FIMM Institiute for Molecular Medicine Finland www fimm
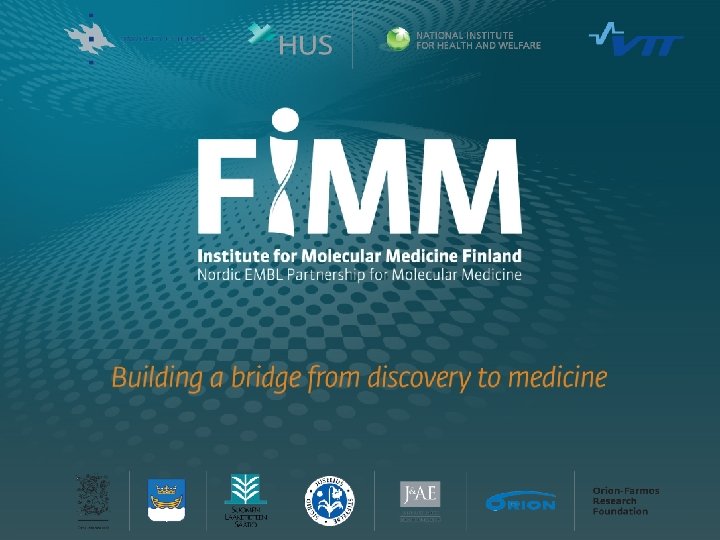
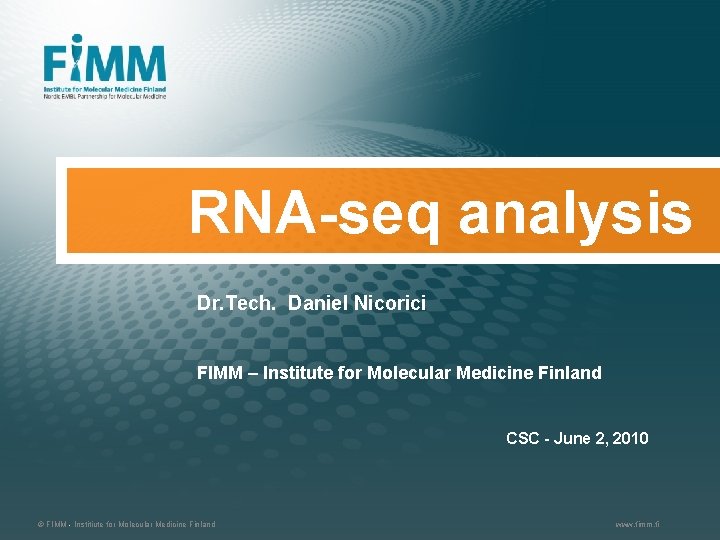
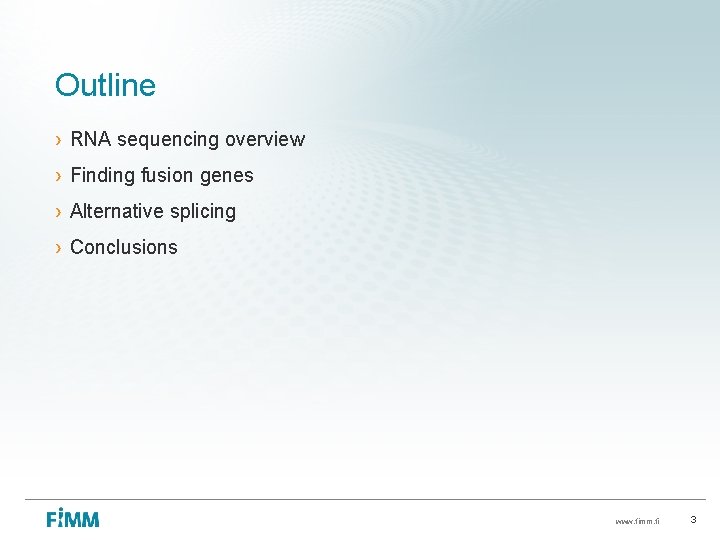
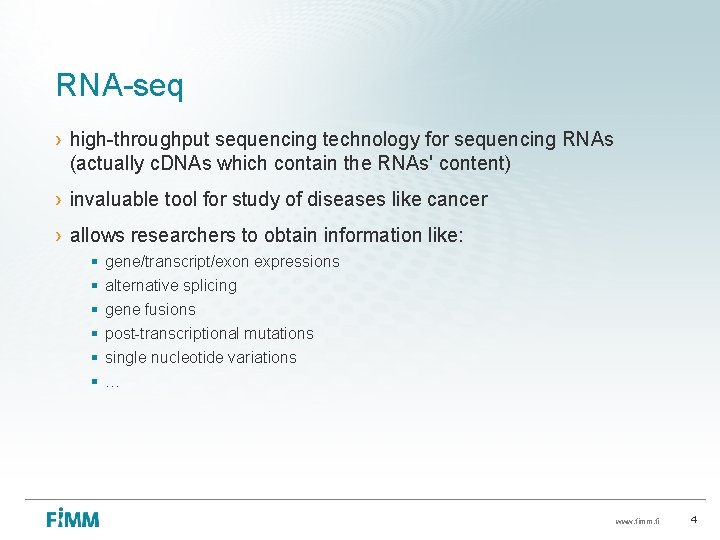
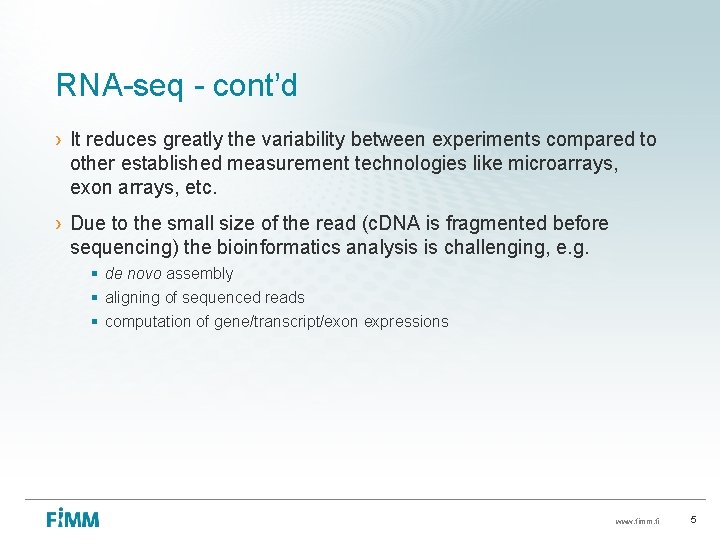
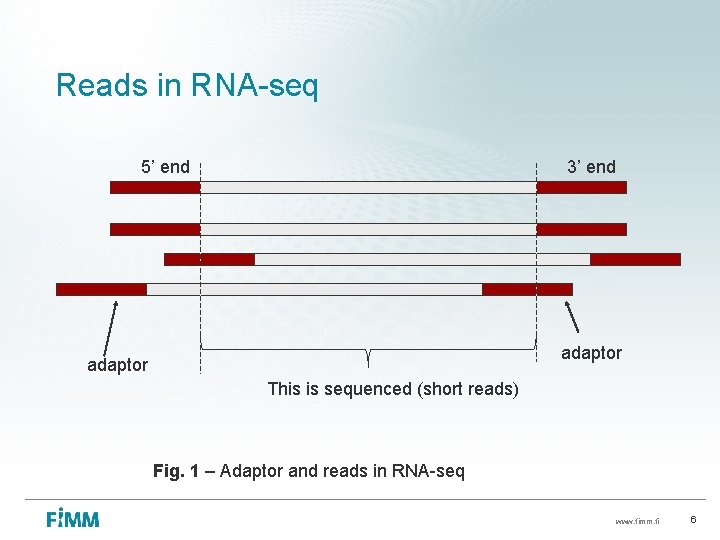
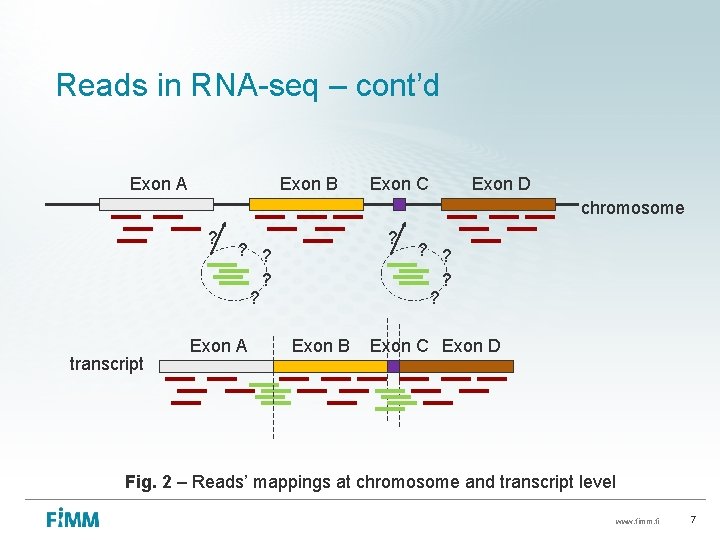
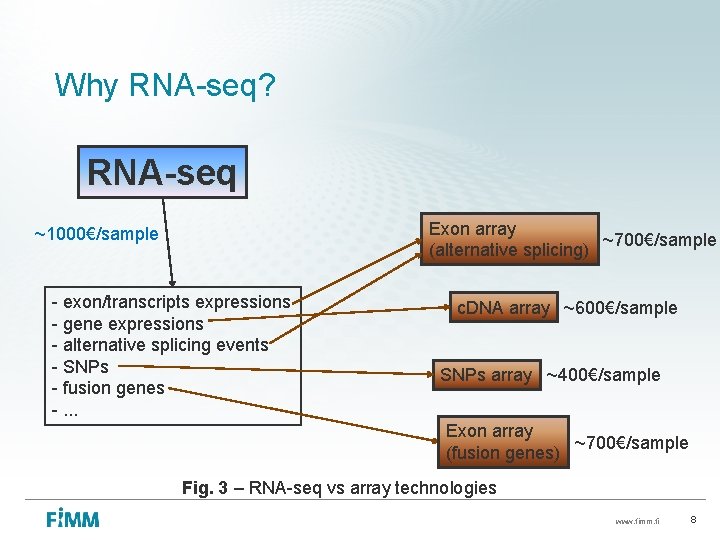
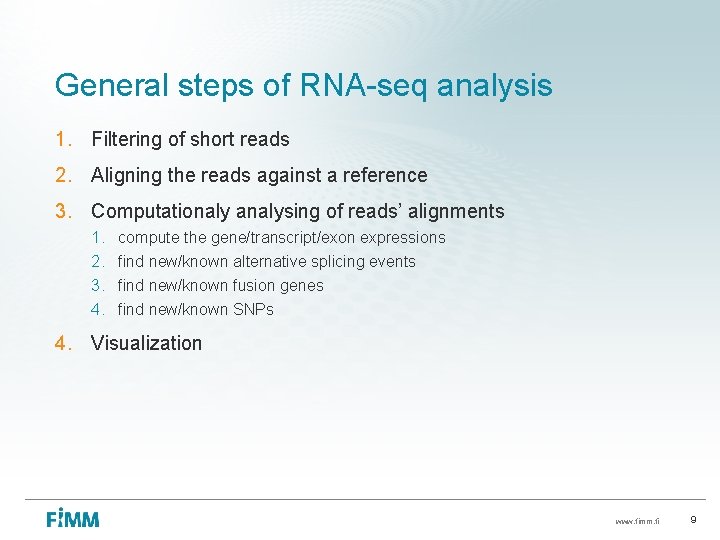
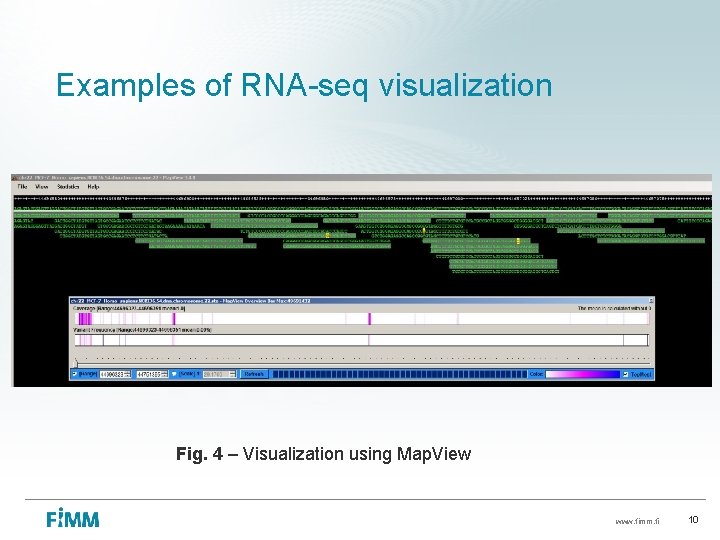
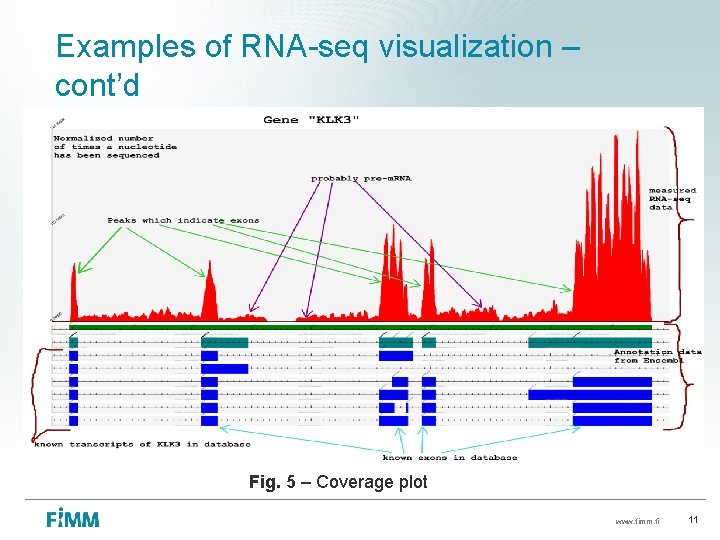
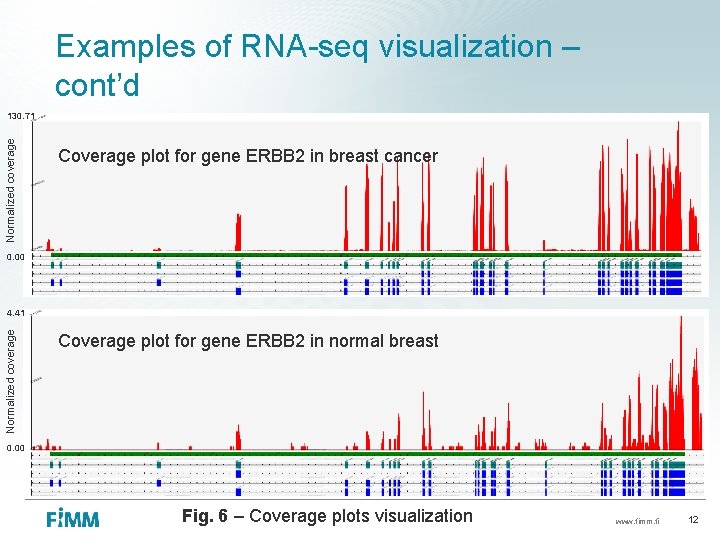
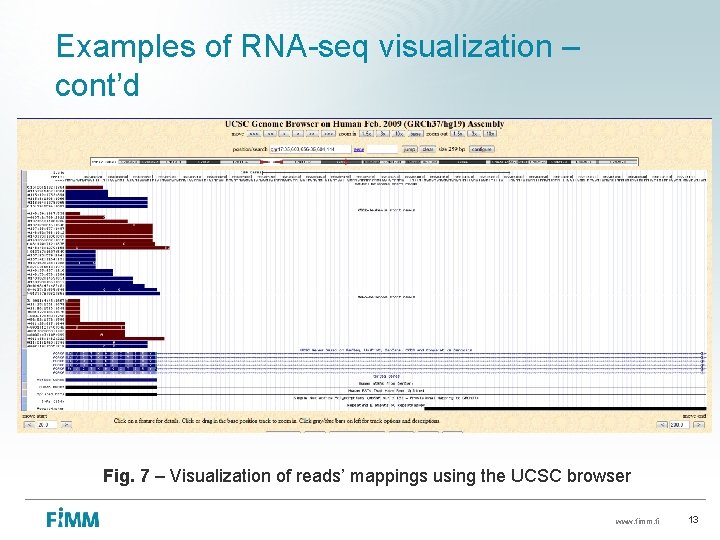
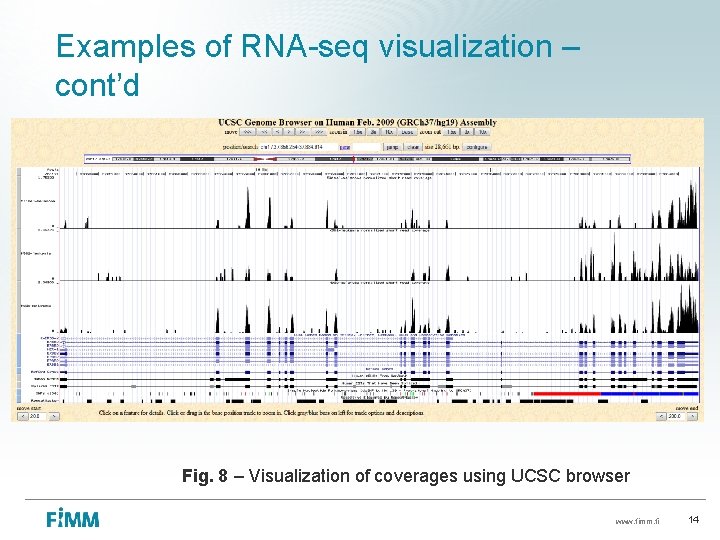
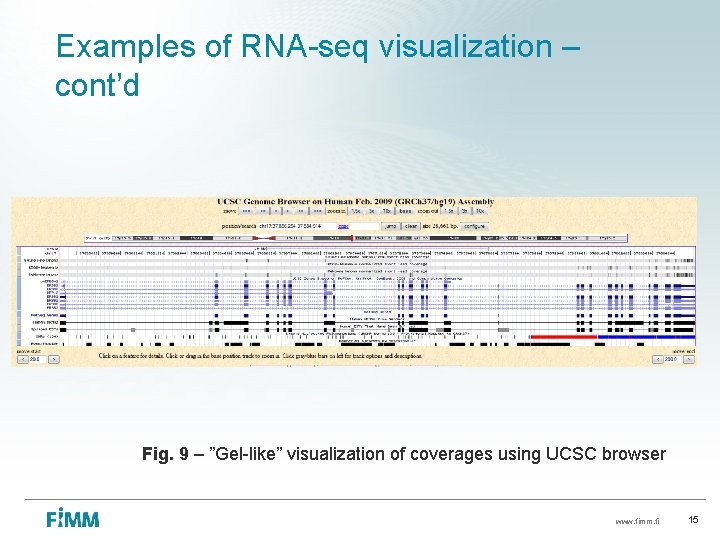
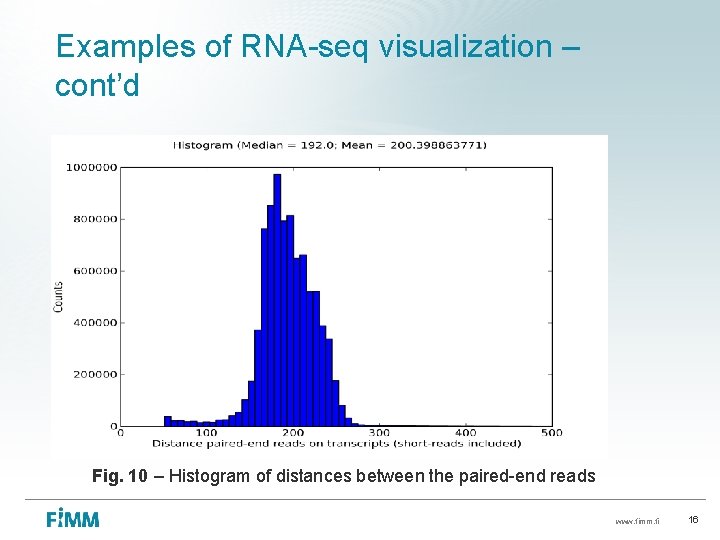
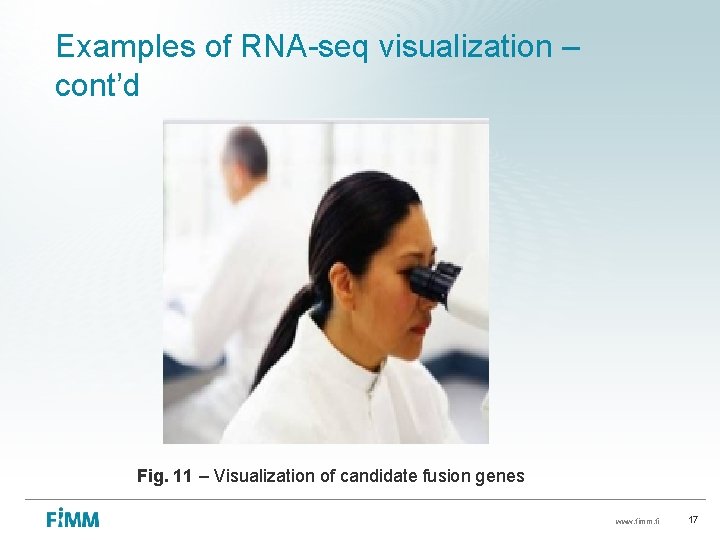
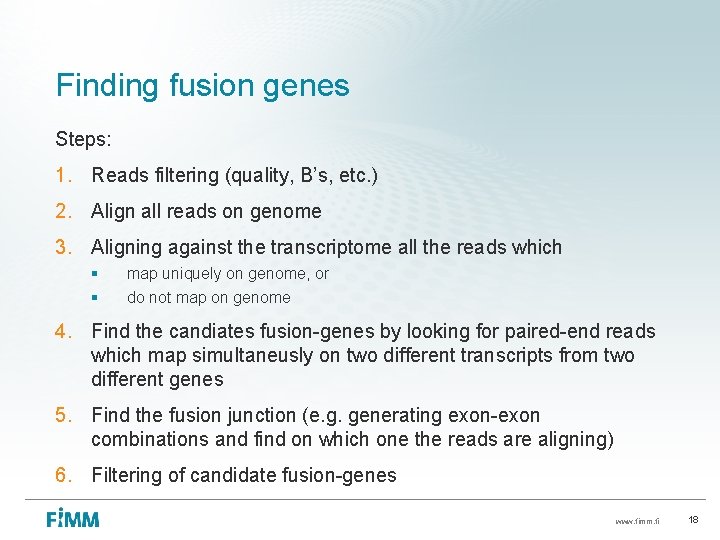
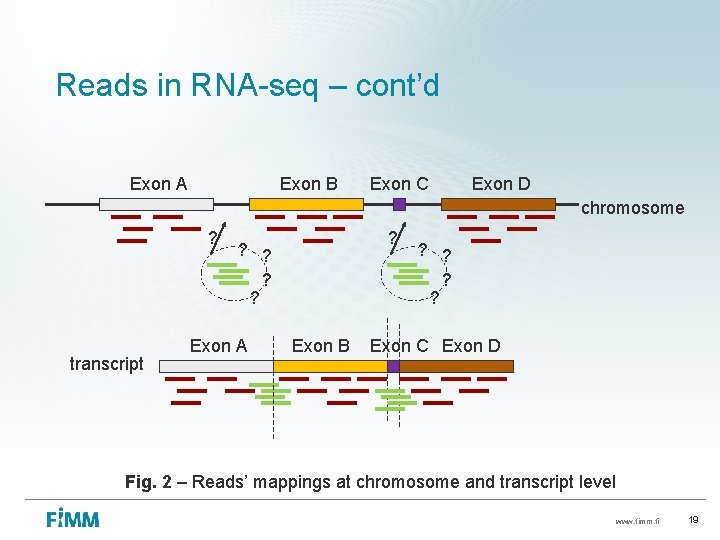
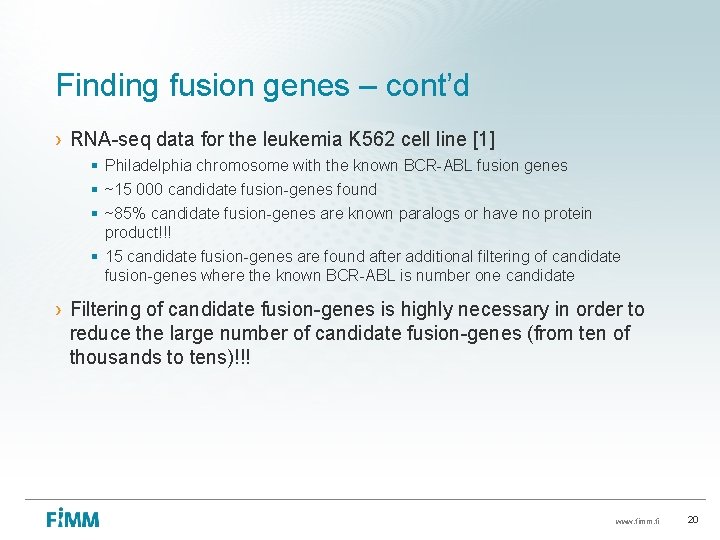
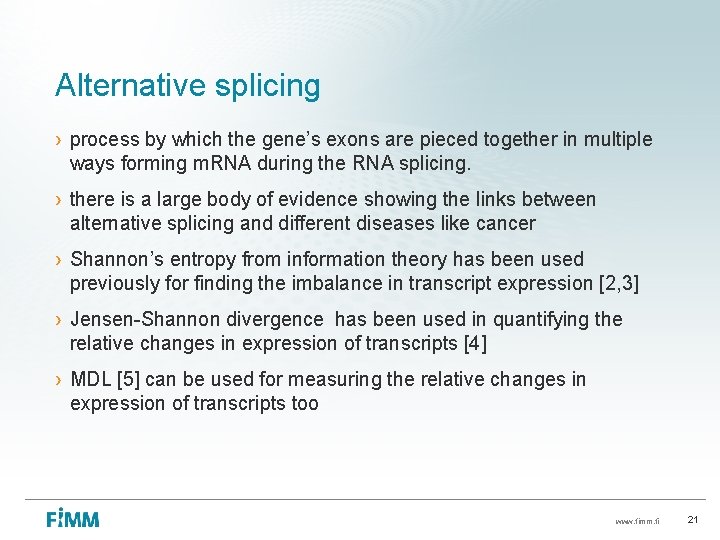
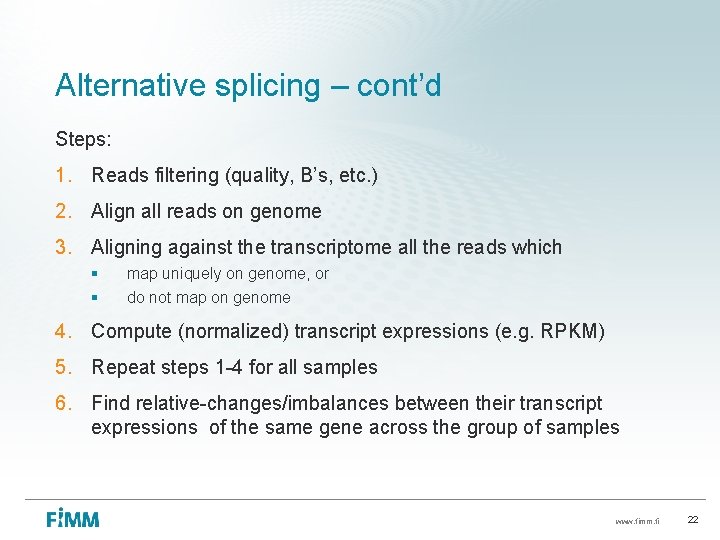
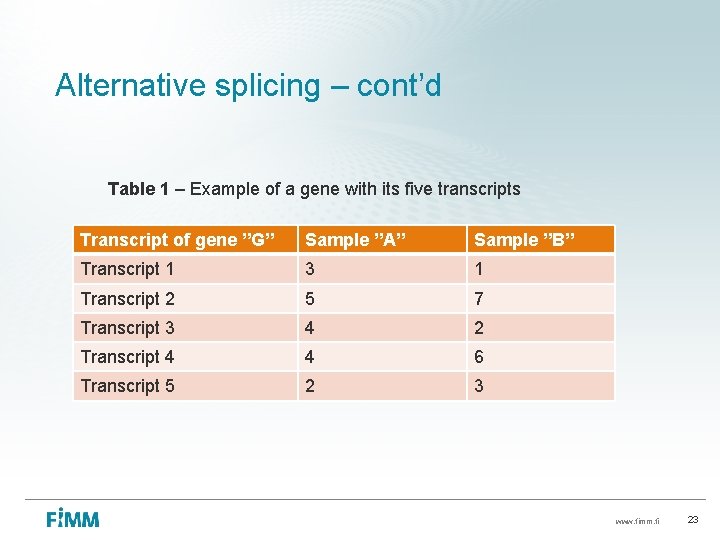
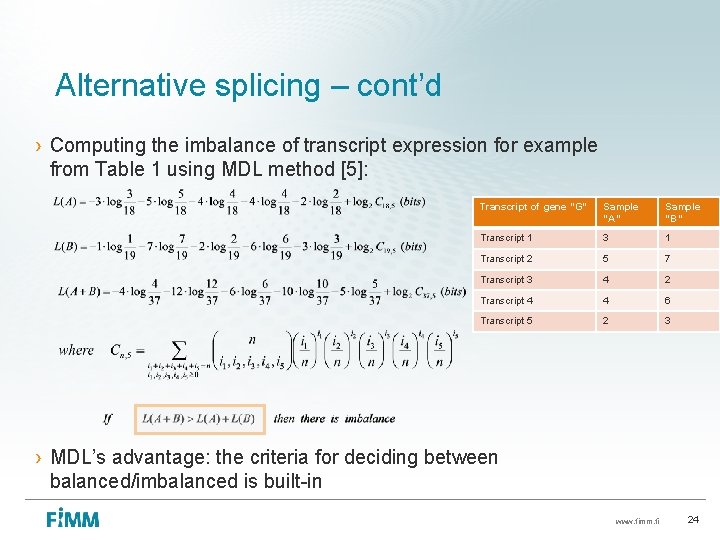
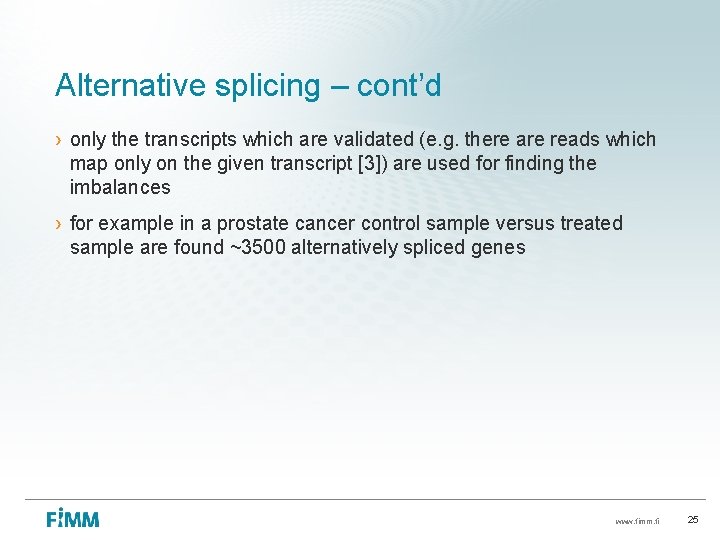
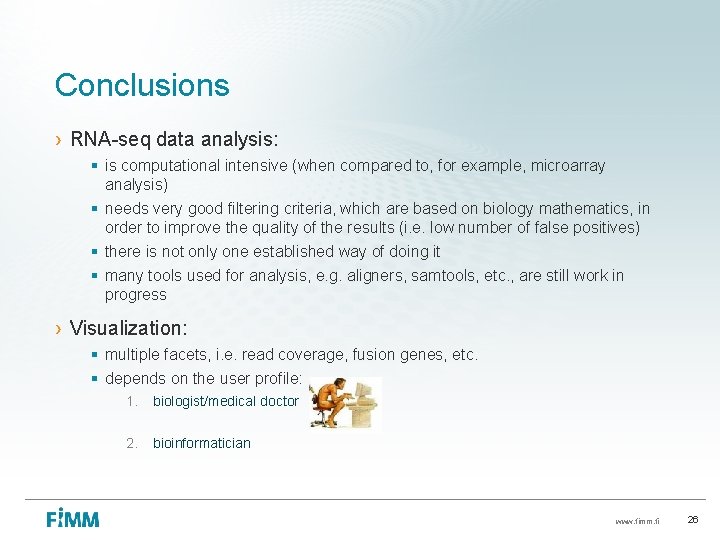
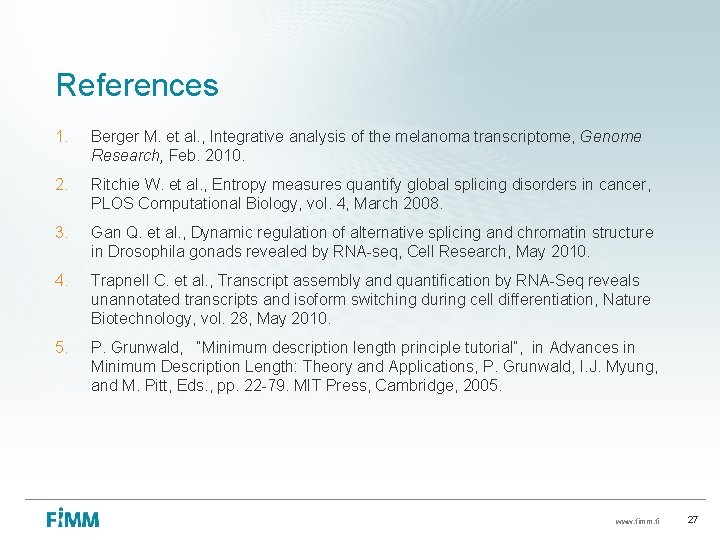
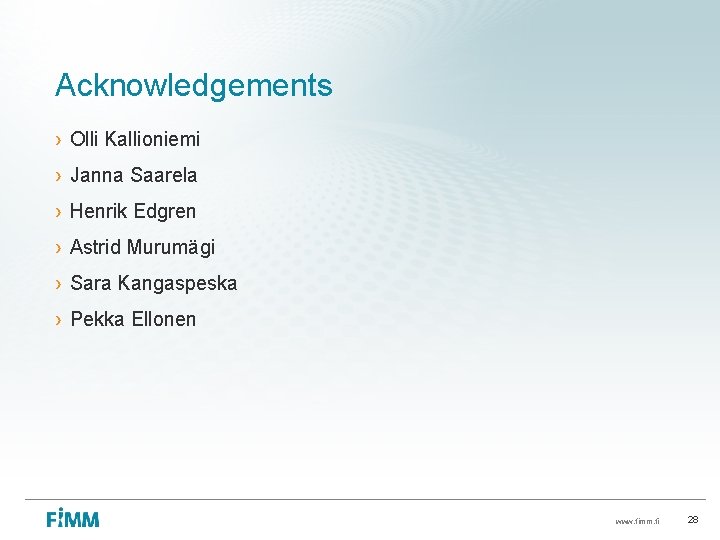
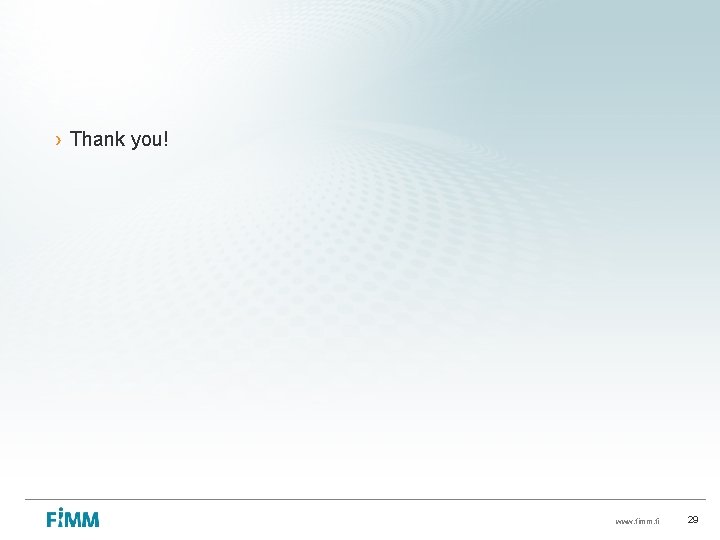
- Slides: 29
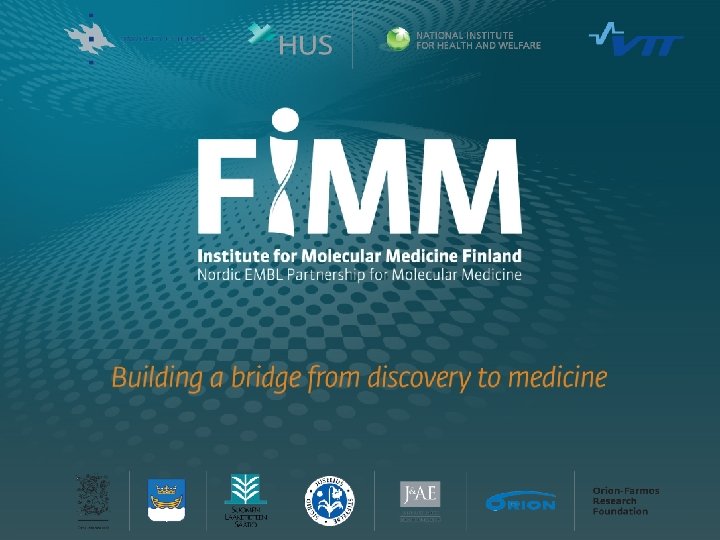
© FIMM - Institiute for Molecular Medicine Finland www. fimm. fi
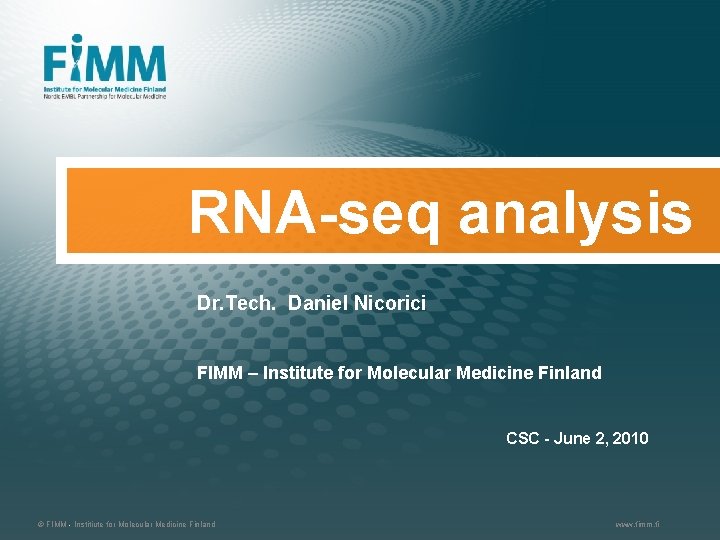
RNA-seq analysis Dr. Tech. Daniel Nicorici FIMM – Institute for Molecular Medicine Finland CSC - June 2, 2010 © FIMM - Institiute for Molecular Medicine Finland www. fimm. fi
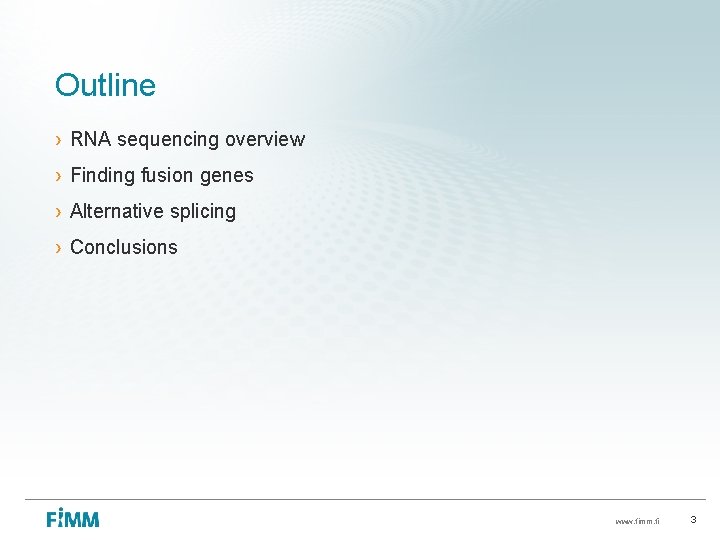
Outline › RNA sequencing overview › Finding fusion genes › Alternative splicing › Conclusions www. fimm. fi 3
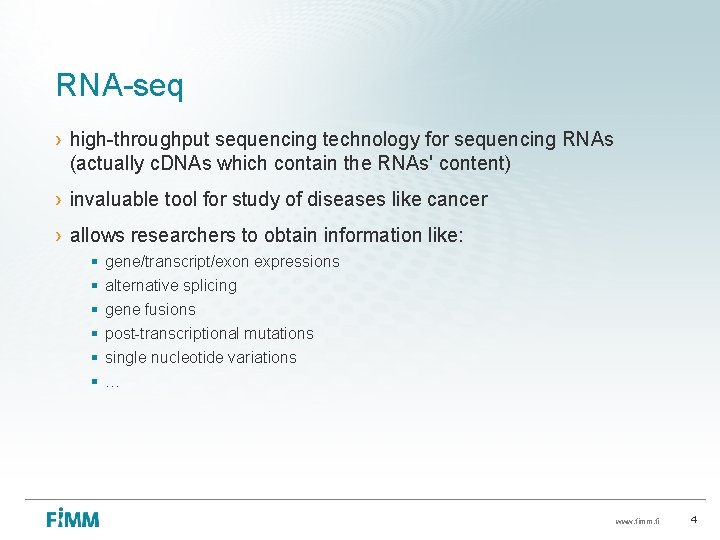
RNA-seq › high-throughput sequencing technology for sequencing RNAs (actually c. DNAs which contain the RNAs' content) › invaluable tool for study of diseases like cancer › allows researchers to obtain information like: § § § gene/transcript/exon expressions alternative splicing gene fusions post-transcriptional mutations single nucleotide variations … www. fimm. fi 4
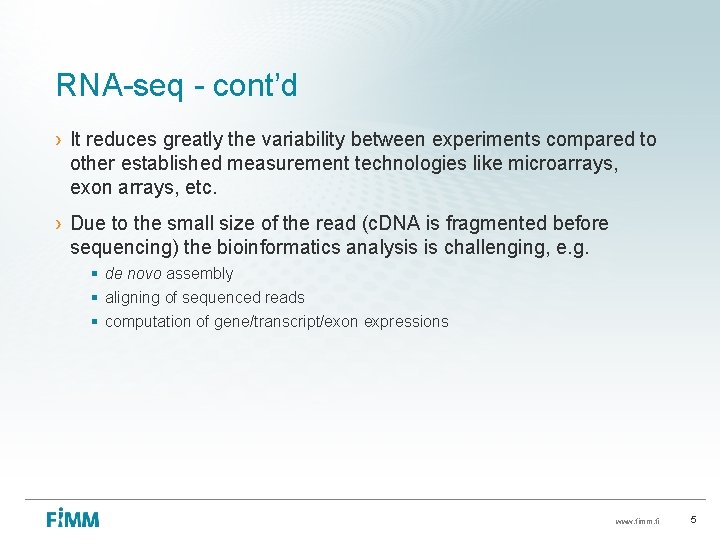
RNA-seq - cont’d › It reduces greatly the variability between experiments compared to other established measurement technologies like microarrays, exon arrays, etc. › Due to the small size of the read (c. DNA is fragmented before sequencing) the bioinformatics analysis is challenging, e. g. § de novo assembly § aligning of sequenced reads § computation of gene/transcript/exon expressions www. fimm. fi 5
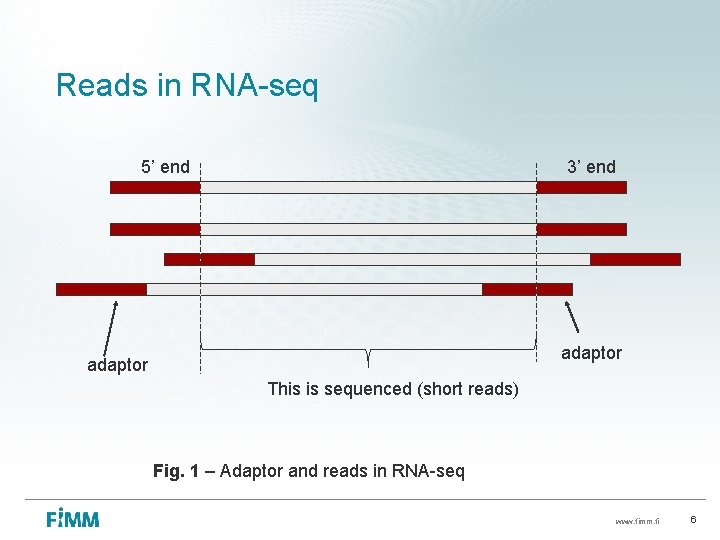
Reads in RNA-seq 5’ end 3’ end adaptor This is sequenced (short reads) Fig. 1 – Adaptor and reads in RNA-seq www. fimm. fi 6
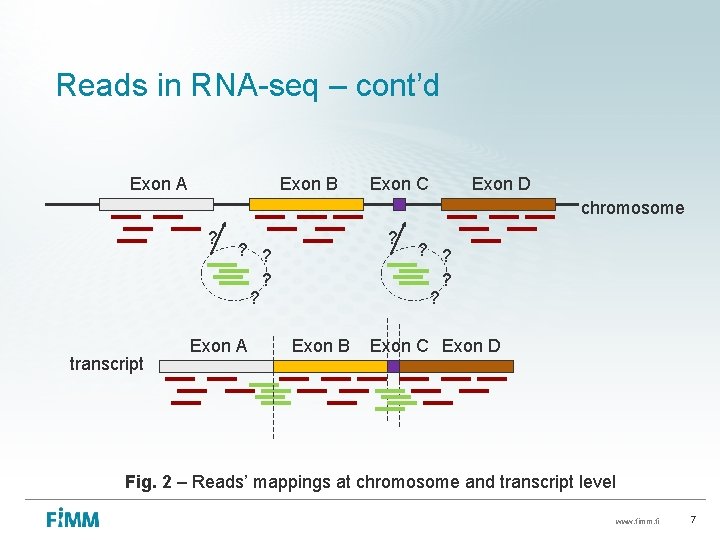
Reads in RNA-seq – cont’d Exon A Exon B Exon C Exon D chromosome ? transcript ? ? ? Exon A Exon B ? ? Exon C Exon D Fig. 2 – Reads’ mappings at chromosome and transcript level www. fimm. fi 7
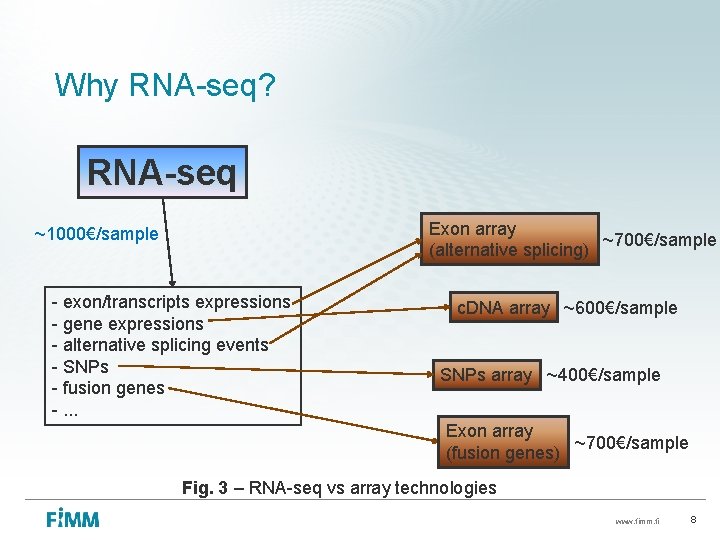
Why RNA-seq? RNA-seq Exon array ~700€/sample (alternative splicing) ~1000€/sample - exon/transcripts expressions - gene expressions - alternative splicing events - SNPs - fusion genes -. . . c. DNA array ~600€/sample SNPs array ~400€/sample Exon array ~700€/sample (fusion genes) Fig. 3 – RNA-seq vs array technologies www. fimm. fi 8
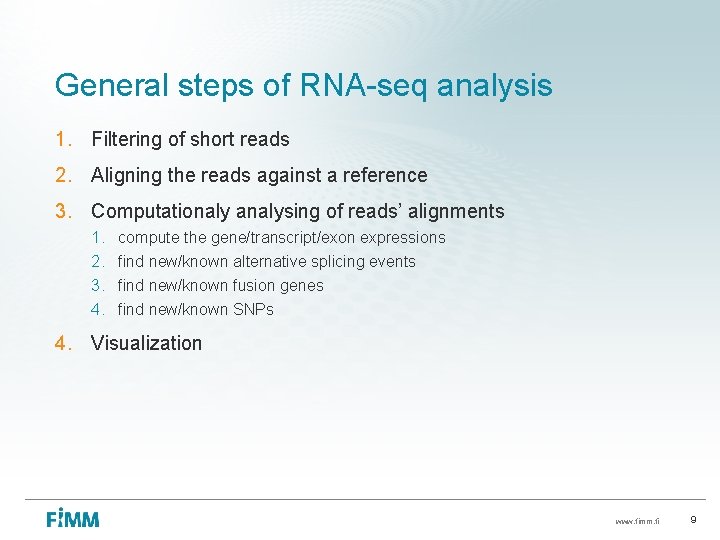
General steps of RNA-seq analysis 1. Filtering of short reads 2. Aligning the reads against a reference 3. Computationaly analysing of reads’ alignments 1. 2. 3. 4. compute the gene/transcript/exon expressions find new/known alternative splicing events find new/known fusion genes find new/known SNPs 4. Visualization www. fimm. fi 9
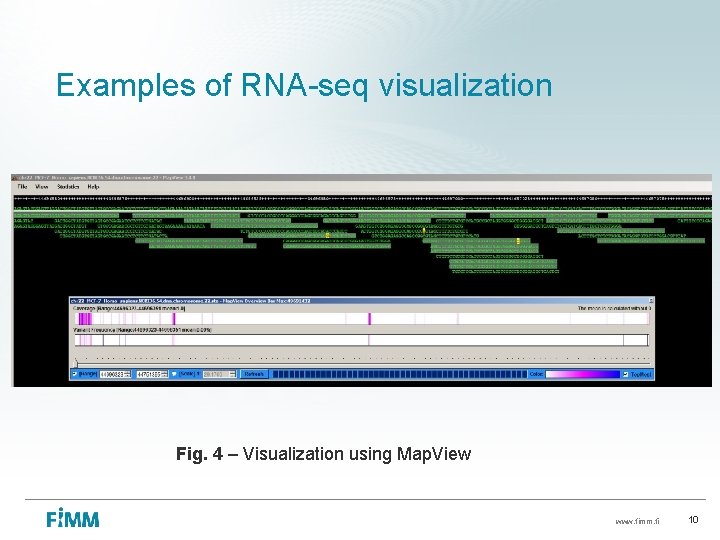
Examples of RNA-seq visualization Fig. 4 – Visualization using Map. View www. fimm. fi 10
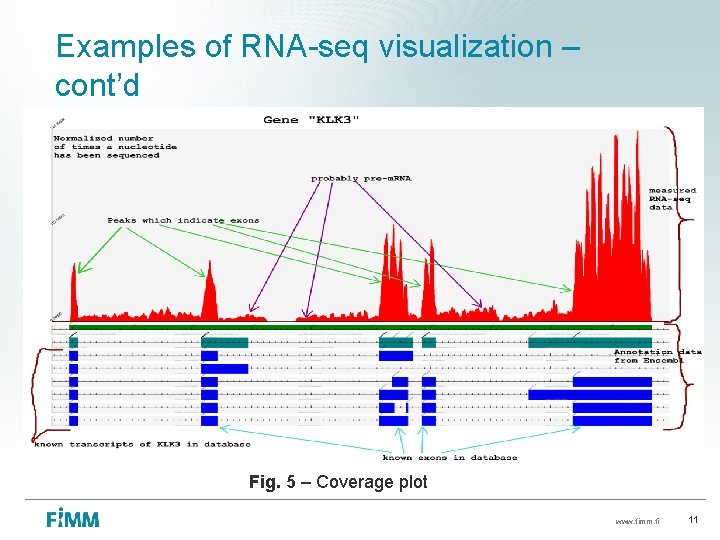
Examples of RNA-seq visualization – cont’d Fig. 5 – Coverage plot www. fimm. fi 11
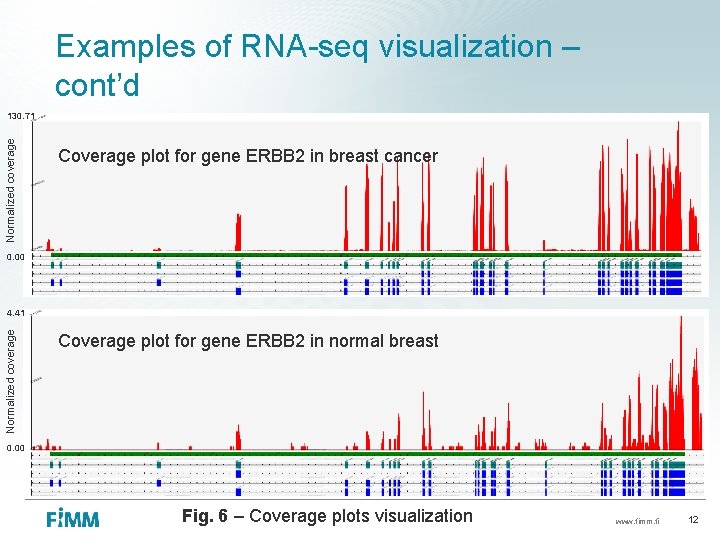
Examples of RNA-seq visualization – cont’d Normalized coverage 130. 71 Coverage plot for gene ERBB 2 in breast cancer 0. 00 Normalized coverage 4. 41 Coverage plot for gene ERBB 2 in normal breast 0. 00 Fig. 6 – Coverage plots visualization www. fimm. fi 12
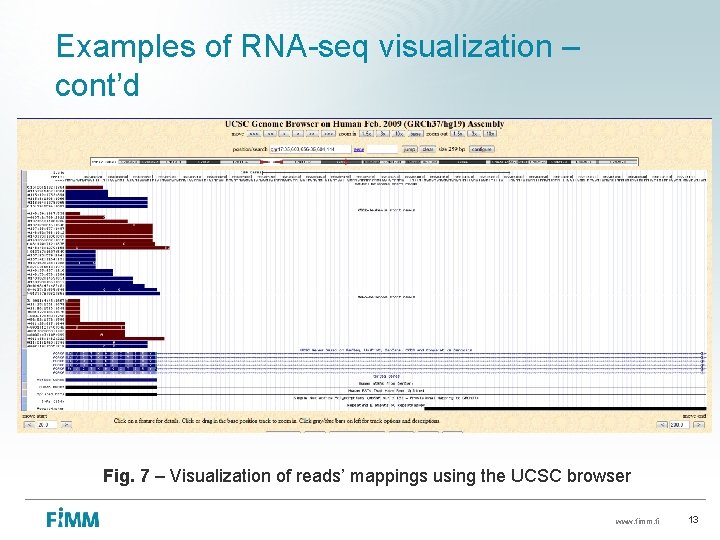
Examples of RNA-seq visualization – cont’d Fig. 7 – Visualization of reads’ mappings using the UCSC browser www. fimm. fi 13
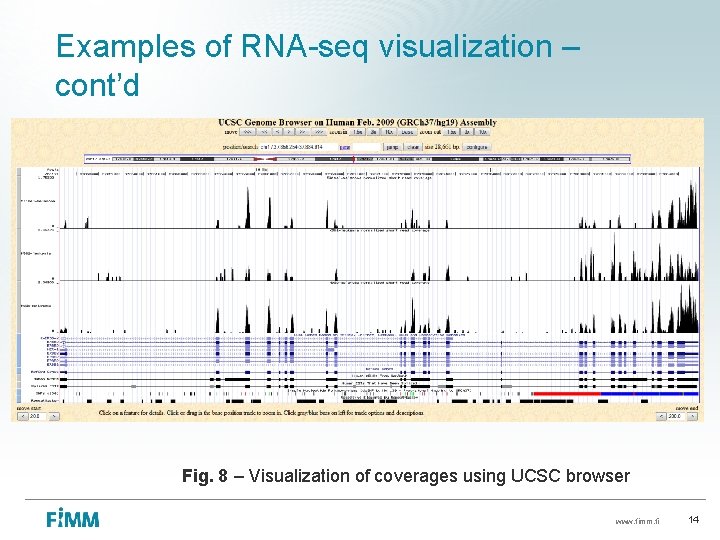
Examples of RNA-seq visualization – cont’d Fig. 8 – Visualization of coverages using UCSC browser www. fimm. fi 14
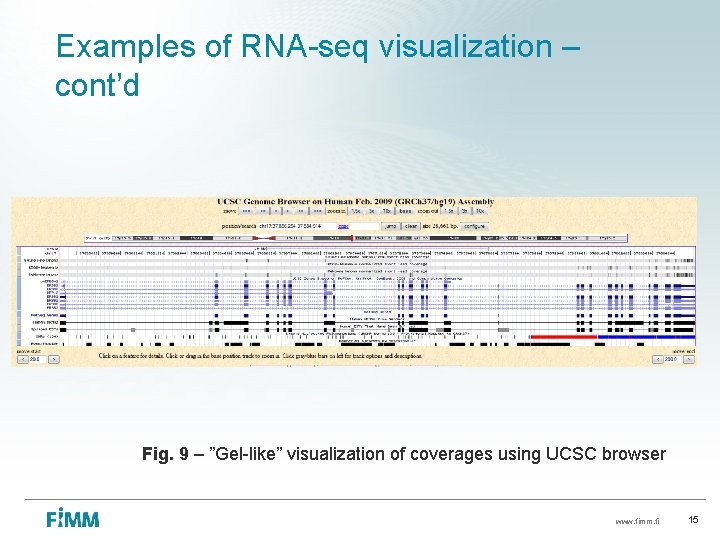
Examples of RNA-seq visualization – cont’d Fig. 9 – ”Gel-like” visualization of coverages using UCSC browser www. fimm. fi 15
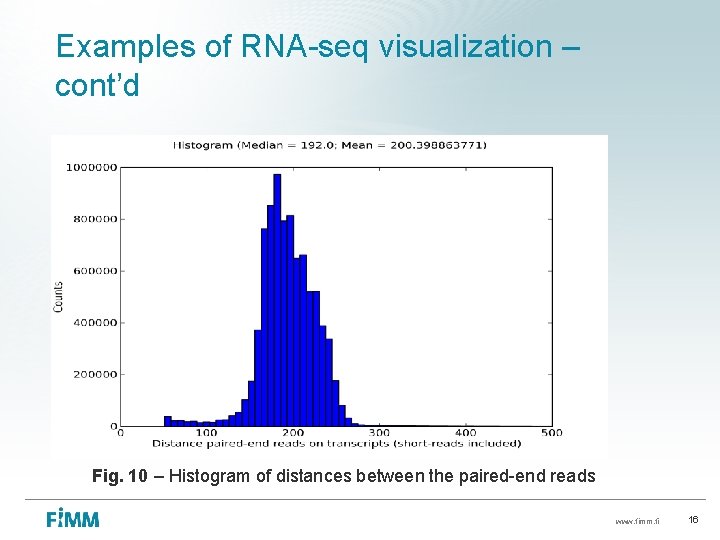
Examples of RNA-seq visualization – cont’d Fig. 10 – Histogram of distances between the paired-end reads www. fimm. fi 16
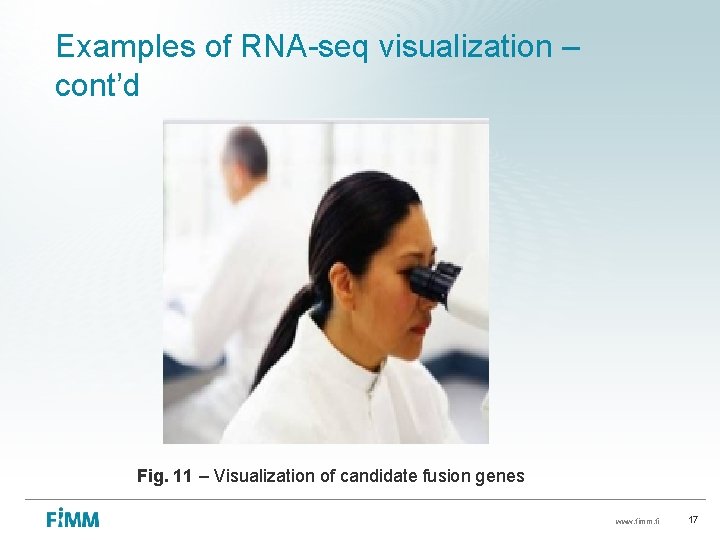
Examples of RNA-seq visualization – cont’d Fig. 11 – Visualization of candidate fusion genes www. fimm. fi 17
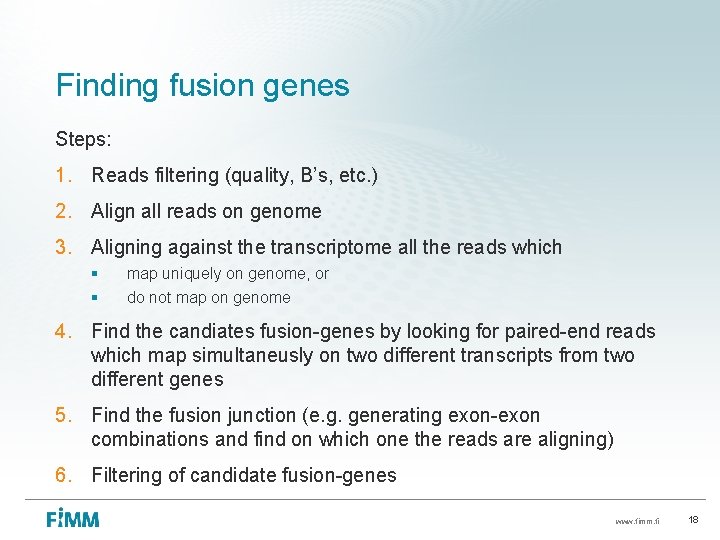
Finding fusion genes Steps: 1. Reads filtering (quality, B’s, etc. ) 2. Align all reads on genome 3. Aligning against the transcriptome all the reads which § § map uniquely on genome, or do not map on genome 4. Find the candiates fusion-genes by looking for paired-end reads which map simultaneusly on two different transcripts from two different genes 5. Find the fusion junction (e. g. generating exon-exon combinations and find on which one the reads are aligning) 6. Filtering of candidate fusion-genes www. fimm. fi 18
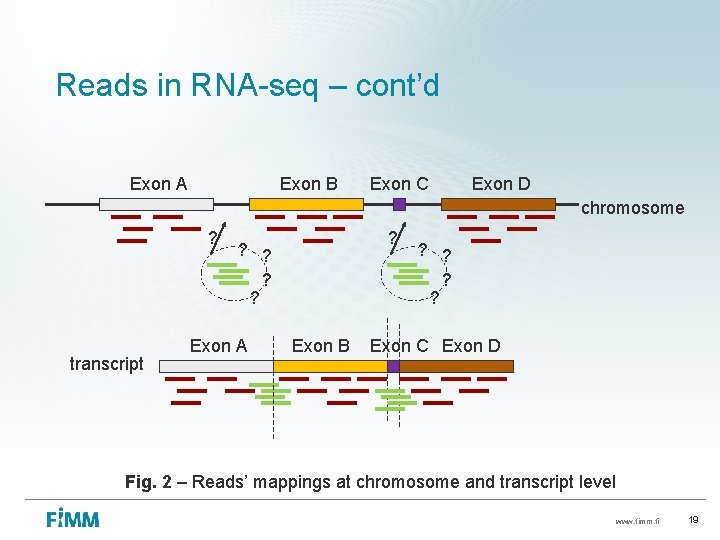
Reads in RNA-seq – cont’d Exon A Exon B Exon C Exon D chromosome ? transcript ? ? ? Exon A Exon B ? ? Exon C Exon D Fig. 2 – Reads’ mappings at chromosome and transcript level www. fimm. fi 19
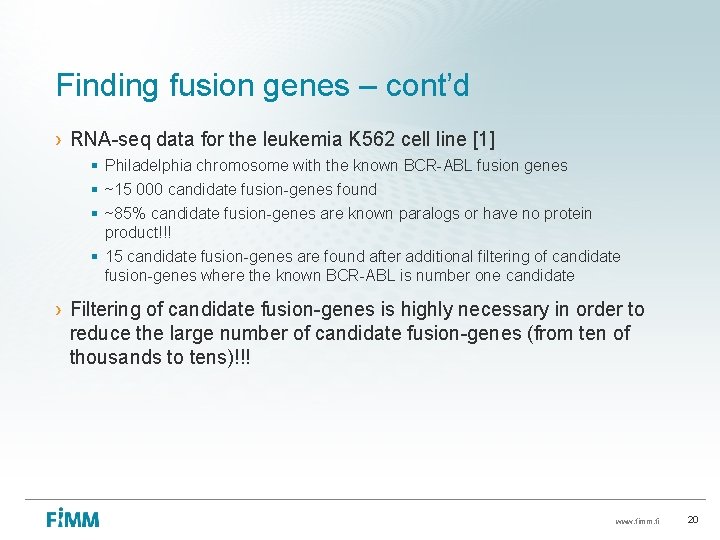
Finding fusion genes – cont’d › RNA-seq data for the leukemia K 562 cell line [1] § Philadelphia chromosome with the known BCR-ABL fusion genes § ~15 000 candidate fusion-genes found § ~85% candidate fusion-genes are known paralogs or have no protein product!!! § 15 candidate fusion-genes are found after additional filtering of candidate fusion-genes where the known BCR-ABL is number one candidate › Filtering of candidate fusion-genes is highly necessary in order to reduce the large number of candidate fusion-genes (from ten of thousands to tens)!!! www. fimm. fi 20
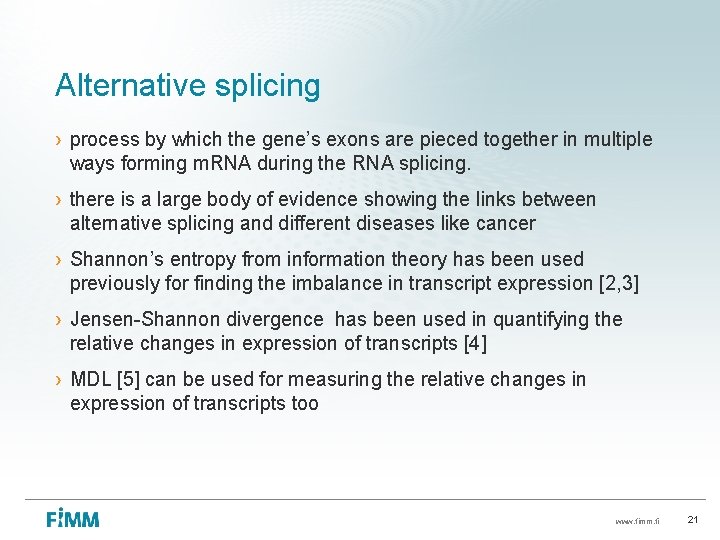
Alternative splicing › process by which the gene’s exons are pieced together in multiple ways forming m. RNA during the RNA splicing. › there is a large body of evidence showing the links between alternative splicing and different diseases like cancer › Shannon’s entropy from information theory has been used previously for finding the imbalance in transcript expression [2, 3] › Jensen-Shannon divergence has been used in quantifying the relative changes in expression of transcripts [4] › MDL [5] can be used for measuring the relative changes in expression of transcripts too www. fimm. fi 21
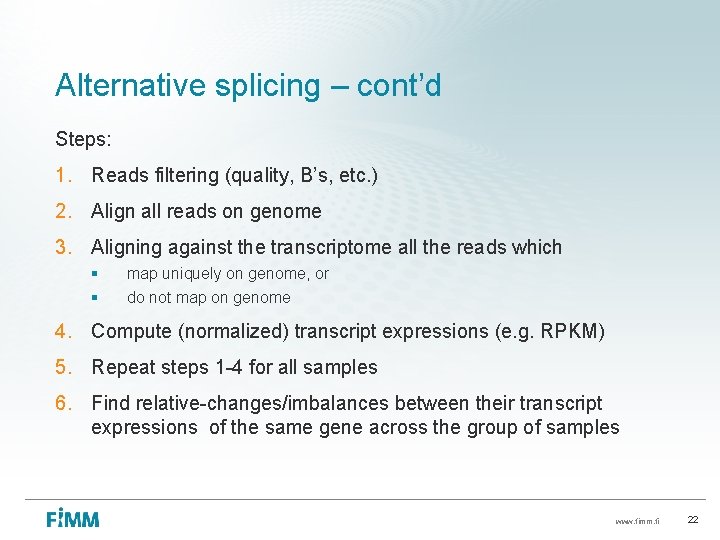
Alternative splicing – cont’d Steps: 1. Reads filtering (quality, B’s, etc. ) 2. Align all reads on genome 3. Aligning against the transcriptome all the reads which § § map uniquely on genome, or do not map on genome 4. Compute (normalized) transcript expressions (e. g. RPKM) 5. Repeat steps 1 -4 for all samples 6. Find relative-changes/imbalances between their transcript expressions of the same gene across the group of samples www. fimm. fi 22
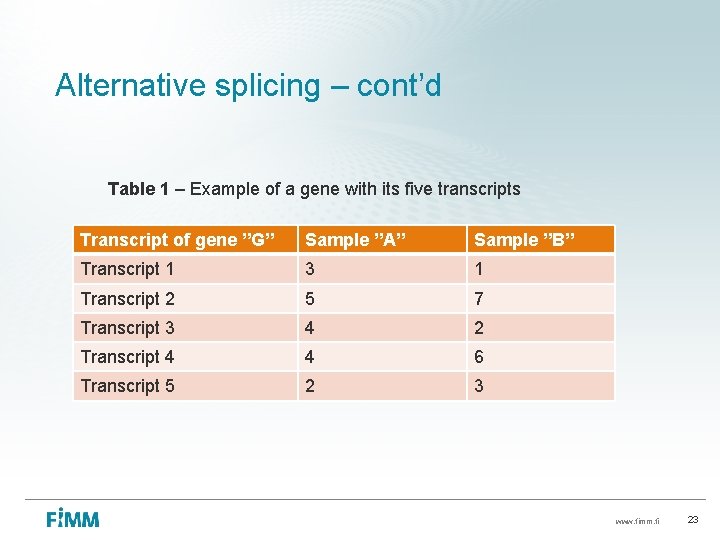
Alternative splicing – cont’d Table 1 – Example of a gene with its five transcripts Transcript of gene ”G” Sample ”A” Sample ”B” Transcript 1 3 1 Transcript 2 5 7 Transcript 3 4 2 Transcript 4 4 6 Transcript 5 2 3 www. fimm. fi 23
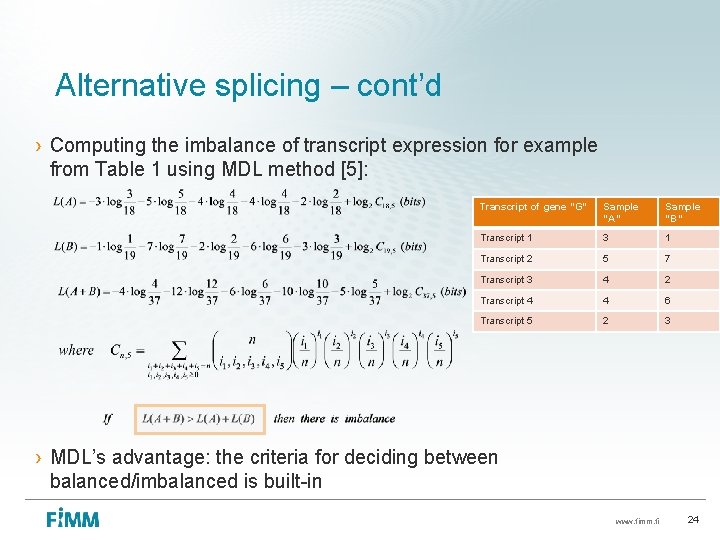
Alternative splicing – cont’d › Computing the imbalance of transcript expression for example from Table 1 using MDL method [5]: Transcript of gene ”G” Sample ”A” Sample ”B” Transcript 1 3 1 Transcript 2 5 7 Transcript 3 4 2 Transcript 4 4 6 Transcript 5 2 3 › MDL’s advantage: the criteria for deciding between balanced/imbalanced is built-in www. fimm. fi 24
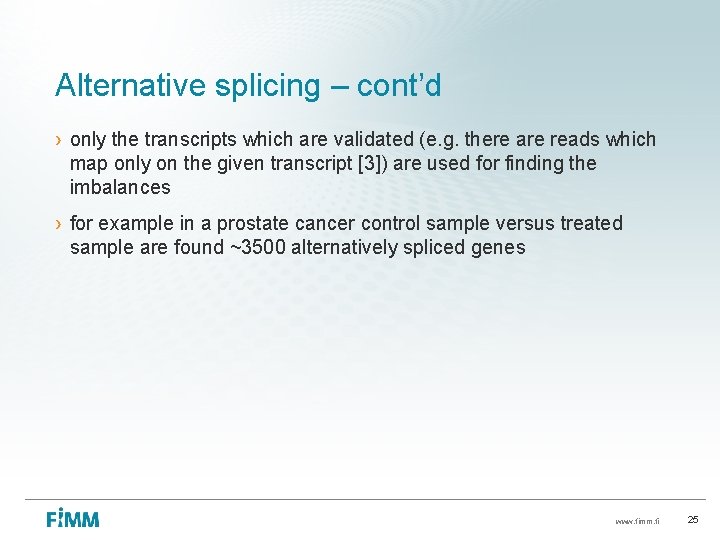
Alternative splicing – cont’d › only the transcripts which are validated (e. g. there are reads which map only on the given transcript [3]) are used for finding the imbalances › for example in a prostate cancer control sample versus treated sample are found ~3500 alternatively spliced genes www. fimm. fi 25
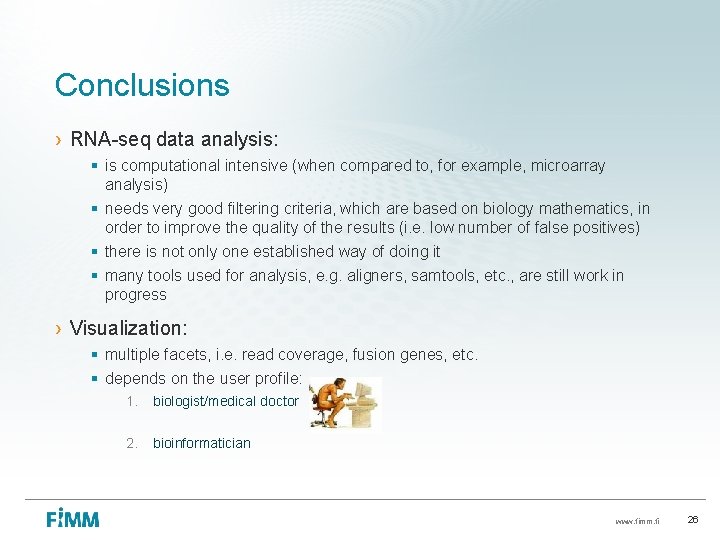
Conclusions › RNA-seq data analysis: § is computational intensive (when compared to, for example, microarray analysis) § needs very good filtering criteria, which are based on biology mathematics, in order to improve the quality of the results (i. e. low number of false positives) § there is not only one established way of doing it § many tools used for analysis, e. g. aligners, samtools, etc. , are still work in progress › Visualization: § multiple facets, i. e. read coverage, fusion genes, etc. § depends on the user profile: 1. biologist/medical doctor 2. bioinformatician www. fimm. fi 26
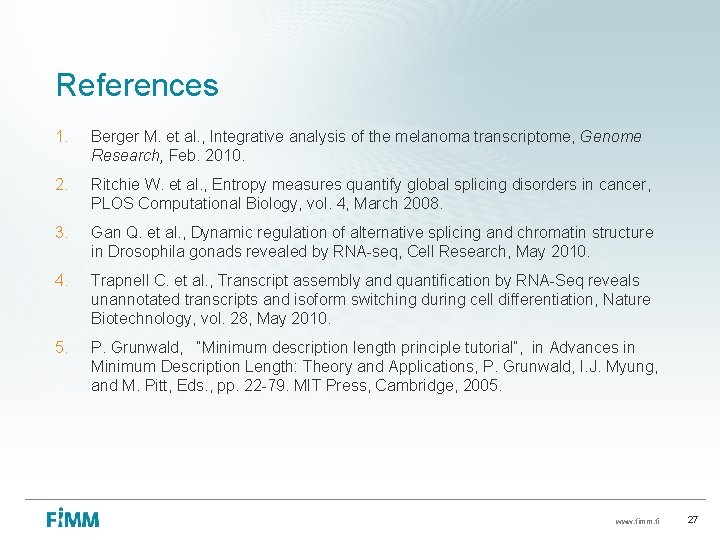
References 1. Berger M. et al. , Integrative analysis of the melanoma transcriptome, Genome Research, Feb. 2010. 2. Ritchie W. et al. , Entropy measures quantify global splicing disorders in cancer, PLOS Computational Biology, vol. 4, March 2008. 3. Gan Q. et al. , Dynamic regulation of alternative splicing and chromatin structure in Drosophila gonads revealed by RNA-seq, Cell Research, May 2010. 4. Trapnell C. et al. , Transcript assembly and quantification by RNA-Seq reveals unannotated transcripts and isoform switching during cell differentiation, Nature Biotechnology, vol. 28, May 2010. 5. P. Grunwald, “Minimum description length principle tutorial”, in Advances in Minimum Description Length: Theory and Applications, P. Grunwald, I. J. Myung, and M. Pitt, Eds. , pp. 22 -79. MIT Press, Cambridge, 2005. www. fimm. fi 27
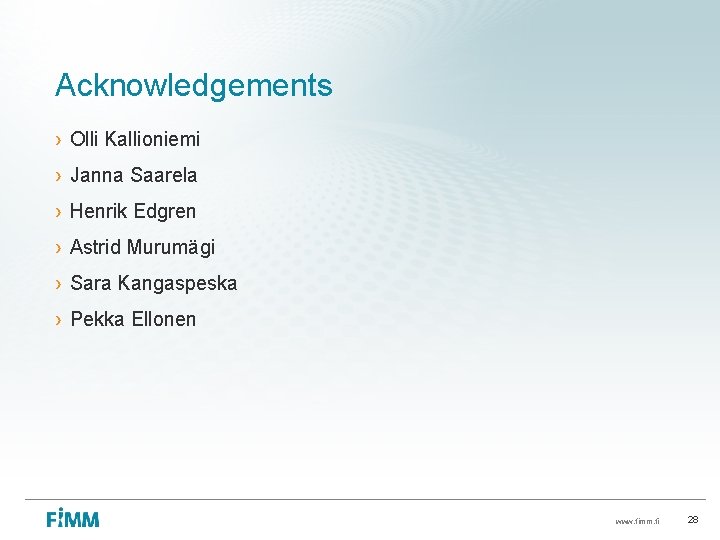
Acknowledgements › Olli Kallioniemi › Janna Saarela › Henrik Edgren › Astrid Murumägi › Sara Kangaspeska › Pekka Ellonen www. fimm. fi 28
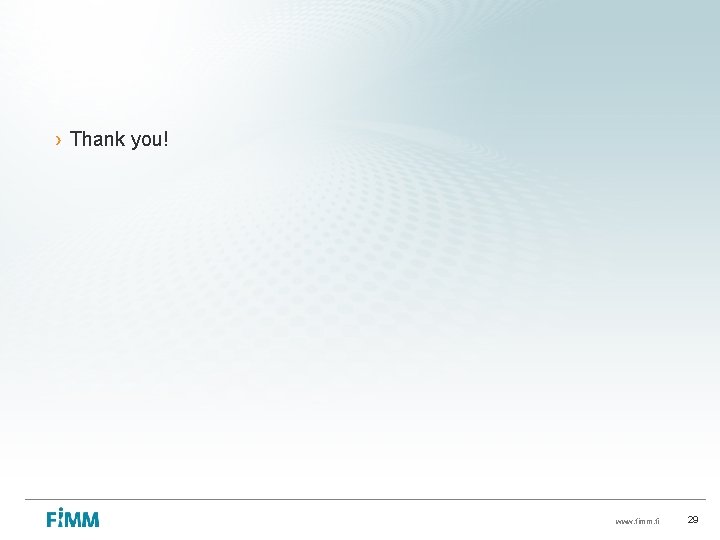
› Thank you! www. fimm. fi 29
Pfarrer fimm exerzitien 2021
Physical state of covalent compounds
Giant molecular structure vs simple molecular structure
Giant molecular structure vs simple molecular structure
Trends in molecular medicine
Y m c a
Technology industries of finland
Finland homelessness solution
Happy godmothers day gif
Technical
Jukka pietarinen
Ytv2012
Pisa
Aqua action
Ytk täysjäsen
Easter origin
Inner wheel finland
Adoption in finland
Sfs finland
Work life balance finland
Agria finland
Regional state administrative agency for southern finland
Silikoninenätyynyt
Finnish oats
Finland facts and information
Recycled material socks
Elematic finland
Dhl joensuu
Finland goose
Facts about finland