Simulation of Tightly Coupled INSGPS Navigator Ade Mulyana
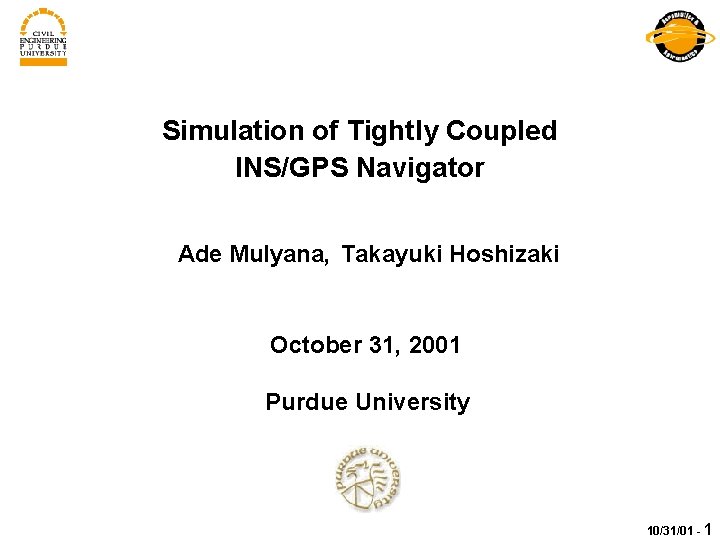
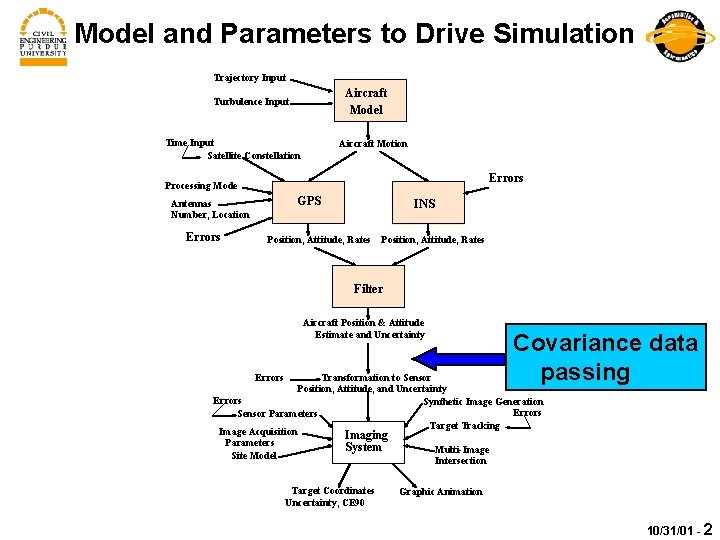
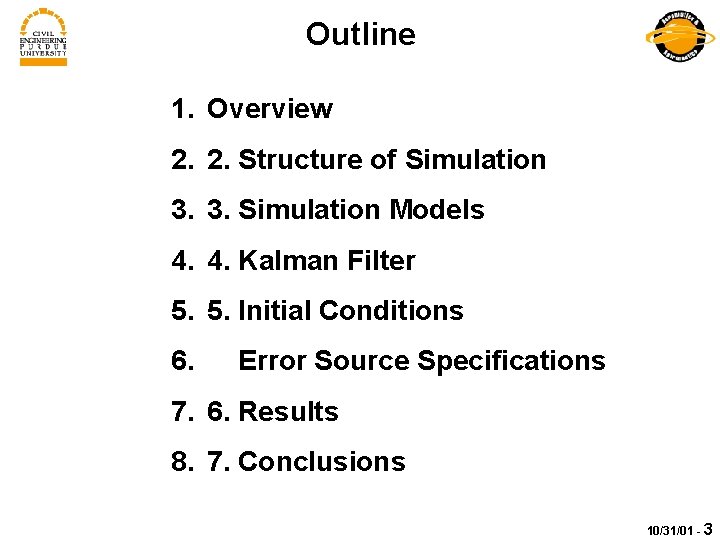
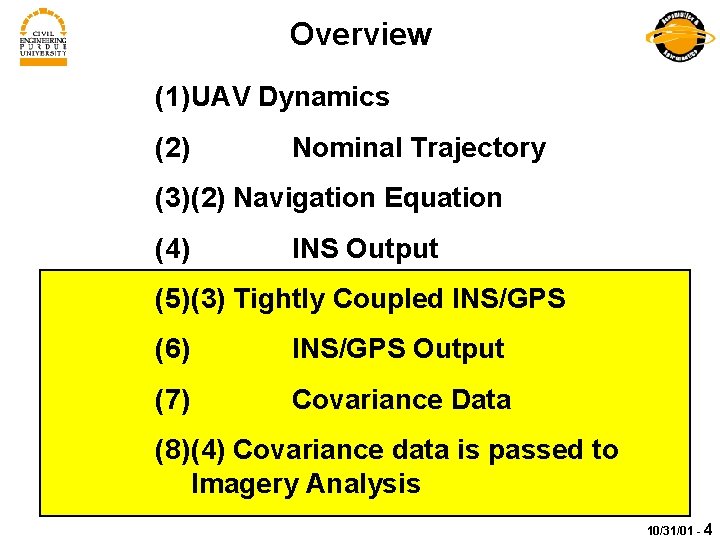
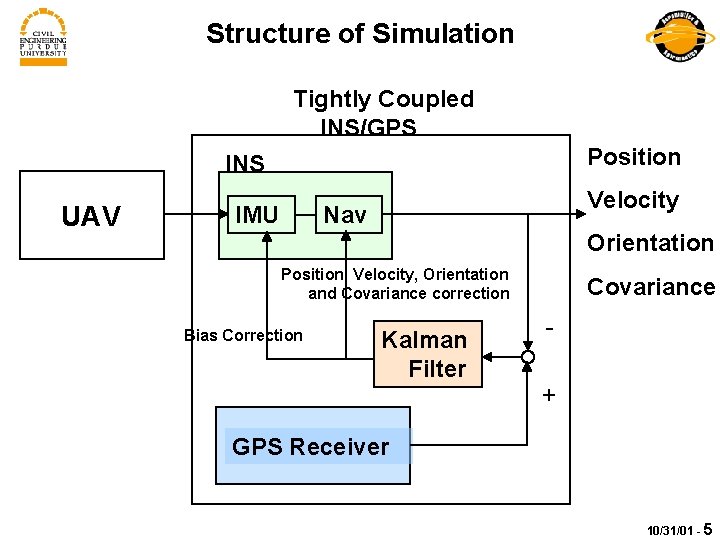
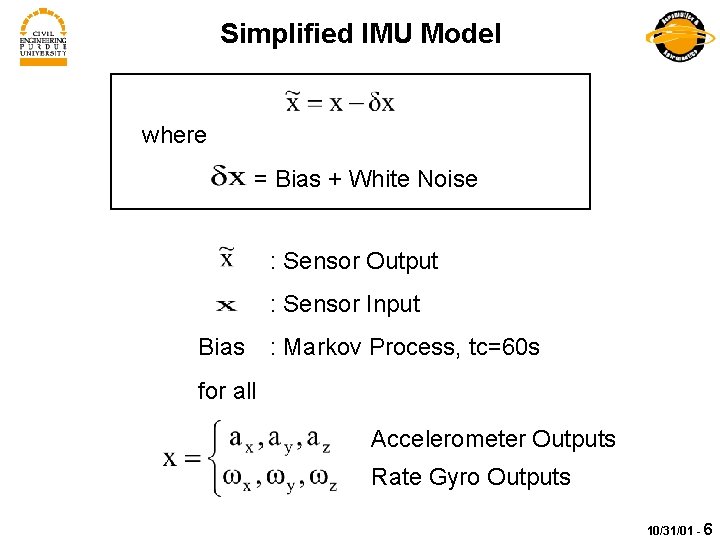
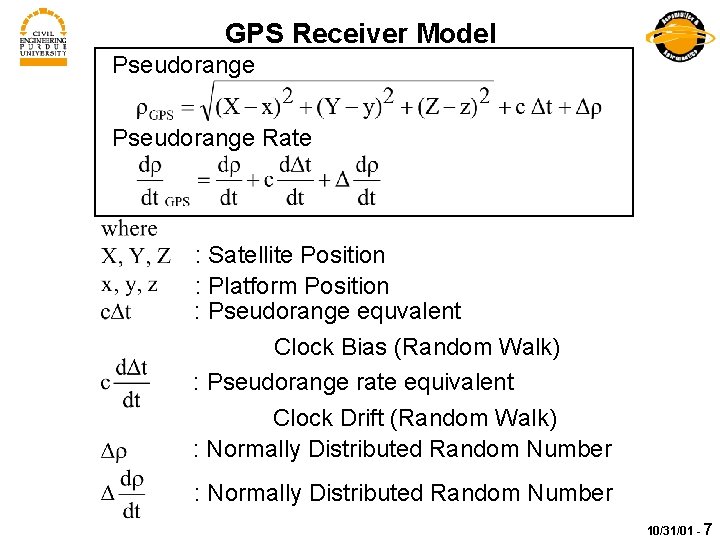
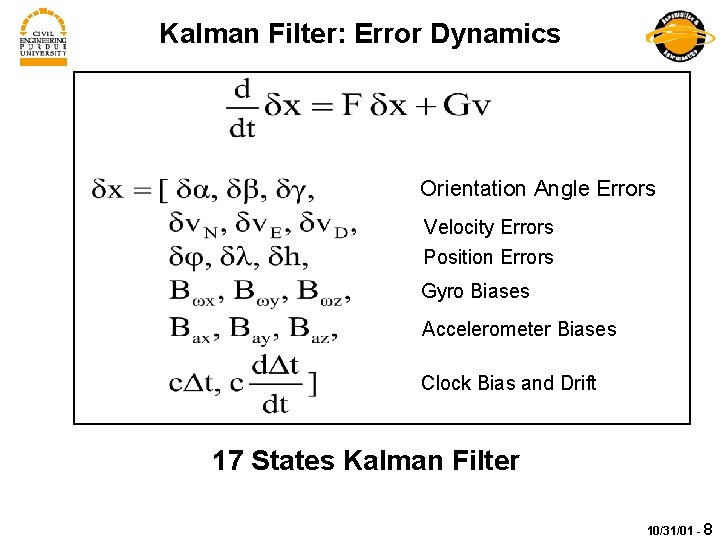
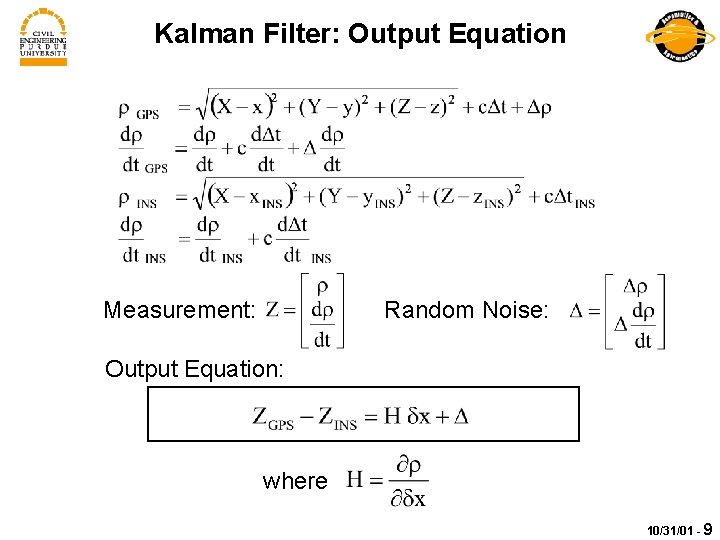
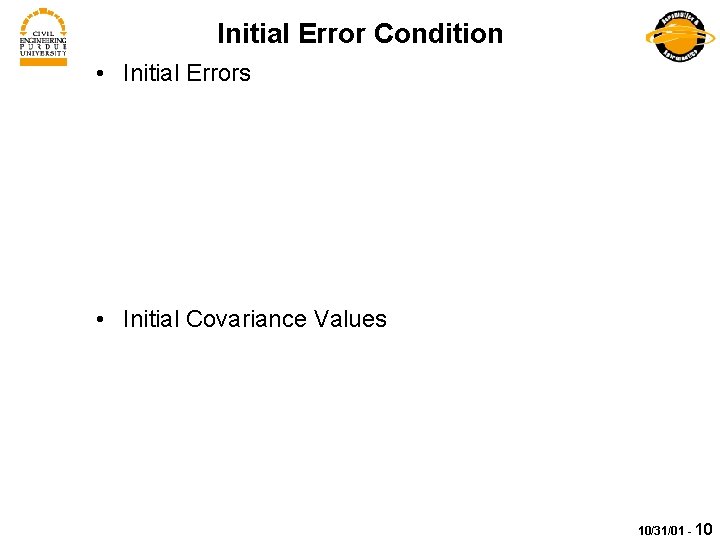
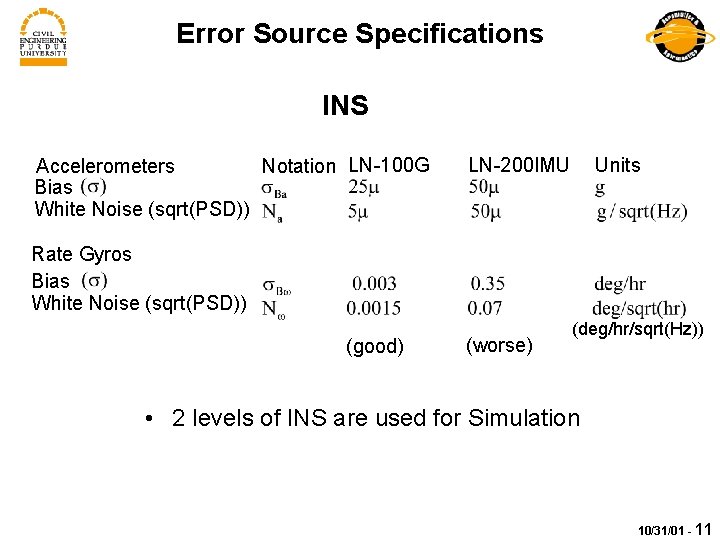
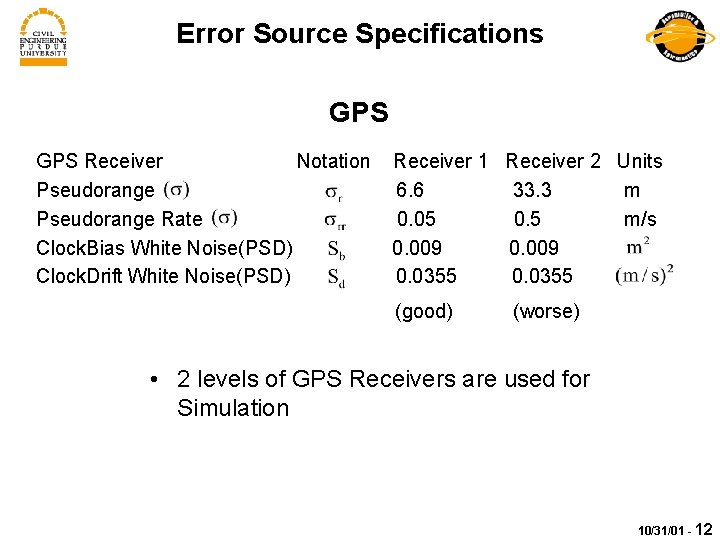
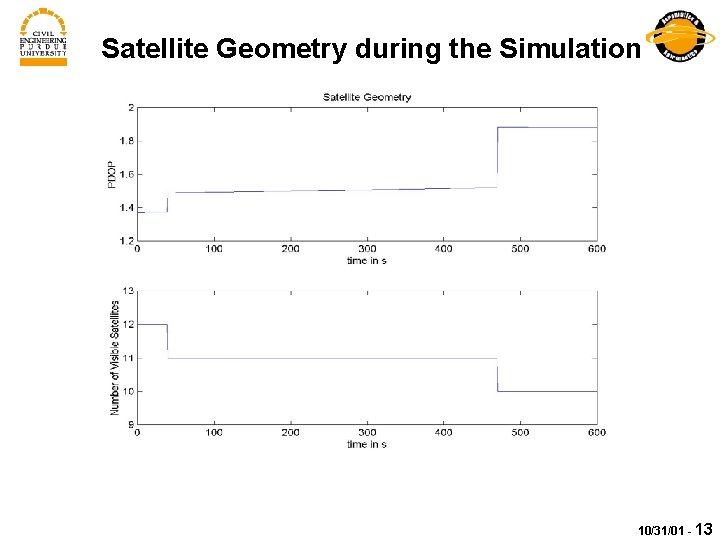
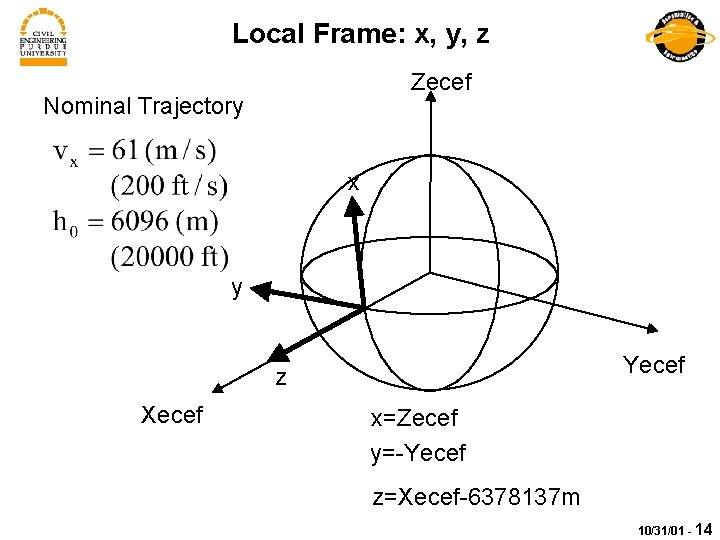
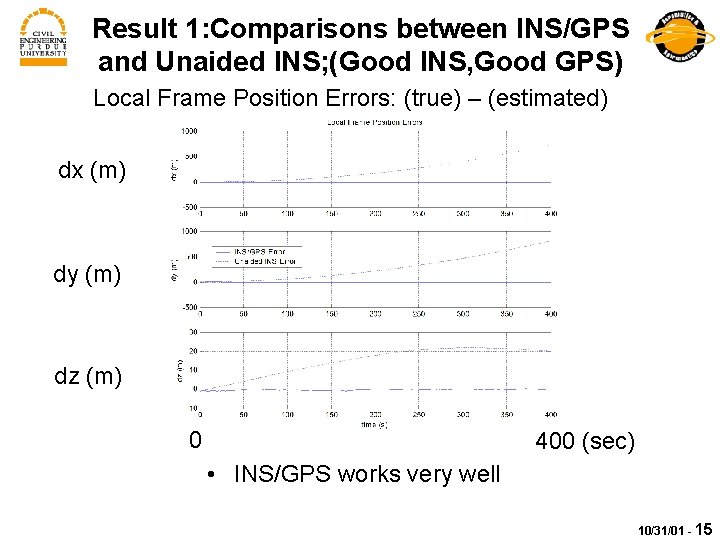
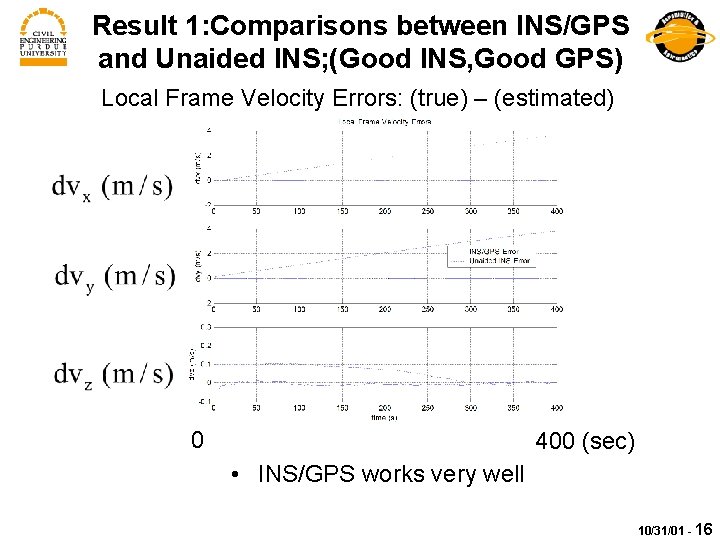
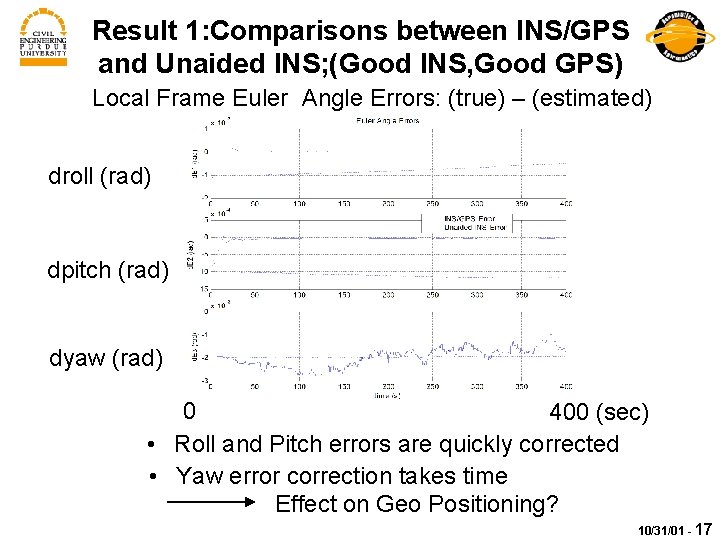
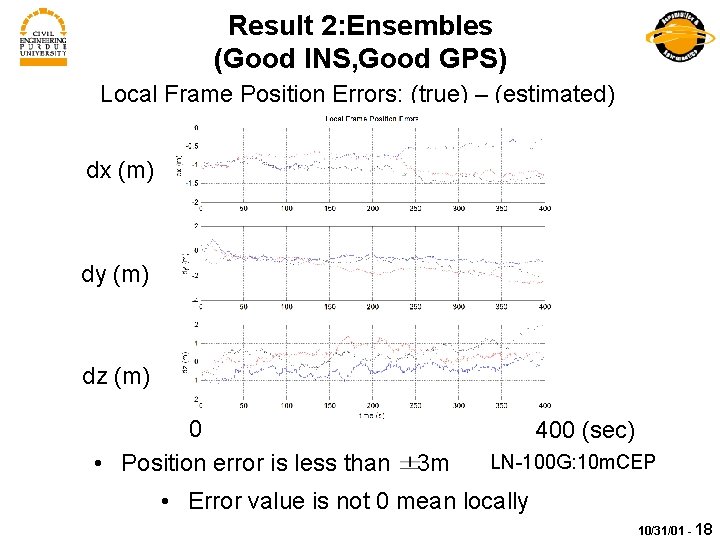
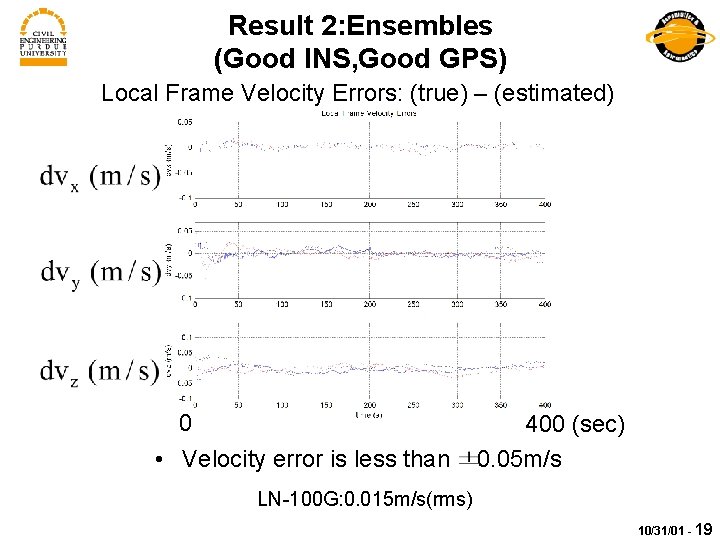
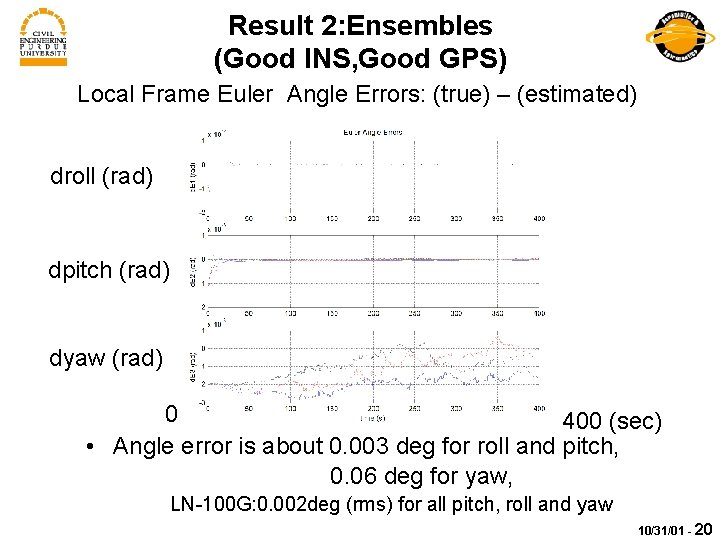
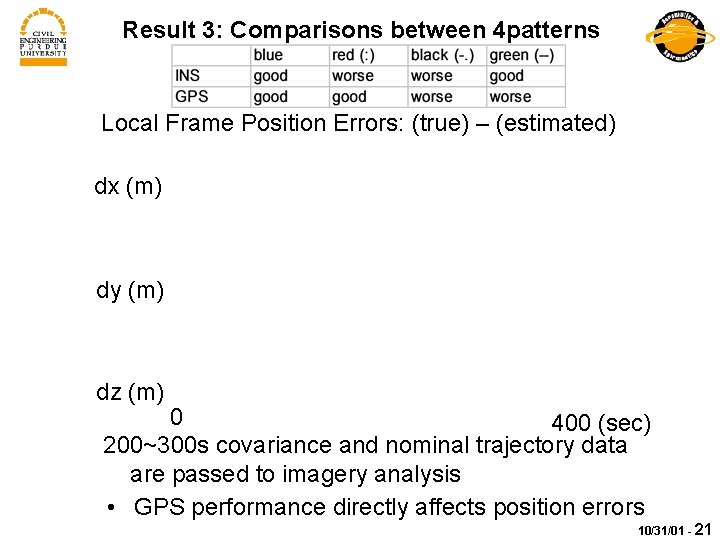
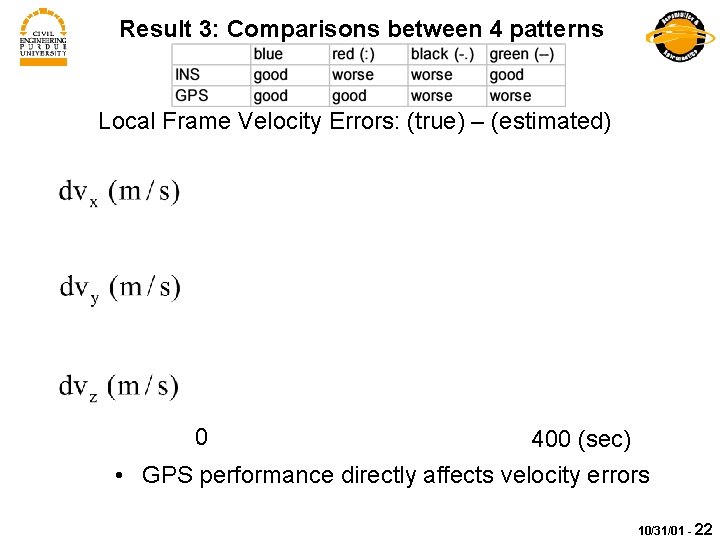
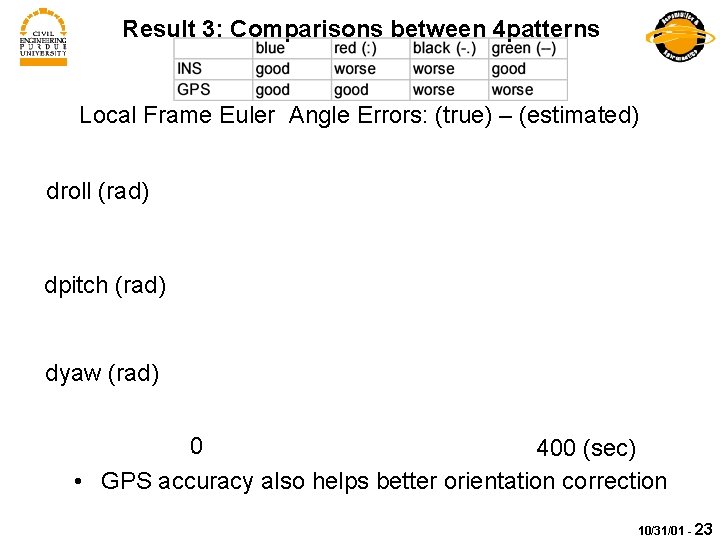
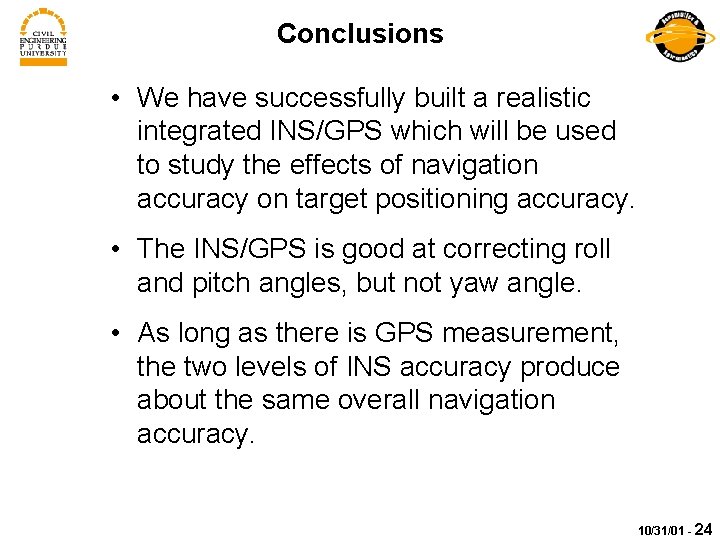
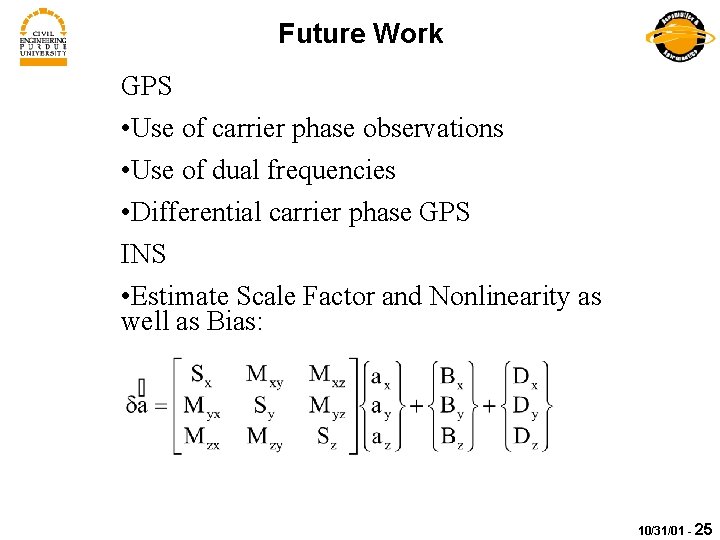
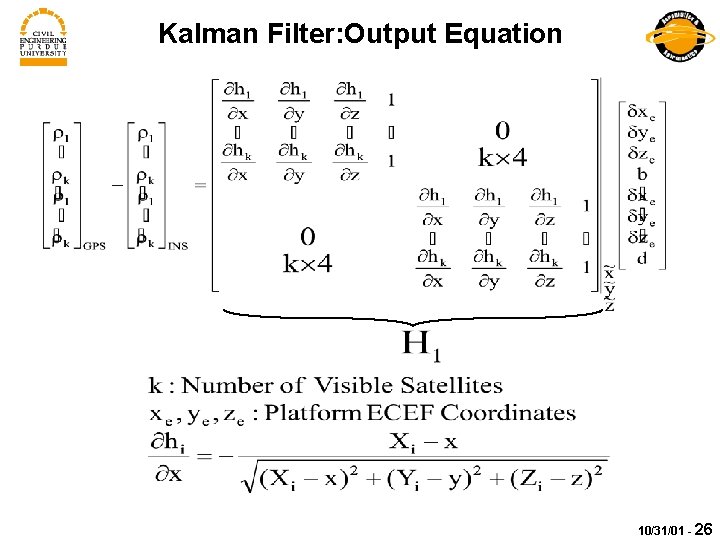
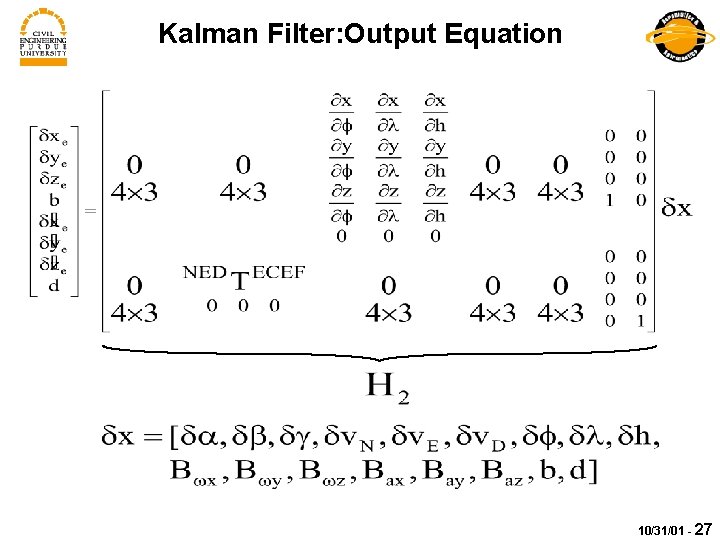
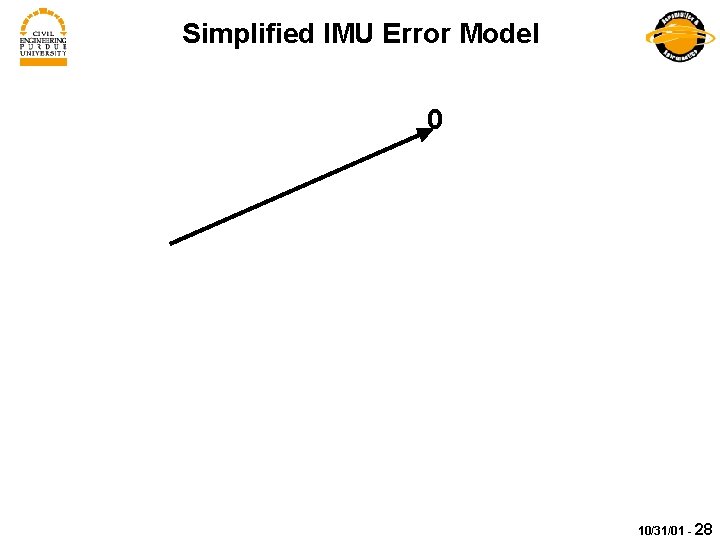
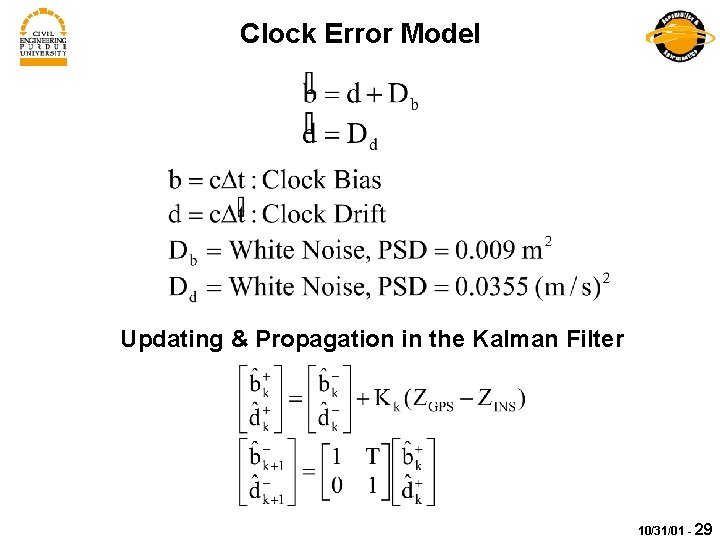
- Slides: 29
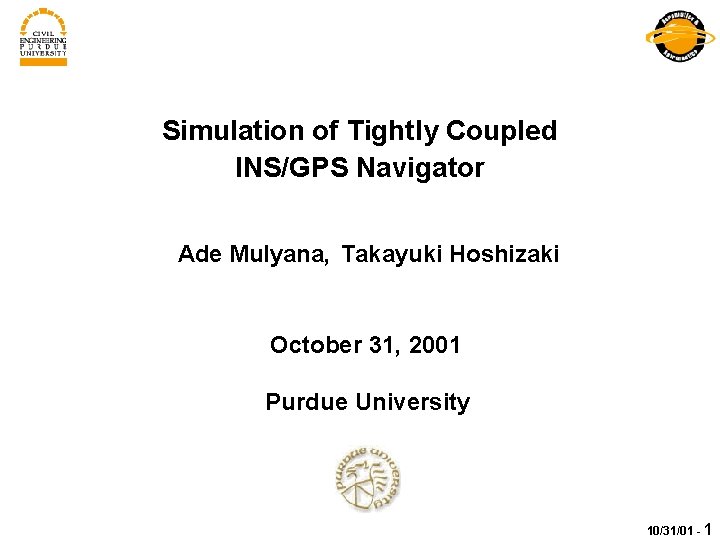
Simulation of Tightly Coupled INS/GPS Navigator Ade Mulyana, Takayuki Hoshizaki October 31, 2001 Purdue University 10/31/01 - 1
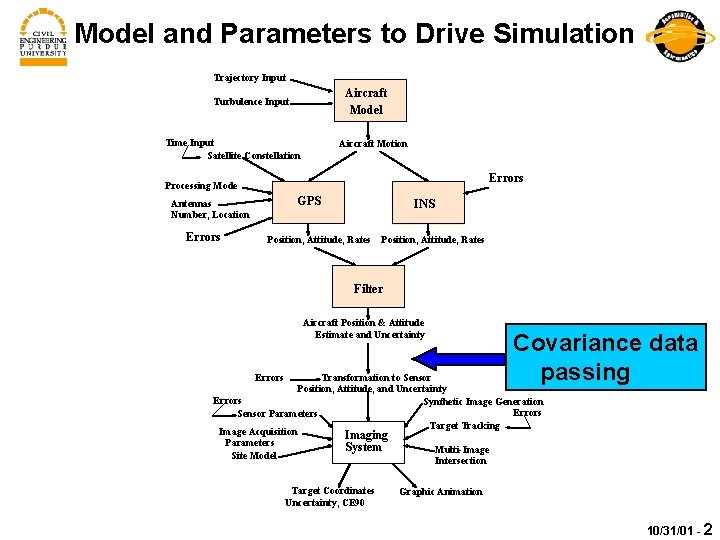
Model and Parameters to Drive Simulation Trajectory Input Aircraft Model Turbulence Input Time Input Satellite Constellation Aircraft Motion Errors Processing Mode GPS Antennas Number, Location Errors INS Position, Attitude, Rates Filter Aircraft Position & Attitude Estimate and Uncertainty Errors Covariance data passing Transformation to Sensor Position, Attitude, and Uncertainty Errors Synthetic Image Generation Errors Sensor Parameters Target Tracking Image Acquisition Imaging Parameters System Multi-Image Site Model Intersection Target Coordinates Uncertainty, CE 90 Graphic Animation 10/31/01 - 2
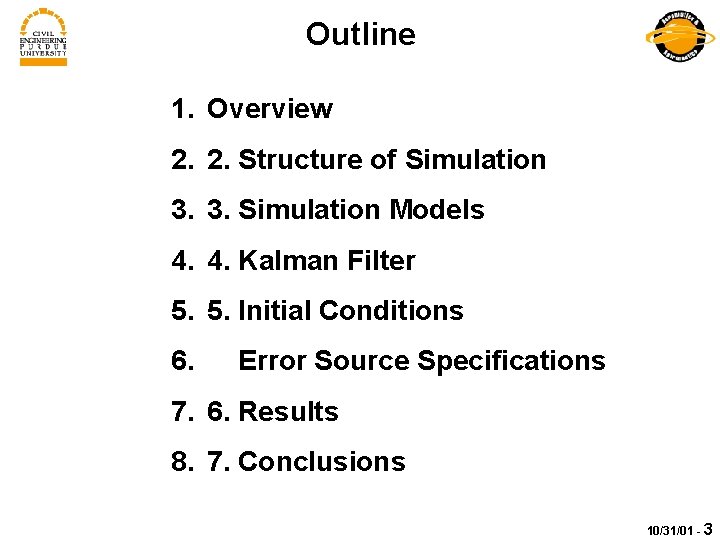
Outline 1. Overview 2. 2. Structure of Simulation 3. 3. Simulation Models 4. 4. Kalman Filter 5. 5. Initial Conditions 6. Error Source Specifications 7. 6. Results 8. 7. Conclusions 10/31/01 - 3
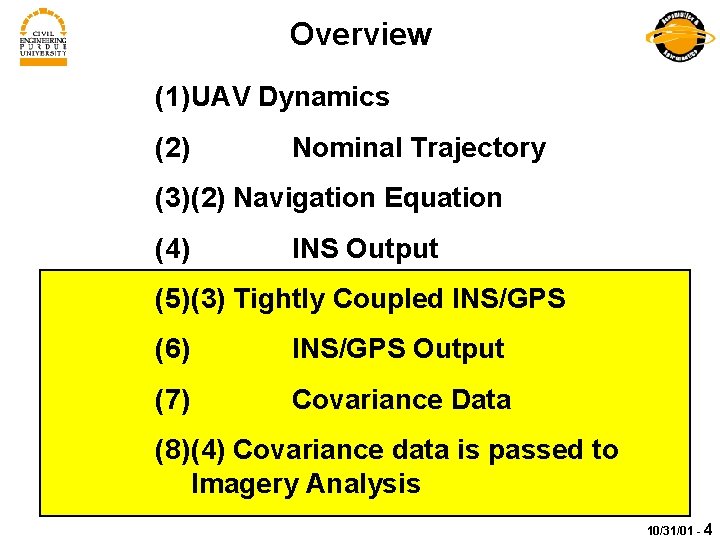
Overview (1) UAV Dynamics (2) Nominal Trajectory (3) (2) Navigation Equation (4) INS Output (5) (3) Tightly Coupled INS/GPS (6) INS/GPS Output (7) Covariance Data (8) (4) Covariance data is passed to Imagery Analysis 10/31/01 - 4
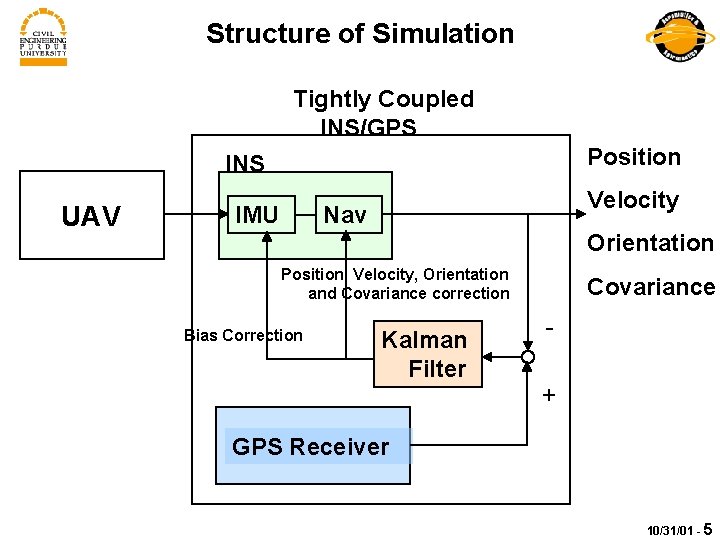
Structure of Simulation Tightly Coupled INS/GPS Position INS UAV IMU Velocity Nav Orientation Position, Velocity, Orientation and Covariance correction Bias Correction Kalman Filter Covariance + GPS Receiver 10/31/01 - 5
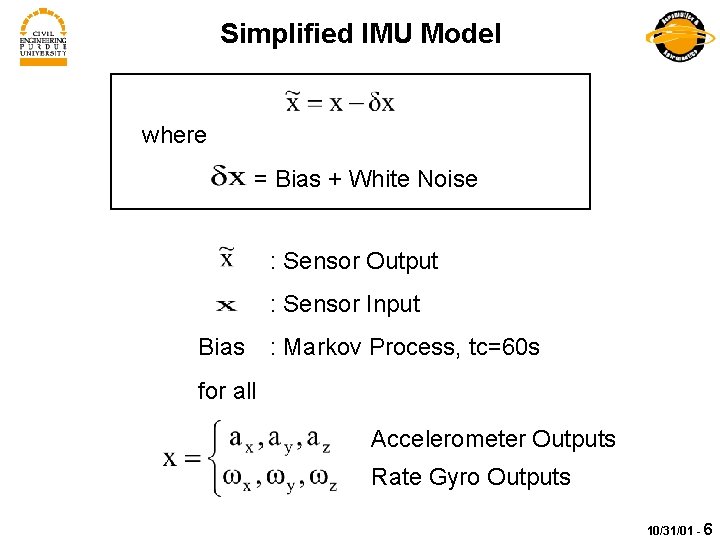
Simplified IMU Model where = Bias + White Noise : Sensor Output : Sensor Input Bias : Markov Process, tc=60 s for all Accelerometer Outputs Rate Gyro Outputs 10/31/01 - 6
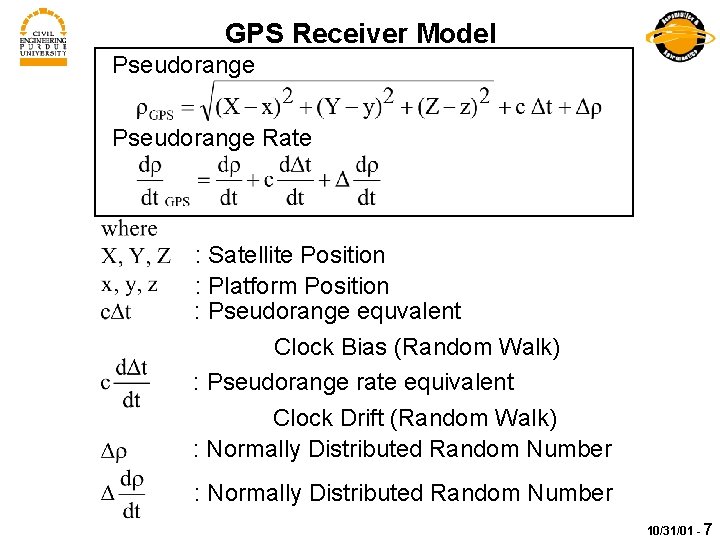
GPS Receiver Model Pseudorange Rate : Satellite Position : Platform Position : Pseudorange equvalent Clock Bias (Random Walk) : Pseudorange rate equivalent Clock Drift (Random Walk) : Normally Distributed Random Number 10/31/01 - 7
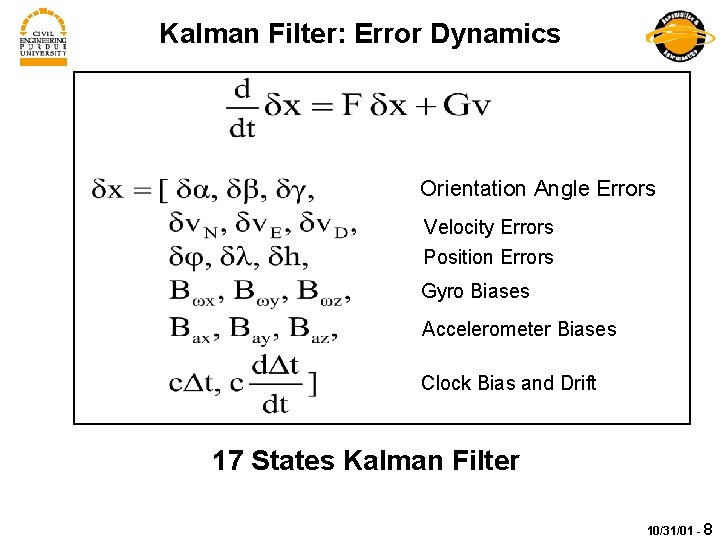
Kalman Filter: Error Dynamics Orientation Angle Errors Velocity Errors Position Errors Gyro Biases Accelerometer Biases Clock Bias and Drift 17 States Kalman Filter 10/31/01 - 8
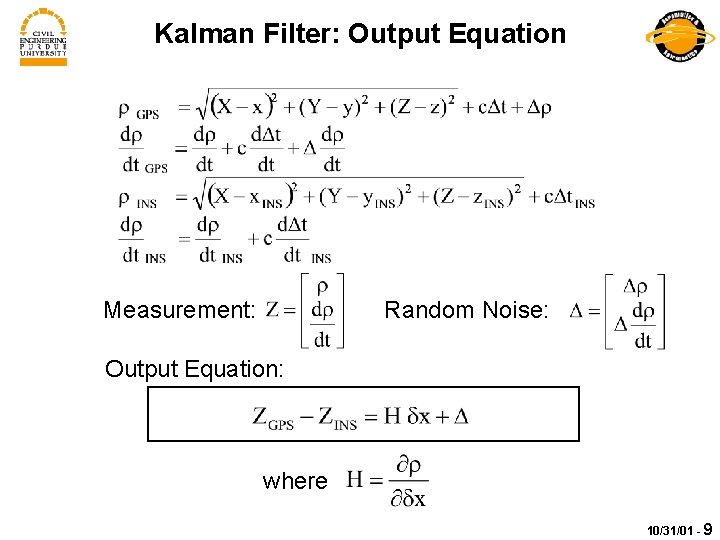
Kalman Filter: Output Equation Measurement: Random Noise: Output Equation: where 10/31/01 - 9
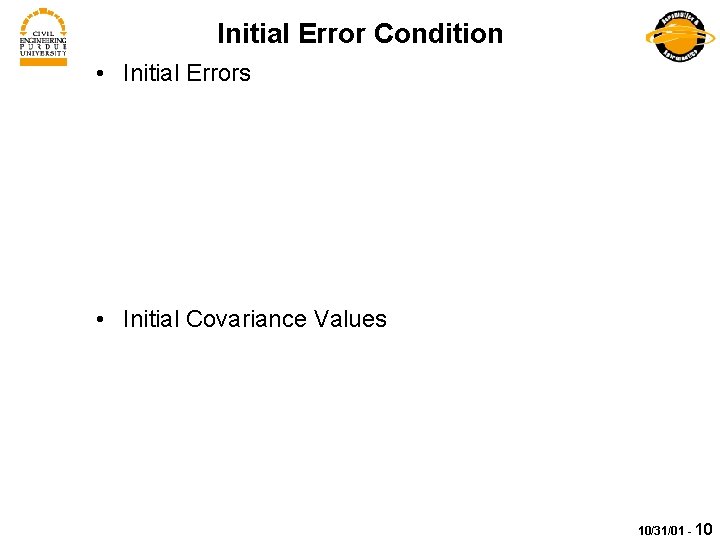
Initial Error Condition • Initial Errors • Initial Covariance Values 10/31/01 - 10
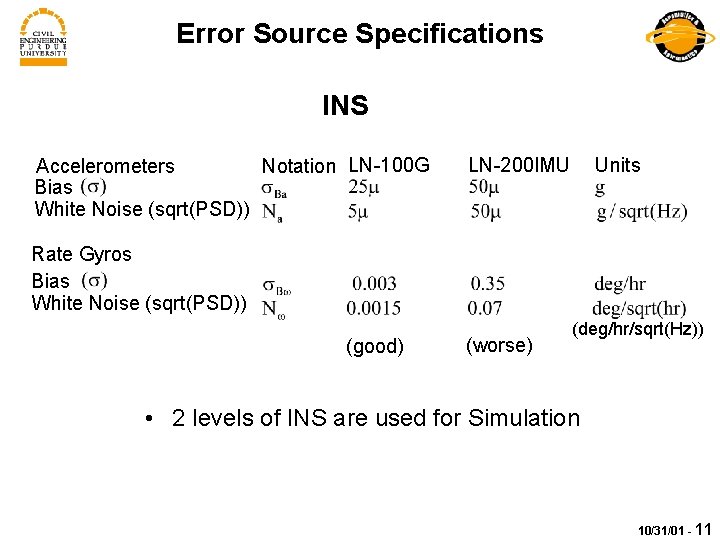
Error Source Specifications INS Accelerometers Notation LN-100 G Bias White Noise (sqrt(PSD)) LN-200 IMU Units Rate Gyros Bias White Noise (sqrt(PSD)) (good) (worse) (deg/hr/sqrt(Hz)) • 2 levels of INS are used for Simulation 10/31/01 - 11
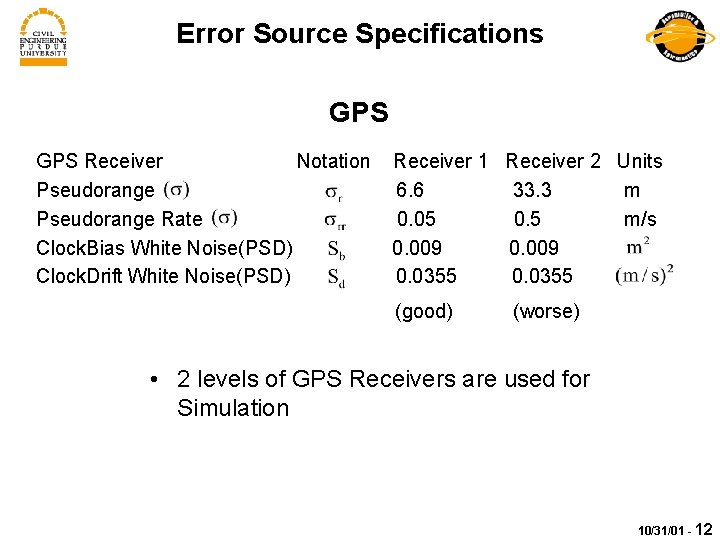
Error Source Specifications GPS Receiver Notation Pseudorange Rate Clock. Bias White Noise(PSD) Clock. Drift White Noise(PSD) Receiver 1 Receiver 2 Units 6. 6 33. 3 m 0. 05 0. 5 m/s 0. 009 0. 0355 (good) (worse) • 2 levels of GPS Receivers are used for Simulation 10/31/01 - 12
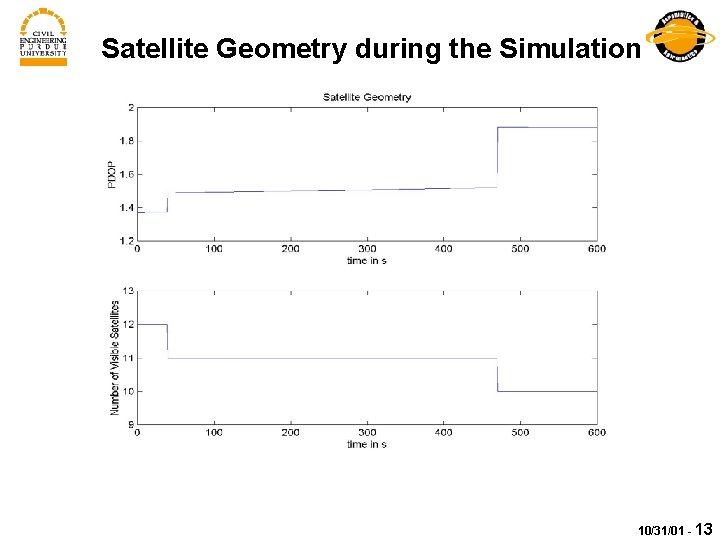
Satellite Geometry during the Simulation 10/31/01 - 13
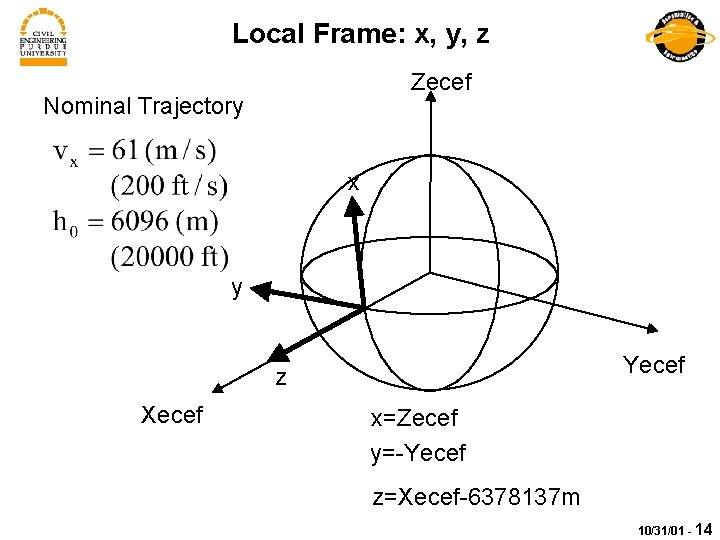
Local Frame: x, y, z Zecef Nominal Trajectory x y Yecef z Xecef x=Zecef y=-Yecef z=Xecef-6378137 m 10/31/01 - 14
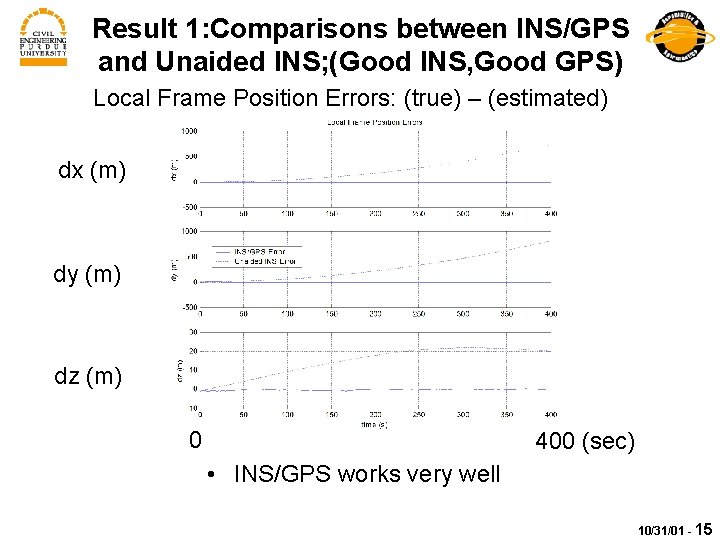
Result 1: Comparisons between INS/GPS and Unaided INS; (Good INS, Good GPS) Local Frame Position Errors: (true) – (estimated) dx (m) dy (m) dz (m) 0 400 (sec) • INS/GPS works very well 10/31/01 - 15
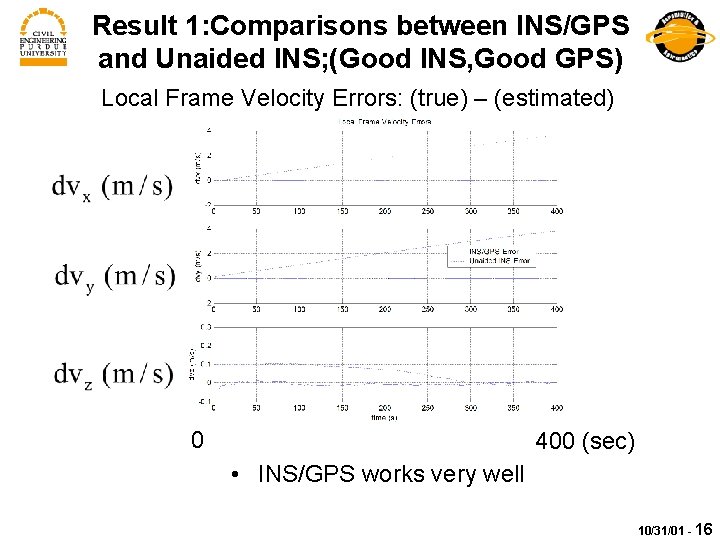
Result 1: Comparisons between INS/GPS and Unaided INS; (Good INS, Good GPS) Local Frame Velocity Errors: (true) – (estimated) 0 400 (sec) • INS/GPS works very well 10/31/01 - 16
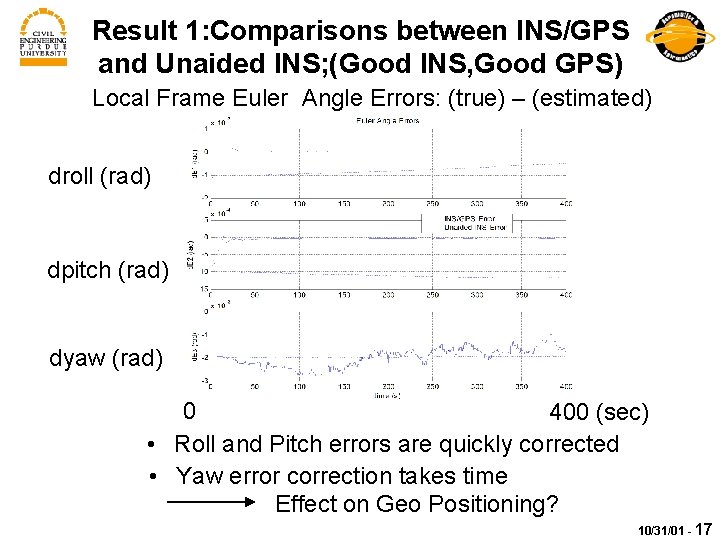
Result 1: Comparisons between INS/GPS and Unaided INS; (Good INS, Good GPS) Local Frame Euler Angle Errors: (true) – (estimated) droll (rad) dpitch (rad) dyaw (rad) 0 400 (sec) • Roll and Pitch errors are quickly corrected • Yaw error correction takes time Effect on Geo Positioning? 10/31/01 - 17
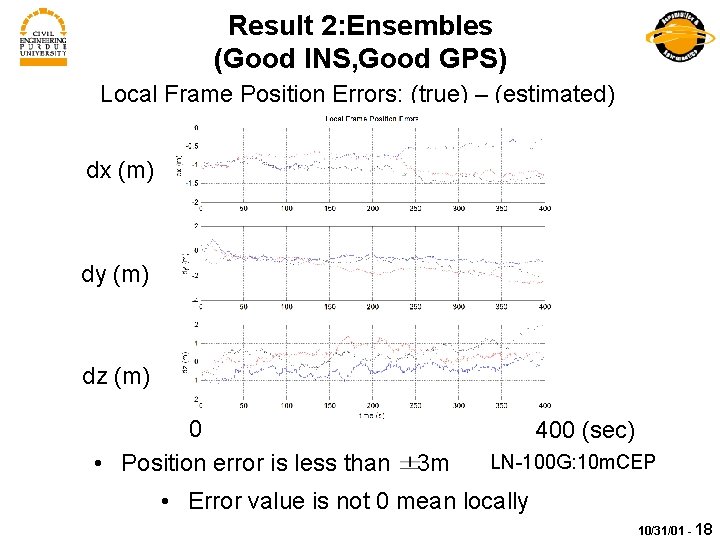
Result 2: Ensembles (Good INS, Good GPS) Local Frame Position Errors: (true) – (estimated) dx (m) dy (m) dz (m) 0 • Position error is less than 400 (sec) 3 m LN-100 G: 10 m. CEP • Error value is not 0 mean locally 10/31/01 - 18
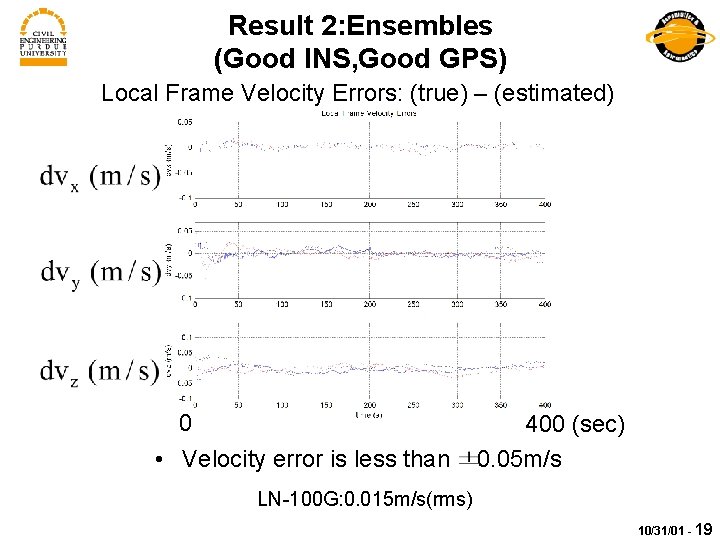
Result 2: Ensembles (Good INS, Good GPS) Local Frame Velocity Errors: (true) – (estimated) 0 • Velocity error is less than 400 (sec) 0. 05 m/s LN-100 G: 0. 015 m/s(rms) 10/31/01 - 19
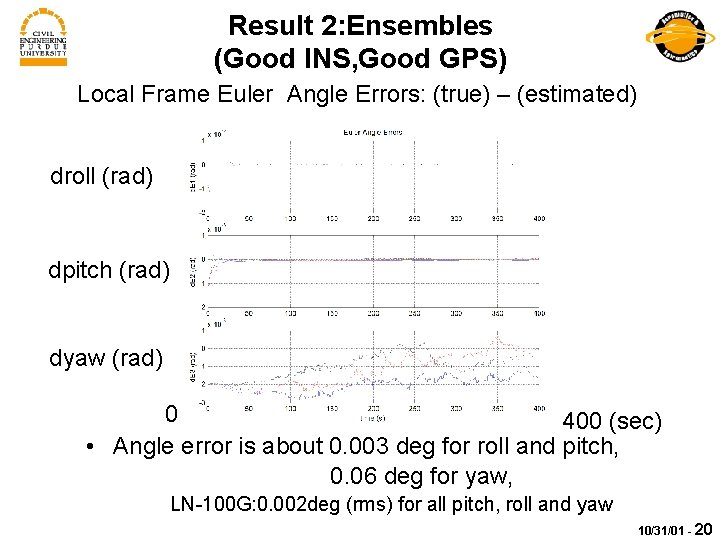
Result 2: Ensembles (Good INS, Good GPS) Local Frame Euler Angle Errors: (true) – (estimated) droll (rad) dpitch (rad) dyaw (rad) 0 400 (sec) • Angle error is about 0. 003 deg for roll and pitch, 0. 06 deg for yaw, LN-100 G: 0. 002 deg (rms) for all pitch, roll and yaw 10/31/01 - 20
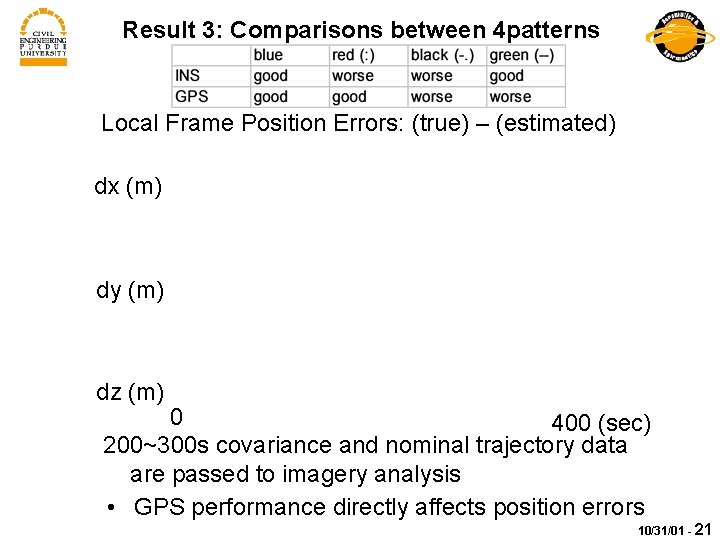
Result 3: Comparisons between 4 patterns Local Frame Position Errors: (true) – (estimated) dx (m) dy (m) dz (m) 0 400 (sec) 200~300 s covariance and nominal trajectory data are passed to imagery analysis • GPS performance directly affects position errors 10/31/01 - 21
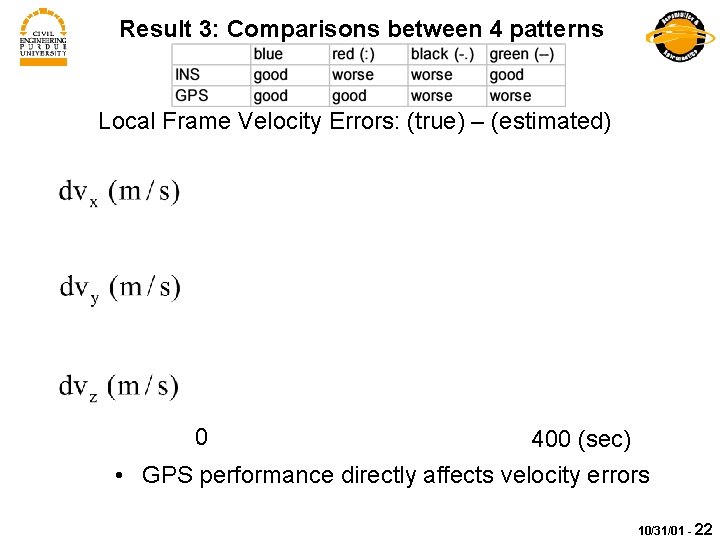
Result 3: Comparisons between 4 patterns Local Frame Velocity Errors: (true) – (estimated) 0 400 (sec) • GPS performance directly affects velocity errors 10/31/01 - 22
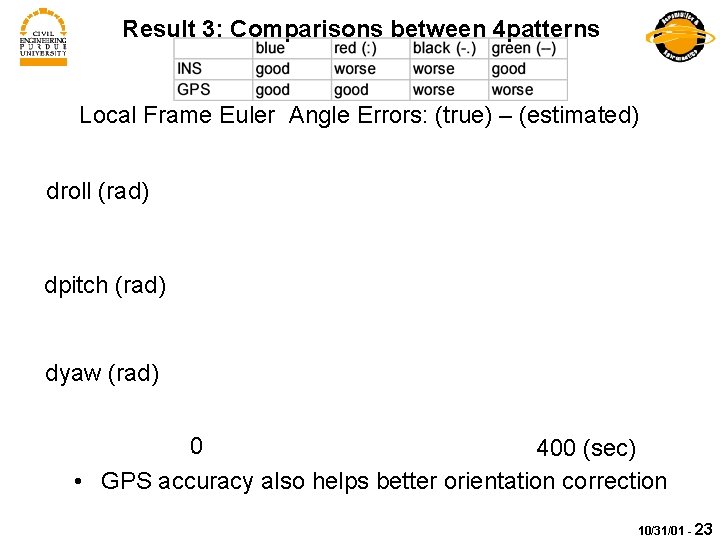
Result 3: Comparisons between 4 patterns Local Frame Euler Angle Errors: (true) – (estimated) droll (rad) dpitch (rad) dyaw (rad) 0 400 (sec) • GPS accuracy also helps better orientation correction 10/31/01 - 23
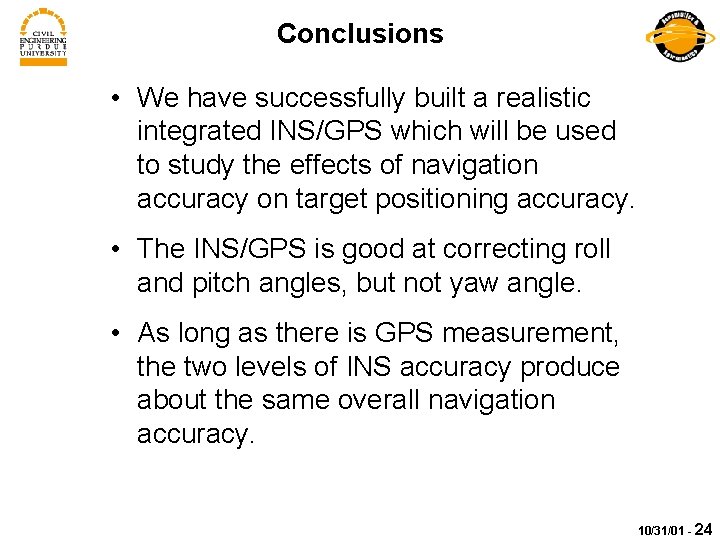
Conclusions • We have successfully built a realistic integrated INS/GPS which will be used to study the effects of navigation accuracy on target positioning accuracy. • The INS/GPS is good at correcting roll and pitch angles, but not yaw angle. • As long as there is GPS measurement, the two levels of INS accuracy produce about the same overall navigation accuracy. 10/31/01 - 24
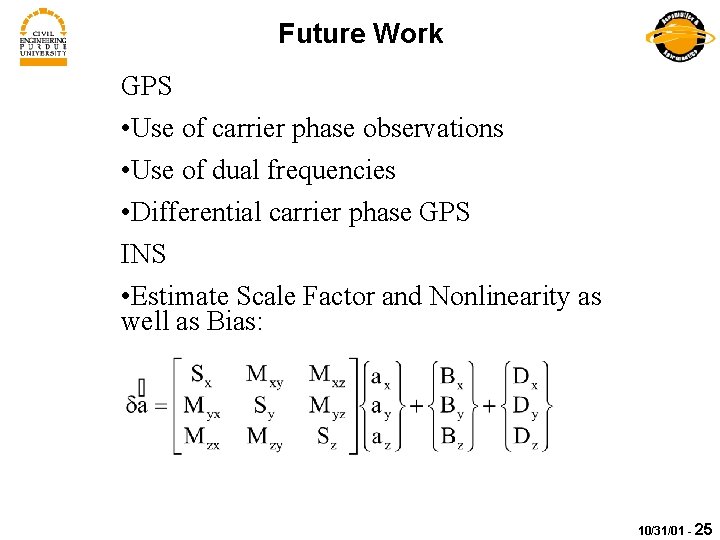
Future Work GPS • Use of carrier phase observations • Use of dual frequencies • Differential carrier phase GPS INS • Estimate Scale Factor and Nonlinearity as well as Bias: 10/31/01 - 25
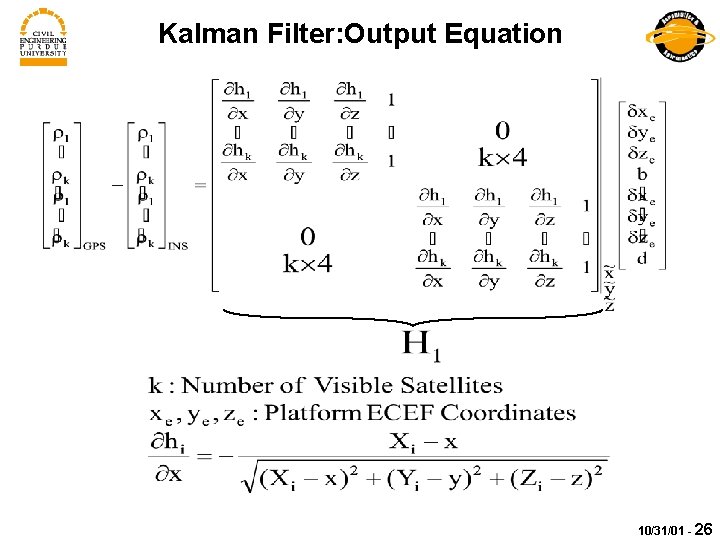
Kalman Filter: Output Equation 10/31/01 - 26
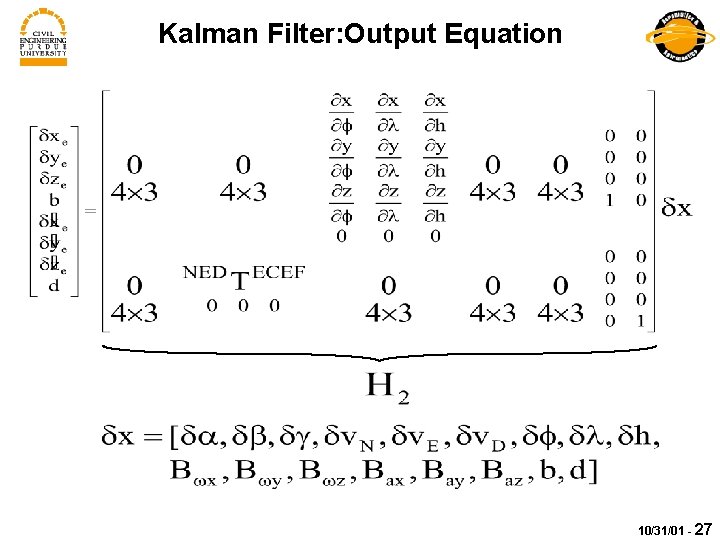
Kalman Filter: Output Equation 10/31/01 - 27
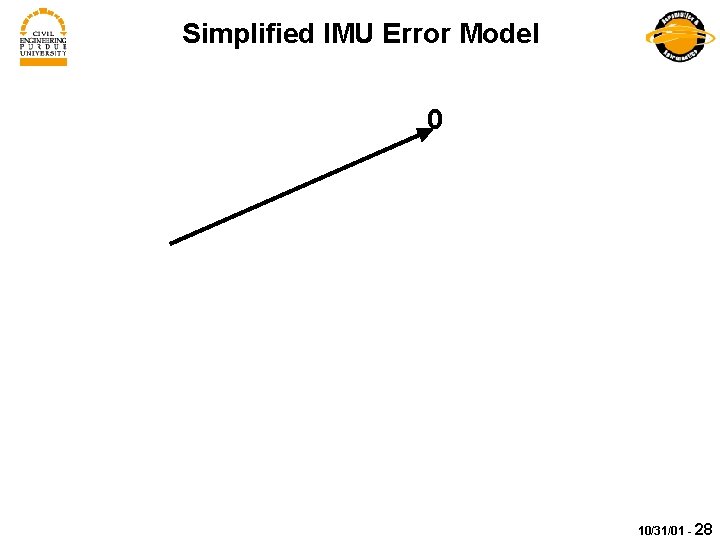
Simplified IMU Error Model 0 10/31/01 - 28
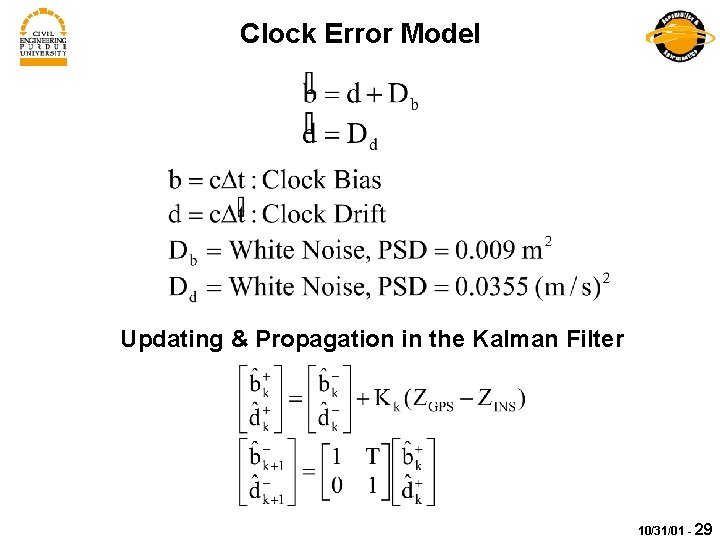
Clock Error Model Updating & Propagation in the Kalman Filter 10/31/01 - 29
Distributed system models in cloud computing
Tightly coupled multiprocessor
All resources are tightly coupled in computing paradigm of
Asep mulyana unpad
Apache mxnet operating system
A belt fits tightly around two pulleys
Philip larkin born yesterday
Unit 10 homework 6 tangent lines
Chapter 11 circles
What is reproductive system
Tightly packed in a disorderly manner
How to write chromosome notation
Tightly attached ceiling
Interstitial ceilings
Cadence deepprobe
Horarios arquitectura upv
Gade uca
Denis bureau esiee
Trend dan issue keperawatan jiwa
Ade heryana
Video procedure text
Ade gafar abdullah
"ade tech" and "support"
Architectural model in distributed system
Ade valdivia
Gestio academica economia uab
Heryana
Ade needs assessment
Ade oelas
Ade oelas