Ingnierie des systmes de soins et de services
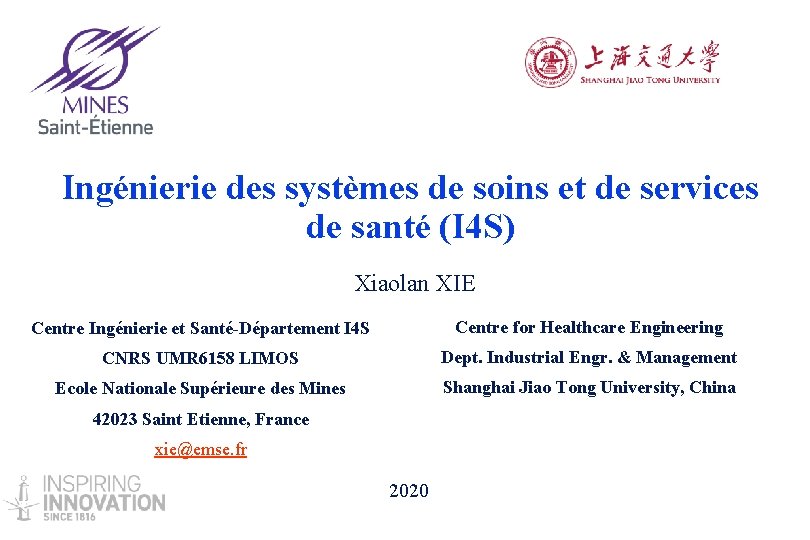
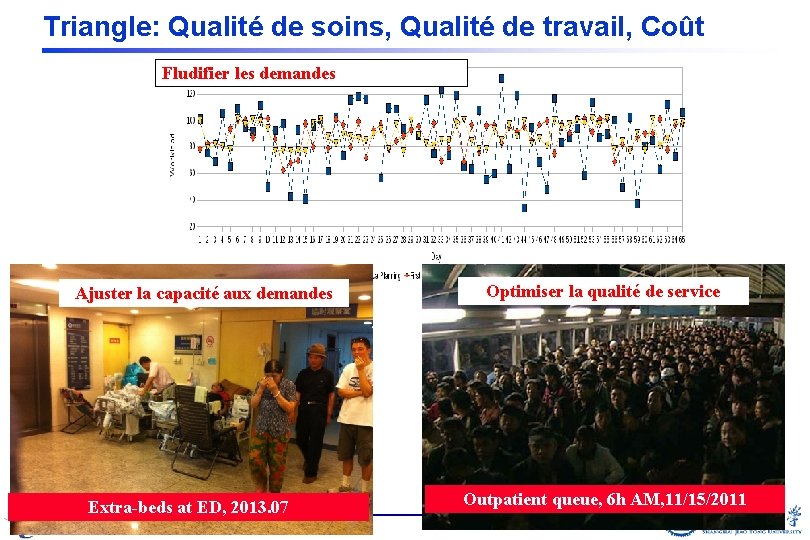
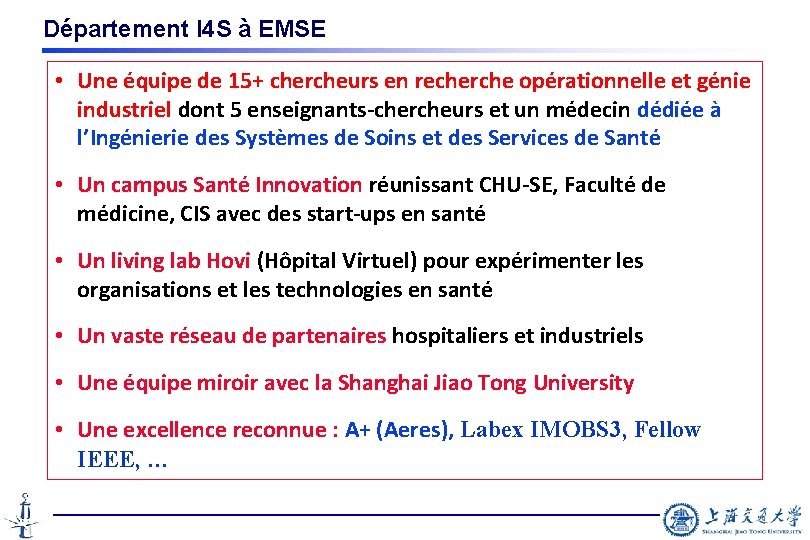
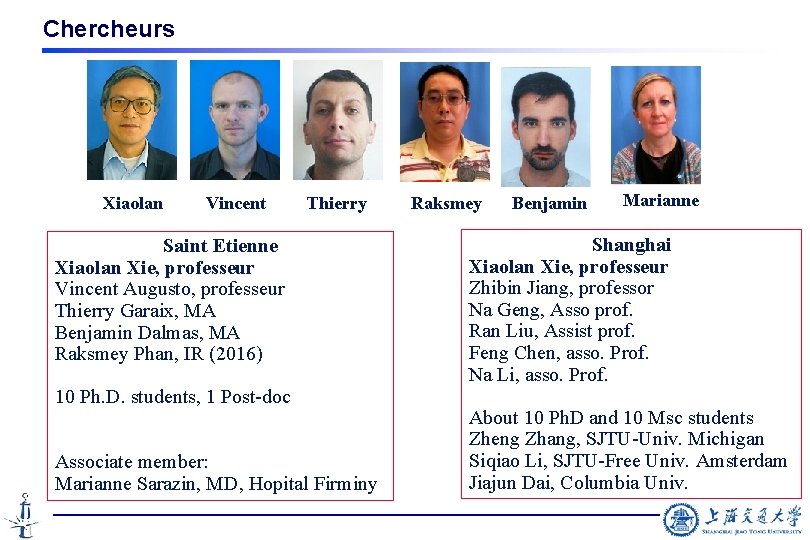
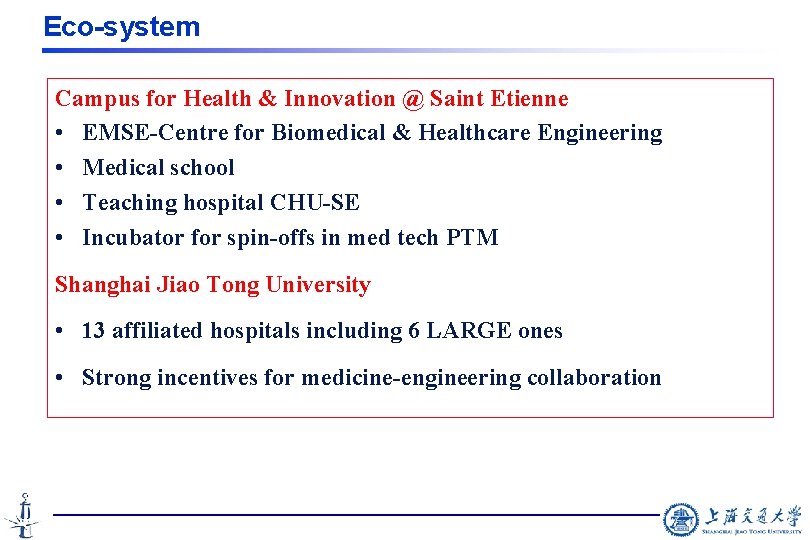
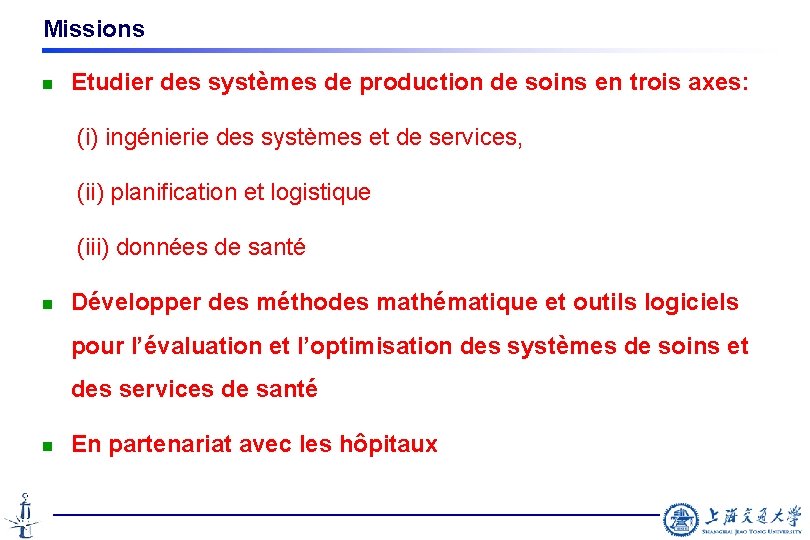
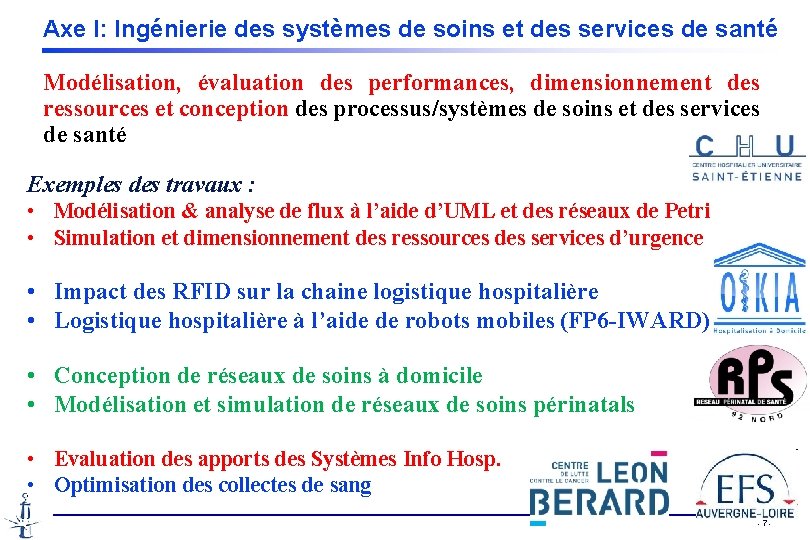
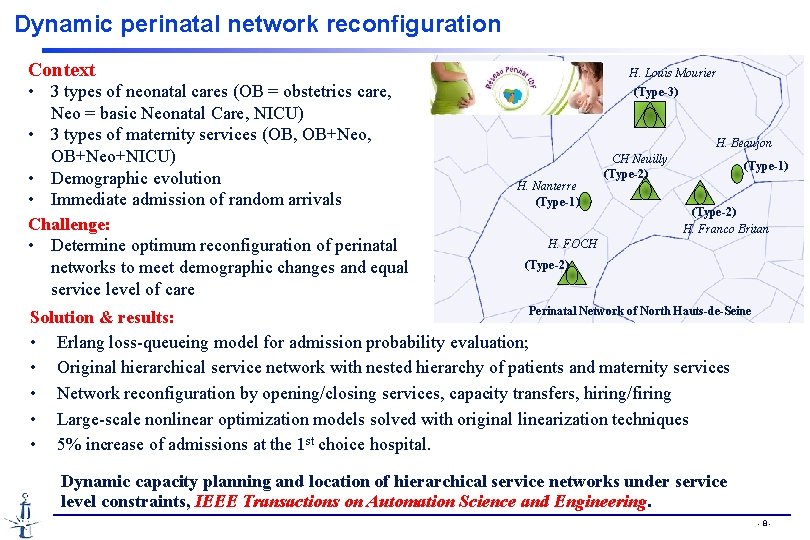
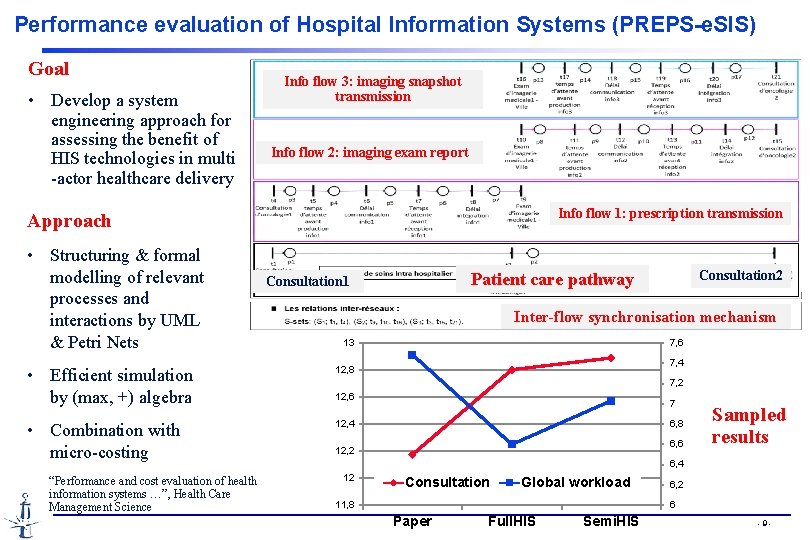
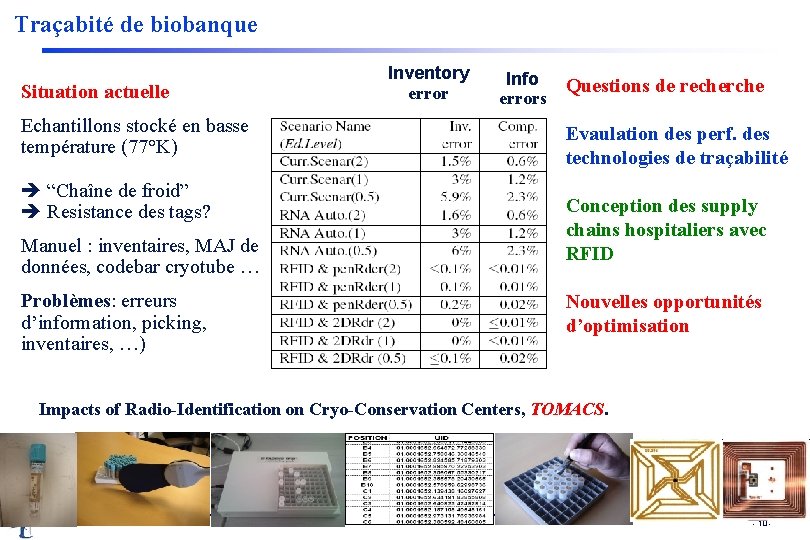
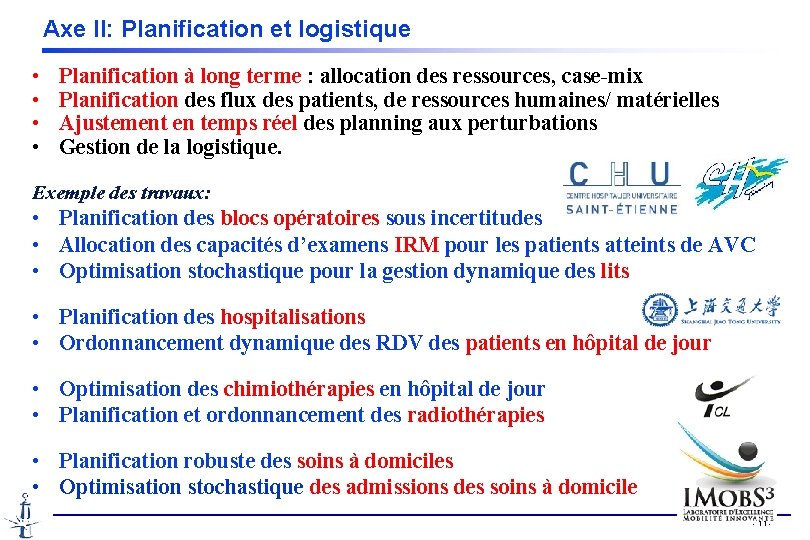
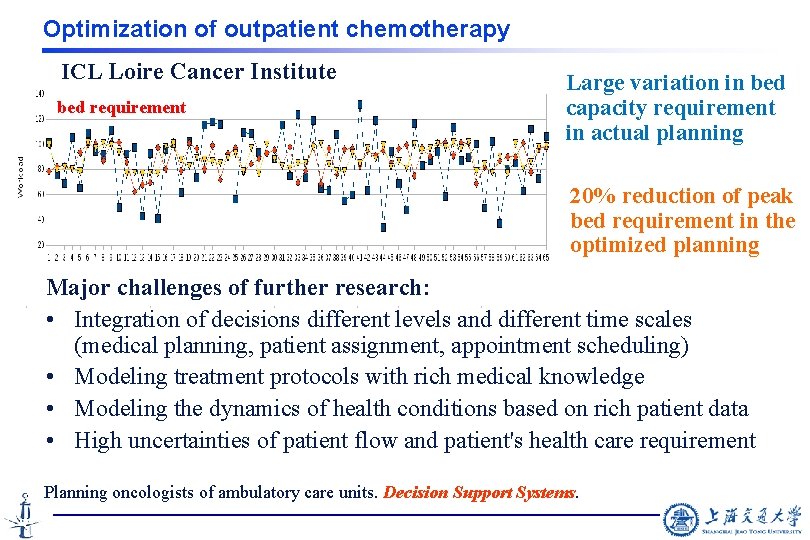
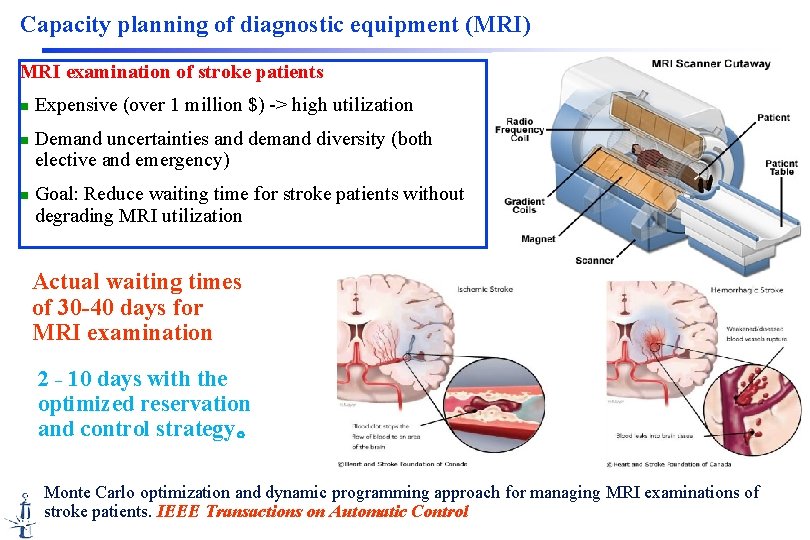
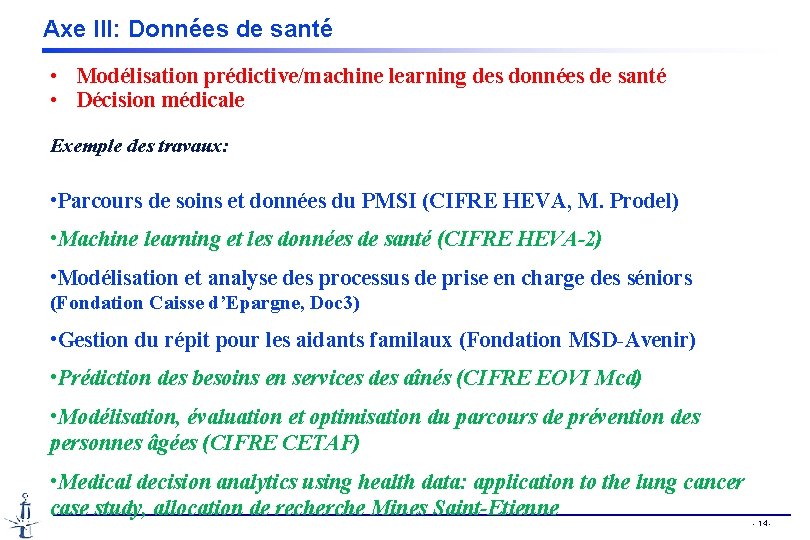
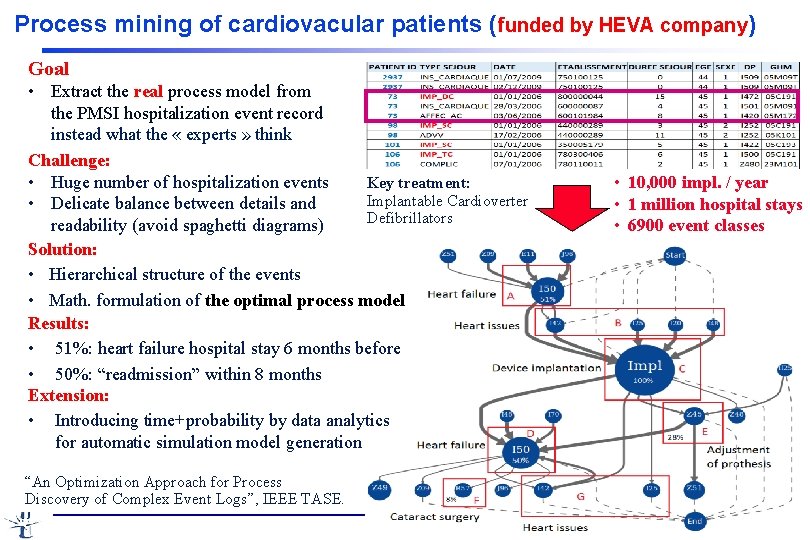
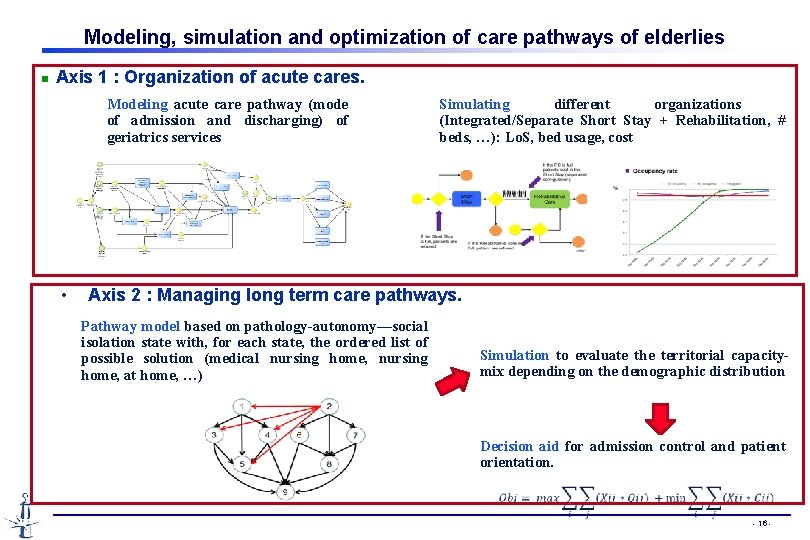
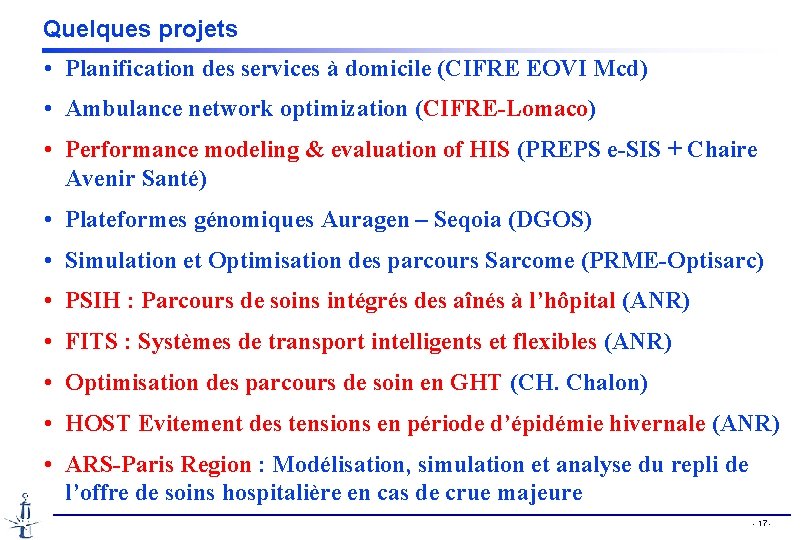
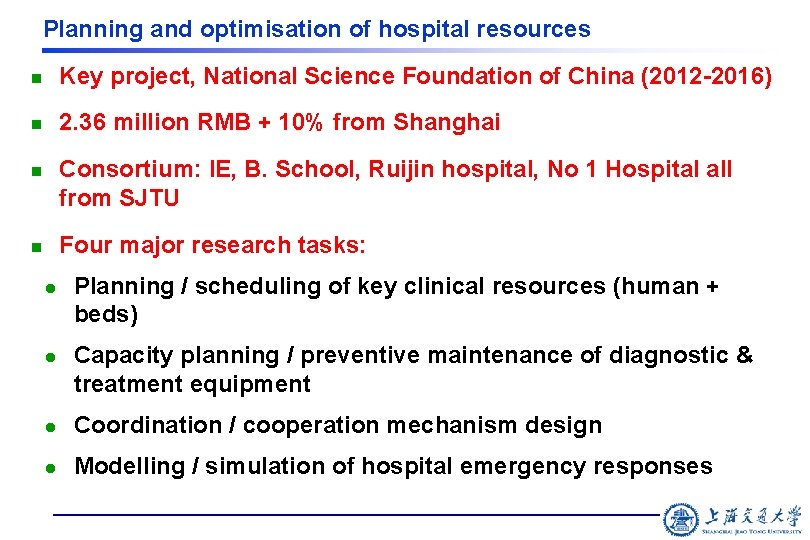
- Slides: 18
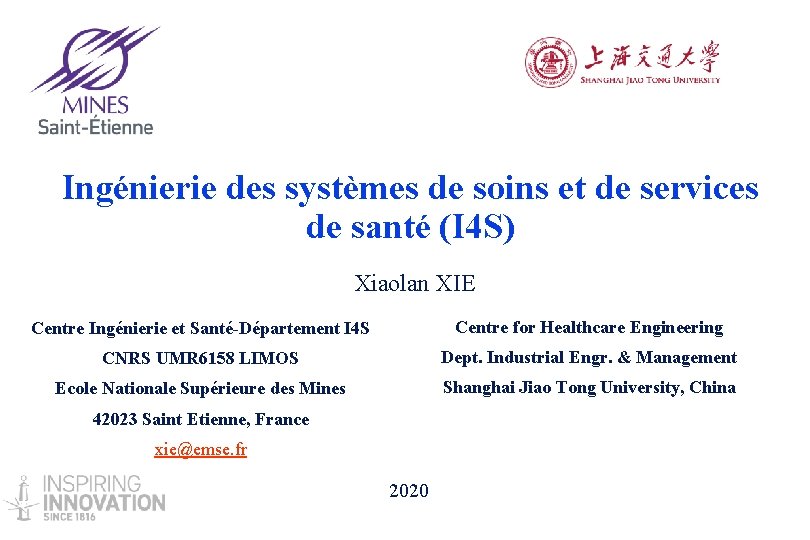
Ingénierie des systèmes de soins et de services de santé (I 4 S) Xiaolan XIE Centre Ingénierie et Santé-Département I 4 S Centre for Healthcare Engineering CNRS UMR 6158 LIMOS Dept. Industrial Engr. & Management Ecole Nationale Supérieure des Mines Shanghai Jiao Tong University, China 42023 Saint Etienne, France xie@emse. fr 2020
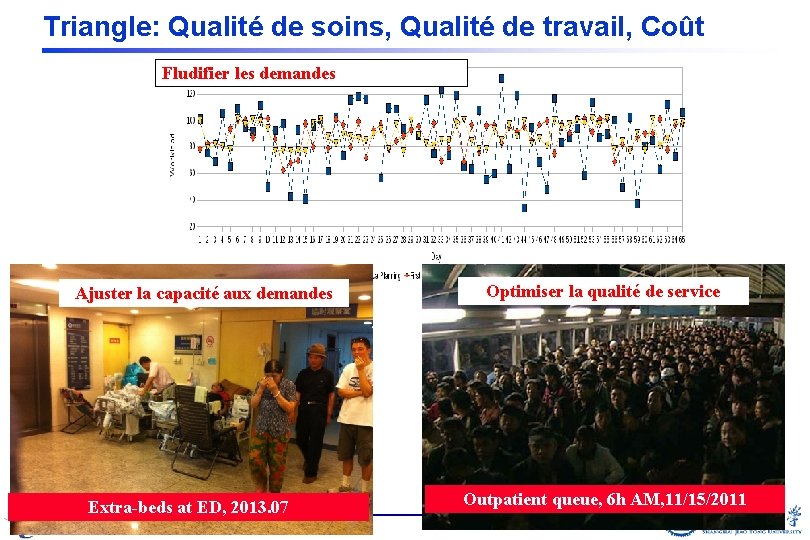
Triangle: Qualité de soins, Qualité de travail, Coût Fludifier les demandes Ajuster la capacité aux demandes Extra-beds at ED, 2013. 07 Optimiser la qualité de service Outpatient queue, 6 h AM, 11/15/2011 -2 -
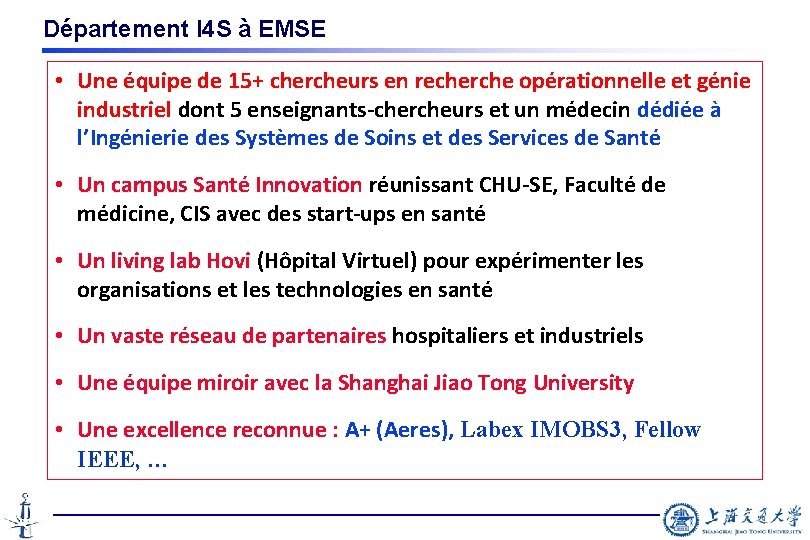
Département I 4 S à EMSE • Une équipe de 15+ chercheurs en recherche opérationnelle et génie industriel dont 5 enseignants-chercheurs et un médecin dédiée à l’Ingénierie des Systèmes de Soins et des Services de Santé • Un campus Santé Innovation réunissant CHU-SE, Faculté de médicine, CIS avec des start-ups en santé • Un living lab Hovi (Hôpital Virtuel) pour expérimenter les organisations et les technologies en santé • Un vaste réseau de partenaires hospitaliers et industriels • Une équipe miroir avec la Shanghai Jiao Tong University • Une excellence reconnue : A+ (Aeres), Labex IMOBS 3, Fellow IEEE, … -3 -
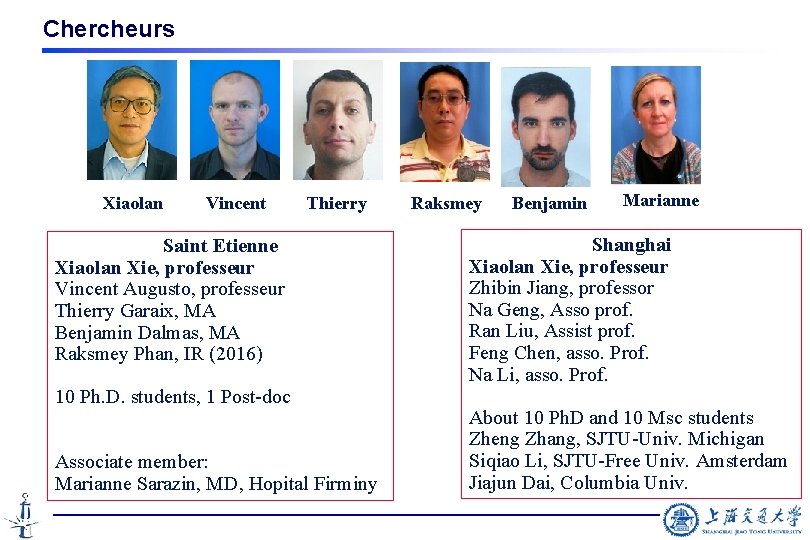
Chercheurs Xiaolan Vincent Thierry Saint Etienne Xiaolan Xie, professeur Vincent Augusto, professeur Thierry Garaix, MA Benjamin Dalmas, MA Raksmey Phan, IR (2016) 10 Ph. D. students, 1 Post-doc Associate member: Marianne Sarazin, MD, Hopital Firminy Raksmey Benjamin Marianne Shanghai Xiaolan Xie, professeur Zhibin Jiang, professor Na Geng, Asso prof. Ran Liu, Assist prof. Feng Chen, asso. Prof. Na Li, asso. Prof. About 10 Ph. D and 10 Msc students Zheng Zhang, SJTU-Univ. Michigan Siqiao Li, SJTU-Free Univ. Amsterdam Jiajun Dai, Columbia Univ. -4 -
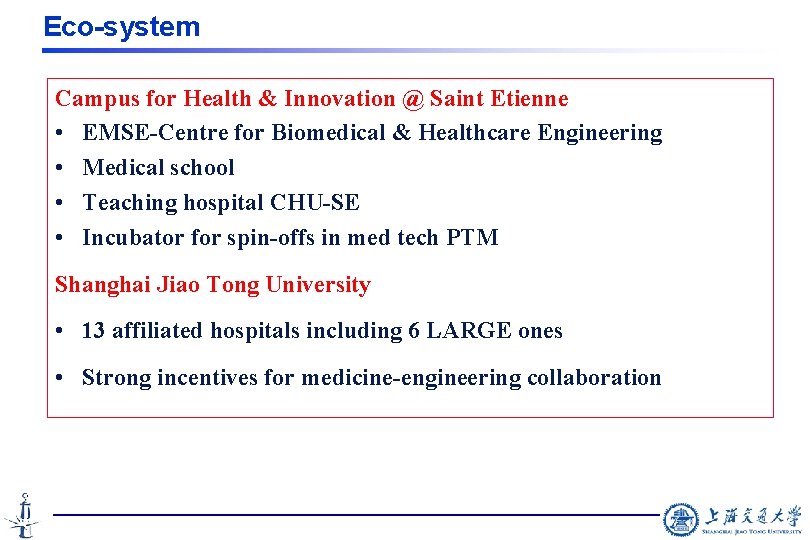
Eco-system Campus for Health & Innovation @ Saint Etienne • EMSE-Centre for Biomedical & Healthcare Engineering • Medical school • Teaching hospital CHU-SE • Incubator for spin-offs in med tech PTM Shanghai Jiao Tong University • 13 affiliated hospitals including 6 LARGE ones • Strong incentives for medicine-engineering collaboration -5 -
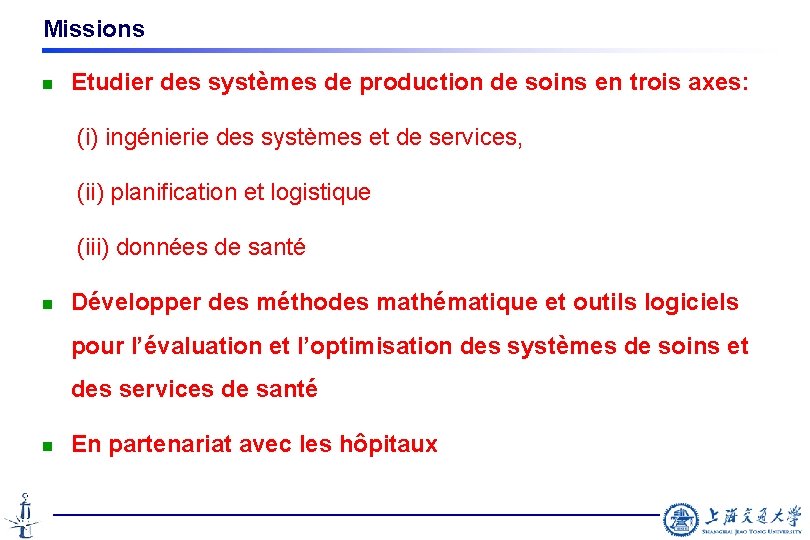
Missions n Etudier des systèmes de production de soins en trois axes: (i) ingénierie des systèmes et de services, (ii) planification et logistique (iii) données de santé n Développer des méthodes mathématique et outils logiciels pour l’évaluation et l’optimisation des systèmes de soins et des services de santé n En partenariat avec les hôpitaux -6 -
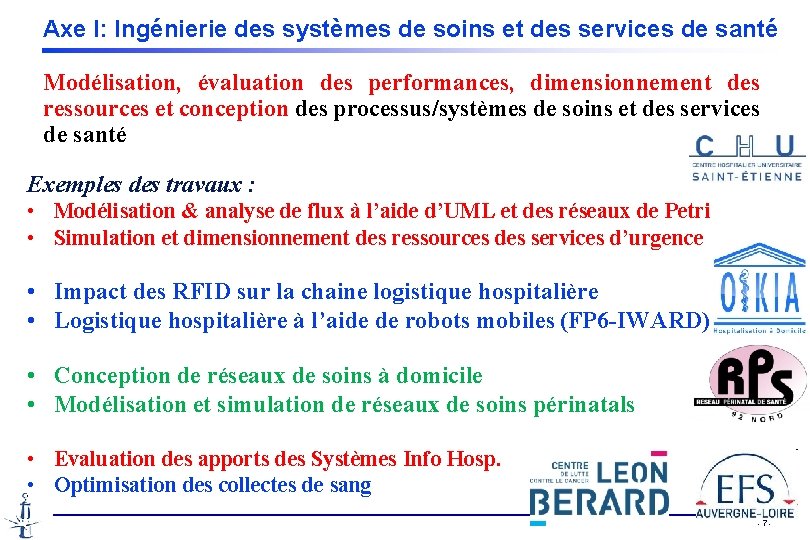
Axe I: Ingénierie des systèmes de soins et des services de santé Modélisation, évaluation des performances, dimensionnement des ressources et conception des processus/systèmes de soins et des services de santé Exemples des travaux : • Modélisation & analyse de flux à l’aide d’UML et des réseaux de Petri • Simulation et dimensionnement des ressources des services d’urgence • Impact des RFID sur la chaine logistique hospitalière • Logistique hospitalière à l’aide de robots mobiles (FP 6 -IWARD) • Conception de réseaux de soins à domicile • Modélisation et simulation de réseaux de soins périnatals • Evaluation des apports des Systèmes Info Hosp. • Optimisation des collectes de sang -7 -
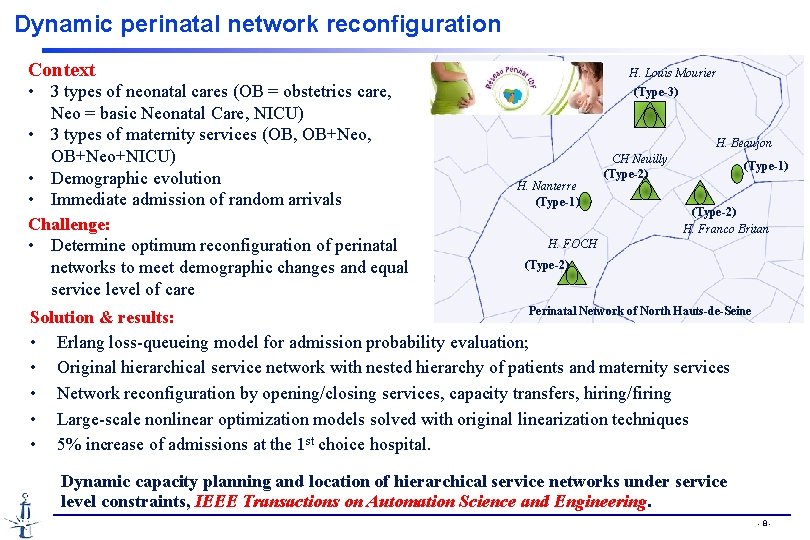
Dynamic perinatal network reconfiguration Context • 3 types of neonatal cares (OB = obstetrics care, Neo = basic Neonatal Care, NICU) • 3 types of maternity services (OB, OB+Neo+NICU) • Demographic evolution • Immediate admission of random arrivals Challenge: • Determine optimum reconfiguration of perinatal networks to meet demographic changes and equal service level of care H. Louis Mourier (Type-3) H. Beaujon H. Nanterre (Type-1) CH Neuilly (Type-2) (Type-1) (Type-2) H. Franco Britan H. FOCH (Type-2) Perinatal Network of North Hauts-de-Seine Solution & results: • Erlang loss-queueing model for admission probability evaluation; • Original hierarchical service network with nested hierarchy of patients and maternity services • Network reconfiguration by opening/closing services, capacity transfers, hiring/firing • Large-scale nonlinear optimization models solved with original linearization techniques • 5% increase of admissions at the 1 st choice hospital. Dynamic capacity planning and location of hierarchical service networks under service level constraints, IEEE Transactions on Automation Science and Engineering. -8 -
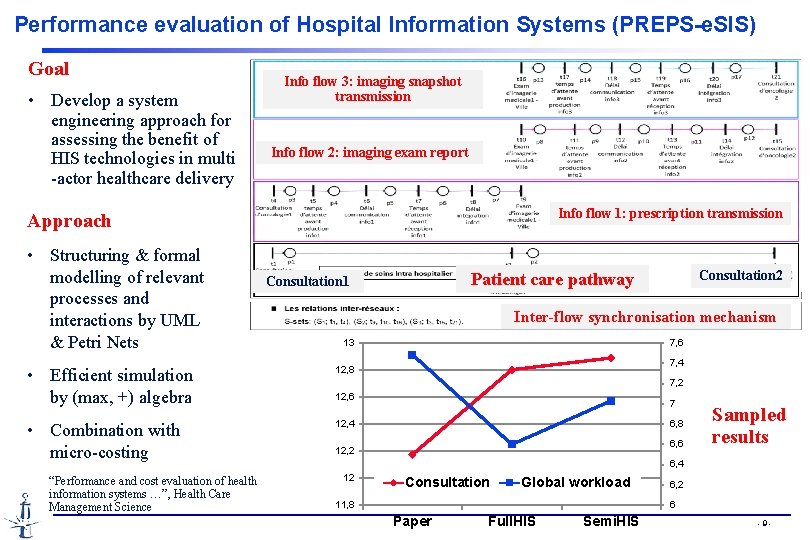
Performance evaluation of Hospital Information Systems (PREPS-e. SIS) Goal • Develop a system engineering approach for assessing the benefit of HIS technologies in multi -actor healthcare delivery Info flow 3: imaging snapshot transmission Info flow 2: imaging exam report Info flow 1: prescription transmission Approach • Structuring & formal modelling of relevant processes and interactions by UML & Petri Nets Inter-flow synchronisation mechanism 13 • Efficient simulation by (max, +) algebra 12, 8 • Combination with micro-costing 12, 4 “Performance and cost evaluation of health information systems …”, Health Care Management Science Consultation 2 Patient care pathway Consultation 1 7, 6 7, 4 7, 2 12, 6 7 6, 8 6, 6 12, 2 Sampled results 6, 4 12 Consultation Global workload 11, 8 6, 2 6 Paper Full. HIS Semi. HIS -9 -
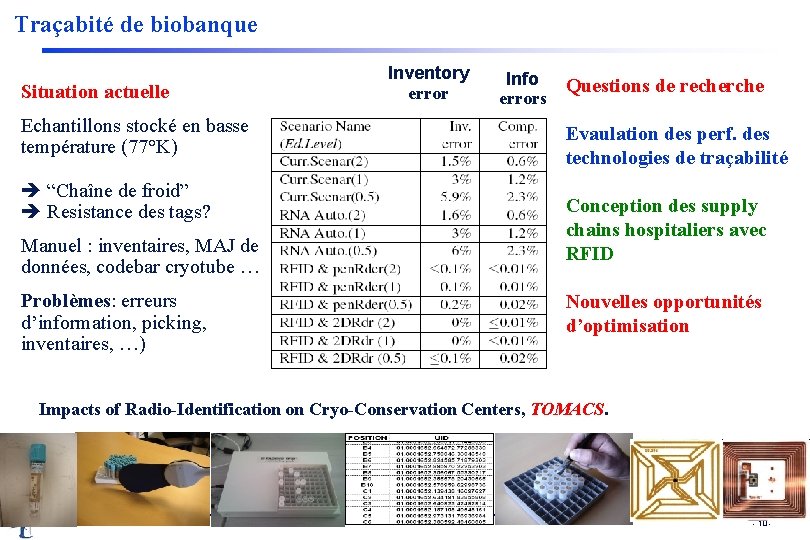
Traçabité de biobanque Situation actuelle Echantillons stocké en basse température (77°K) “Chaîne de froid” Resistance des tags? Manuel : inventaires, MAJ de données, codebar cryotube … Problèmes: erreurs d’information, picking, inventaires, …) Inventory error Info errors Questions de recherche Evaulation des perf. des technologies de traçabilité Conception des supply chains hospitaliers avec RFID Nouvelles opportunités d’optimisation Impacts of Radio-Identification on Cryo-Conservation Centers, TOMACS. - 10 -
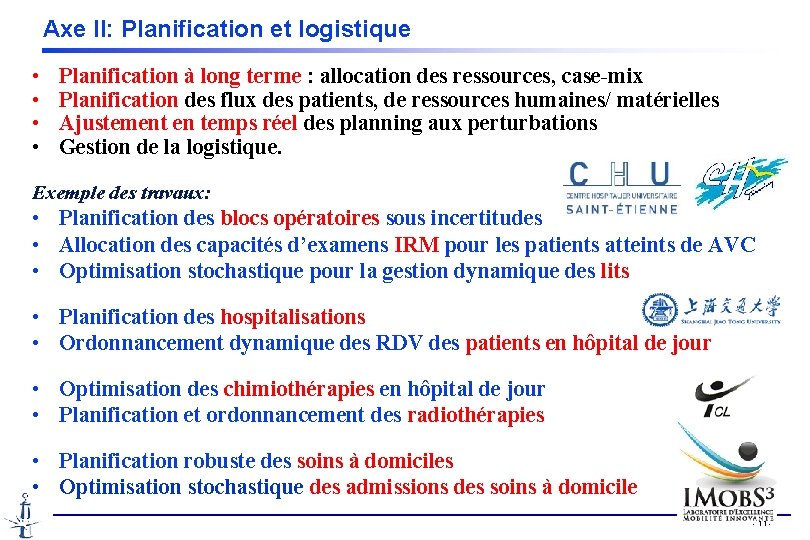
Axe II: Planification et logistique • • Planification à long terme : allocation des ressources, case-mix Planification des flux des patients, de ressources humaines/ matérielles Ajustement en temps réel des planning aux perturbations Gestion de la logistique. Exemple des travaux: • Planification des blocs opératoires sous incertitudes • Allocation des capacités d’examens IRM pour les patients atteints de AVC • Optimisation stochastique pour la gestion dynamique des lits • Planification des hospitalisations • Ordonnancement dynamique des RDV des patients en hôpital de jour • Optimisation des chimiothérapies en hôpital de jour • Planification et ordonnancement des radiothérapies • Planification robuste des soins à domiciles • Optimisation stochastique des admissions des soins à domicile - 11 -
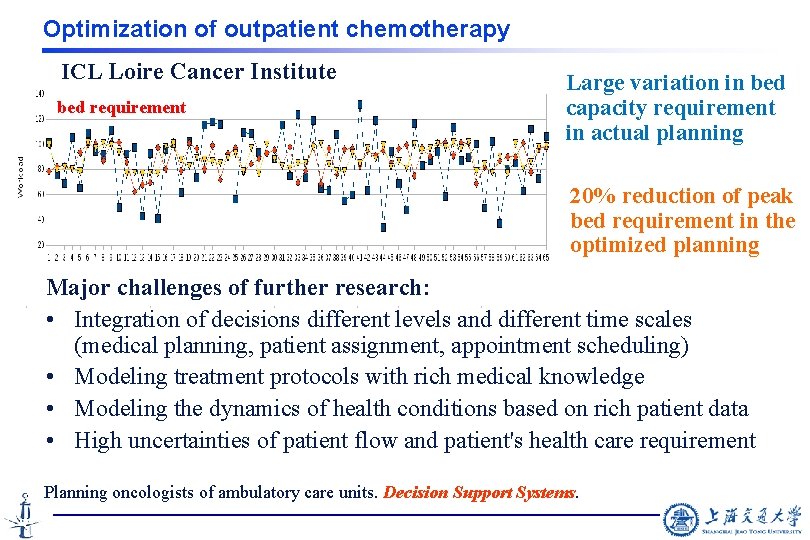
Optimization of outpatient chemotherapy ICL Loire Cancer Institute bed requirement Large variation in bed capacity requirement in actual planning 20% reduction of peak bed requirement in the optimized planning Major challenges of further research: • Integration of decisions different levels and different time scales (medical planning, patient assignment, appointment scheduling) • Modeling treatment protocols with rich medical knowledge • Modeling the dynamics of health conditions based on rich patient data • High uncertainties of patient flow and patient's health care requirement Planning oncologists of ambulatory care units. Decision Support Systems. - 12 -
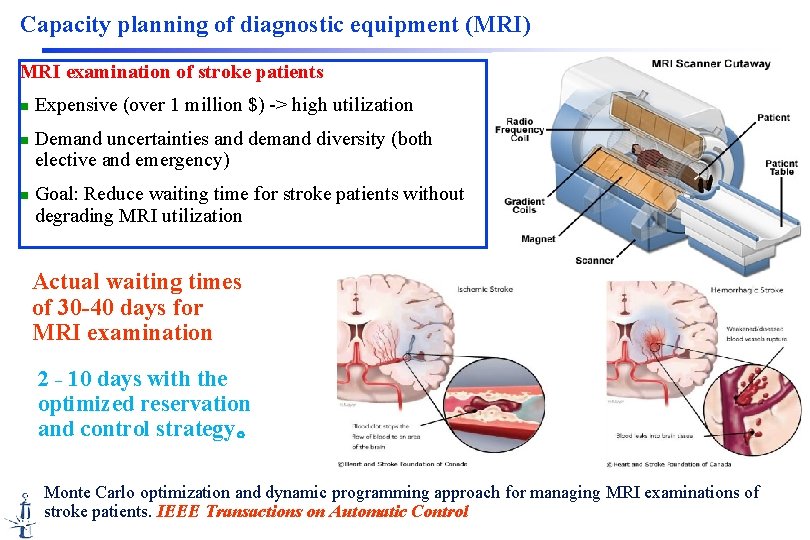
Capacity planning of diagnostic equipment (MRI) MRI examination of stroke patients n n n Expensive (over 1 million $) -> high utilization Demand uncertainties and demand diversity (both elective and emergency) Goal: Reduce waiting time for stroke patients without degrading MRI utilization Actual waiting times of 30 -40 days for MRI examination 2 - 10 days with the optimized reservation and control strategy。 Monte Carlo optimization and dynamic programming approach for managing MRI examinations of stroke patients. IEEE Transactions on Automatic Control - 13 -
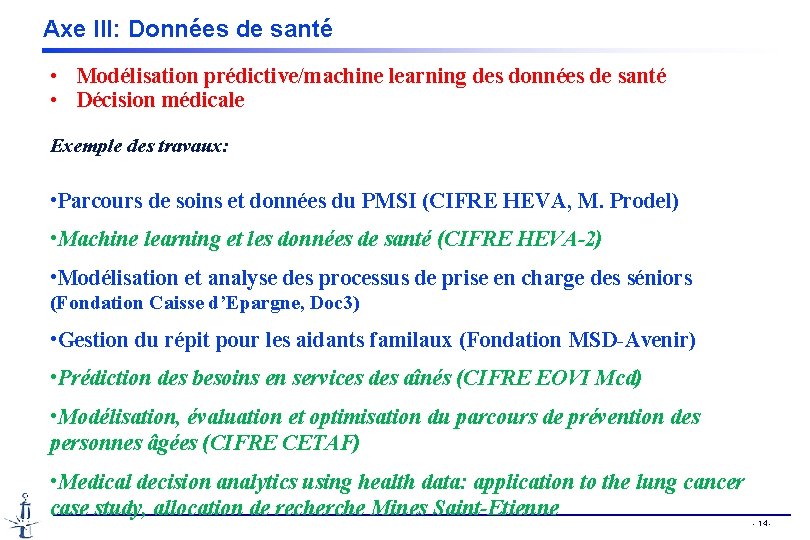
Axe III: Données de santé • Modélisation prédictive/machine learning des données de santé • Décision médicale Exemple des travaux: • Parcours de soins et données du PMSI (CIFRE HEVA, M. Prodel) • Machine learning et les données de santé (CIFRE HEVA-2) • Modélisation et analyse des processus de prise en charge des séniors (Fondation Caisse d’Epargne, Doc 3) • Gestion du répit pour les aidants familaux (Fondation MSD-Avenir) • Prédiction des besoins en services des aînés (CIFRE EOVI Mcd) • Modélisation, évaluation et optimisation du parcours de prévention des personnes âgées (CIFRE CETAF) • Medical decision analytics using health data: application to the lung cancer case study, allocation de recherche Mines Saint-Etienne - 14 -
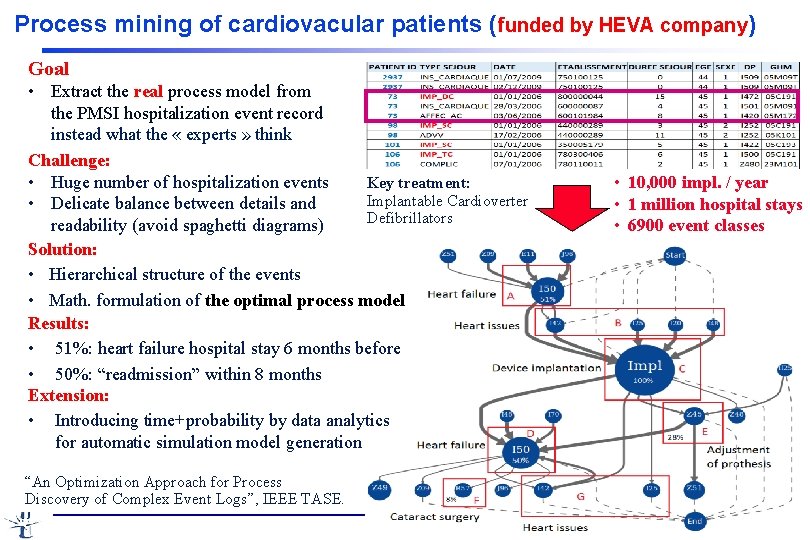
Process mining of cardiovacular patients (funded by HEVA company) Goal • Extract the real process model from the PMSI hospitalization event record instead what the « experts » think Challenge: • Huge number of hospitalization events Key treatment: Implantable Cardioverter • Delicate balance between details and Defibrillators readability (avoid spaghetti diagrams) Solution: • Hierarchical structure of the events • Math. formulation of the optimal process model Results: • 51%: heart failure hospital stay 6 months before • 50%: “readmission” within 8 months Extension: • Introducing time+probability by data analytics for automatic simulation model generation • 10, 000 impl. / year • 1 million hospital stays • 6900 event classes “An Optimization Approach for Process Discovery of Complex Event Logs”, IEEE TASE. - 15 -
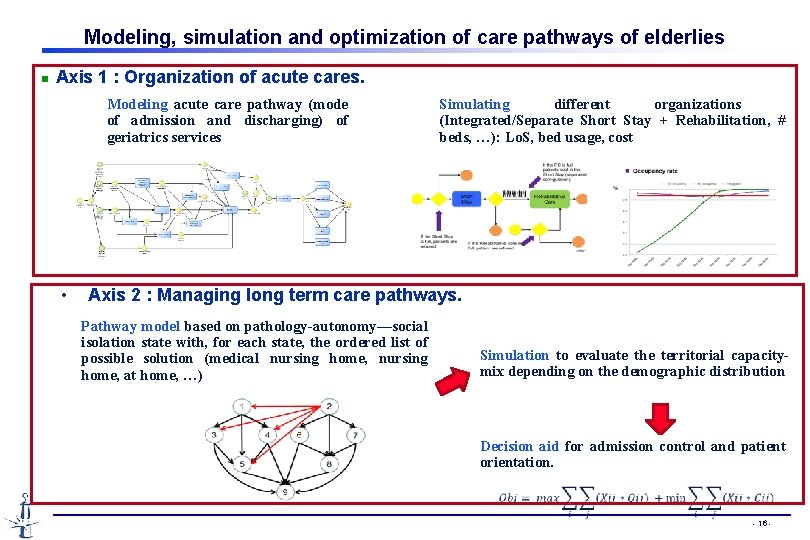
Modeling, simulation and optimization of care pathways of elderlies n Axis 1 : Organization of acute cares. Modeling acute care pathway (mode of admission and discharging) of geriatrics services • Simulating different organizations (Integrated/Separate Short Stay + Rehabilitation, # beds, …): Lo. S, bed usage, cost Axis 2 : Managing long term care pathways. Pathway model based on pathology-autonomy—social isolation state with, for each state, the ordered list of possible solution (medical nursing home, at home, …) Simulation to evaluate the territorial capacitymix depending on the demographic distribution Decision aid for admission control and patient orientation. - 16 -
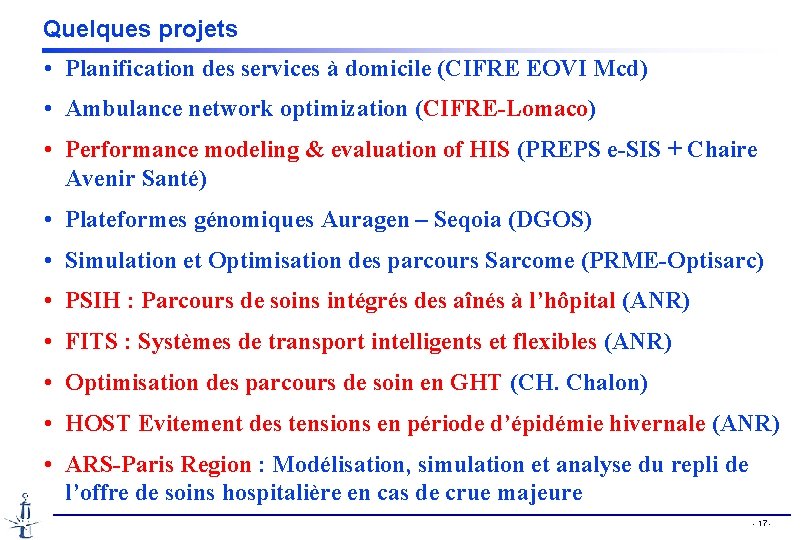
Quelques projets • Planification des services à domicile (CIFRE EOVI Mcd) • Ambulance network optimization (CIFRE-Lomaco) • Performance modeling & evaluation of HIS (PREPS e-SIS + Chaire Avenir Santé) • Plateformes génomiques Auragen – Seqoia (DGOS) • Simulation et Optimisation des parcours Sarcome (PRME-Optisarc) • PSIH : Parcours de soins intégrés des aînés à l’hôpital (ANR) • FITS : Systèmes de transport intelligents et flexibles (ANR) • Optimisation des parcours de soin en GHT (CH. Chalon) • HOST Evitement des tensions en période d’épidémie hivernale (ANR) • ARS-Paris Region : Modélisation, simulation et analyse du repli de l’offre de soins hospitalière en cas de crue majeure - 17 -
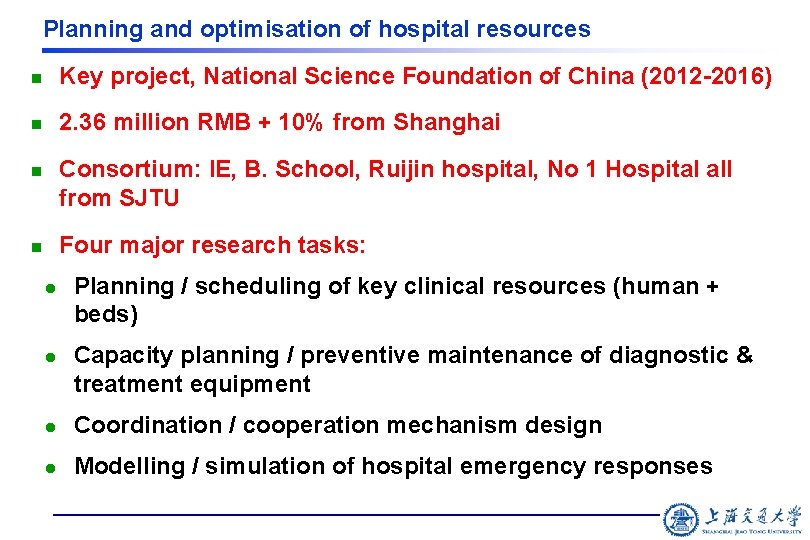
Planning and optimisation of hospital resources n Key project, National Science Foundation of China (2012 -2016) n 2. 36 million RMB + 10% from Shanghai Consortium: IE, B. School, Ruijin hospital, No 1 Hospital all from SJTU n Four major research tasks: n l l Planning / scheduling of key clinical resources (human + beds) Capacity planning / preventive maintenance of diagnostic & treatment equipment l Coordination / cooperation mechanism design l Modelling / simulation of hospital emergency responses - 18 -
Système technique définition
Systmes
Systmes
Ingnierie
Atlas dsl grammar
Ingnierie
Saphia bouchentouf
Des des des
Demarche de soins infirmier
Traçabilité des soins infirmiers législation
Les 5 etapes de la demarche de soins aide soignante
La diffusion des idées des lumières
Il existe des personnes qui sont des lumières pour tous
Mode verbal
Plan marshall affiche
Diversification des espaces et des acteurs de la production
Volume d'un corps
Mesure des liquides
Cartographie des risques gestion des stocks