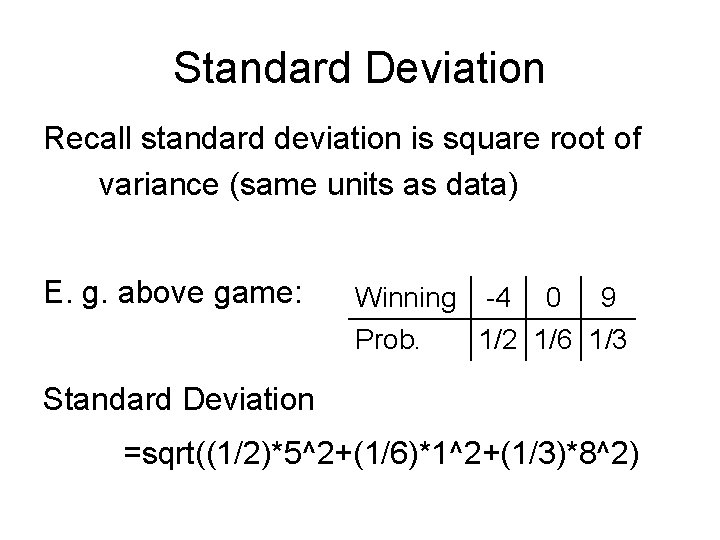
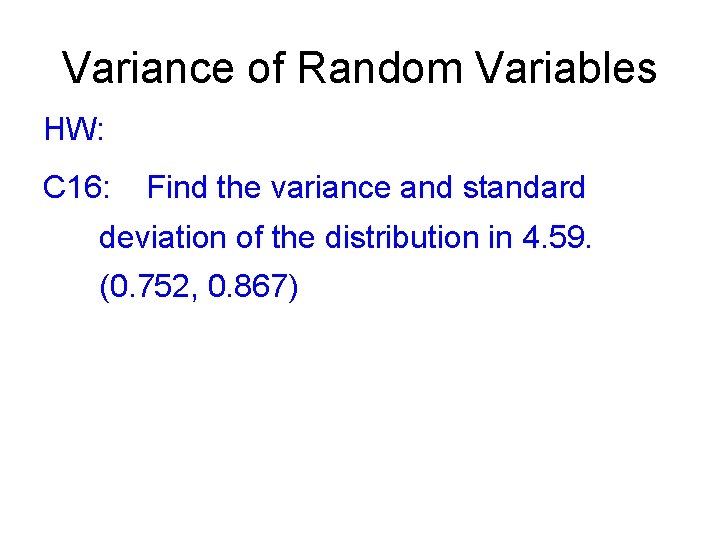
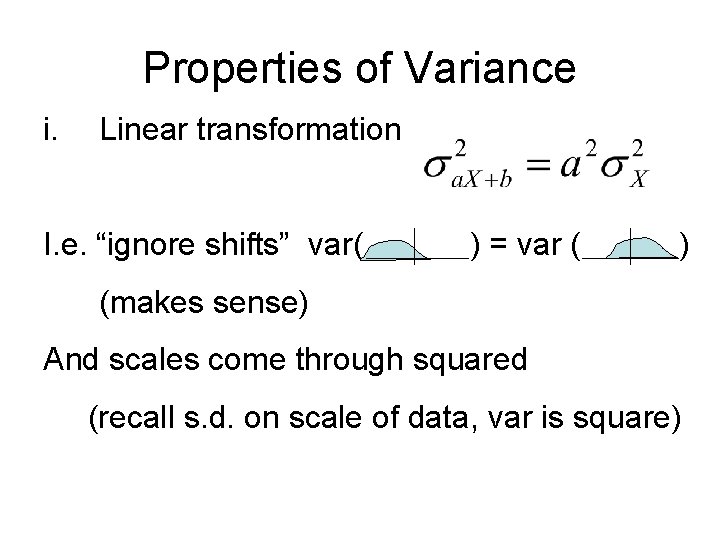
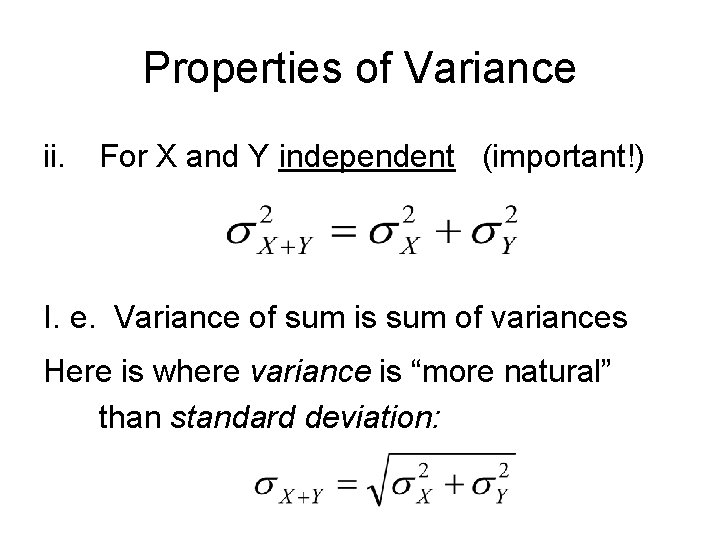
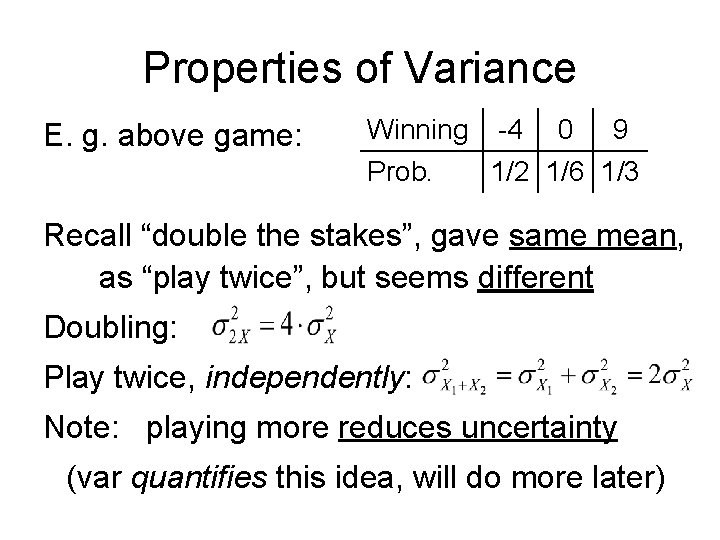
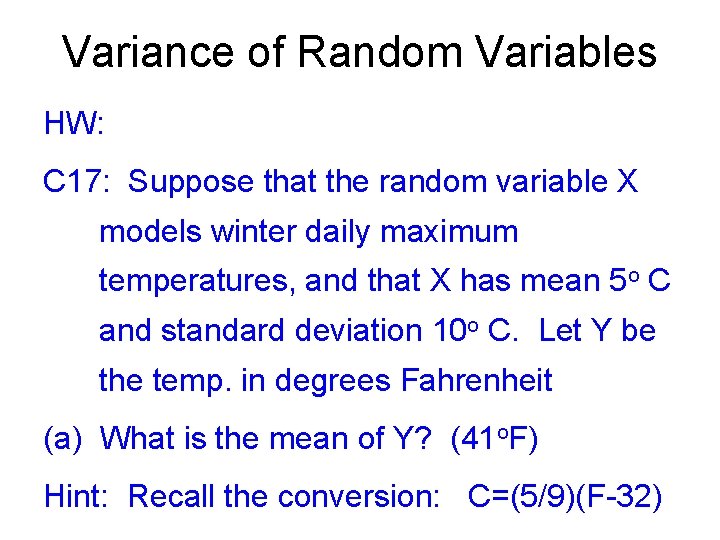
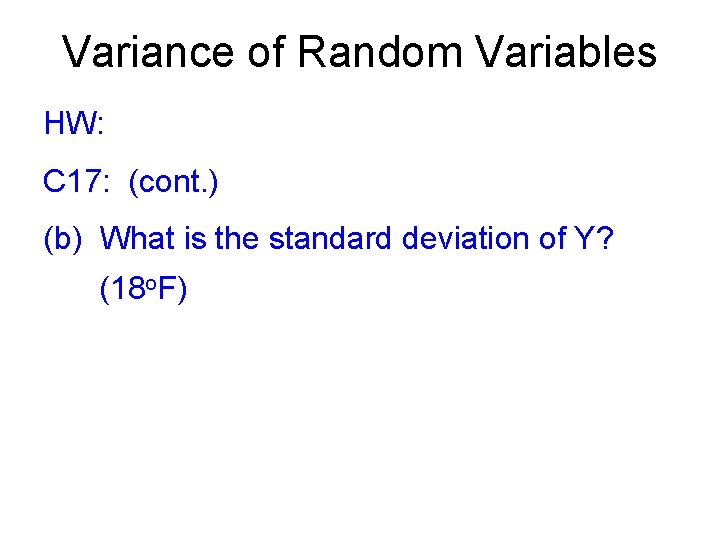
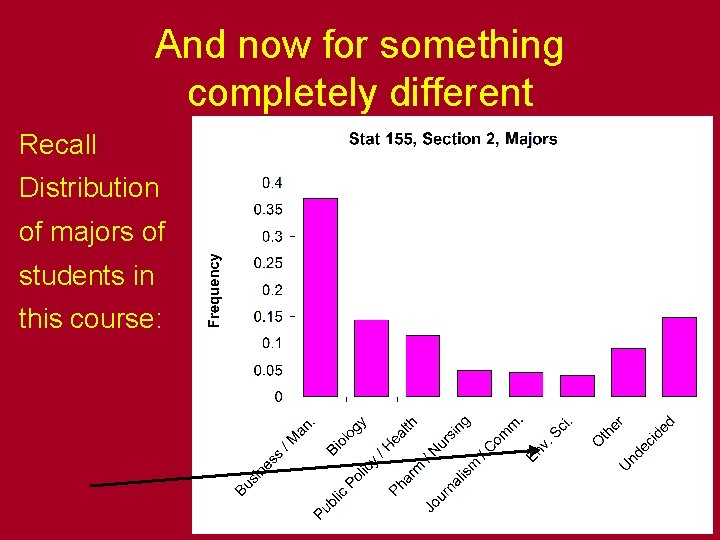
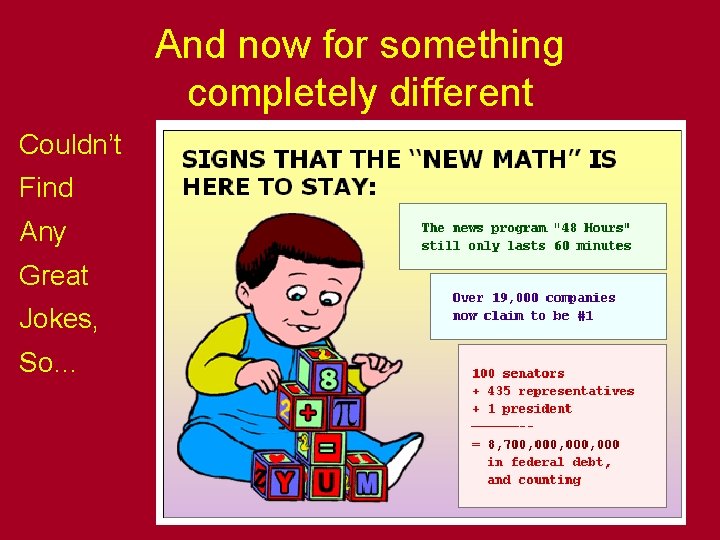
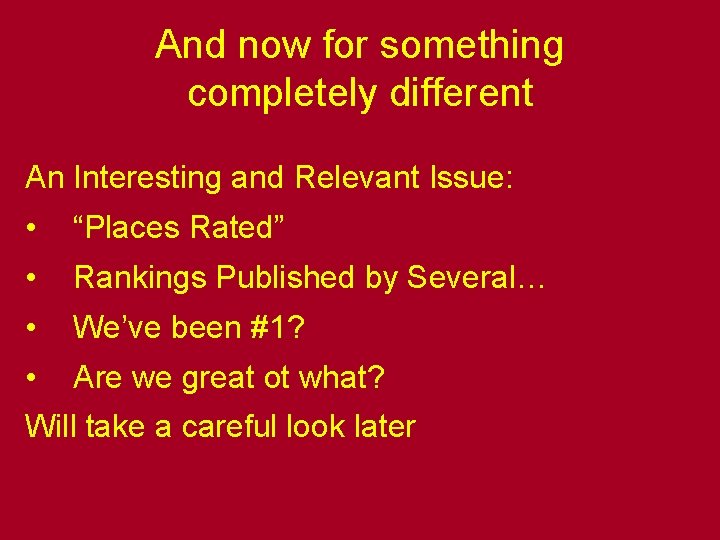
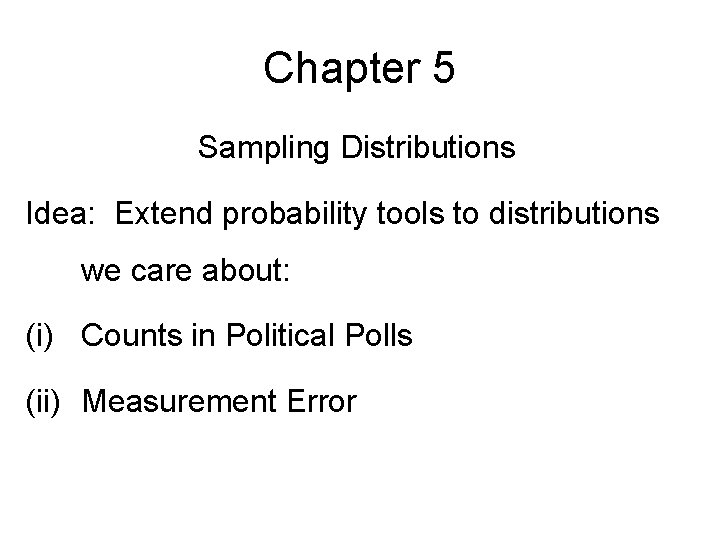
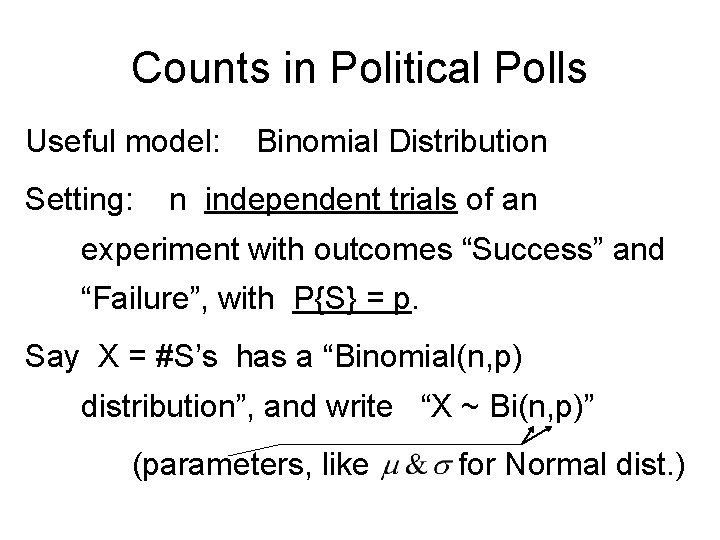
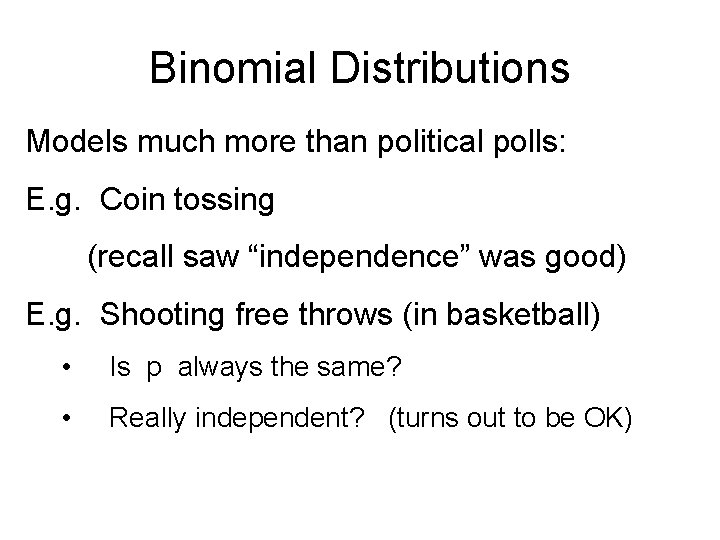
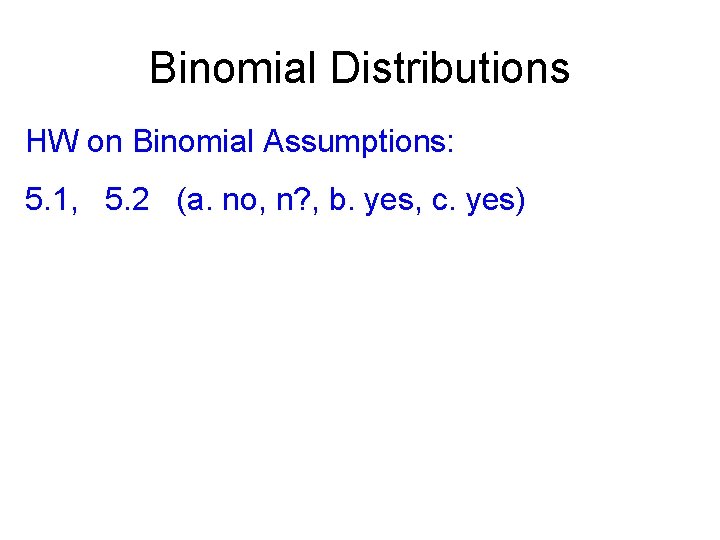
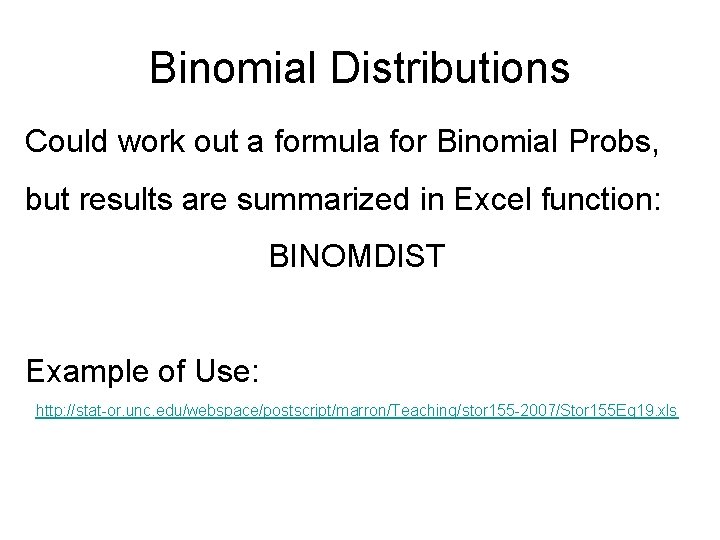
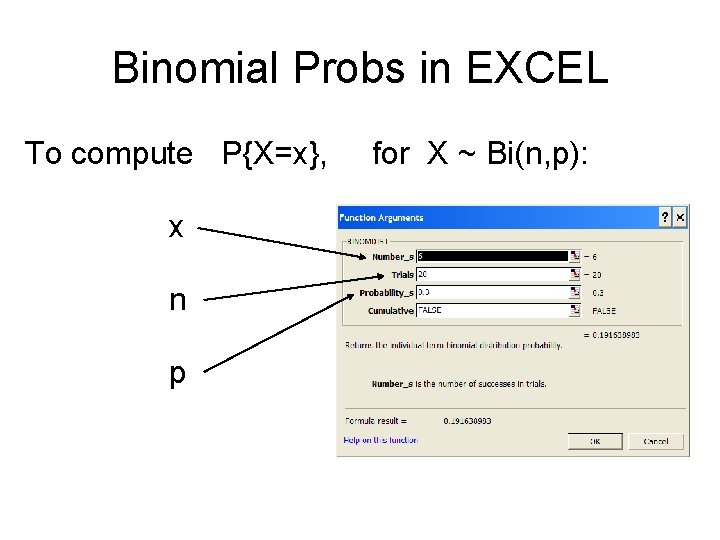
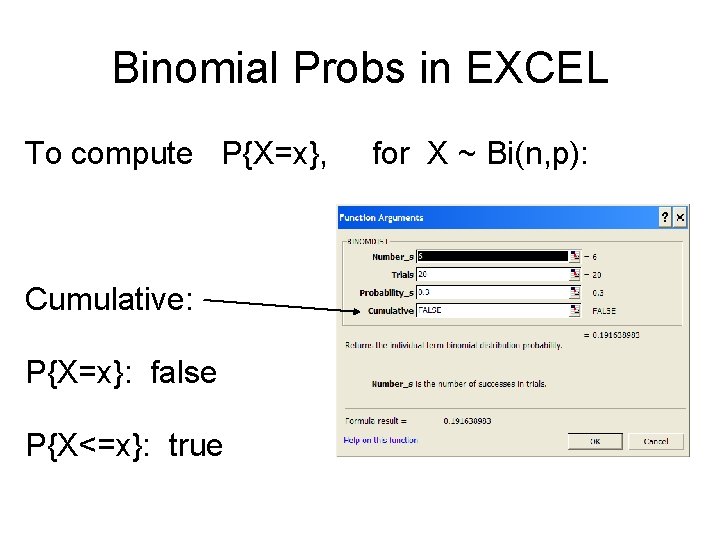
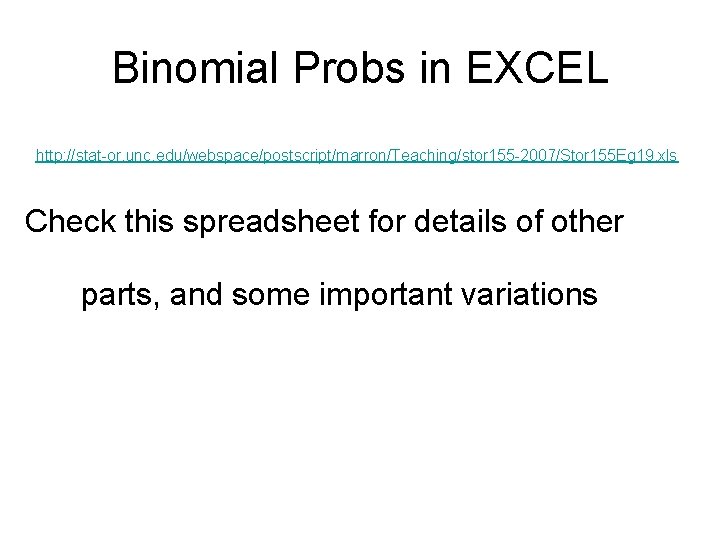
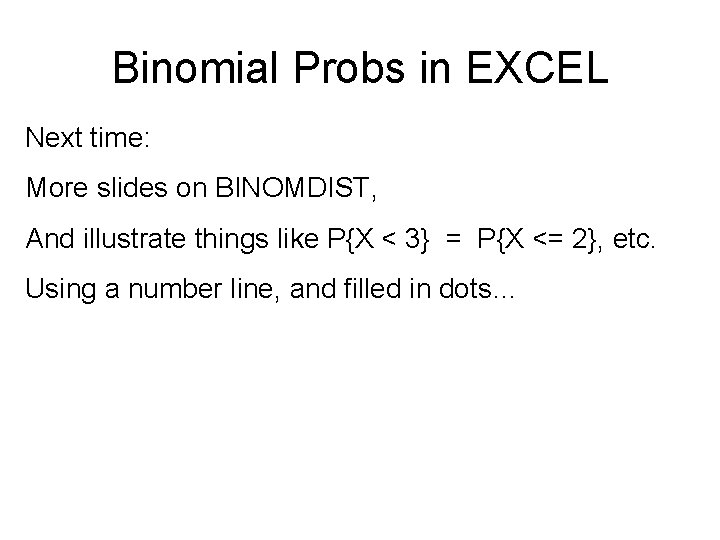
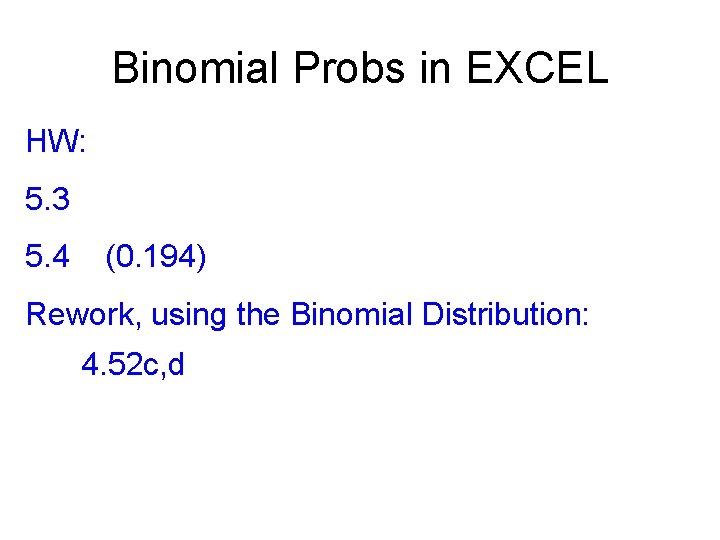
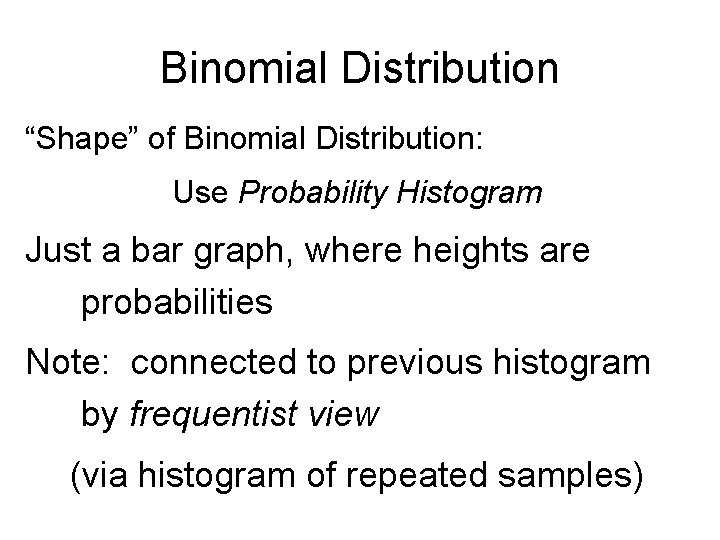
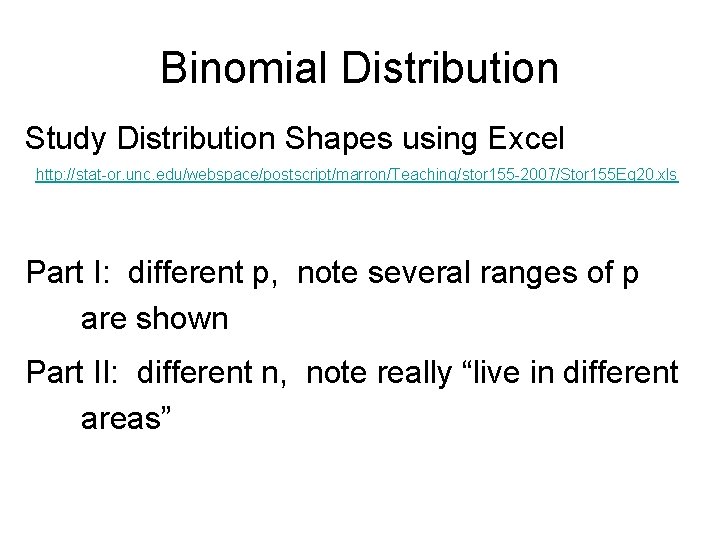
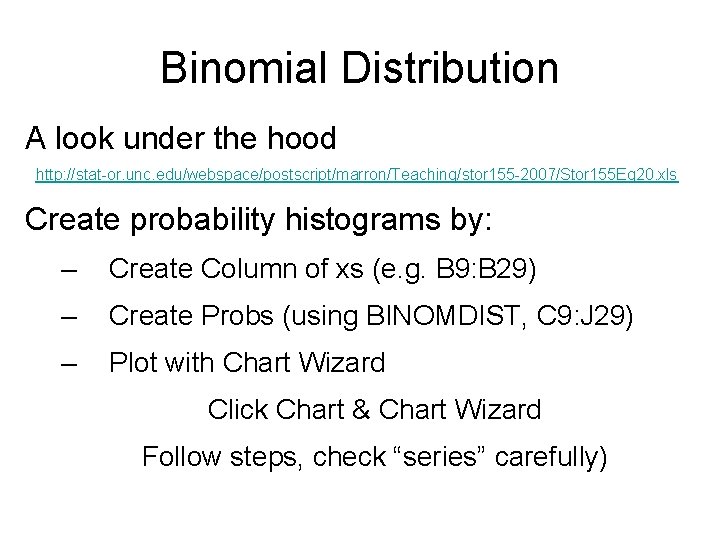
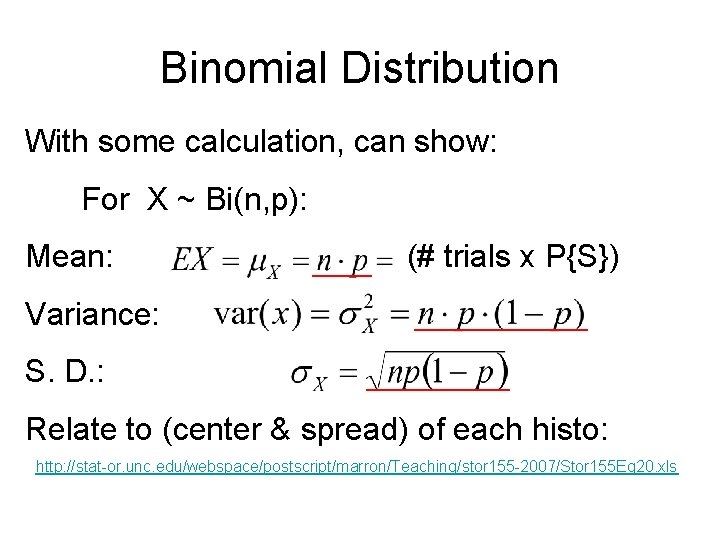
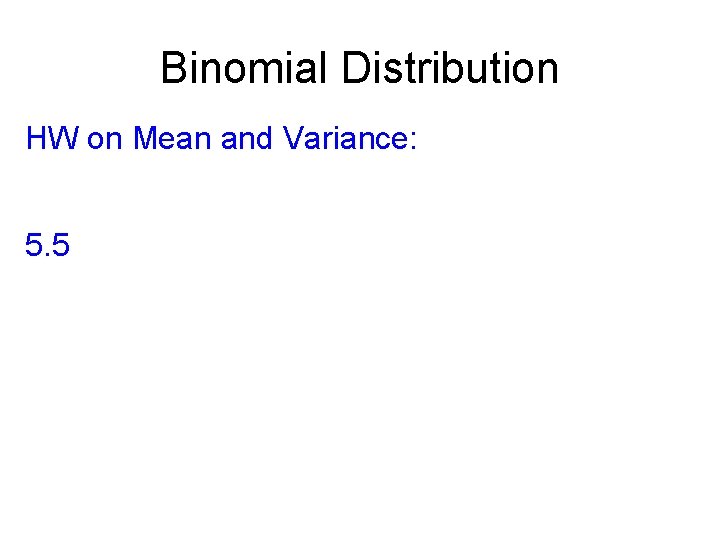
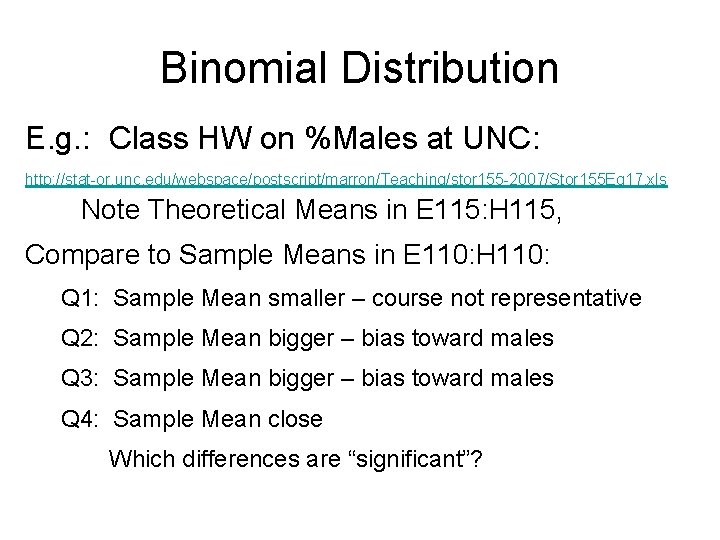
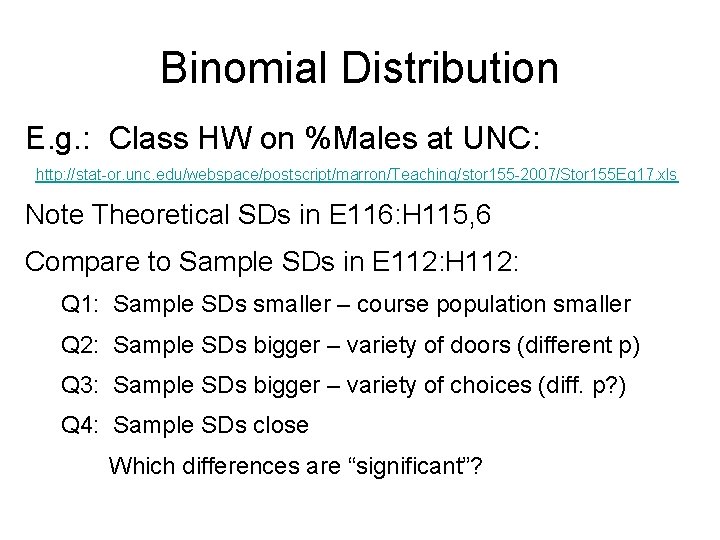
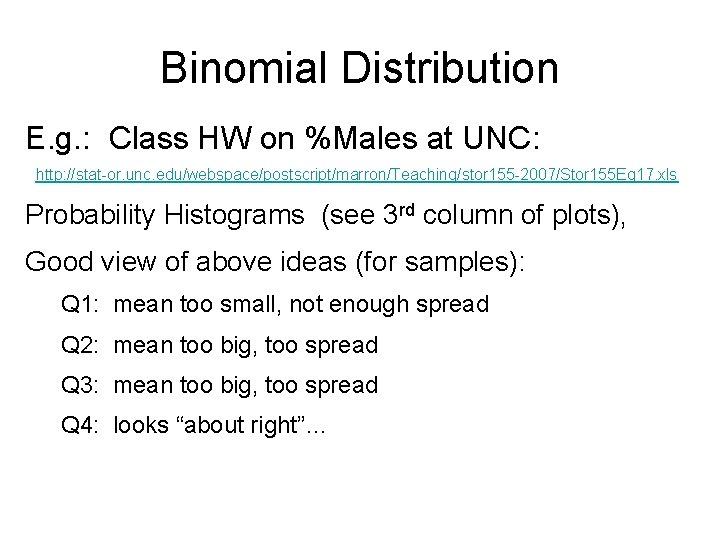
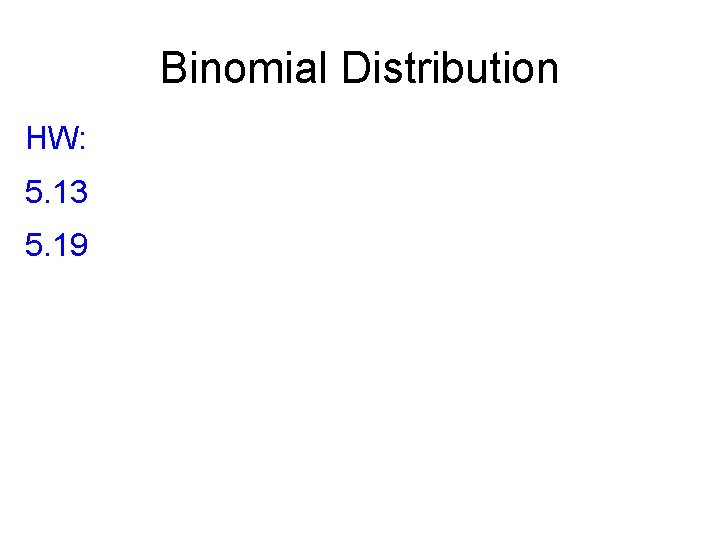
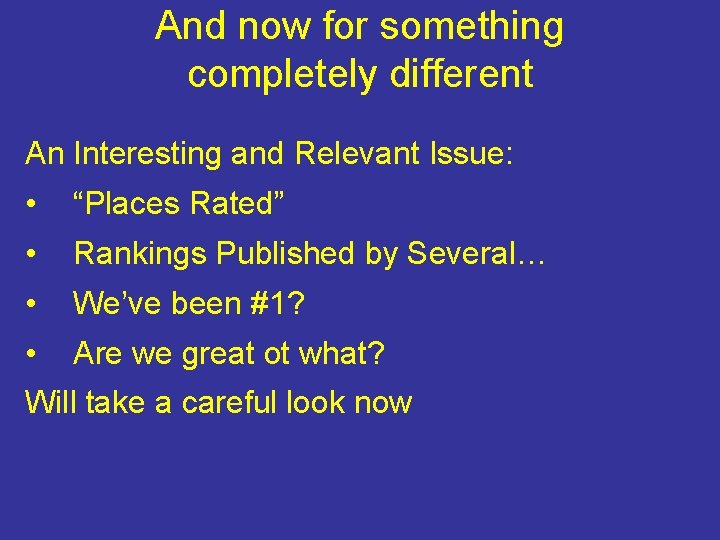
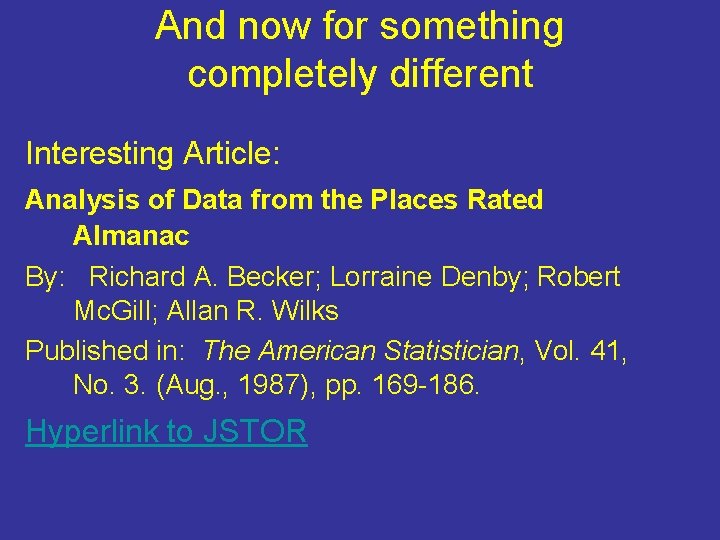
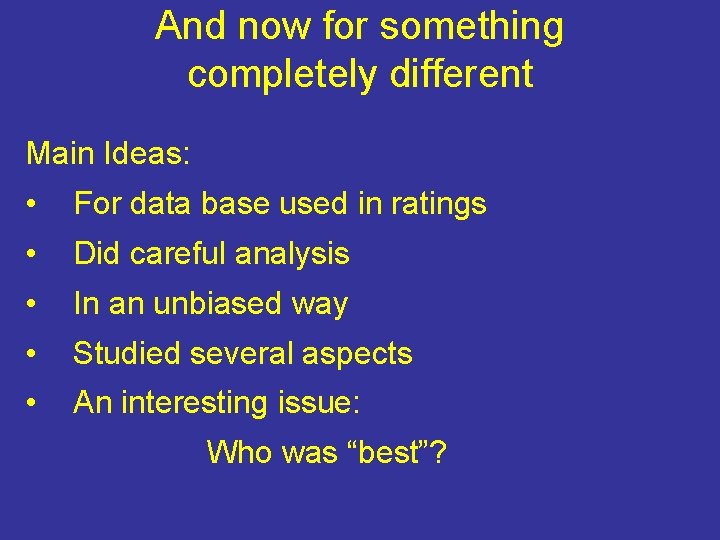
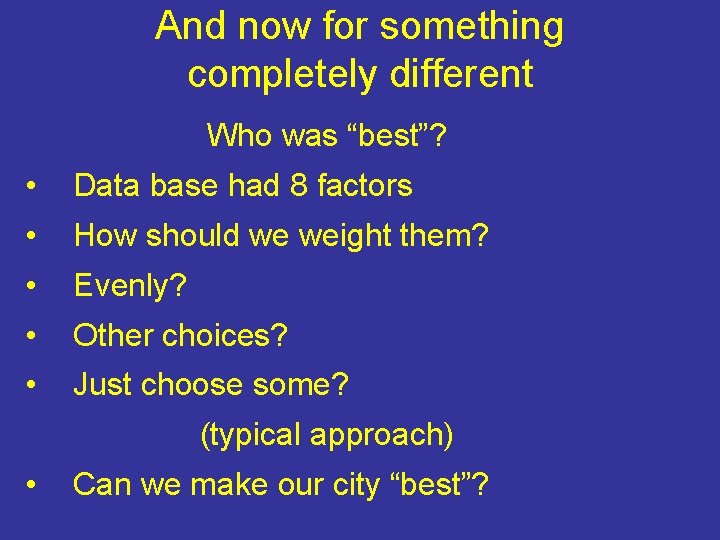
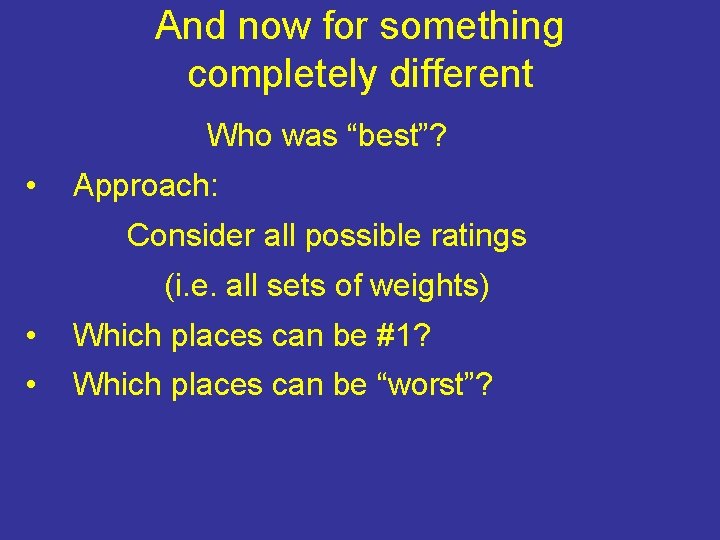
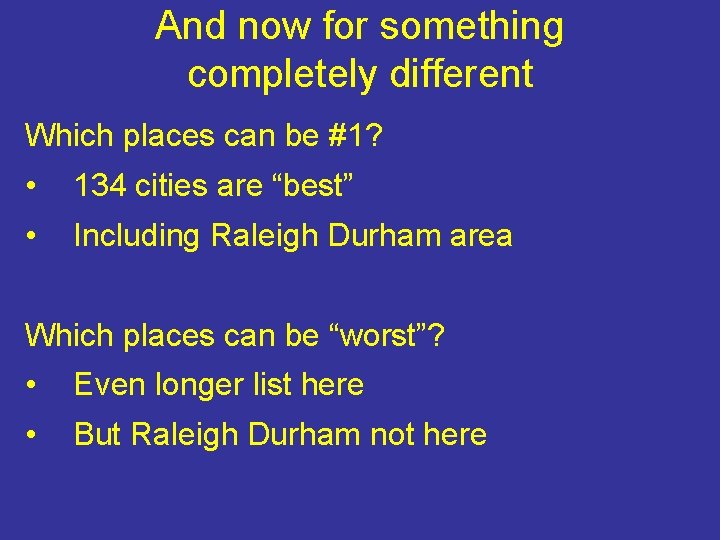
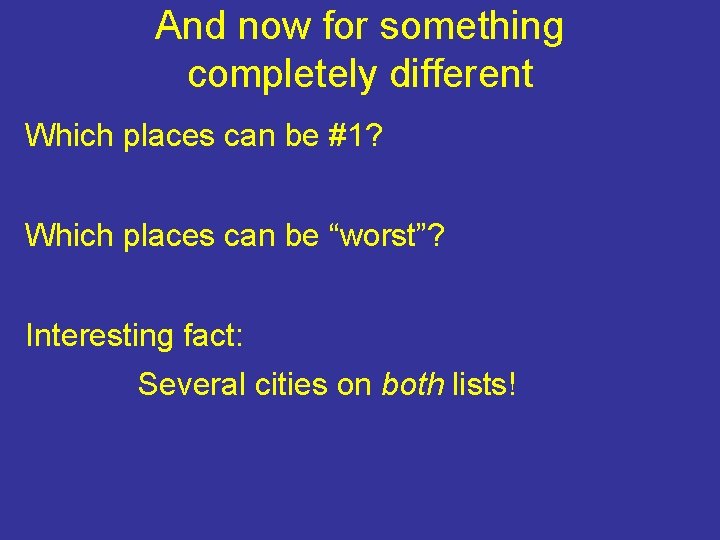
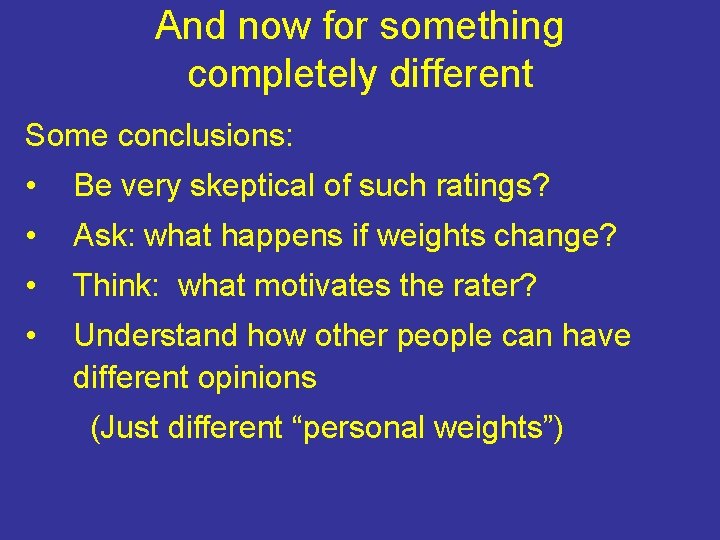
- Slides: 51
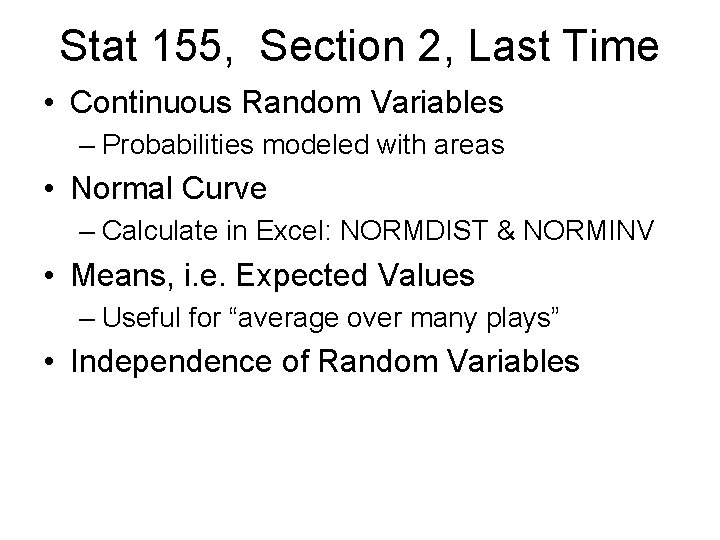
Stat 155, Section 2, Last Time • Continuous Random Variables – Probabilities modeled with areas • Normal Curve – Calculate in Excel: NORMDIST & NORMINV • Means, i. e. Expected Values – Useful for “average over many plays” • Independence of Random Variables
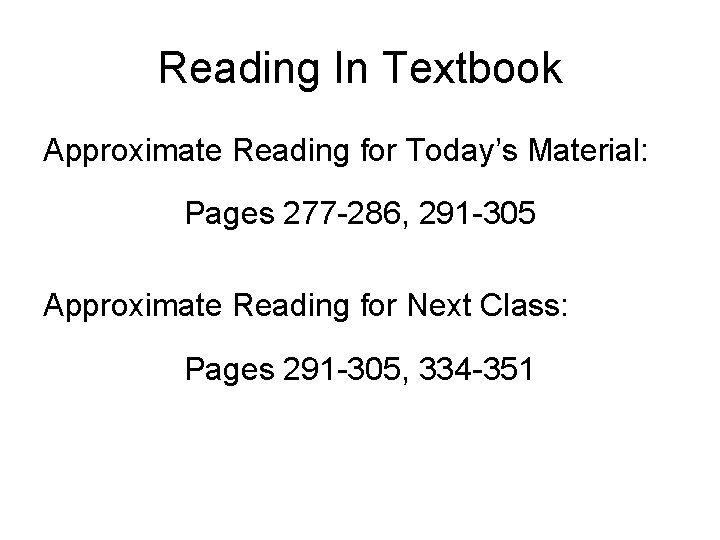
Reading In Textbook Approximate Reading for Today’s Material: Pages 277 -286, 291 -305 Approximate Reading for Next Class: Pages 291 -305, 334 -351
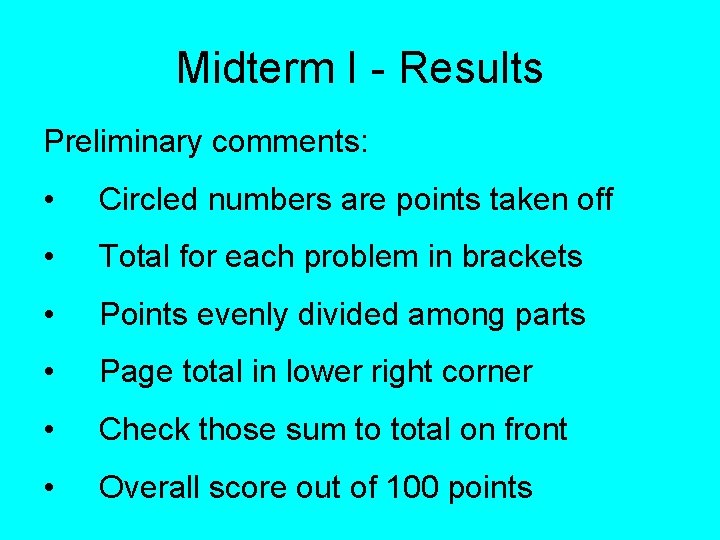
Midterm I - Results Preliminary comments: • Circled numbers are points taken off • Total for each problem in brackets • Points evenly divided among parts • Page total in lower right corner • Check those sum to total on front • Overall score out of 100 points
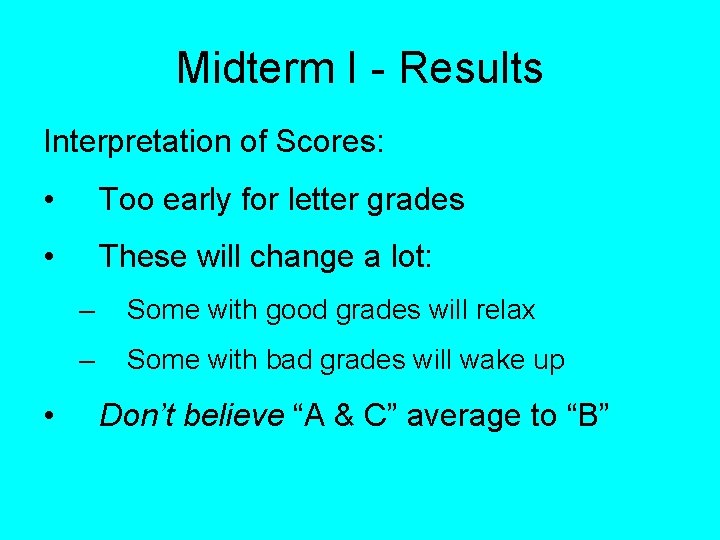
Midterm I - Results Interpretation of Scores: • Too early for letter grades • These will change a lot: • – Some with good grades will relax – Some with bad grades will wake up Don’t believe “A & C” average to “B”
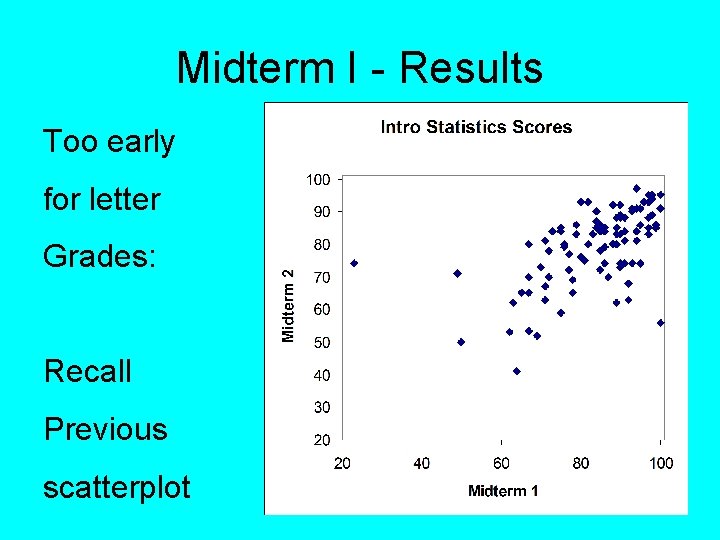
Midterm I - Results Too early for letter Grades: Recall Previous scatterplot
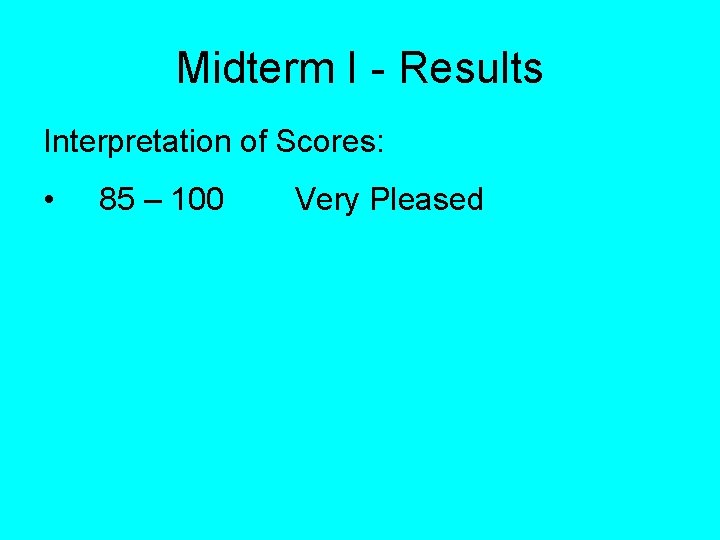
Midterm I - Results Interpretation of Scores: • 85 – 100 Very Pleased
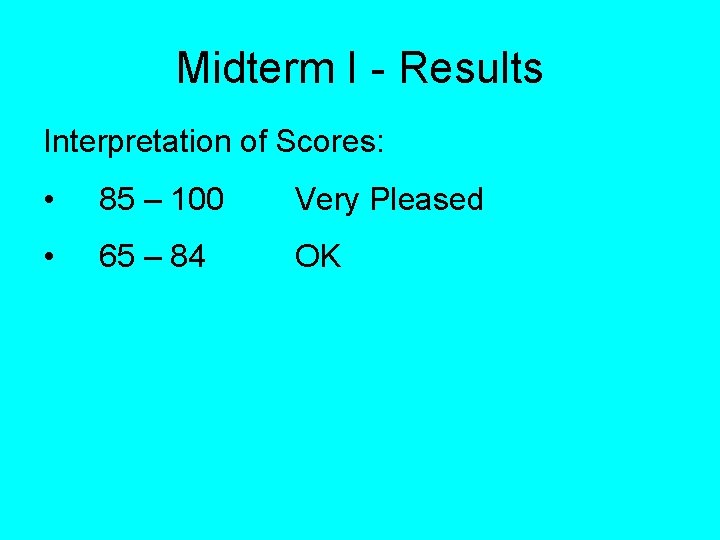
Midterm I - Results Interpretation of Scores: • 85 – 100 Very Pleased • 65 – 84 OK
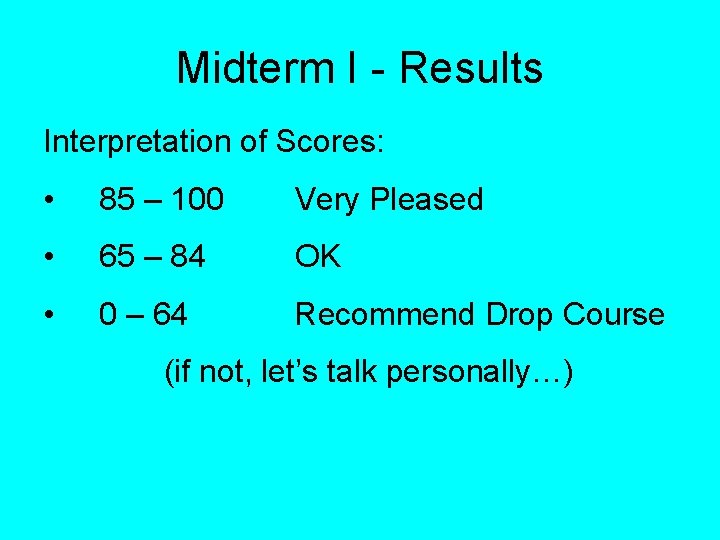
Midterm I - Results Interpretation of Scores: • 85 – 100 Very Pleased • 65 – 84 OK • 0 – 64 Recommend Drop Course (if not, let’s talk personally…)
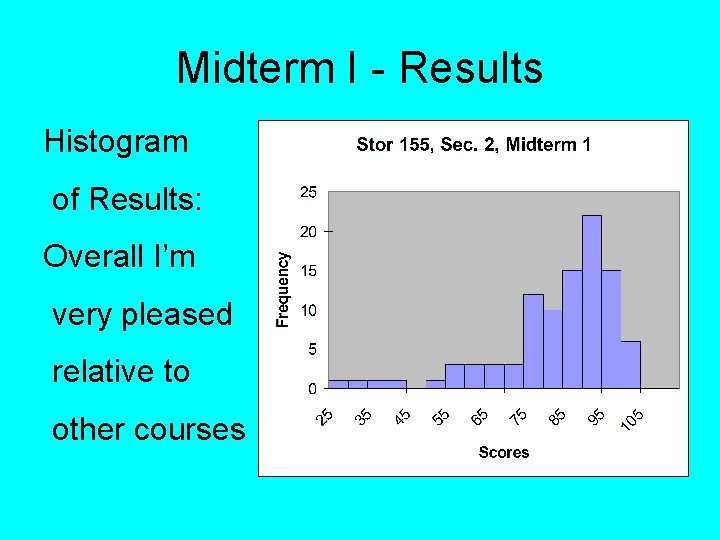
Midterm I - Results Histogram of Results: Overall I’m very pleased relative to other courses
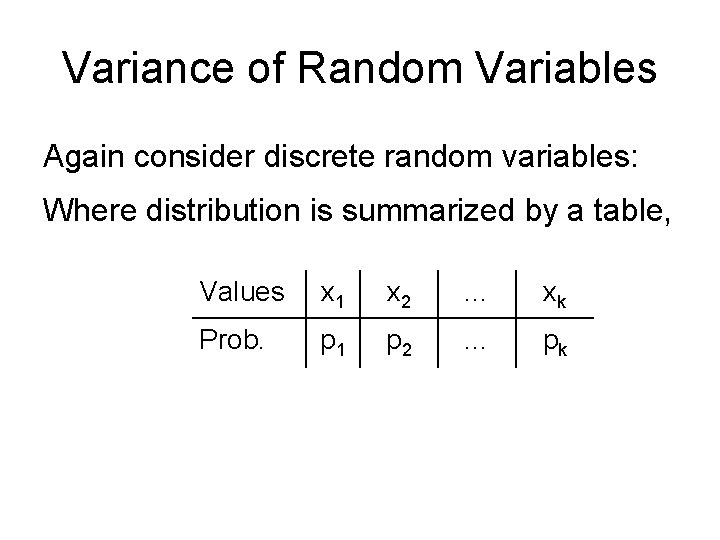
Variance of Random Variables Again consider discrete random variables: Where distribution is summarized by a table, Values x 1 x 2 … xk Prob. p 1 p 2 … pk
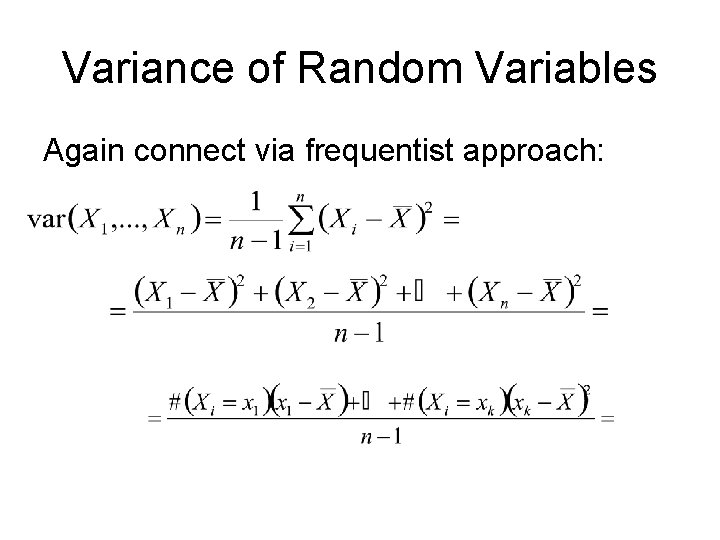
Variance of Random Variables Again connect via frequentist approach:
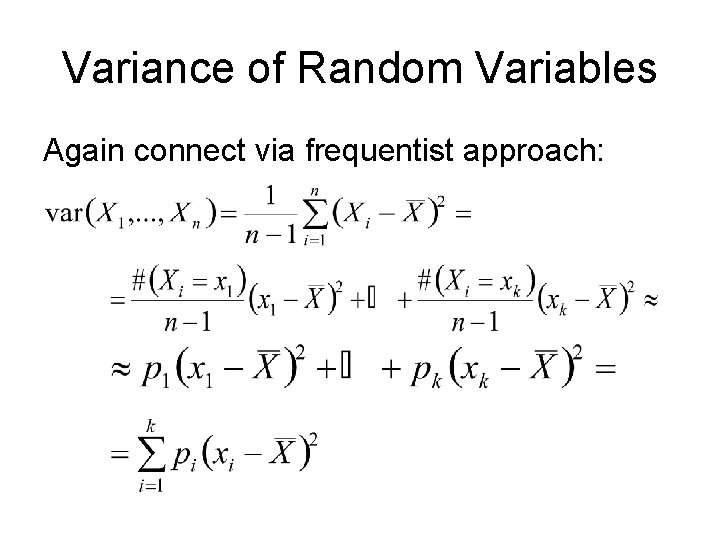
Variance of Random Variables Again connect via frequentist approach:
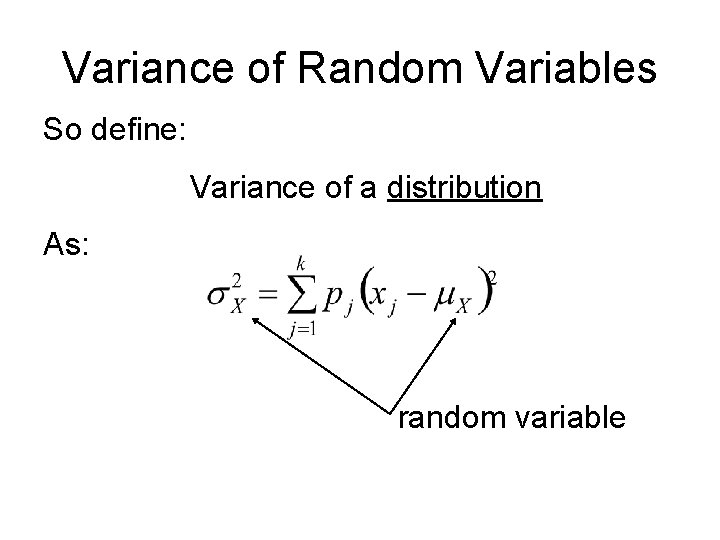
Variance of Random Variables So define: Variance of a distribution As: random variable
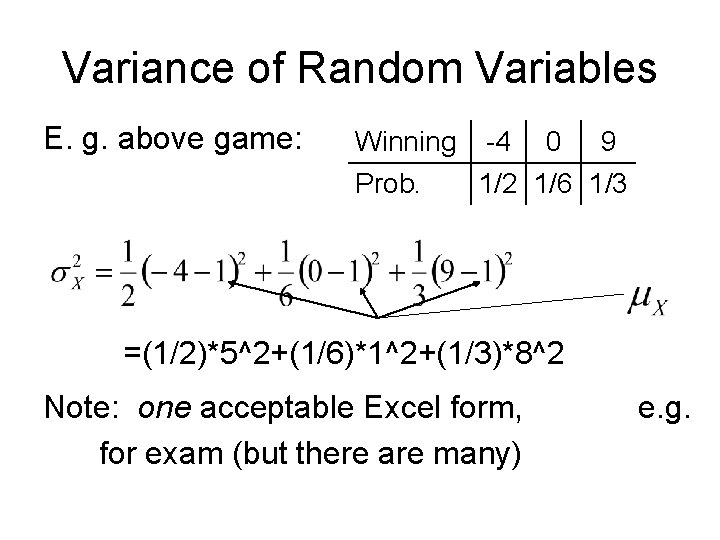
Variance of Random Variables E. g. above game: Winning -4 0 9 Prob. 1/2 1/6 1/3 =(1/2)*5^2+(1/6)*1^2+(1/3)*8^2 Note: one acceptable Excel form, for exam (but there are many) e. g.
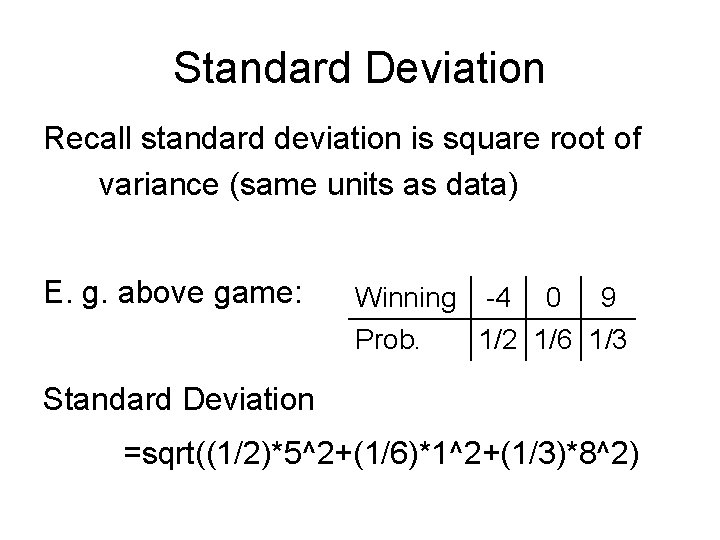
Standard Deviation Recall standard deviation is square root of variance (same units as data) E. g. above game: Winning -4 0 9 Prob. 1/2 1/6 1/3 Standard Deviation =sqrt((1/2)*5^2+(1/6)*1^2+(1/3)*8^2)
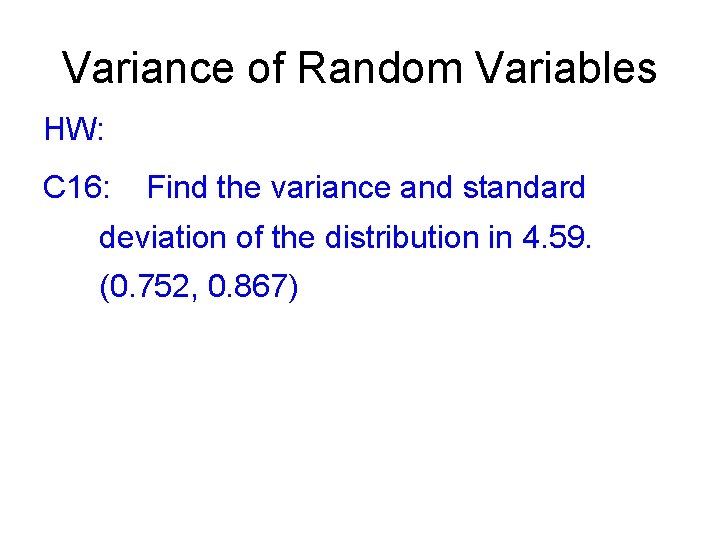
Variance of Random Variables HW: C 16: Find the variance and standard deviation of the distribution in 4. 59. (0. 752, 0. 867)
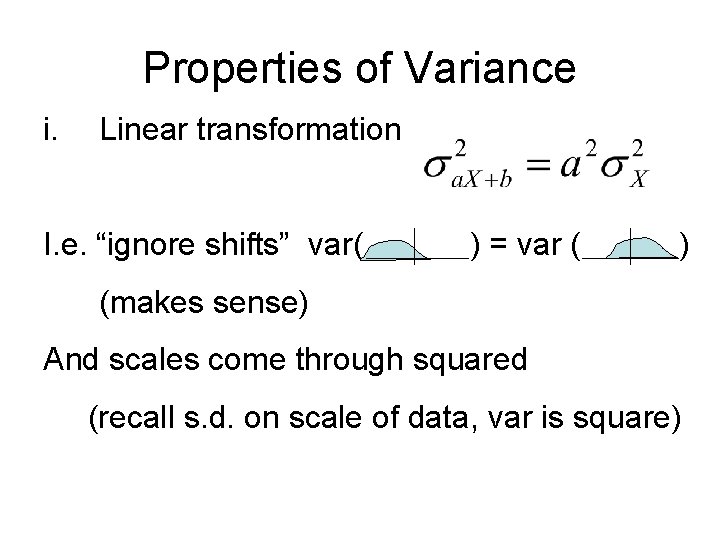
Properties of Variance i. Linear transformation I. e. “ignore shifts” var( ) = var ( ) (makes sense) And scales come through squared (recall s. d. on scale of data, var is square)
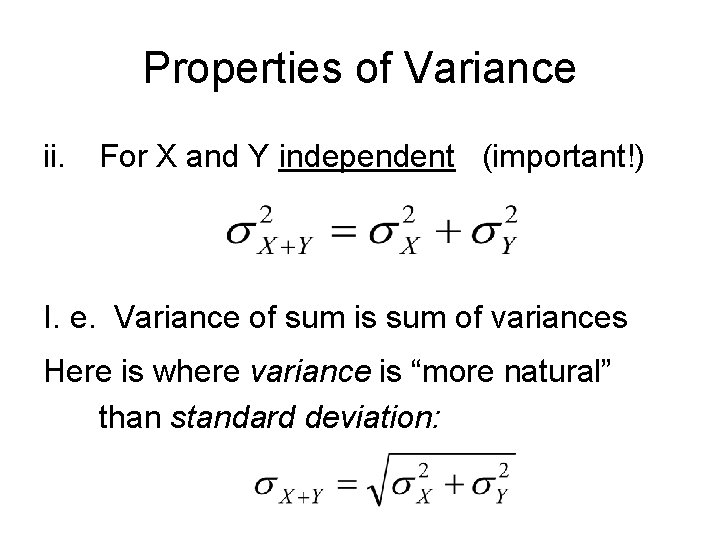
Properties of Variance ii. For X and Y independent (important!) I. e. Variance of sum is sum of variances Here is where variance is “more natural” than standard deviation:
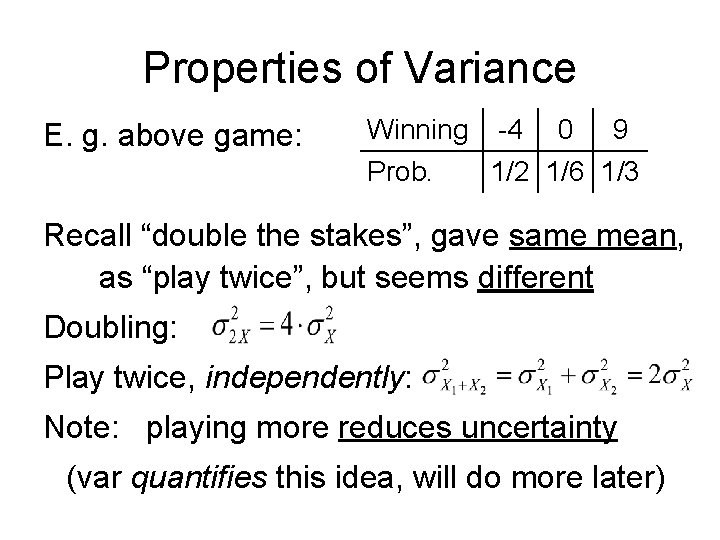
Properties of Variance E. g. above game: Winning -4 0 9 Prob. 1/2 1/6 1/3 Recall “double the stakes”, gave same mean, as “play twice”, but seems different Doubling: Play twice, independently: Note: playing more reduces uncertainty (var quantifies this idea, will do more later)
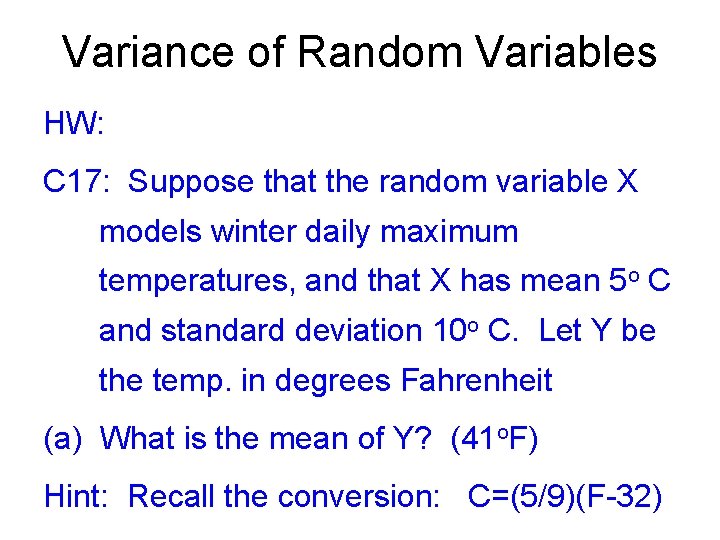
Variance of Random Variables HW: C 17: Suppose that the random variable X models winter daily maximum temperatures, and that X has mean 5 o C and standard deviation 10 o C. Let Y be the temp. in degrees Fahrenheit (a) What is the mean of Y? (41 o. F) Hint: Recall the conversion: C=(5/9)(F-32)
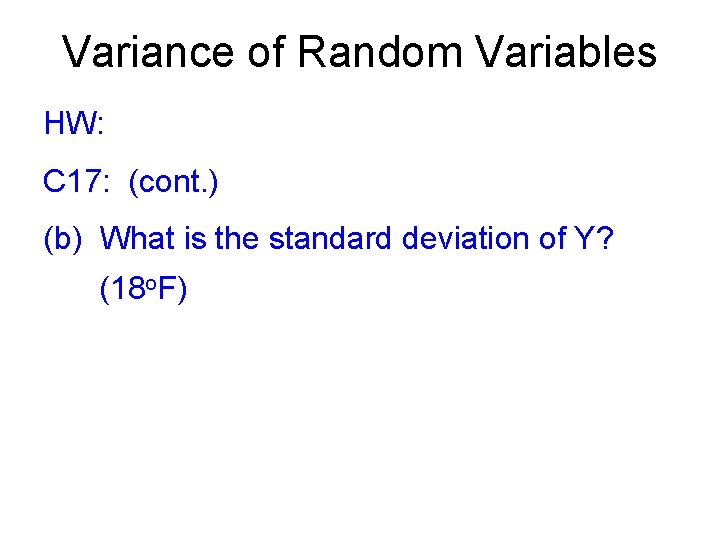
Variance of Random Variables HW: C 17: (cont. ) (b) What is the standard deviation of Y? (18 o. F)
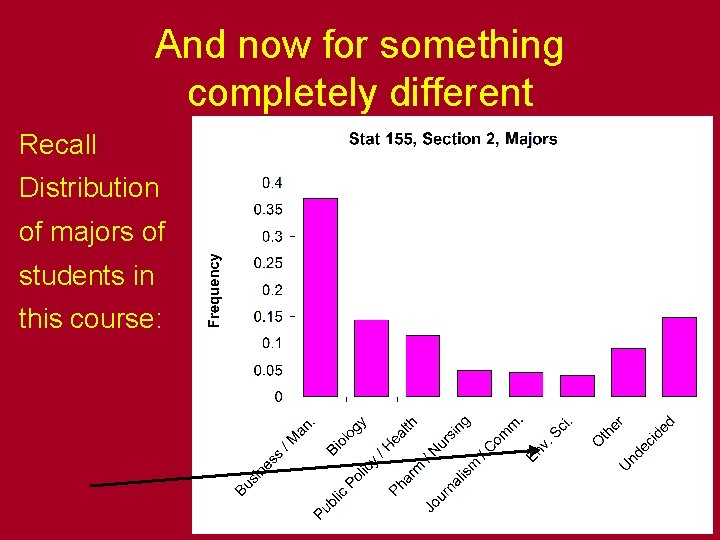
And now for something completely different Recall Distribution of majors of students in this course:
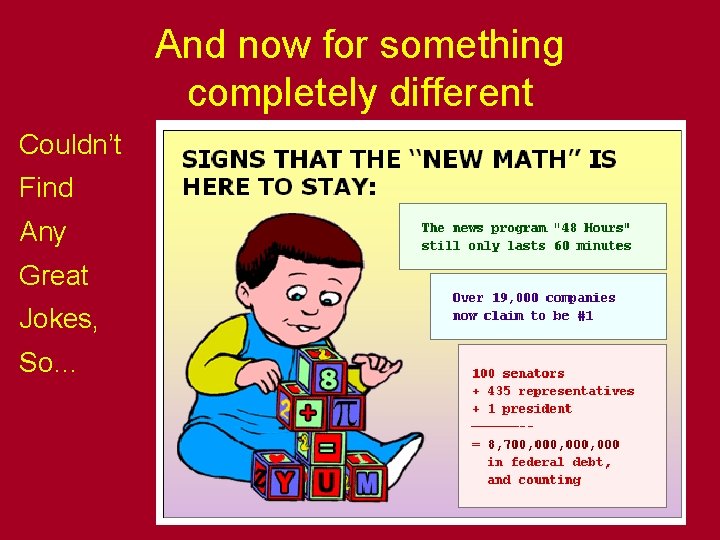
And now for something completely different Couldn’t Find Any Great Jokes, So…
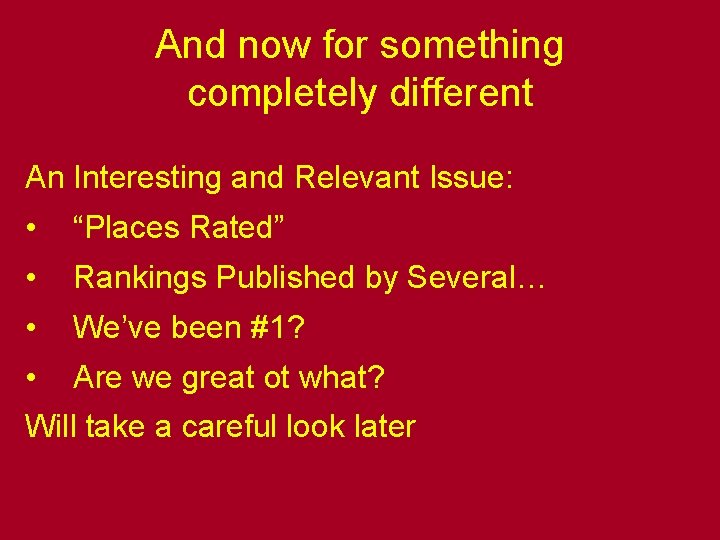
And now for something completely different An Interesting and Relevant Issue: • “Places Rated” • Rankings Published by Several… • We’ve been #1? • Are we great ot what? Will take a careful look later
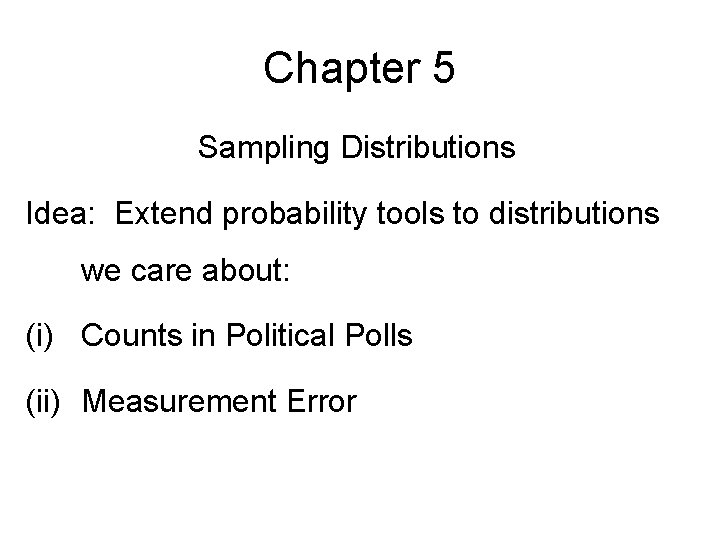
Chapter 5 Sampling Distributions Idea: Extend probability tools to distributions we care about: (i) Counts in Political Polls (ii) Measurement Error
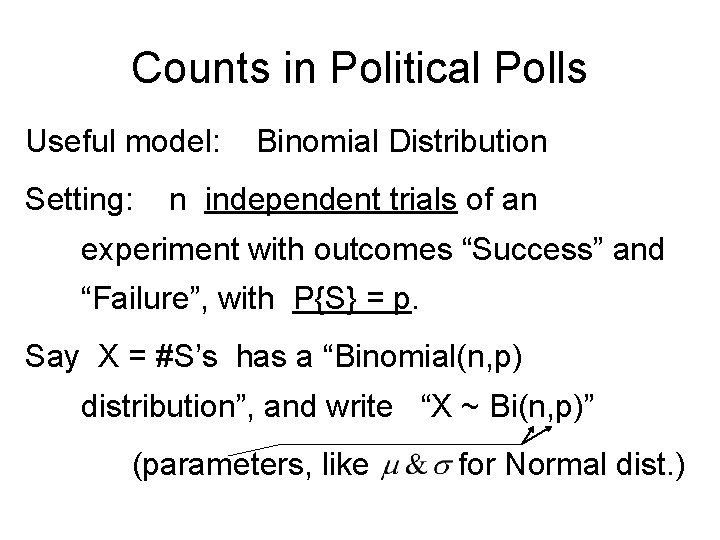
Counts in Political Polls Useful model: Setting: Binomial Distribution n independent trials of an experiment with outcomes “Success” and “Failure”, with P{S} = p. Say X = #S’s has a “Binomial(n, p) distribution”, and write “X ~ Bi(n, p)” (parameters, like for Normal dist. )
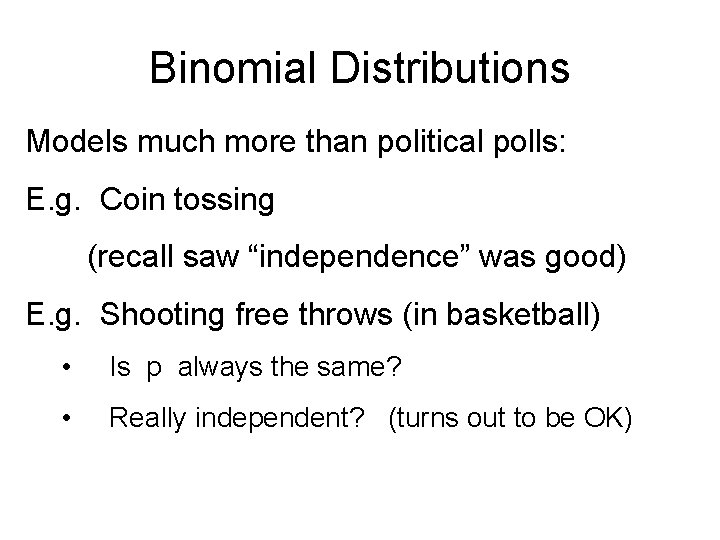
Binomial Distributions Models much more than political polls: E. g. Coin tossing (recall saw “independence” was good) E. g. Shooting free throws (in basketball) • Is p always the same? • Really independent? (turns out to be OK)
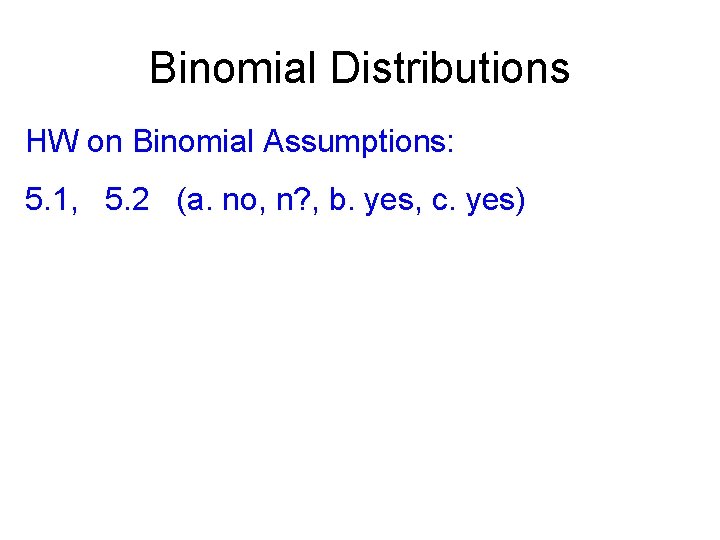
Binomial Distributions HW on Binomial Assumptions: 5. 1, 5. 2 (a. no, n? , b. yes, c. yes)
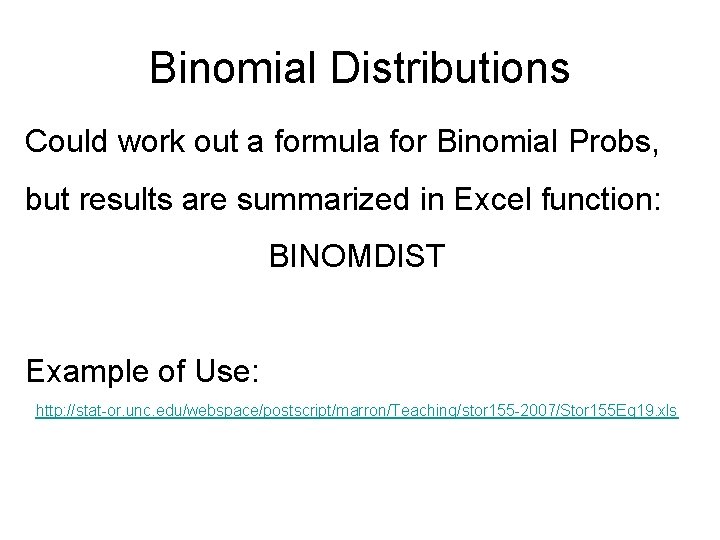
Binomial Distributions Could work out a formula for Binomial Probs, but results are summarized in Excel function: BINOMDIST Example of Use: http: //stat-or. unc. edu/webspace/postscript/marron/Teaching/stor 155 -2007/Stor 155 Eg 19. xls
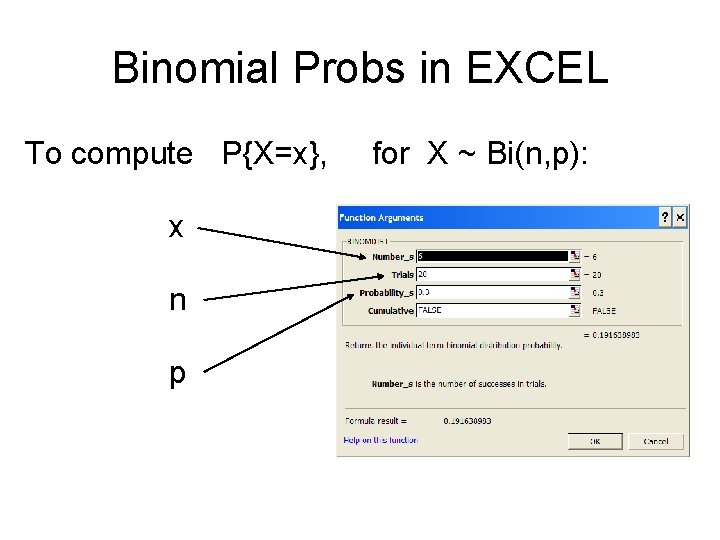
Binomial Probs in EXCEL To compute P{X=x}, x n p for X ~ Bi(n, p):
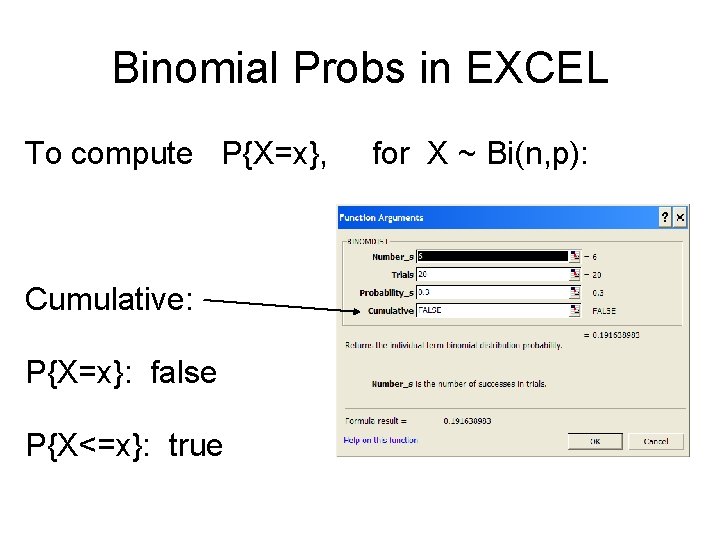
Binomial Probs in EXCEL To compute P{X=x}, Cumulative: P{X=x}: false P{X<=x}: true for X ~ Bi(n, p):
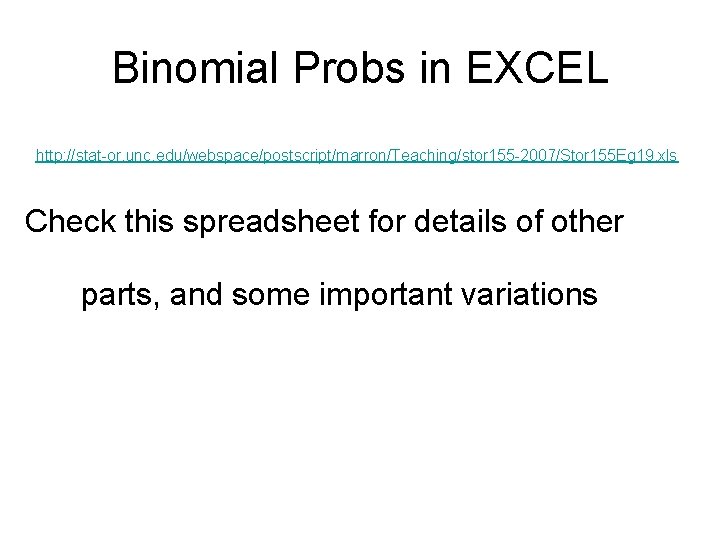
Binomial Probs in EXCEL http: //stat-or. unc. edu/webspace/postscript/marron/Teaching/stor 155 -2007/Stor 155 Eg 19. xls Check this spreadsheet for details of other parts, and some important variations
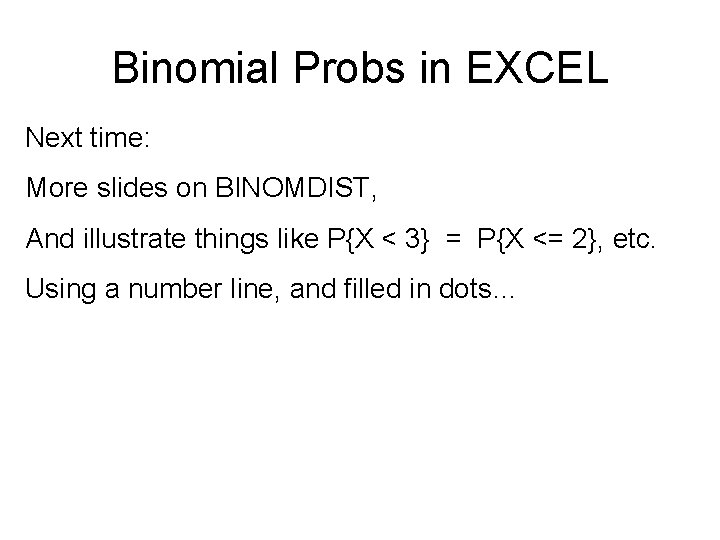
Binomial Probs in EXCEL Next time: More slides on BINOMDIST, And illustrate things like P{X < 3} = P{X <= 2}, etc. Using a number line, and filled in dots…
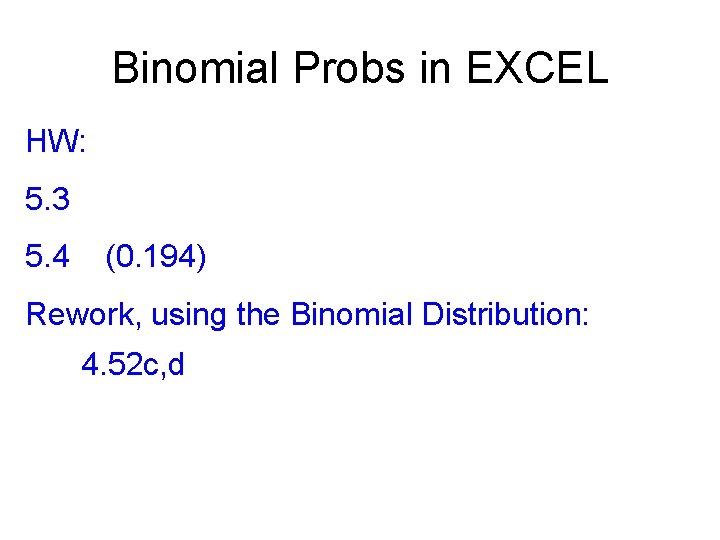
Binomial Probs in EXCEL HW: 5. 3 5. 4 (0. 194) Rework, using the Binomial Distribution: 4. 52 c, d
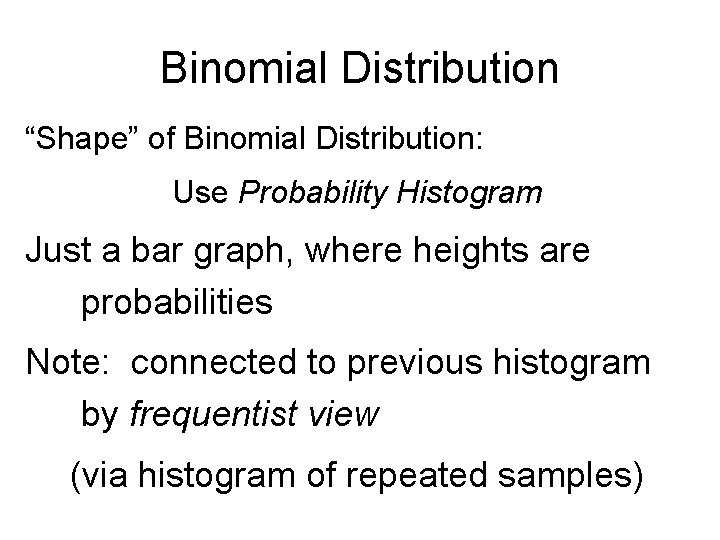
Binomial Distribution “Shape” of Binomial Distribution: Use Probability Histogram Just a bar graph, where heights are probabilities Note: connected to previous histogram by frequentist view (via histogram of repeated samples)
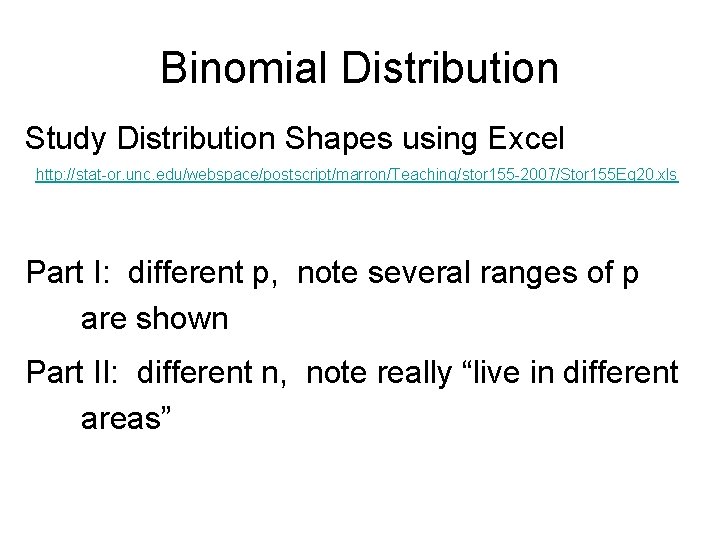
Binomial Distribution Study Distribution Shapes using Excel http: //stat-or. unc. edu/webspace/postscript/marron/Teaching/stor 155 -2007/Stor 155 Eg 20. xls Part I: different p, note several ranges of p are shown Part II: different n, note really “live in different areas”
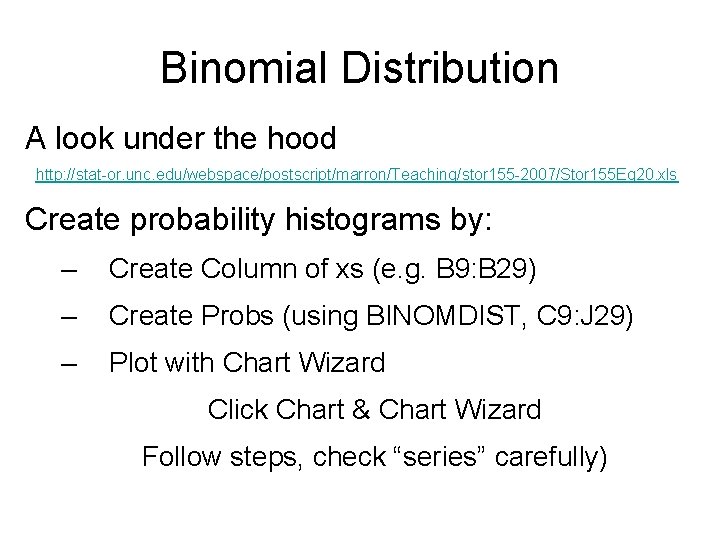
Binomial Distribution A look under the hood http: //stat-or. unc. edu/webspace/postscript/marron/Teaching/stor 155 -2007/Stor 155 Eg 20. xls Create probability histograms by: – Create Column of xs (e. g. B 9: B 29) – Create Probs (using BINOMDIST, C 9: J 29) – Plot with Chart Wizard Click Chart & Chart Wizard Follow steps, check “series” carefully)
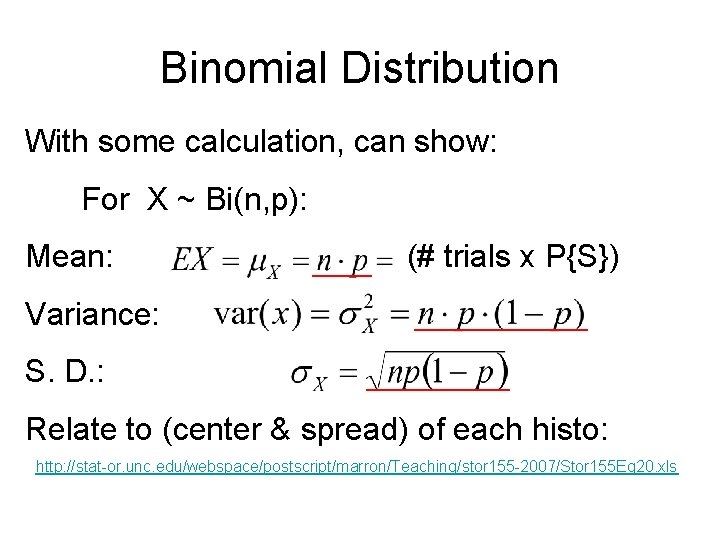
Binomial Distribution With some calculation, can show: For X ~ Bi(n, p): Mean: (# trials x P{S}) Variance: S. D. : Relate to (center & spread) of each histo: http: //stat-or. unc. edu/webspace/postscript/marron/Teaching/stor 155 -2007/Stor 155 Eg 20. xls
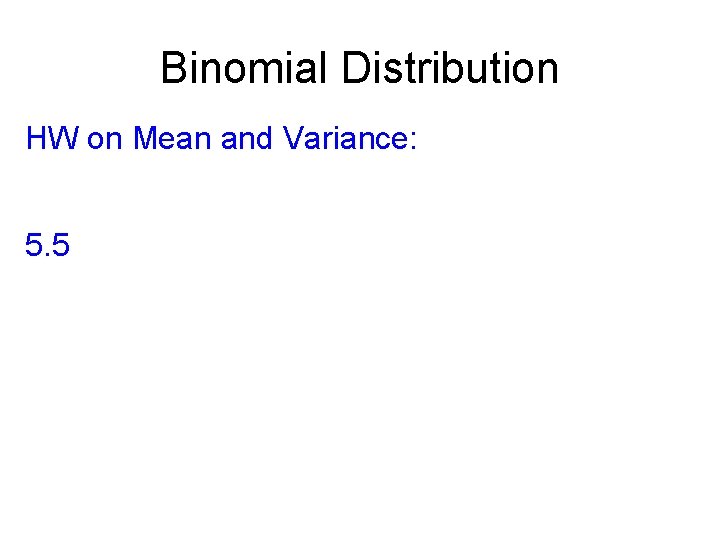
Binomial Distribution HW on Mean and Variance: 5. 5
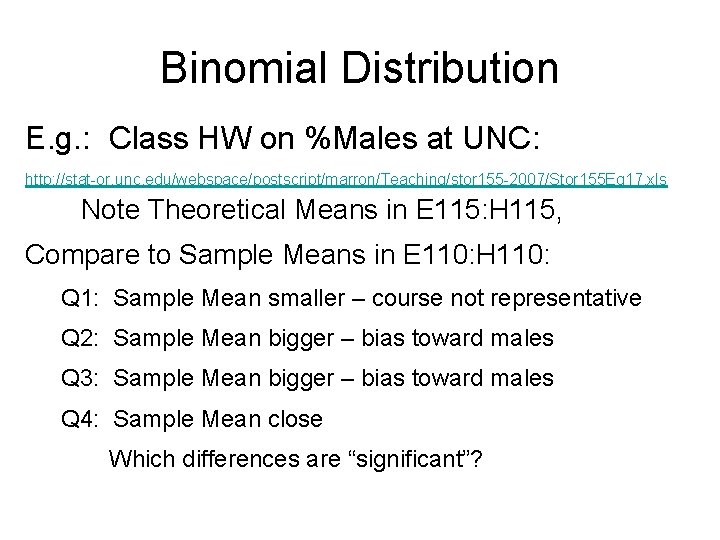
Binomial Distribution E. g. : Class HW on %Males at UNC: http: //stat-or. unc. edu/webspace/postscript/marron/Teaching/stor 155 -2007/Stor 155 Eg 17. xls Note Theoretical Means in E 115: H 115, Compare to Sample Means in E 110: H 110: Q 1: Sample Mean smaller – course not representative Q 2: Sample Mean bigger – bias toward males Q 3: Sample Mean bigger – bias toward males Q 4: Sample Mean close Which differences are “significant”?
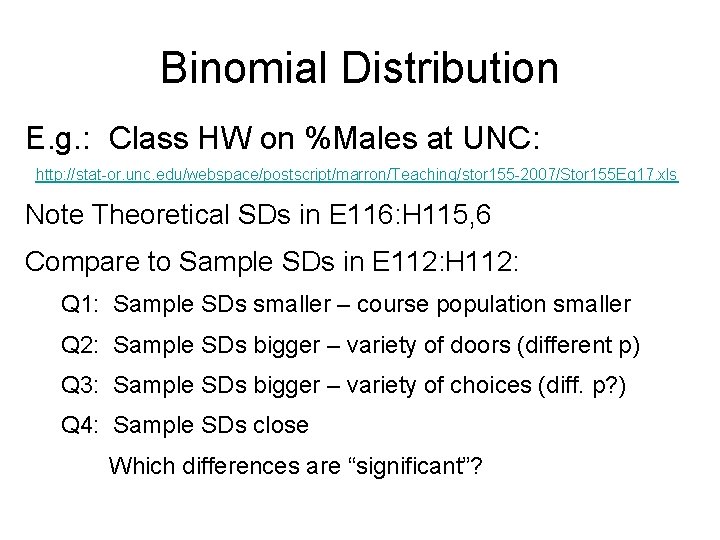
Binomial Distribution E. g. : Class HW on %Males at UNC: http: //stat-or. unc. edu/webspace/postscript/marron/Teaching/stor 155 -2007/Stor 155 Eg 17. xls Note Theoretical SDs in E 116: H 115, 6 Compare to Sample SDs in E 112: H 112: Q 1: Sample SDs smaller – course population smaller Q 2: Sample SDs bigger – variety of doors (different p) Q 3: Sample SDs bigger – variety of choices (diff. p? ) Q 4: Sample SDs close Which differences are “significant”?
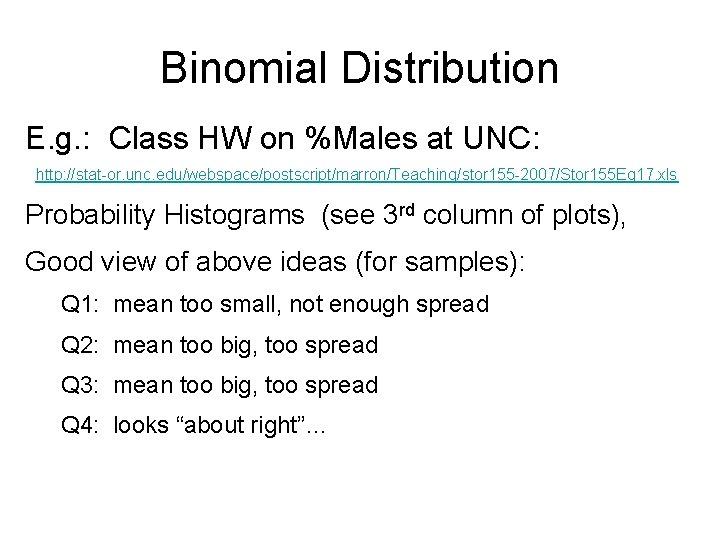
Binomial Distribution E. g. : Class HW on %Males at UNC: http: //stat-or. unc. edu/webspace/postscript/marron/Teaching/stor 155 -2007/Stor 155 Eg 17. xls Probability Histograms (see 3 rd column of plots), Good view of above ideas (for samples): Q 1: mean too small, not enough spread Q 2: mean too big, too spread Q 3: mean too big, too spread Q 4: looks “about right”…
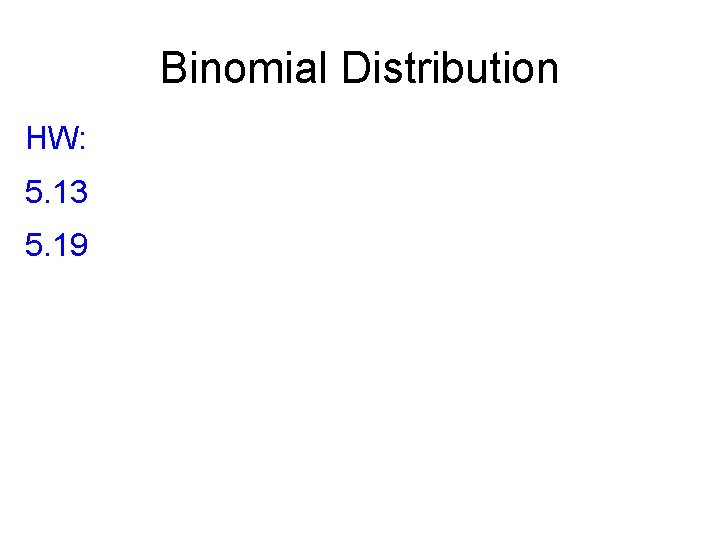
Binomial Distribution HW: 5. 13 5. 19
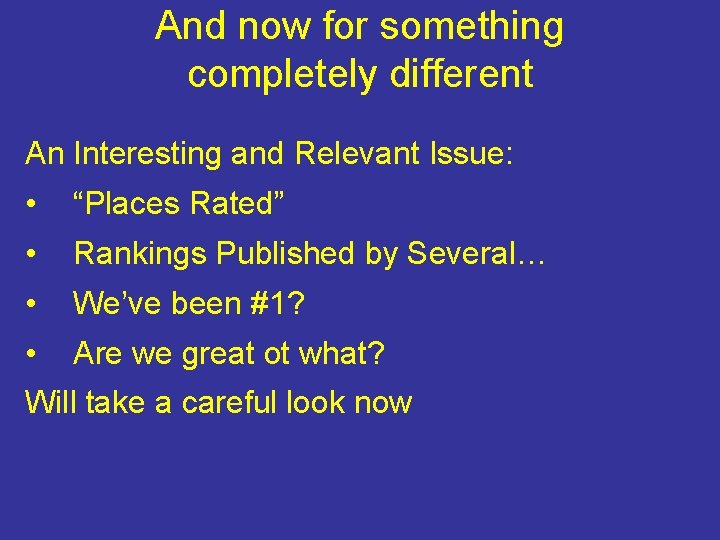
And now for something completely different An Interesting and Relevant Issue: • “Places Rated” • Rankings Published by Several… • We’ve been #1? • Are we great ot what? Will take a careful look now
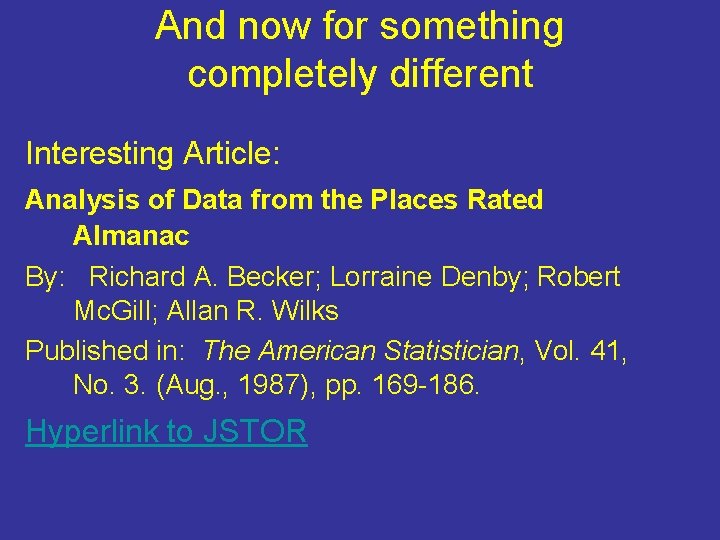
And now for something completely different Interesting Article: Analysis of Data from the Places Rated Almanac By: Richard A. Becker; Lorraine Denby; Robert Mc. Gill; Allan R. Wilks Published in: The American Statistician, Vol. 41, No. 3. (Aug. , 1987), pp. 169 -186. Hyperlink to JSTOR
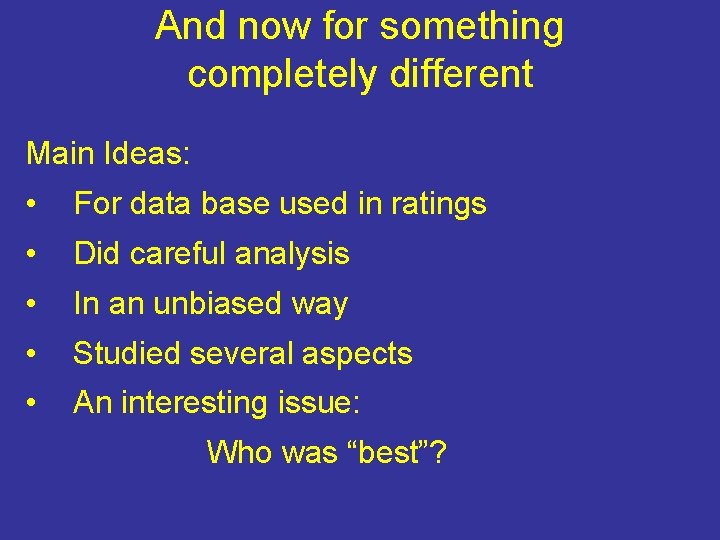
And now for something completely different Main Ideas: • For data base used in ratings • Did careful analysis • In an unbiased way • Studied several aspects • An interesting issue: Who was “best”?
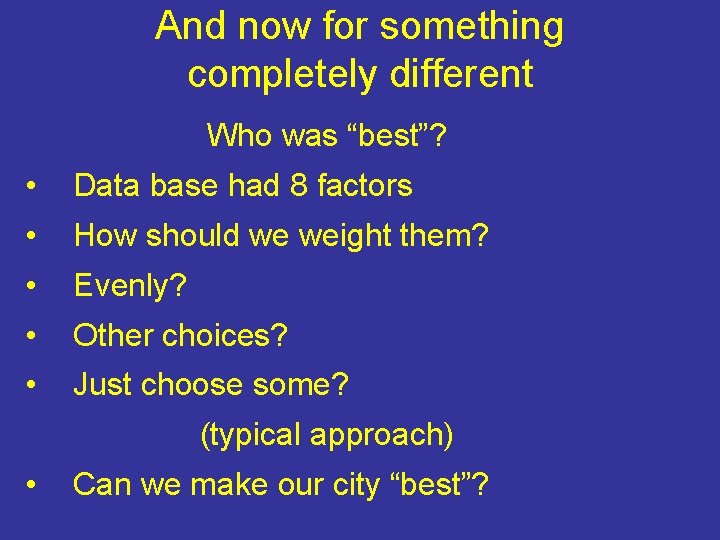
And now for something completely different Who was “best”? • Data base had 8 factors • How should we weight them? • Evenly? • Other choices? • Just choose some? (typical approach) • Can we make our city “best”?
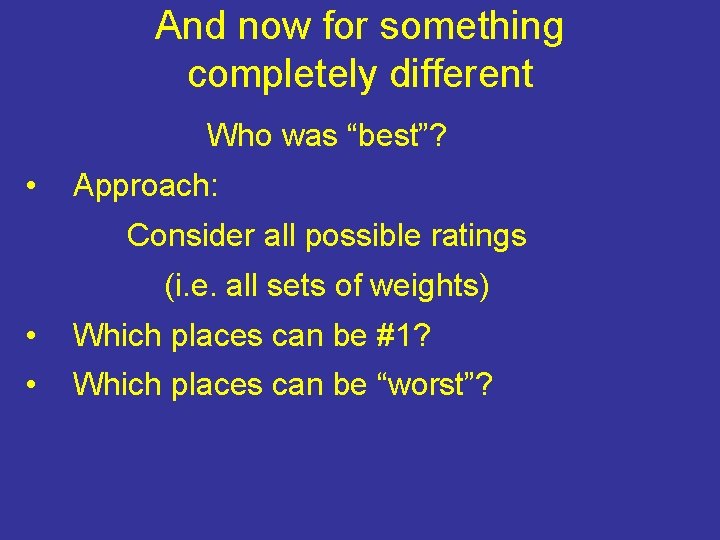
And now for something completely different Who was “best”? • Approach: Consider all possible ratings (i. e. all sets of weights) • Which places can be #1? • Which places can be “worst”?
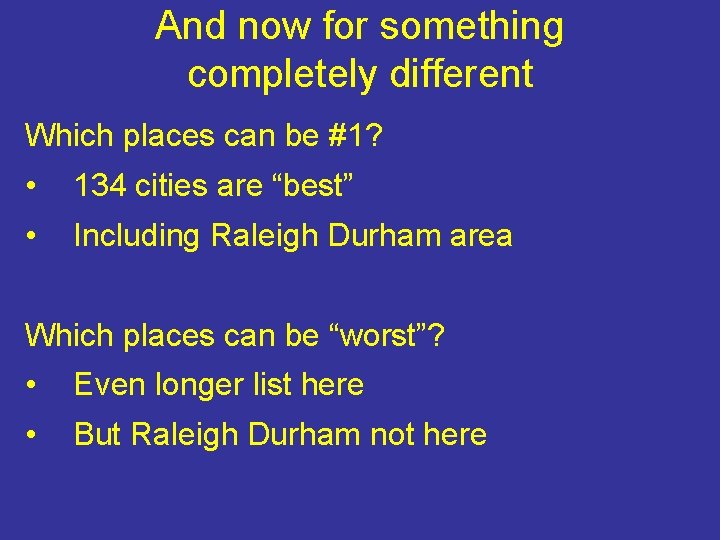
And now for something completely different Which places can be #1? • 134 cities are “best” • Including Raleigh Durham area Which places can be “worst”? • Even longer list here • But Raleigh Durham not here
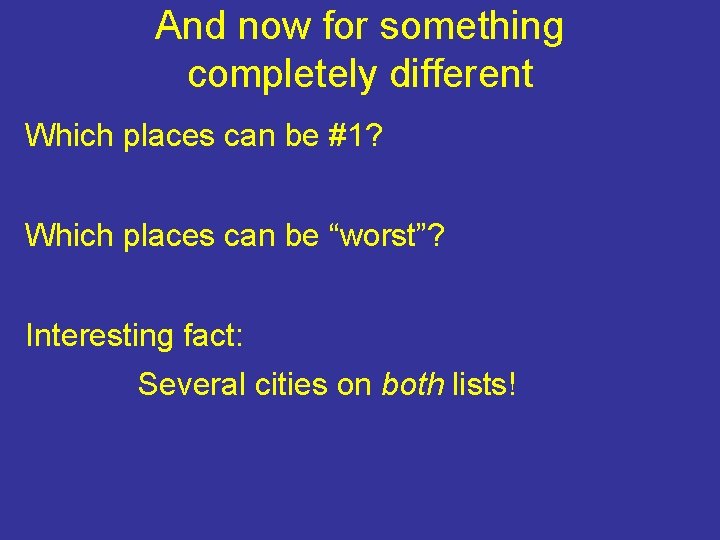
And now for something completely different Which places can be #1? Which places can be “worst”? Interesting fact: Several cities on both lists!
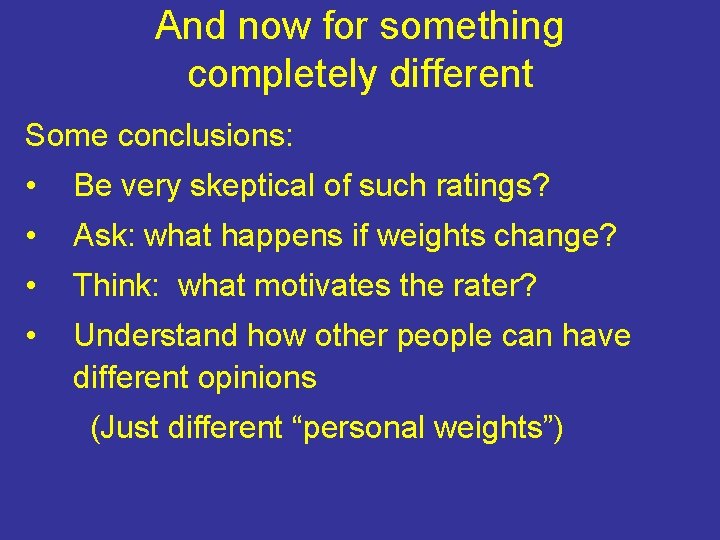
And now for something completely different Some conclusions: • Be very skeptical of such ratings? • Ask: what happens if weights change? • Think: what motivates the rater? • Understand how other people can have different opinions (Just different “personal weights”)
Random assignment vs random sampling
Random assignment vs random selection
Past and future continuous tense
Past simple future simple present simple
Discrete time processing of continuous time signals
What do salir, decir and venir have in common?
Wac 296 155
Monarc howitzer
Stanford cs 155
Track design handbook for light rail transit
Cs 155
Convenio 155
155 as a fraction in simplest form
Scalar is to vector as regents
Cs 155
Cs 155
Cs 155
Aapm tg 155
Cs 155
Convenio 155
Cos½
3 doğal sayının toplamı 155'tir
Pout tif
Nom 155
Math learning center wvu
La promesse 155
Variabel acak adalah
Probability variance formula
Ojikax.com
Continuous variable
Continuous random variable example
Properties of continuous random variable
Continuous random variable example
Variance of a continuous random variable
Median of continuous distribution
For minutes. start.
Random moment time study texas
Fairbanks time study
Present simple vs present continuous
Wake up past simple form
The past of add
For the last time sentence
When do you your homework
Last time we discussed
Last time we discussed
Ziua drapelului republicii moldova ppt
Idexx urine protein creatinine ratio
Qualcomm xr apis unreal engine unityverge
Otrokářský stát znaky
Quirks stat testing
Sys stat h
Statquest