New Techniques and Technologies for Statistics NTTS 2017
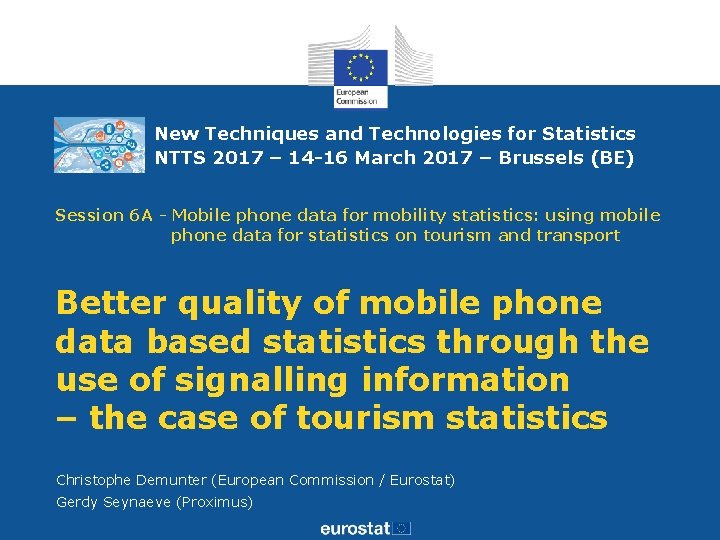
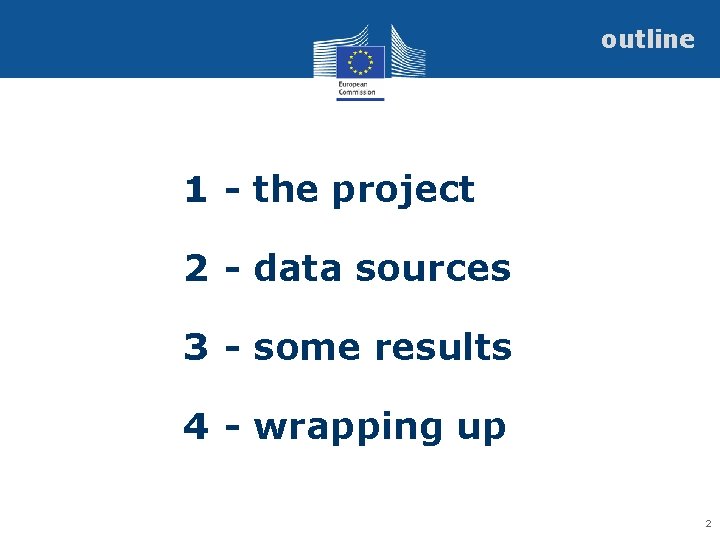
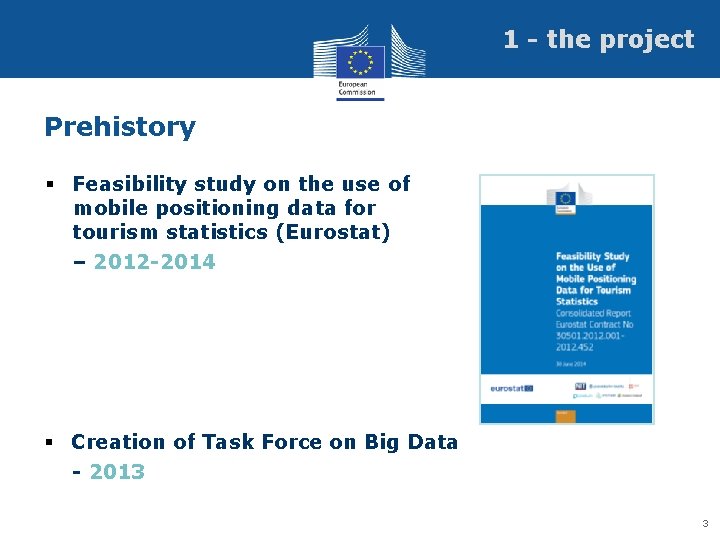
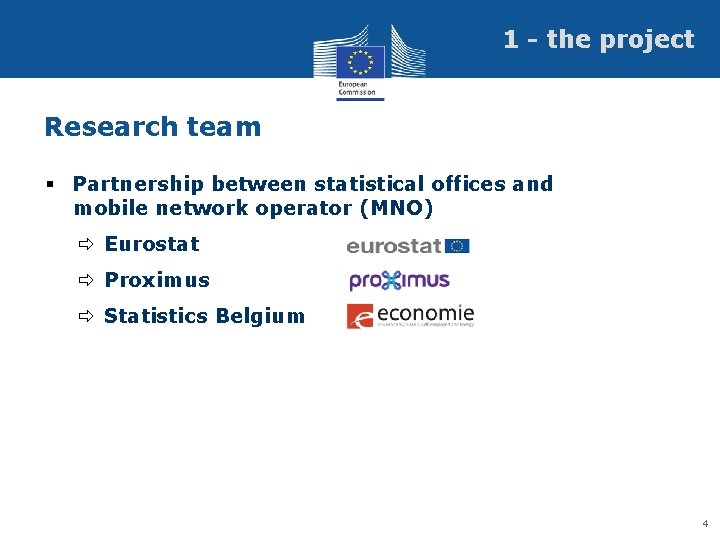
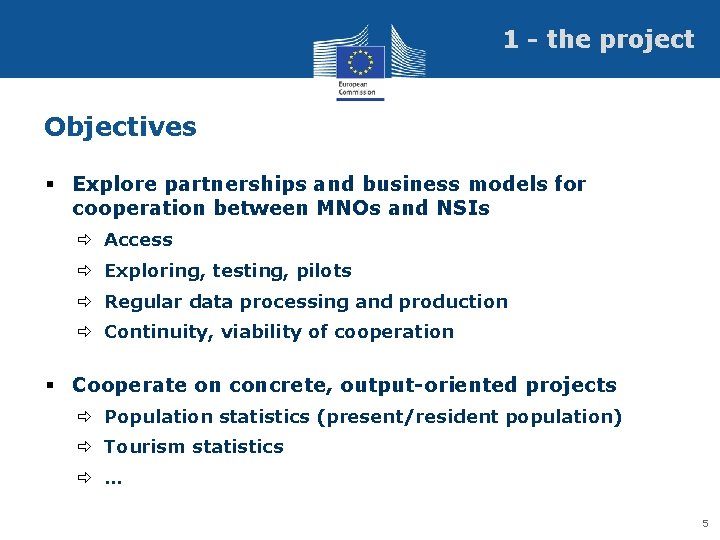
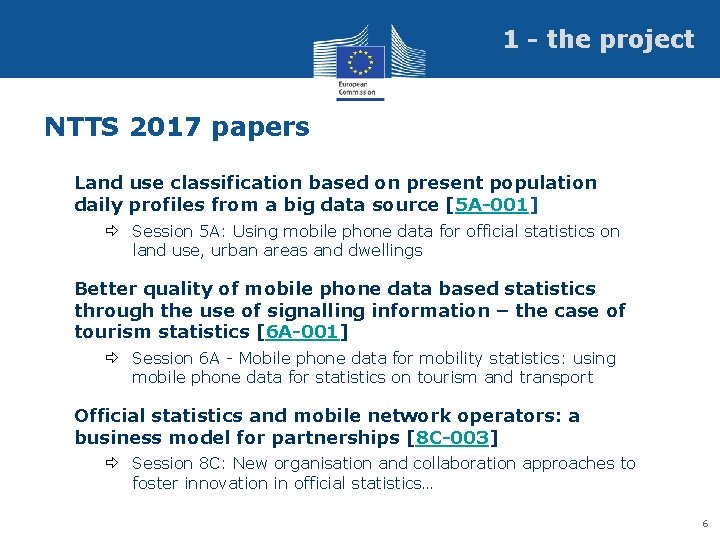
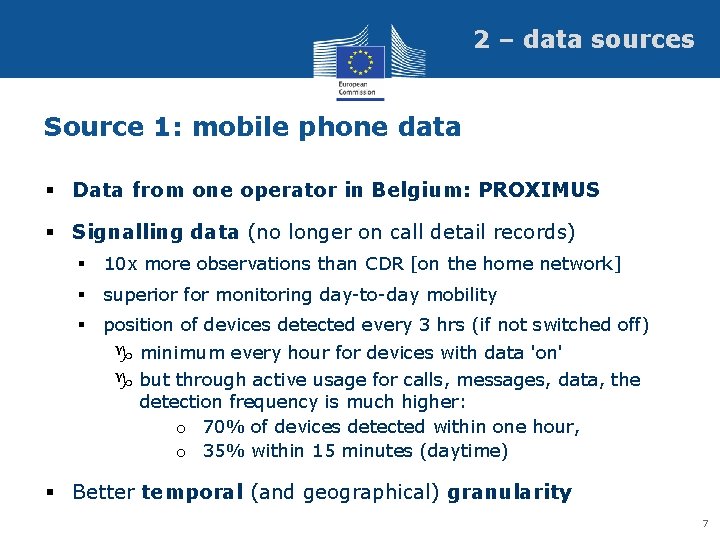
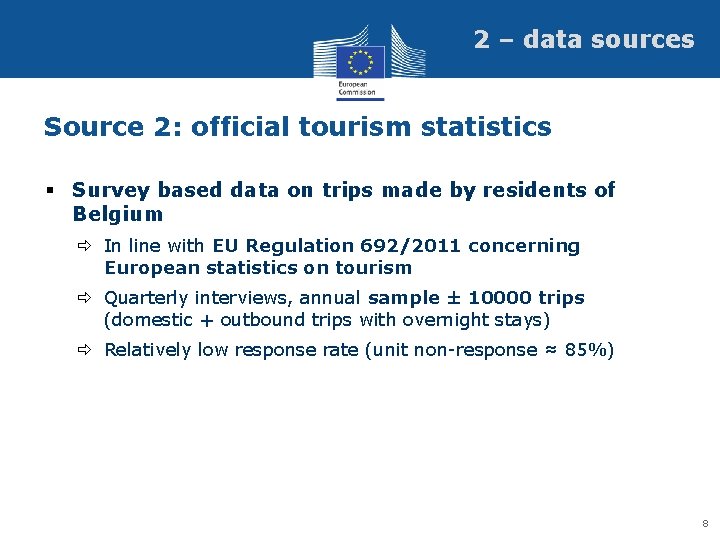
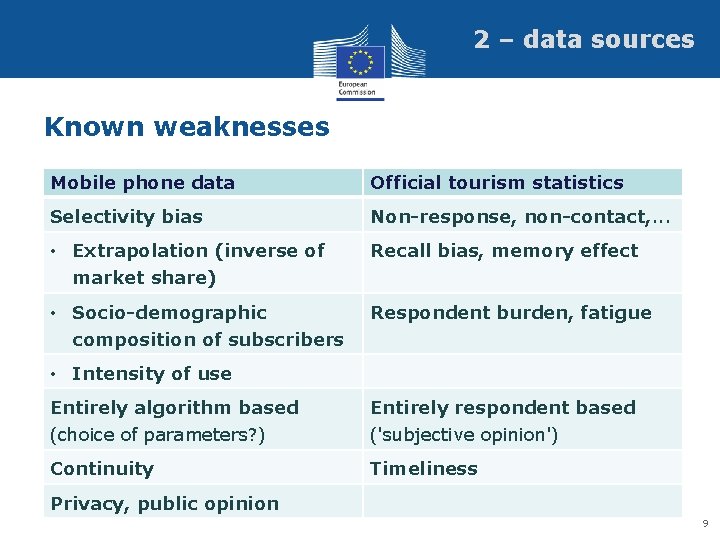
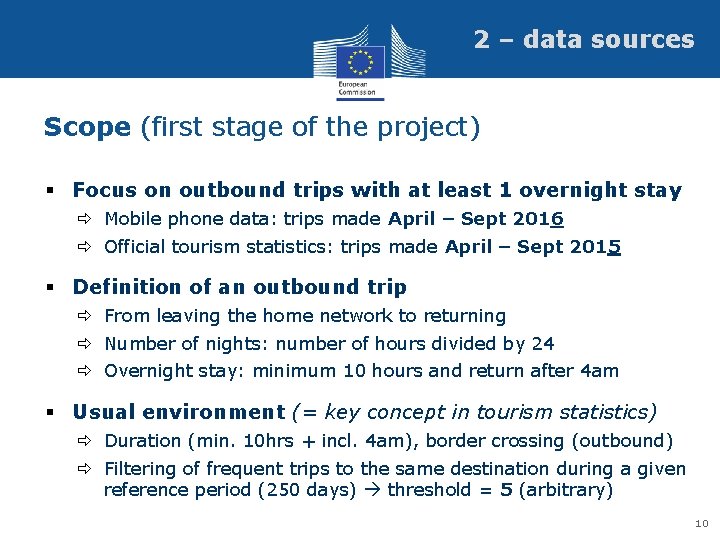
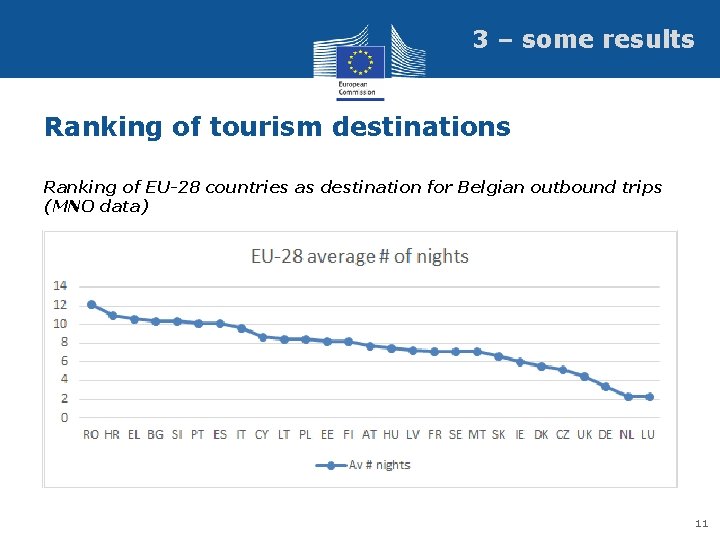
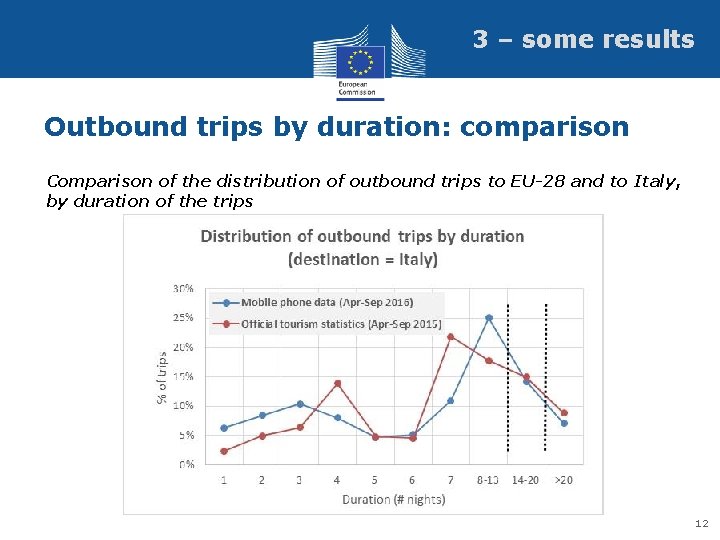
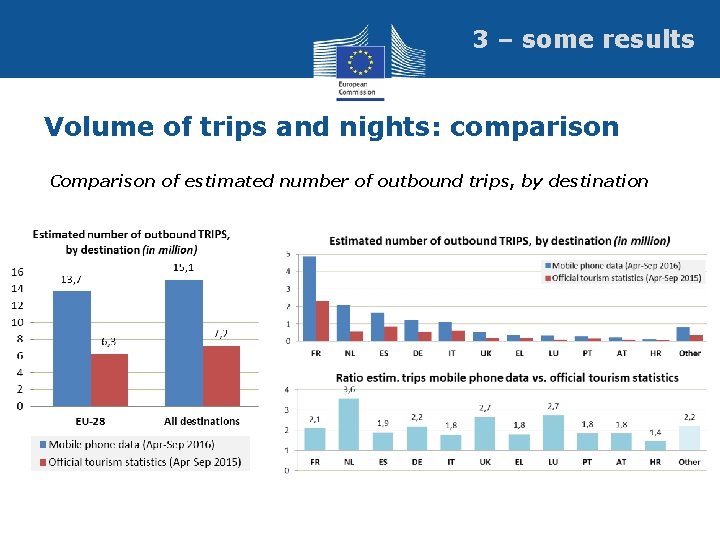
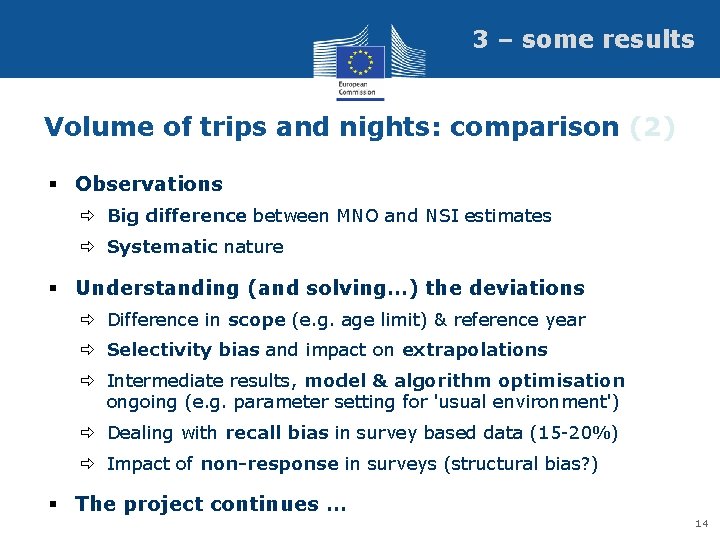
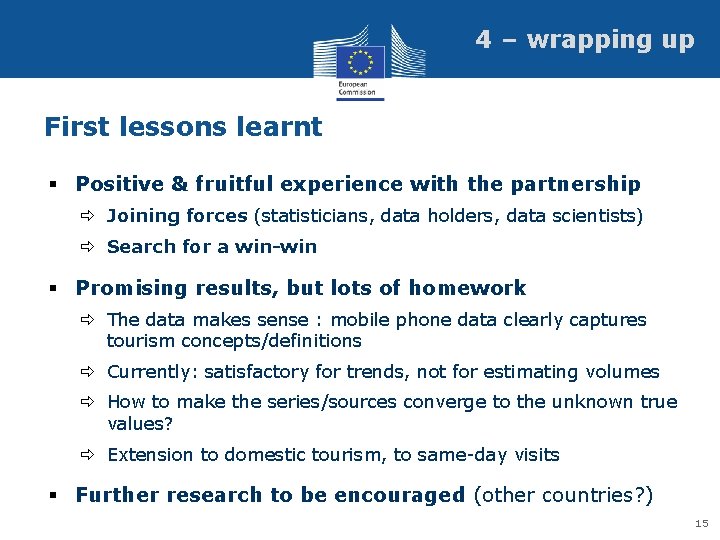
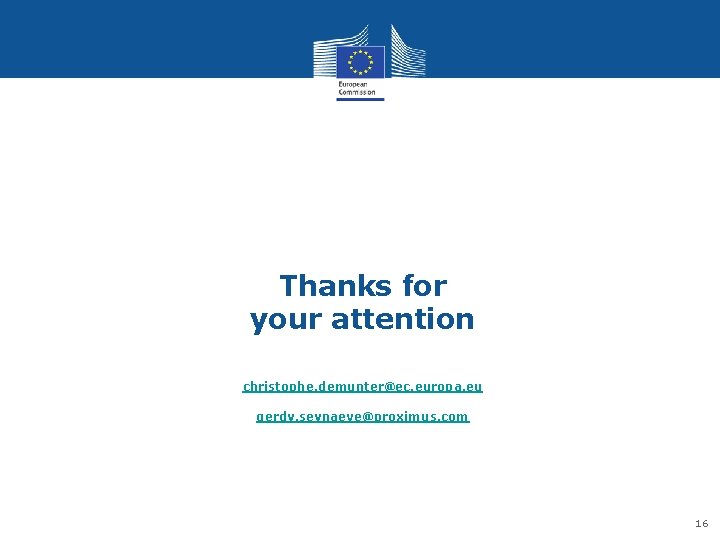
- Slides: 16
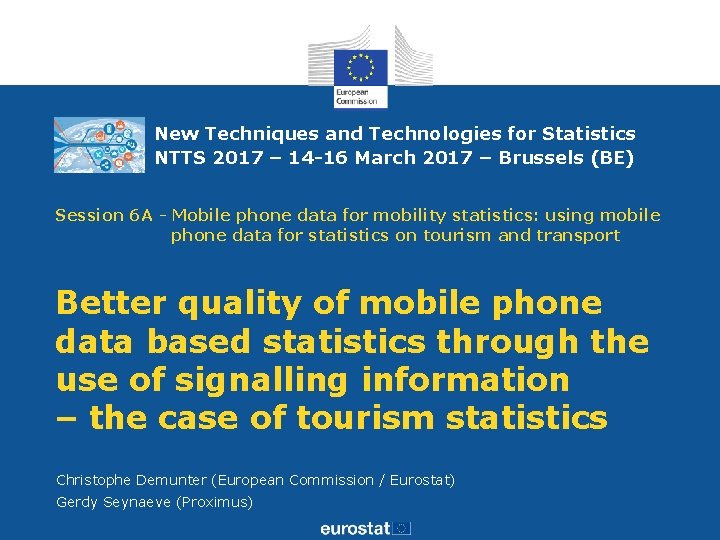
New Techniques and Technologies for Statistics NTTS 2017 – 14 -16 March 2017 – Brussels (BE) Session 6 A - Mobile phone data for mobility statistics: using mobile phone data for statistics on tourism and transport Better quality of mobile phone data based statistics through the use of signalling information – the case of tourism statistics Christophe Demunter (European Commission / Eurostat) Gerdy Seynaeve (Proximus)
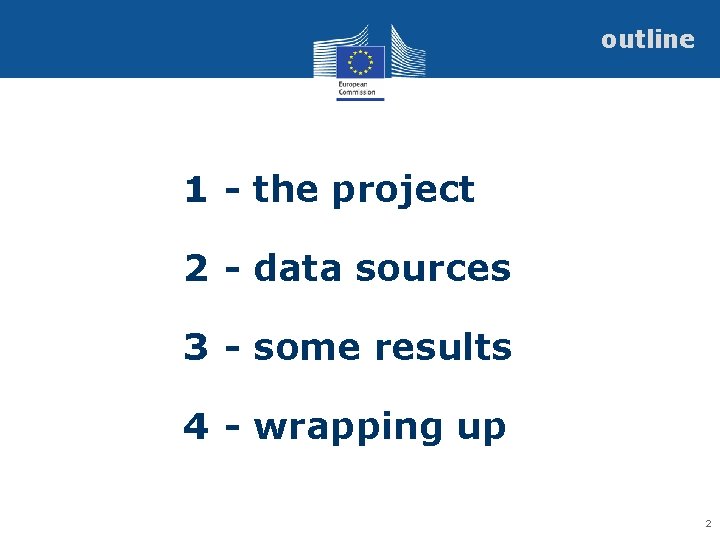
outline 1 - the project 2 - data sources 3 - some results 4 - wrapping up 2
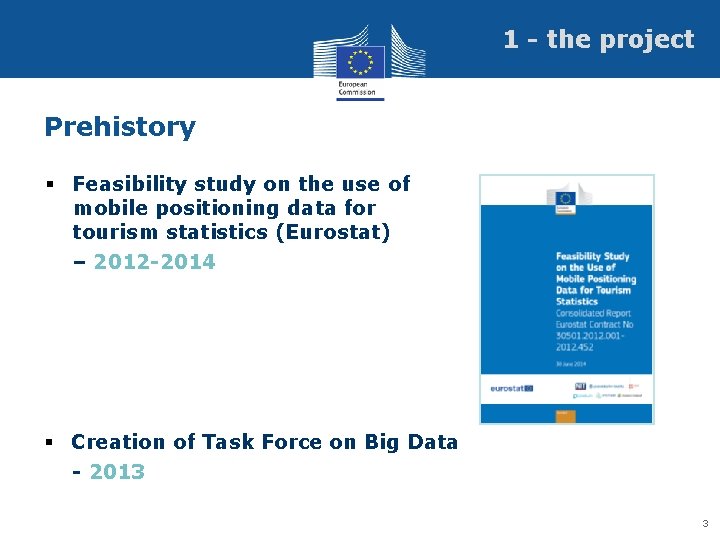
1 - the project Prehistory § Feasibility study on the use of mobile positioning data for tourism statistics (Eurostat) – 2012 -2014 § Creation of Task Force on Big Data - 2013 3
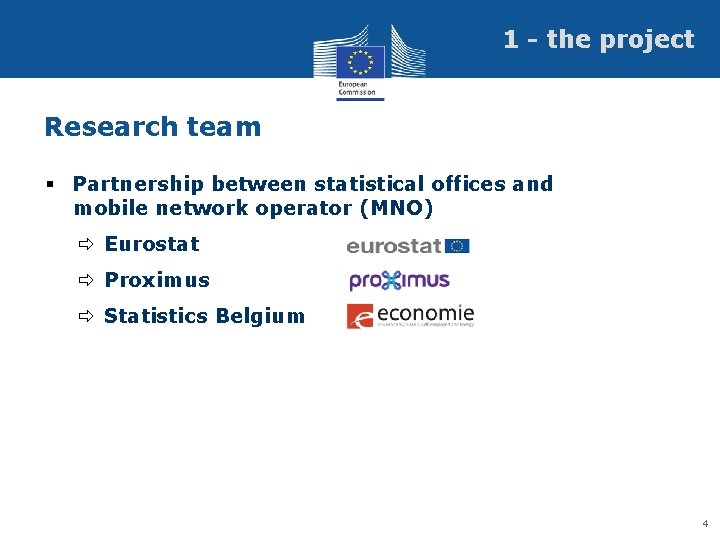
1 - the project Research team § Partnership between statistical offices and mobile network operator (MNO) ð Eurostat ð Proximus ð Statistics Belgium 4
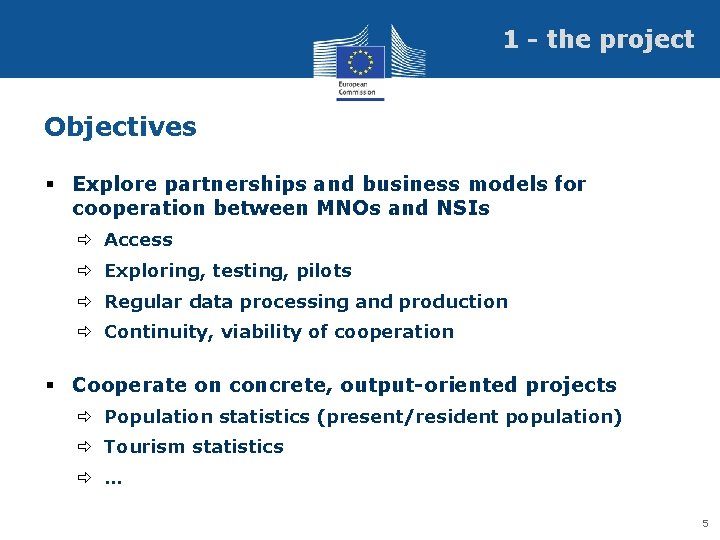
1 - the project Objectives § Explore partnerships and business models for cooperation between MNOs and NSIs ð Access ð Exploring, testing, pilots ð Regular data processing and production ð Continuity, viability of cooperation § Cooperate on concrete, output-oriented projects ð Population statistics (present/resident population) ð Tourism statistics ð … 5
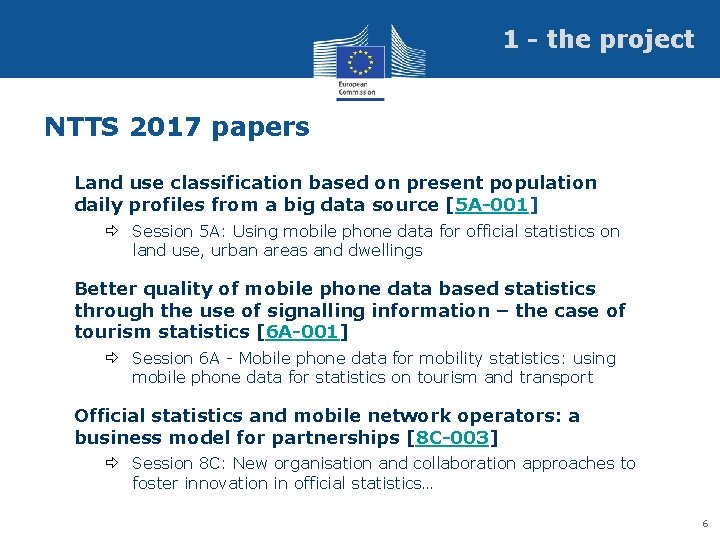
1 - the project NTTS 2017 papers Land use classification based on present population daily profiles from a big data source [5 A-001] ð Session 5 A: Using mobile phone data for official statistics on land use, urban areas and dwellings Better quality of mobile phone data based statistics through the use of signalling information – the case of tourism statistics [6 A-001] ð Session 6 A - Mobile phone data for mobility statistics: using mobile phone data for statistics on tourism and transport Official statistics and mobile network operators: a business model for partnerships [8 C-003] ð Session 8 C: New organisation and collaboration approaches to foster innovation in official statistics… 6
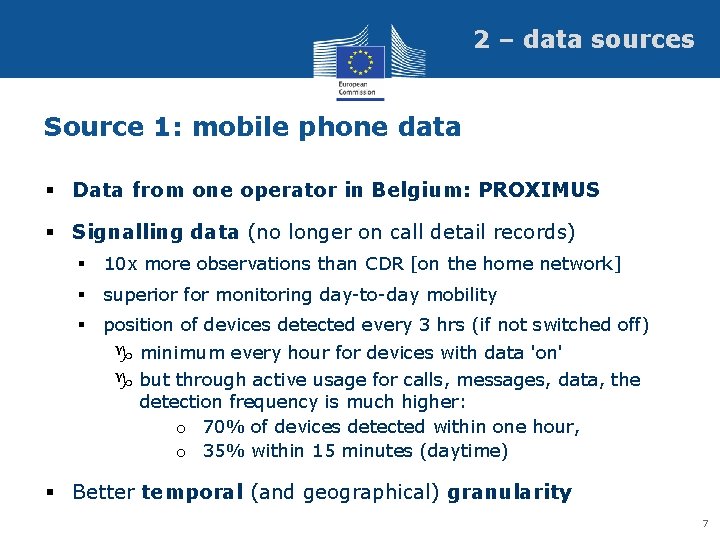
2 – data sources Source 1: mobile phone data § Data from one operator in Belgium: PROXIMUS § Signalling data (no longer on call detail records) § 10 x more observations than CDR [on the home network] § superior for monitoring day-to-day mobility § position of devices detected every 3 hrs (if not switched off) g minimum every hour for devices with data 'on' g but through active usage for calls, messages, data, the detection frequency is much higher: o 70% of devices detected within one hour, o 35% within 15 minutes (daytime) § Better temporal (and geographical) granularity 7
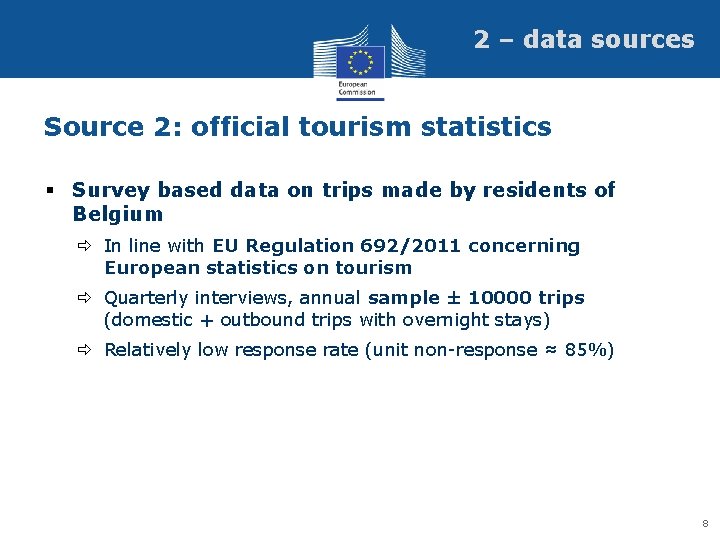
2 – data sources Source 2: official tourism statistics § Survey based data on trips made by residents of Belgium ð In line with EU Regulation 692/2011 concerning European statistics on tourism ð Quarterly interviews, annual sample ± 10000 trips (domestic + outbound trips with overnight stays) ð Relatively low response rate (unit non-response ≈ 85%) 8
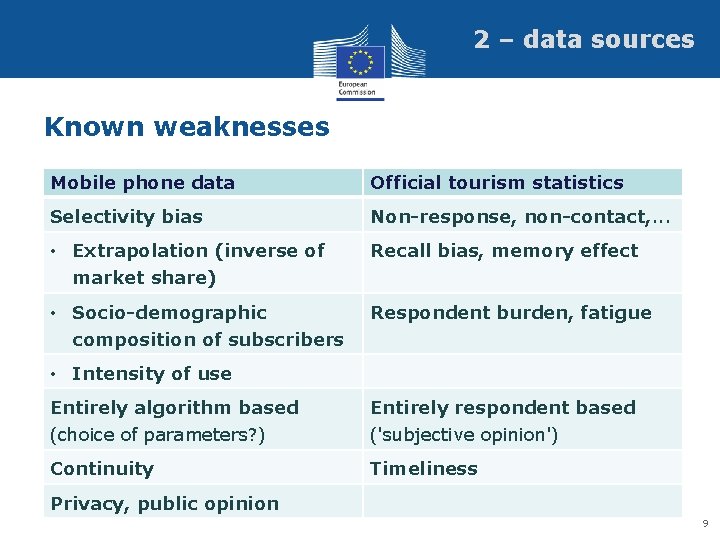
2 – data sources Known weaknesses Mobile phone data Official tourism statistics Selectivity bias Non-response, non-contact, . . . • Extrapolation (inverse of market share) Recall bias, memory effect • Socio-demographic composition of subscribers Respondent burden, fatigue • Intensity of use Entirely algorithm based (choice of parameters? ) Entirely respondent based ('subjective opinion') Continuity Timeliness Privacy, public opinion 9
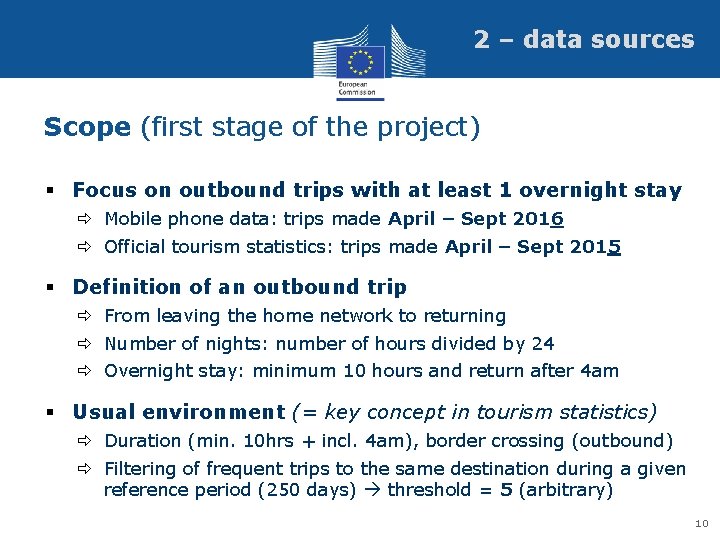
2 – data sources Scope (first stage of the project) § Focus on outbound trips with at least 1 overnight stay ð Mobile phone data: trips made April – Sept 2016 ð Official tourism statistics: trips made April – Sept 2015 § Definition of an outbound trip ð From leaving the home network to returning ð Number of nights: number of hours divided by 24 ð Overnight stay: minimum 10 hours and return after 4 am § Usual environment (= key concept in tourism statistics) ð Duration (min. 10 hrs + incl. 4 am), border crossing (outbound) ð Filtering of frequent trips to the same destination during a given reference period (250 days) threshold = 5 (arbitrary) 10
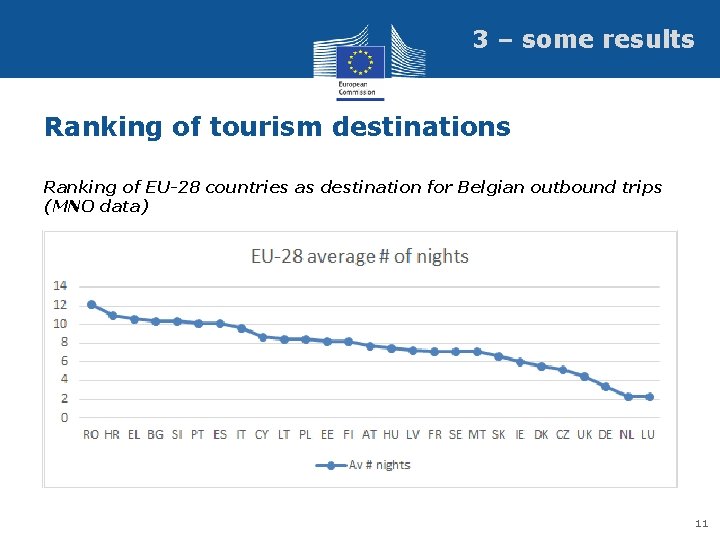
3 – some results Ranking of tourism destinations Ranking of EU-28 countries as destination for Belgian outbound trips (MNO data) 11
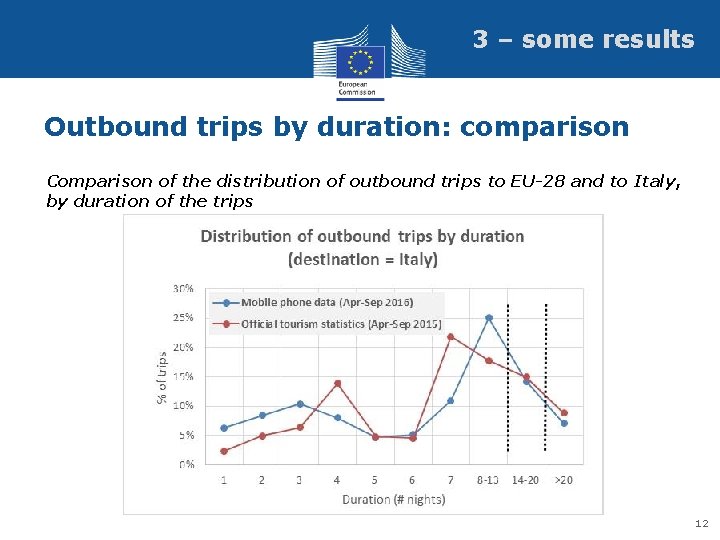
3 – some results Outbound trips by duration: comparison Comparison of the distribution of outbound trips to EU-28 and to Italy, by duration of the trips 12
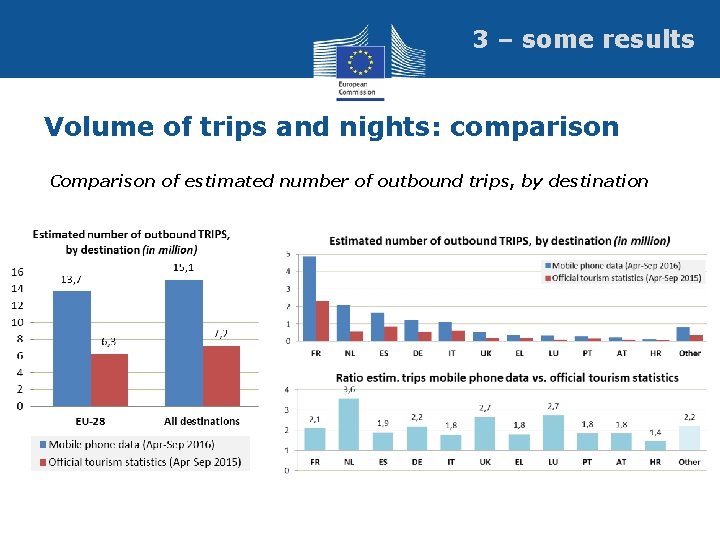
3 – some results Volume of trips and nights: comparison Comparison of estimated number of outbound trips, by destination
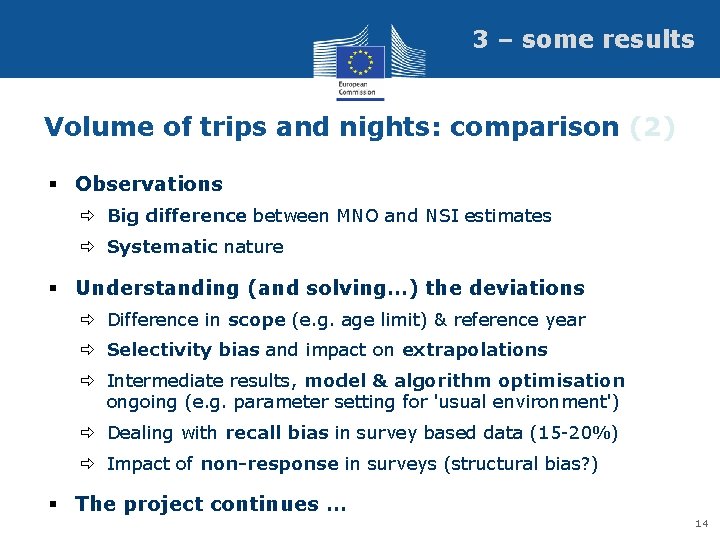
3 – some results Volume of trips and nights: comparison (2) § Observations ð Big difference between MNO and NSI estimates ð Systematic nature § Understanding (and solving…) the deviations ð Difference in scope (e. g. age limit) & reference year ð Selectivity bias and impact on extrapolations ð Intermediate results, model & algorithm optimisation ongoing (e. g. parameter setting for 'usual environment') ð Dealing with recall bias in survey based data (15 -20%) ð Impact of non-response in surveys (structural bias? ) § The project continues … 14
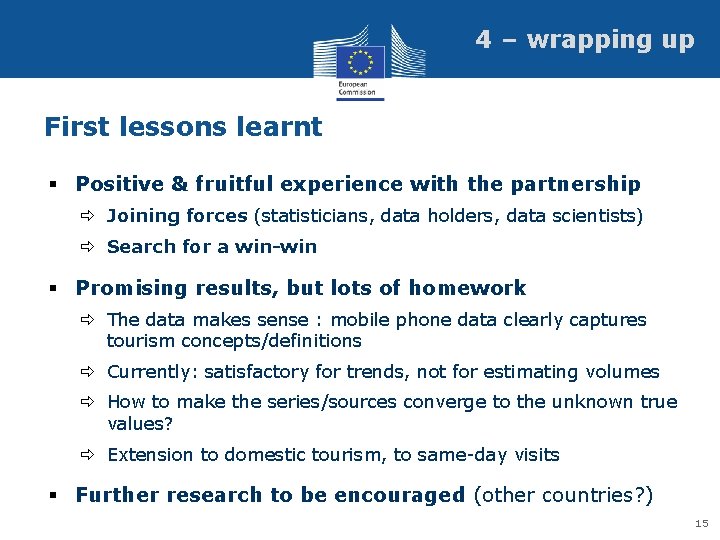
4 – wrapping up First lessons learnt § Positive & fruitful experience with the partnership ð Joining forces (statisticians, data holders, data scientists) ð Search for a win-win § Promising results, but lots of homework ð The data makes sense : mobile phone data clearly captures tourism concepts/definitions ð Currently: satisfactory for trends, not for estimating volumes ð How to make the series/sources converge to the unknown true values? ð Extension to domestic tourism, to same-day visits § Further research to be encouraged (other countries? ) 15
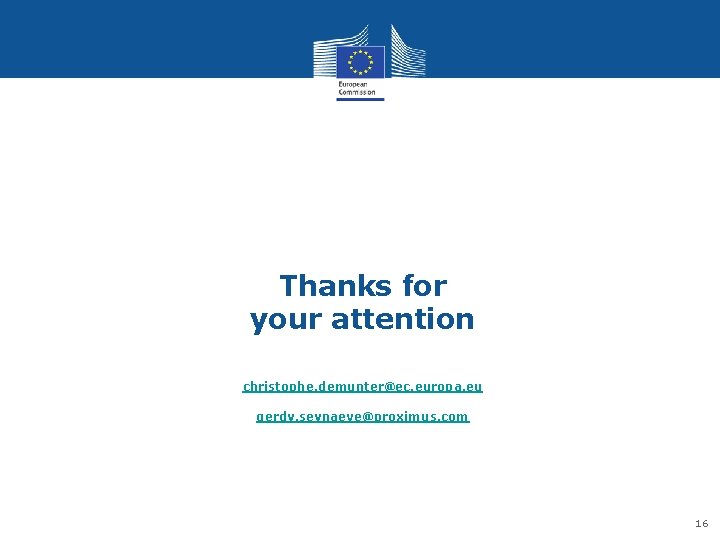
Thanks for your attention christophe. demunter@ec. europa. eu gerdy. seynaeve@proximus. com 16
Ntts cost
Ntts conference 2021
Ntts conference
Immigration and urbanization new technologies lesson 4
Introduction to statistics what is statistics
Name-calling examples
Linearly independen
New disruptive technologies 2021
New disruptive technologies
New casino october 2017
Fonction technique scooter
Kontinuitetshantering i praktiken
Typiska novell drag
Nationell inriktning för artificiell intelligens
Returpilarna
Varför kallas perioden 1918-1939 för mellankrigstiden?
En lathund för arbete med kontinuitetshantering