MODELLING INTERACTOMES RAM SAMUDRALA ASSOCIATE PROFESSOR UNIVERSITY OF
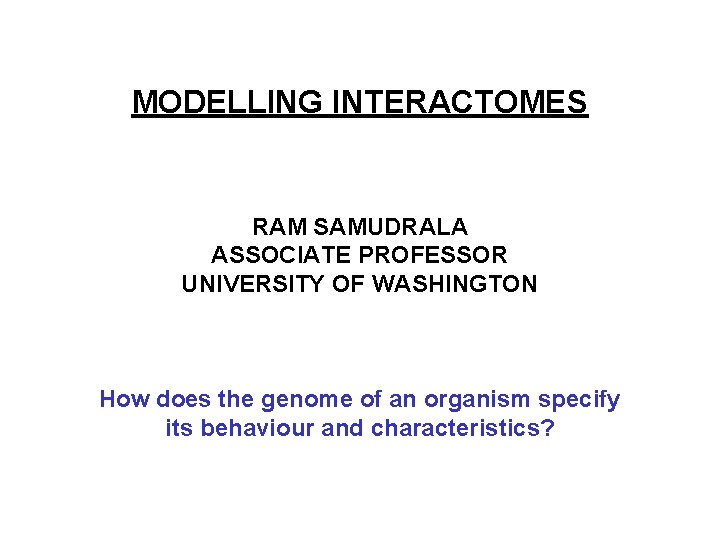
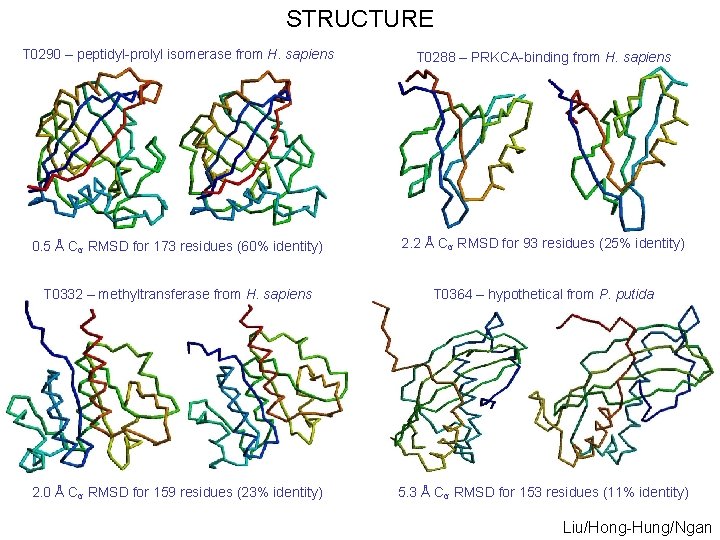
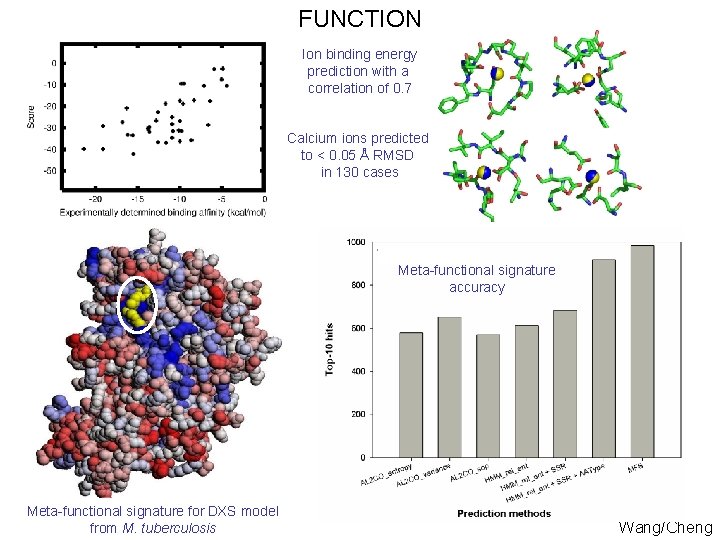
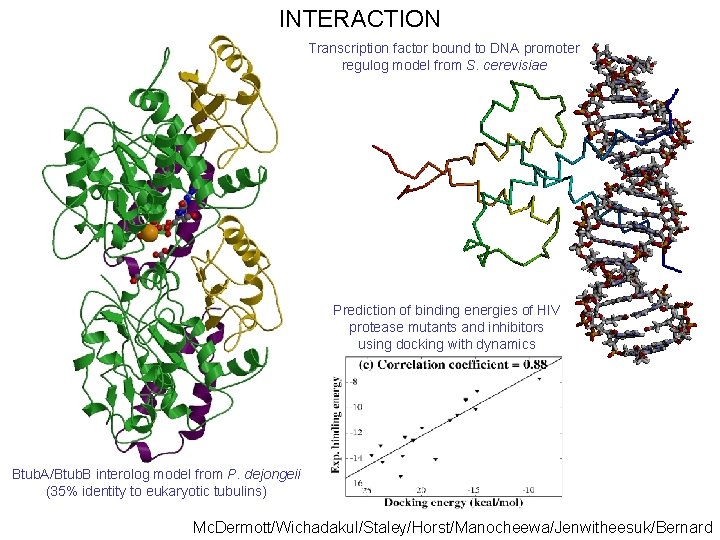
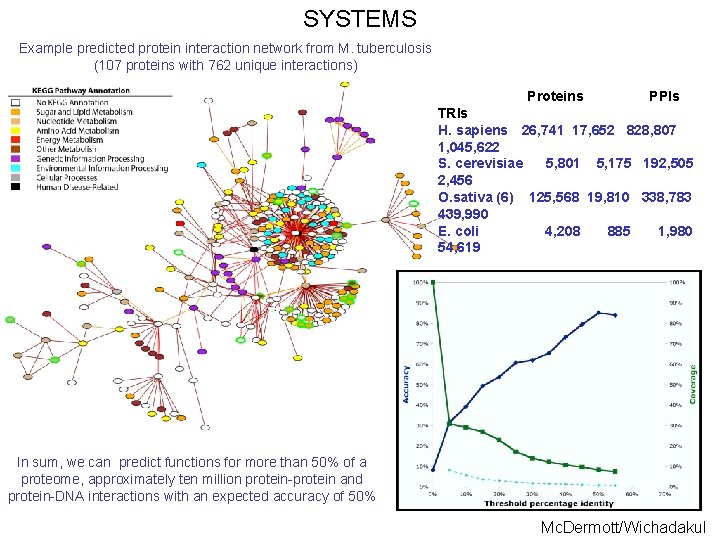
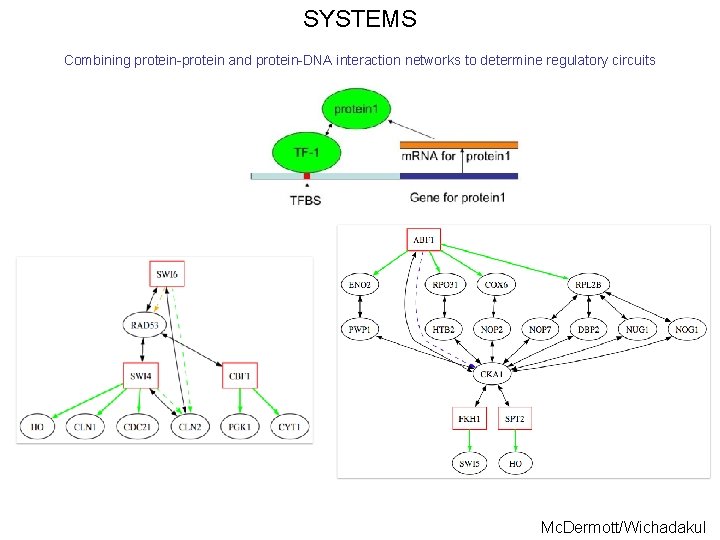
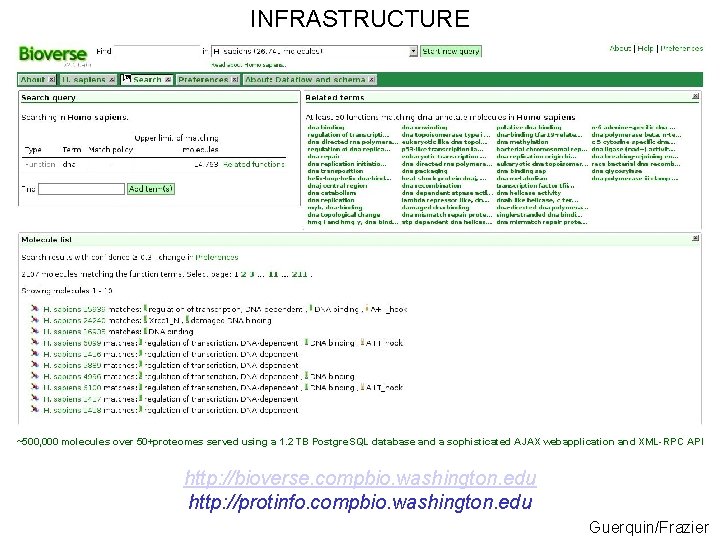
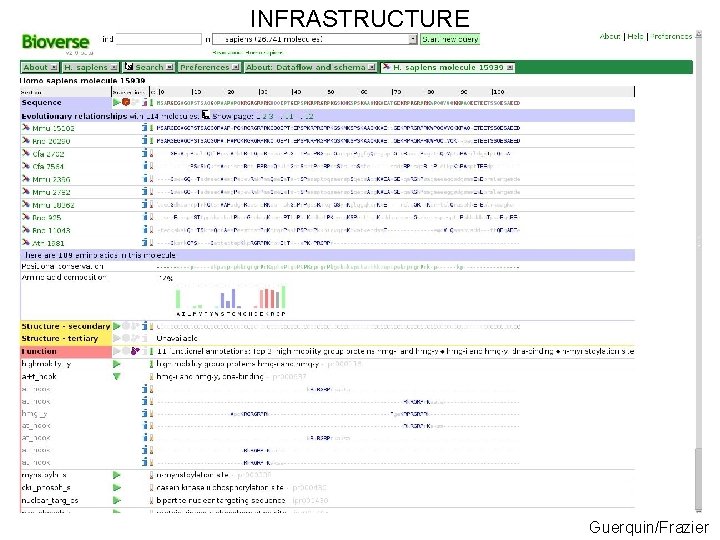
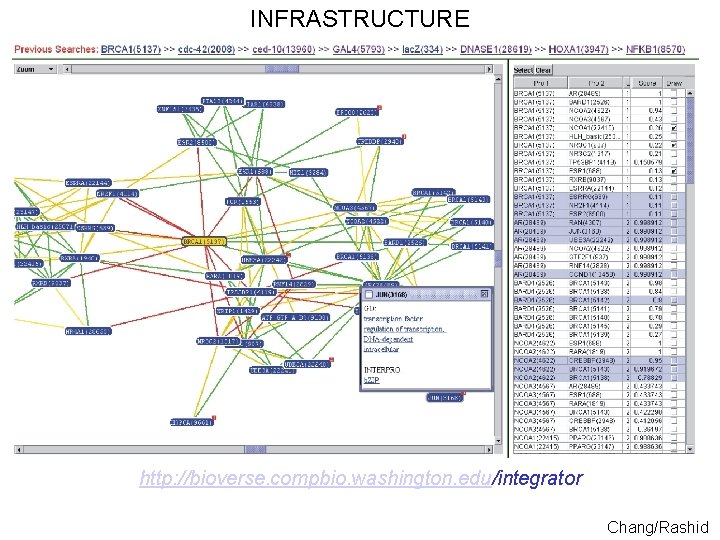
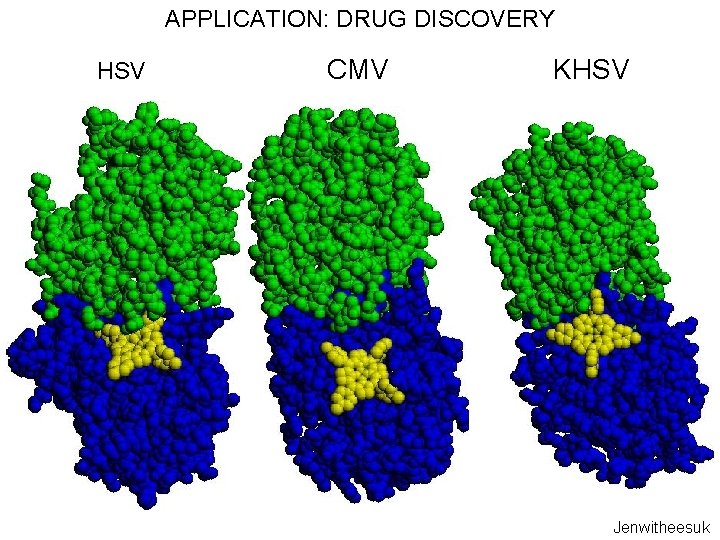
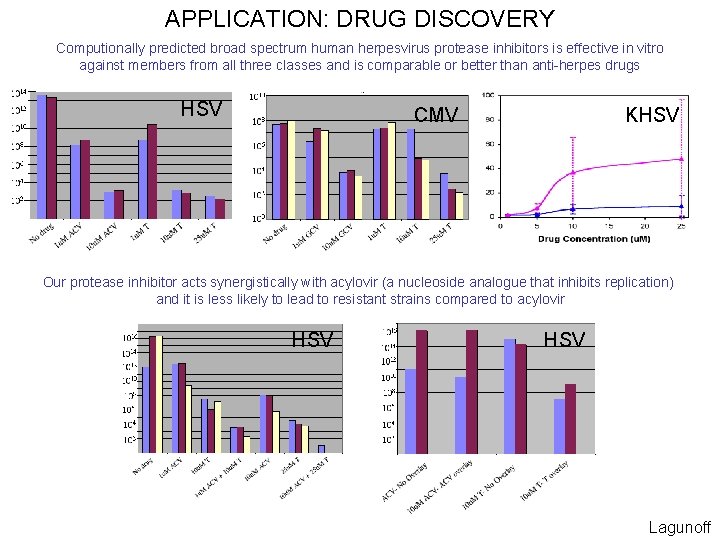
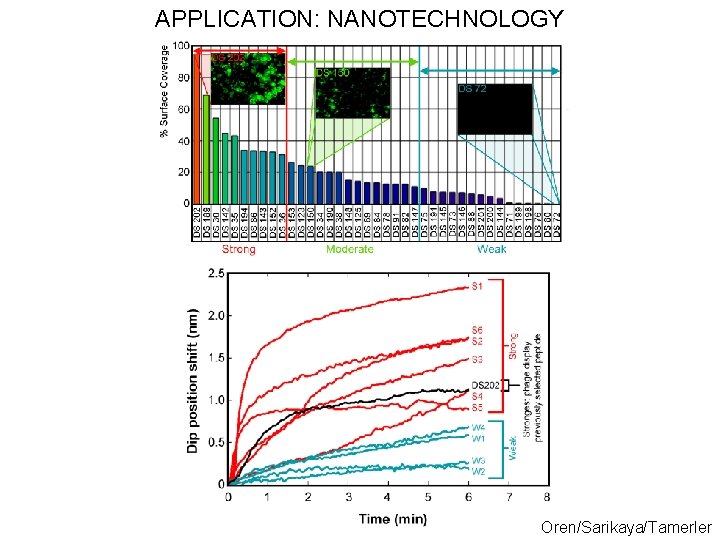
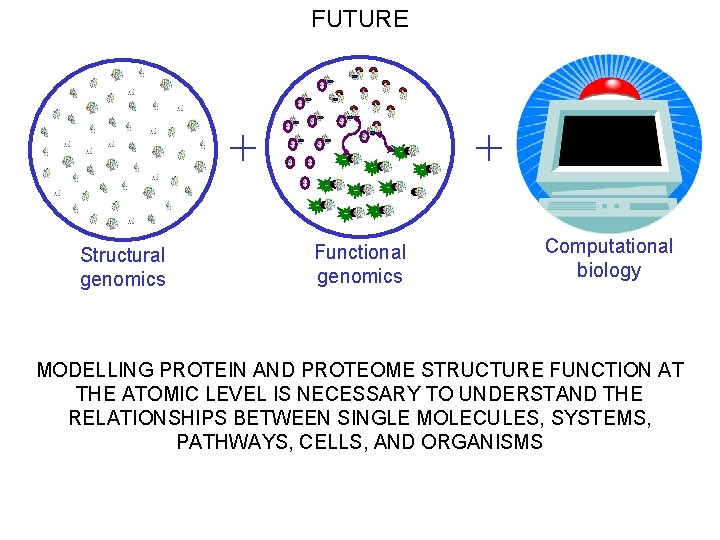
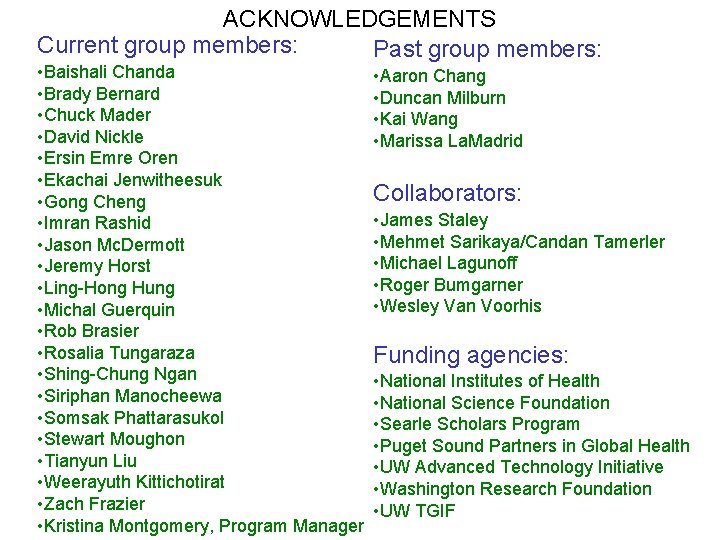
- Slides: 14
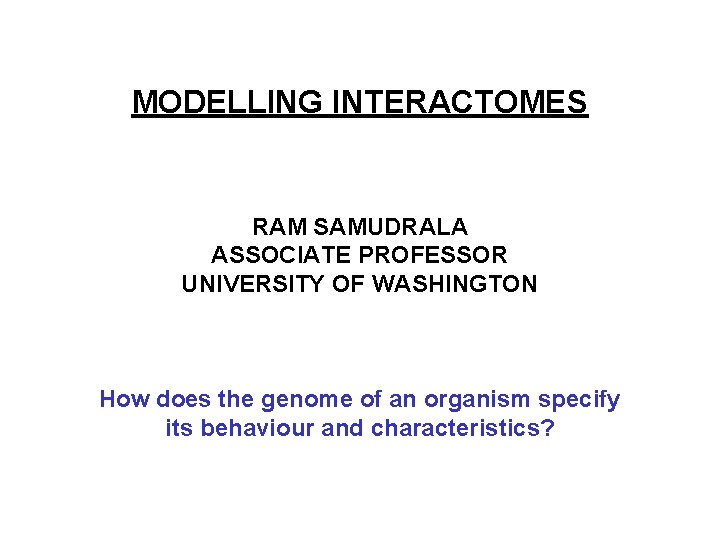
MODELLING INTERACTOMES RAM SAMUDRALA ASSOCIATE PROFESSOR UNIVERSITY OF WASHINGTON How does the genome of an organism specify its behaviour and characteristics?
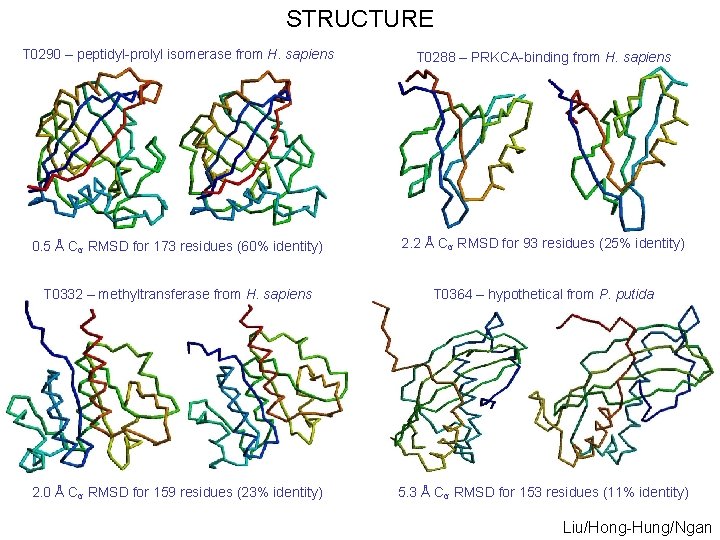
STRUCTURE T 0290 – peptidyl-prolyl isomerase from H. sapiens T 0288 – PRKCA-binding from H. sapiens 0. 5 Å Cα RMSD for 173 residues (60% identity) 2. 2 Å Cα RMSD for 93 residues (25% identity) T 0332 – methyltransferase from H. sapiens T 0364 – hypothetical from P. putida 2. 0 Å Cα RMSD for 159 residues (23% identity) 5. 3 Å Cα RMSD for 153 residues (11% identity) Liu/Hong-Hung/Ngan
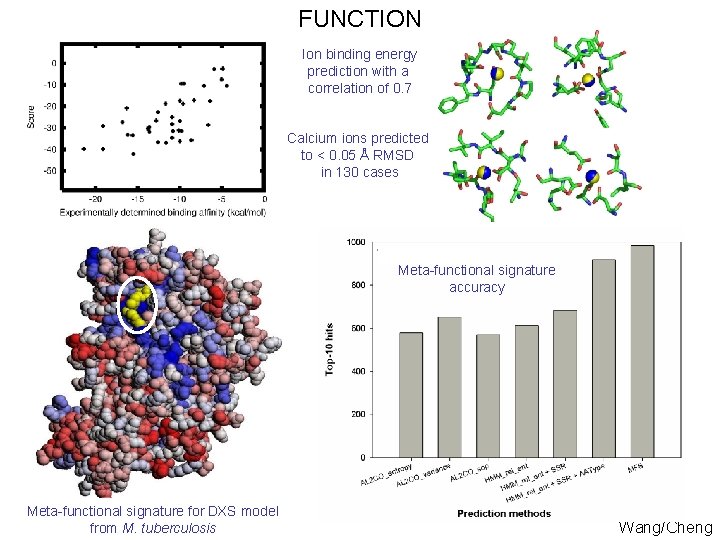
FUNCTION Ion binding energy prediction with a correlation of 0. 7 Calcium ions predicted to < 0. 05 Å RMSD in 130 cases Meta-functional signature accuracy Meta-functional signature for DXS model from M. tuberculosis Wang/Cheng
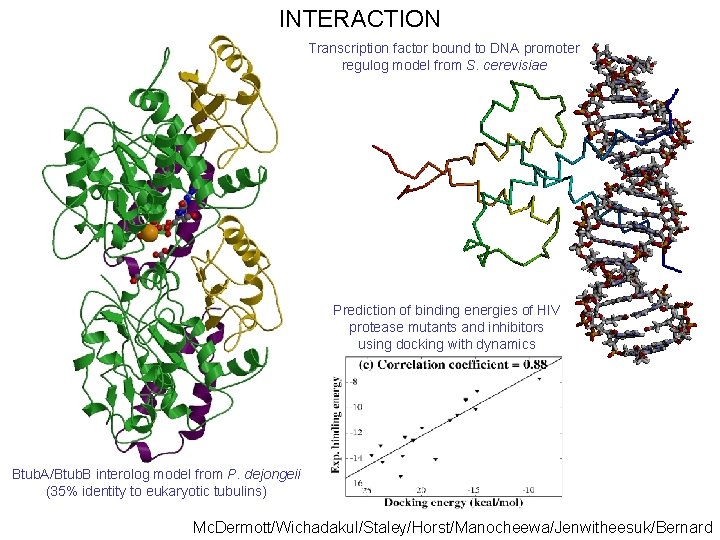
INTERACTION Transcription factor bound to DNA promoter regulog model from S. cerevisiae Prediction of binding energies of HIV protease mutants and inhibitors using docking with dynamics Btub. A/Btub. B interolog model from P. dejongeii (35% identity to eukaryotic tubulins) Mc. Dermott/Wichadakul/Staley/Horst/Manocheewa/Jenwitheesuk/Bernard
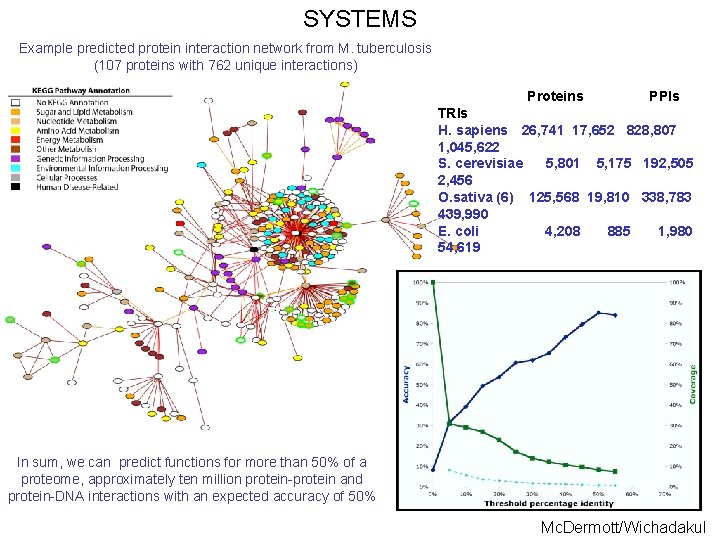
SYSTEMS Example predicted protein interaction network from M. tuberculosis (107 proteins with 762 unique interactions) Proteins PPIs TRIs H. sapiens 26, 741 17, 652 828, 807 1, 045, 622 S. cerevisiae 5, 801 5, 175 192, 505 2, 456 O. sativa (6) 125, 568 19, 810 338, 783 439, 990 E. coli 4, 208 885 1, 980 54, 619 In sum, we can predict functions for more than 50% of a proteome, approximately ten million protein-protein and protein-DNA interactions with an expected accuracy of 50% Mc. Dermott/Wichadakul
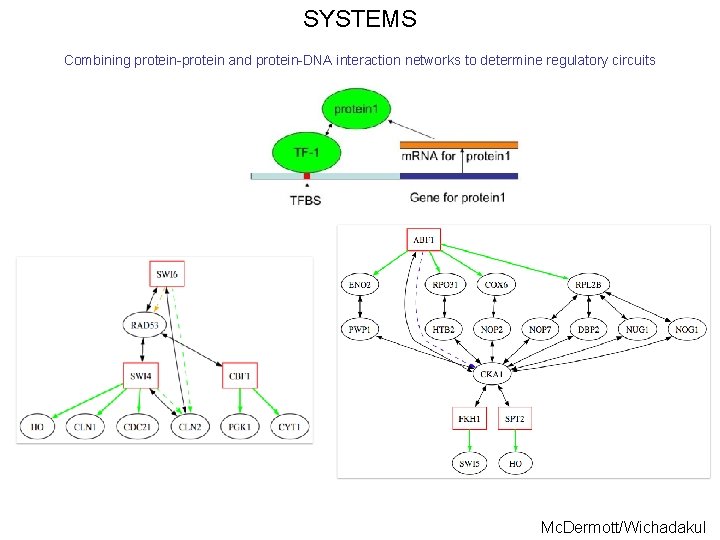
SYSTEMS Combining protein-protein and protein-DNA interaction networks to determine regulatory circuits Mc. Dermott/Wichadakul
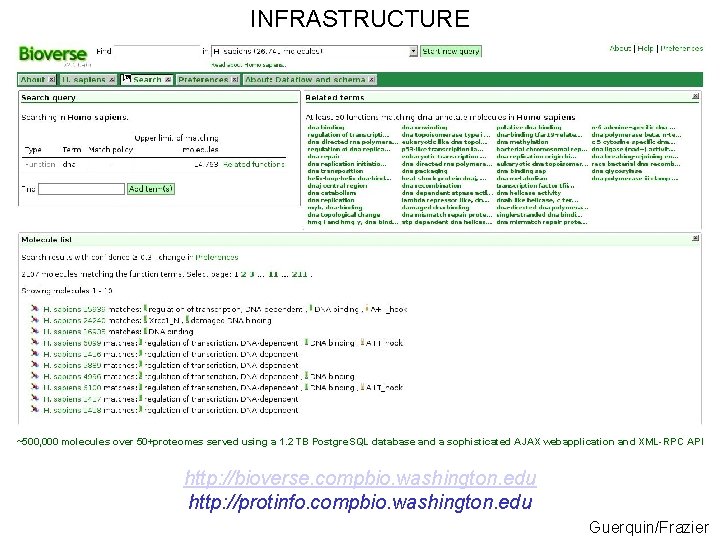
INFRASTRUCTURE ~500, 000 molecules over 50+proteomes served using a 1. 2 TB Postgre. SQL database and a sophisticated AJAX webapplication and XML-RPC API http: //bioverse. compbio. washington. edu http: //protinfo. compbio. washington. edu Guerquin/Frazier
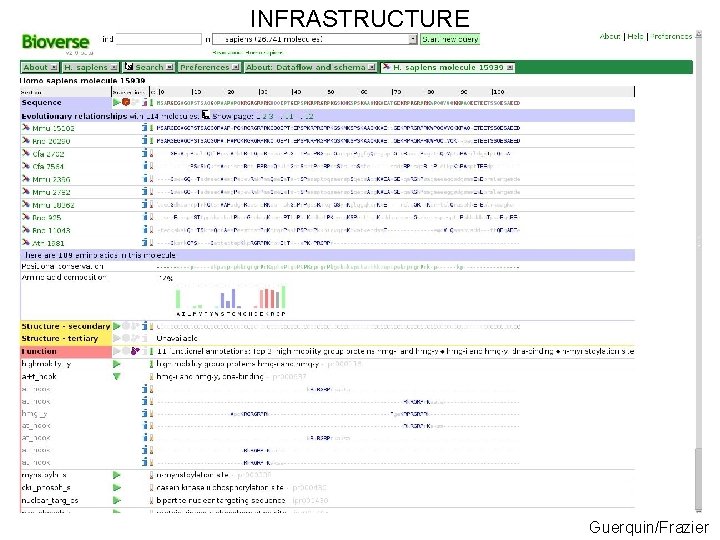
INFRASTRUCTURE Guerquin/Frazier
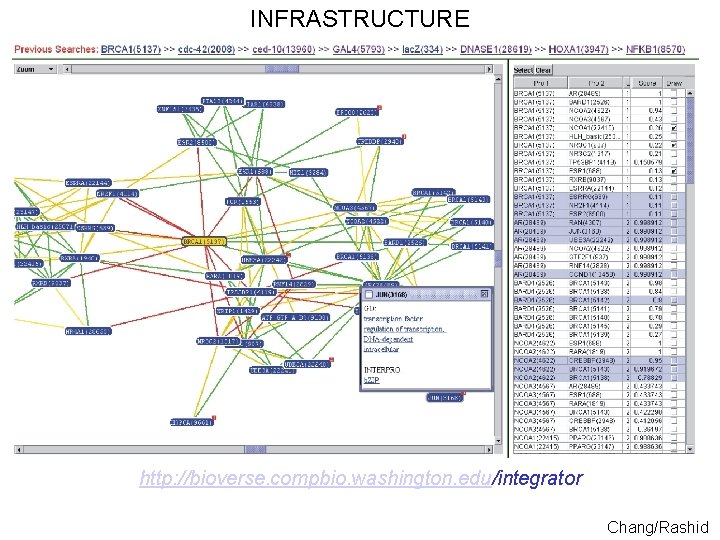
INFRASTRUCTURE http: //bioverse. compbio. washington. edu/integrator Chang/Rashid
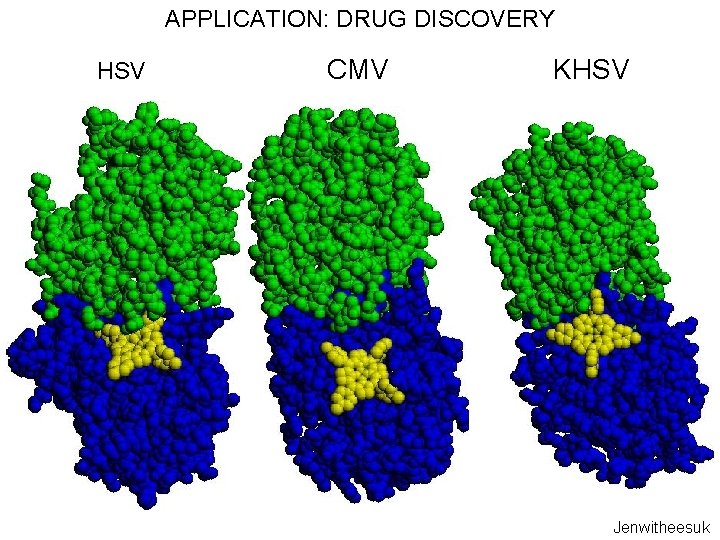
APPLICATION: DRUG DISCOVERY HSV CMV KHSV Jenwitheesuk
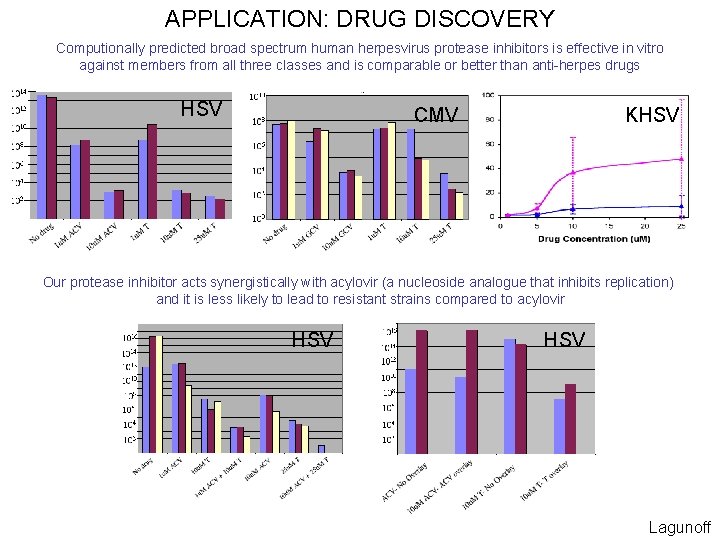
APPLICATION: DRUG DISCOVERY Computionally predicted broad spectrum human herpesvirus protease inhibitors is effective in vitro against members from all three classes and is comparable or better than anti-herpes drugs HSV KHSV CMV Our protease inhibitor acts synergistically with acylovir (a nucleoside analogue that inhibits replication) and it is less likely to lead to resistant strains compared to acylovir HSV Lagunoff
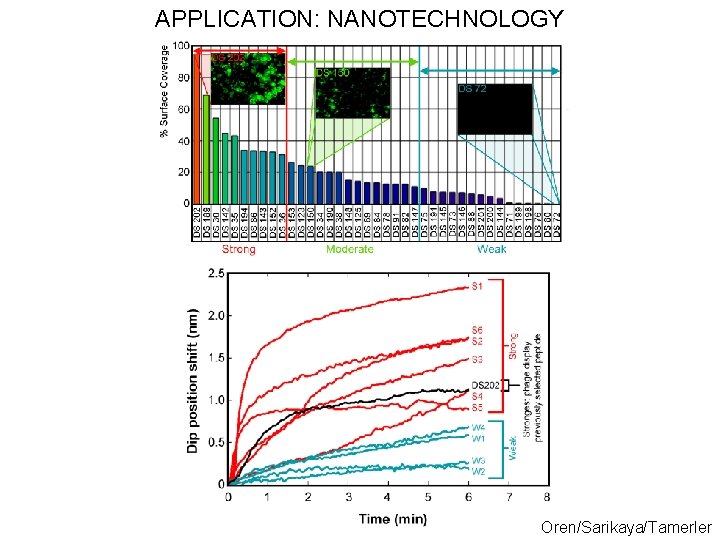
APPLICATION: NANOTECHNOLOGY Oren/Sarikaya/Tamerler
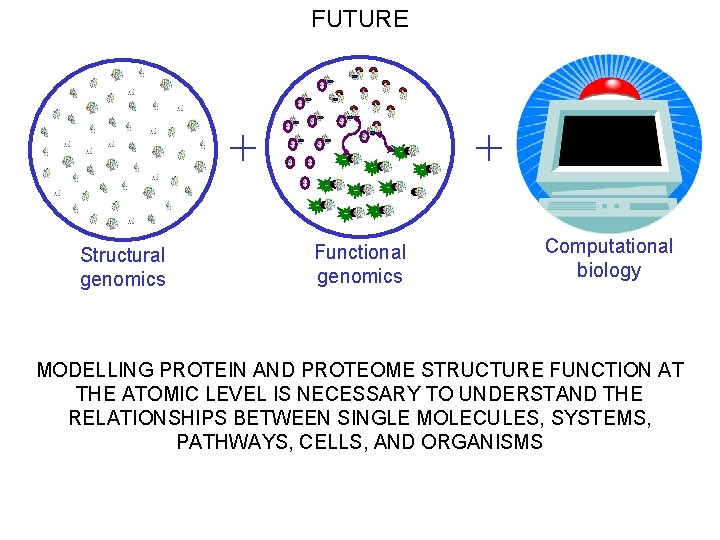
FUTURE + Structural genomics + Functional genomics Computational biology MODELLING PROTEIN AND PROTEOME STRUCTURE FUNCTION AT THE ATOMIC LEVEL IS NECESSARY TO UNDERSTAND THE RELATIONSHIPS BETWEEN SINGLE MOLECULES, SYSTEMS, PATHWAYS, CELLS, AND ORGANISMS
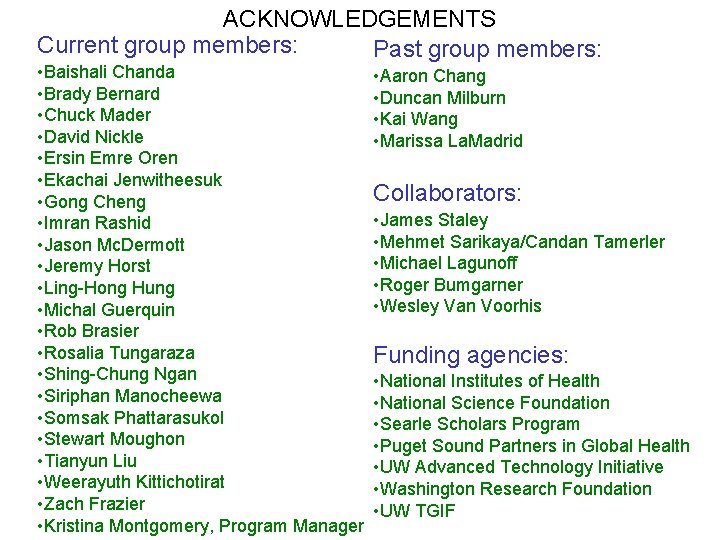
ACKNOWLEDGEMENTS Current group members: Past group members: • Baishali Chanda • Brady Bernard • Chuck Mader • David Nickle • Ersin Emre Oren • Ekachai Jenwitheesuk • Gong Cheng • Imran Rashid • Jason Mc. Dermott • Jeremy Horst • Ling-Hong Hung • Michal Guerquin • Rob Brasier • Rosalia Tungaraza • Shing-Chung Ngan • Siriphan Manocheewa • Somsak Phattarasukol • Stewart Moughon • Tianyun Liu • Weerayuth Kittichotirat • Zach Frazier • Kristina Montgomery, Program Manager • Aaron Chang • Duncan Milburn • Kai Wang • Marissa La. Madrid Collaborators: • James Staley • Mehmet Sarikaya/Candan Tamerler • Michael Lagunoff • Roger Bumgarner • Wesley Van Voorhis Funding agencies: • National Institutes of Health • National Science Foundation • Searle Scholars Program • Puget Sound Partners in Global Health • UW Advanced Technology Initiative • Washington Research Foundation • UW TGIF
Ram samudrala
Ram samudrala
Promotion from assistant to associate professor
Sruthi samudrala
Ram nam me lin hai dekhat sabme ram
Bcs professional membership
Tecniche associate al pensiero computazionale
Inca civilization
Lone star college nursing program deadlines
Certified system engineering professional
What is the drawback of direct mapping?
Project associate cern
Associate degrees in the netherlands
Berstoff gearbox repair
Physician associate lecturer