PSY 369 Psycholinguistics Language Comprehension Sentence comprehension n
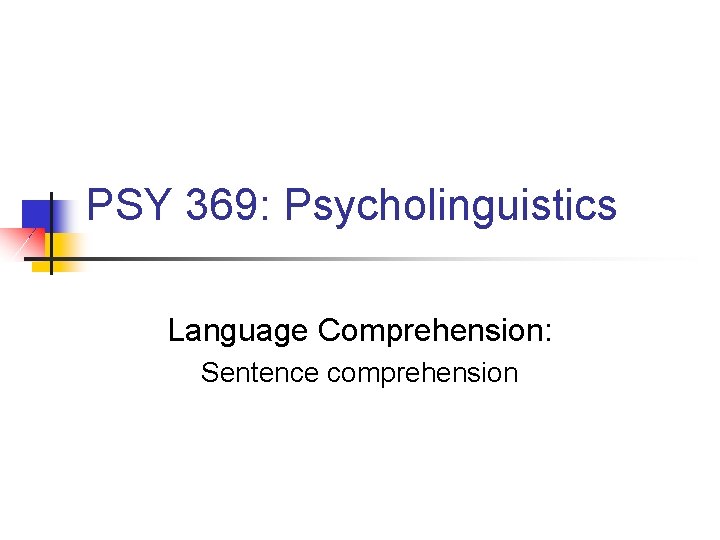
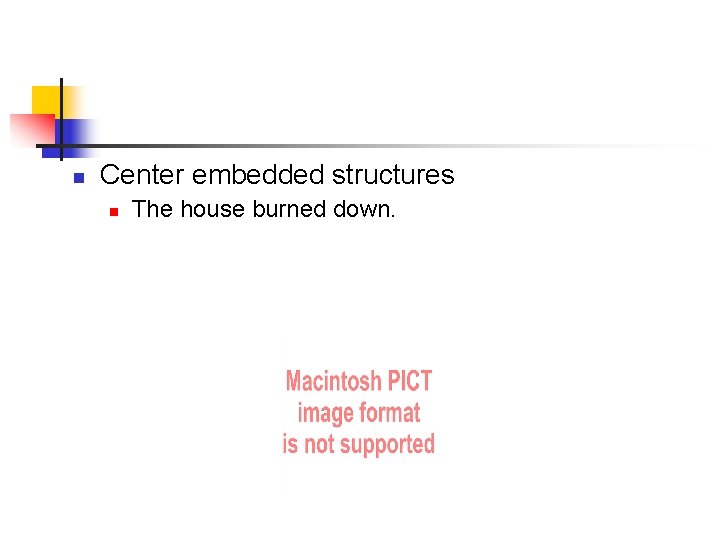
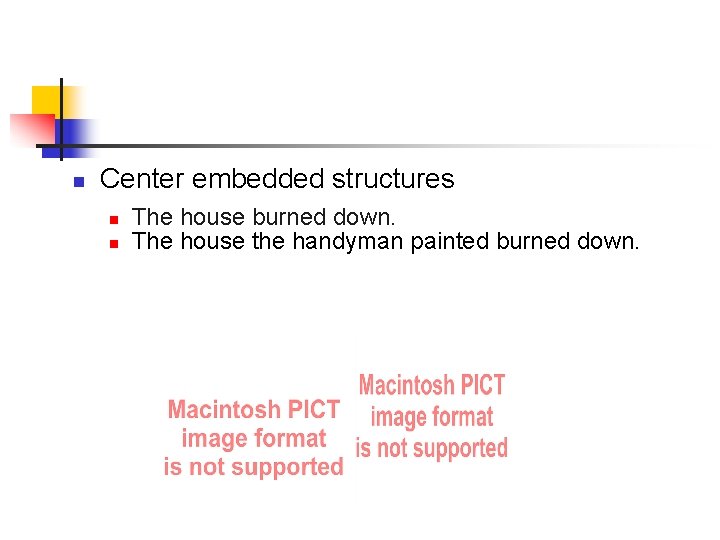
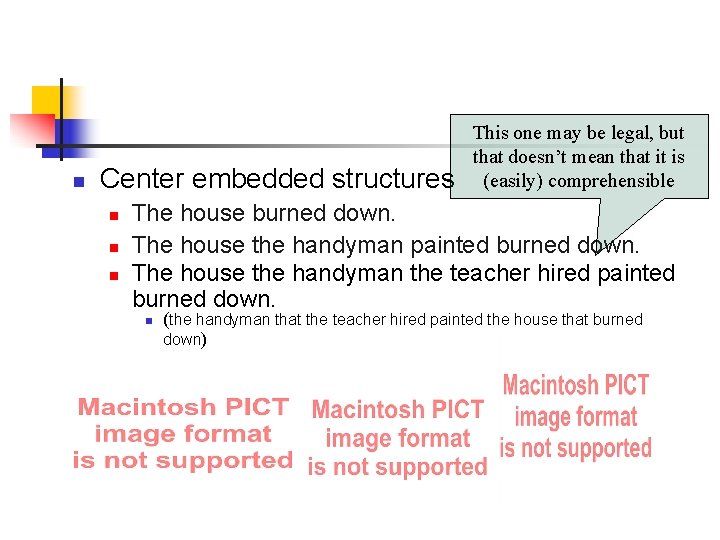
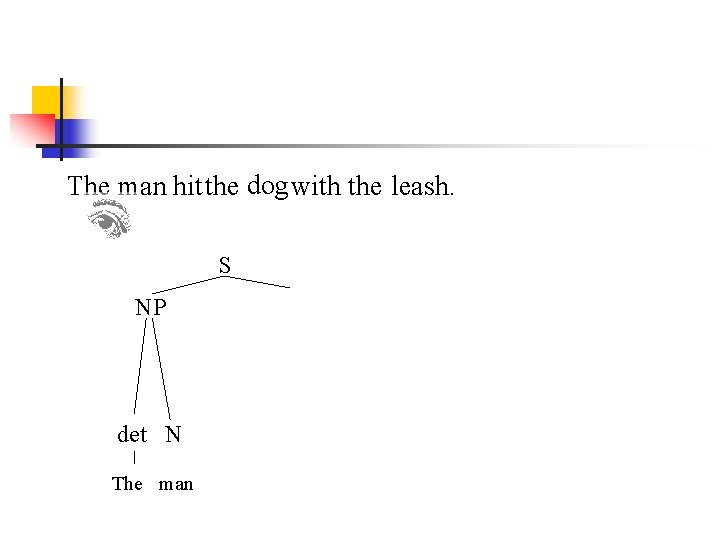
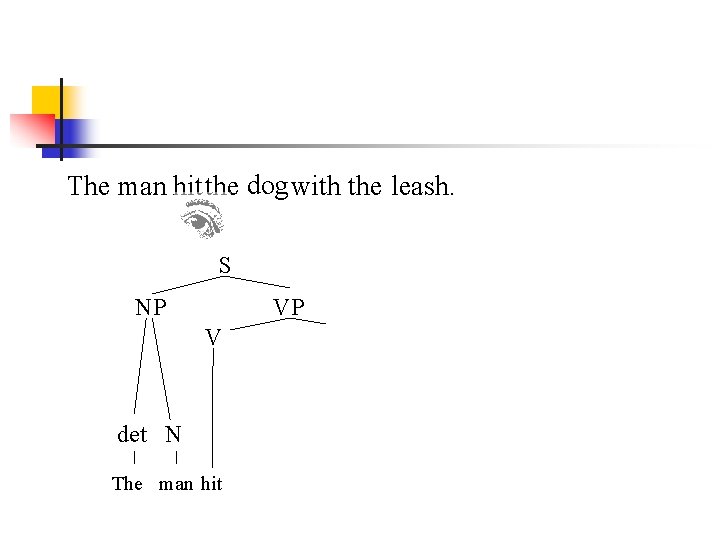
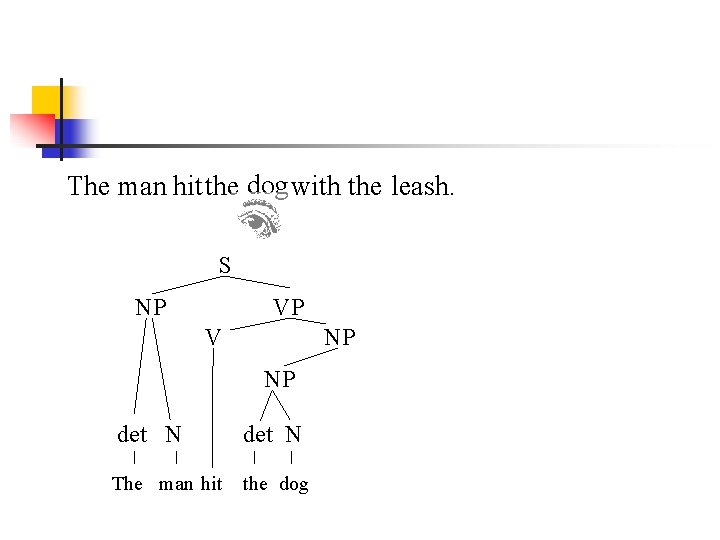
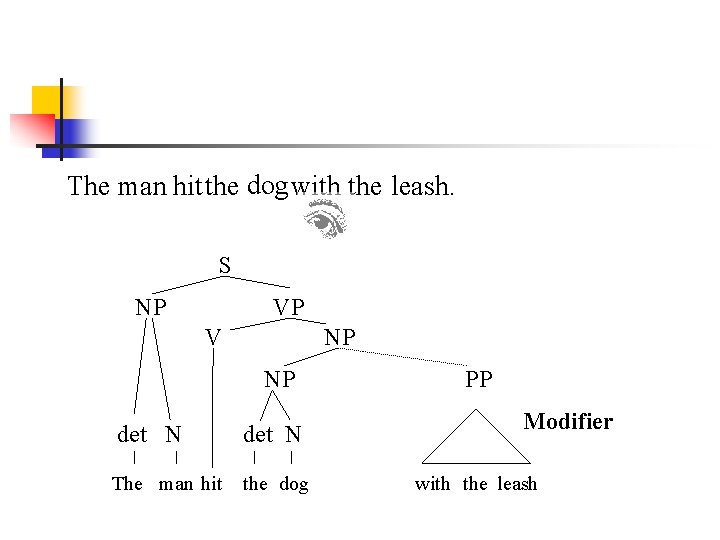
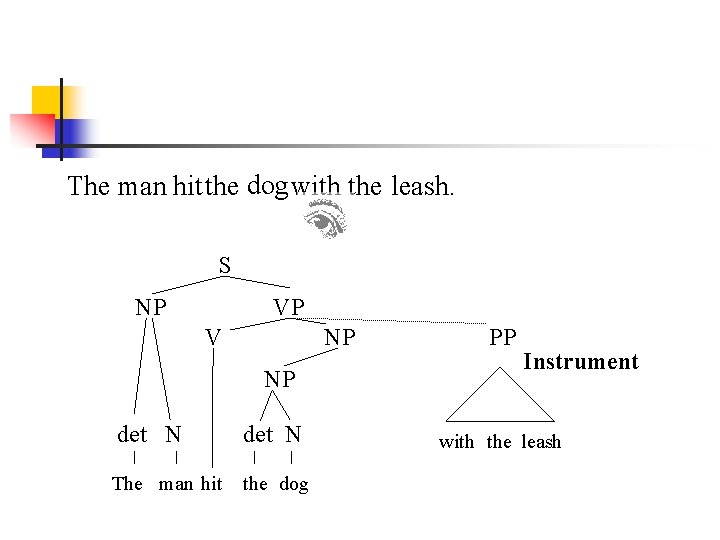
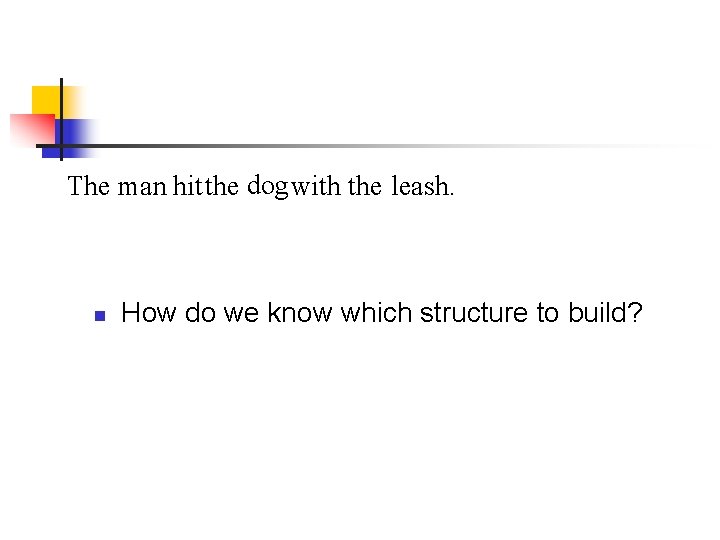
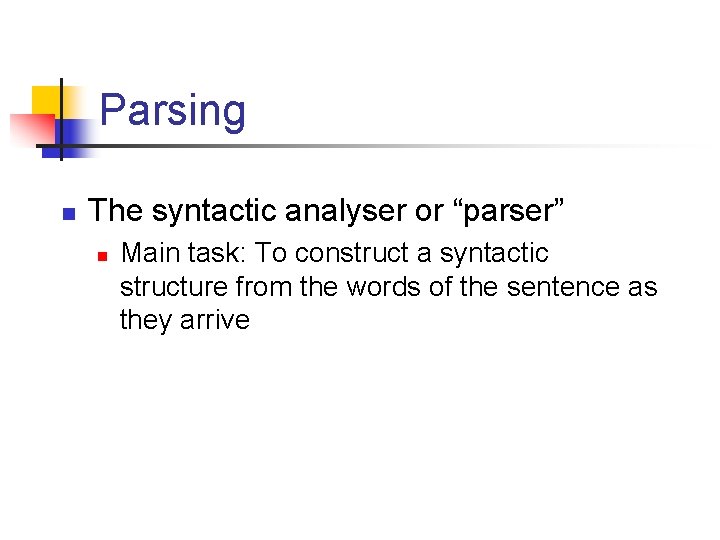
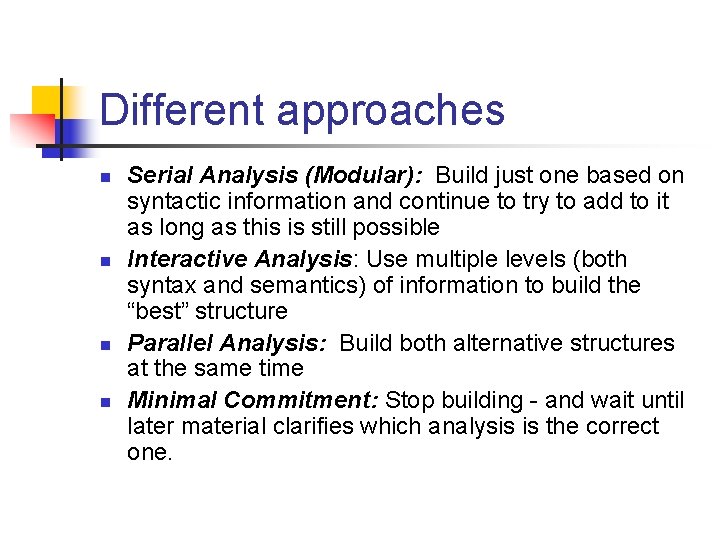
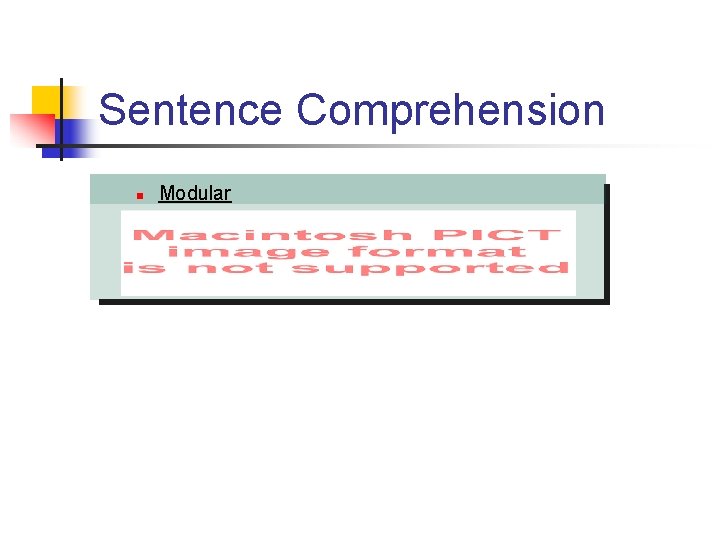
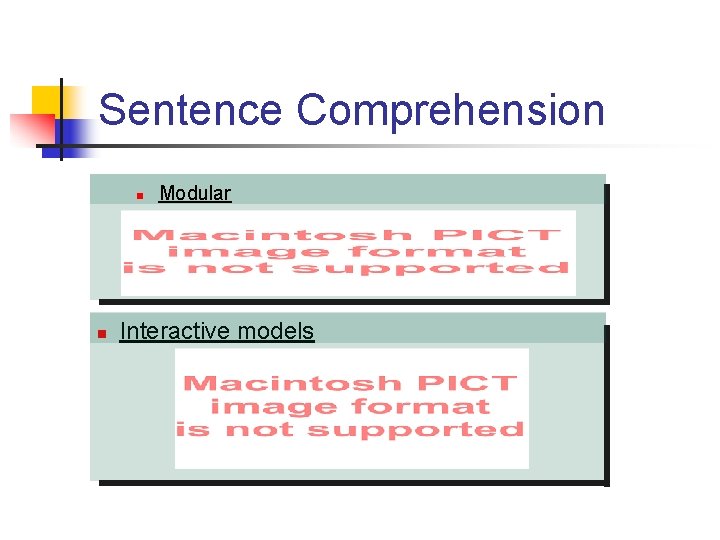
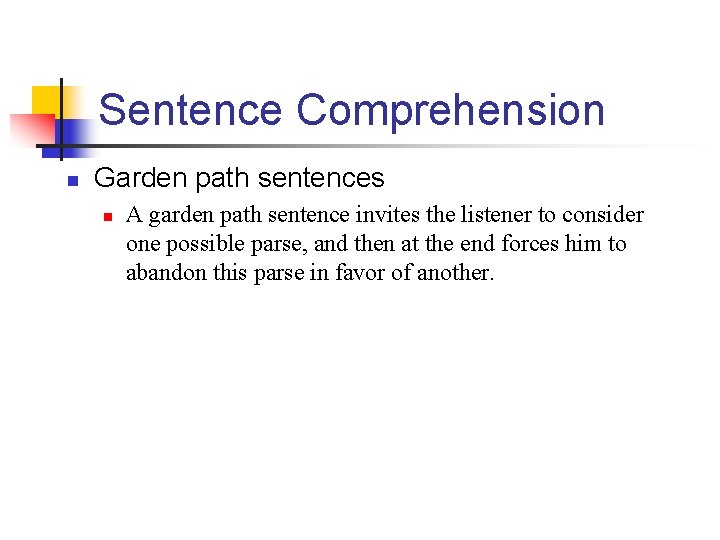
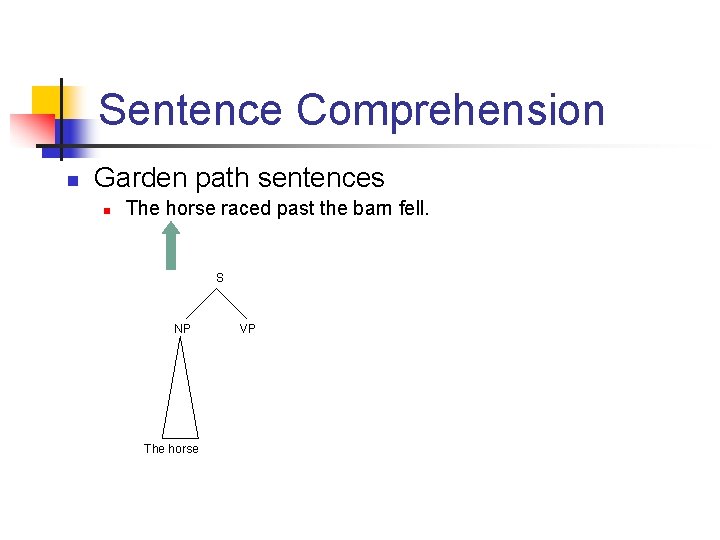
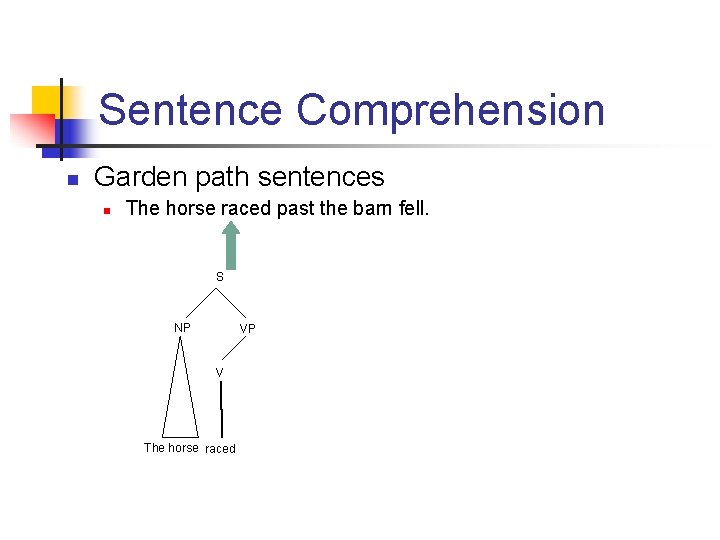
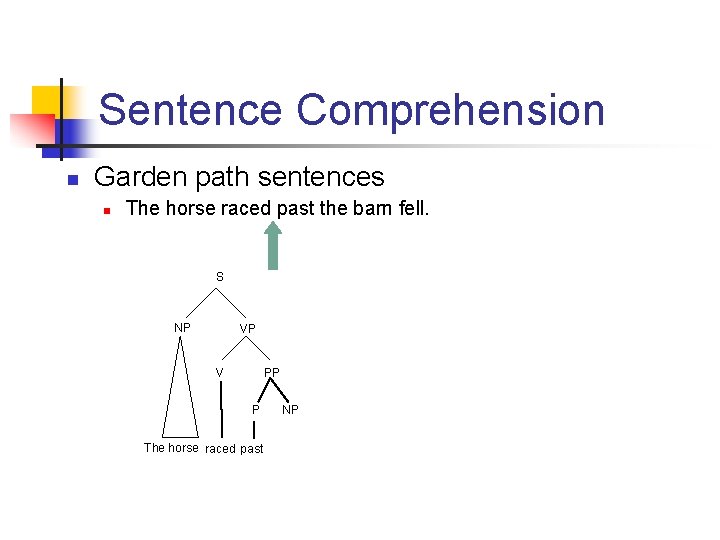
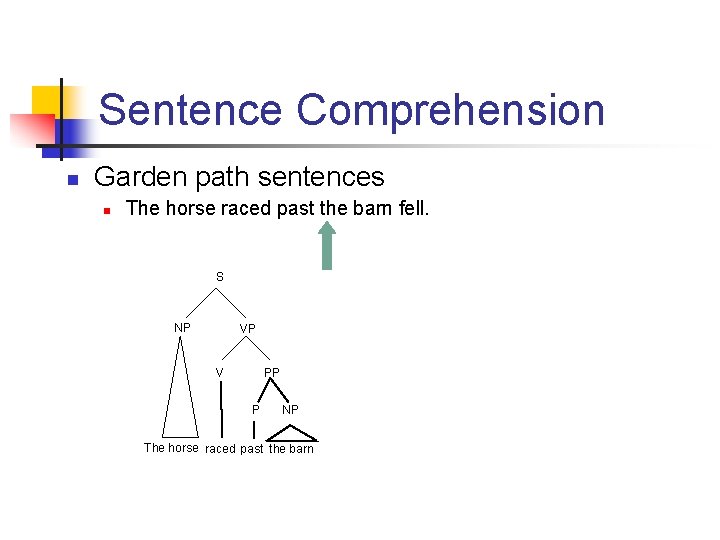
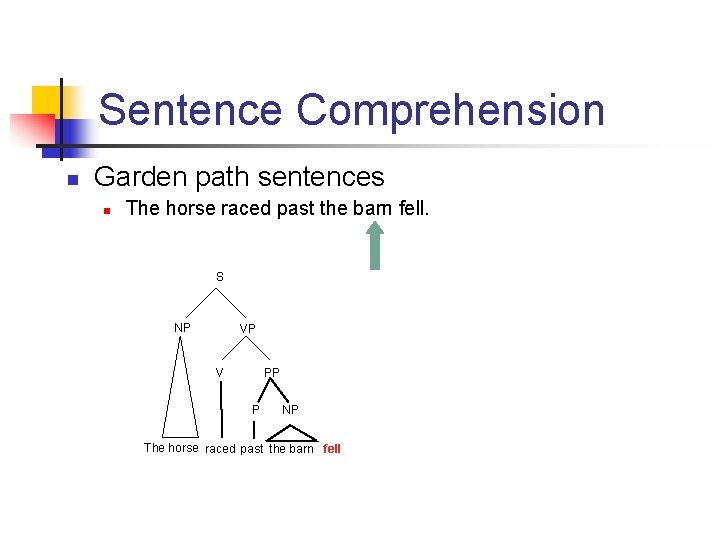
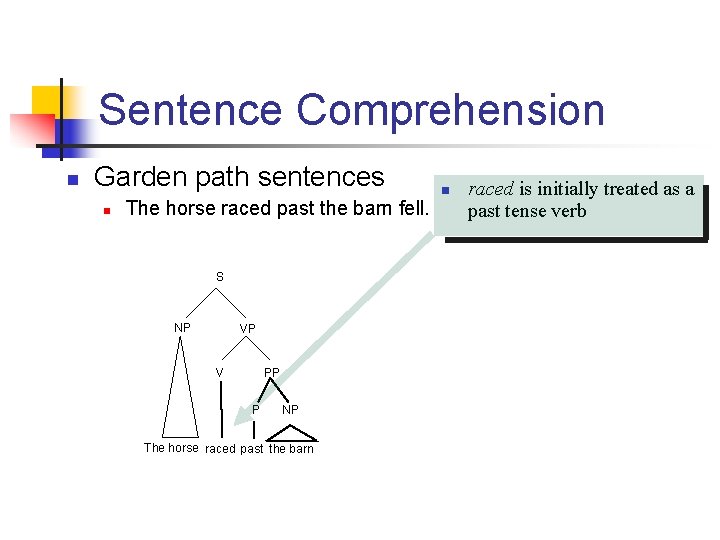
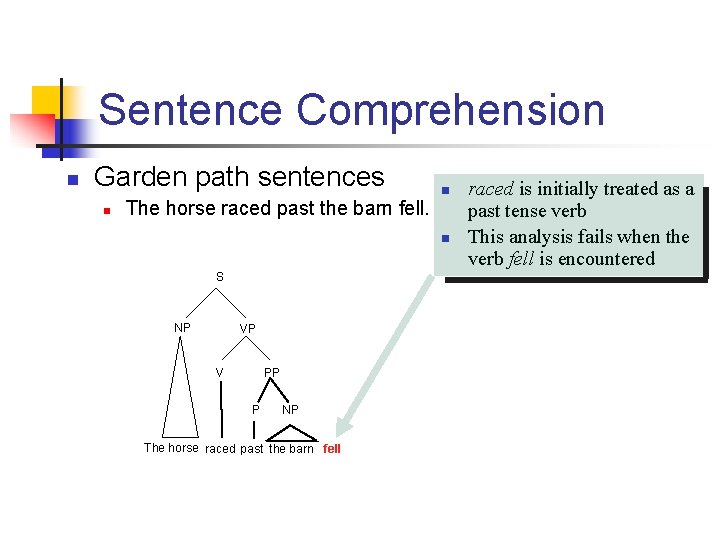
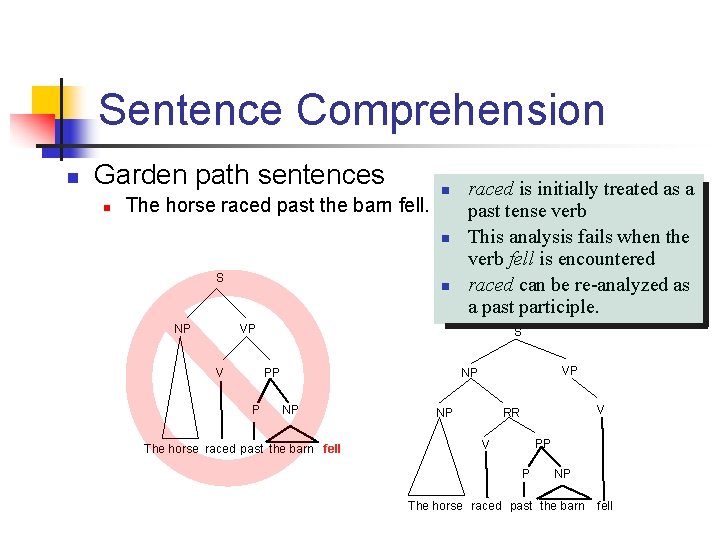
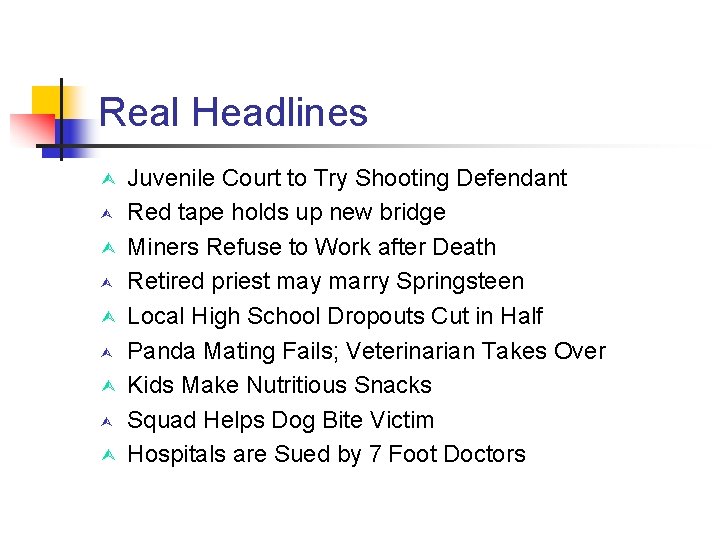
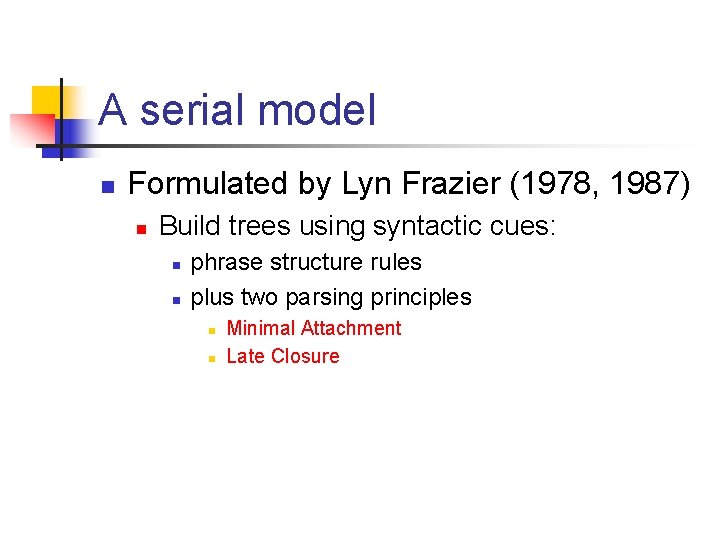
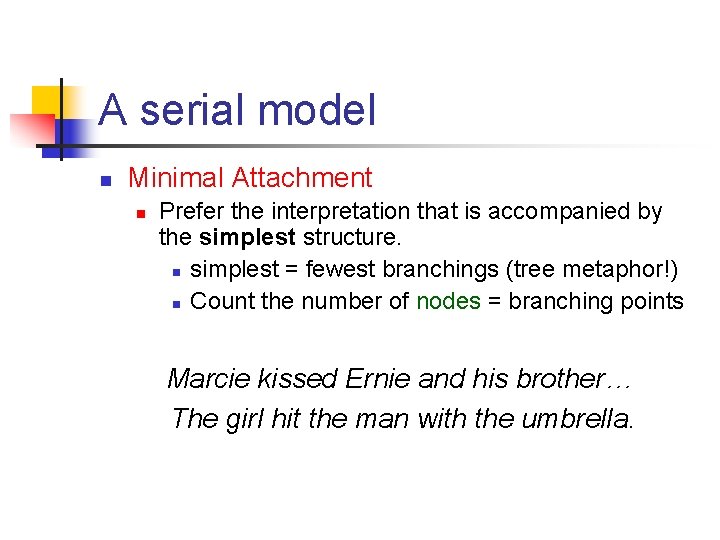
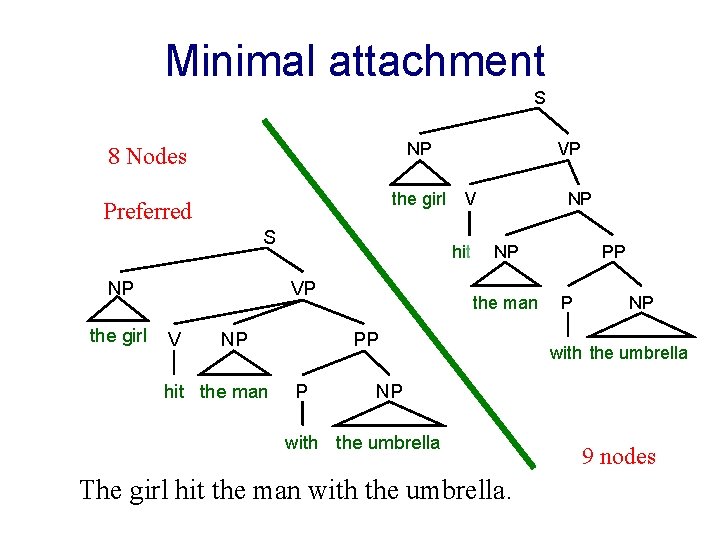
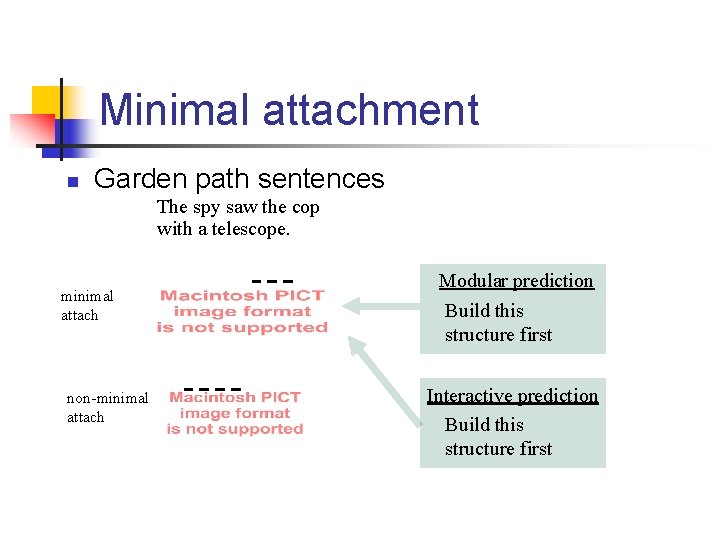
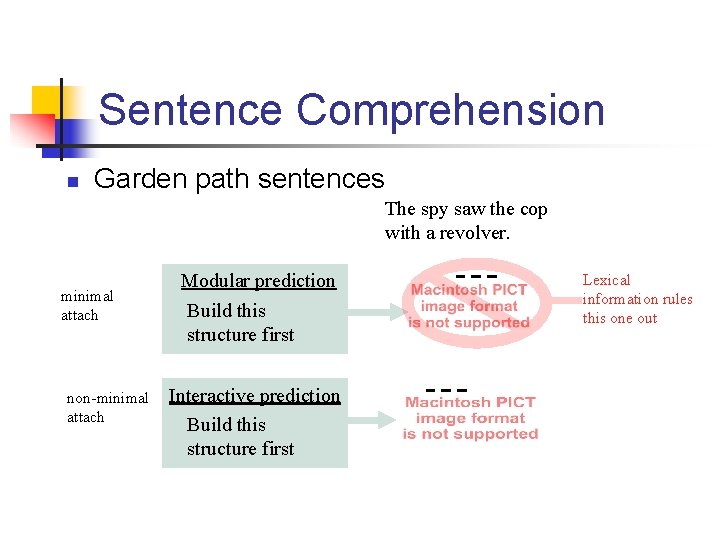
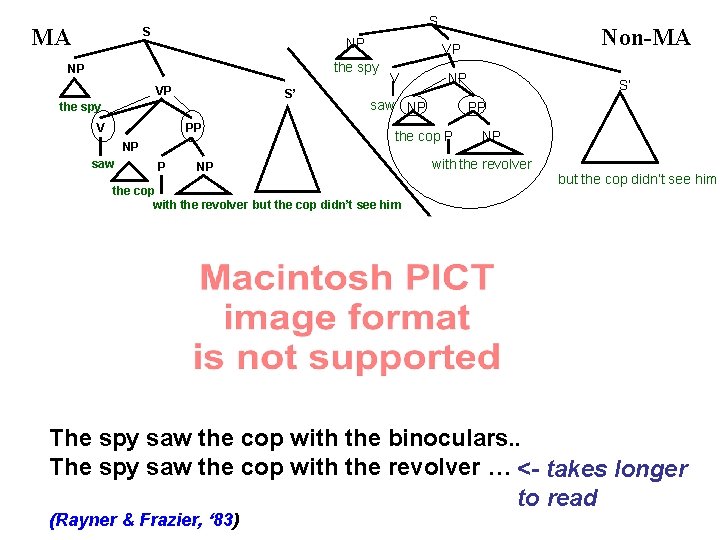
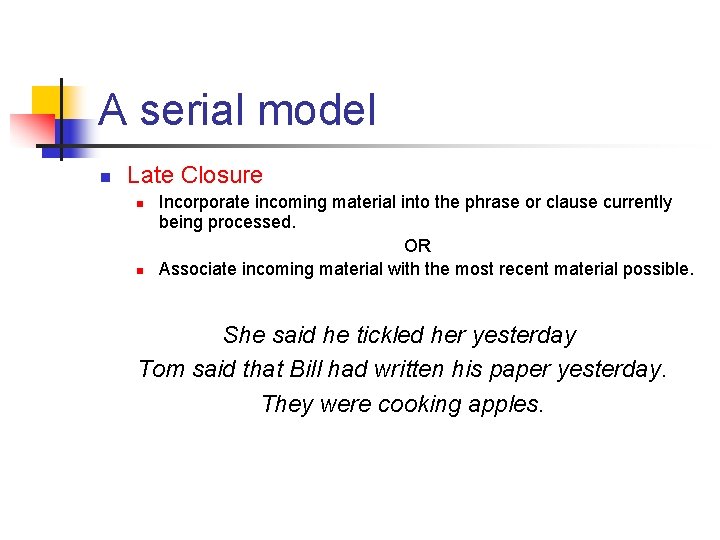
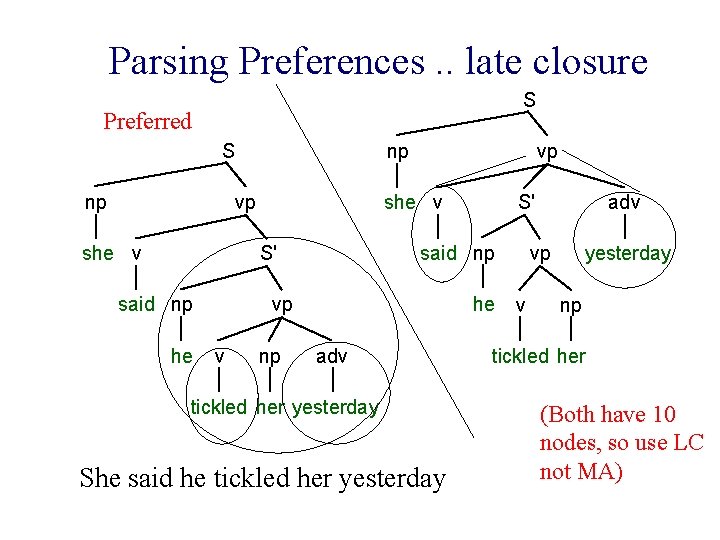
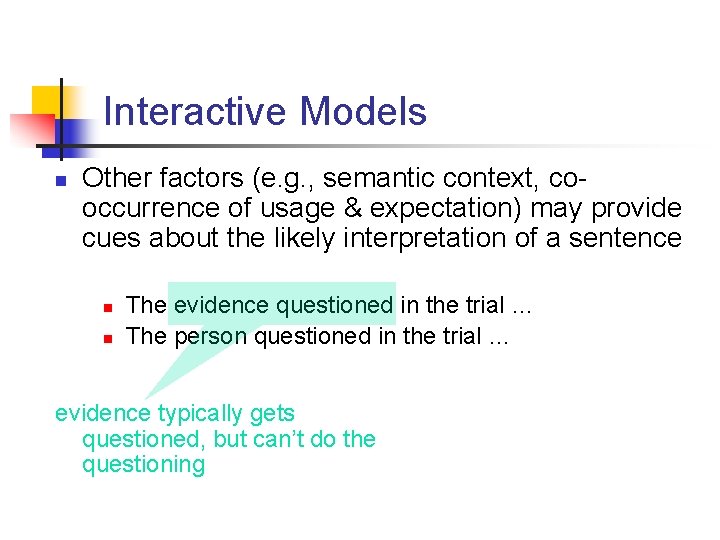
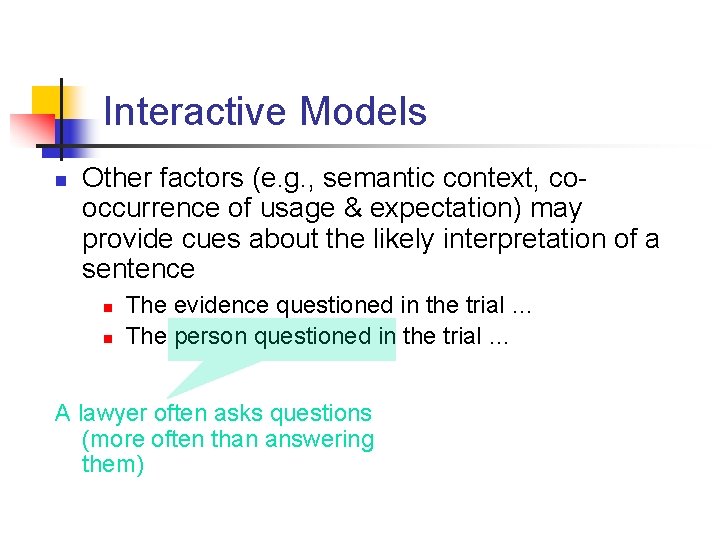
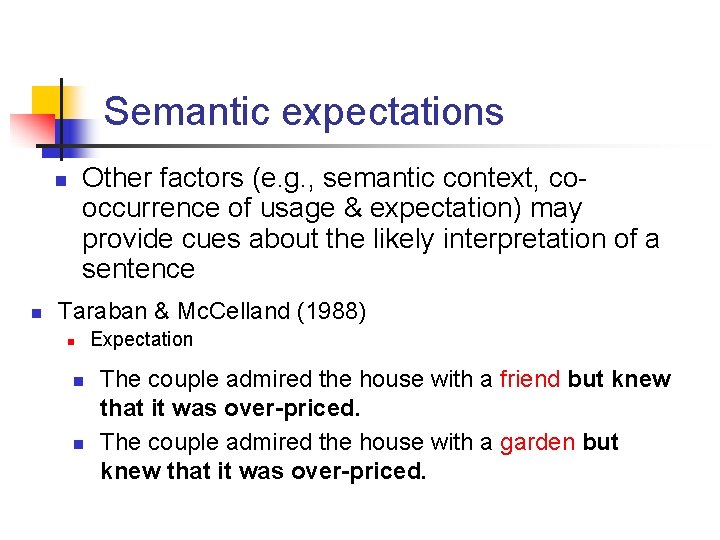
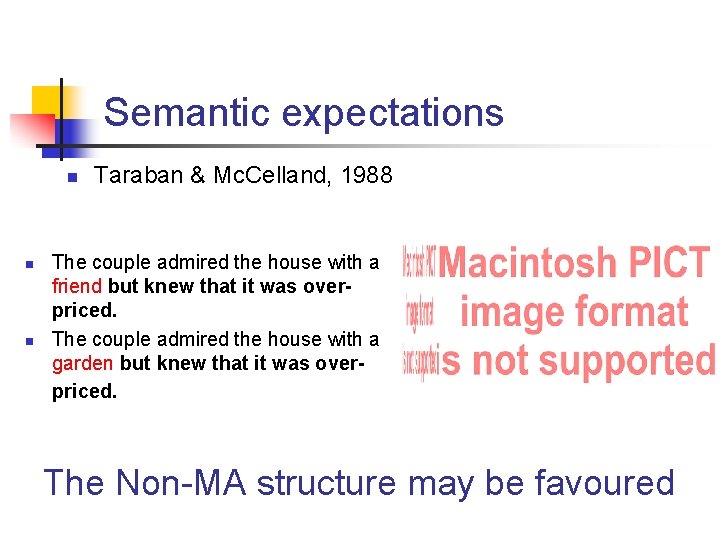
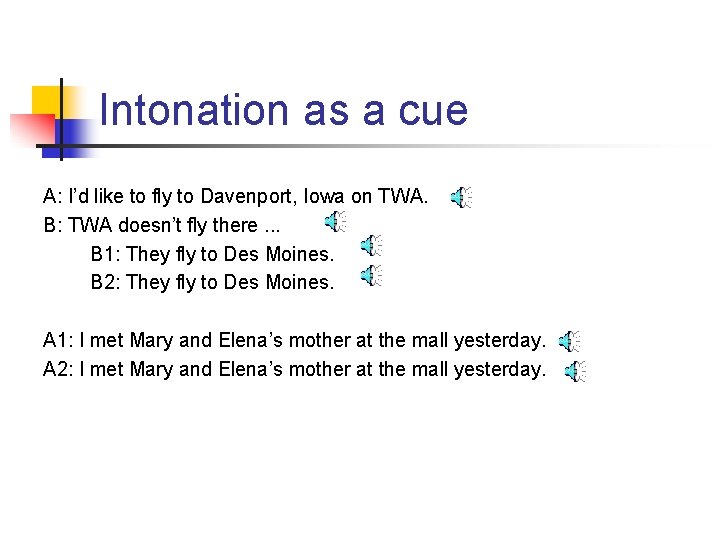
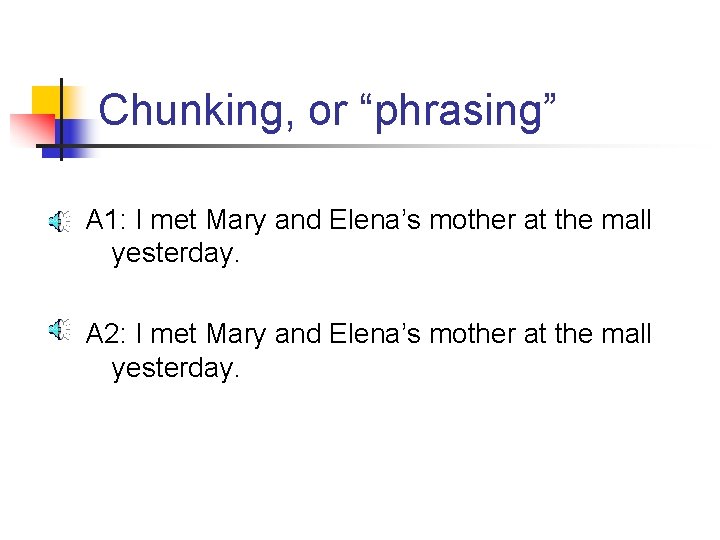
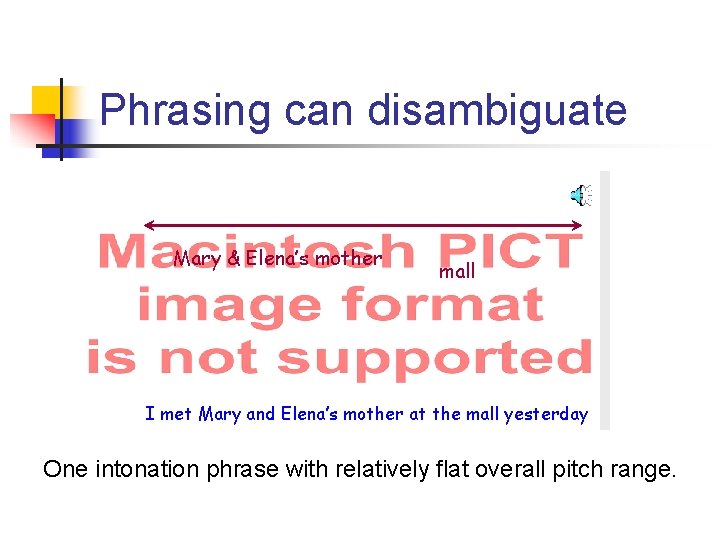
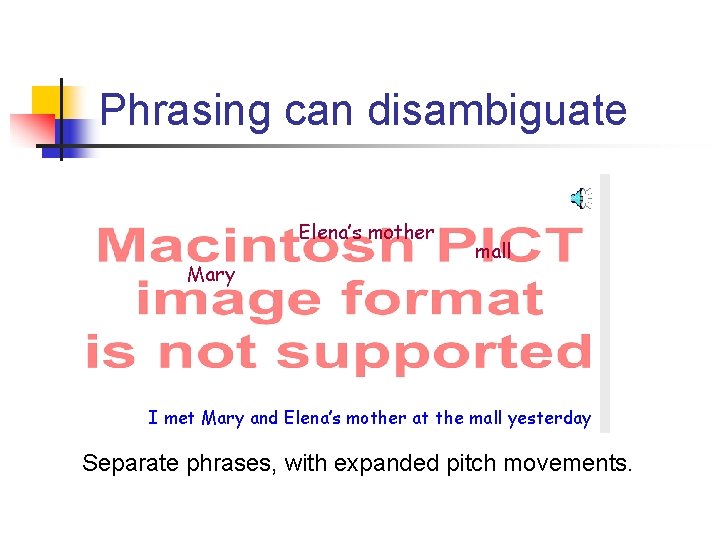
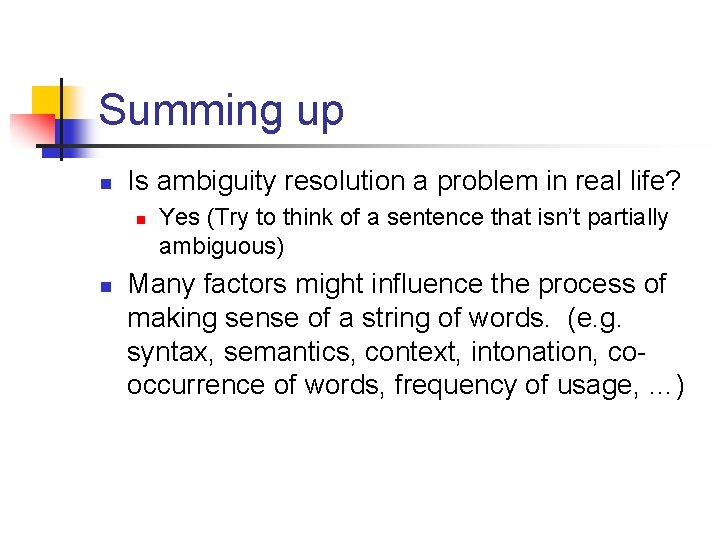
- Slides: 41
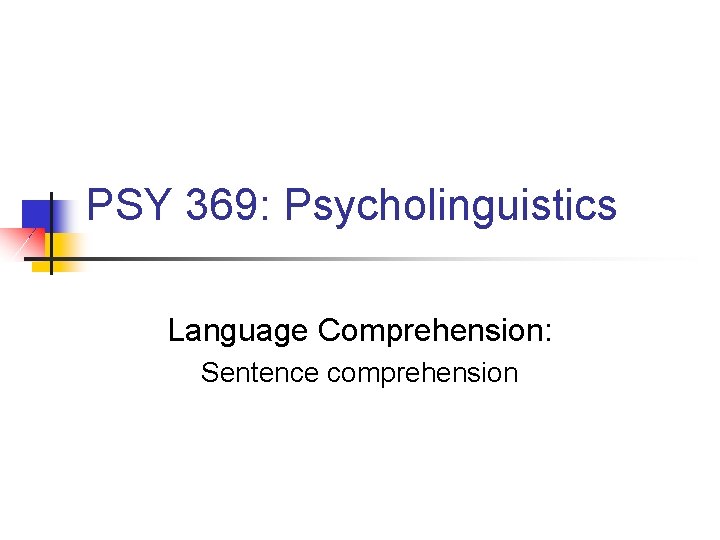
PSY 369: Psycholinguistics Language Comprehension: Sentence comprehension
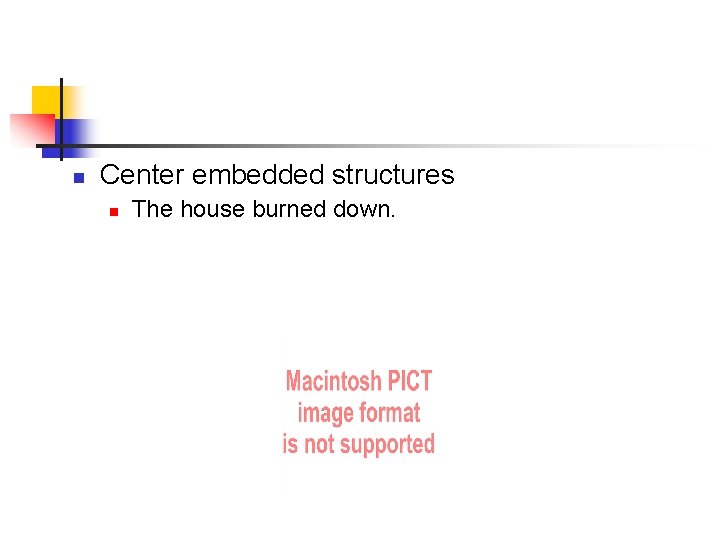
n Center embedded structures n The house burned down.
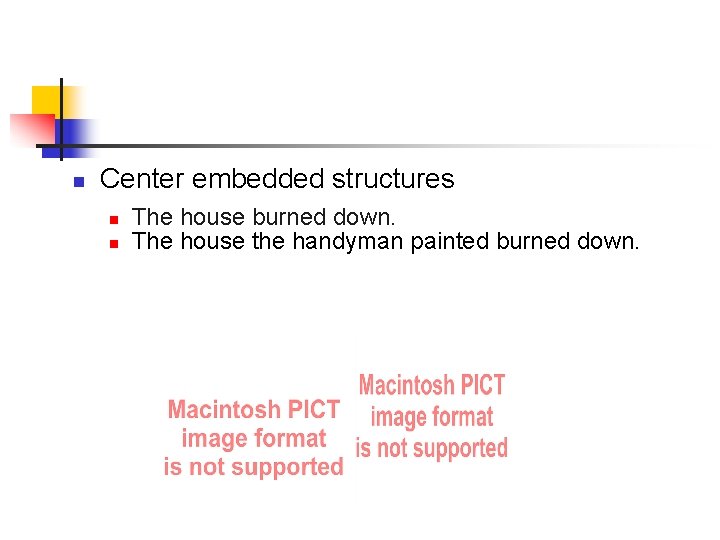
n Center embedded structures n n The house burned down. The house the handyman painted burned down.
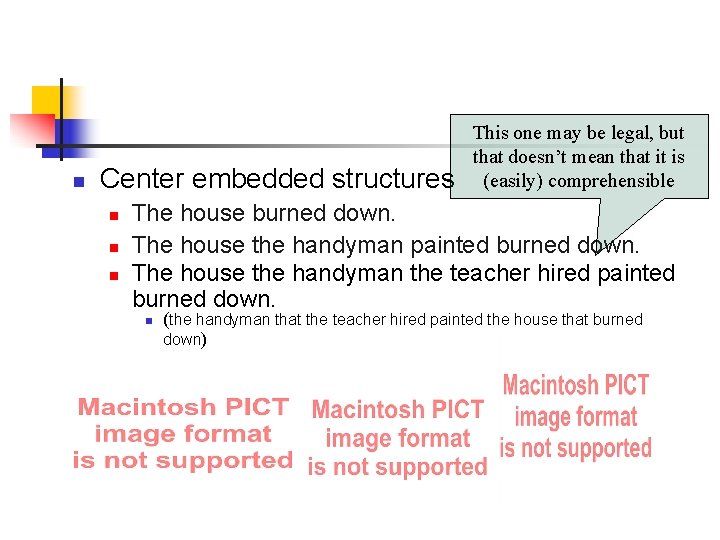
n Center embedded structures n n n This one may be legal, but that doesn’t mean that it is (easily) comprehensible The house burned down. The house the handyman painted burned down. The house the handyman the teacher hired painted burned down. n (the handyman that the teacher hired painted the house that burned down)
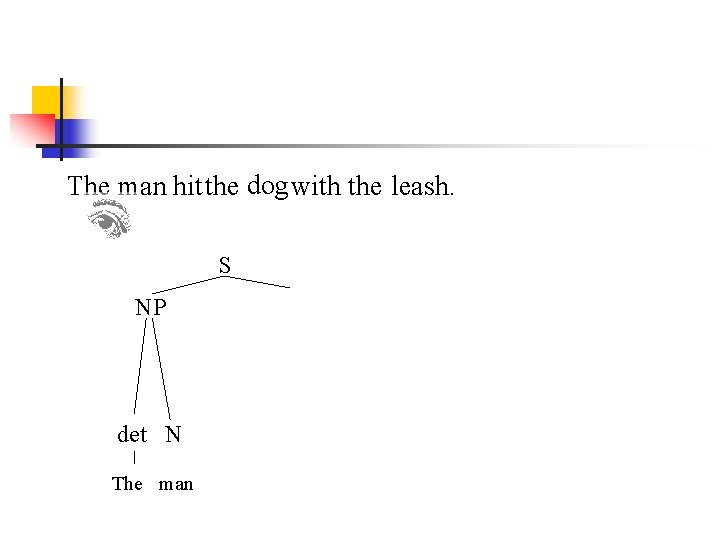
The man hit the dog with the leash. S NP det N The man
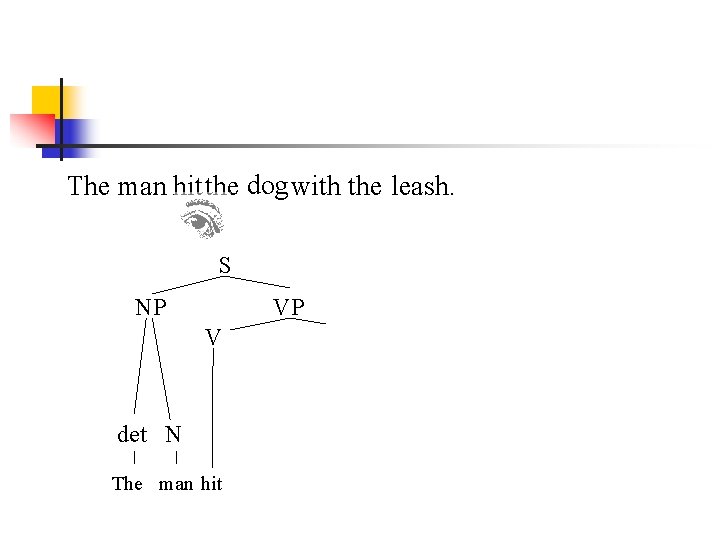
The man hit the dog with the leash. S NP VP V det N The man hit
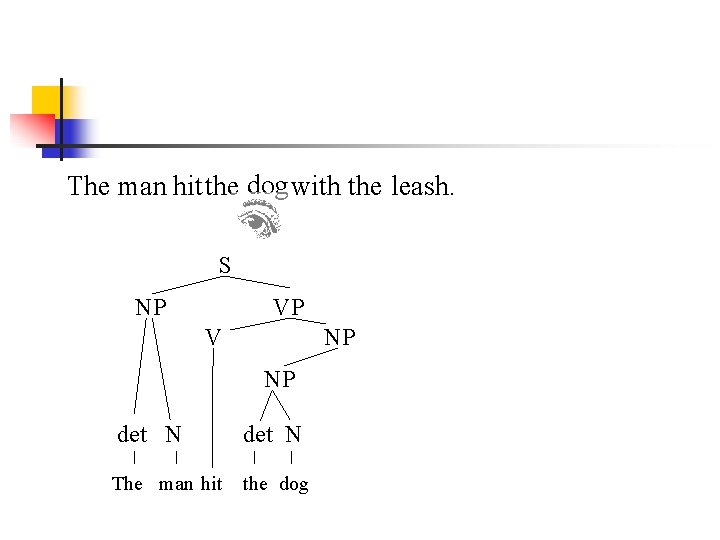
The man hit the dog with the leash. S NP VP V NP NP det N The man hit the dog
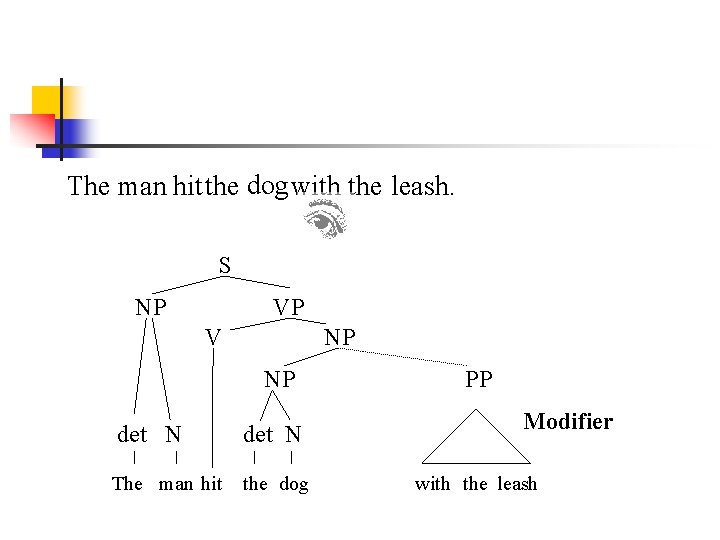
The man hit the dog with the leash. S NP VP V NP NP det N The man hit the dog PP Modifier with the leash
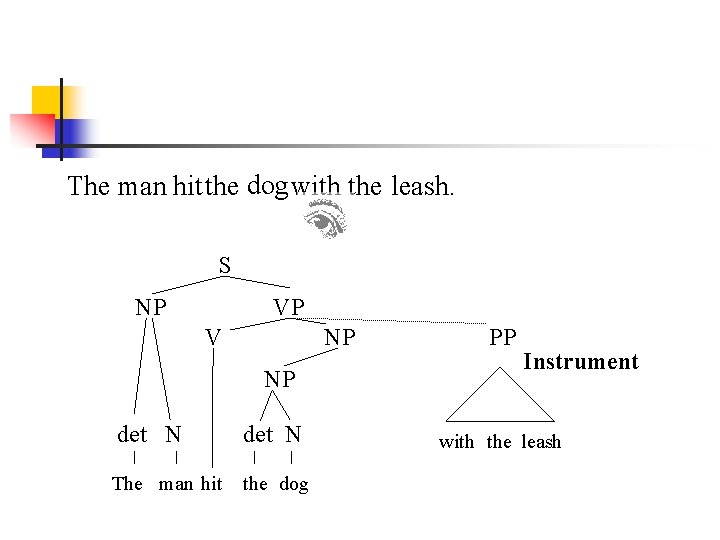
The man hit the dog with the leash. S NP VP V NP NP det N The man hit the dog PP Instrument with the leash
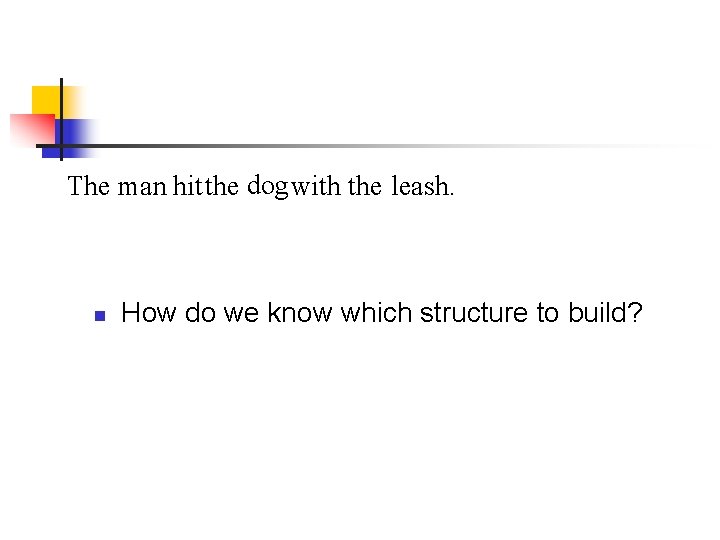
The man hit the dog with the leash. n How do we know which structure to build?
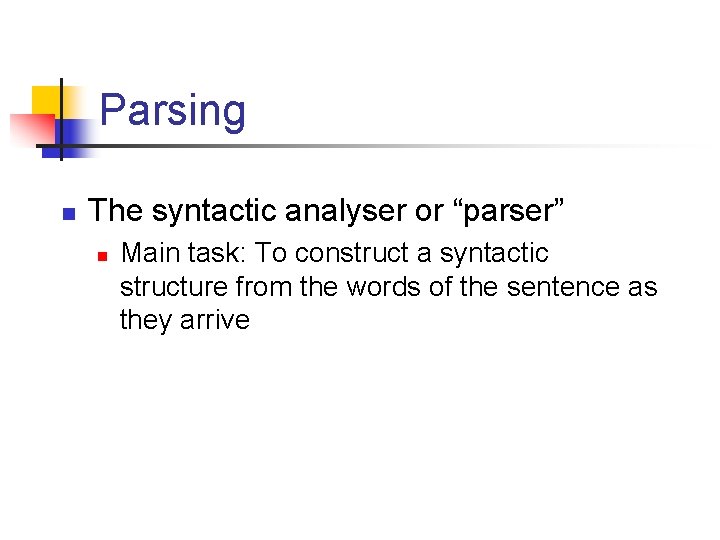
Parsing n The syntactic analyser or “parser” n Main task: To construct a syntactic structure from the words of the sentence as they arrive
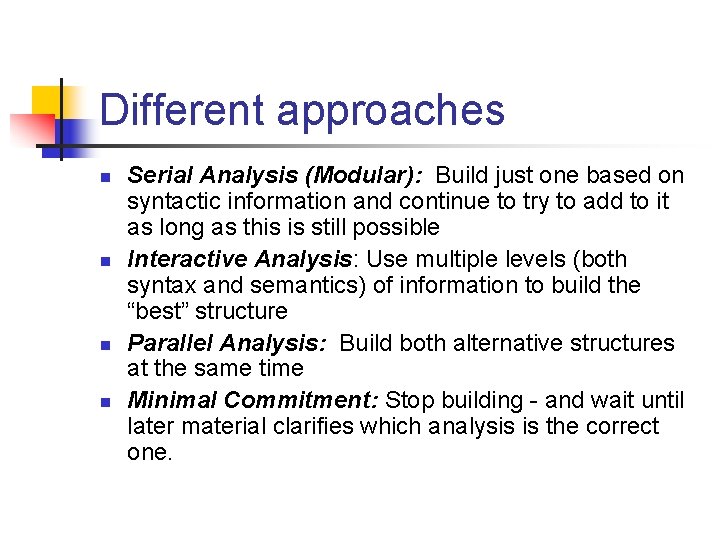
Different approaches n n Serial Analysis (Modular): Build just one based on syntactic information and continue to try to add to it as long as this is still possible Interactive Analysis: Use multiple levels (both syntax and semantics) of information to build the “best” structure Parallel Analysis: Build both alternative structures at the same time Minimal Commitment: Stop building - and wait until later material clarifies which analysis is the correct one.
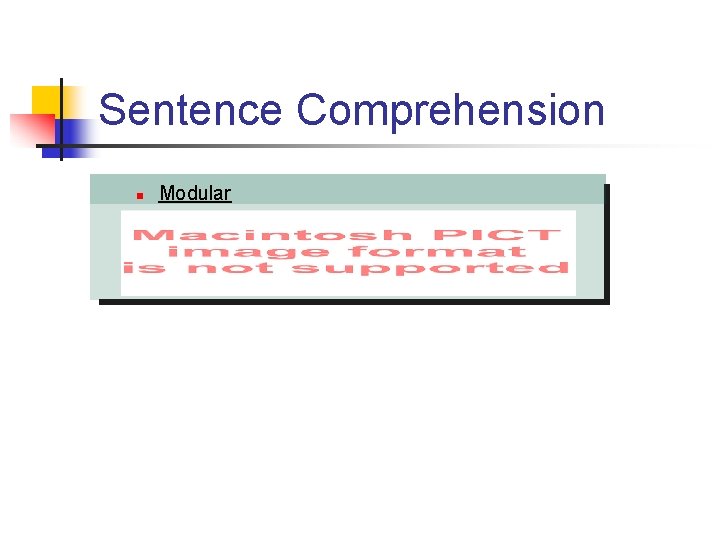
Sentence Comprehension n Modular
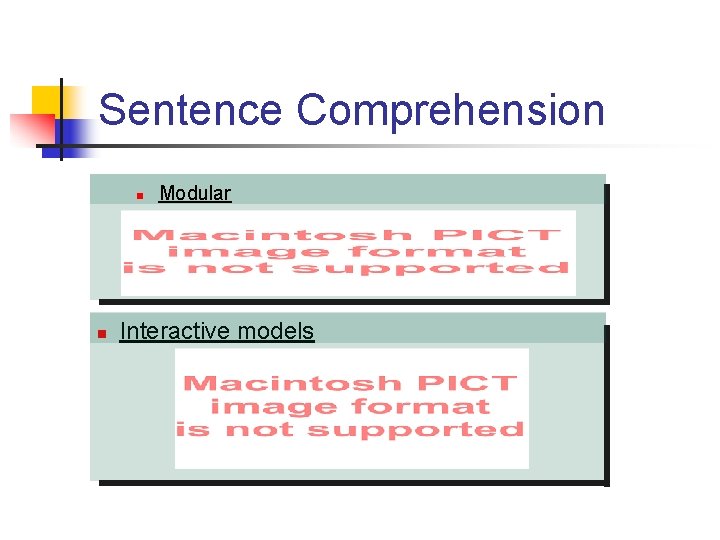
Sentence Comprehension n n Modular Interactive models
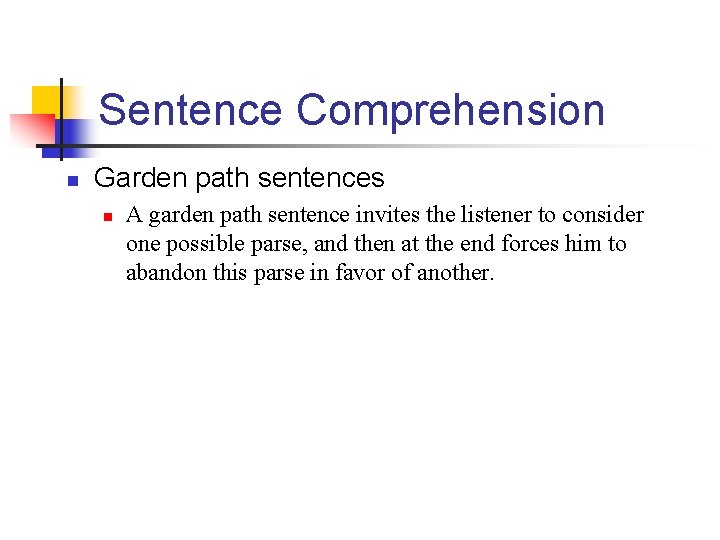
Sentence Comprehension n Garden path sentences n A garden path sentence invites the listener to consider one possible parse, and then at the end forces him to abandon this parse in favor of another.
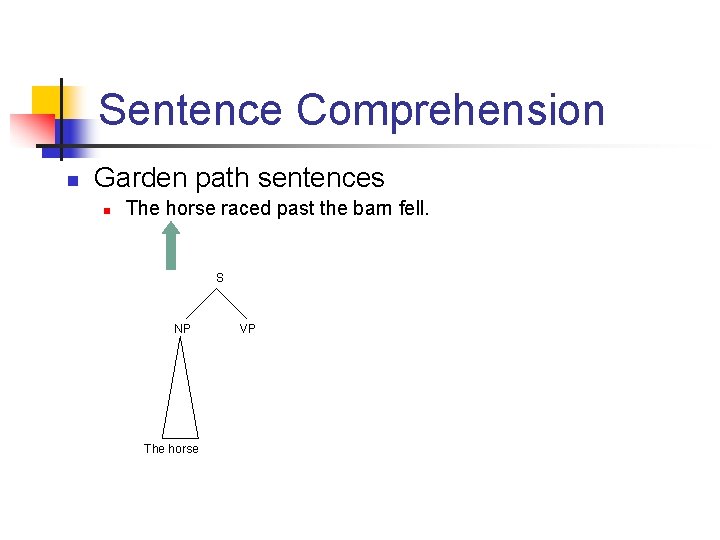
Sentence Comprehension n Garden path sentences n The horse raced past the barn fell. S NP The horse VP
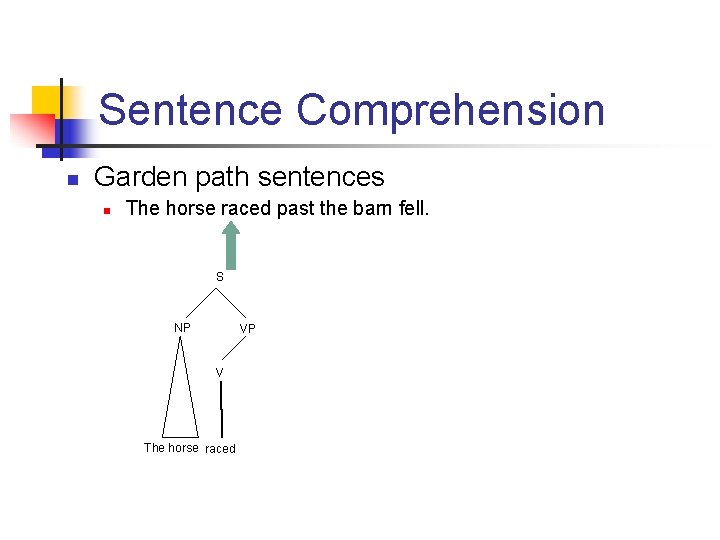
Sentence Comprehension n Garden path sentences n The horse raced past the barn fell. S NP VP V The horse raced
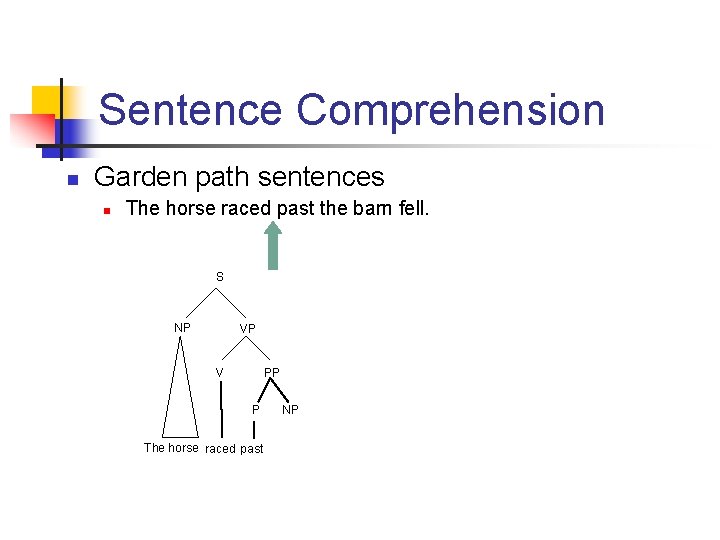
Sentence Comprehension n Garden path sentences n The horse raced past the barn fell. S NP VP V PP P The horse raced past NP
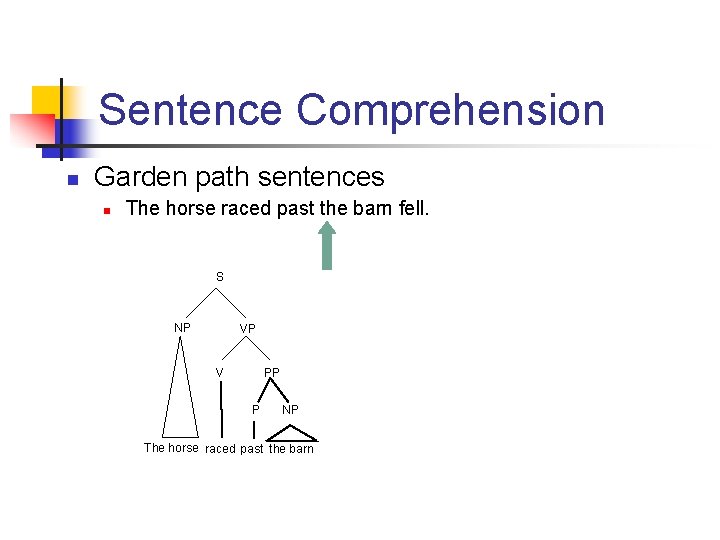
Sentence Comprehension n Garden path sentences n The horse raced past the barn fell. S NP VP V PP P NP The horse raced past the barn
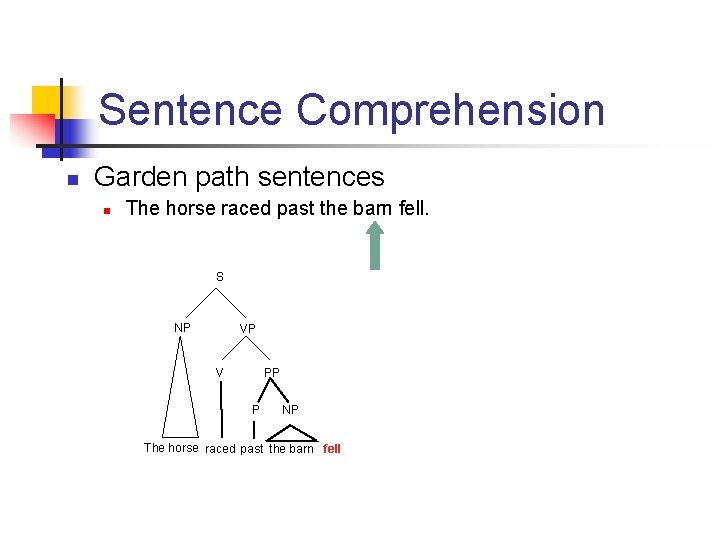
Sentence Comprehension n Garden path sentences n The horse raced past the barn fell. S NP VP V PP P NP The horse raced past the barn fell
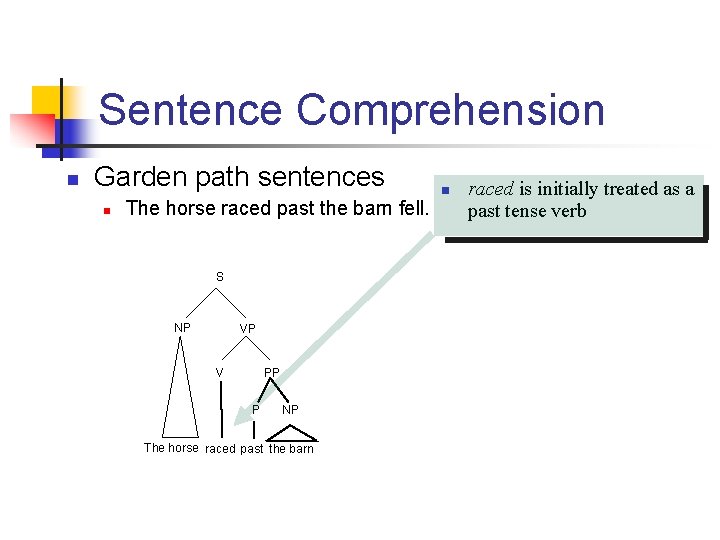
Sentence Comprehension n Garden path sentences n The horse raced past the barn fell. S NP VP V PP P NP The horse raced past the barn n raced is initially treated as a past tense verb
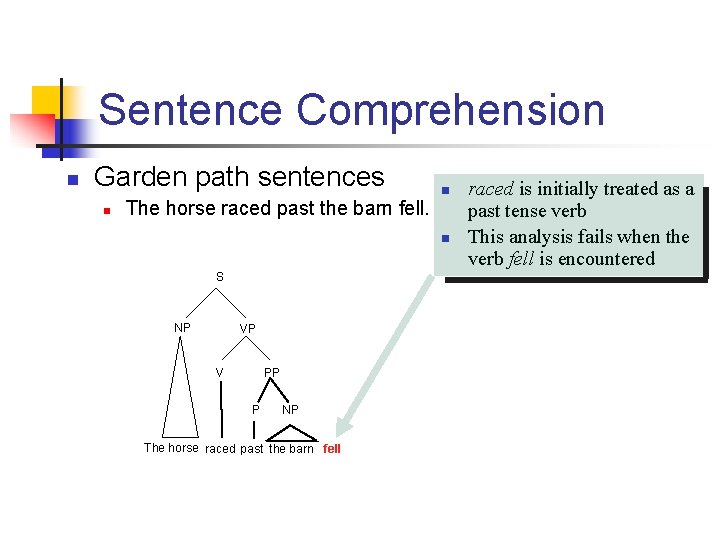
Sentence Comprehension n Garden path sentences n The horse raced past the barn fell. n n S NP VP V PP P NP The horse raced past the barn fell raced is initially treated as a past tense verb This analysis fails when the verb fell is encountered
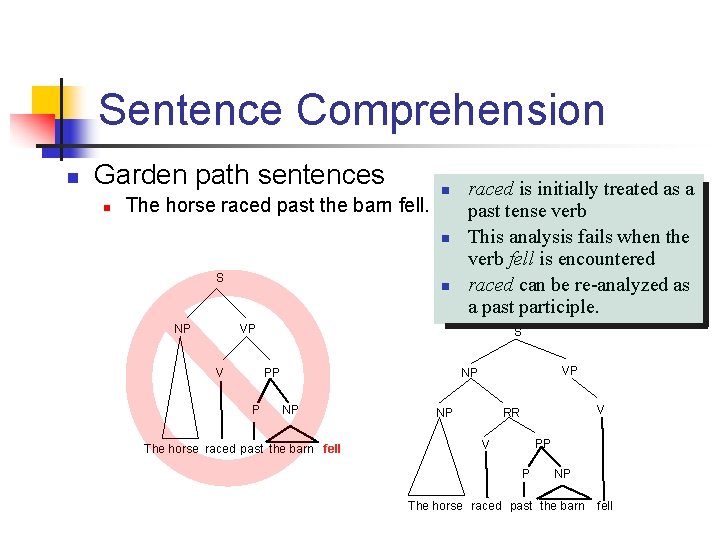
Sentence Comprehension n Garden path sentences n The horse raced past the barn fell. n n S NP n raced is initially treated as a past tense verb This analysis fails when the verb fell is encountered raced can be re-analyzed as a past participle. VP V S PP P VP NP NP The horse raced past the barn fell NP V RR PP V P NP The horse raced past the barn fell
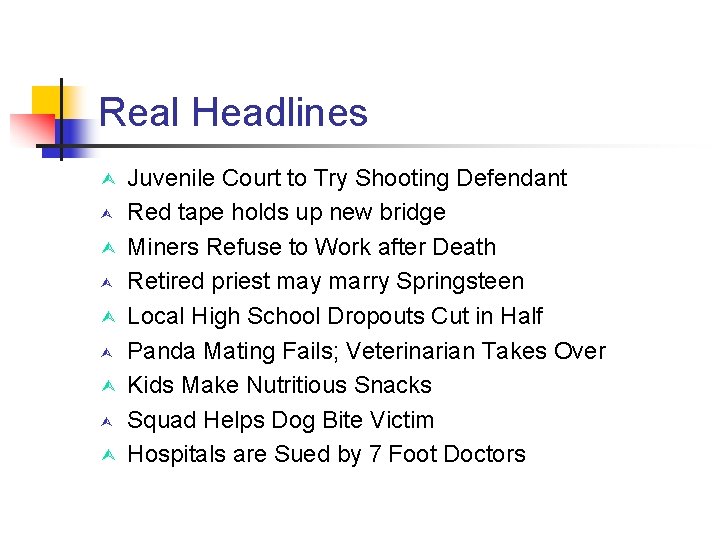
Real Headlines Juvenile Court to Try Shooting Defendant Red tape holds up new bridge Miners Refuse to Work after Death Retired priest may marry Springsteen Local High School Dropouts Cut in Half Panda Mating Fails; Veterinarian Takes Over Kids Make Nutritious Snacks Squad Helps Dog Bite Victim Hospitals are Sued by 7 Foot Doctors
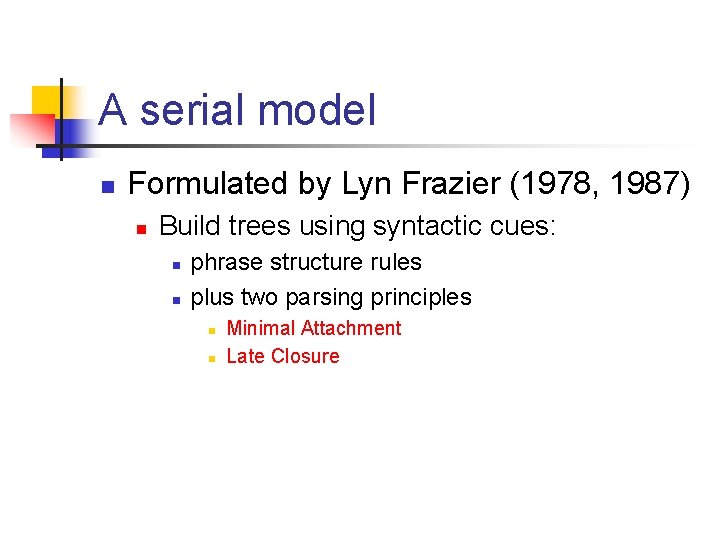
A serial model n Formulated by Lyn Frazier (1978, 1987) n Build trees using syntactic cues: n n phrase structure rules plus two parsing principles n n Minimal Attachment Late Closure
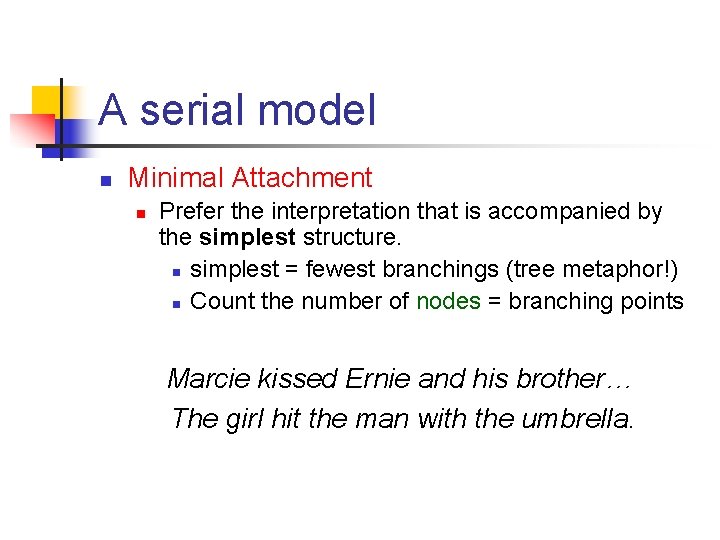
A serial model n Minimal Attachment n Prefer the interpretation that is accompanied by the simplest structure. n simplest = fewest branchings (tree metaphor!) n Count the number of nodes = branching points Marcie kissed Ernie and his brother… The girl hit the man with the umbrella.
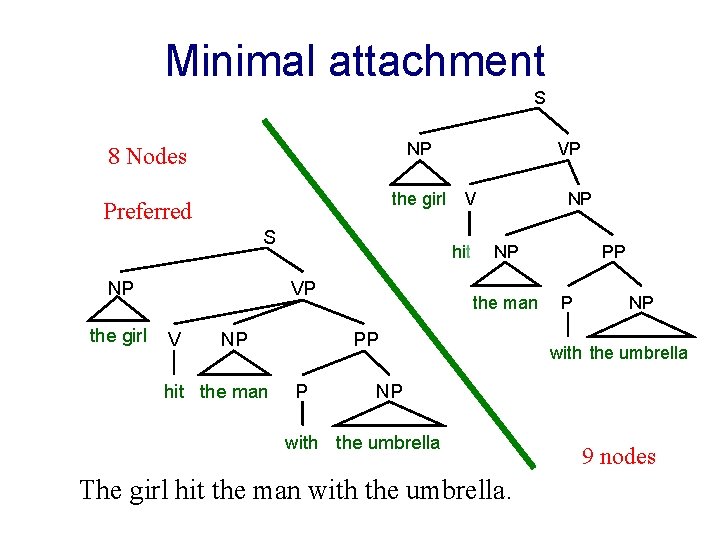
Minimal attachment S 8 Nodes NP Preferred the girl S NP the girl NP hit the man V hit VP V VP NP NP the man PP P NP with the umbrella The girl hit the man with the umbrella. 9 nodes
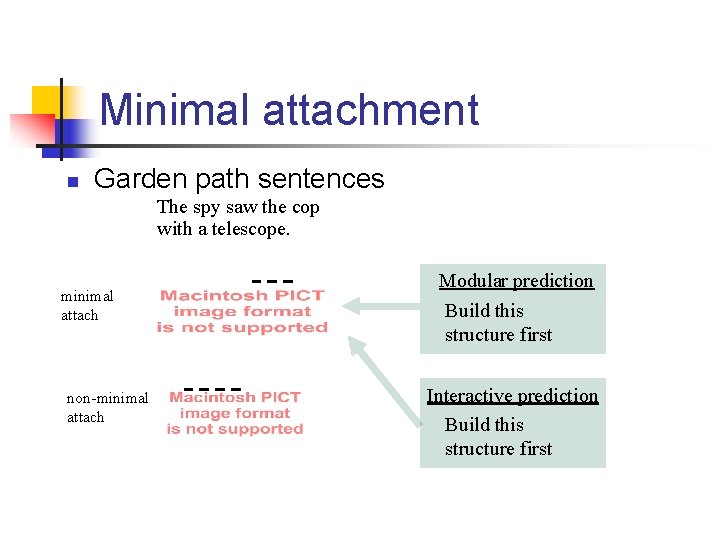
Minimal attachment n Garden path sentences The spy saw the cop with a telescope. minimal attach non-minimal attach Modular prediction Build this structure first Interactive prediction Build this structure first
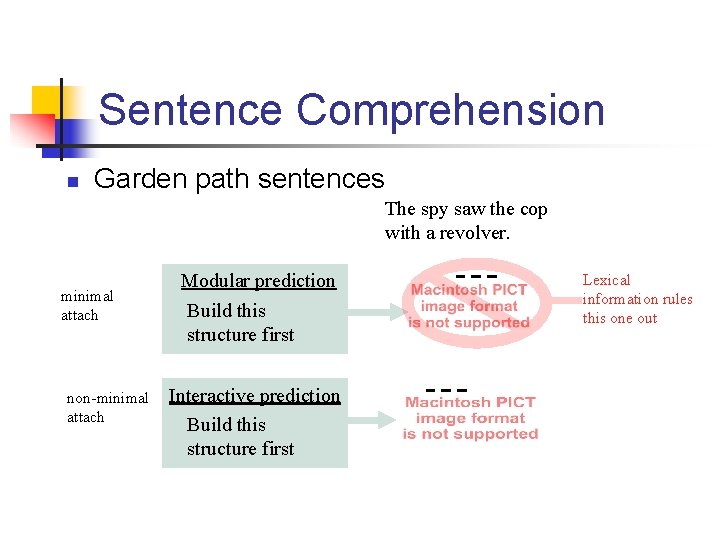
Sentence Comprehension n Garden path sentences The spy saw the cop with a revolver. minimal attach non-minimal attach Modular prediction Build this structure first Interactive prediction Build this structure first Lexical information rules this one out
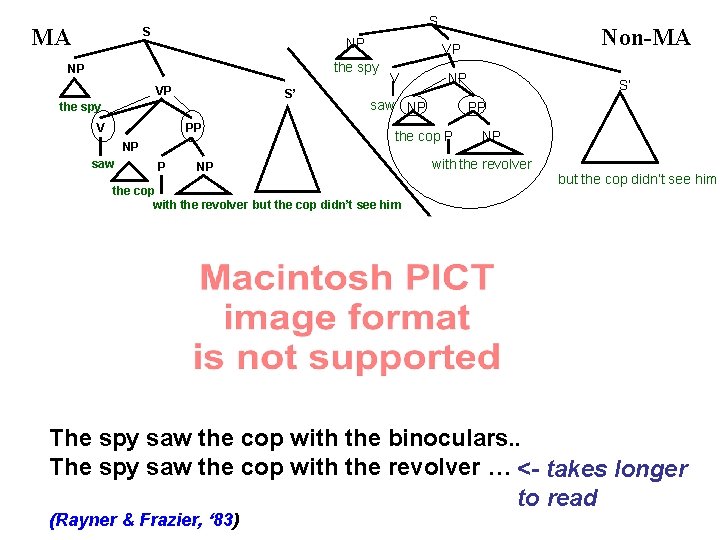
MA S S NP the spy NP VP S’ the spy V PP NP saw P Non-MA VP V NP saw NP PP the cop P NP the cop with the revolver but the cop didn’t see him S’ NP with the revolver but the cop didn’t see him The spy saw the cop with the binoculars. . The spy saw the cop with the revolver … <- takes longer to read (Rayner & Frazier, ‘ 83)
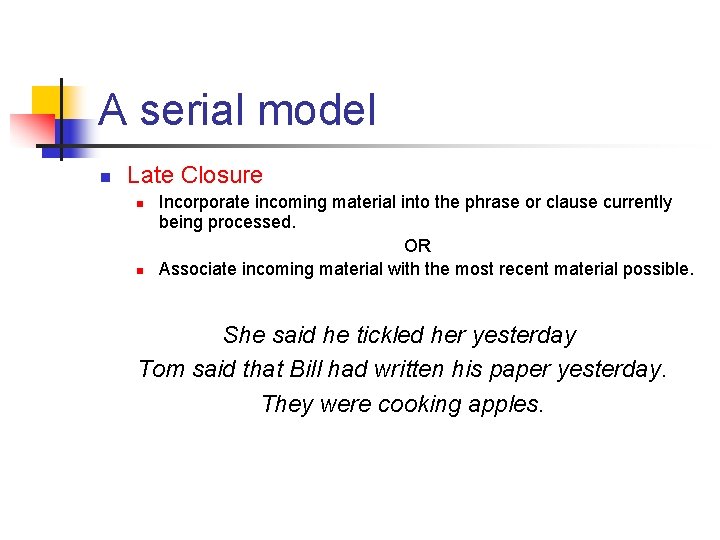
A serial model n Late Closure n n Incorporate incoming material into the phrase or clause currently being processed. OR Associate incoming material with the most recent material possible. She said he tickled her yesterday Tom said that Bill had written his paper yesterday. They were cooking apples.
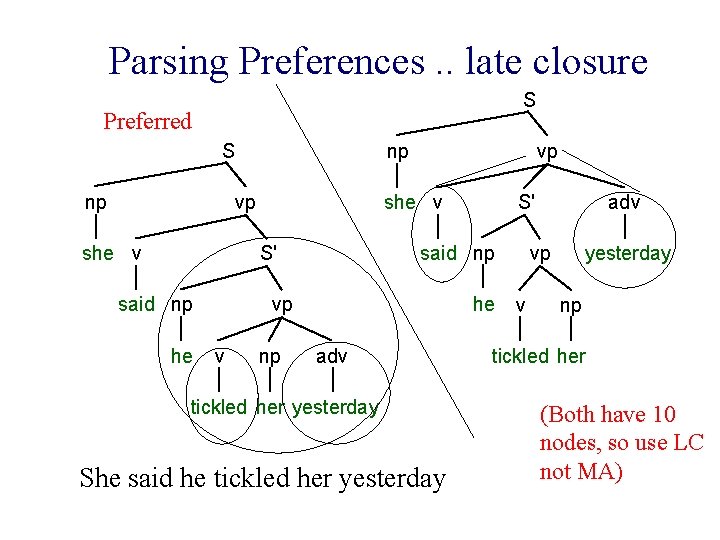
Parsing Preferences. . late closure S Preferred S np np vp she v S' said np he vp said np vp v np S' he adv tickled her yesterday She said he tickled her yesterday adv vp v yesterday np tickled her (Both have 10 nodes, so use LC not MA)
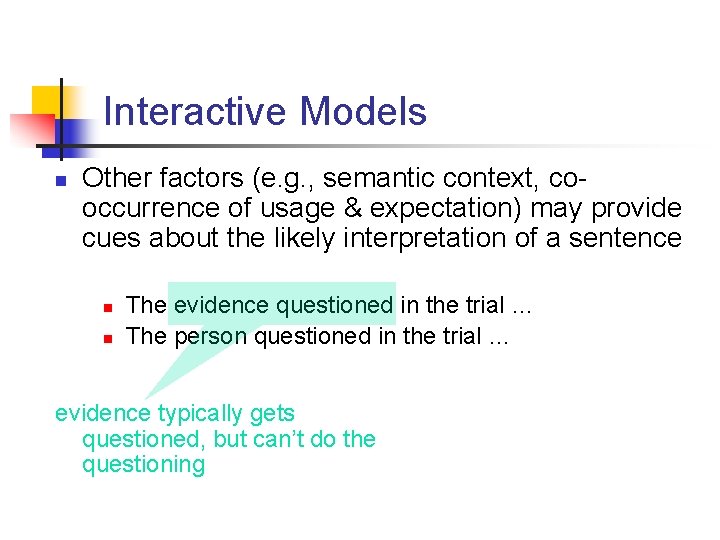
Interactive Models n Other factors (e. g. , semantic context, cooccurrence of usage & expectation) may provide cues about the likely interpretation of a sentence n n The evidence questioned in the trial … The person questioned in the trial … evidence typically gets questioned, but can’t do the questioning
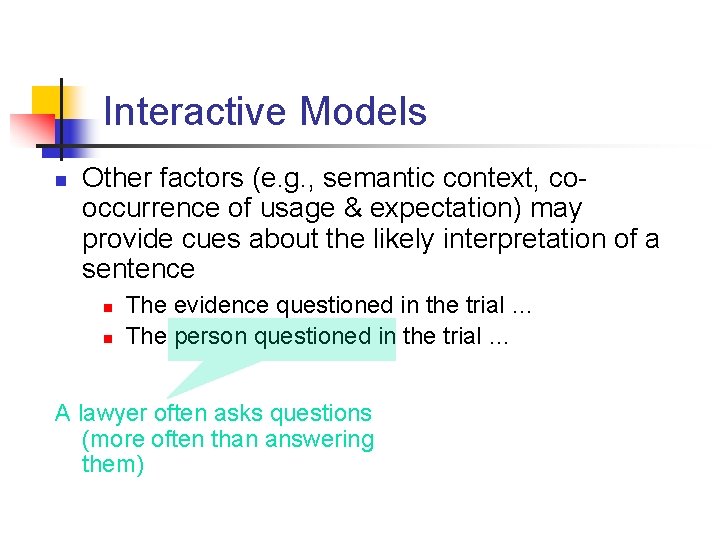
Interactive Models n Other factors (e. g. , semantic context, cooccurrence of usage & expectation) may provide cues about the likely interpretation of a sentence n n The evidence questioned in the trial … The person questioned in the trial … A lawyer often asks questions (more often than answering them)
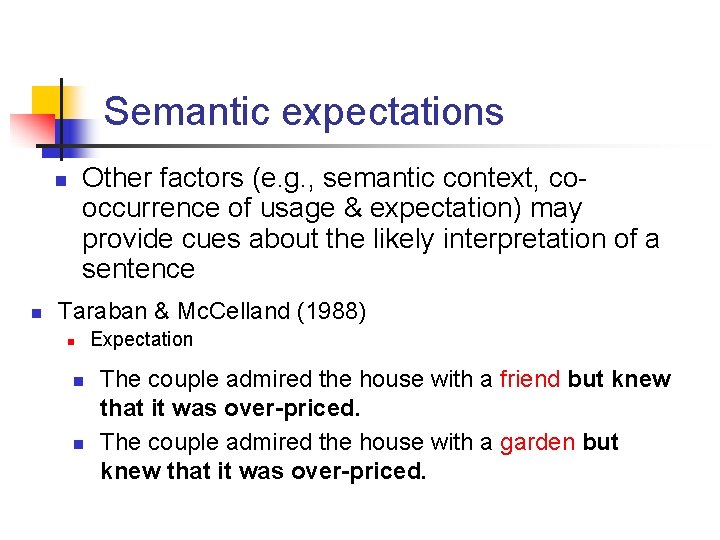
Semantic expectations Other factors (e. g. , semantic context, cooccurrence of usage & expectation) may provide cues about the likely interpretation of a sentence n n Taraban & Mc. Celland (1988) n n n Expectation The couple admired the house with a friend but knew that it was over-priced. The couple admired the house with a garden but knew that it was over-priced.
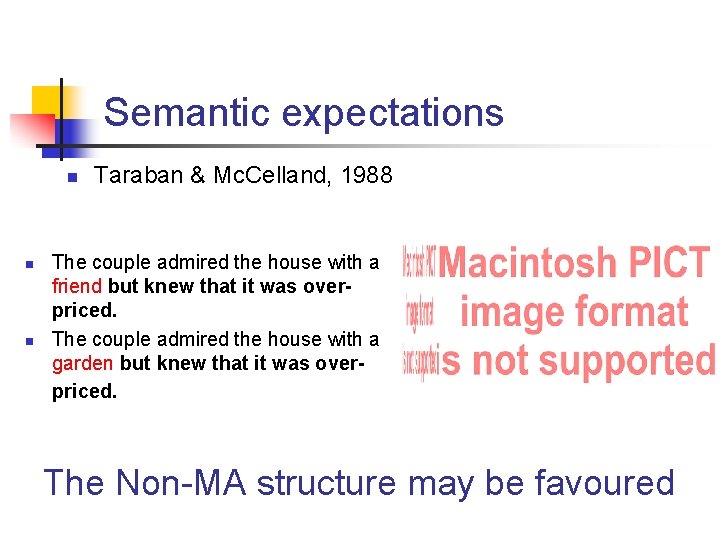
Semantic expectations n n n Taraban & Mc. Celland, 1988 The couple admired the house with a friend but knew that it was overpriced. The couple admired the house with a garden but knew that it was overpriced. The Non-MA structure may be favoured
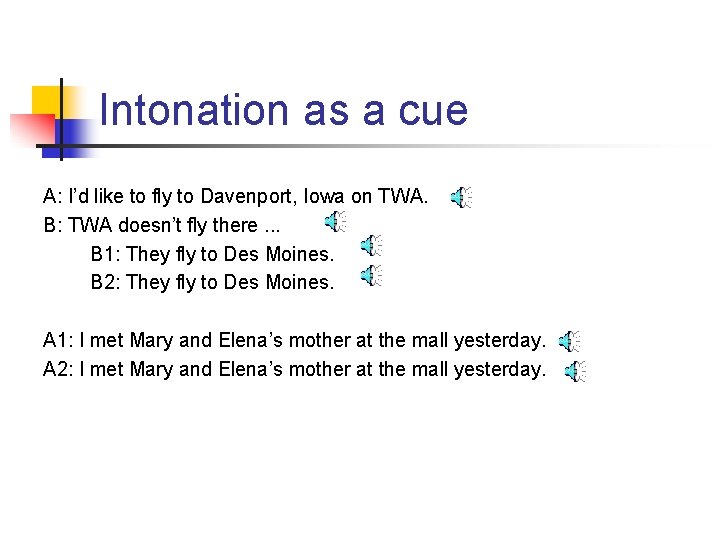
Intonation as a cue A: I’d like to fly to Davenport, Iowa on TWA. B: TWA doesn’t fly there. . . B 1: They fly to Des Moines. B 2: They fly to Des Moines. A 1: I met Mary and Elena’s mother at the mall yesterday. A 2: I met Mary and Elena’s mother at the mall yesterday.
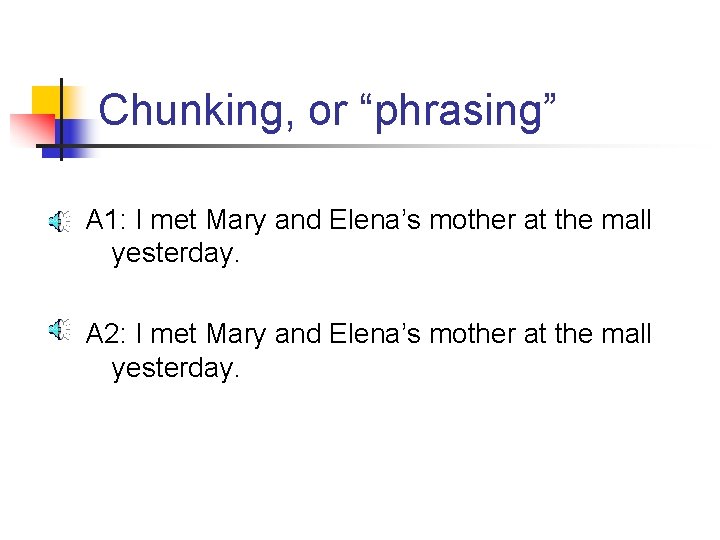
Chunking, or “phrasing” A 1: I met Mary and Elena’s mother at the mall yesterday. A 2: I met Mary and Elena’s mother at the mall yesterday.
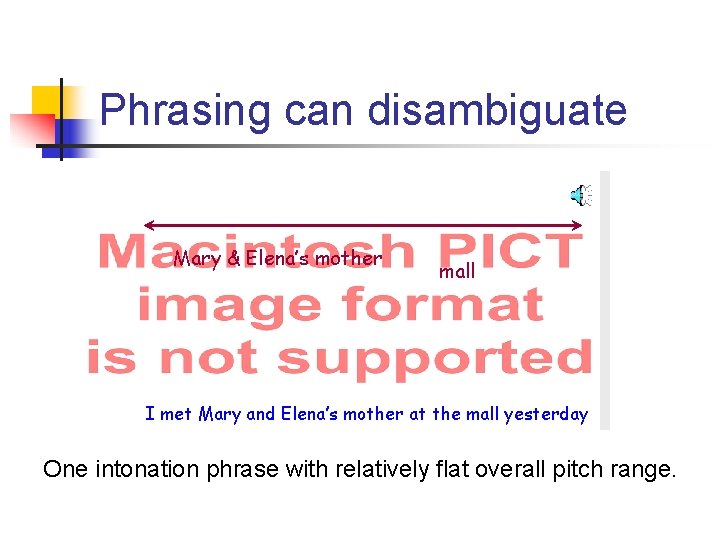
Phrasing can disambiguate Mary & Elena’s mother mall I met Mary and Elena’s mother at the mall yesterday One intonation phrase with relatively flat overall pitch range.
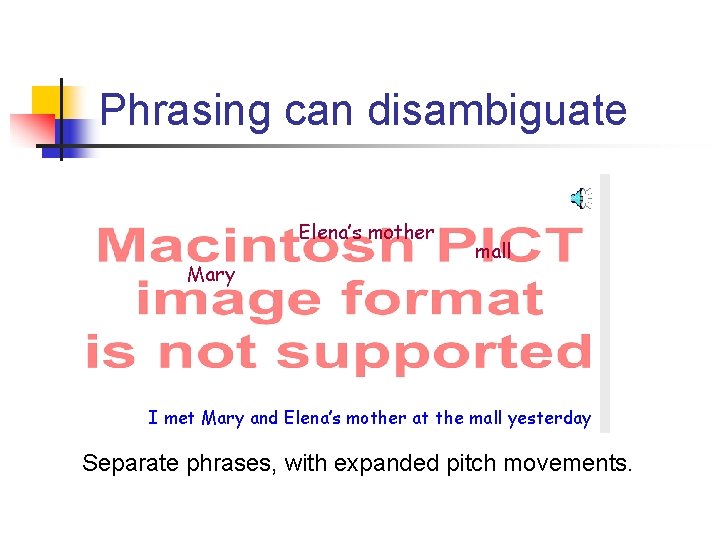
Phrasing can disambiguate Elena’s mother Mary mall I met Mary and Elena’s mother at the mall yesterday Separate phrases, with expanded pitch movements.
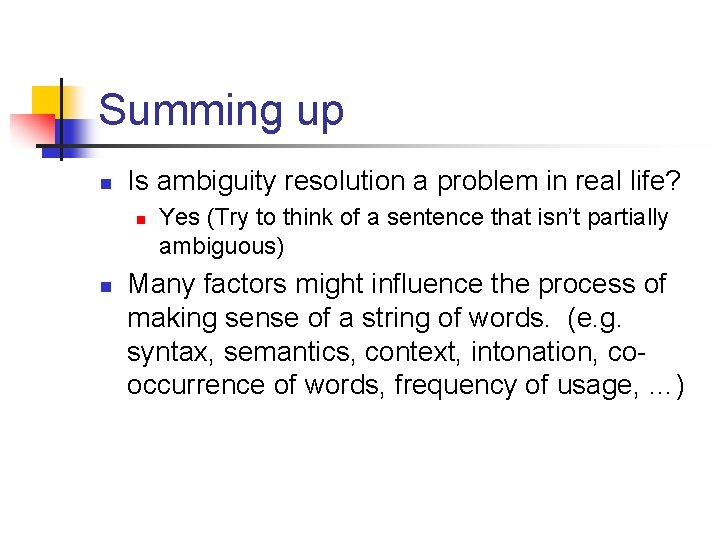
Summing up n Is ambiguity resolution a problem in real life? n n Yes (Try to think of a sentence that isn’t partially ambiguous) Many factors might influence the process of making sense of a string of words. (e. g. syntax, semantics, context, intonation, cooccurrence of words, frequency of usage, …)
Xxxxyyyzzzz
Cratily
Comprehension of words in psycholinguistics
Language loss in psycholinguistics
Short division vs long division
Cs 369
369
Ge power management
Encm 369
Beyond comprehension meaning
369 power
Bio 369
369 project
Tom overbye
369 power
파이썬 369 짝짝
369 power
Ece 369
Euro psy
Ucf io psychology
Psy2055
Psy 2055
Xxxxblue
Psy
Psy sanin
Psy
Psy 421
Phonological loop
Psy
Psy 226
Suppose twin teenagers are vying
Psy academy
Damasio
Psy walsh
Opis mačky po anglicky
Vzor gazdina
11 psy
Regression psy
Psy
What is adoloscence
Topic sentence and supporting sentence examples
Incomplete sentences