Identification of Prognostic Factors Related to Survival Time
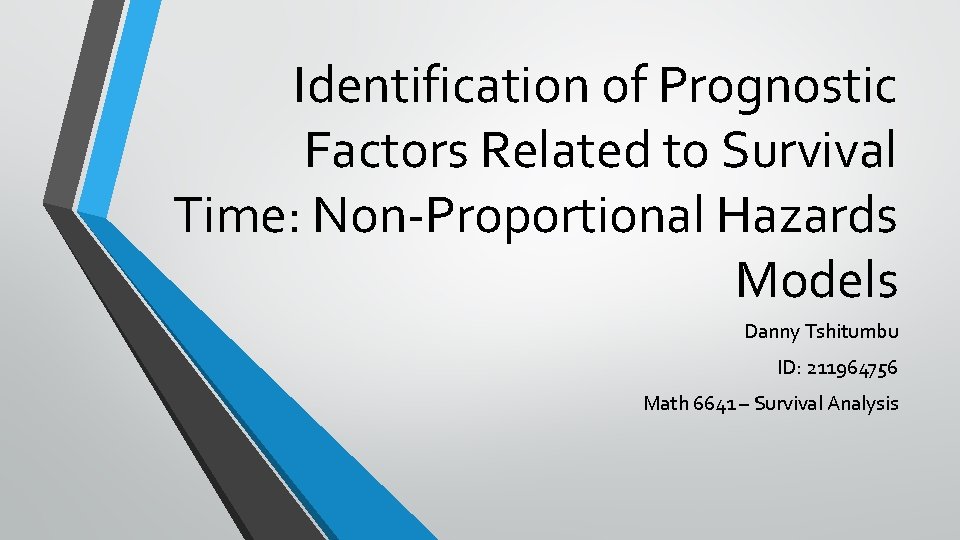
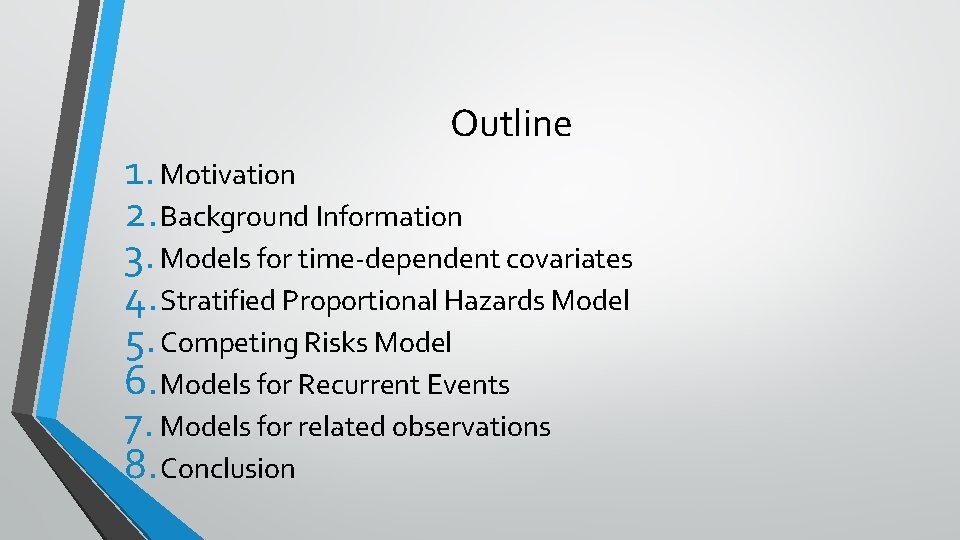
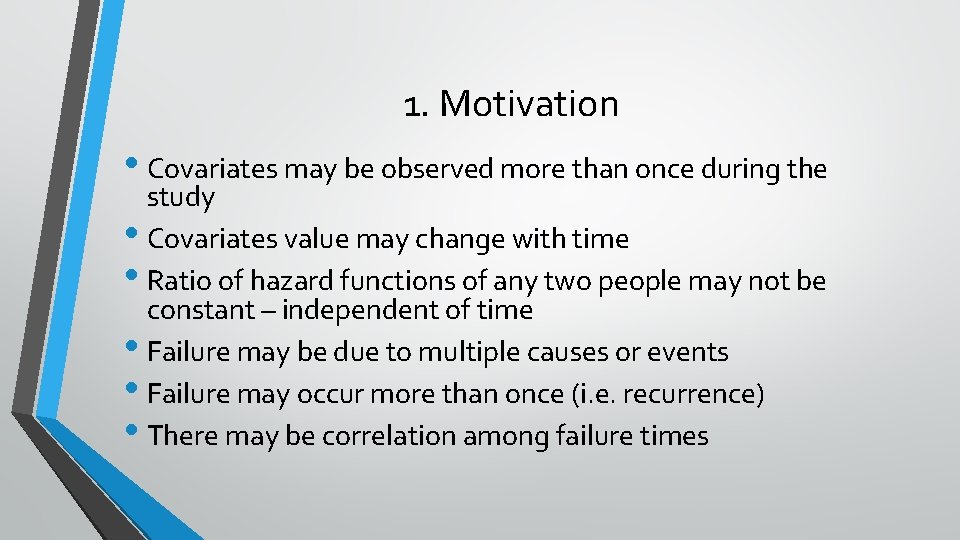
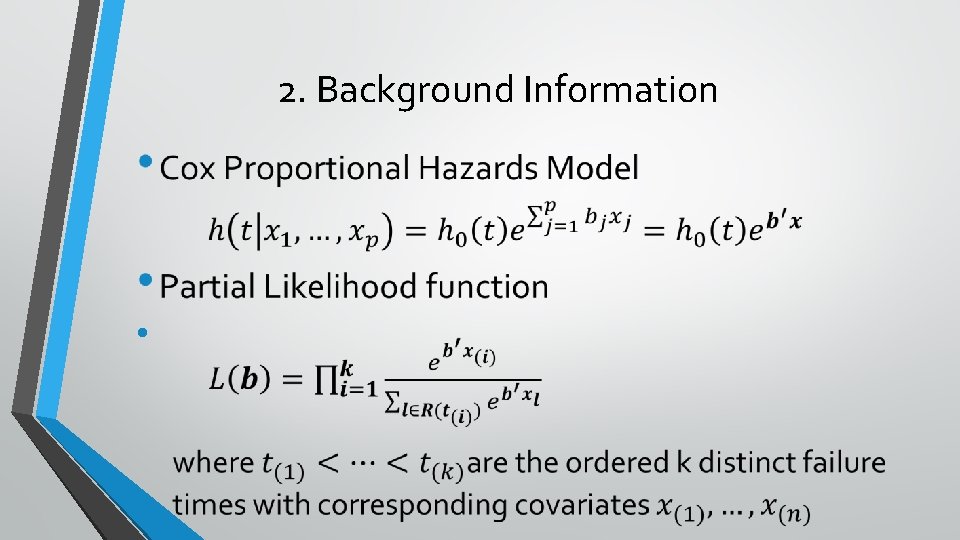
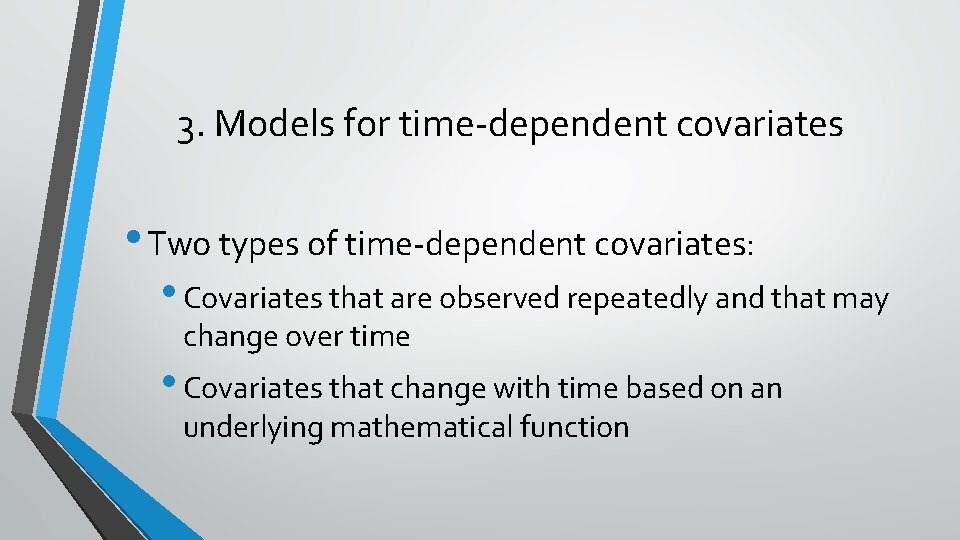
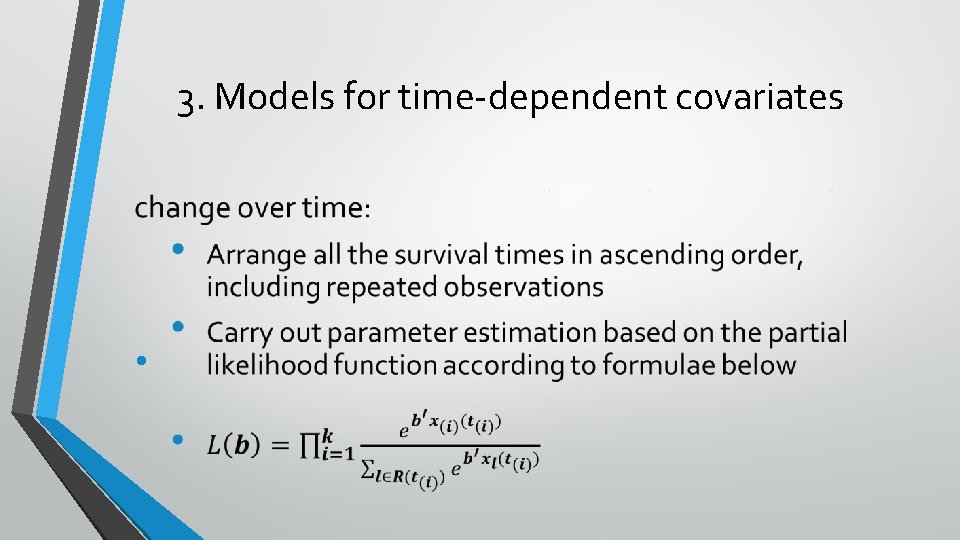
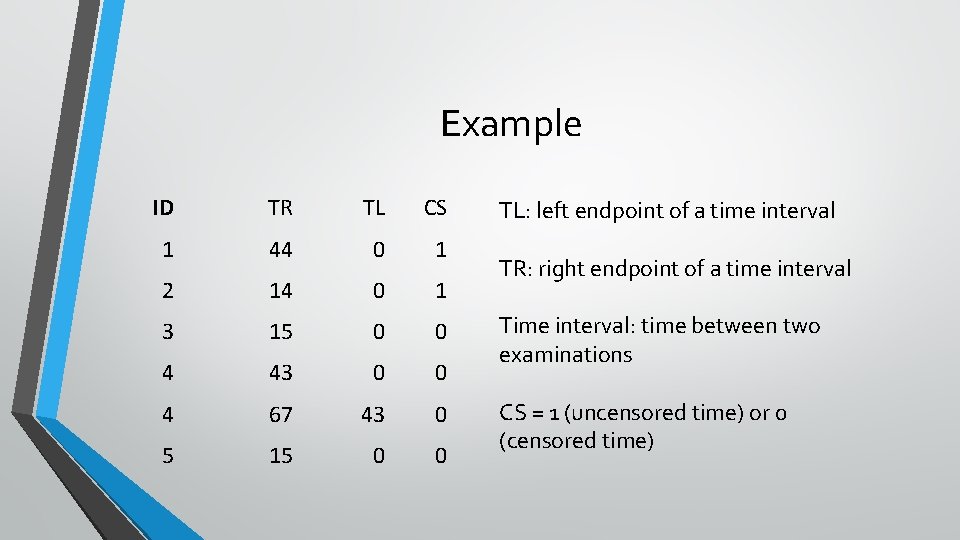
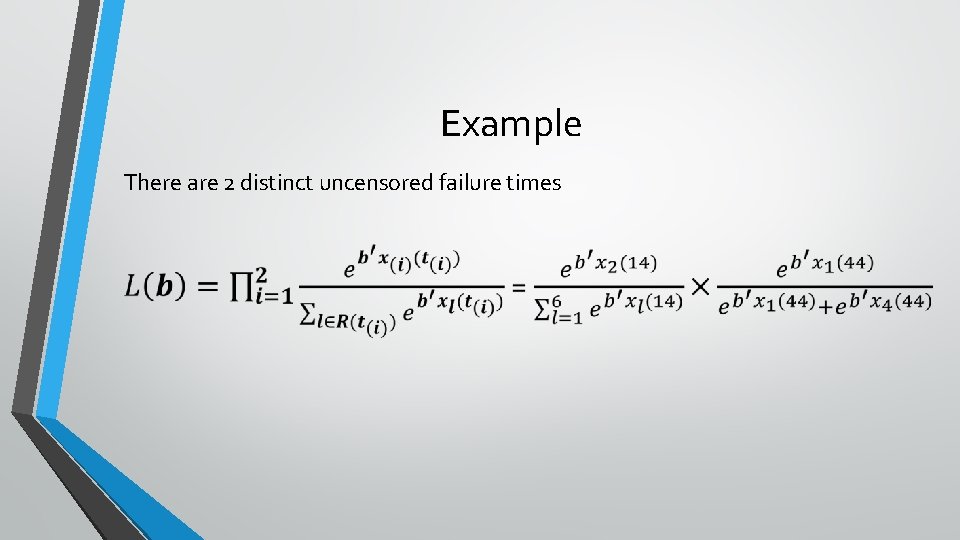
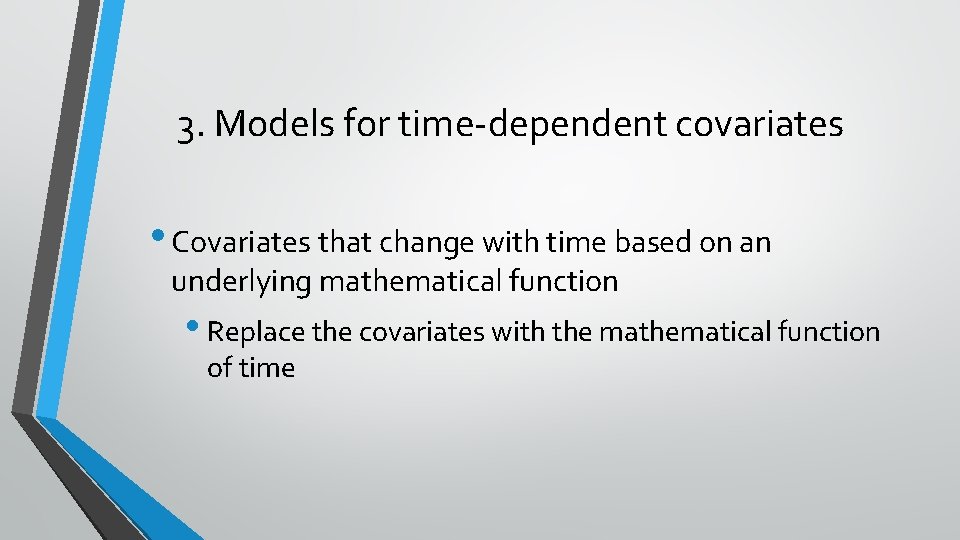
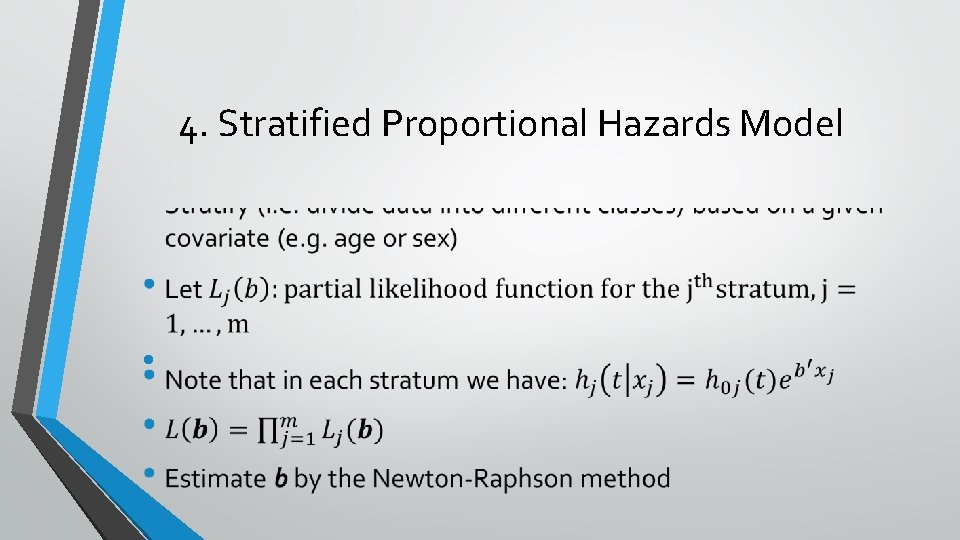
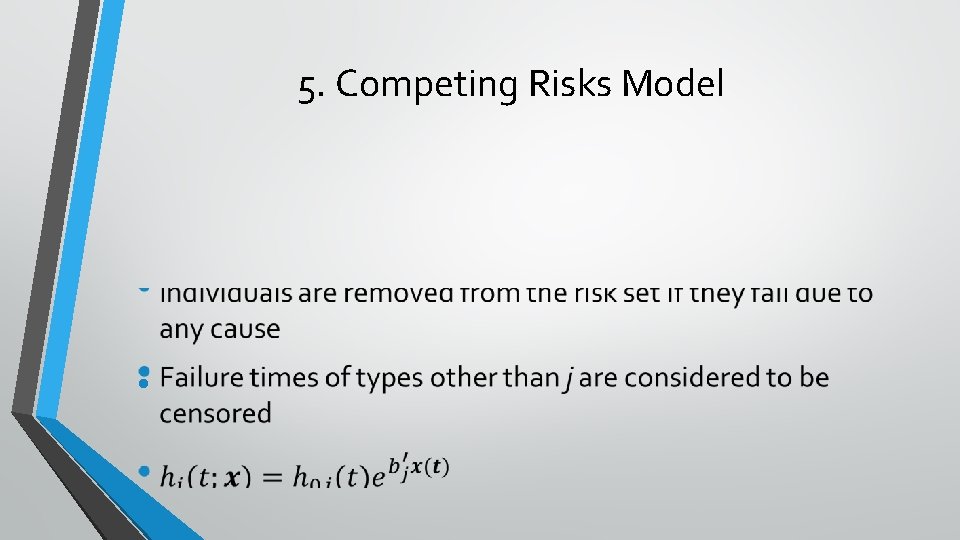
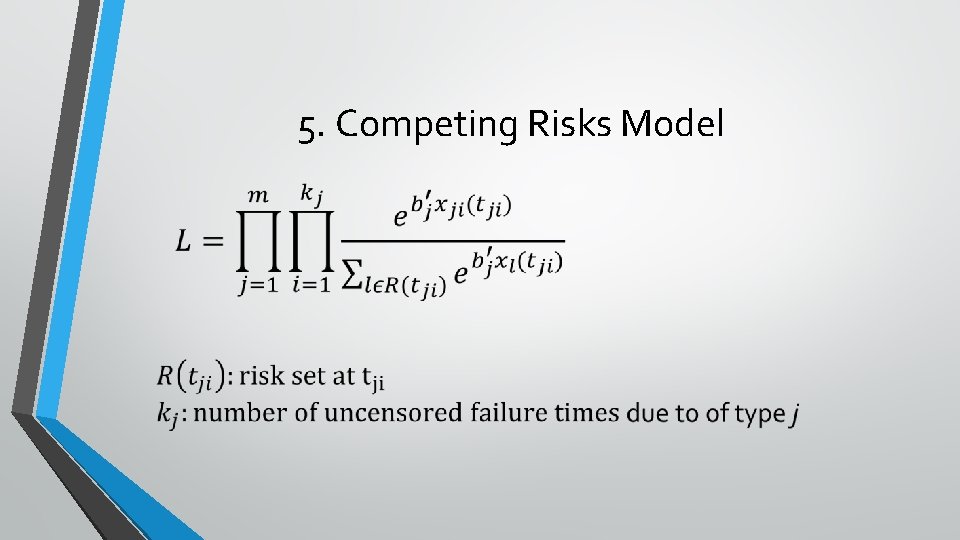
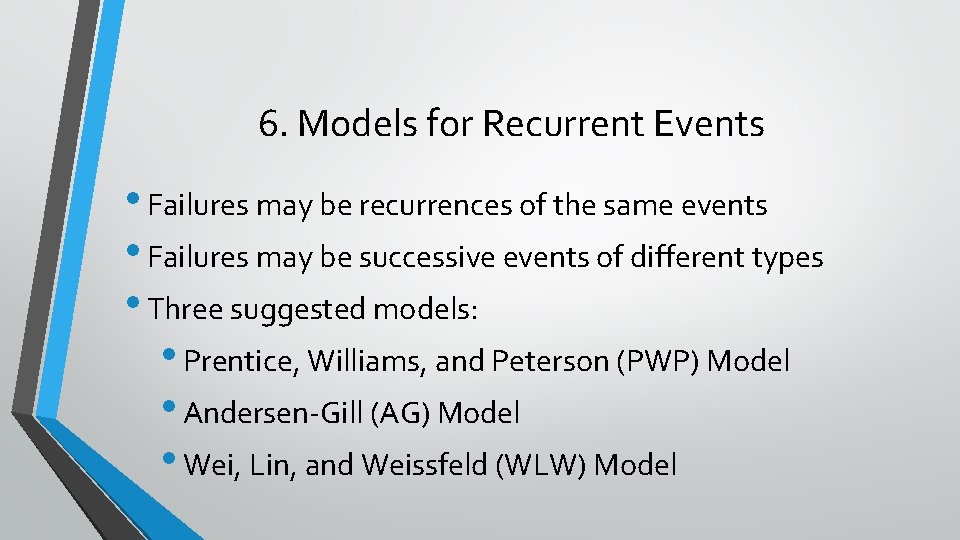
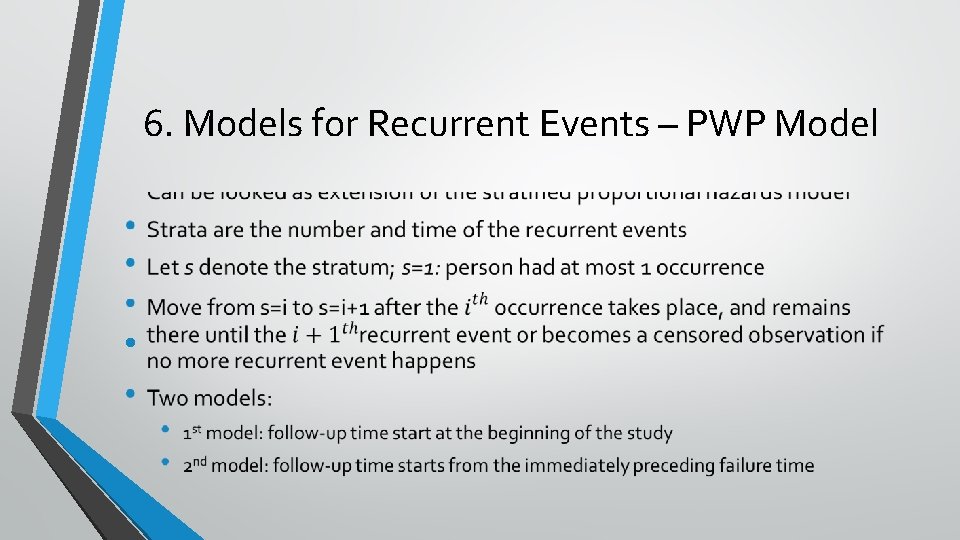
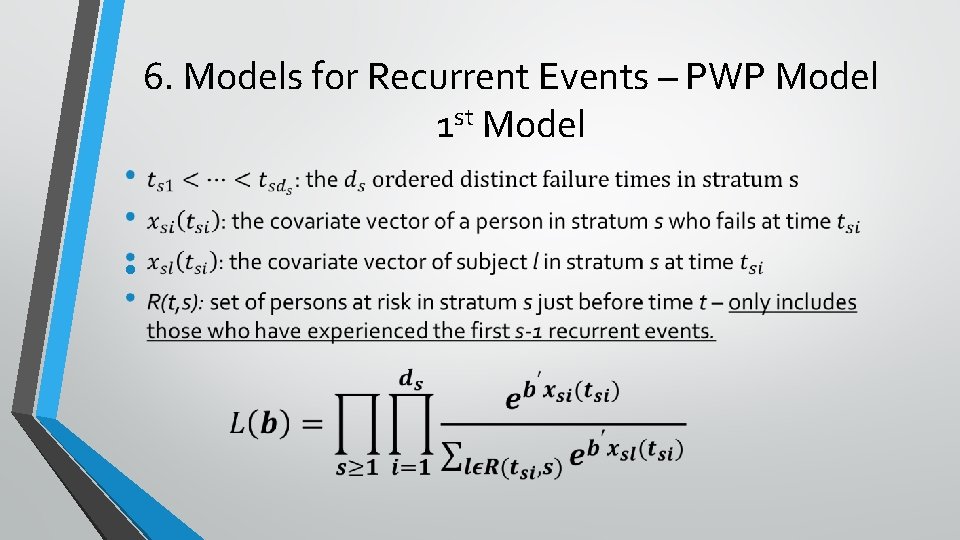
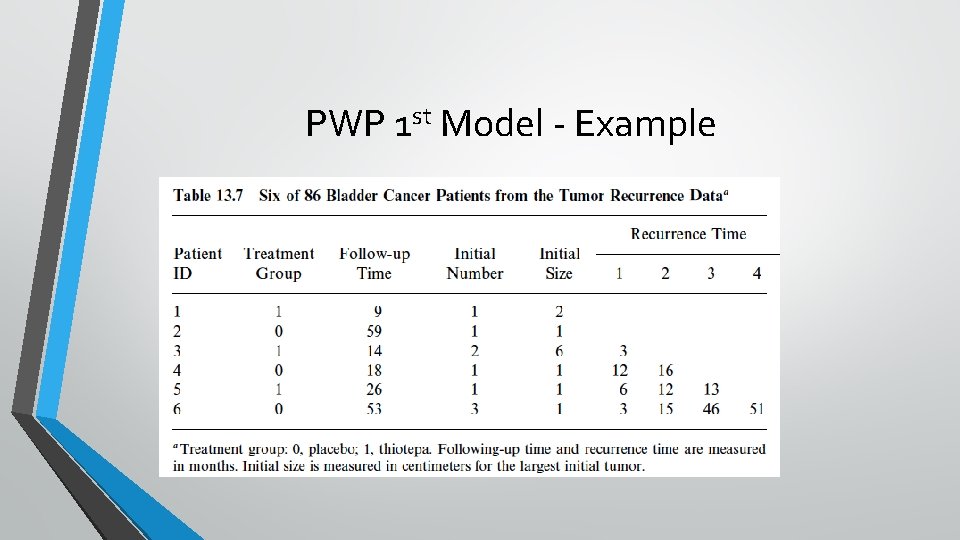
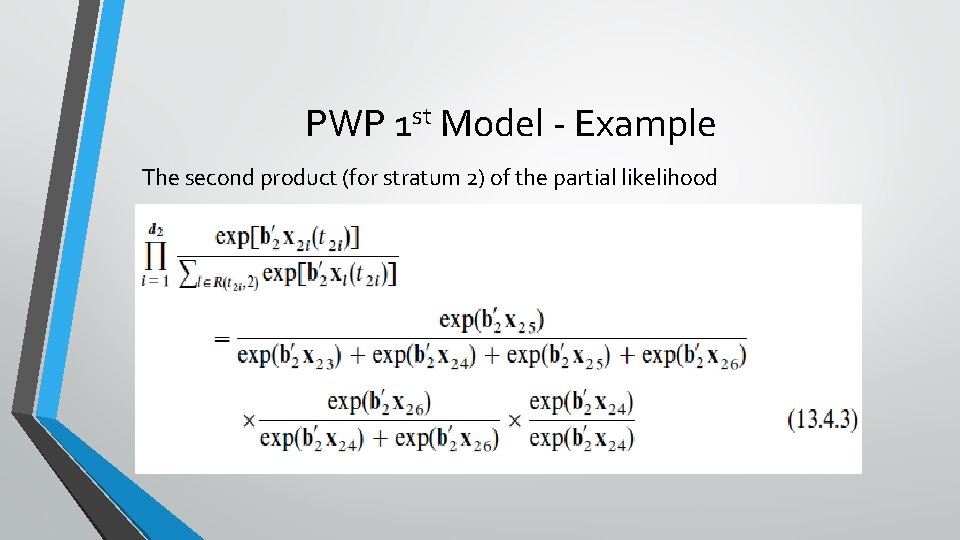
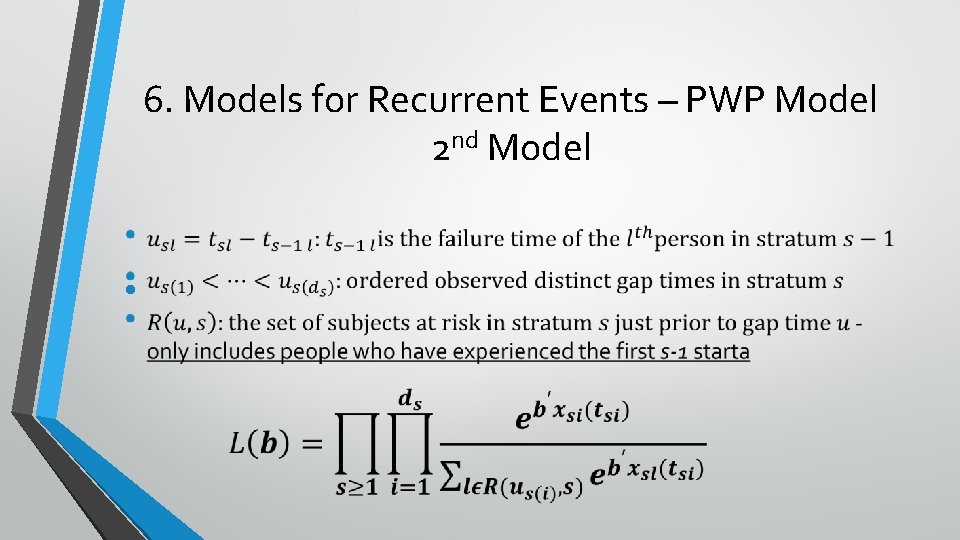
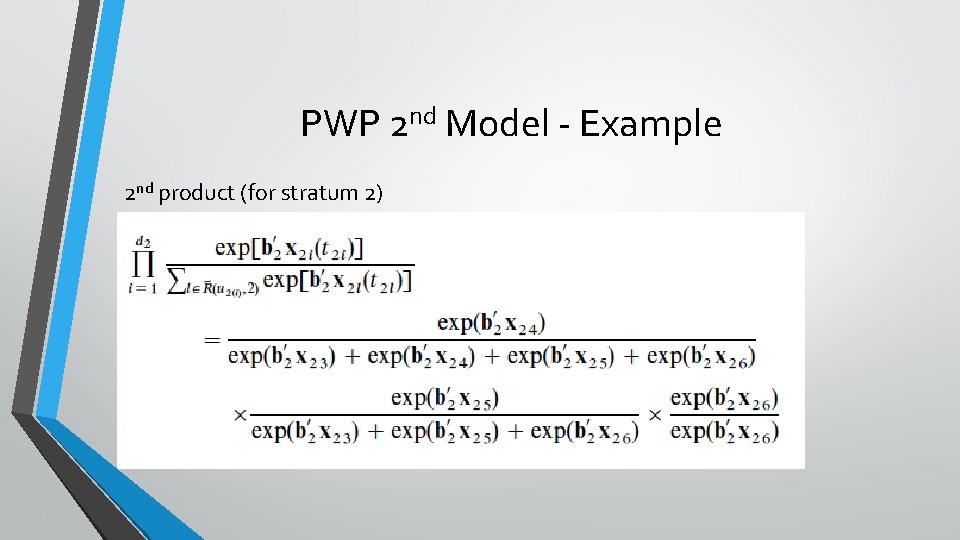
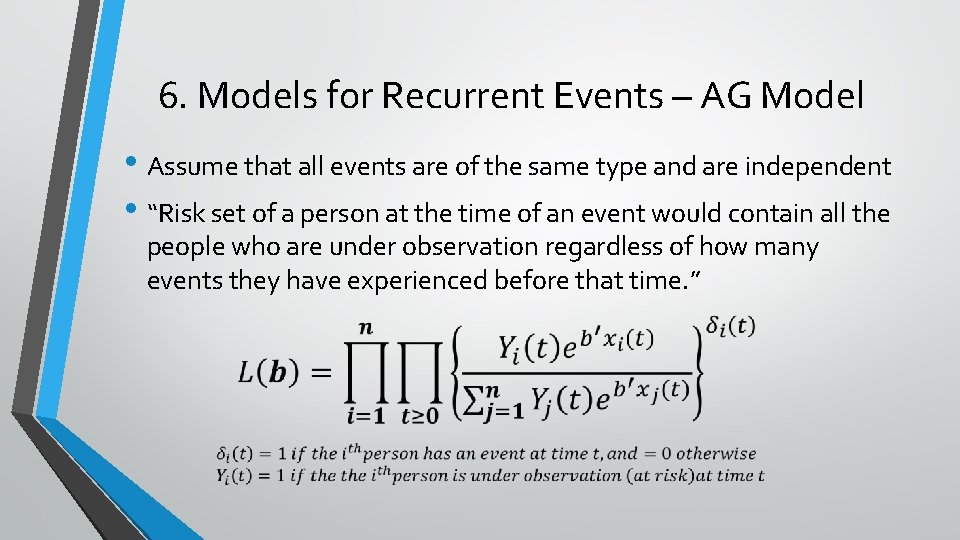
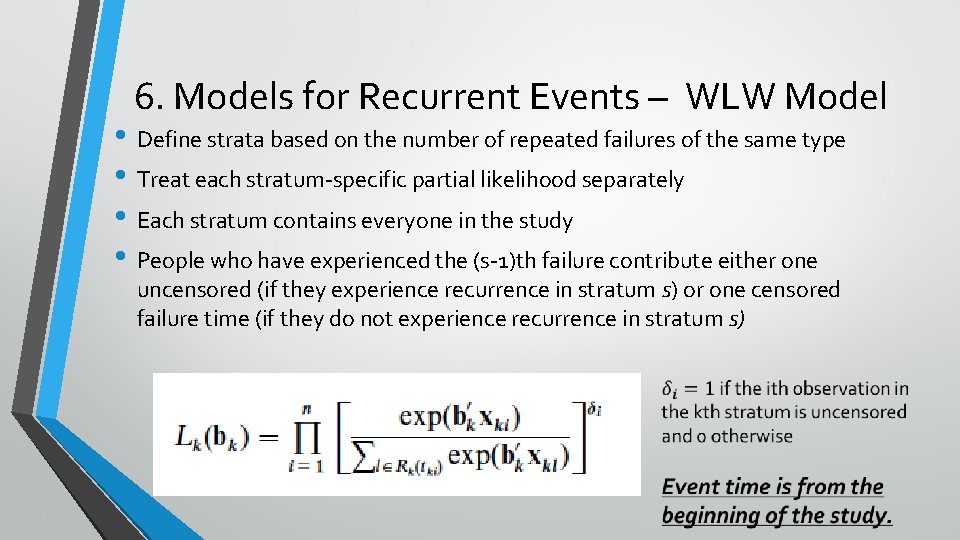
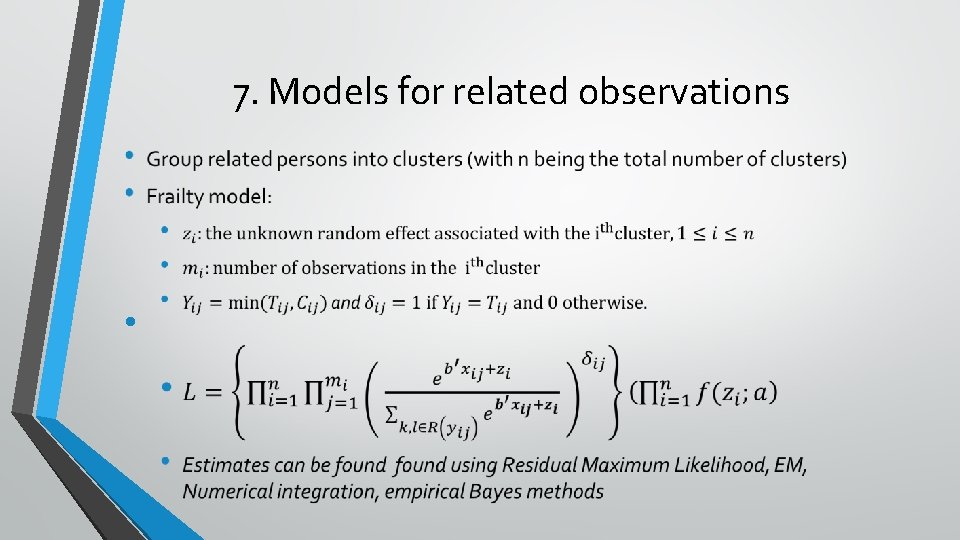
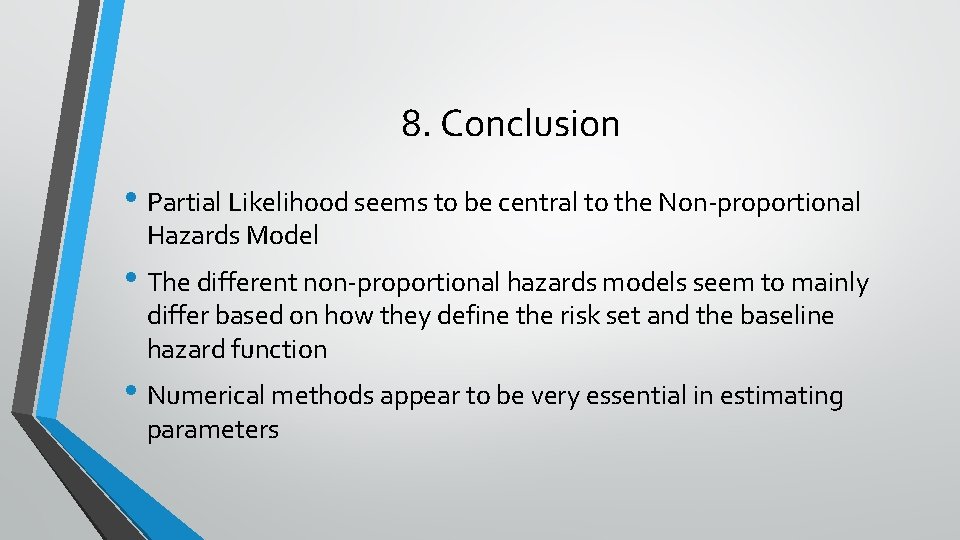
- Slides: 23
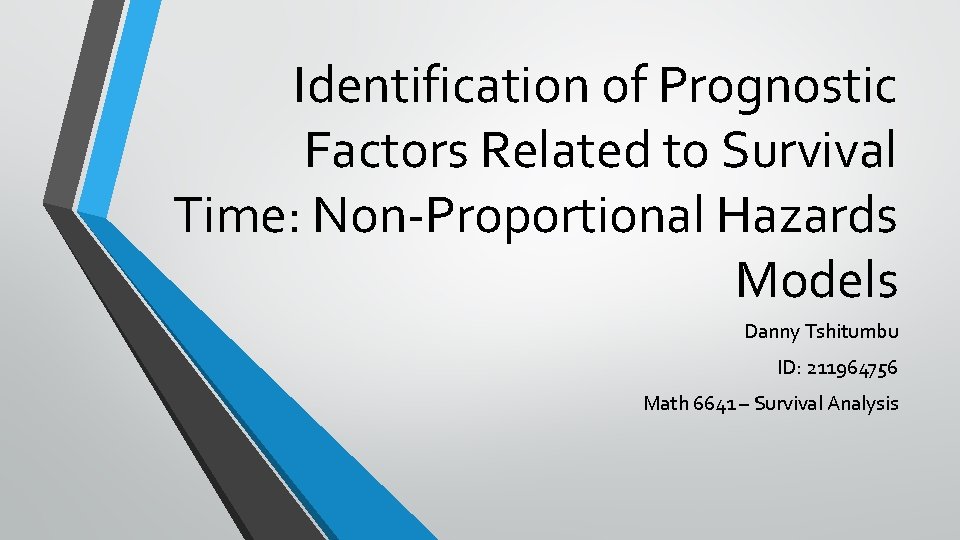
Identification of Prognostic Factors Related to Survival Time: Non-Proportional Hazards Models Danny Tshitumbu ID: 211964756 Math 6641 – Survival Analysis
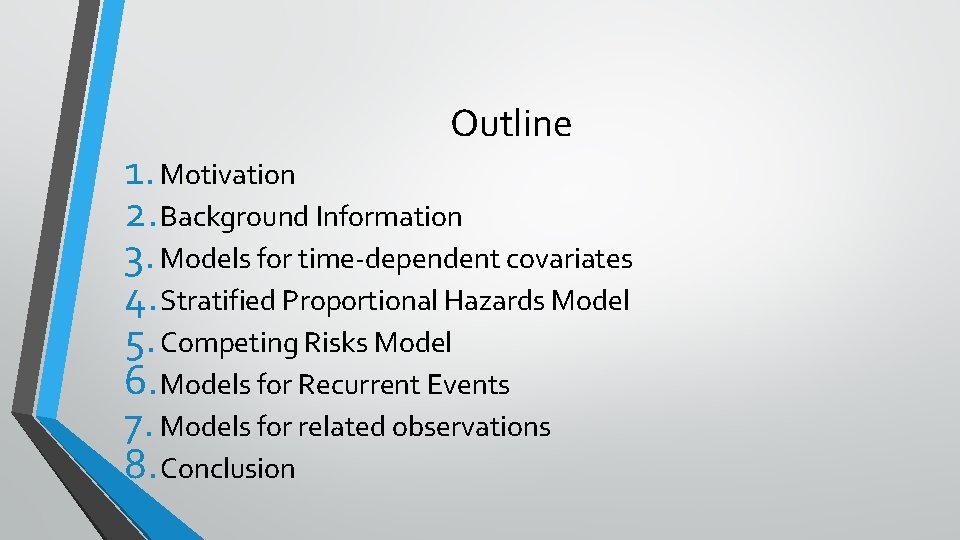
Outline 1. Motivation 2. Background Information 3. Models for time-dependent covariates 4. Stratified Proportional Hazards Model 5. Competing Risks Model 6. Models for Recurrent Events 7. Models for related observations 8. Conclusion
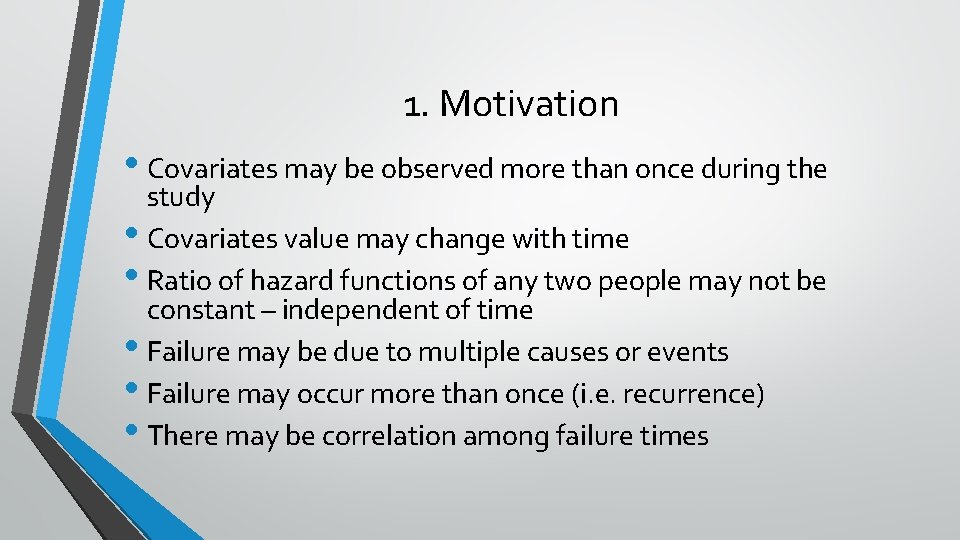
1. Motivation • Covariates may be observed more than once during the study • Covariates value may change with time • Ratio of hazard functions of any two people may not be constant – independent of time • Failure may be due to multiple causes or events • Failure may occur more than once (i. e. recurrence) • There may be correlation among failure times
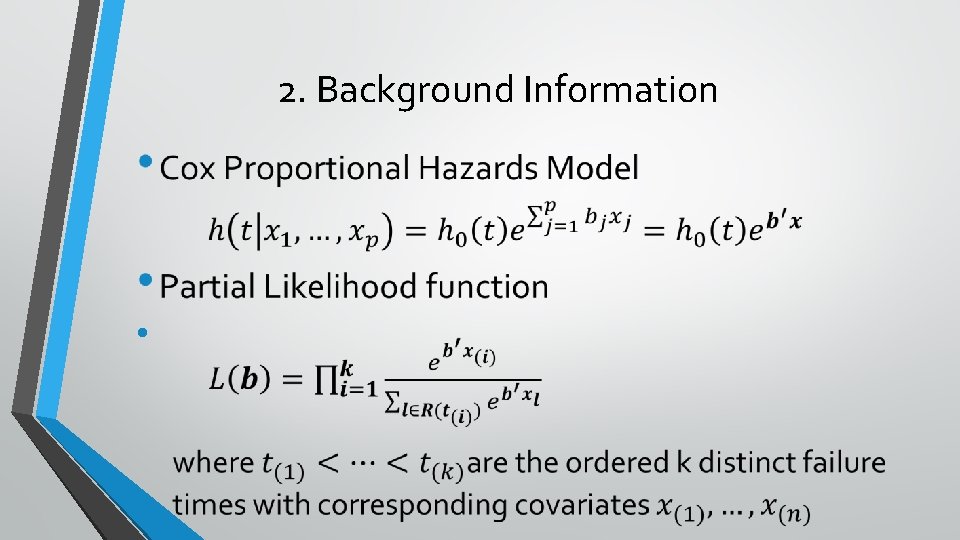
2. Background Information •
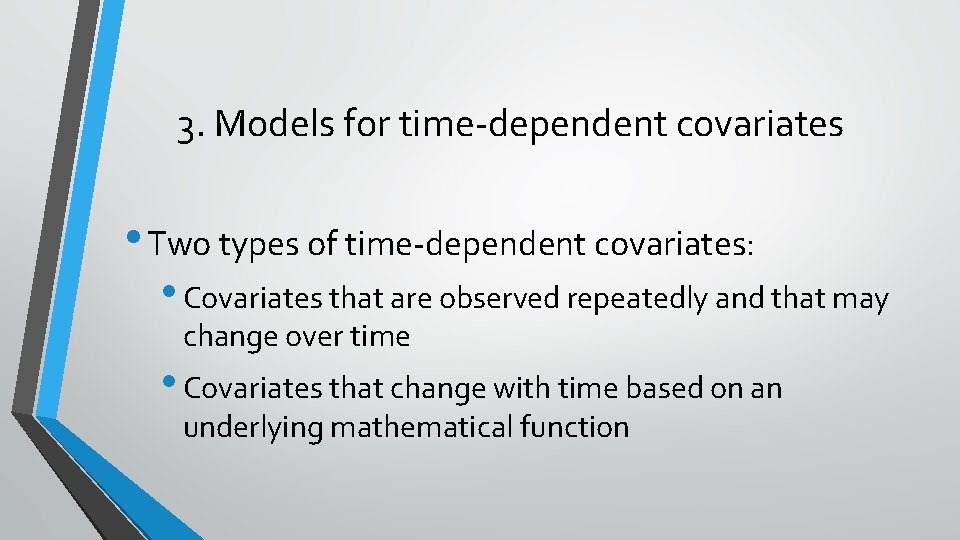
3. Models for time-dependent covariates • Two types of time-dependent covariates: • Covariates that are observed repeatedly and that may change over time • Covariates that change with time based on an underlying mathematical function
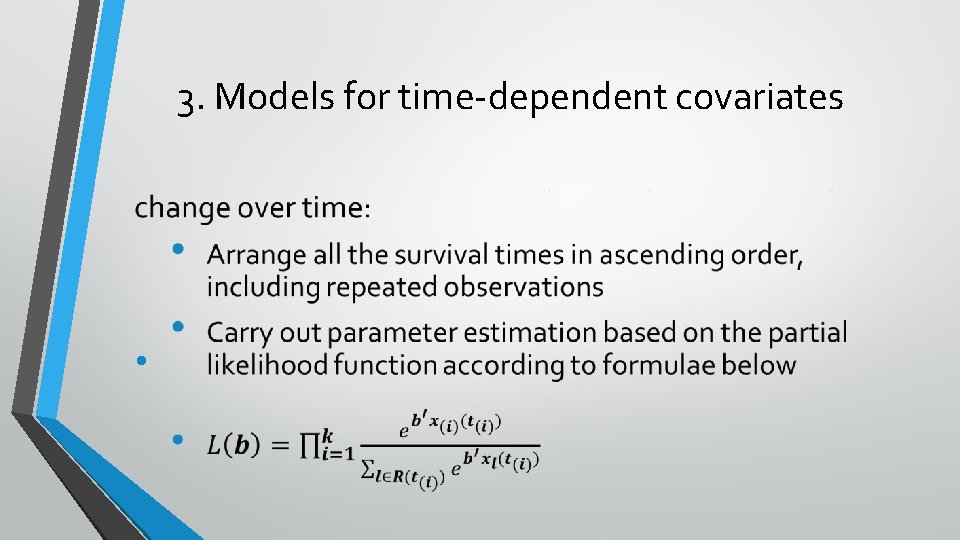
3. Models for time-dependent covariates •
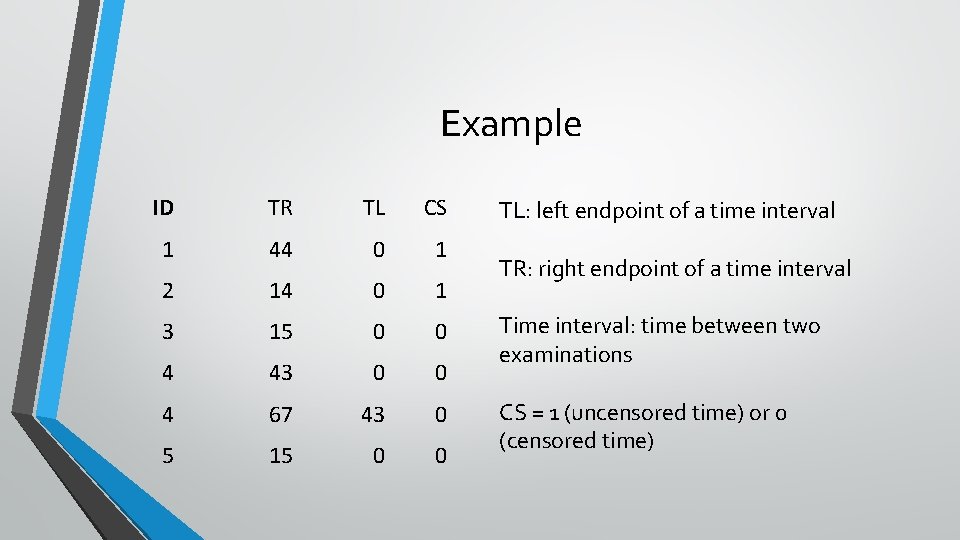
Example ID TR TL CS 1 44 0 1 2 14 0 1 3 15 0 0 4 43 0 0 4 67 43 0 5 15 0 0 TL: left endpoint of a time interval TR: right endpoint of a time interval Time interval: time between two examinations CS = 1 (uncensored time) or 0 (censored time)
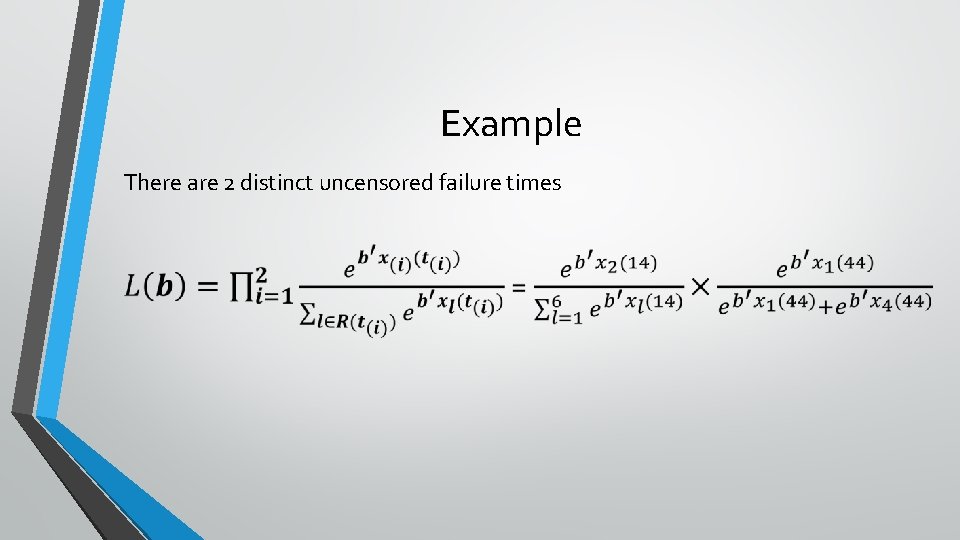
Example There are 2 distinct uncensored failure times
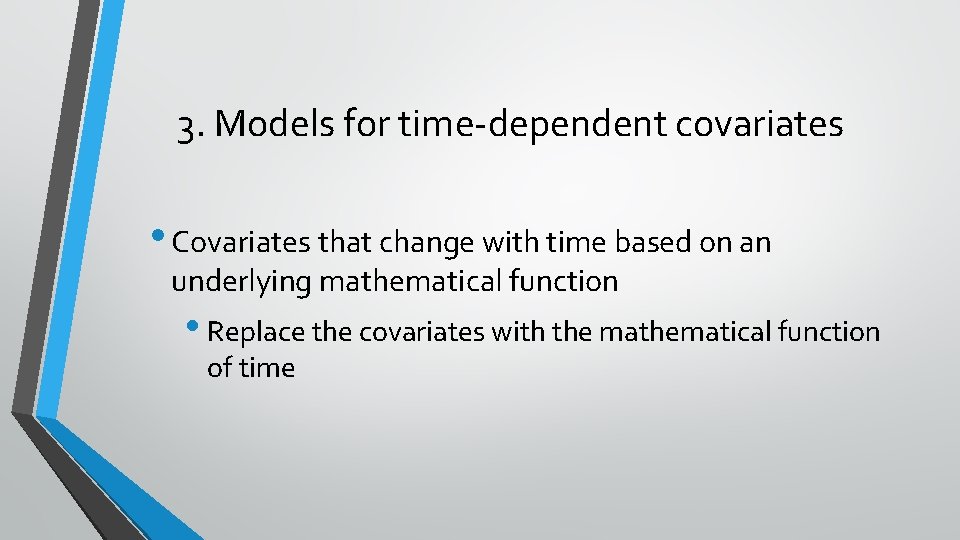
3. Models for time-dependent covariates • Covariates that change with time based on an underlying mathematical function • Replace the covariates with the mathematical function of time
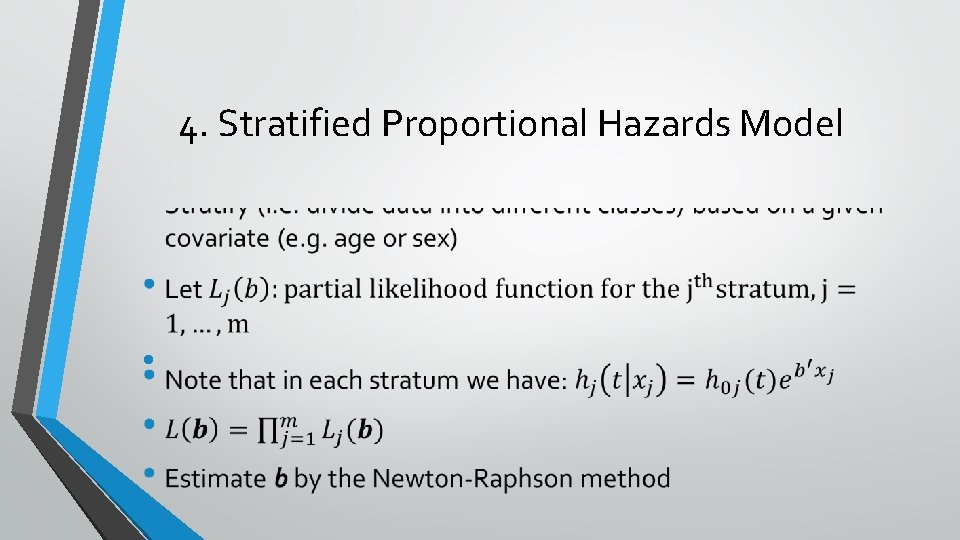
4. Stratified Proportional Hazards Model •
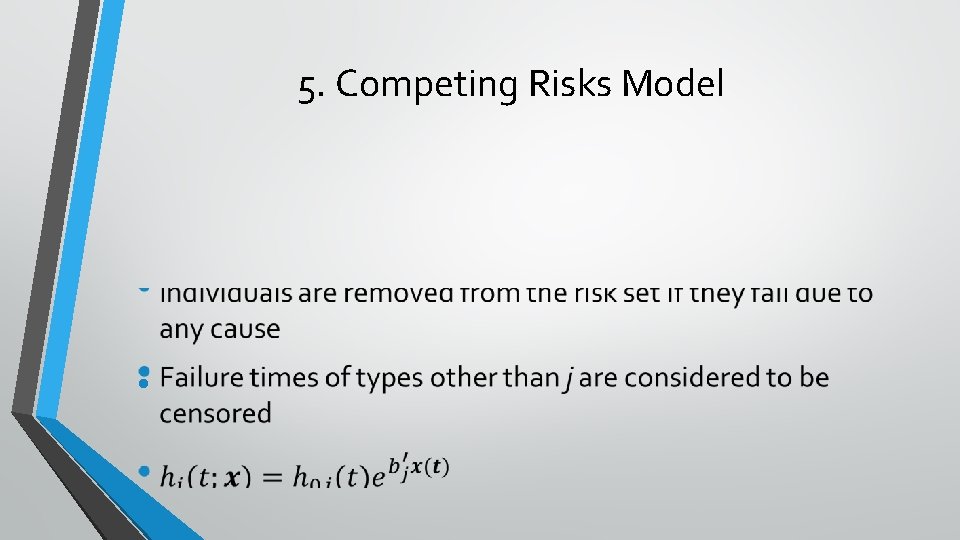
5. Competing Risks Model •
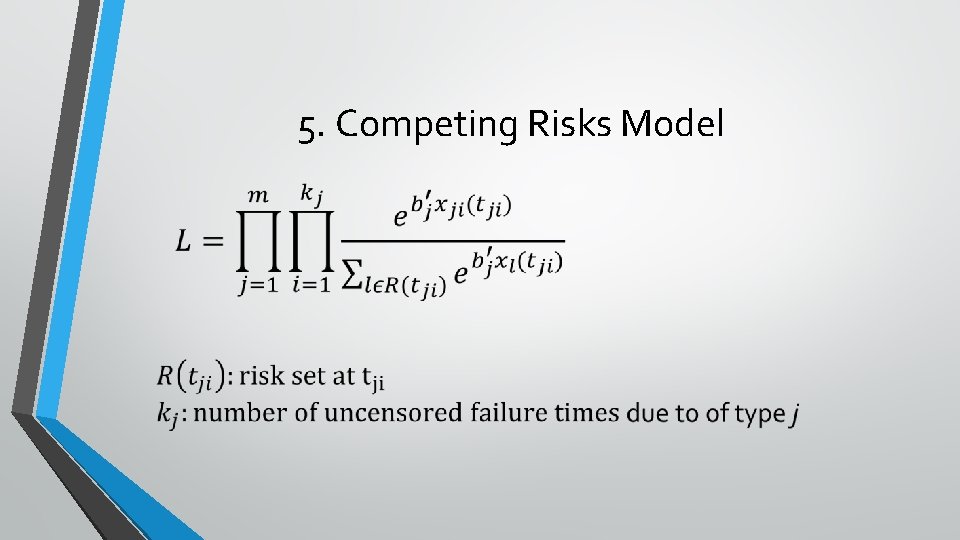
5. Competing Risks Model
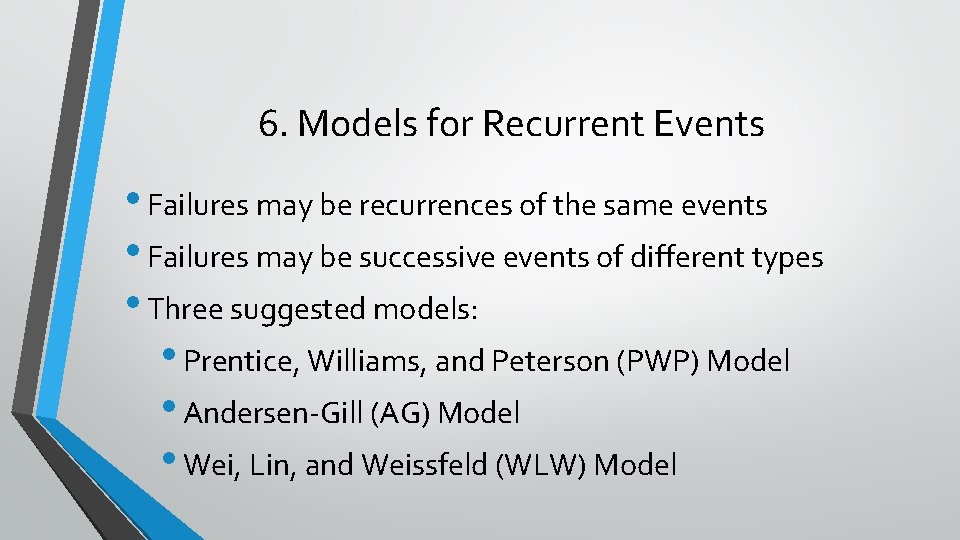
6. Models for Recurrent Events • Failures may be recurrences of the same events • Failures may be successive events of different types • Three suggested models: • Prentice, Williams, and Peterson (PWP) Model • Andersen-Gill (AG) Model • Wei, Lin, and Weissfeld (WLW) Model
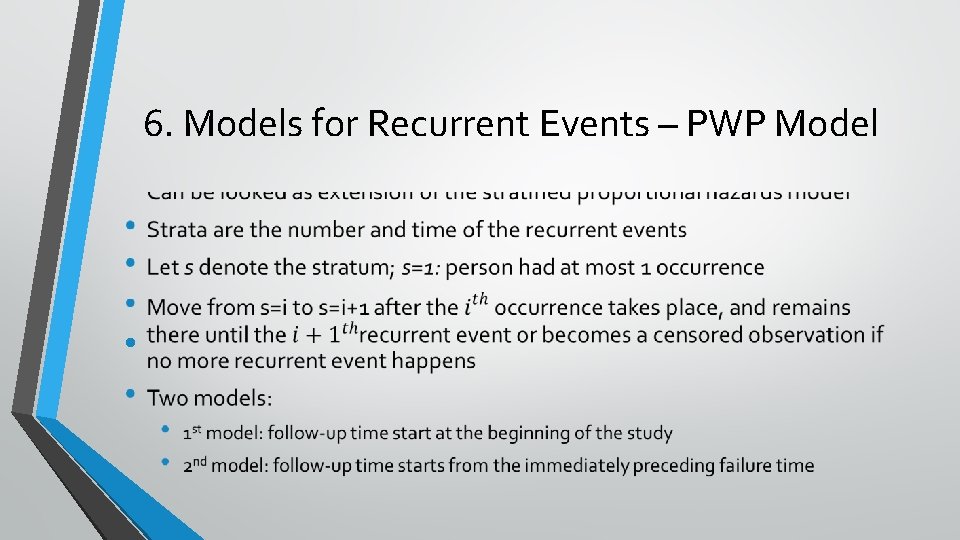
6. Models for Recurrent Events – PWP Model •
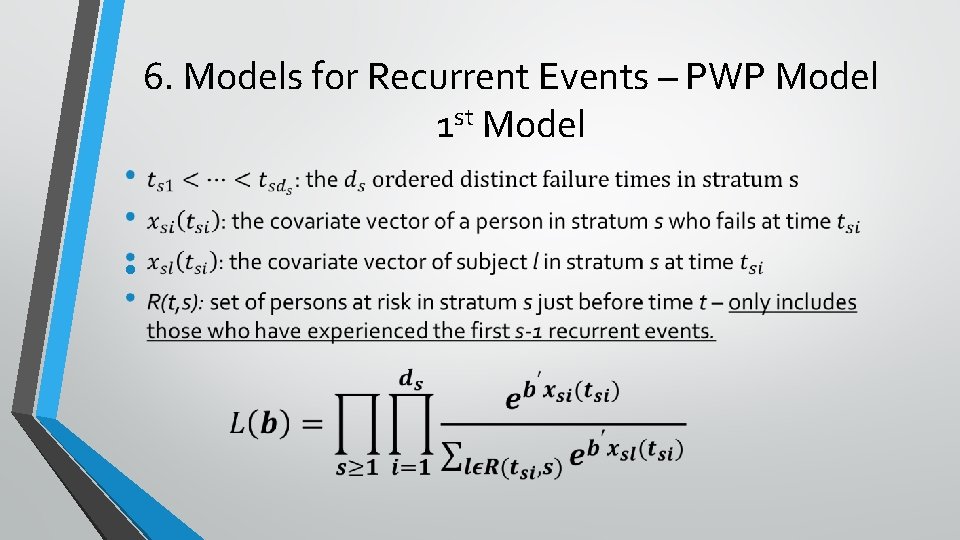
6. Models for Recurrent Events – PWP Model 1 st Model •
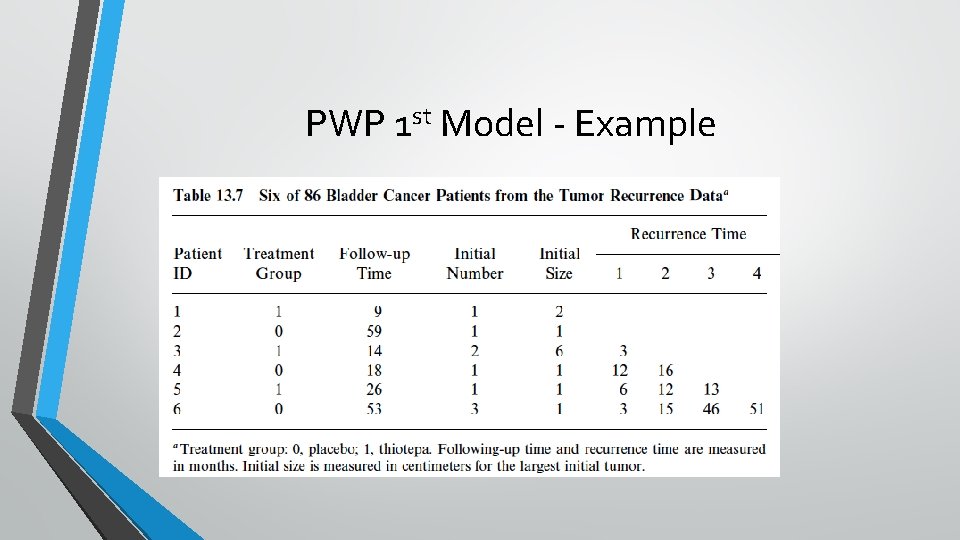
PWP 1 st Model - Example
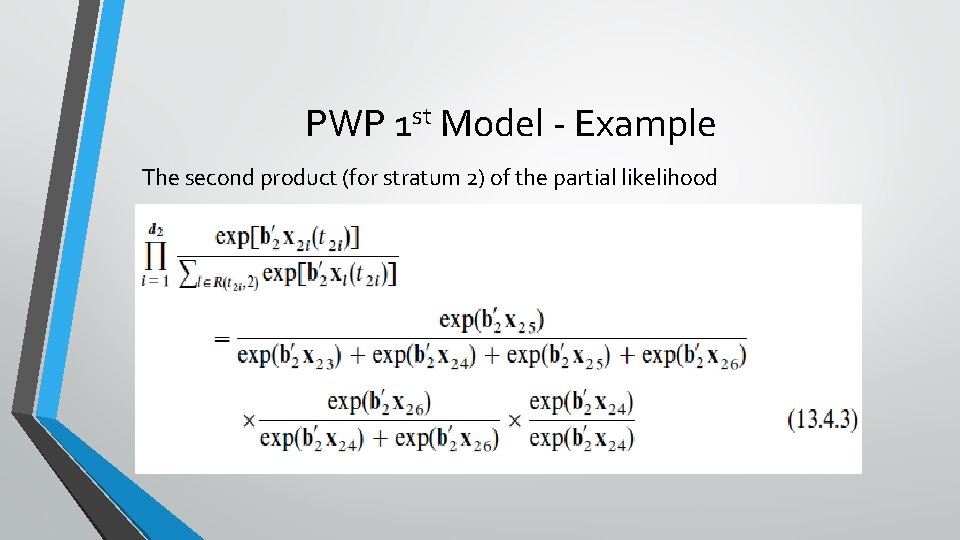
PWP 1 st Model - Example The second product (for stratum 2) of the partial likelihood
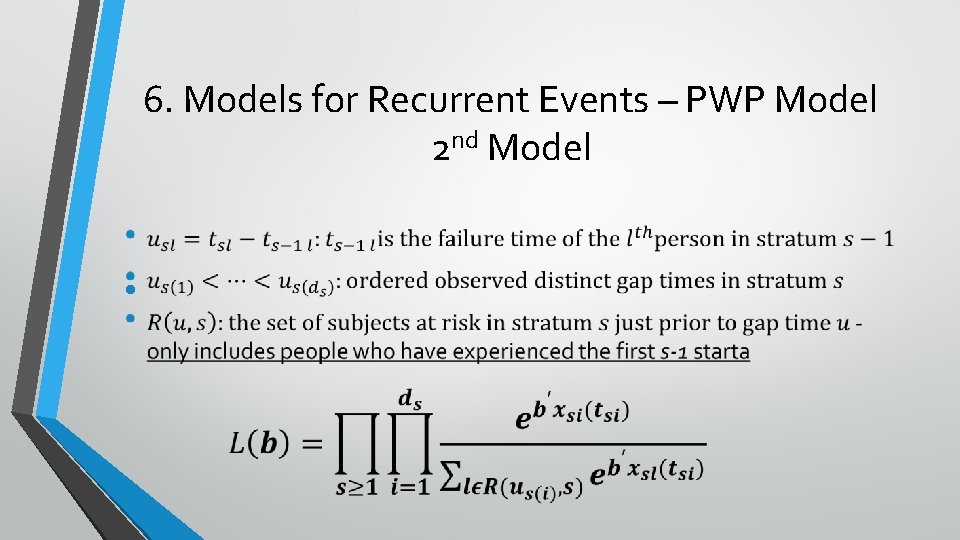
6. Models for Recurrent Events – PWP Model 2 nd Model •
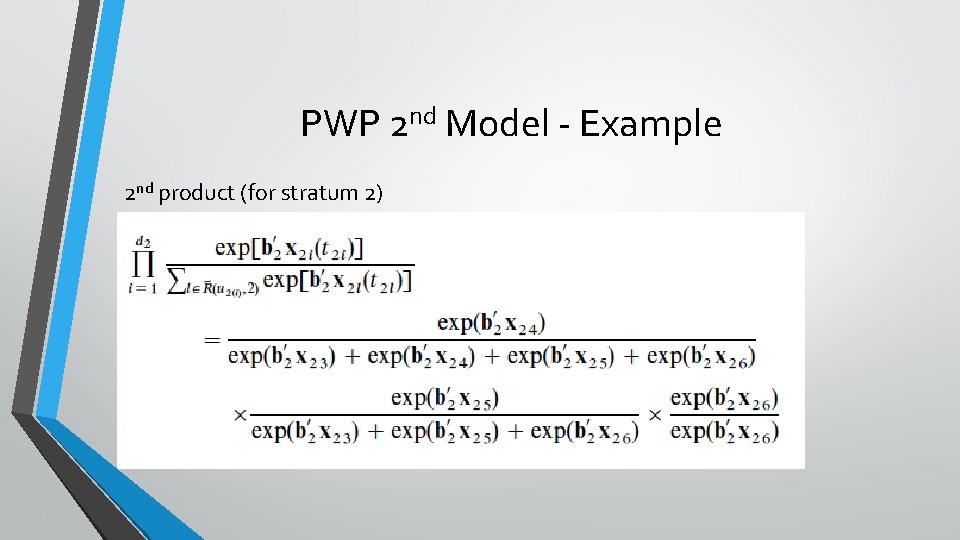
PWP 2 nd Model - Example 2 nd product (for stratum 2)
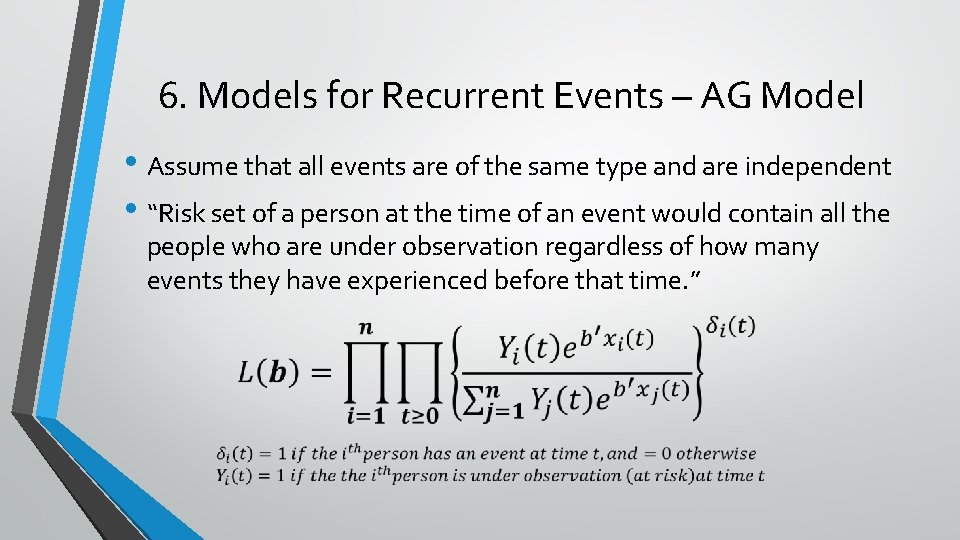
6. Models for Recurrent Events – AG Model • Assume that all events are of the same type and are independent • “Risk set of a person at the time of an event would contain all the people who are under observation regardless of how many events they have experienced before that time. ”
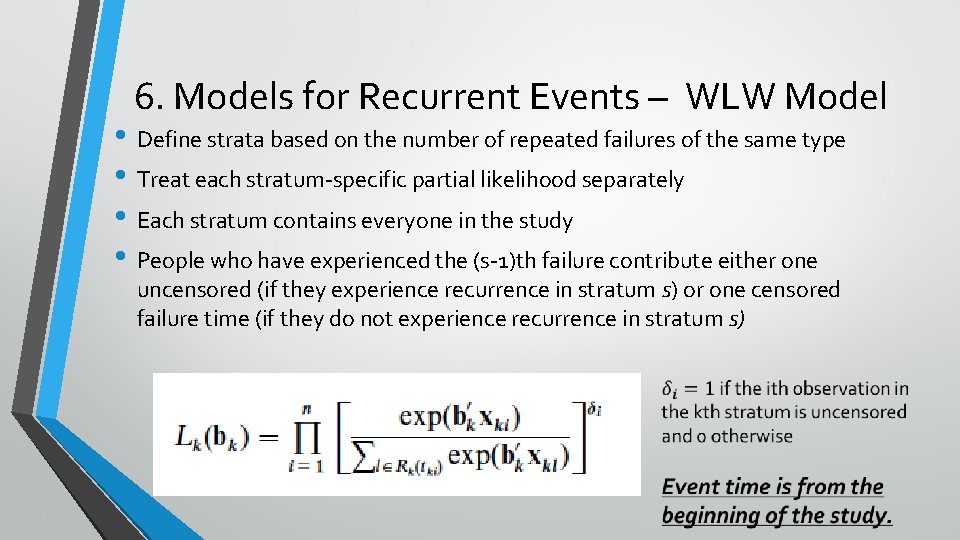
6. Models for Recurrent Events – WLW Model • Define strata based on the number of repeated failures of the same type • Treat each stratum-specific partial likelihood separately • Each stratum contains everyone in the study • People who have experienced the (s-1)th failure contribute either one uncensored (if they experience recurrence in stratum s) or one censored failure time (if they do not experience recurrence in stratum s)
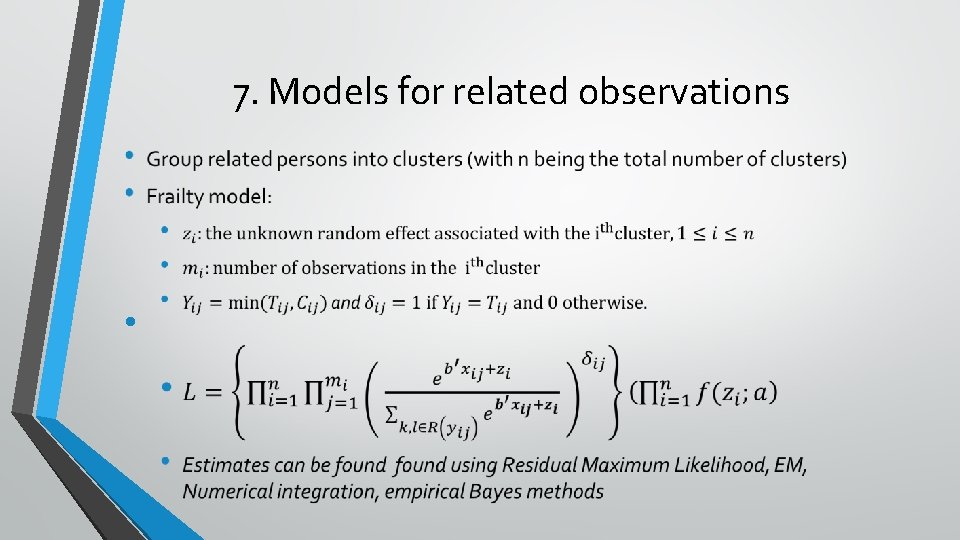
7. Models for related observations •
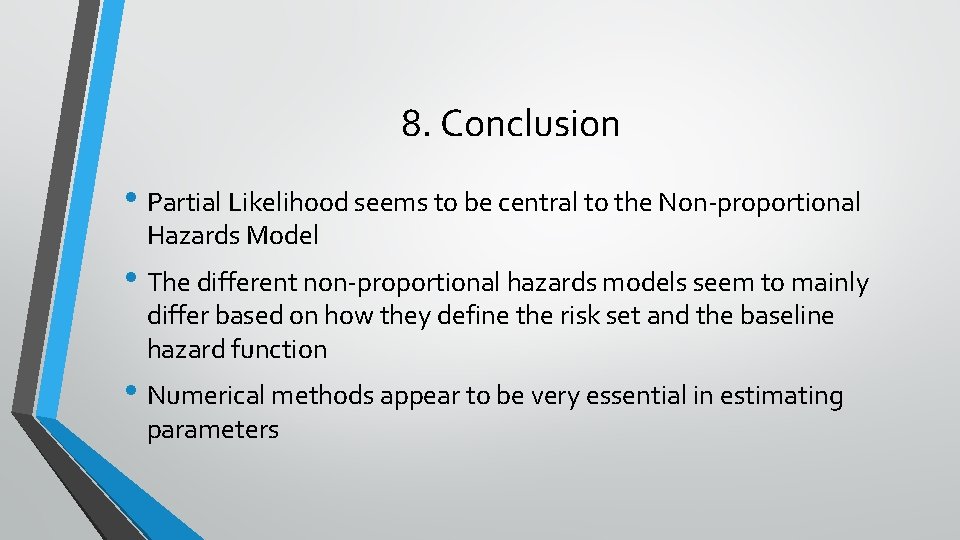
8. Conclusion • Partial Likelihood seems to be central to the Non-proportional Hazards Model • The different non-proportional hazards models seem to mainly differ based on how they define the risk set and the baseline hazard function • Numerical methods appear to be very essential in estimating parameters
State of survival survival of the fittest tweak
State of survival survival of the fittest stages
Central pocket vs plain whorl
2 types of fitness
Skill related fitness
Surface prog chart
Who prognostic scoring system gtn
Who prognostic scoring system gtn
Choriocarcinoma staging figo
Palliative prognostic index
Molar pregnancy karyotype
Van nuys grading system
Concept map of measurement assessment and evaluation
Start time end time and elapsed time
General observation schedule pe
Site vs situation examples
Abiotic
Abiotic vs biotic factors
Abiotic factors and biotic factors
Is a tulip abiotic or biotic
Location
Common factors of 10 and 25
Factors of 7
Factos of 8