Soc 3306 a Lecture 3 Developing the Research
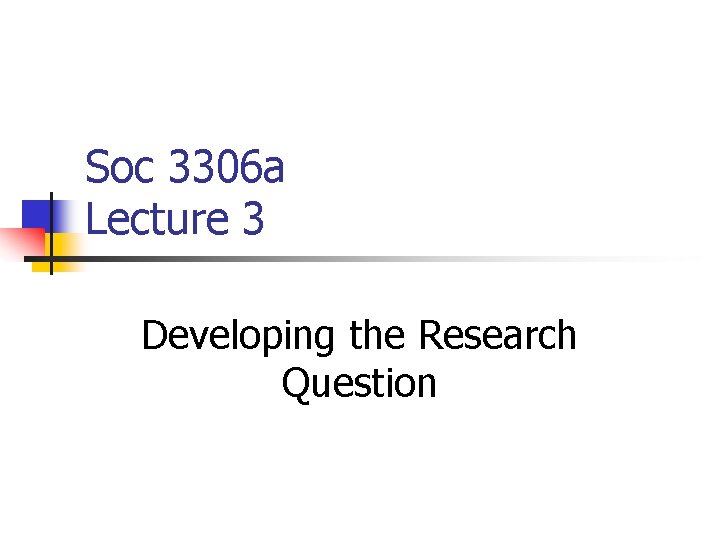
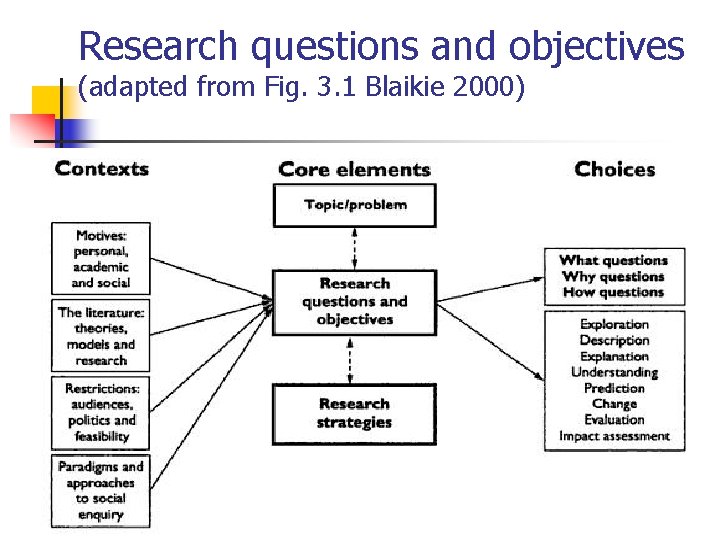
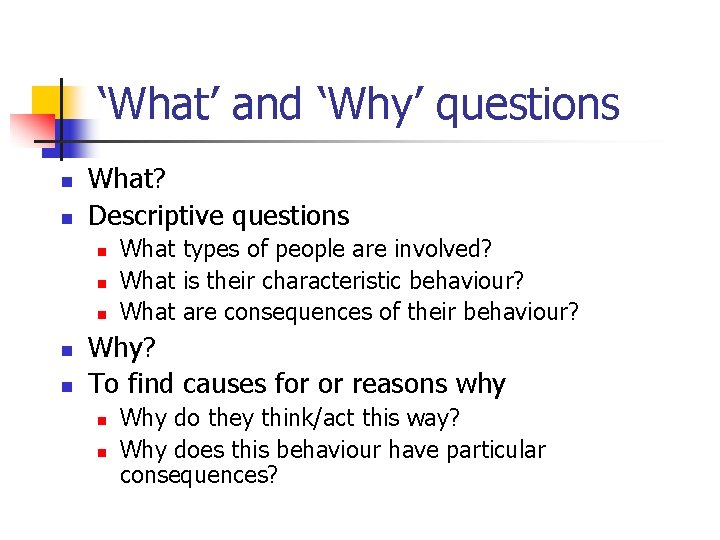
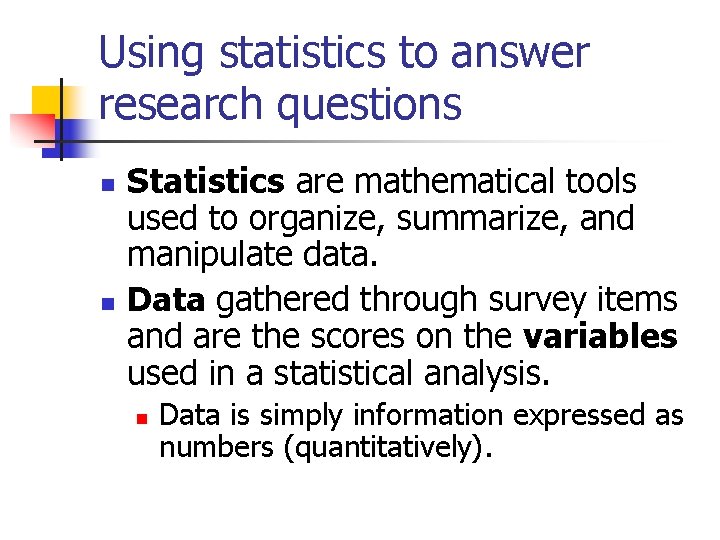
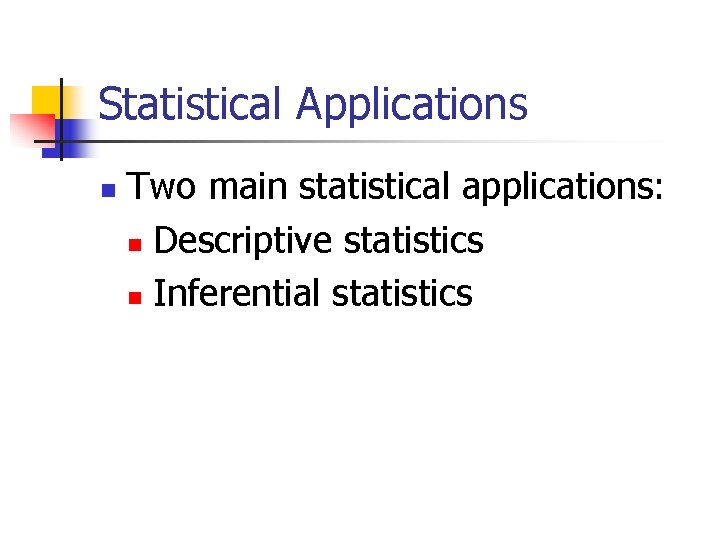
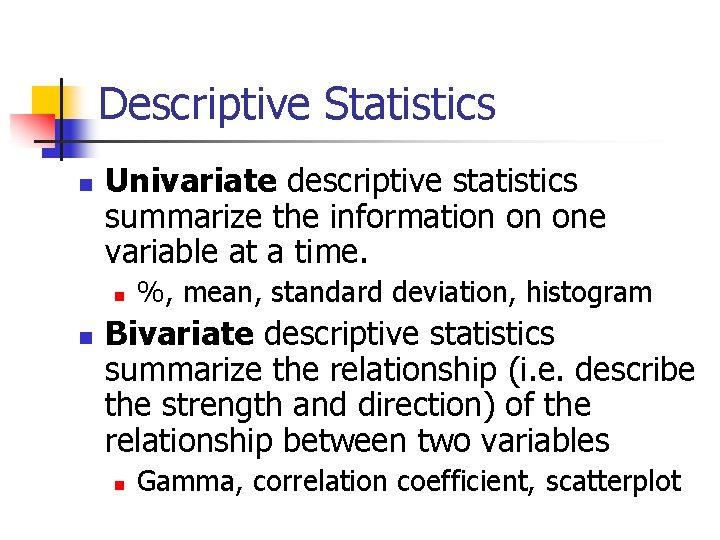
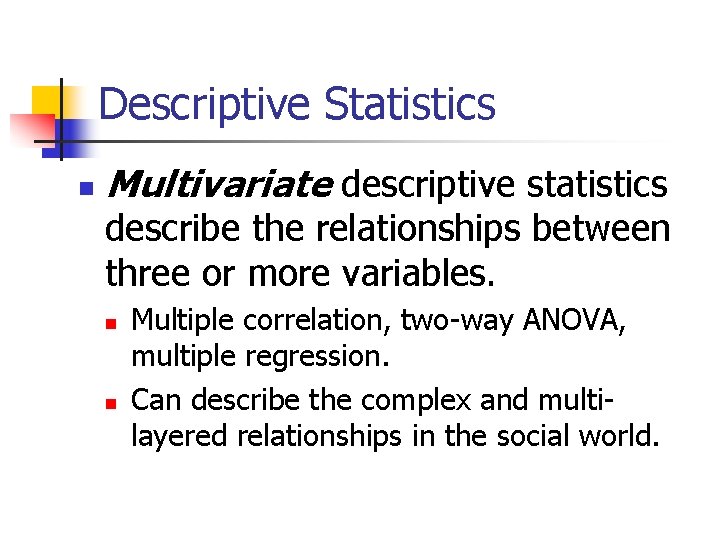
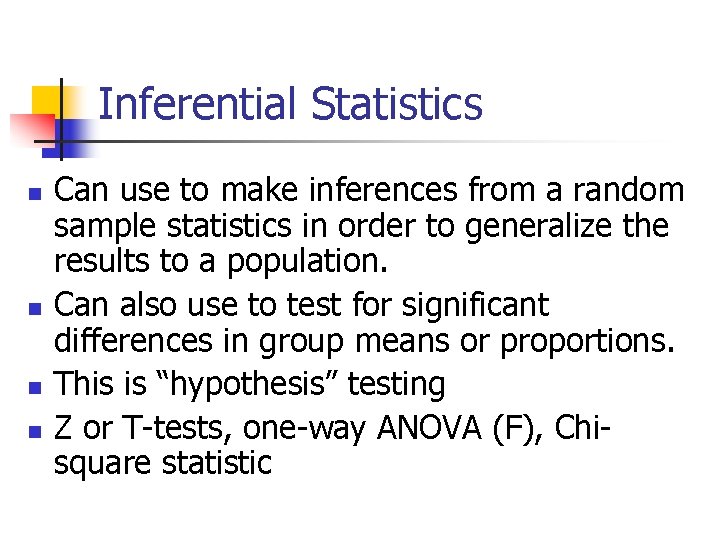
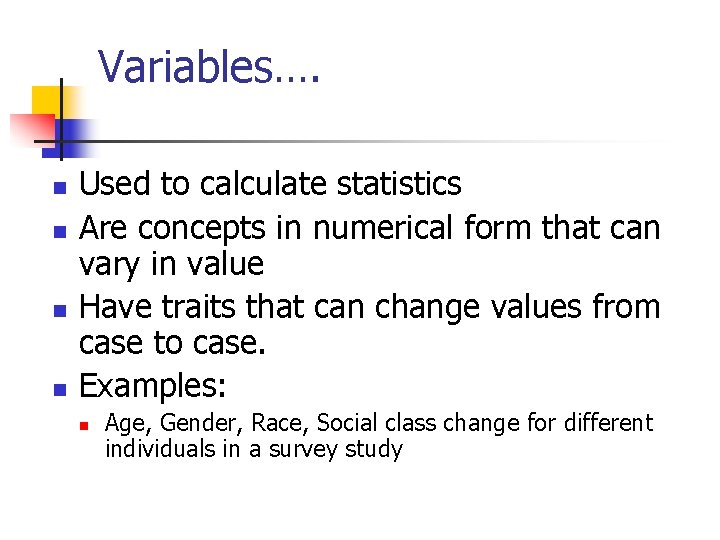
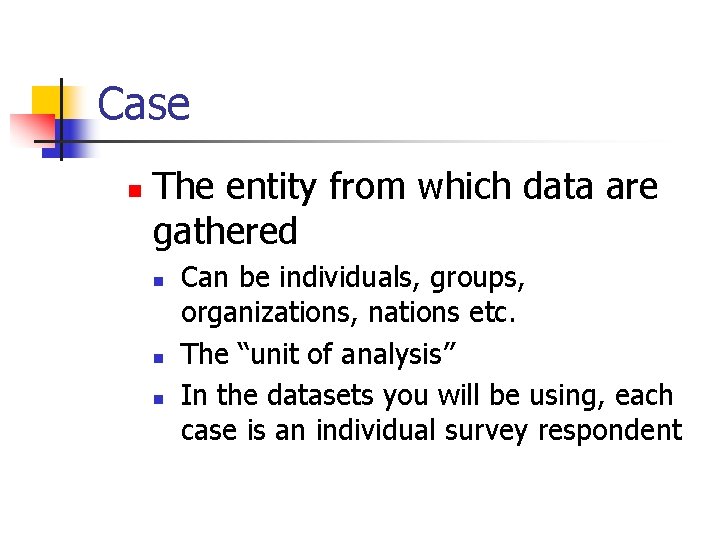
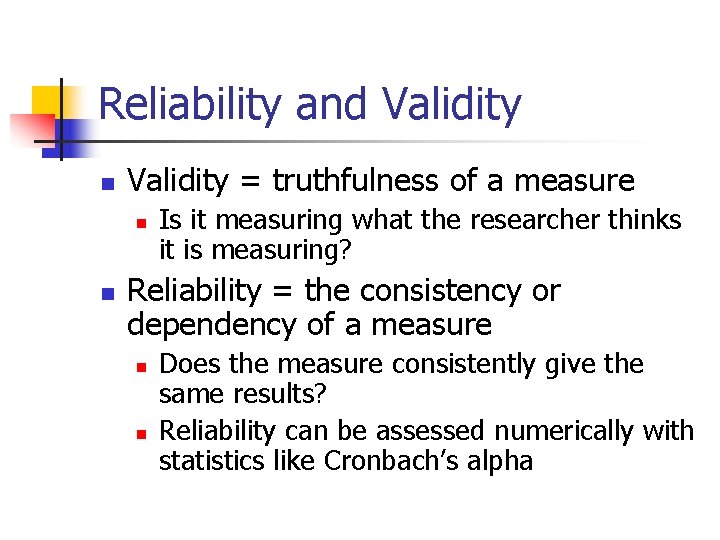
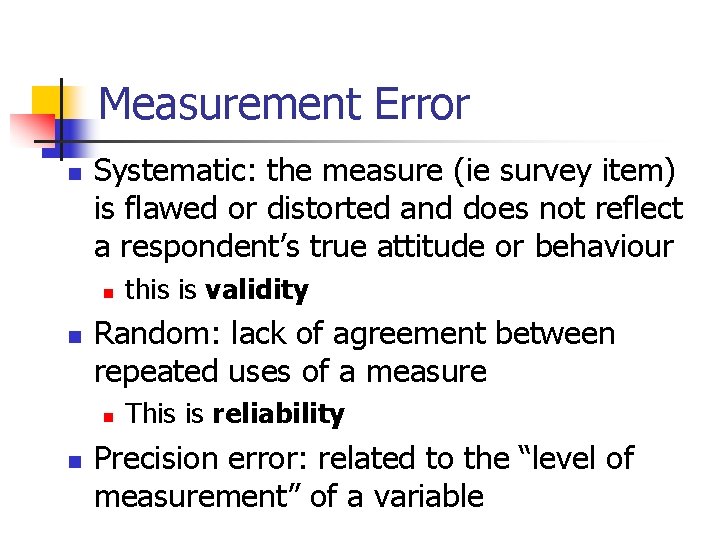
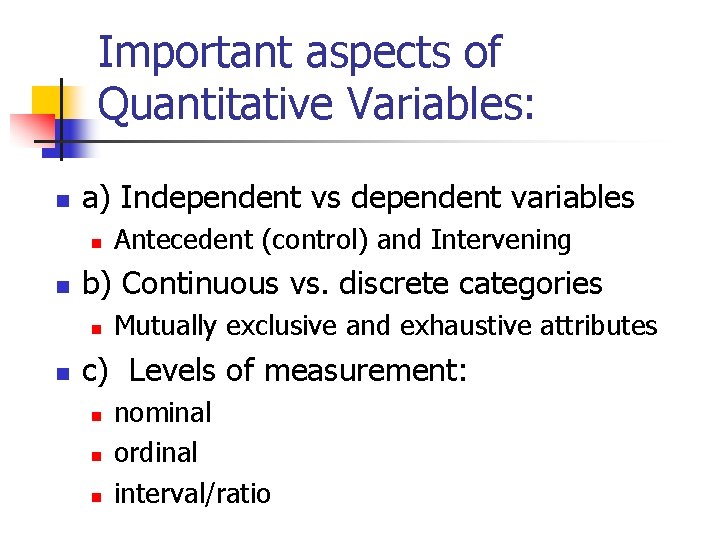
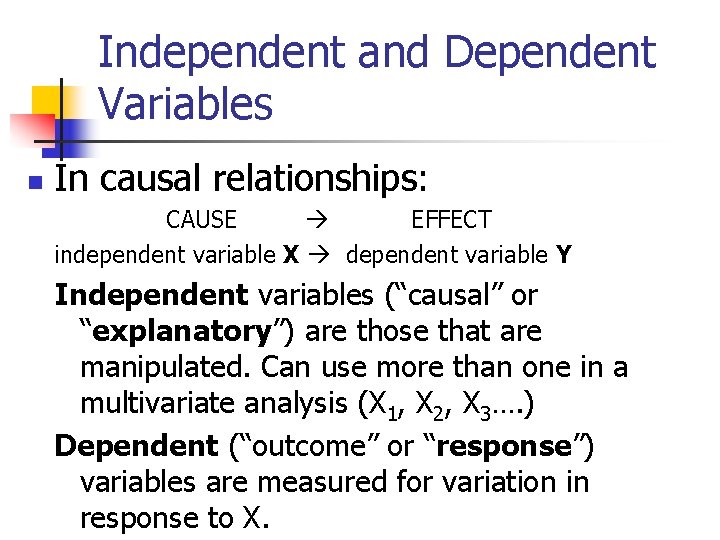
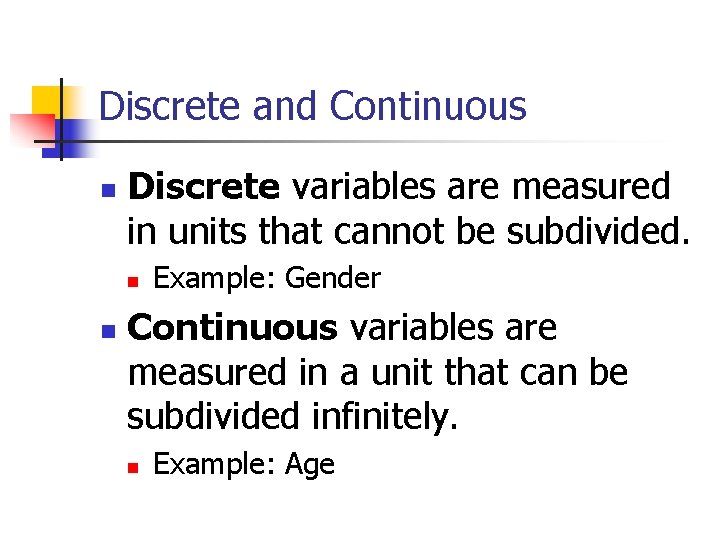
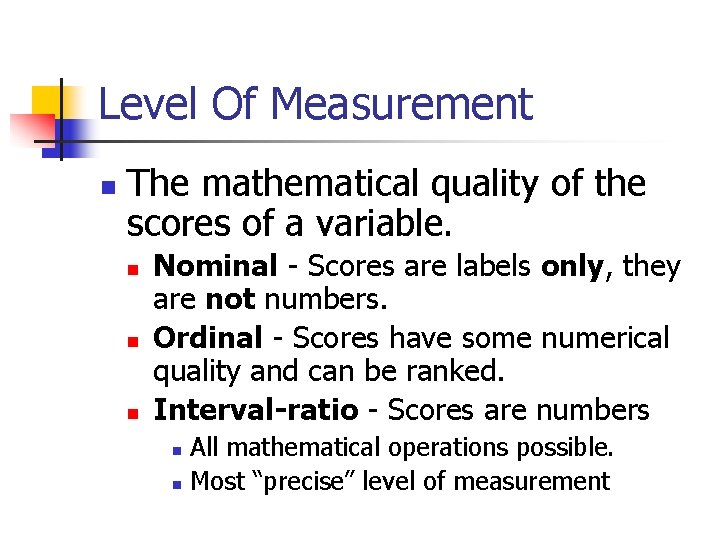
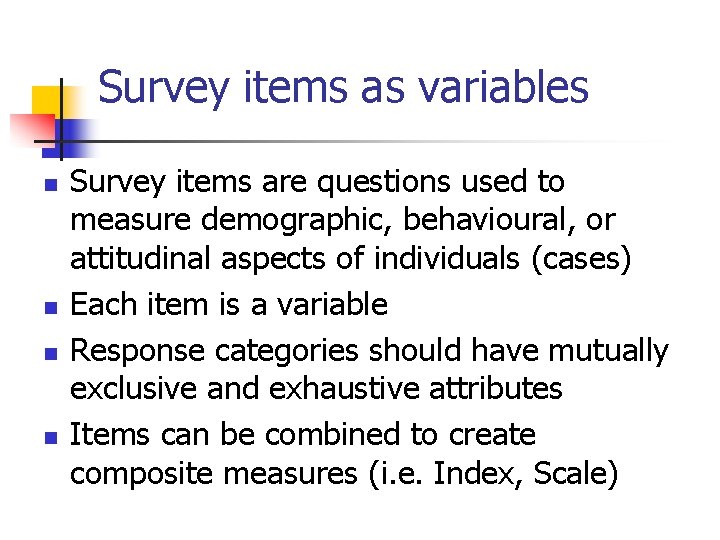
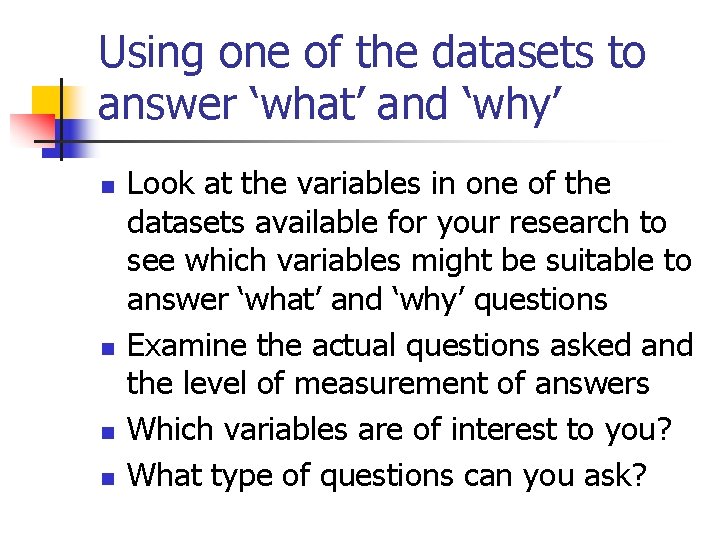
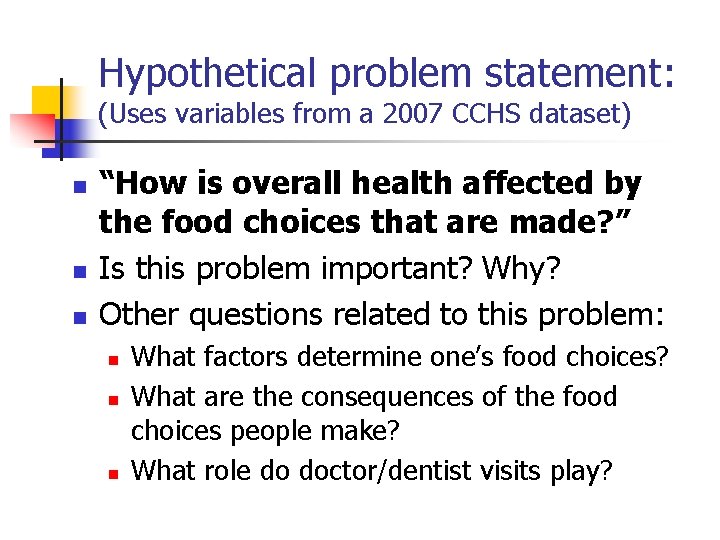
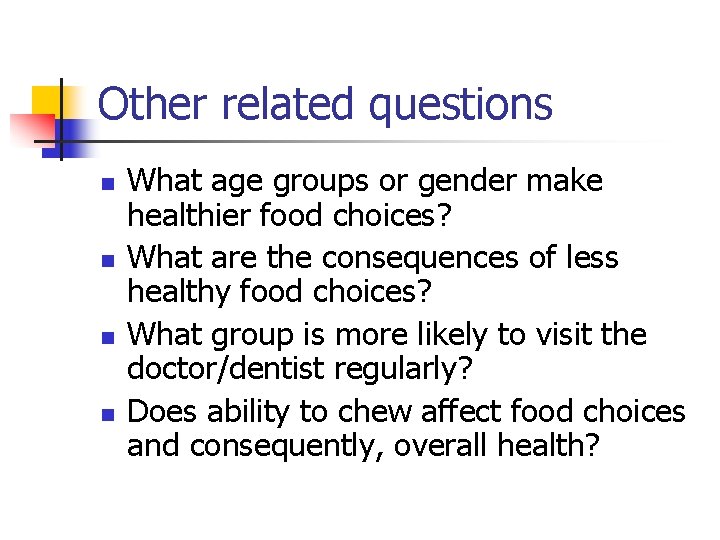
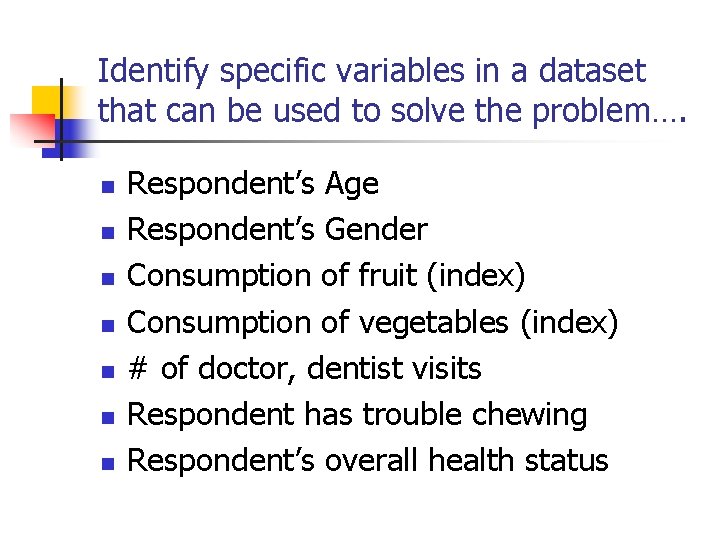
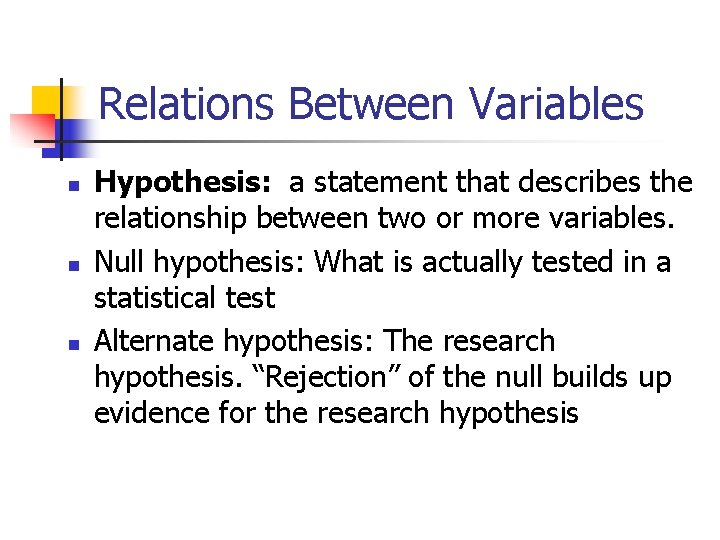
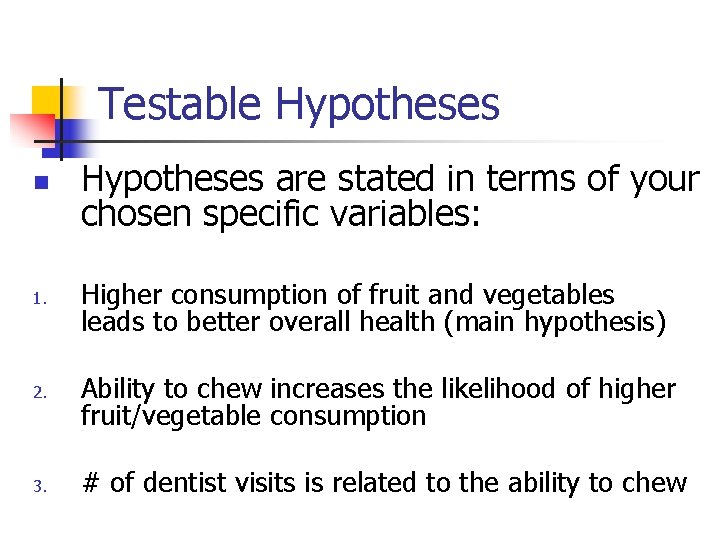
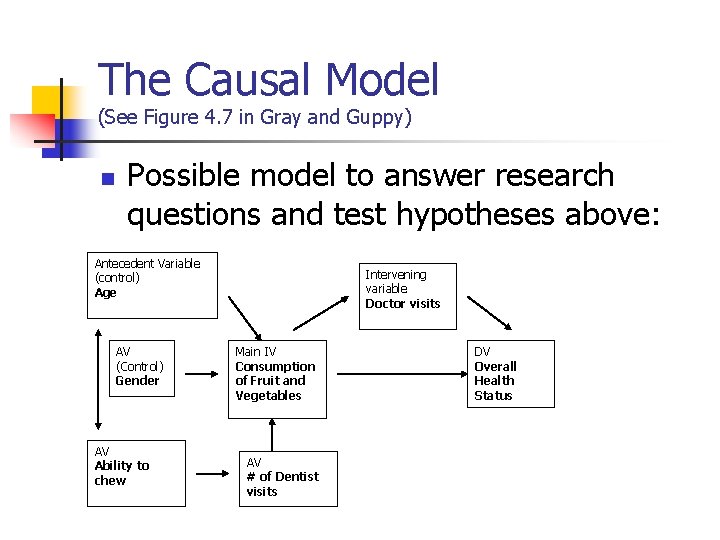
- Slides: 24
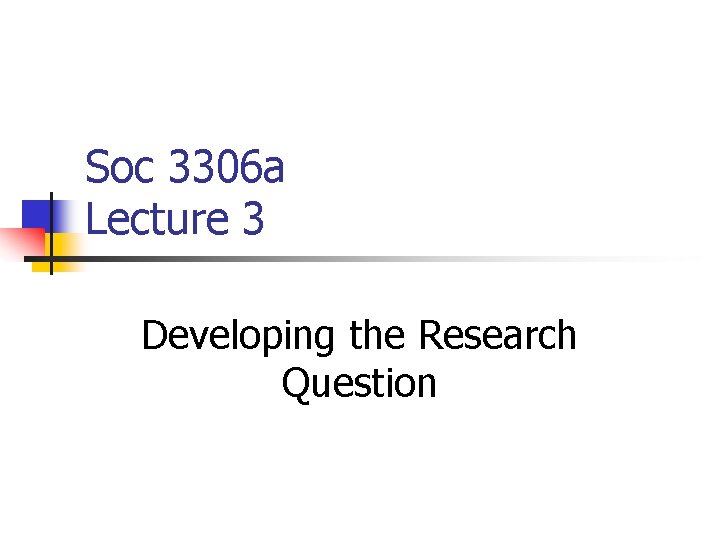
Soc 3306 a Lecture 3 Developing the Research Question
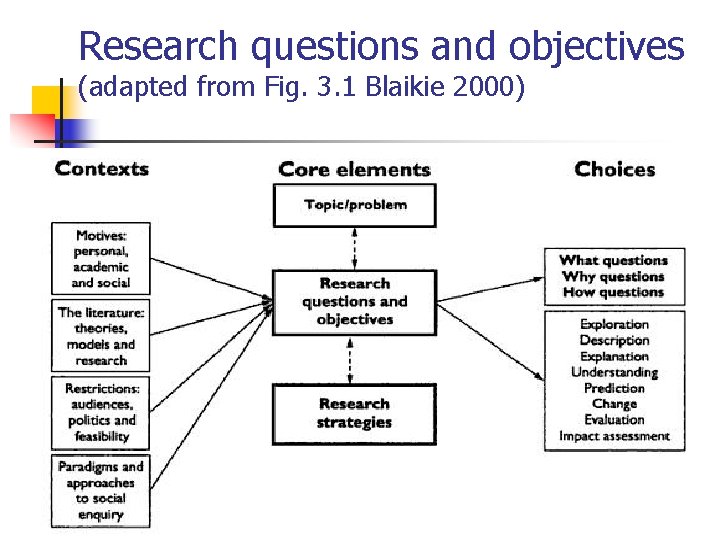
Research questions and objectives (adapted from Fig. 3. 1 Blaikie 2000)
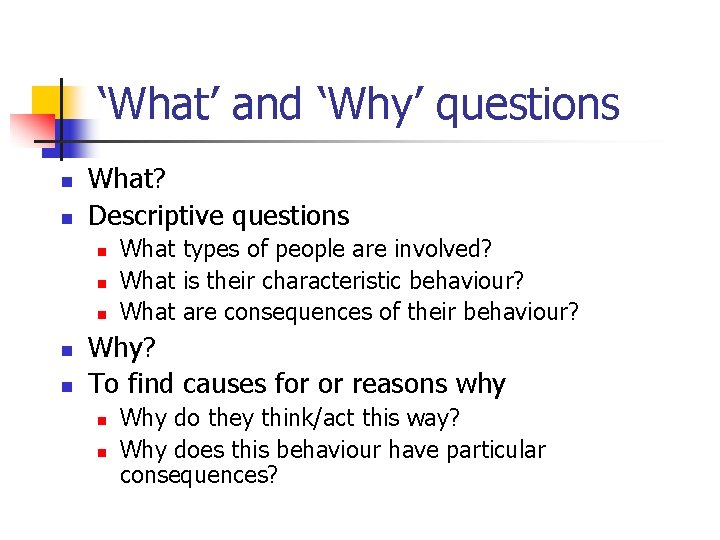
‘What’ and ‘Why’ questions n n What? Descriptive questions n n n What types of people are involved? What is their characteristic behaviour? What are consequences of their behaviour? Why? To find causes for or reasons why n n Why do they think/act this way? Why does this behaviour have particular consequences?
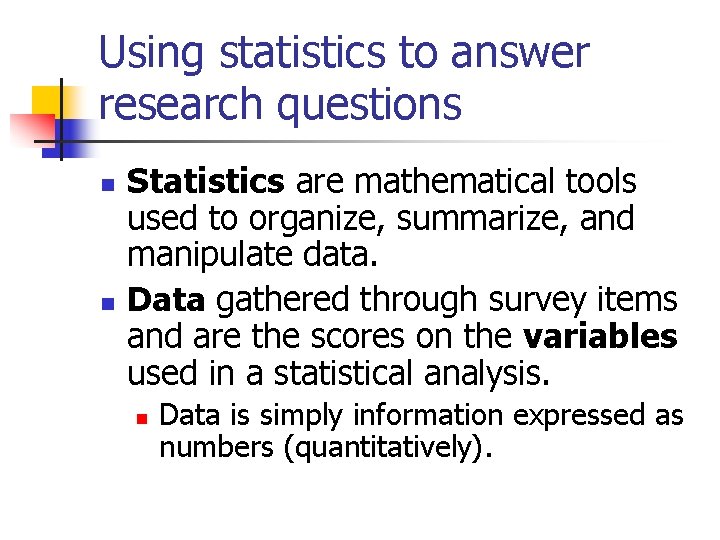
Using statistics to answer research questions n n Statistics are mathematical tools used to organize, summarize, and manipulate data. Data gathered through survey items and are the scores on the variables used in a statistical analysis. n Data is simply information expressed as numbers (quantitatively).
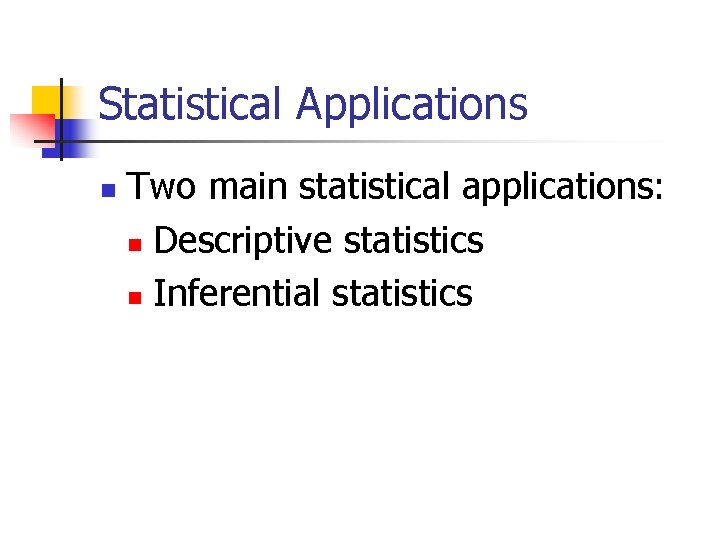
Statistical Applications n Two main statistical applications: n Descriptive statistics n Inferential statistics
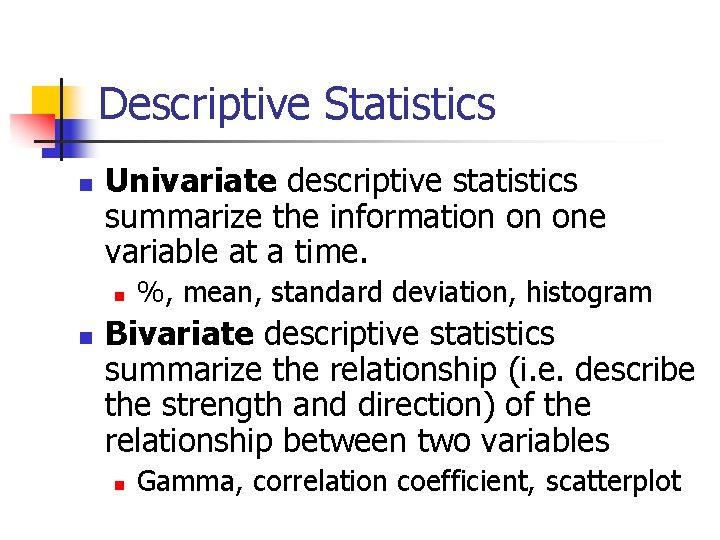
Descriptive Statistics n Univariate descriptive statistics summarize the information on one variable at a time. n n %, mean, standard deviation, histogram Bivariate descriptive statistics summarize the relationship (i. e. describe the strength and direction) of the relationship between two variables n Gamma, correlation coefficient, scatterplot
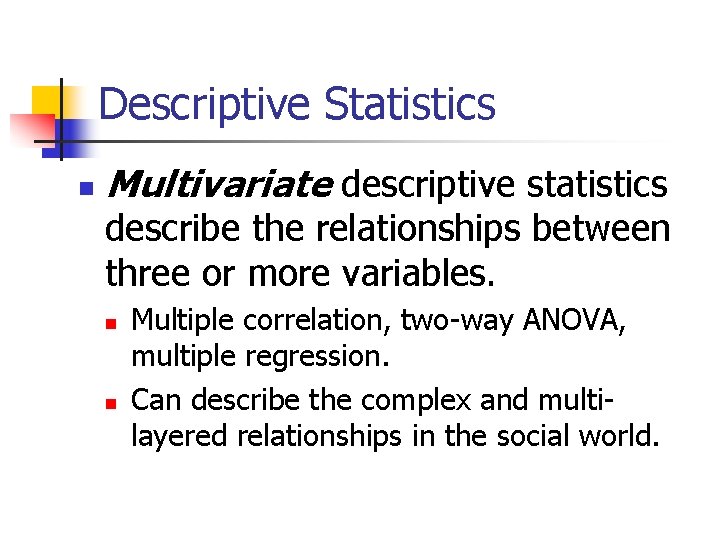
Descriptive Statistics n Multivariate descriptive statistics describe the relationships between three or more variables. n n Multiple correlation, two-way ANOVA, multiple regression. Can describe the complex and multilayered relationships in the social world.
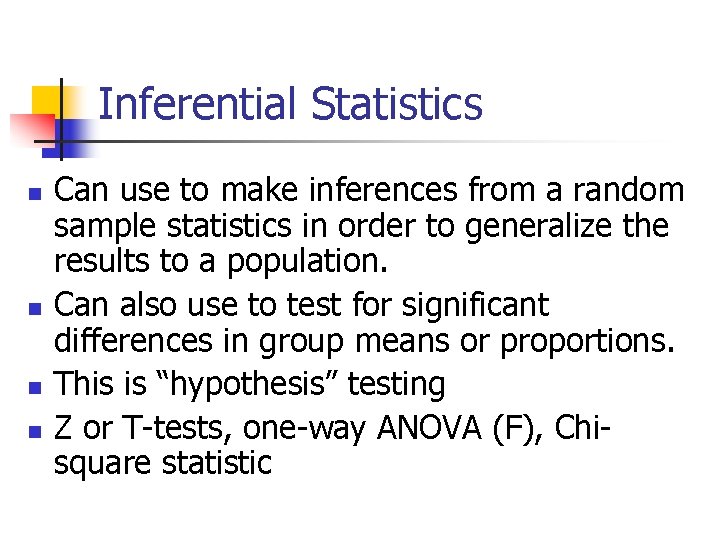
Inferential Statistics n n Can use to make inferences from a random sample statistics in order to generalize the results to a population. Can also use to test for significant differences in group means or proportions. This is “hypothesis” testing Z or T-tests, one-way ANOVA (F), Chisquare statistic
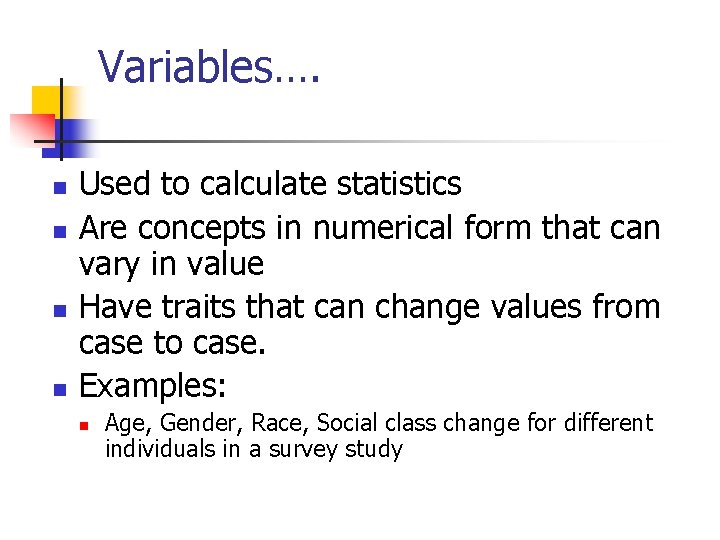
Variables…. n n Used to calculate statistics Are concepts in numerical form that can vary in value Have traits that can change values from case to case. Examples: n Age, Gender, Race, Social class change for different individuals in a survey study
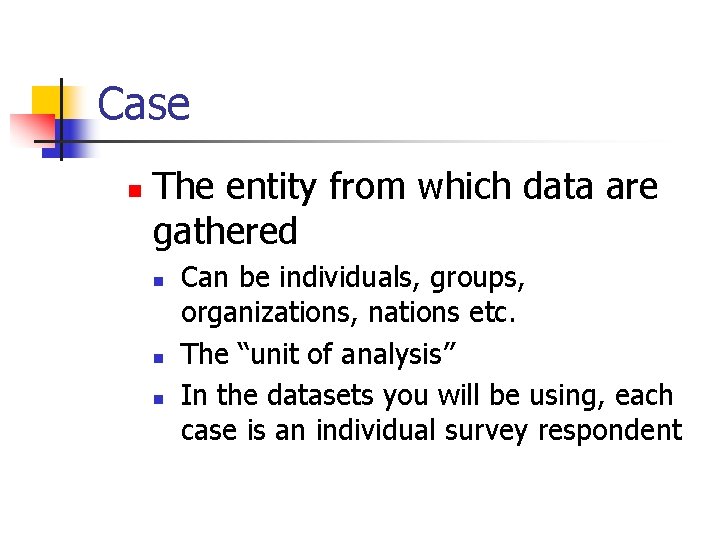
Case n The entity from which data are gathered n n n Can be individuals, groups, organizations, nations etc. The “unit of analysis” In the datasets you will be using, each case is an individual survey respondent
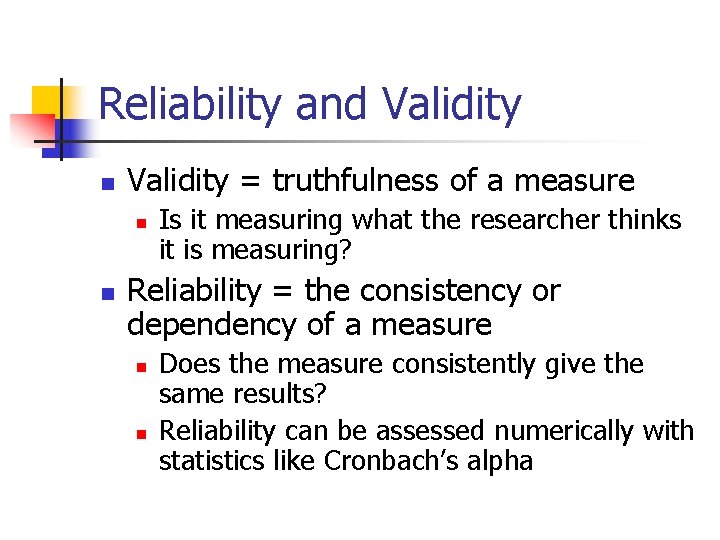
Reliability and Validity n Validity = truthfulness of a measure n n Is it measuring what the researcher thinks it is measuring? Reliability = the consistency or dependency of a measure n n Does the measure consistently give the same results? Reliability can be assessed numerically with statistics like Cronbach’s alpha
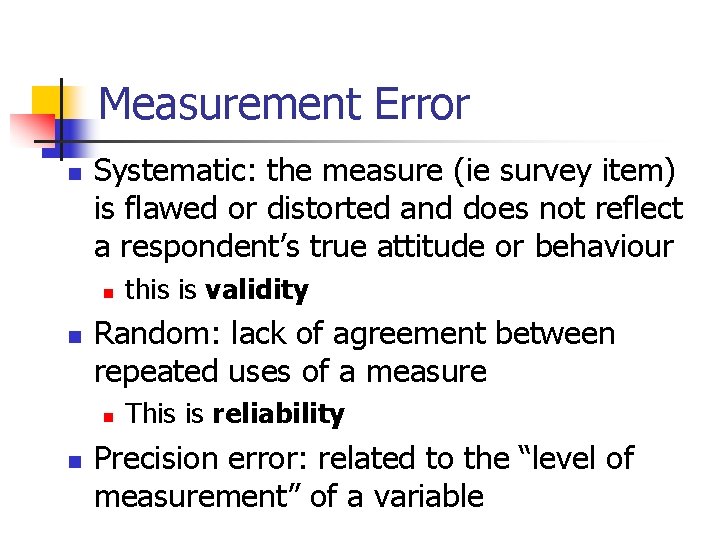
Measurement Error n Systematic: the measure (ie survey item) is flawed or distorted and does not reflect a respondent’s true attitude or behaviour n n Random: lack of agreement between repeated uses of a measure n n this is validity This is reliability Precision error: related to the “level of measurement” of a variable
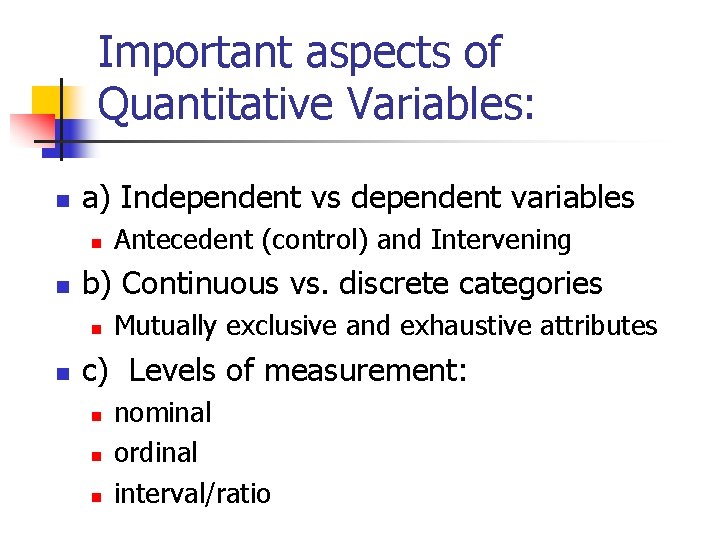
Important aspects of Quantitative Variables: n a) Independent vs dependent variables n n b) Continuous vs. discrete categories n n Antecedent (control) and Intervening Mutually exclusive and exhaustive attributes c) Levels of measurement: n nominal ordinal interval/ratio
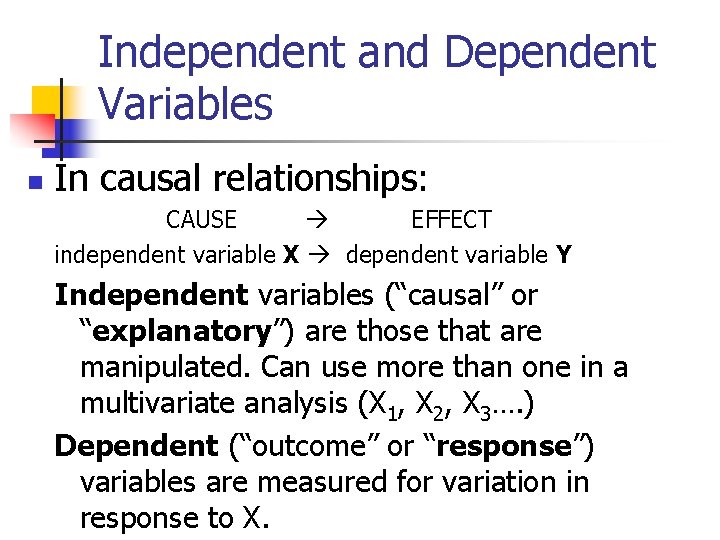
Independent and Dependent Variables n In causal relationships: CAUSE EFFECT independent variable X dependent variable Y Independent variables (“causal” or “explanatory”) are those that are manipulated. Can use more than one in a multivariate analysis (X 1, X 2, X 3…. ) Dependent (“outcome” or “response”) variables are measured for variation in response to X.
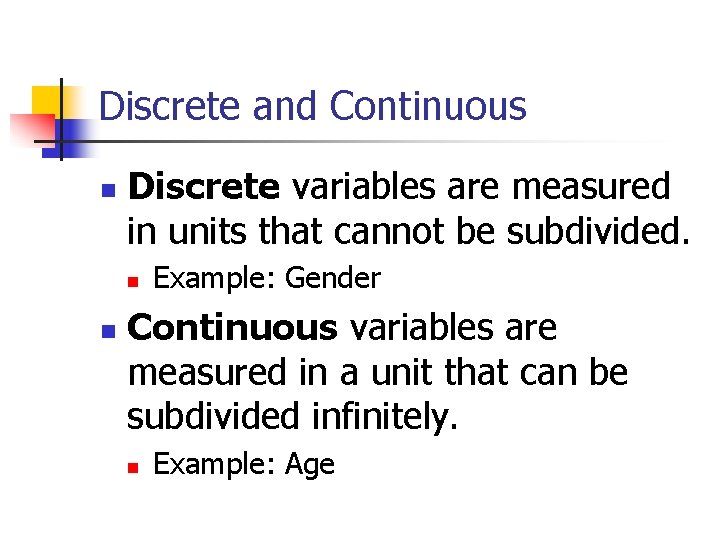
Discrete and Continuous n Discrete variables are measured in units that cannot be subdivided. n n Example: Gender Continuous variables are measured in a unit that can be subdivided infinitely. n Example: Age
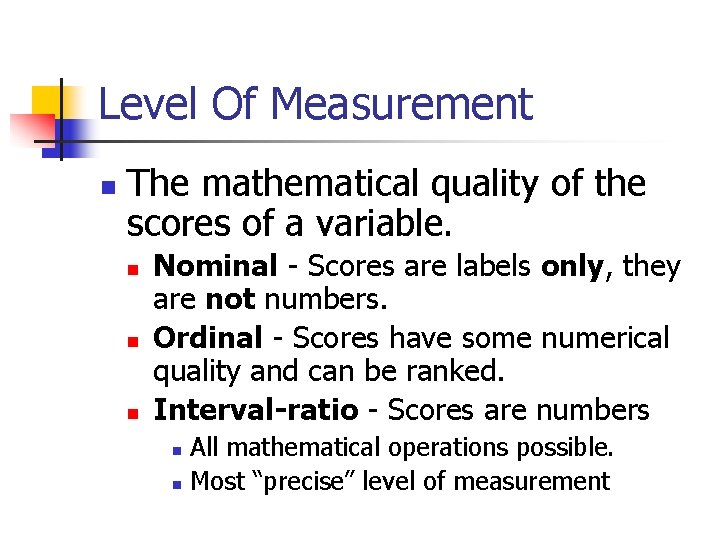
Level Of Measurement n The mathematical quality of the scores of a variable. n n n Nominal - Scores are labels only, they are not numbers. Ordinal - Scores have some numerical quality and can be ranked. Interval-ratio - Scores are numbers n n All mathematical operations possible. Most “precise” level of measurement
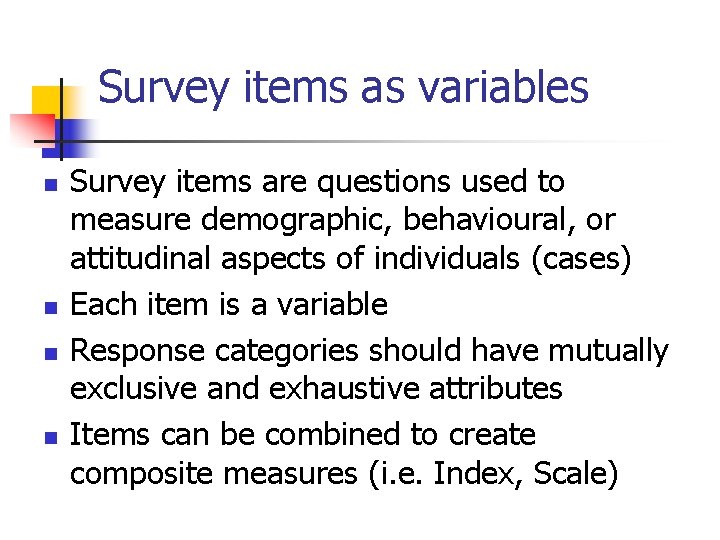
Survey items as variables n n Survey items are questions used to measure demographic, behavioural, or attitudinal aspects of individuals (cases) Each item is a variable Response categories should have mutually exclusive and exhaustive attributes Items can be combined to create composite measures (i. e. Index, Scale)
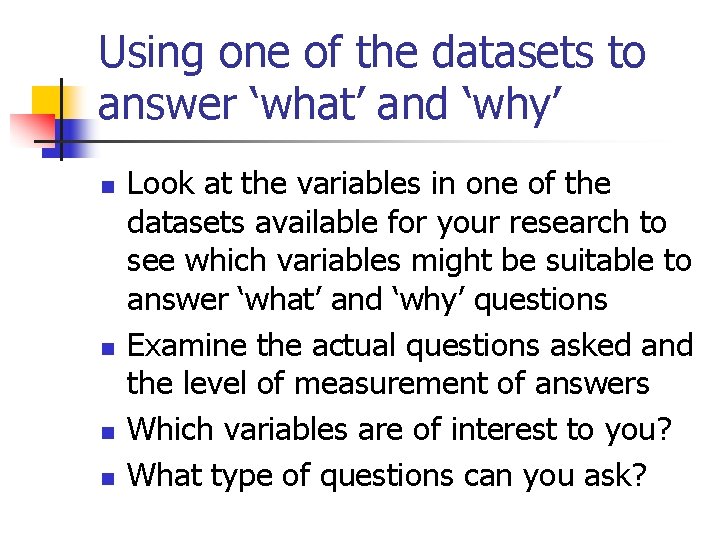
Using one of the datasets to answer ‘what’ and ‘why’ n n Look at the variables in one of the datasets available for your research to see which variables might be suitable to answer ‘what’ and ‘why’ questions Examine the actual questions asked and the level of measurement of answers Which variables are of interest to you? What type of questions can you ask?
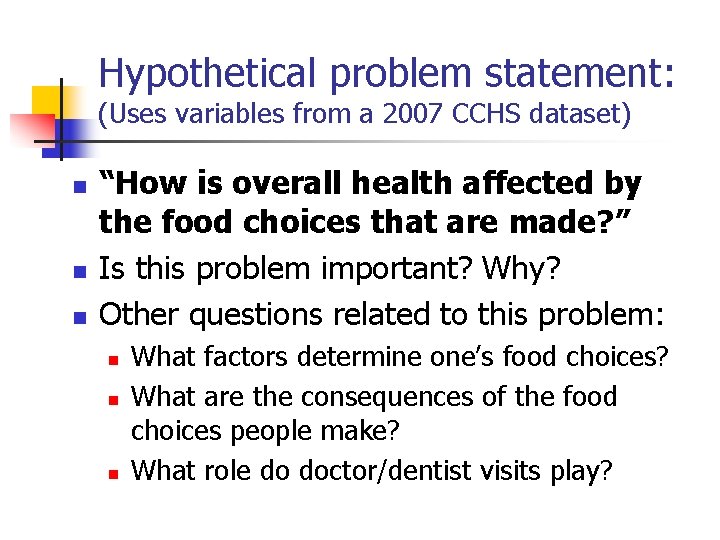
Hypothetical problem statement: (Uses variables from a 2007 CCHS dataset) n n n “How is overall health affected by the food choices that are made? ” Is this problem important? Why? Other questions related to this problem: n n n What factors determine one’s food choices? What are the consequences of the food choices people make? What role do doctor/dentist visits play?
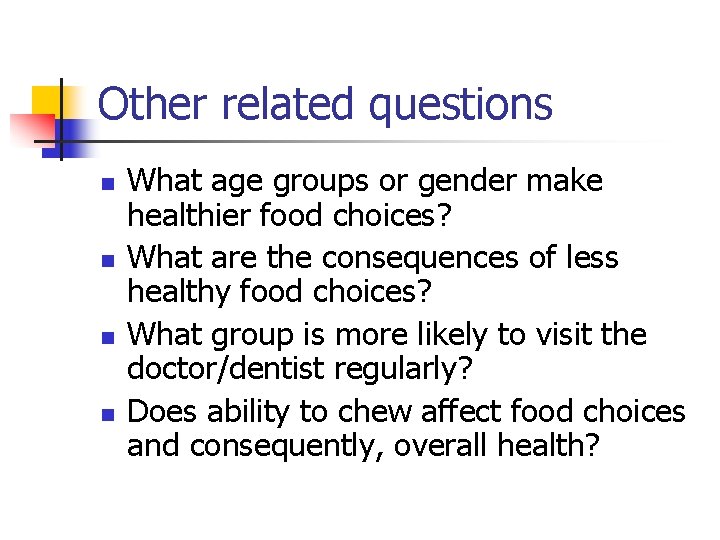
Other related questions n n What age groups or gender make healthier food choices? What are the consequences of less healthy food choices? What group is more likely to visit the doctor/dentist regularly? Does ability to chew affect food choices and consequently, overall health?
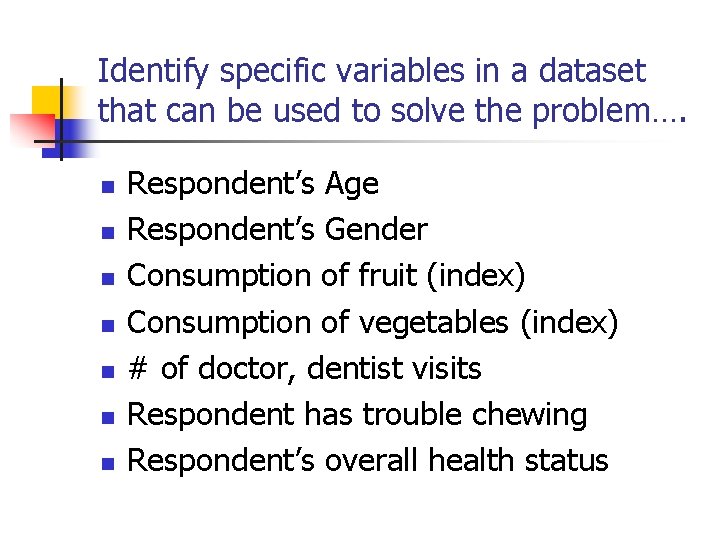
Identify specific variables in a dataset that can be used to solve the problem…. n n n n Respondent’s Age Respondent’s Gender Consumption of fruit (index) Consumption of vegetables (index) # of doctor, dentist visits Respondent has trouble chewing Respondent’s overall health status
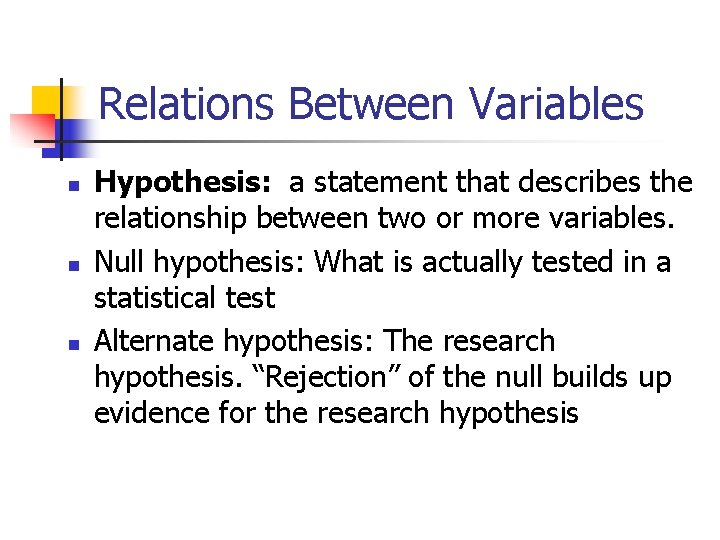
Relations Between Variables n n n Hypothesis: a statement that describes the relationship between two or more variables. Null hypothesis: What is actually tested in a statistical test Alternate hypothesis: The research hypothesis. “Rejection” of the null builds up evidence for the research hypothesis
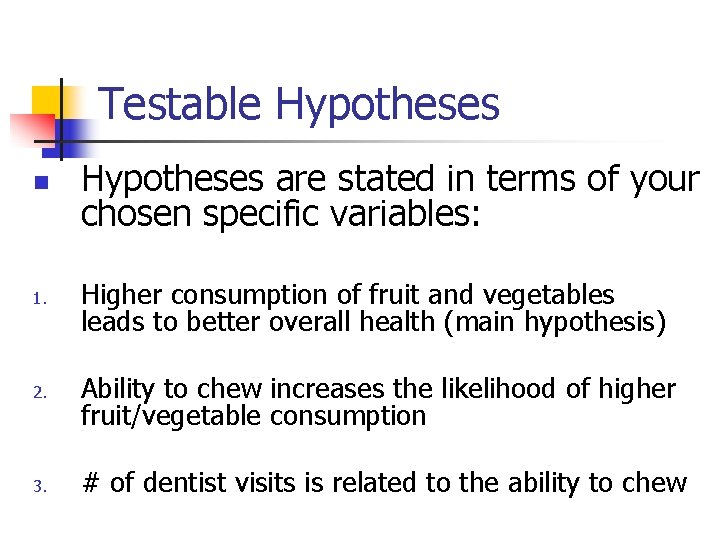
Testable Hypotheses n 1. 2. 3. Hypotheses are stated in terms of your chosen specific variables: Higher consumption of fruit and vegetables leads to better overall health (main hypothesis) Ability to chew increases the likelihood of higher fruit/vegetable consumption # of dentist visits is related to the ability to chew
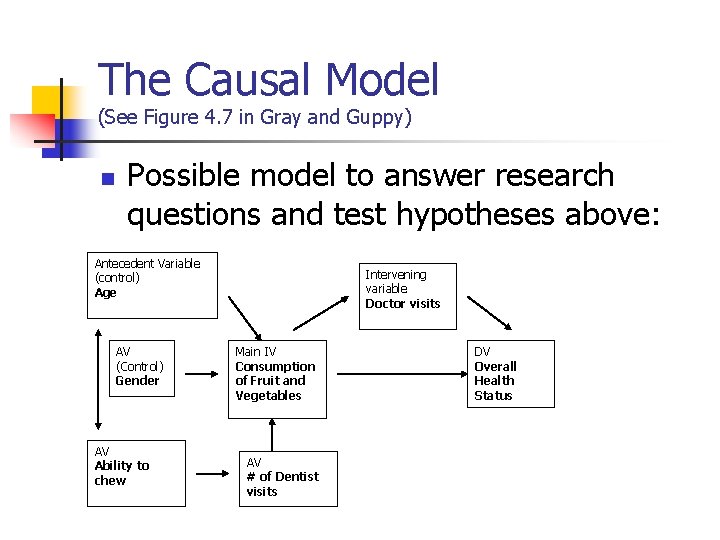
The Causal Model (See Figure 4. 7 in Gray and Guppy) n Possible model to answer research questions and test hypotheses above: Antecedent Variable (control) Age AV (Control) Gender AV Ability to chew Intervening variable Doctor visits Main IV Consumption of Fruit and Vegetables AV # of Dentist visits DV Overall Health Status
Soc2 vs soc3
01:640:244 lecture notes - lecture 15: plat, idah, farad
Global vision market research
Developing a global vision through marketing research
Developing effective research proposals
Global vision marketing definition
Developing a global vision through marketing research
Research methods notes
Https://slidetodoc.com
Research methodology lecture
Workday soc 1 report 2020
Czss zagreb
Mag paed soc
Escala soc
Siov soc
Soc 2255
Soc cissp
Nus soc ffmpeg
Intelligence driven defense
Soc charter template
Raspberry pi gpio4
Dss-soc
Uci ics catalogue
60s greaser girl
để nguyên nước chấm cổ truyền