Faithfulness of Electronic Healthcare Record Data a Shared
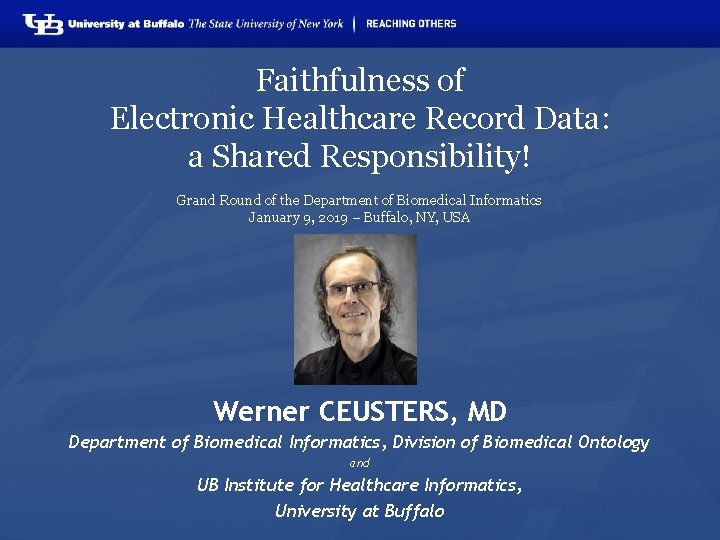
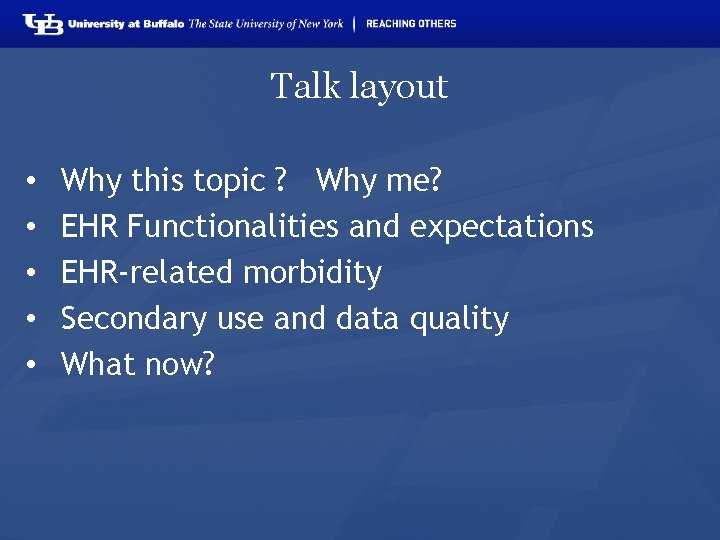
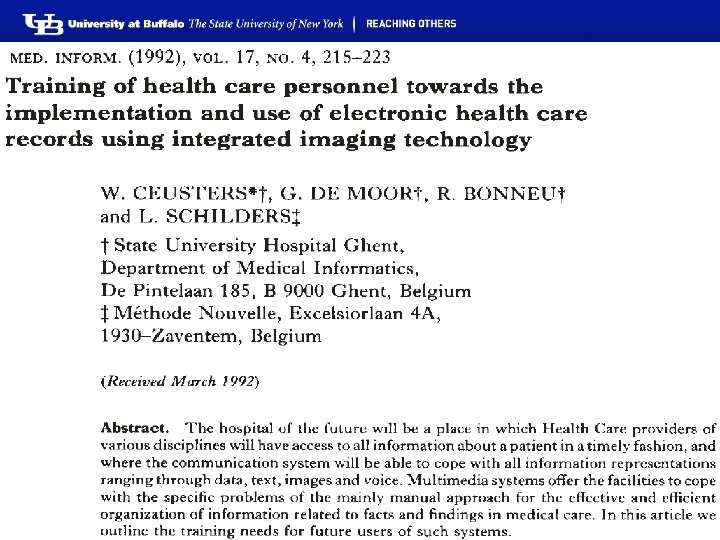
![Pubmed – [EHR] OR [EMR] count (January 3, 2019) 80 70 60 50 40 Pubmed – [EHR] OR [EMR] count (January 3, 2019) 80 70 60 50 40](https://slidetodoc.com/presentation_image_h2/9b2cf8fbee77a52cd672e0a1d00cf216/image-4.jpg)
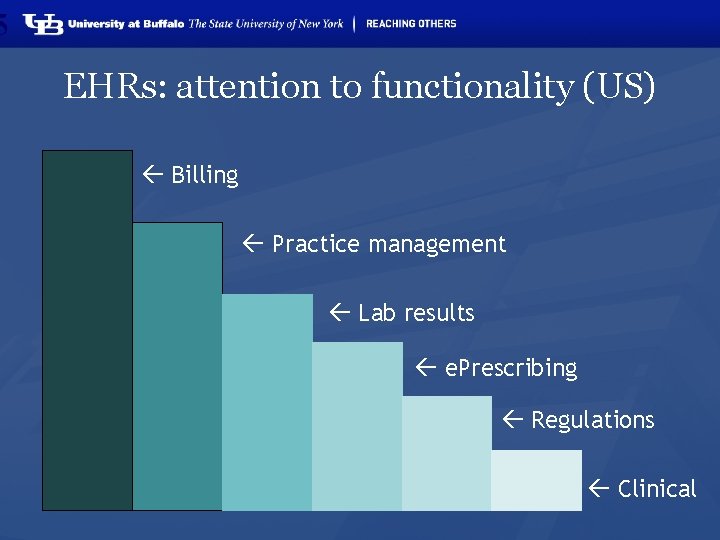
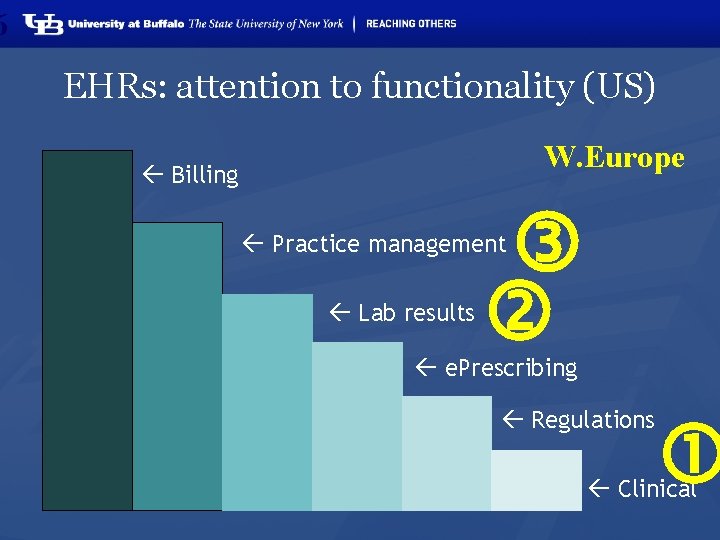
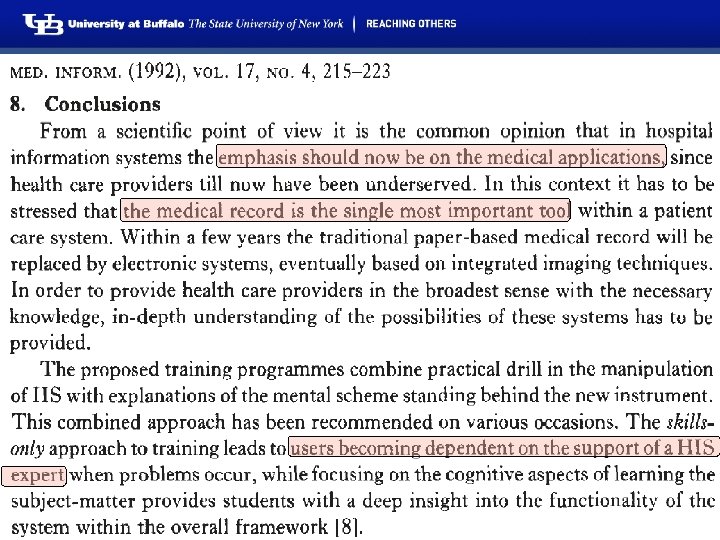
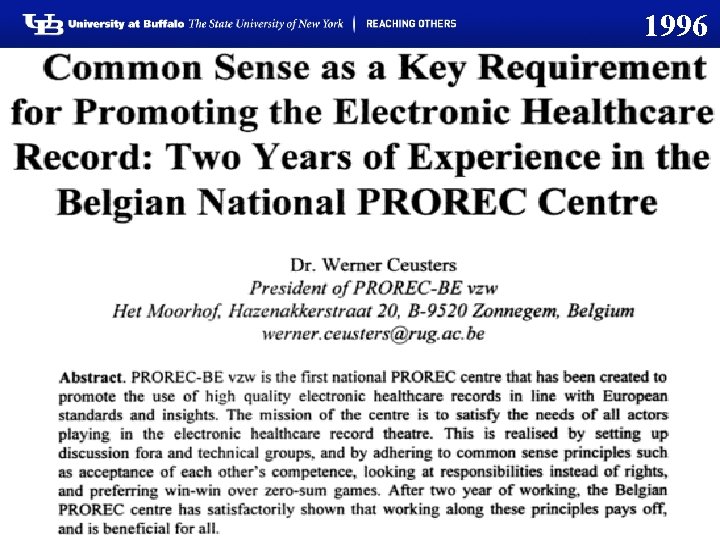
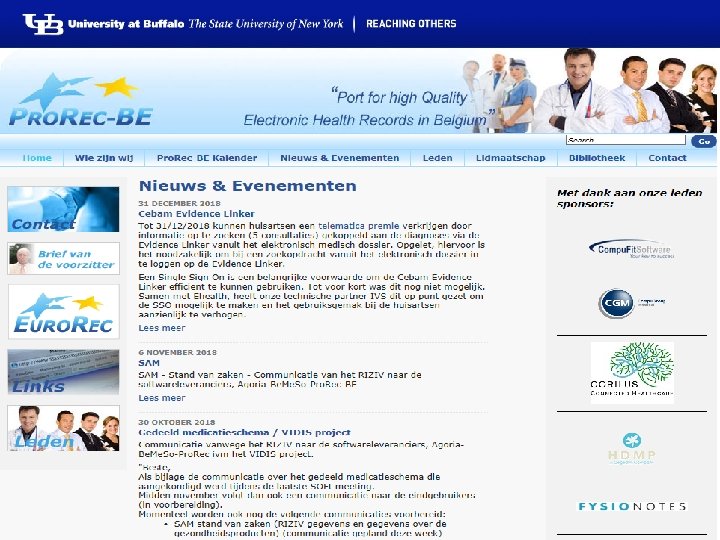
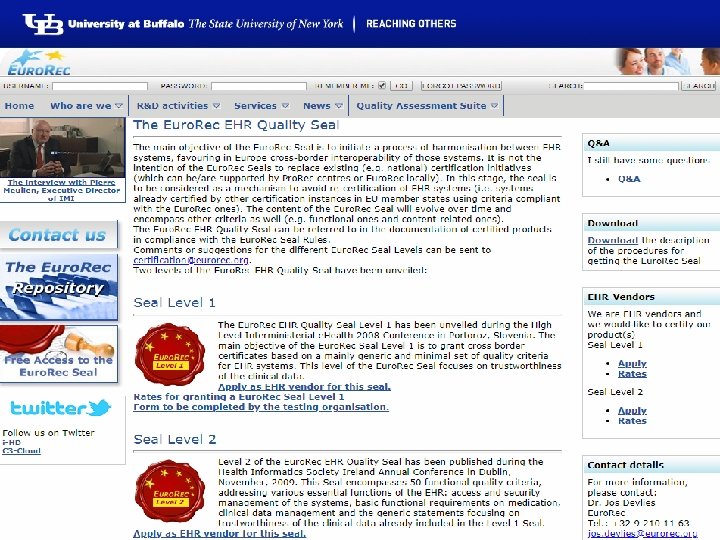
![Pubmed – [EHR] OR [EMR] count (January 3, 2019) 80 70 60 50 40 Pubmed – [EHR] OR [EMR] count (January 3, 2019) 80 70 60 50 40](https://slidetodoc.com/presentation_image_h2/9b2cf8fbee77a52cd672e0a1d00cf216/image-11.jpg)
![Pubmed – [EHR] OR [EMR] count (January 3, 2019) 1800 1600 1400 1200 1000 Pubmed – [EHR] OR [EMR] count (January 3, 2019) 1800 1600 1400 1200 1000](https://slidetodoc.com/presentation_image_h2/9b2cf8fbee77a52cd672e0a1d00cf216/image-12.jpg)
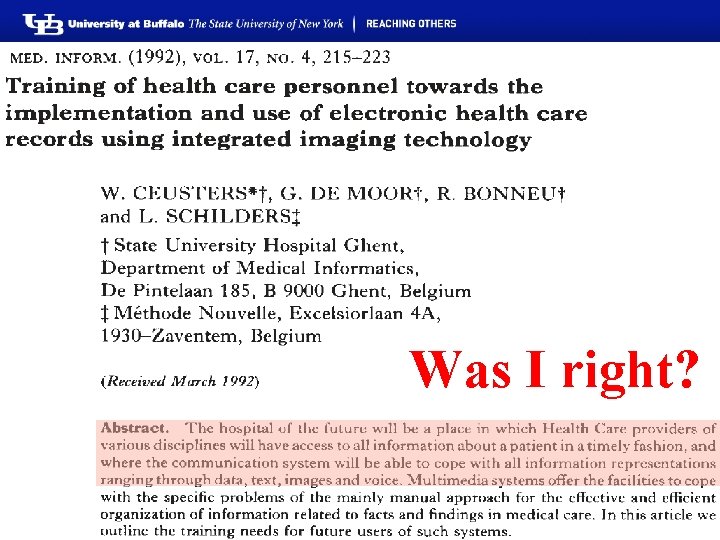
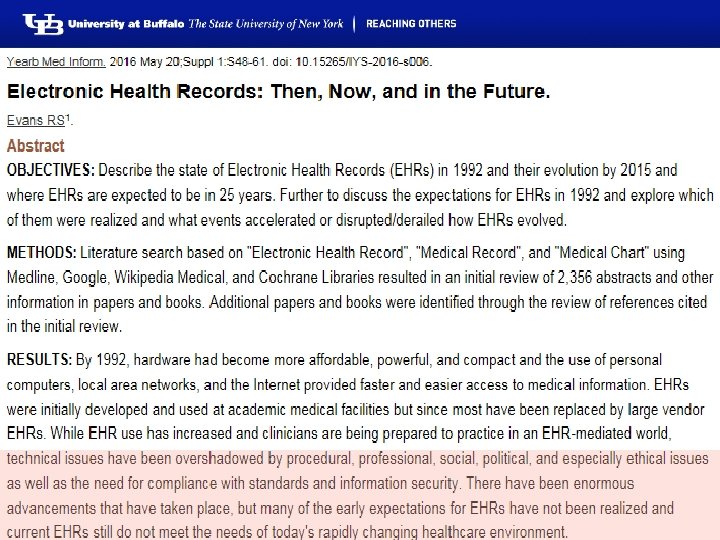
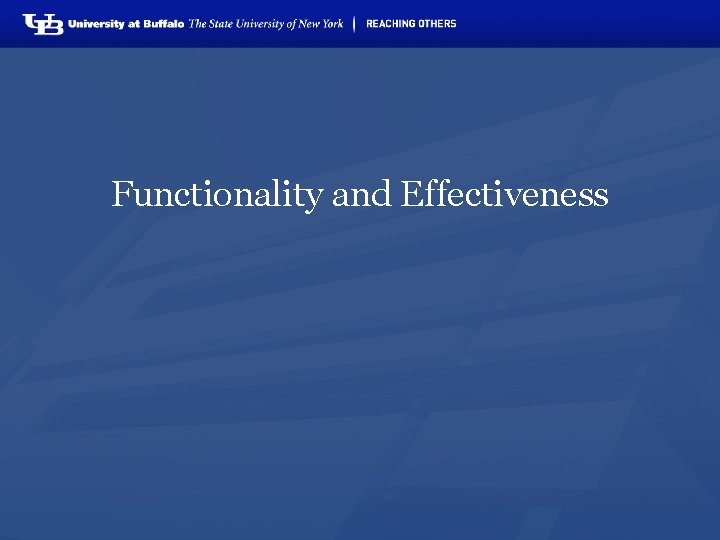
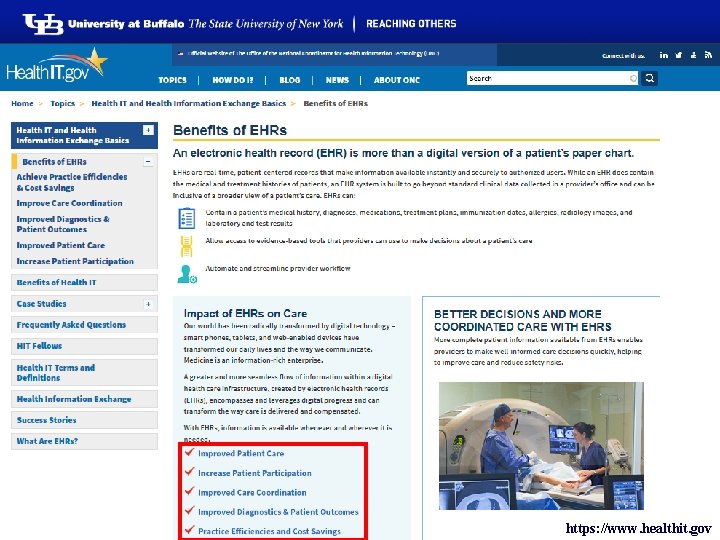
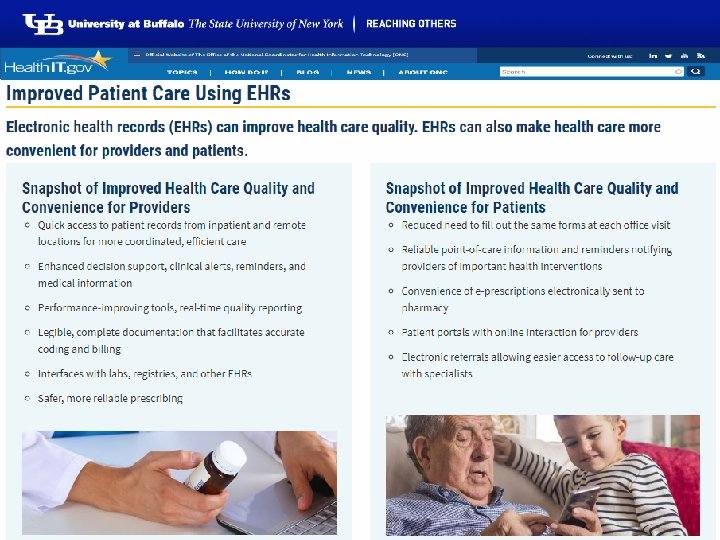
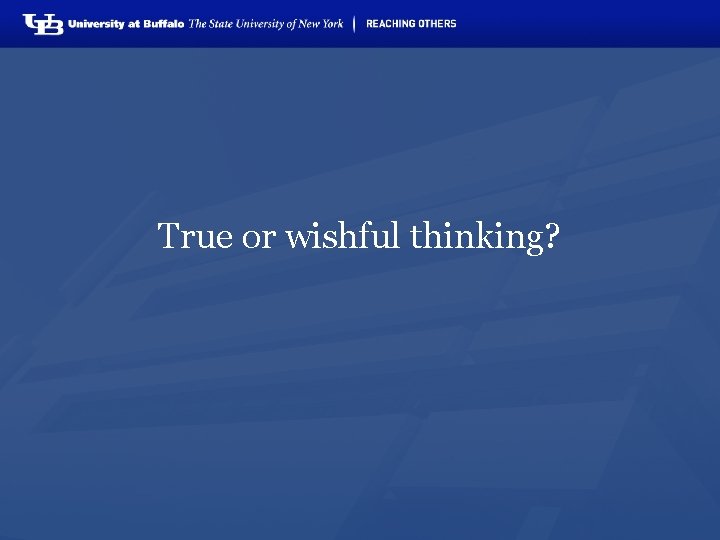
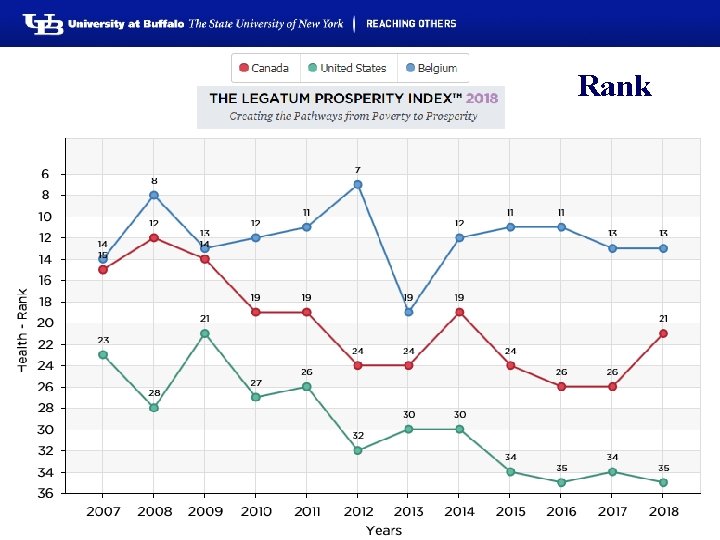
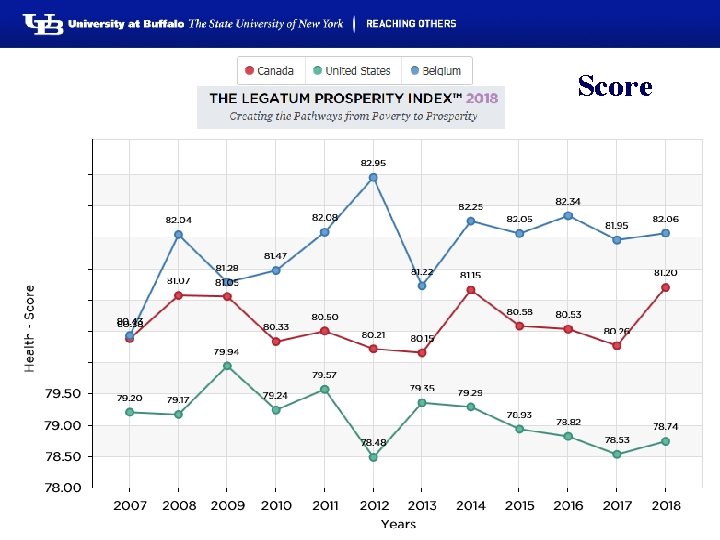
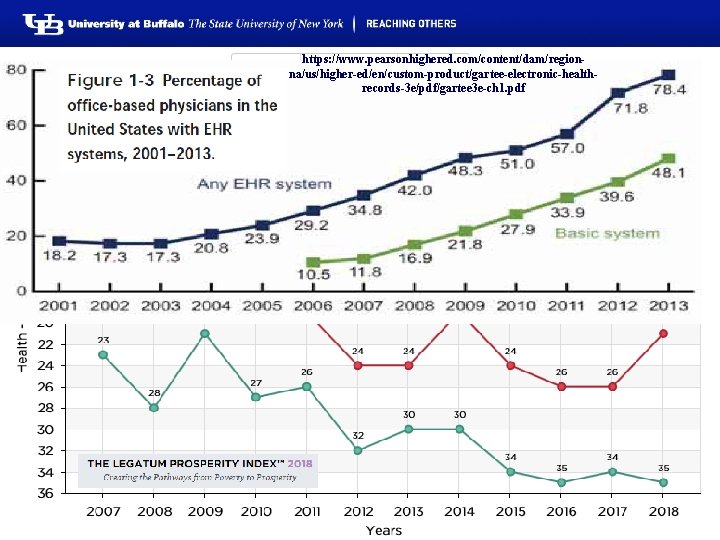
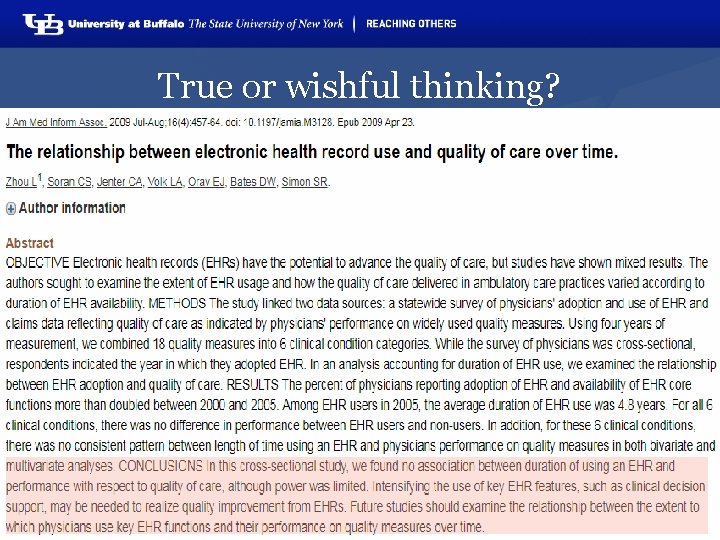
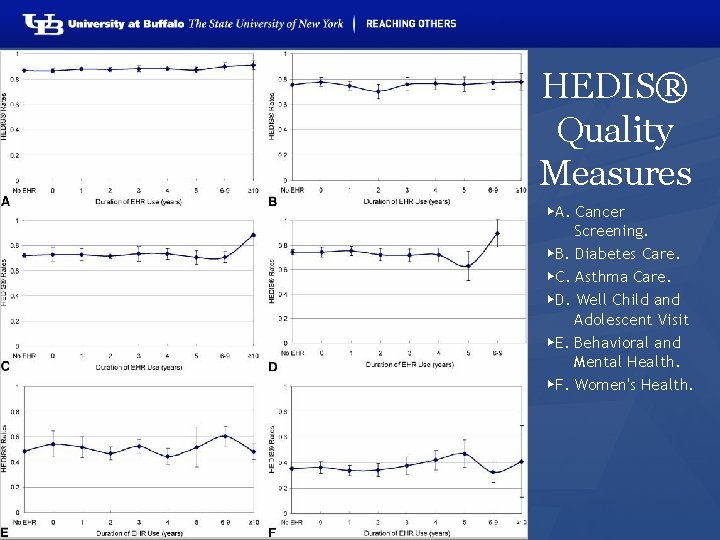
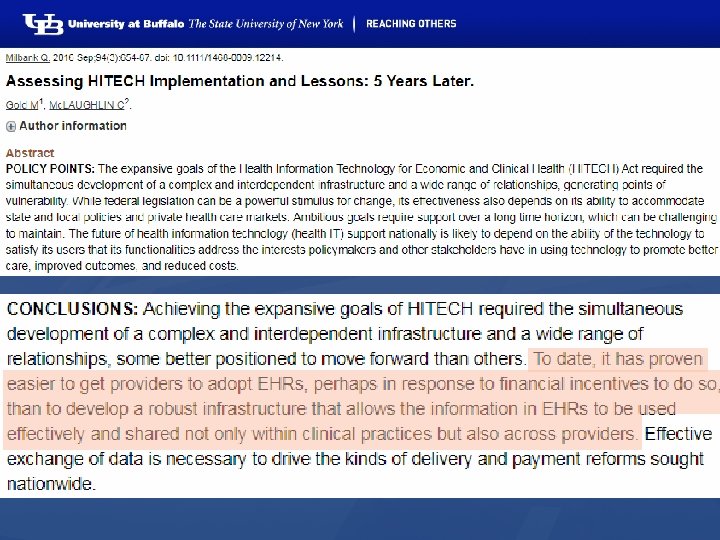
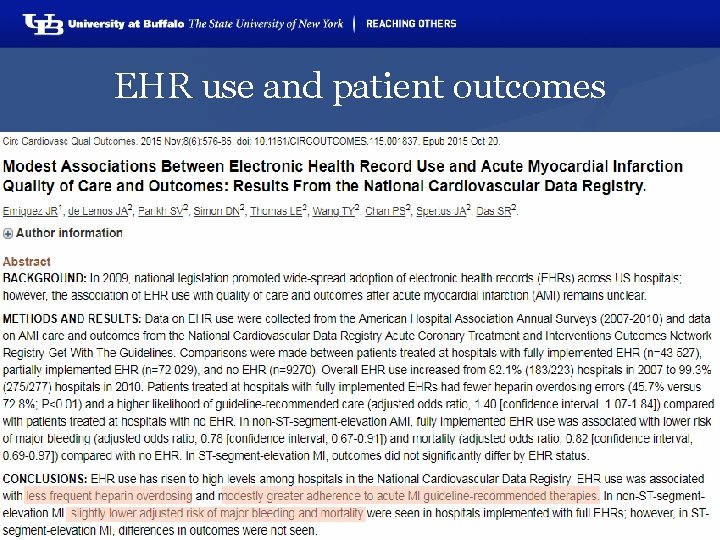
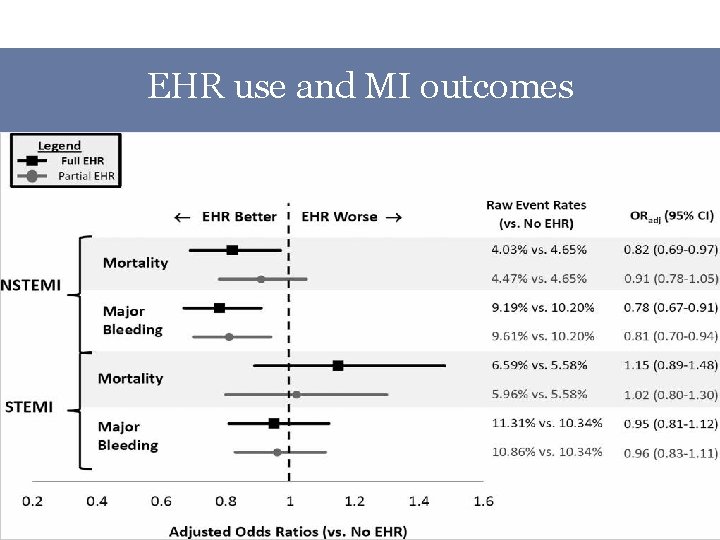
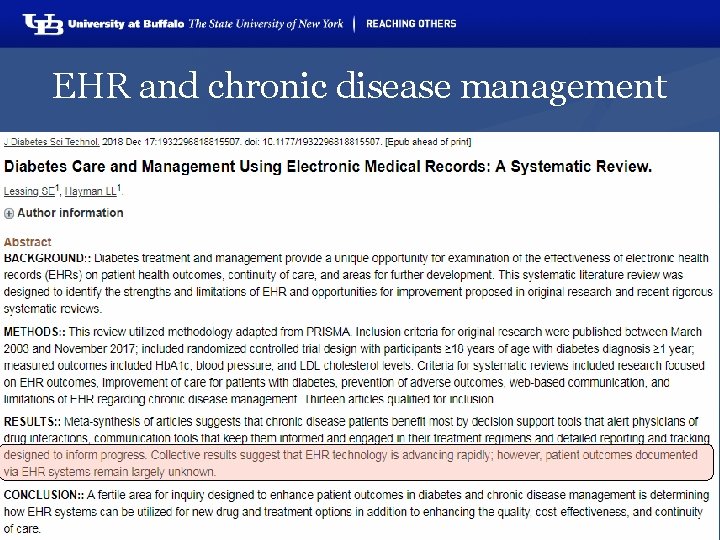
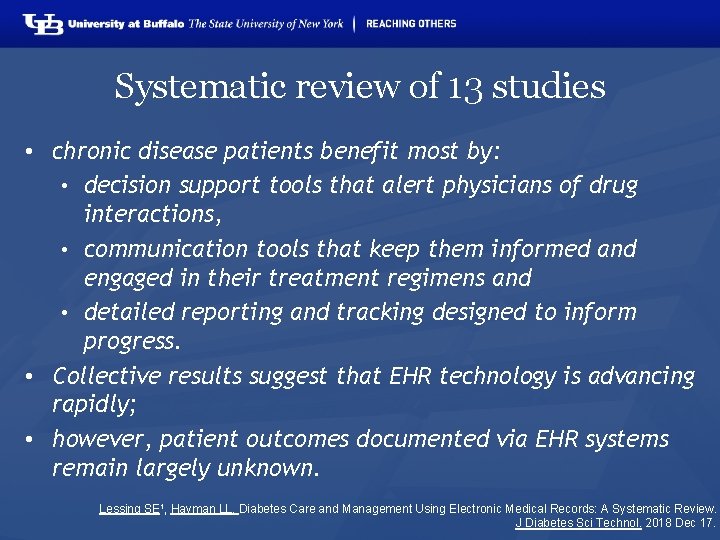
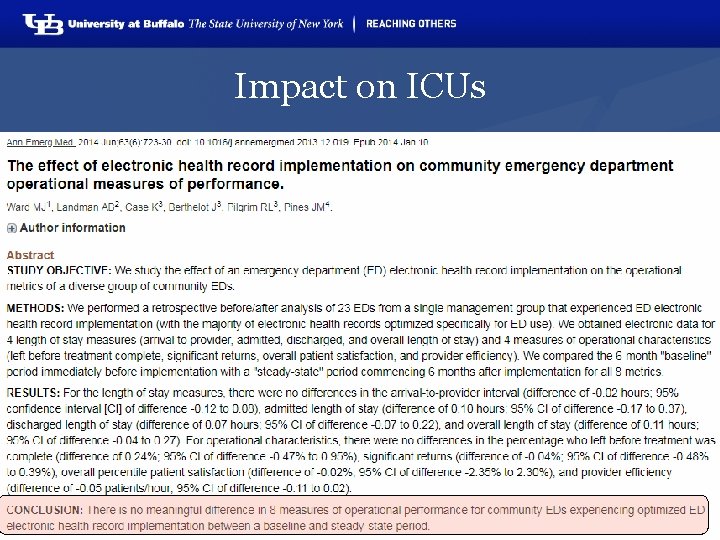
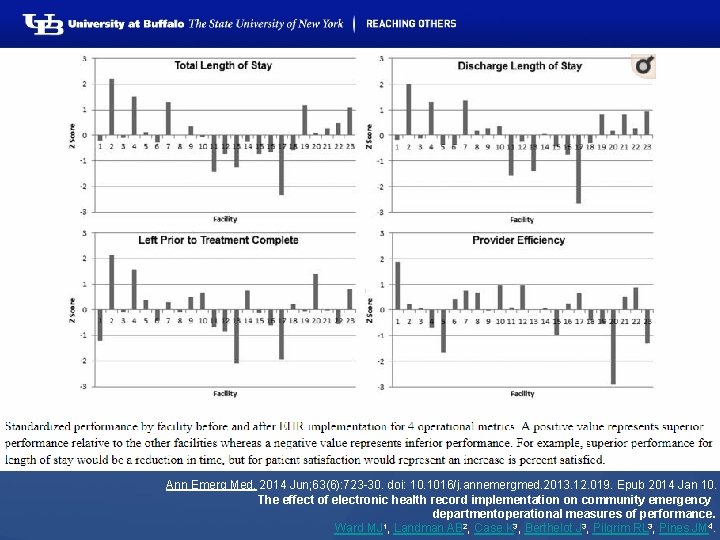
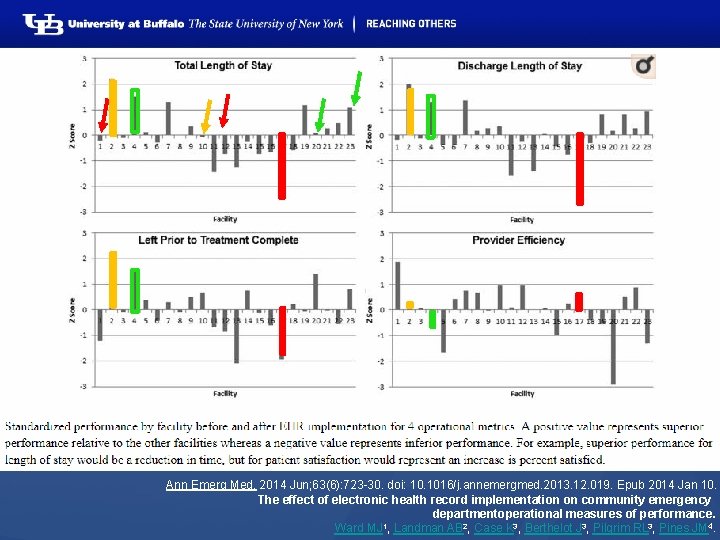
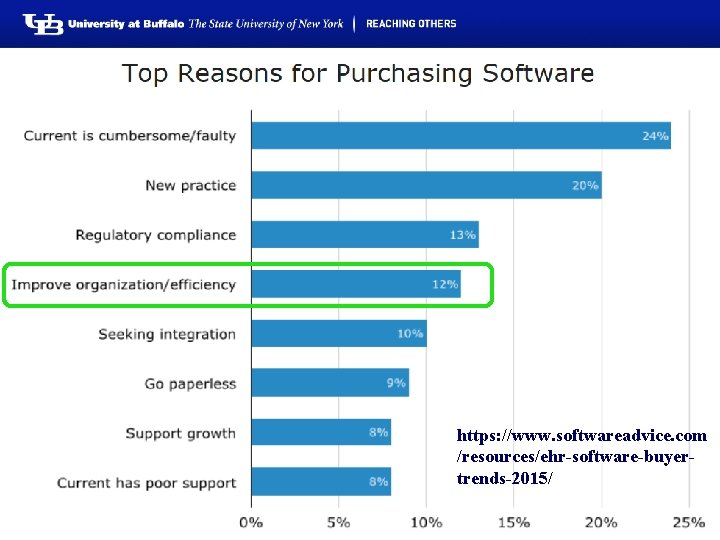
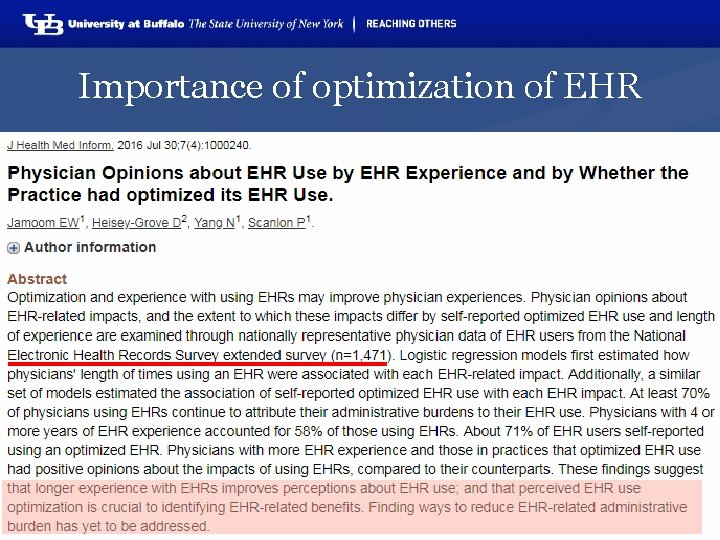
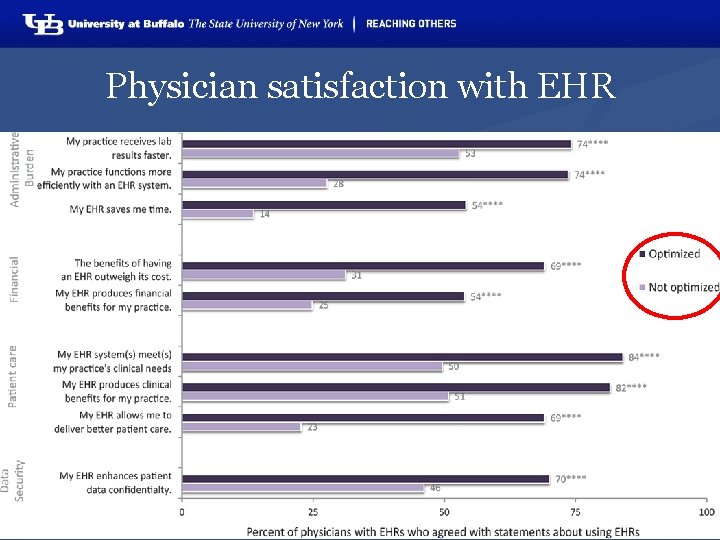
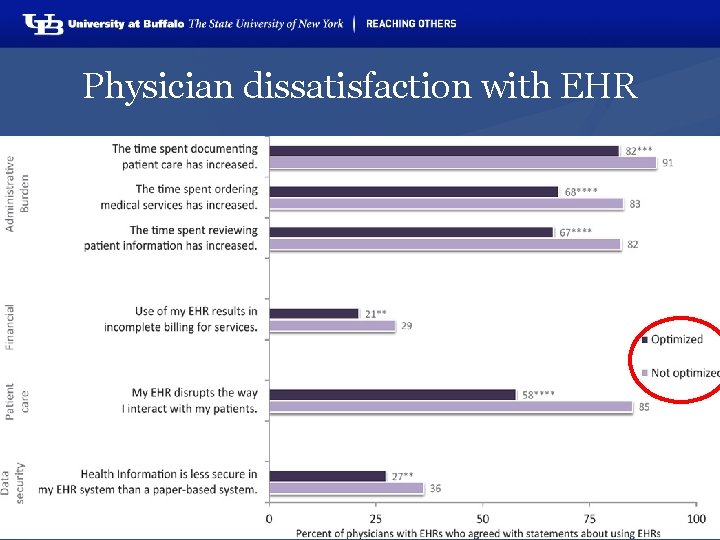
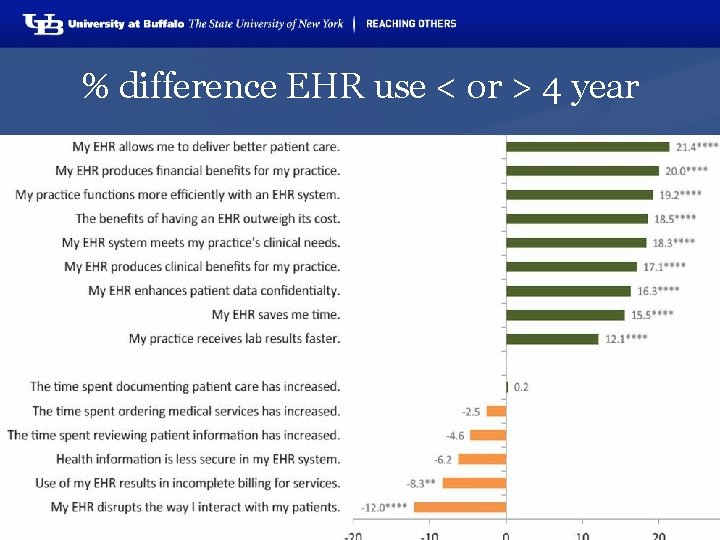
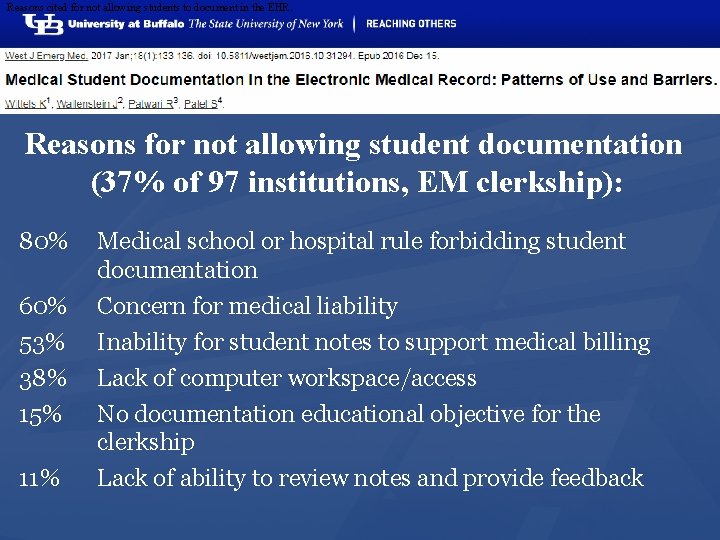
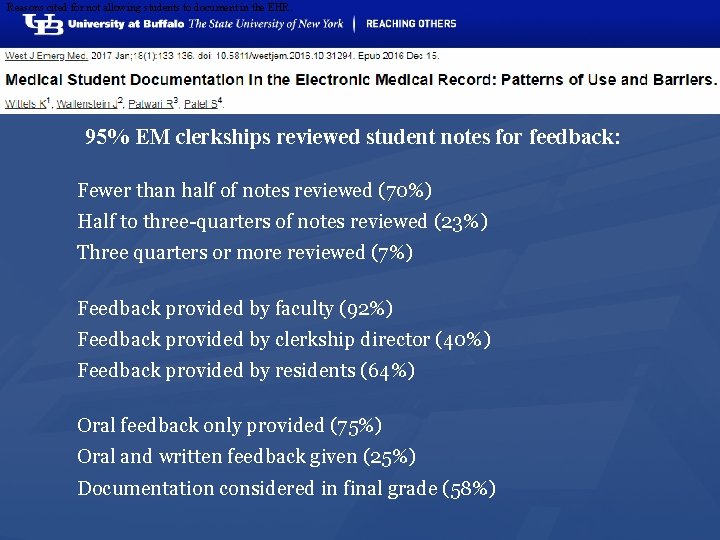
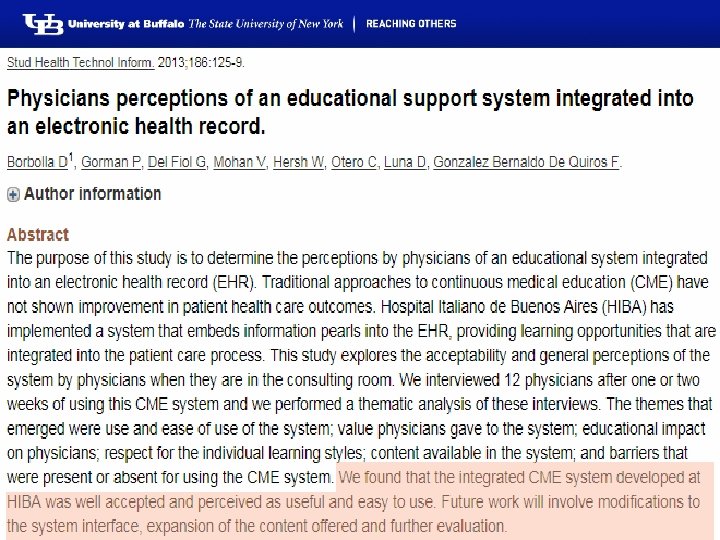
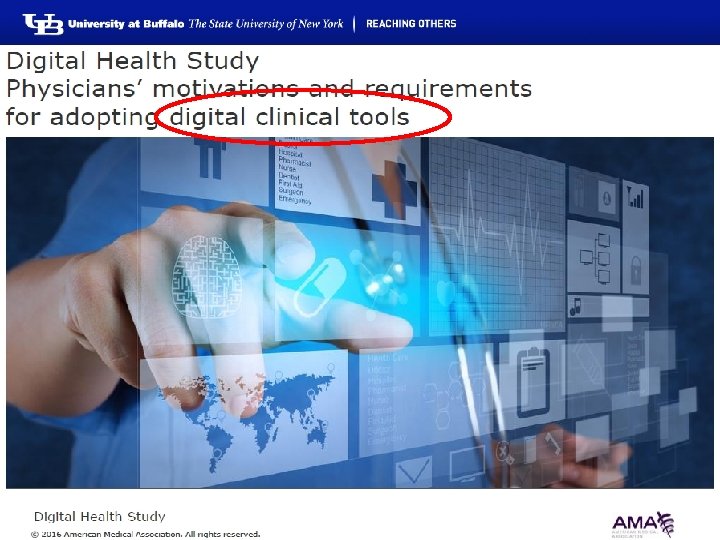
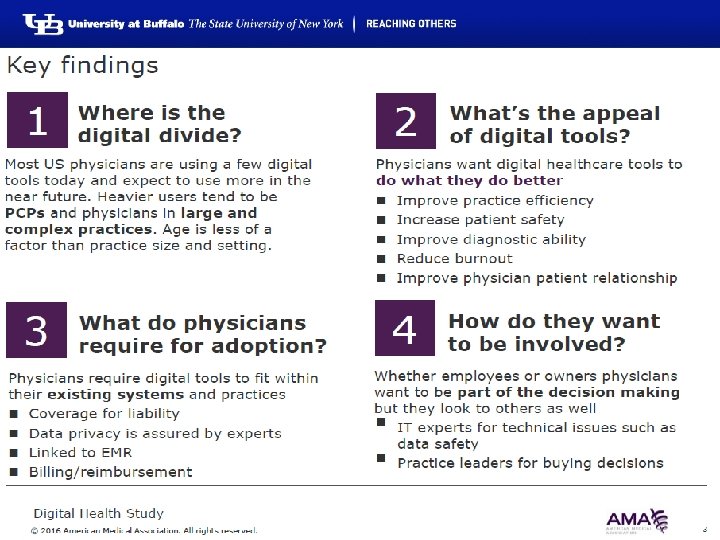
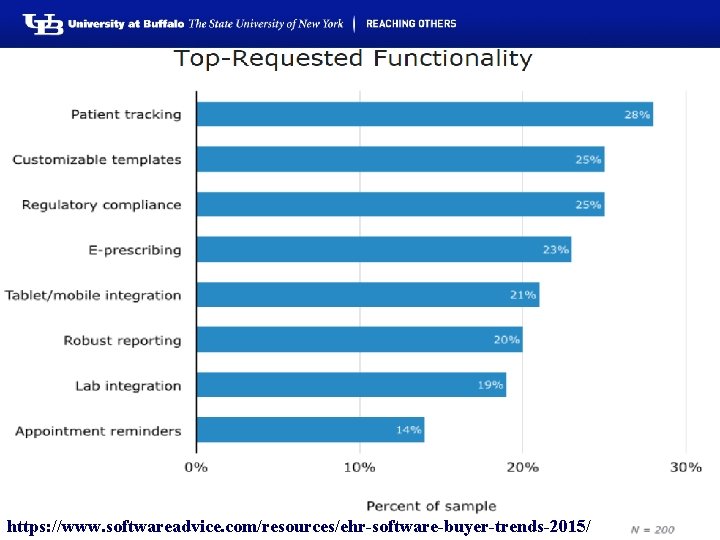
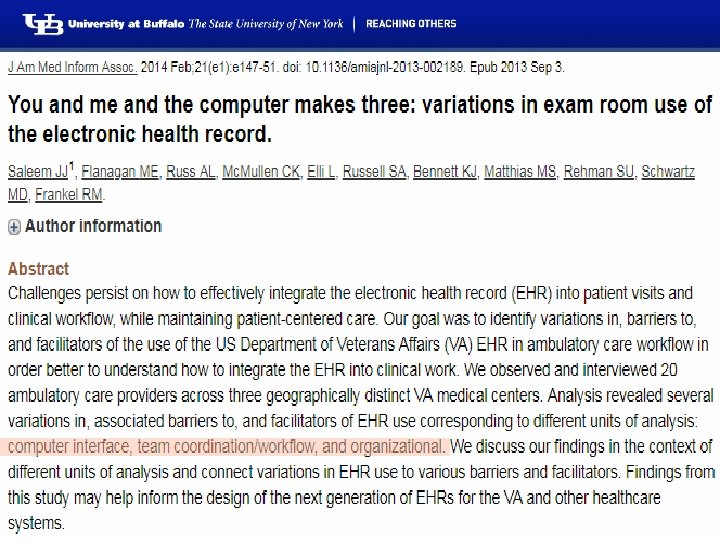
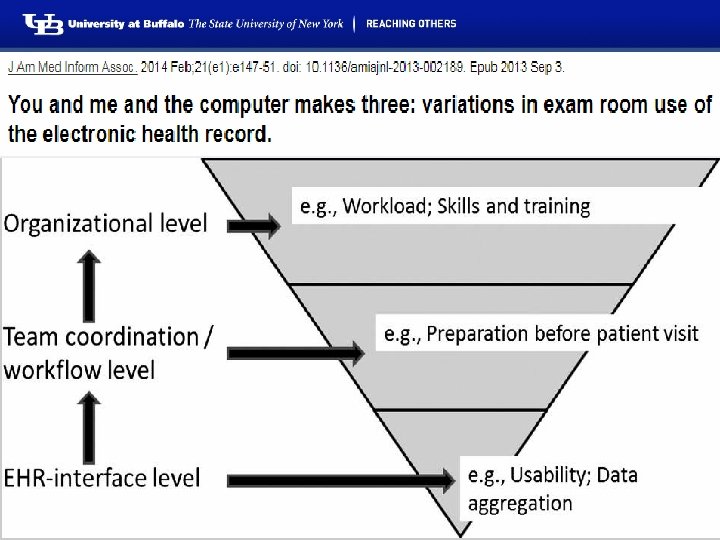
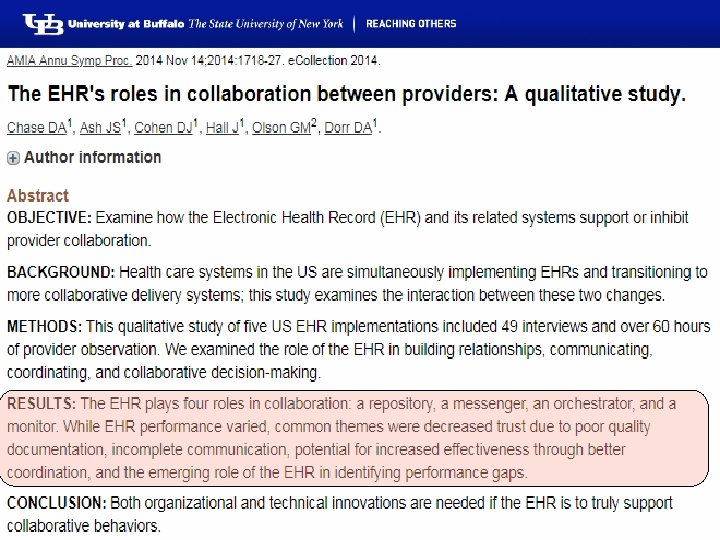
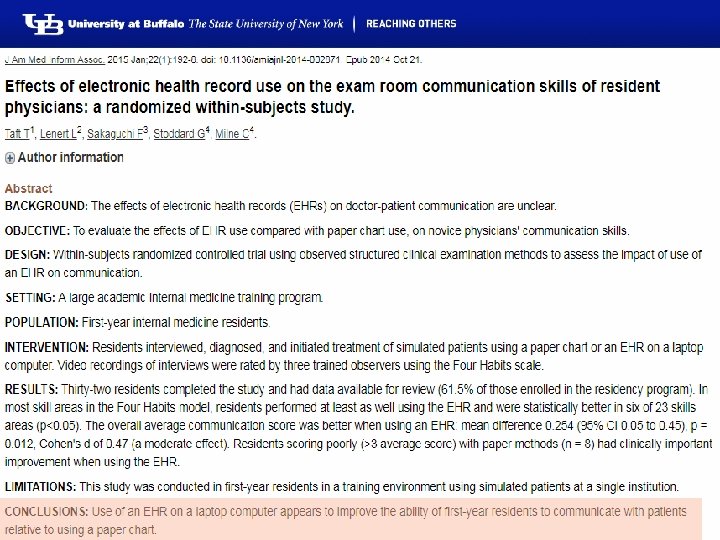
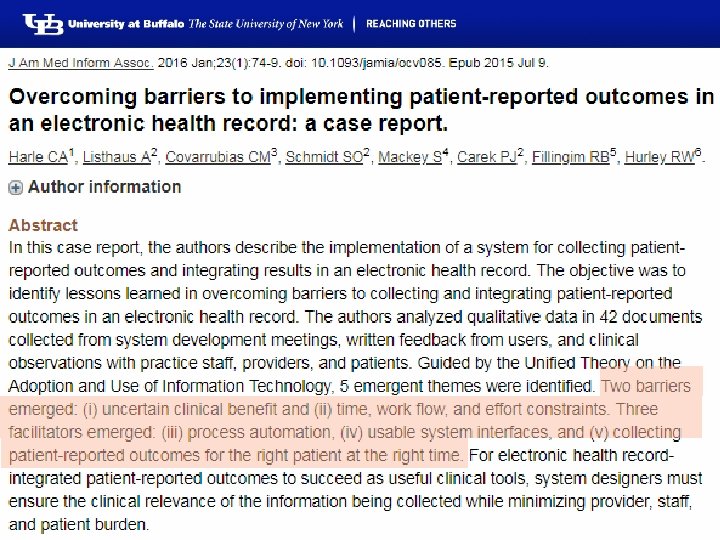
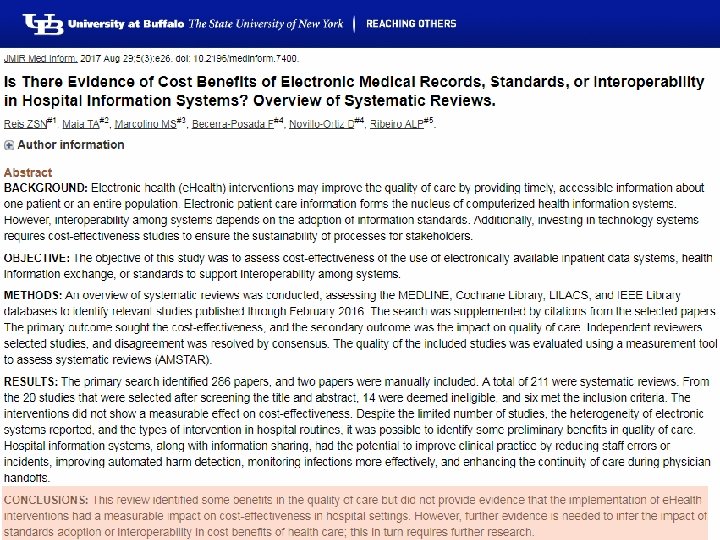
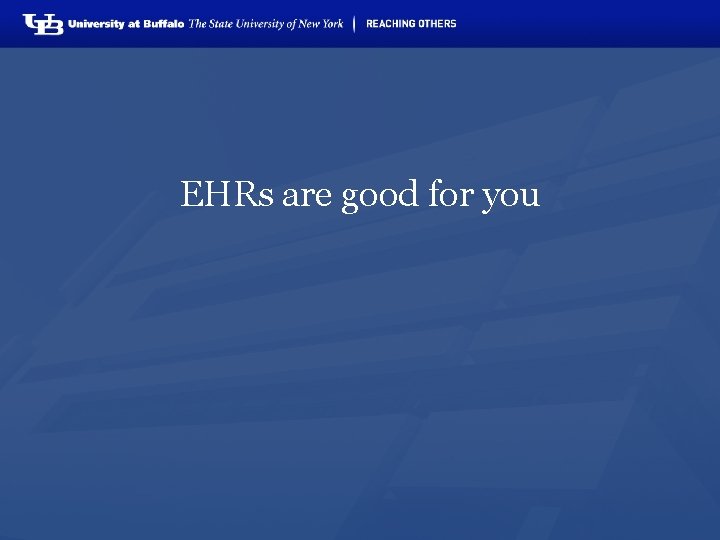
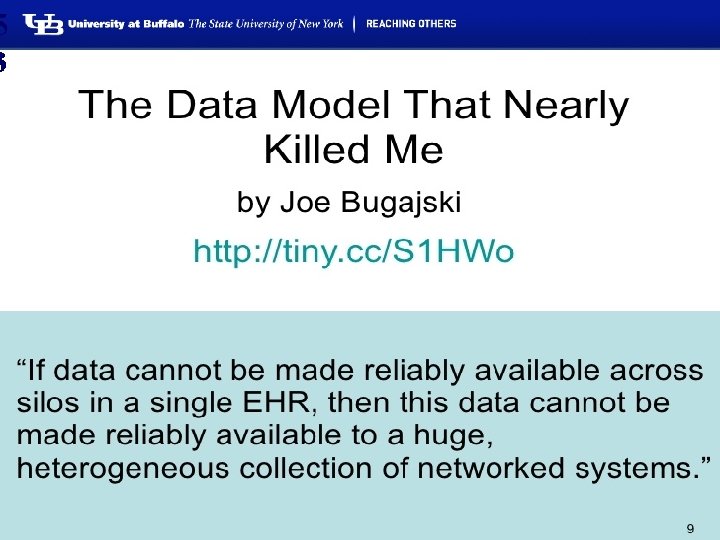
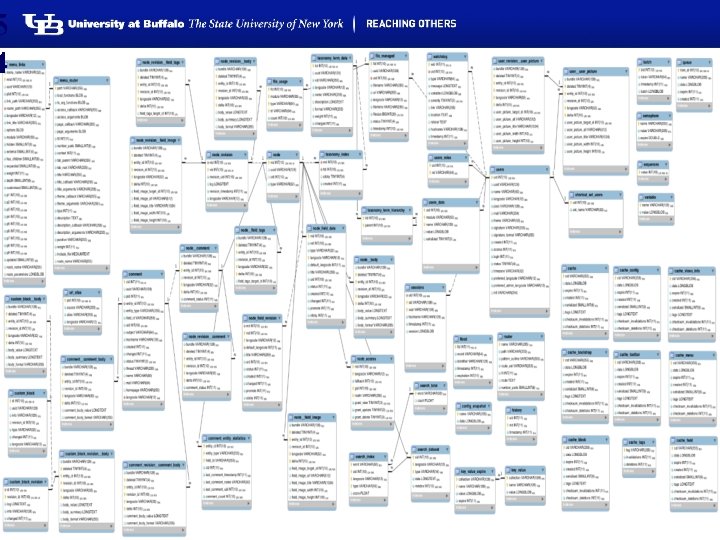
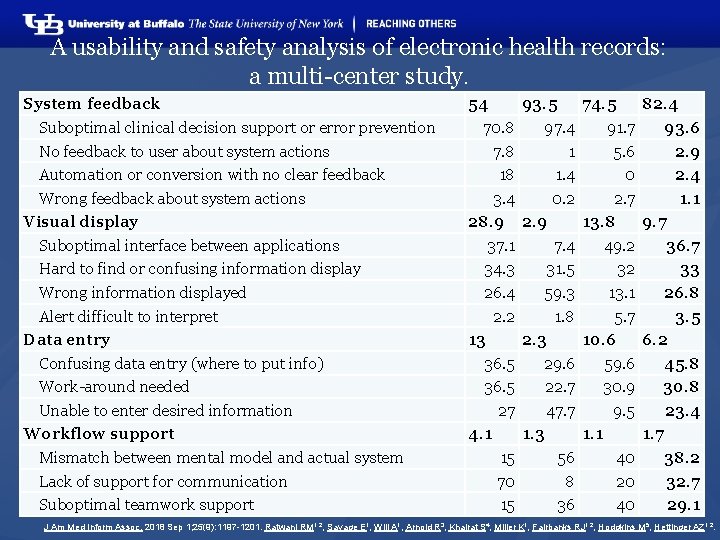
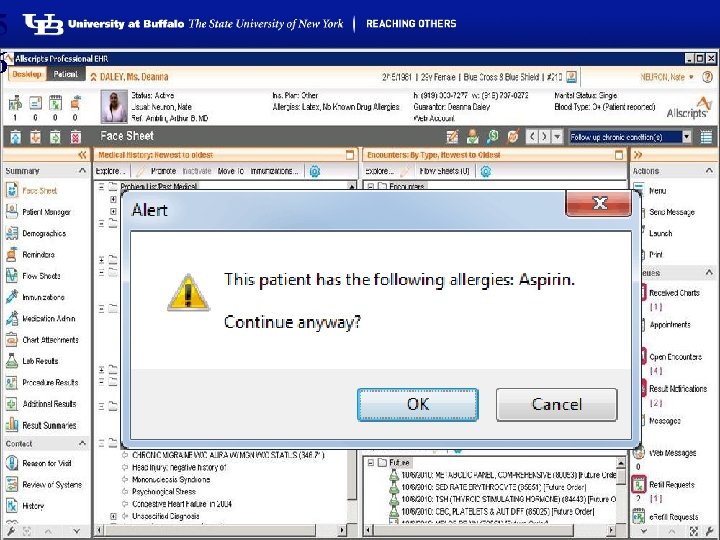
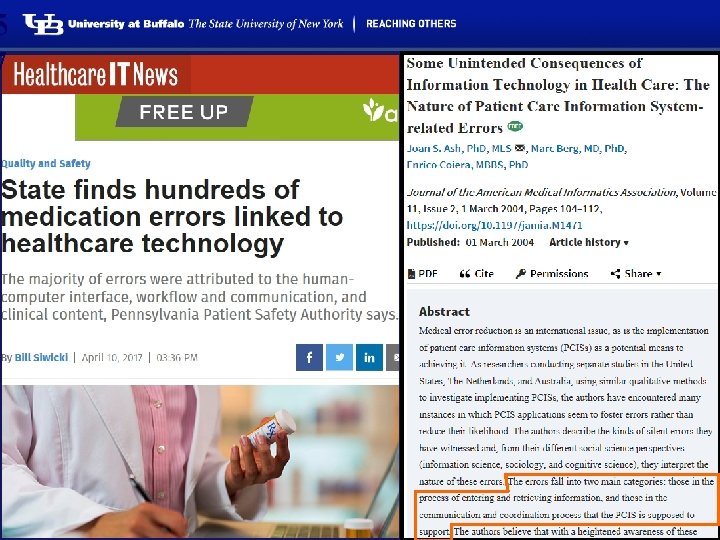
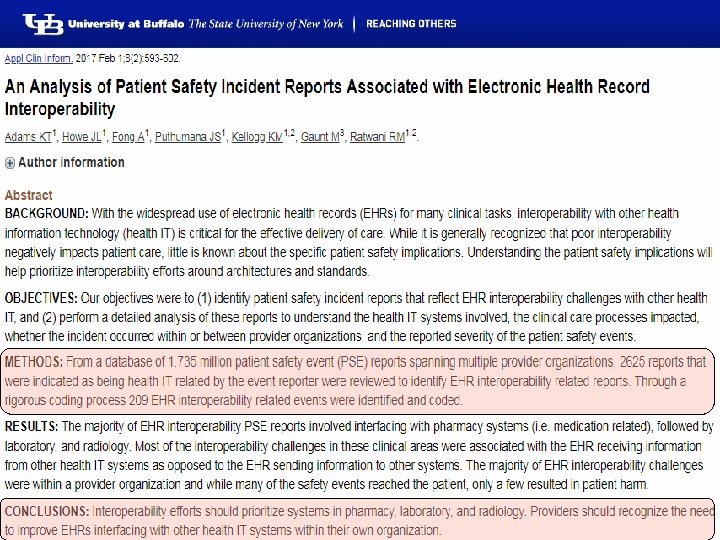
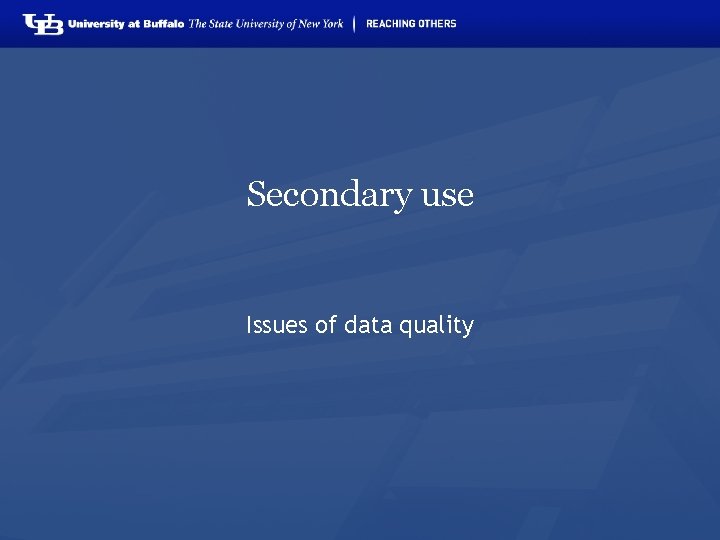
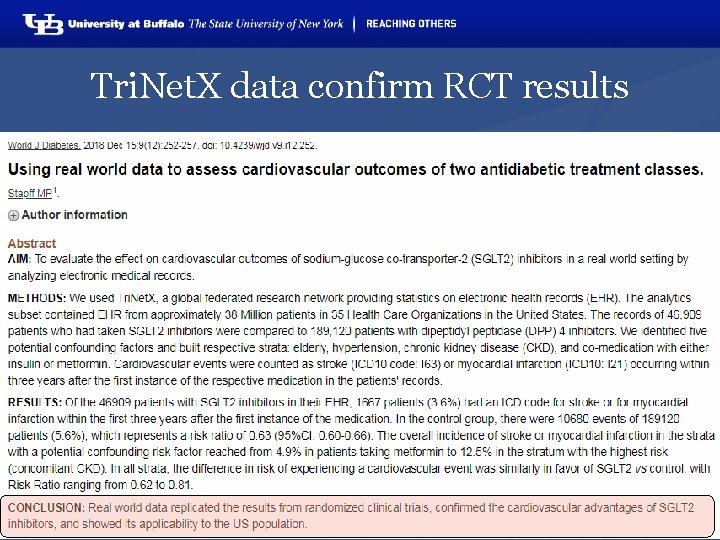
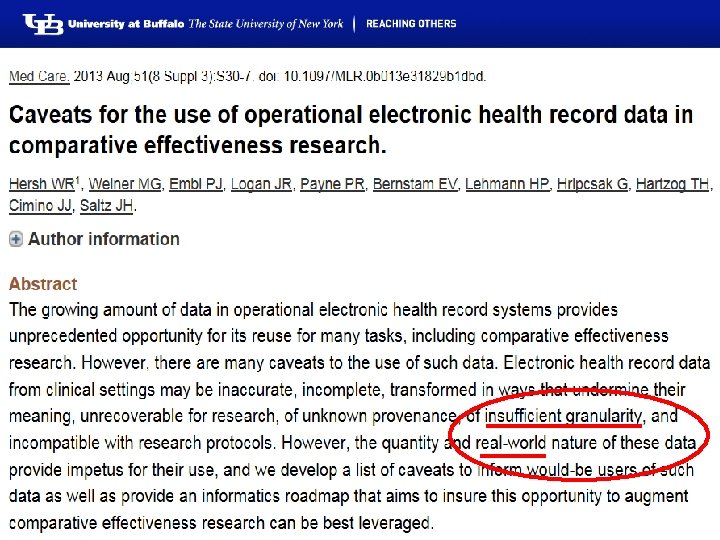
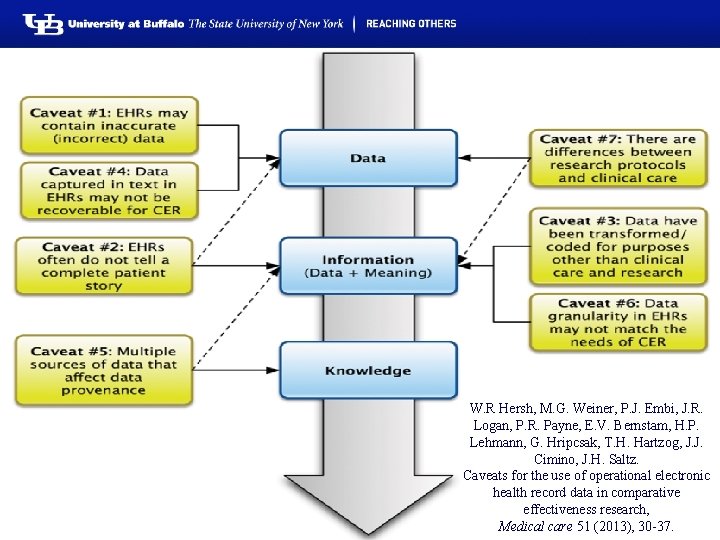
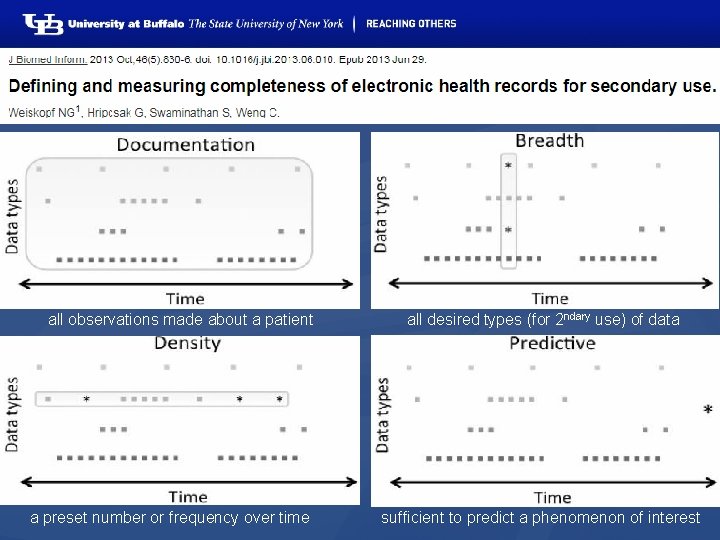
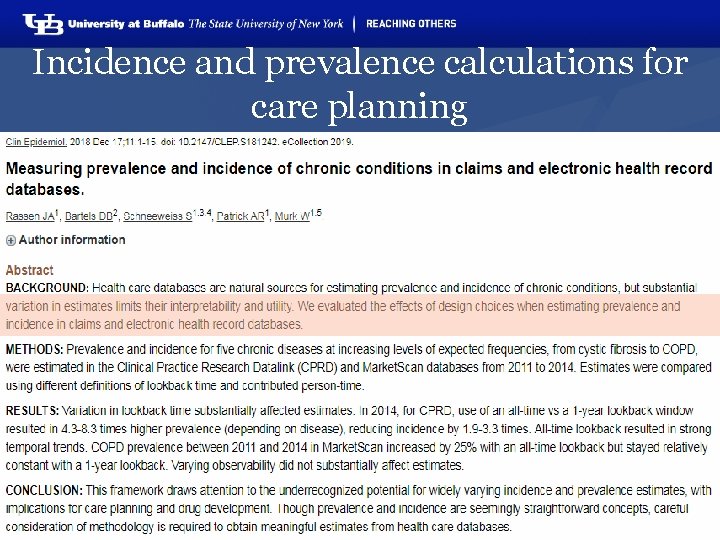
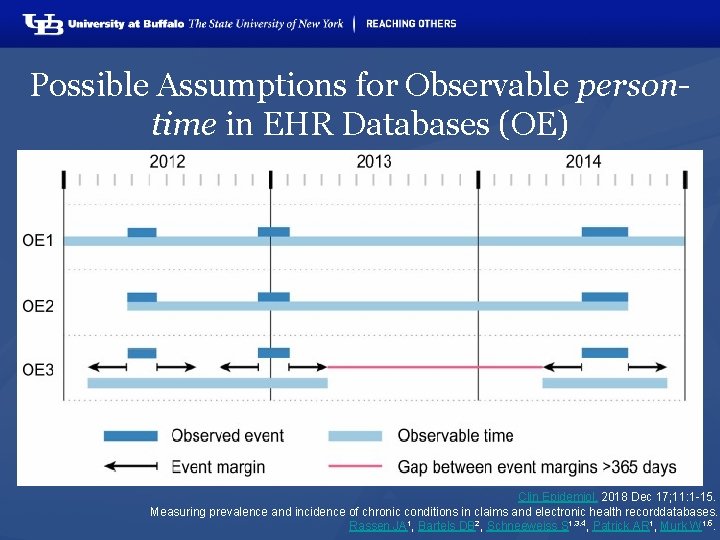
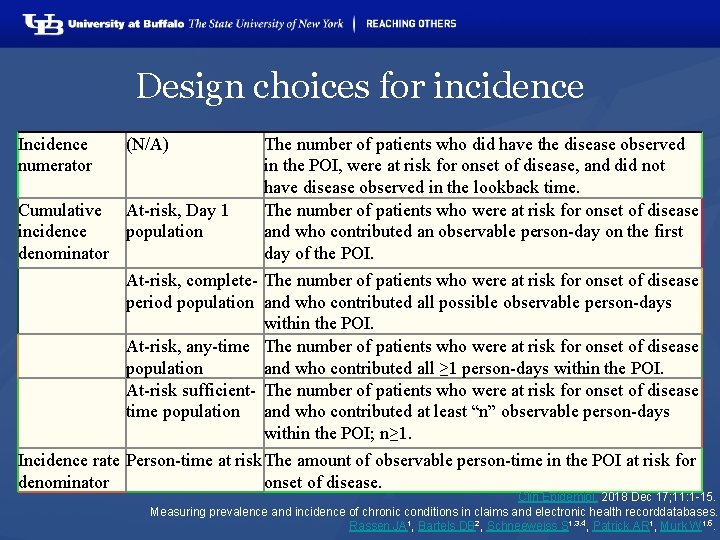
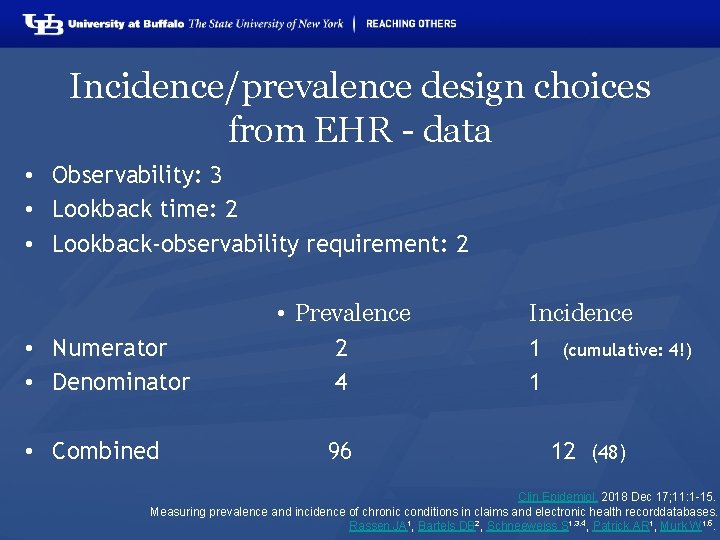
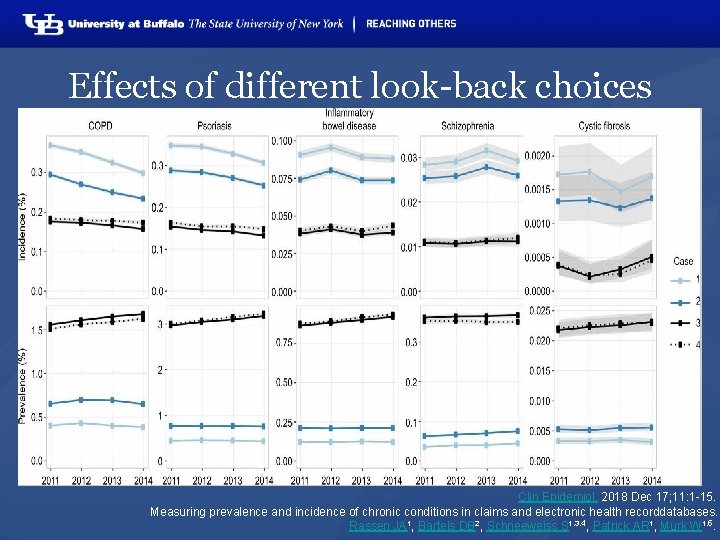
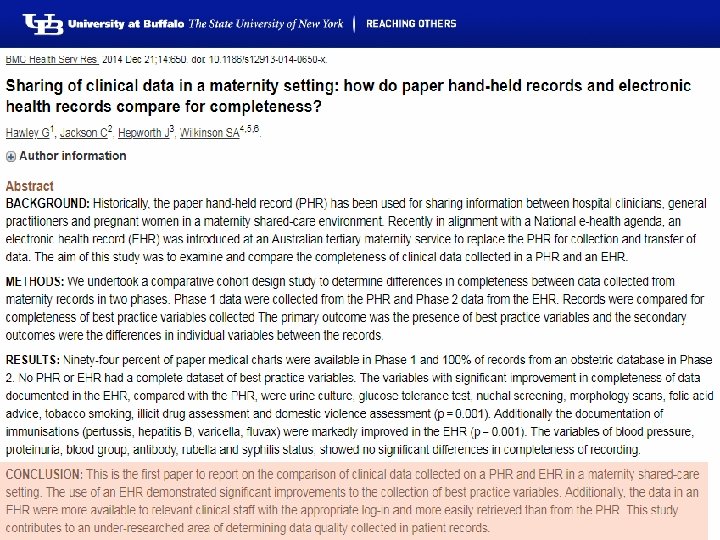
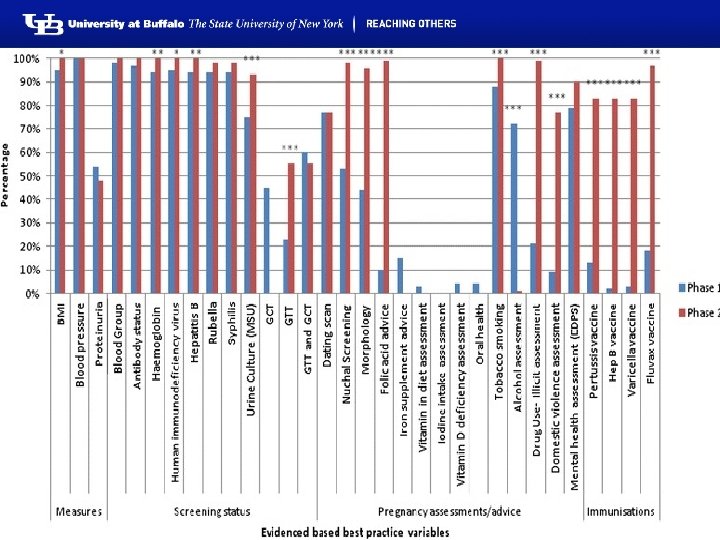
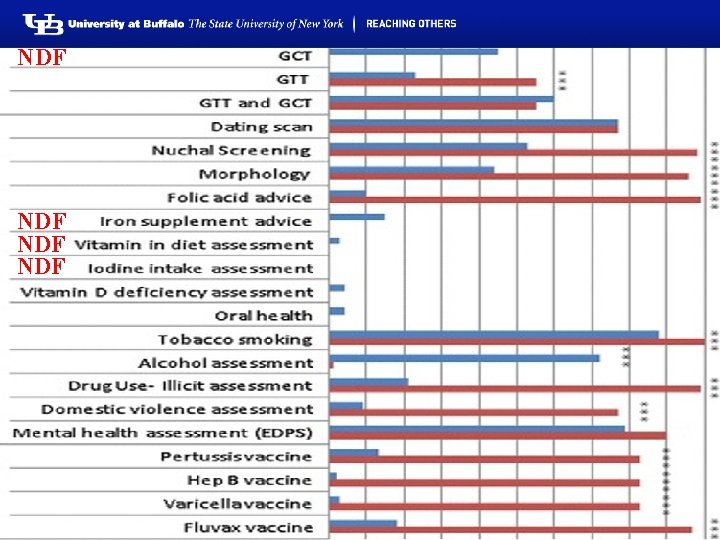
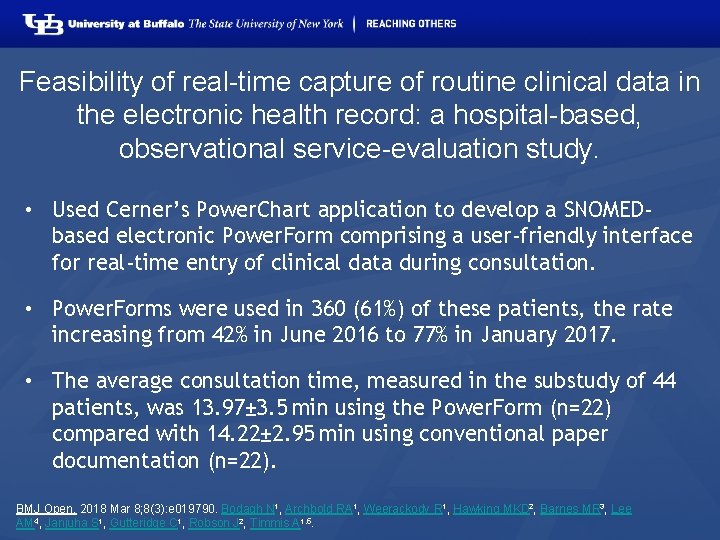
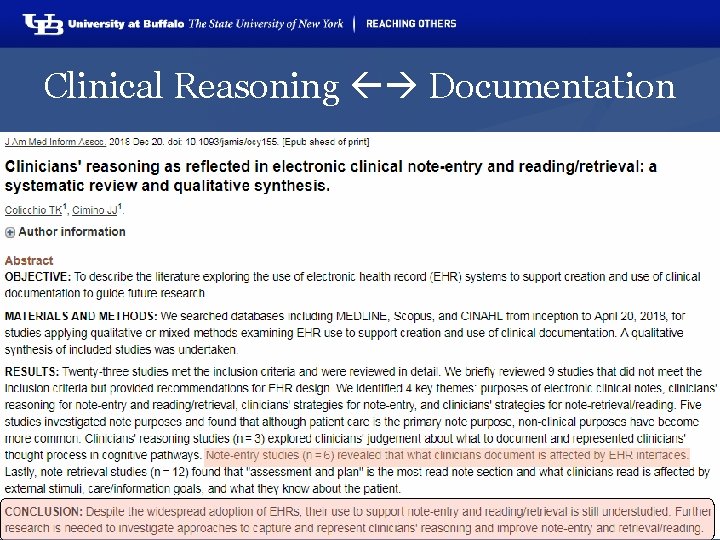
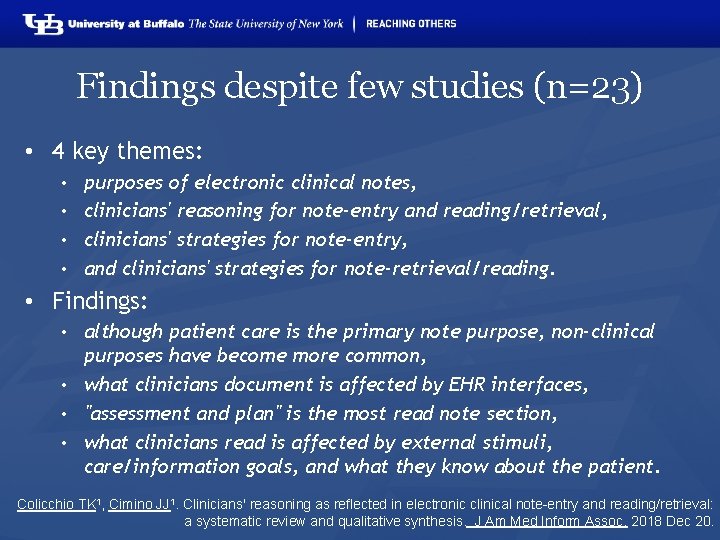
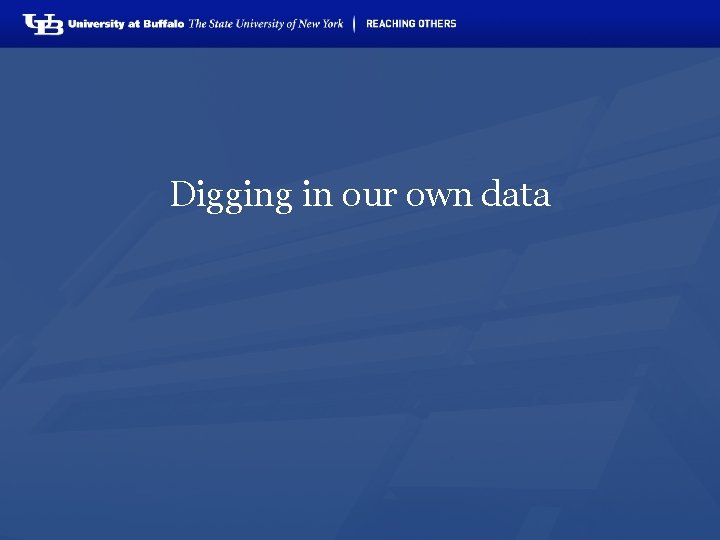
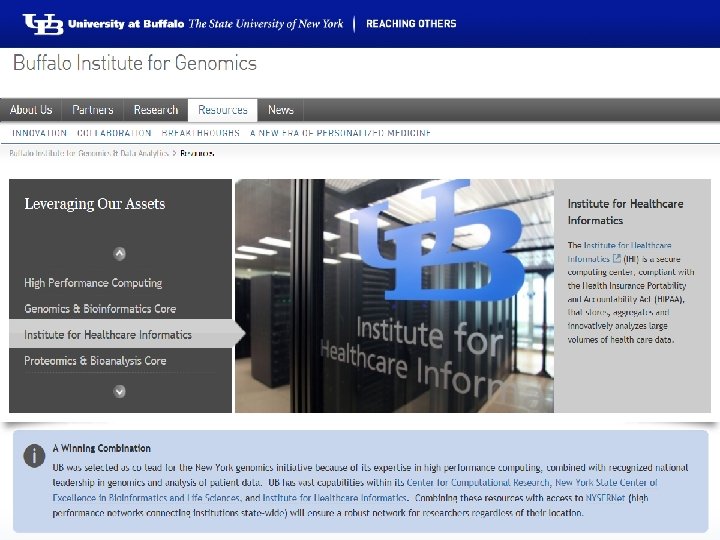
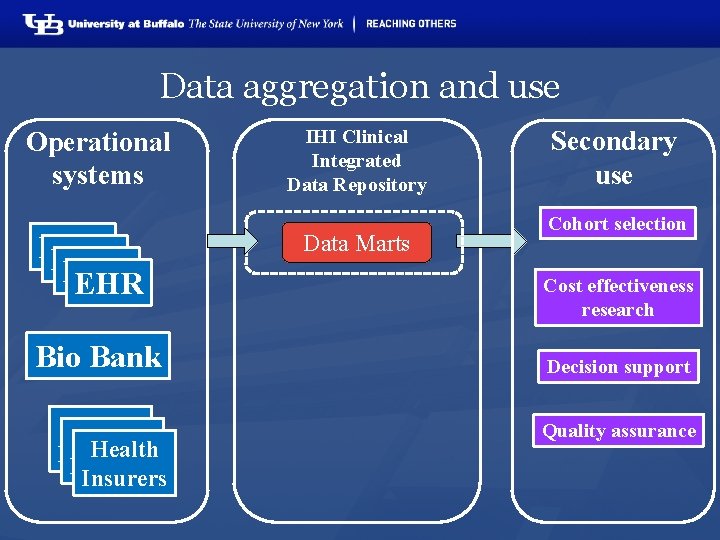
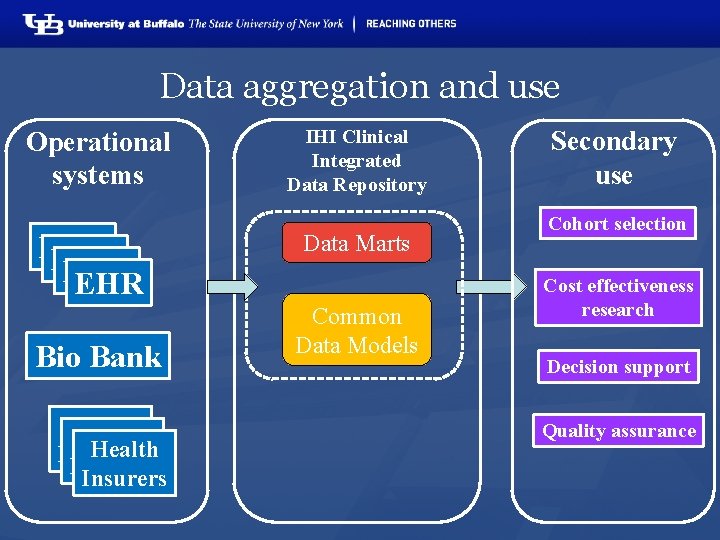
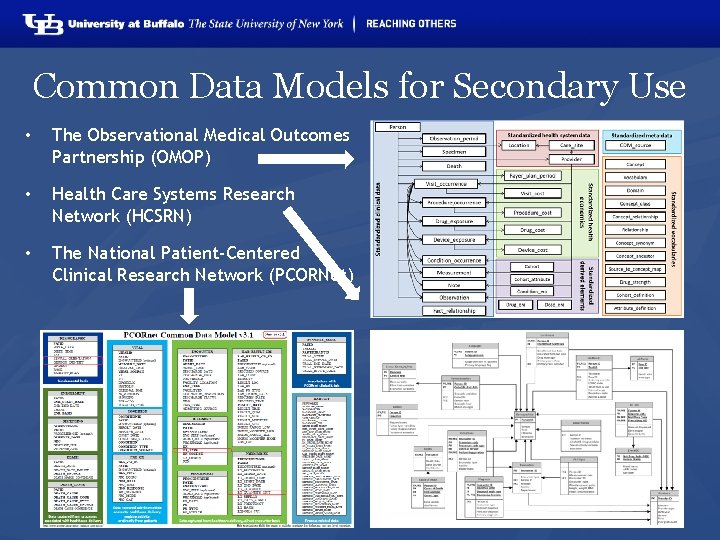
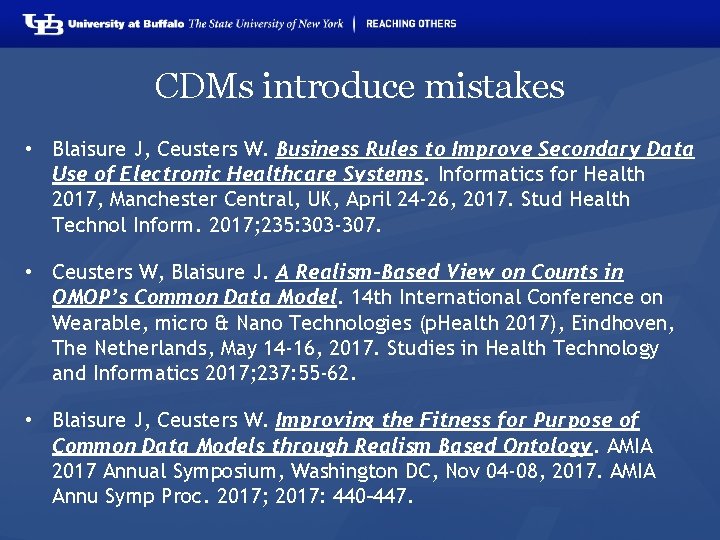
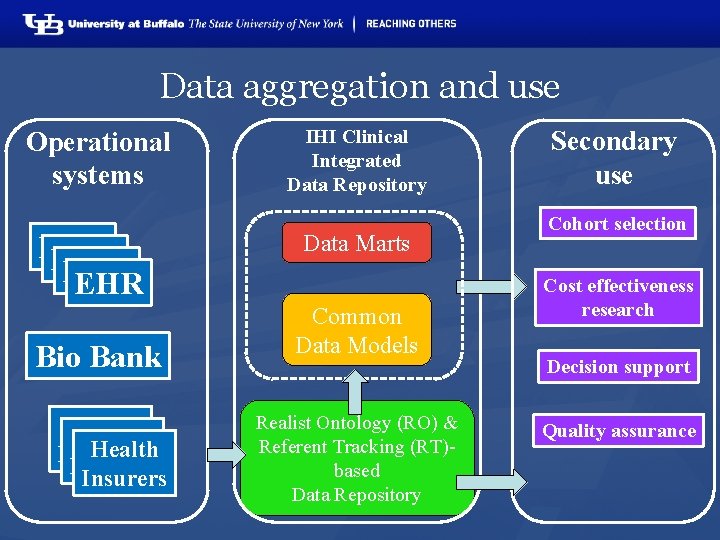
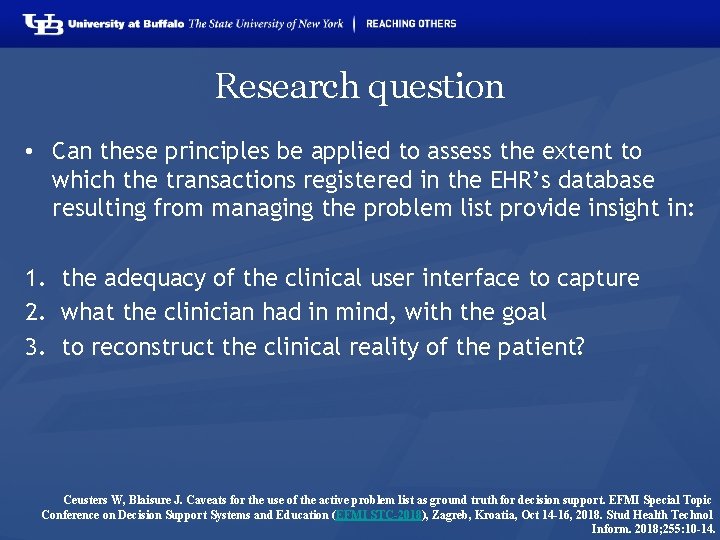
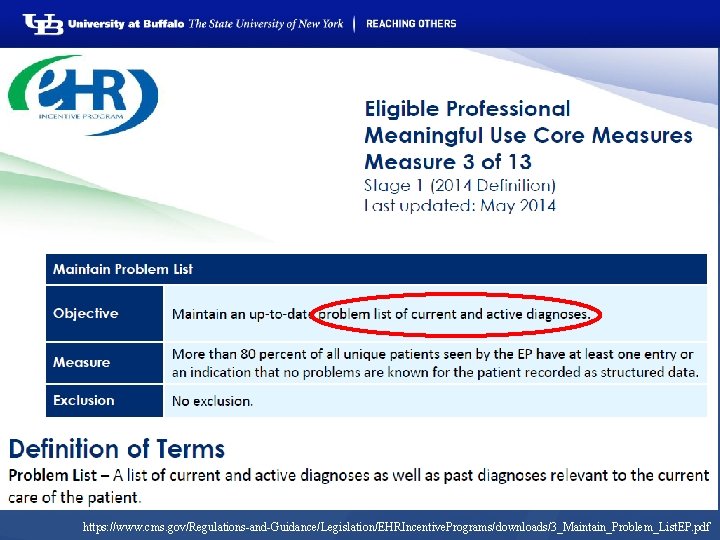
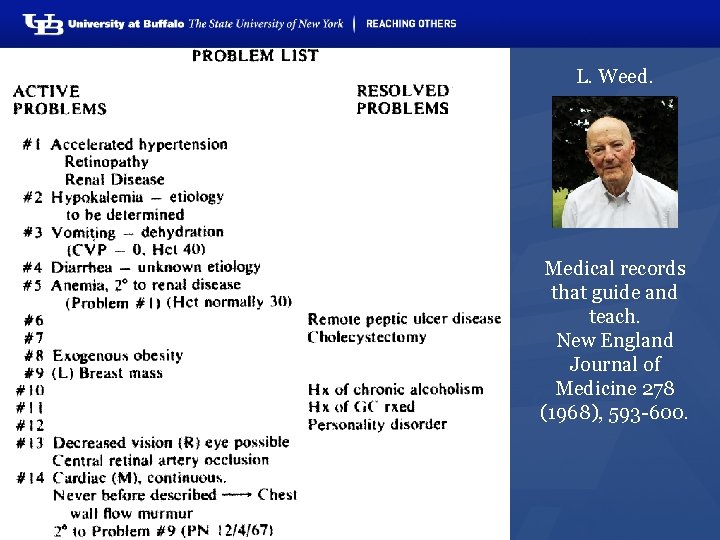
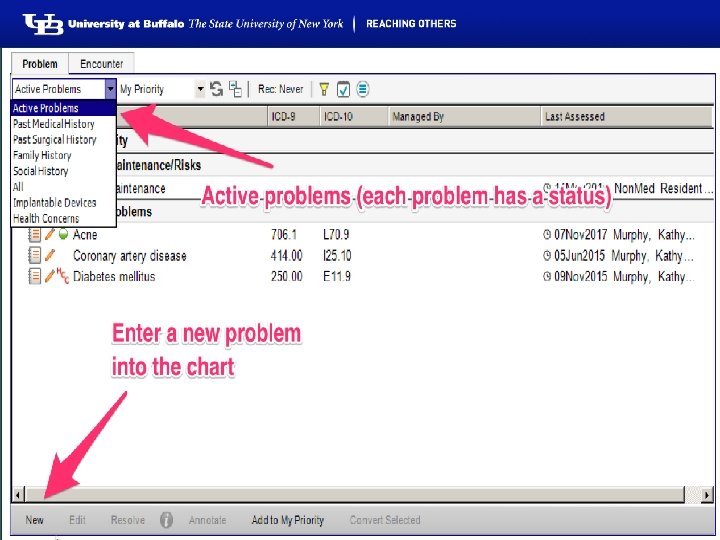
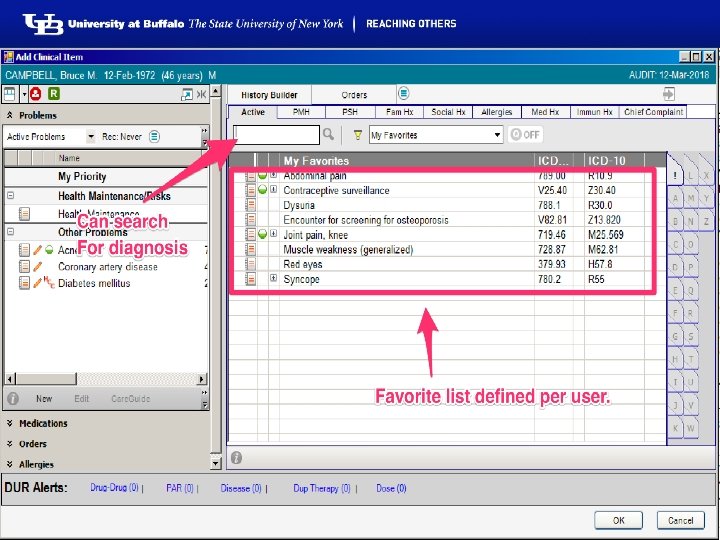
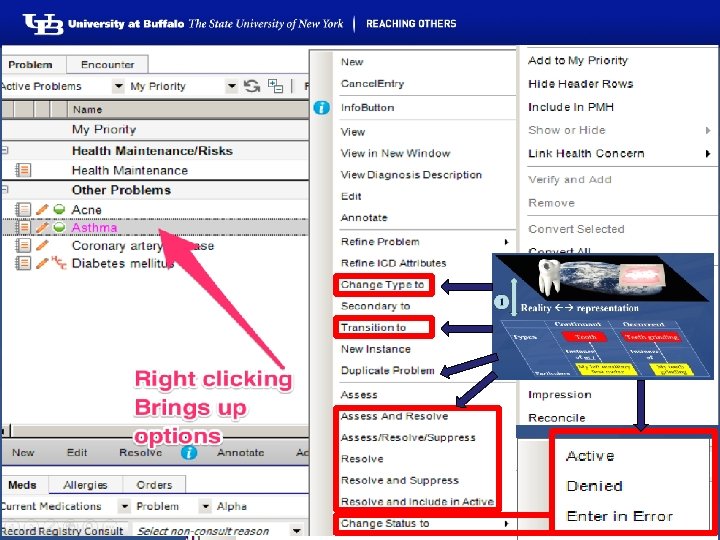
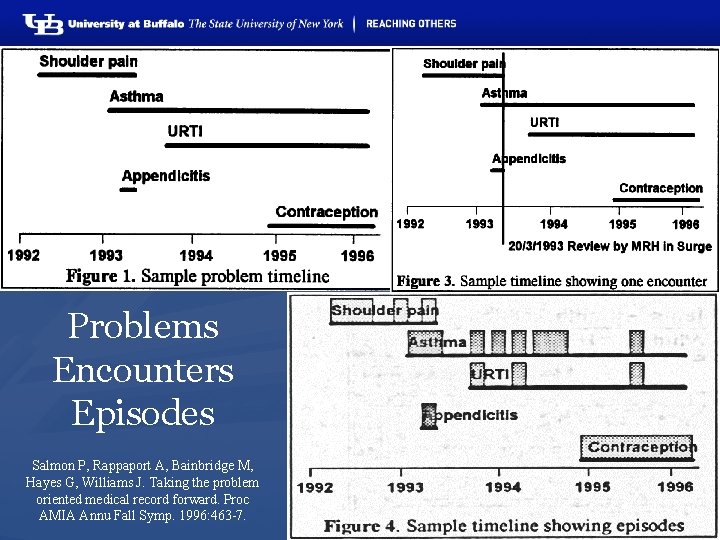
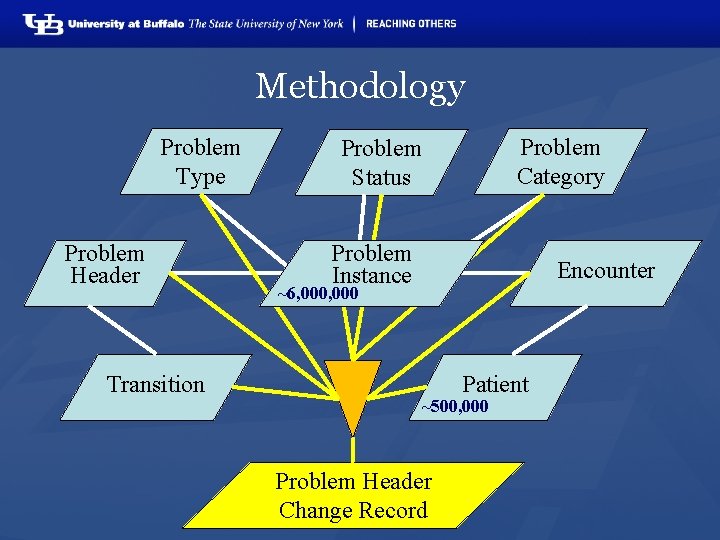
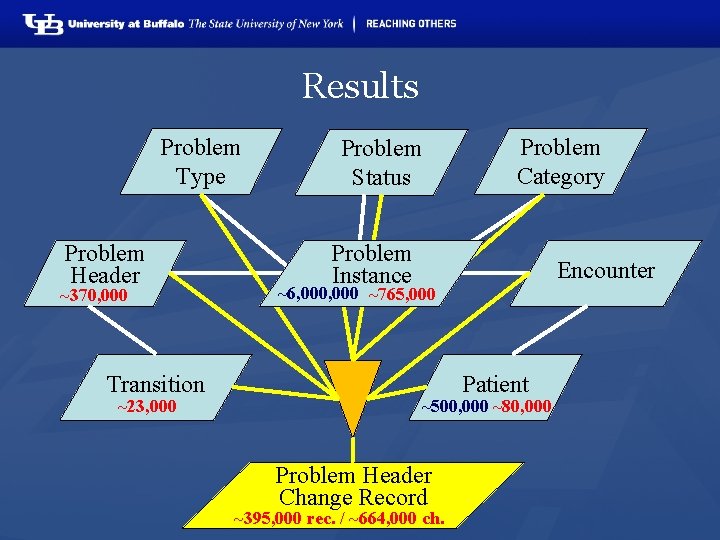
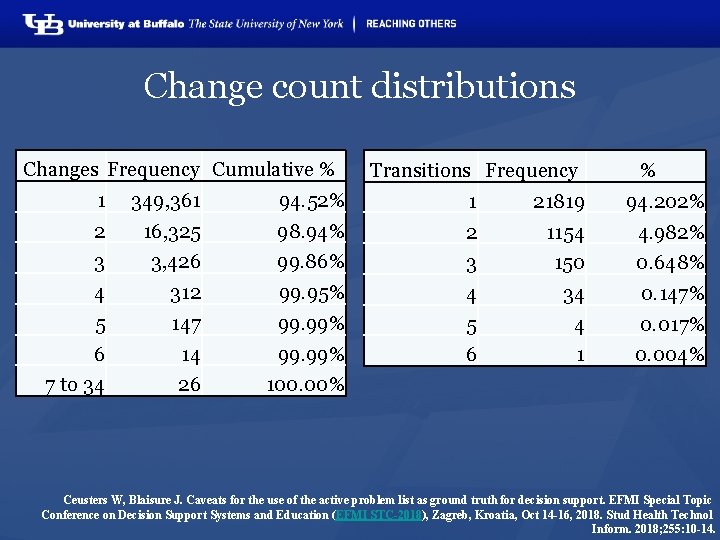
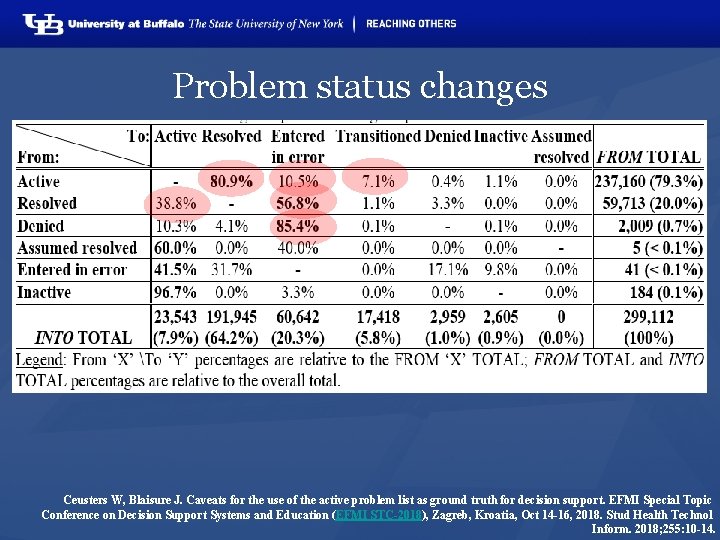
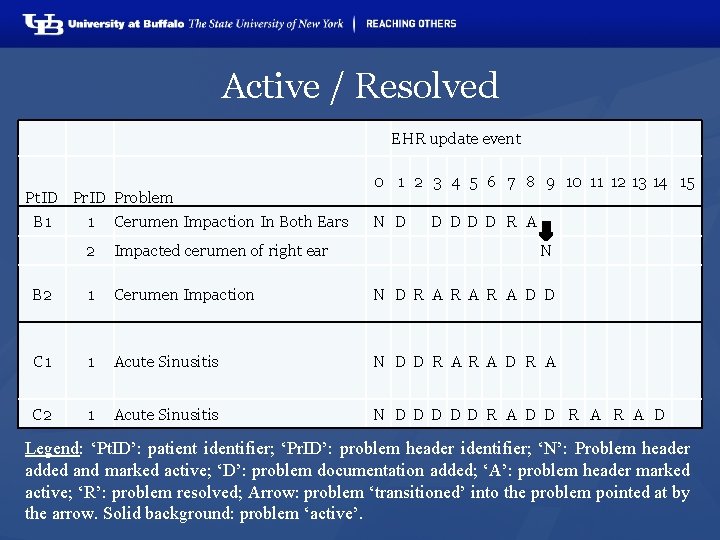
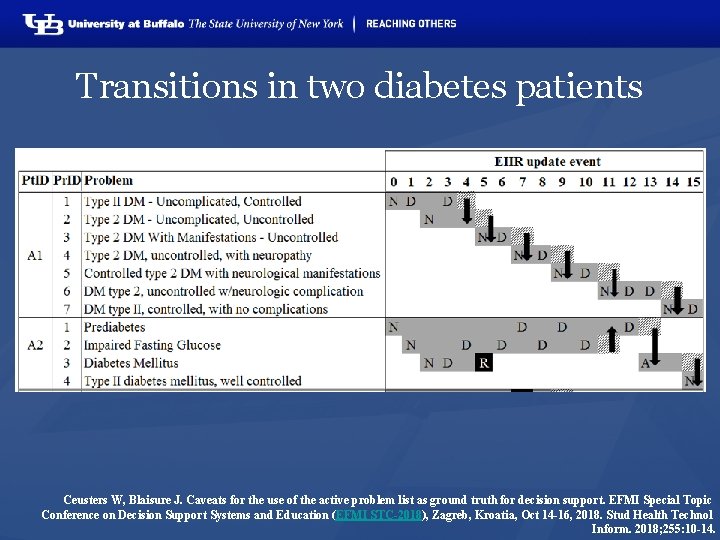
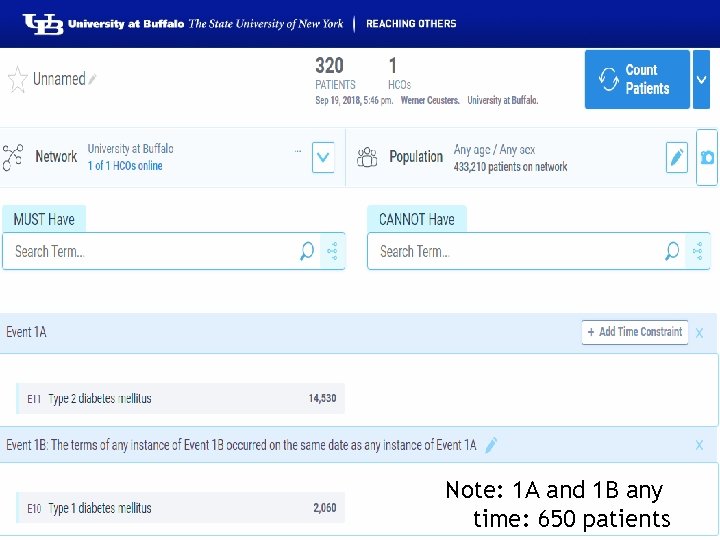
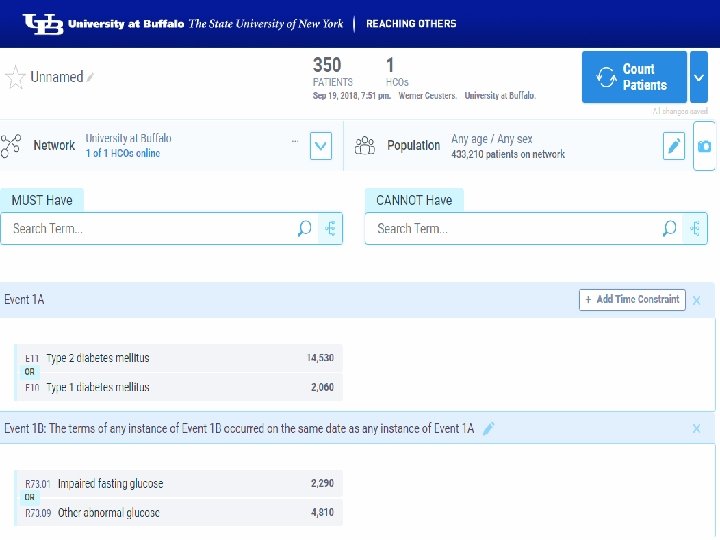
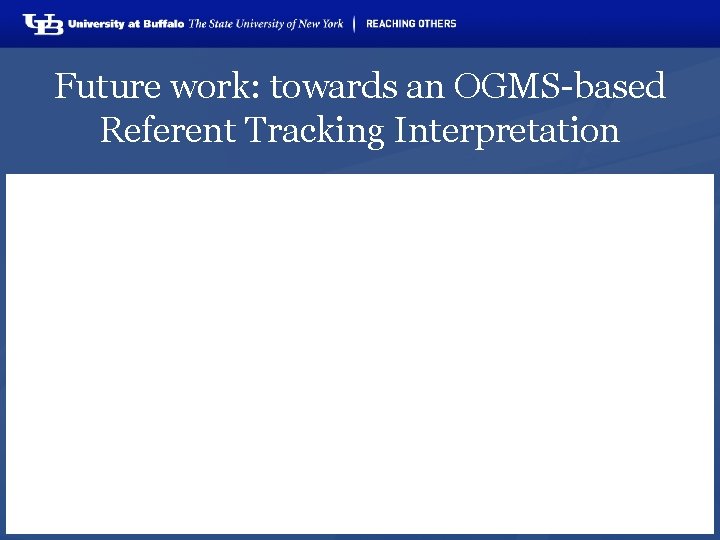
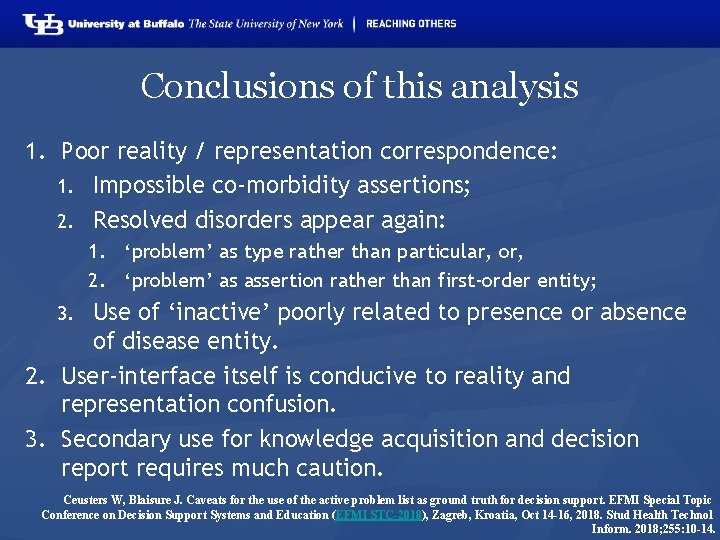
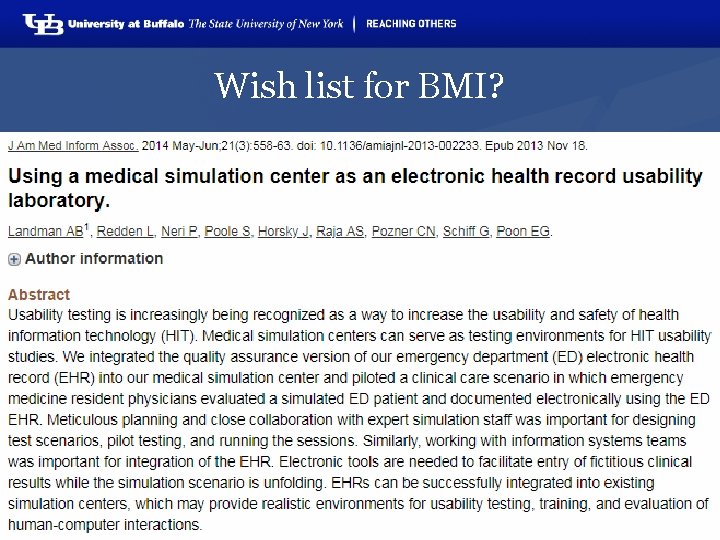
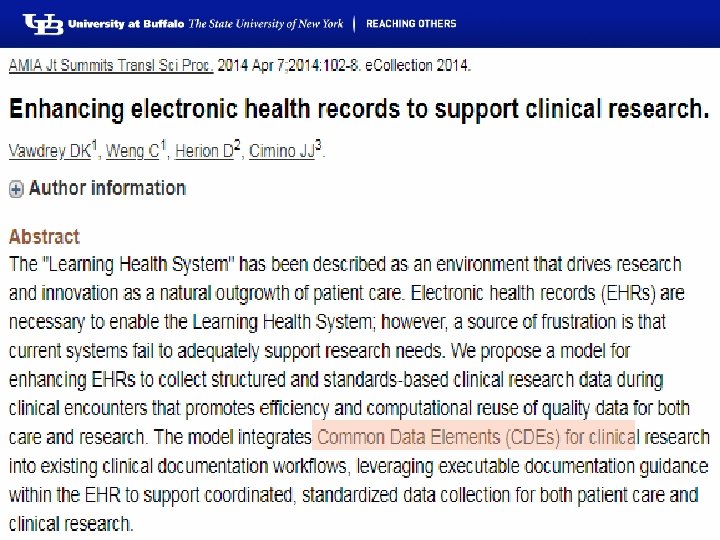
- Slides: 97
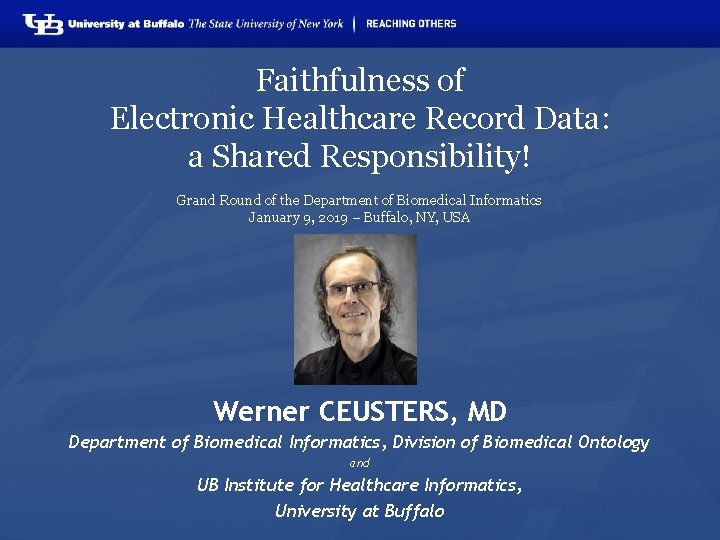
Faithfulness of Electronic Healthcare Record Data: a Shared Responsibility! Grand Round of the Department of Biomedical Informatics January 9, 2019 – Buffalo, NY, USA Werner CEUSTERS, MD Department of Biomedical Informatics, Division of Biomedical Ontology and UB Institute for Healthcare Informatics, University at Buffalo
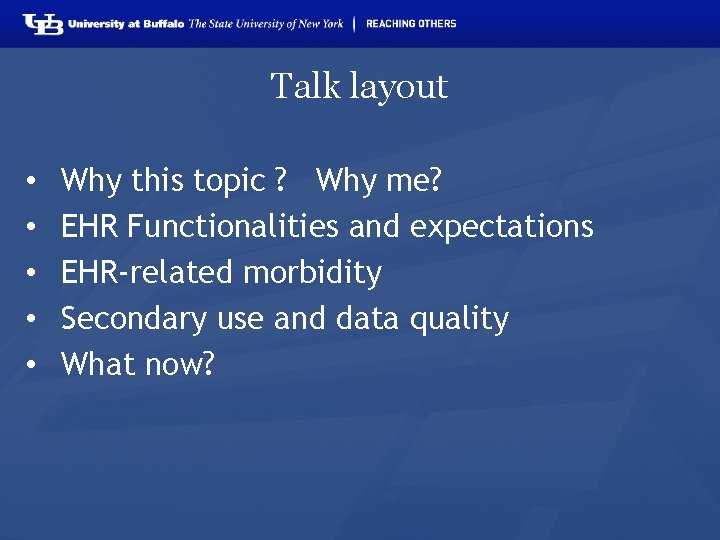
Talk layout • • • Why this topic ? Why me? EHR Functionalities and expectations EHR-related morbidity Secondary use and data quality What now?
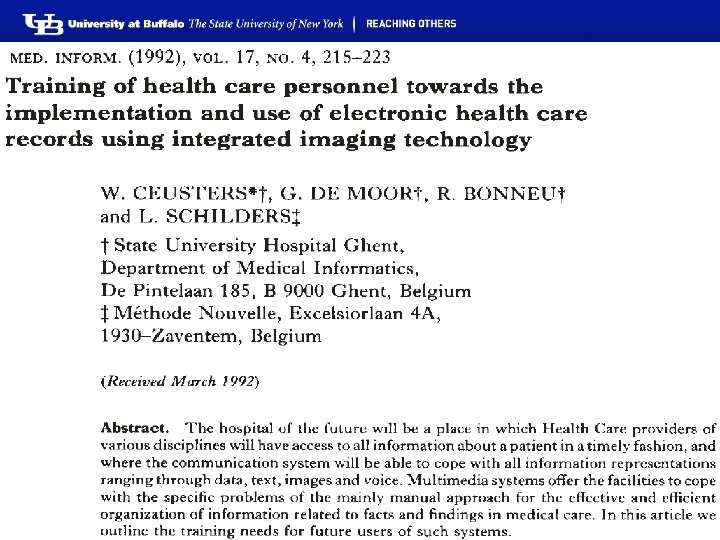
![Pubmed EHR OR EMR count January 3 2019 80 70 60 50 40 Pubmed – [EHR] OR [EMR] count (January 3, 2019) 80 70 60 50 40](https://slidetodoc.com/presentation_image_h2/9b2cf8fbee77a52cd672e0a1d00cf216/image-4.jpg)
Pubmed – [EHR] OR [EMR] count (January 3, 2019) 80 70 60 50 40 30 20 10 0 1940 1950 1960 1970 1980 1990 2000 2010
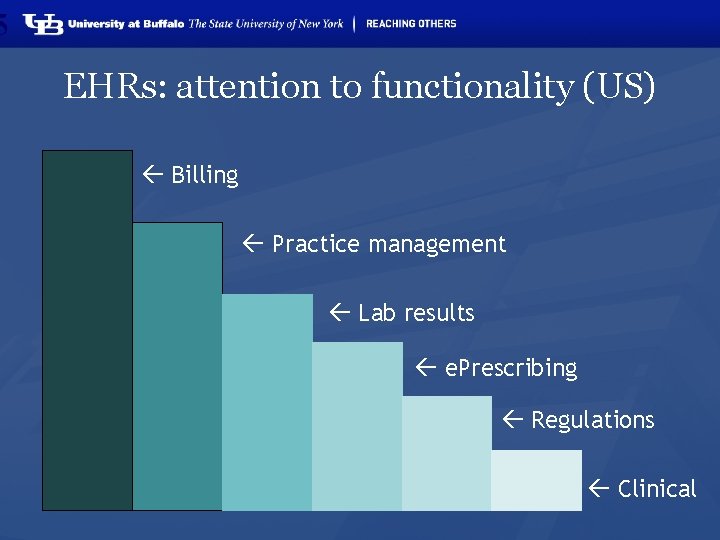
5 EHRs: attention to functionality (US) Billing Practice management Lab results e. Prescribing Regulations Clinical
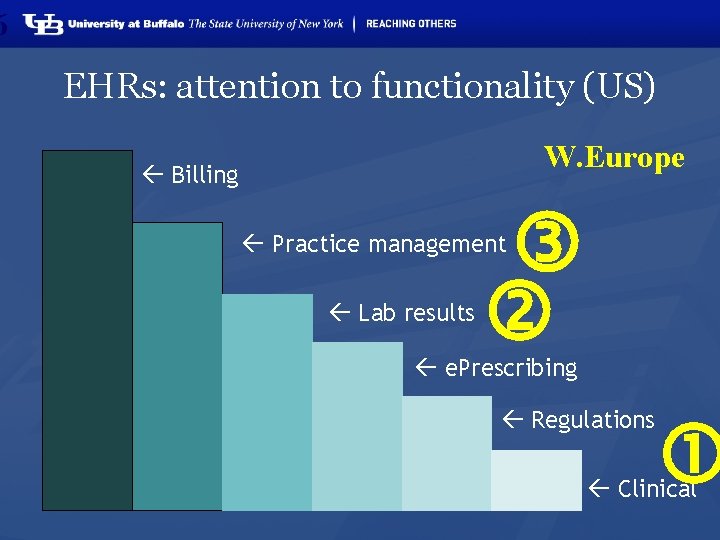
6 EHRs: attention to functionality (US) W. Europe Billing Practice management Lab results e. Prescribing Regulations Clinical
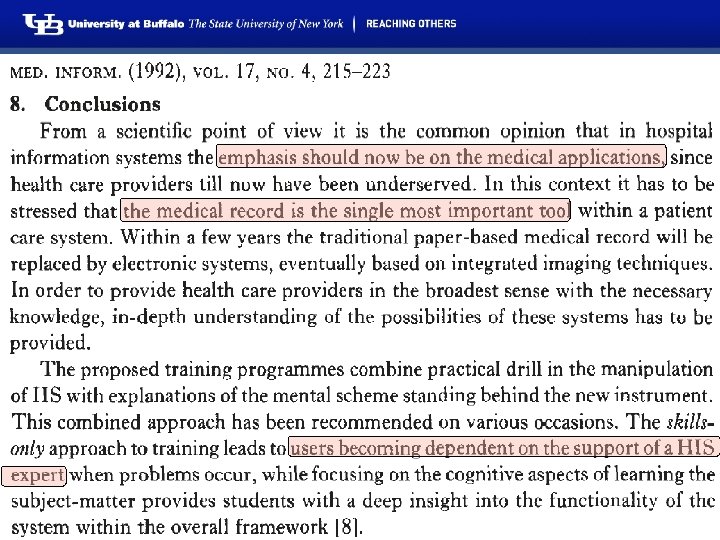
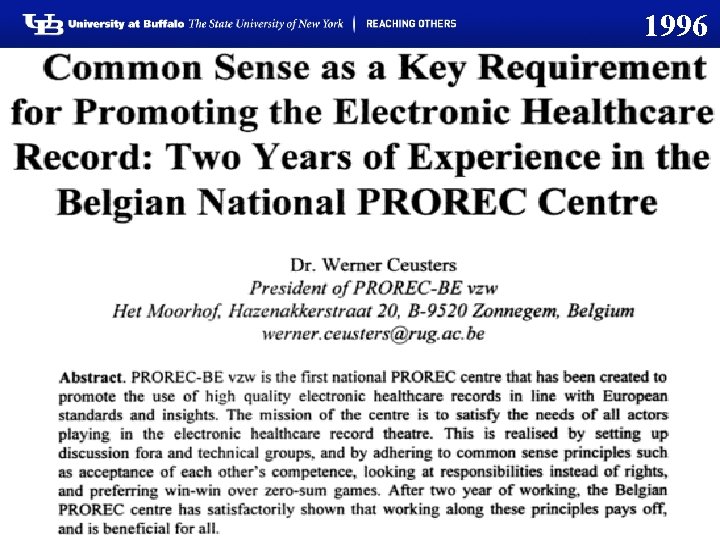
1996
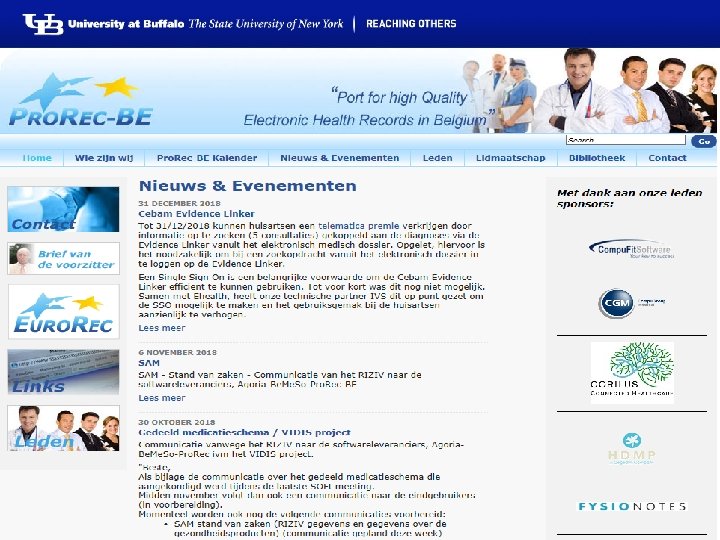
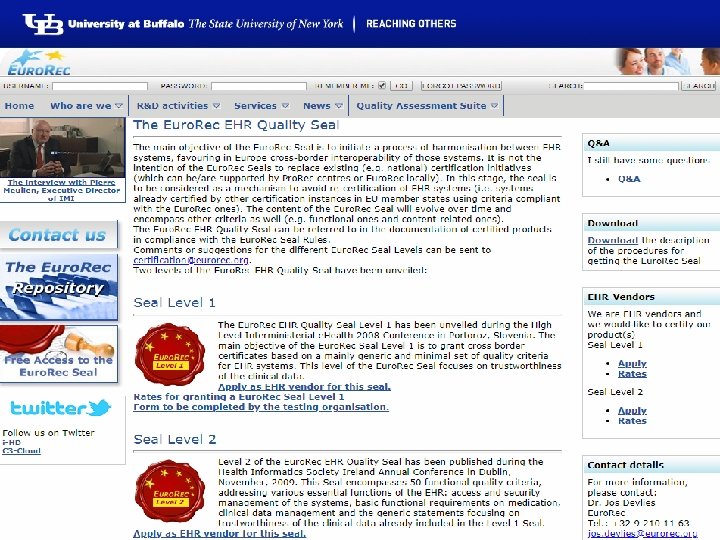
![Pubmed EHR OR EMR count January 3 2019 80 70 60 50 40 Pubmed – [EHR] OR [EMR] count (January 3, 2019) 80 70 60 50 40](https://slidetodoc.com/presentation_image_h2/9b2cf8fbee77a52cd672e0a1d00cf216/image-11.jpg)
Pubmed – [EHR] OR [EMR] count (January 3, 2019) 80 70 60 50 40 30 20 10 0 1940 1950 1960 1970 1980 1990 2000 2010
![Pubmed EHR OR EMR count January 3 2019 1800 1600 1400 1200 1000 Pubmed – [EHR] OR [EMR] count (January 3, 2019) 1800 1600 1400 1200 1000](https://slidetodoc.com/presentation_image_h2/9b2cf8fbee77a52cd672e0a1d00cf216/image-12.jpg)
Pubmed – [EHR] OR [EMR] count (January 3, 2019) 1800 1600 1400 1200 1000 800 600 400 200 0 1940 1950 1960 1970 1980 1990 2000 2010 2020 2030
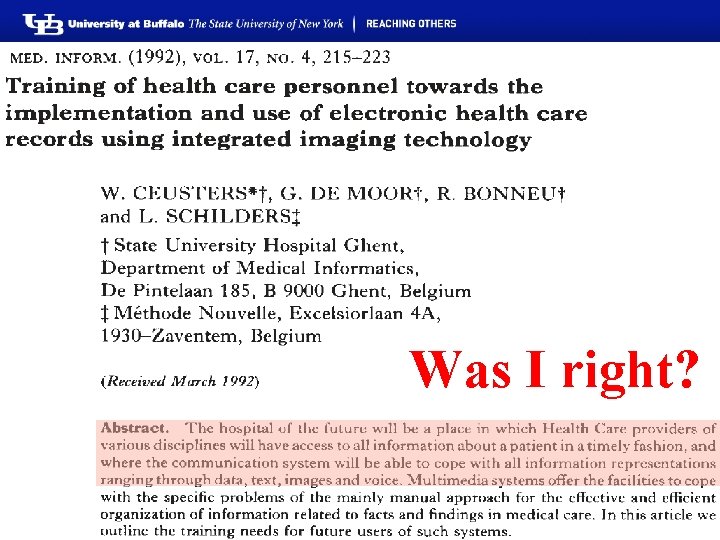
Was I right?
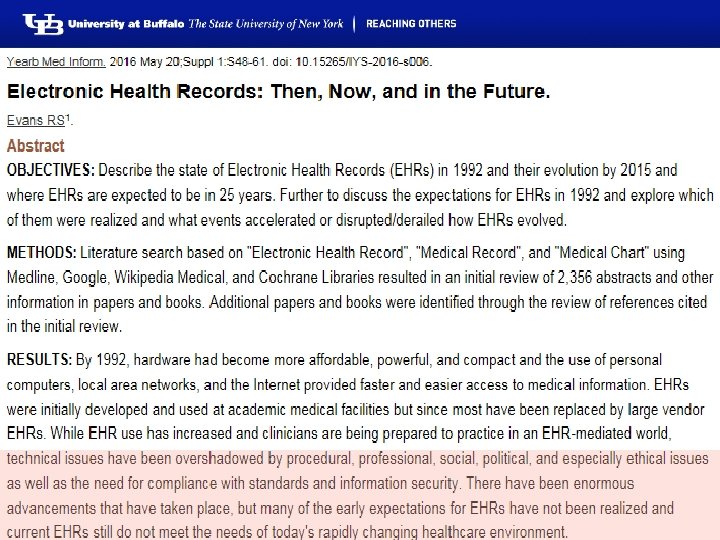
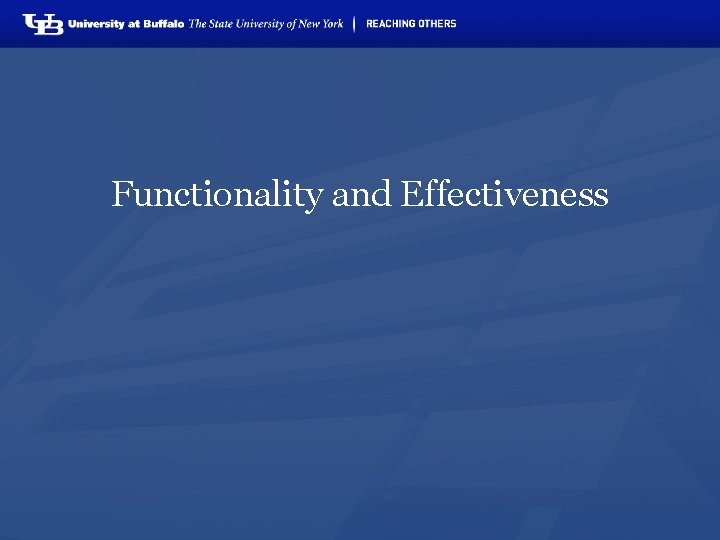
Functionality and Effectiveness
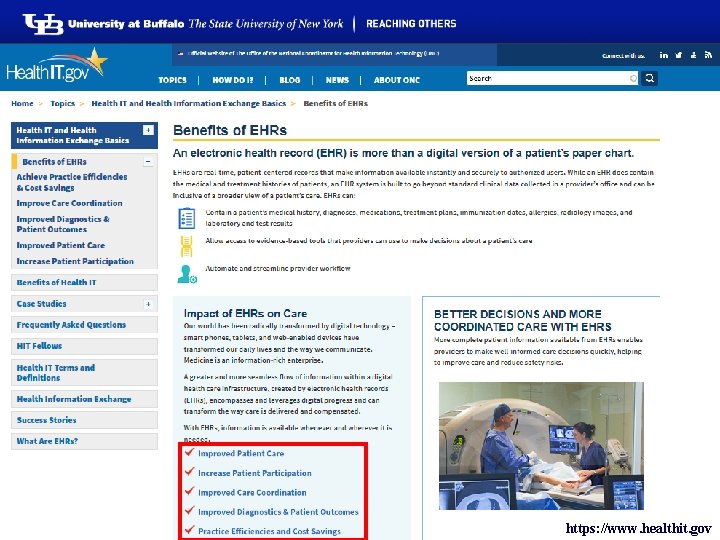
https: //www. healthit. gov
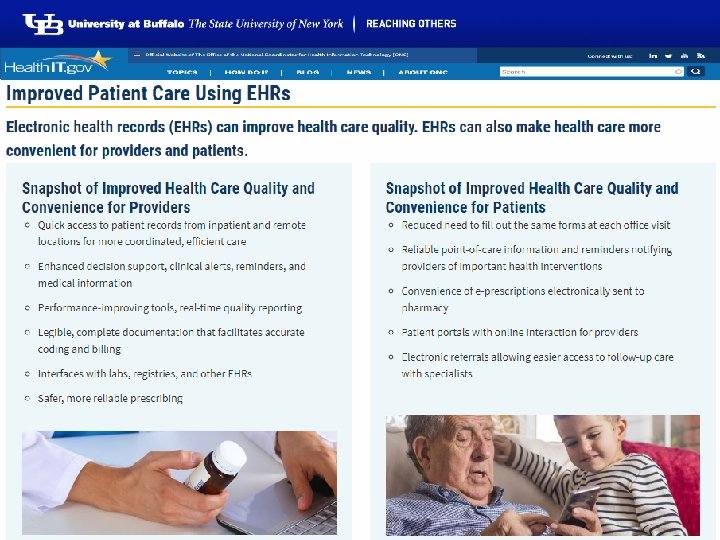
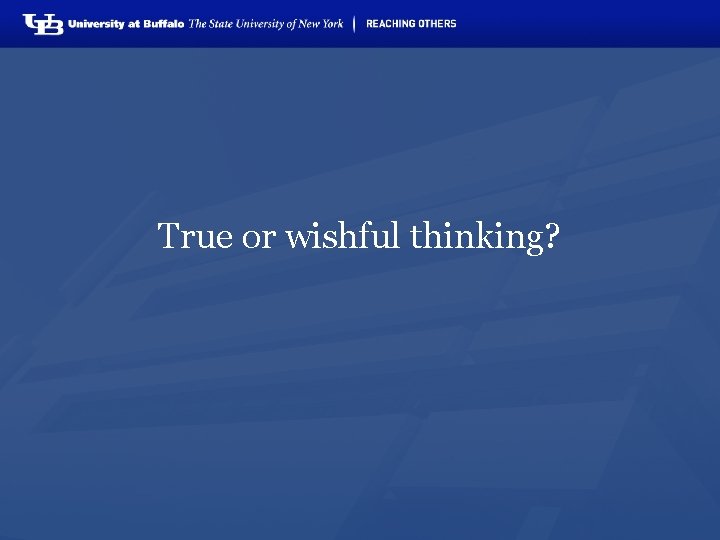
True or wishful thinking?
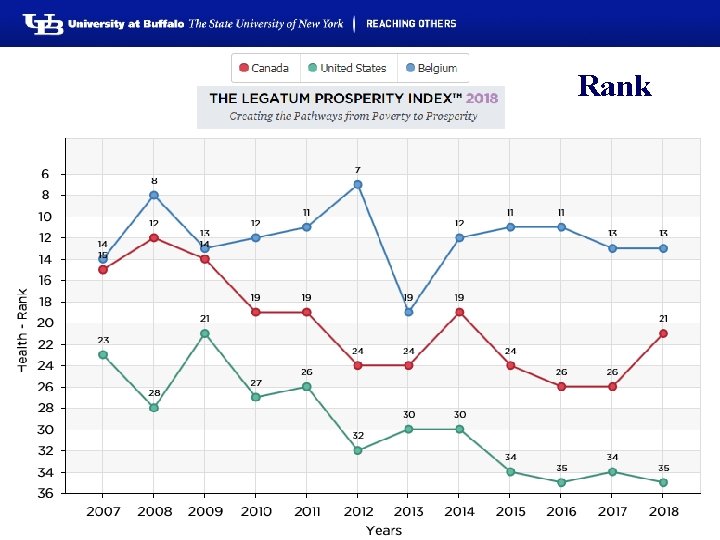
Rank
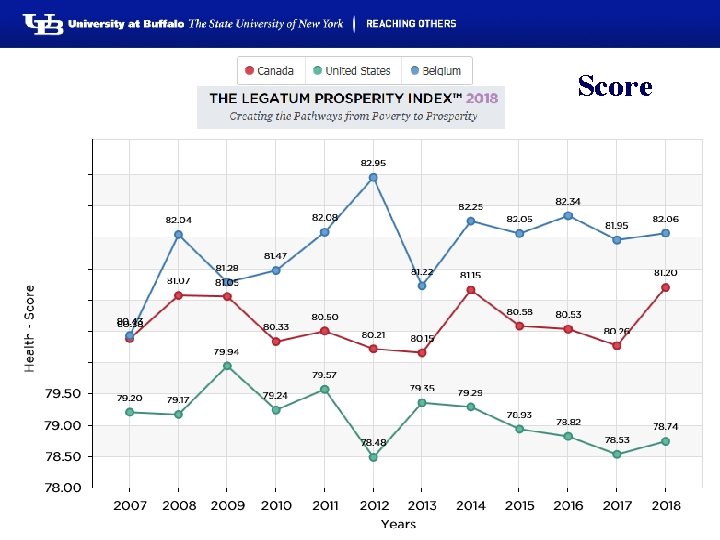
Score
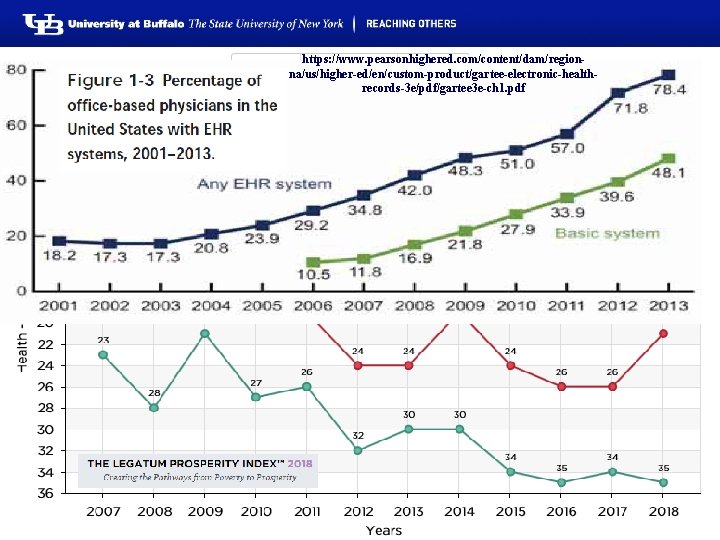
https: //www. pearsonhighered. com/content/dam/regionna/us/higher-ed/en/custom-product/gartee-electronic-healthrecords-3 e/pdf/gartee 3 e-ch 1. pdf
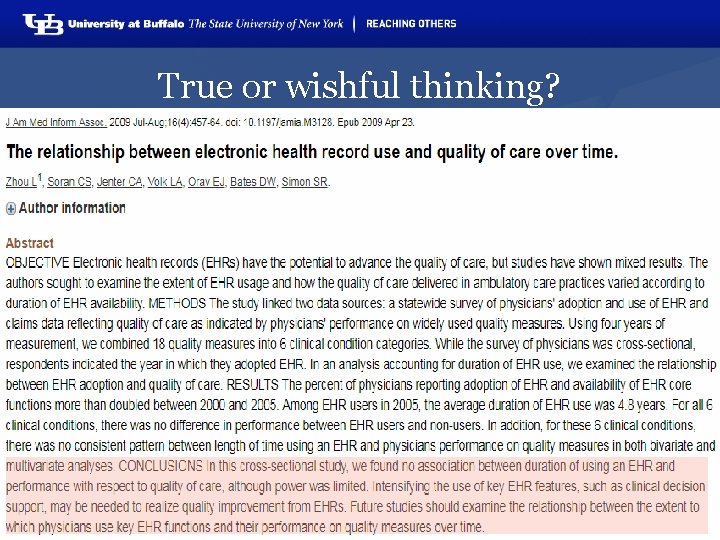
True or wishful thinking?
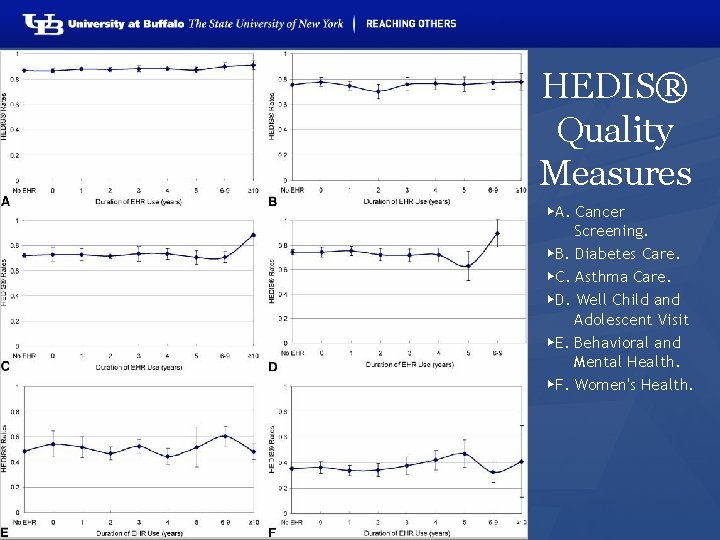
HEDIS® Quality Measures ▶A. Cancer Screening. ▶B. Diabetes Care. ▶C. Asthma Care. ▶D. Well Child and Adolescent Visit ▶E. Behavioral and Mental Health. ▶F. Women's Health.
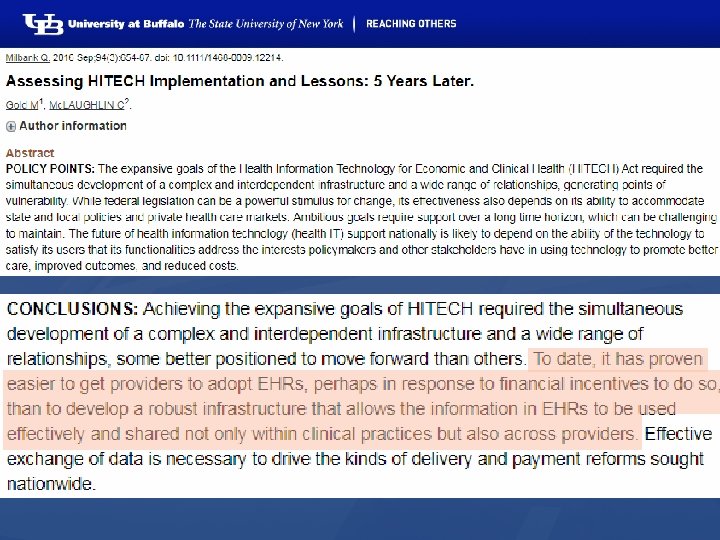
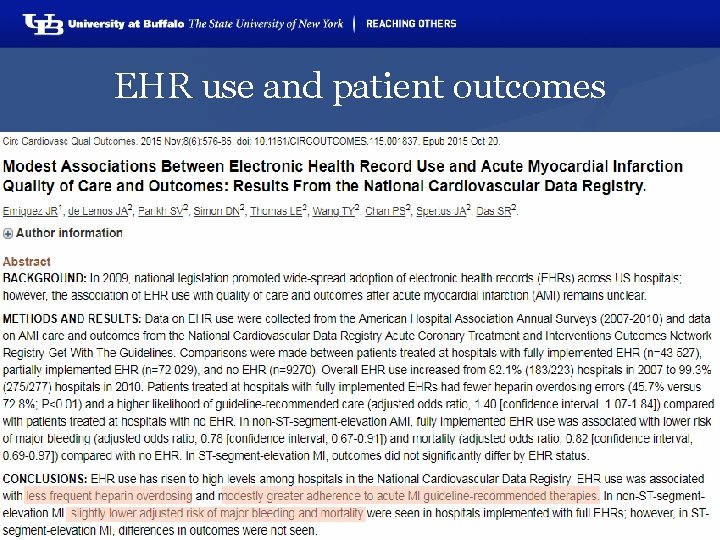
EHR use and patient outcomes
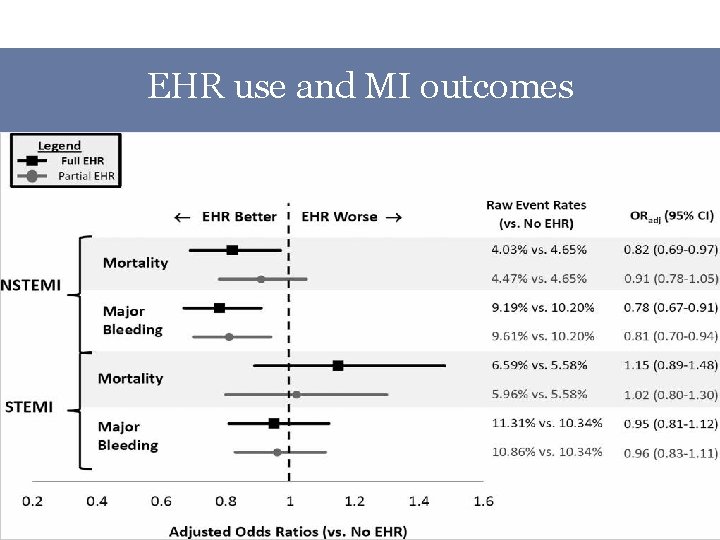
EHR use and MI outcomes
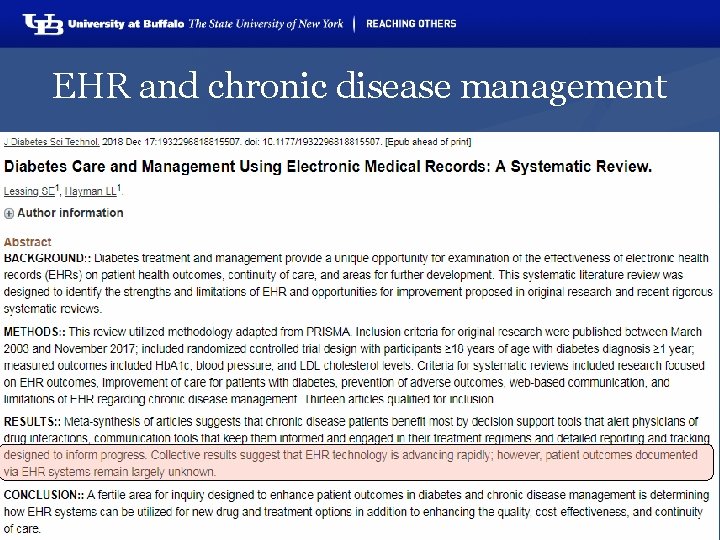
EHR and chronic disease management
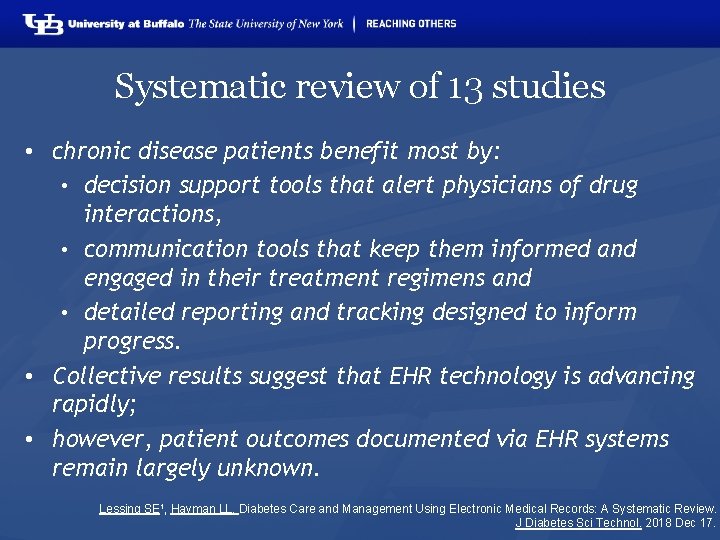
Systematic review of 13 studies • chronic disease patients benefit most by: • decision support tools that alert physicians of drug interactions, • communication tools that keep them informed and engaged in their treatment regimens and • detailed reporting and tracking designed to inform progress. • Collective results suggest that EHR technology is advancing rapidly; • however, patient outcomes documented via EHR systems remain largely unknown. Lessing SE 1, Hayman LL. Diabetes Care and Management Using Electronic Medical Records: A Systematic Review. J Diabetes Sci Technol. 2018 Dec 17.
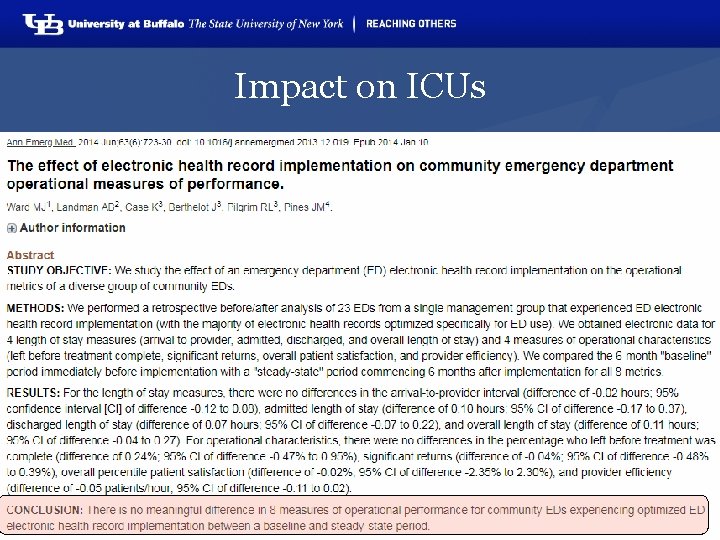
Impact on ICUs
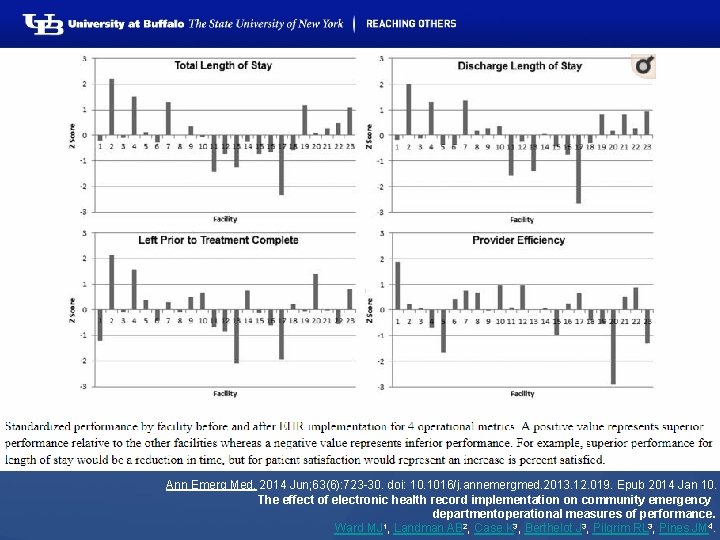
Ann Emerg Med. 2014 Jun; 63(6): 723 -30. doi: 10. 1016/j. annemergmed. 2013. 12. 019. Epub 2014 Jan 10. The effect of electronic health record implementation on community emergency departmentoperational measures of performance. 1 Ward MJ , Landman AB 2, Case K 3, Berthelot J 3, Pilgrim RL 3, Pines JM 4.
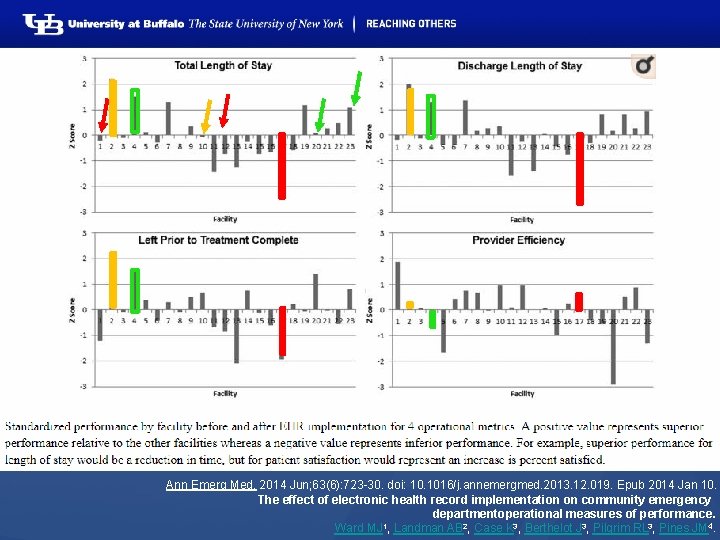
Ann Emerg Med. 2014 Jun; 63(6): 723 -30. doi: 10. 1016/j. annemergmed. 2013. 12. 019. Epub 2014 Jan 10. The effect of electronic health record implementation on community emergency departmentoperational measures of performance. 1 Ward MJ , Landman AB 2, Case K 3, Berthelot J 3, Pilgrim RL 3, Pines JM 4.
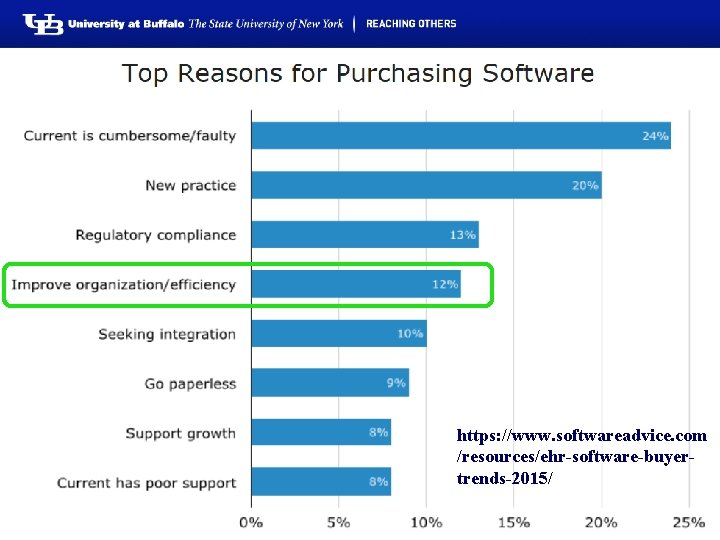
https: //www. softwareadvice. com /resources/ehr-software-buyertrends-2015/
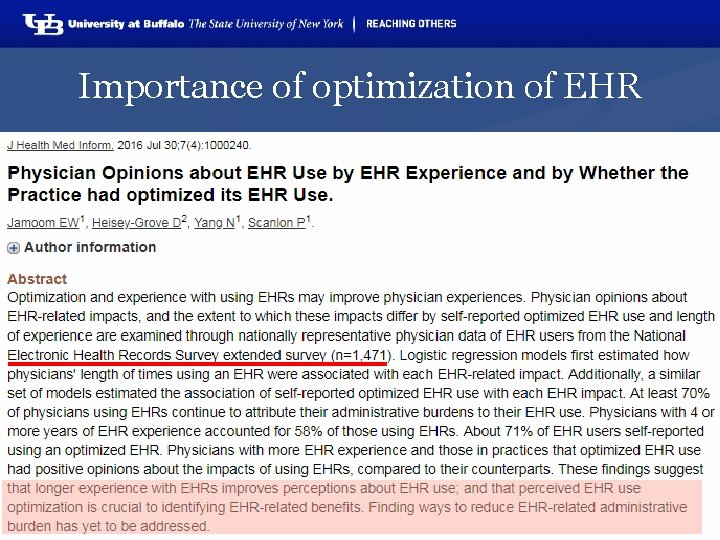
Importance of optimization of EHR
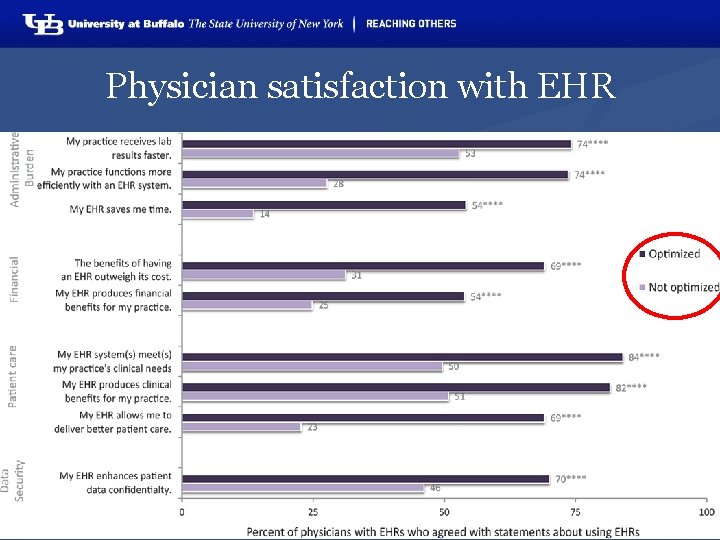
Physician satisfaction with EHR
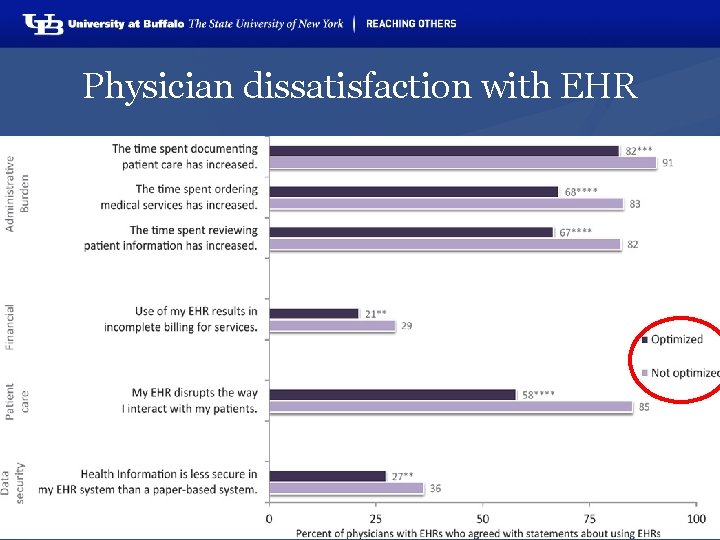
Physician dissatisfaction with EHR
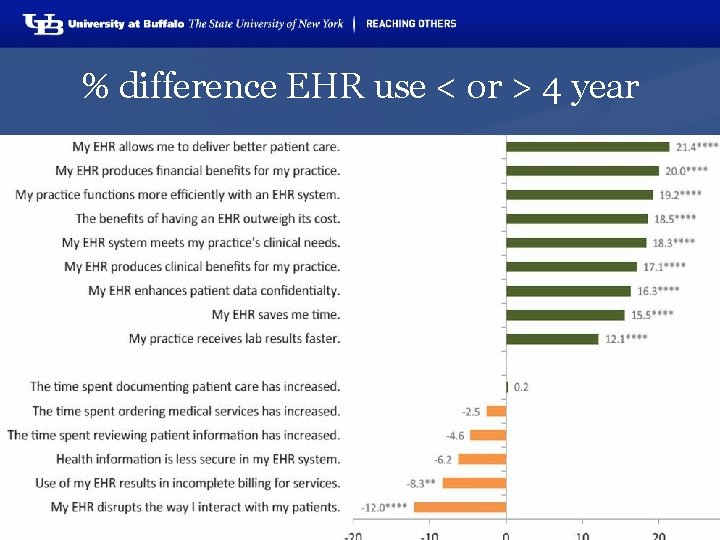
% difference EHR use < or > 4 year
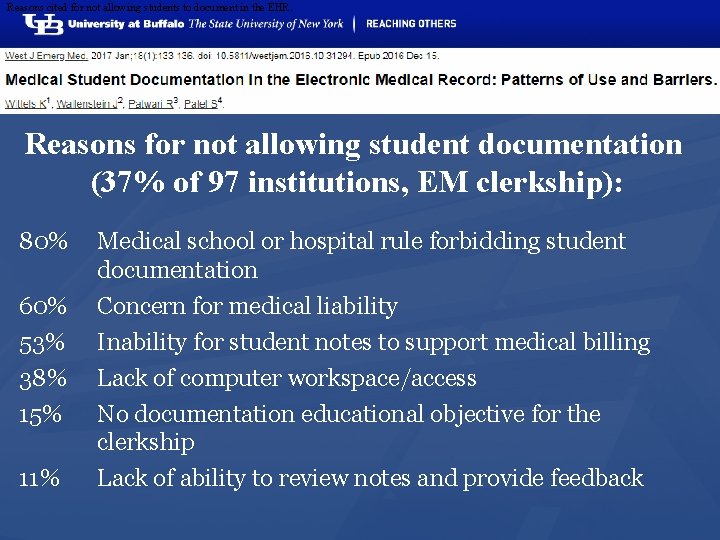
Reasons cited for not allowing students to document in the EHR. Reasons for not allowing student documentation (37% of 97 institutions, EM clerkship): 80% 60% 53% 38% 15% 11% Medical school or hospital rule forbidding student documentation Concern for medical liability Inability for student notes to support medical billing Lack of computer workspace/access No documentation educational objective for the clerkship Lack of ability to review notes and provide feedback
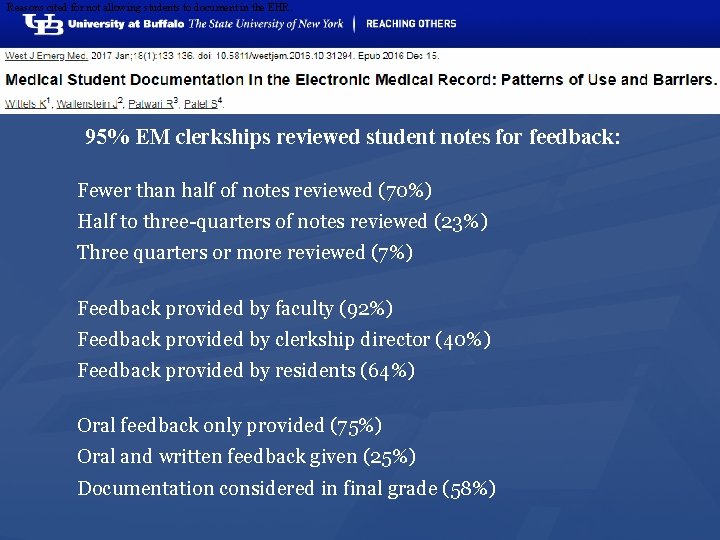
Reasons cited for not allowing students to document in the EHR. 95% EM clerkships reviewed student notes for feedback: Fewer than half of notes reviewed (70%) Half to three-quarters of notes reviewed (23%) Three quarters or more reviewed (7%) Feedback provided by faculty (92%) Feedback provided by clerkship director (40%) Feedback provided by residents (64%) Oral feedback only provided (75%) Oral and written feedback given (25%) Documentation considered in final grade (58%)
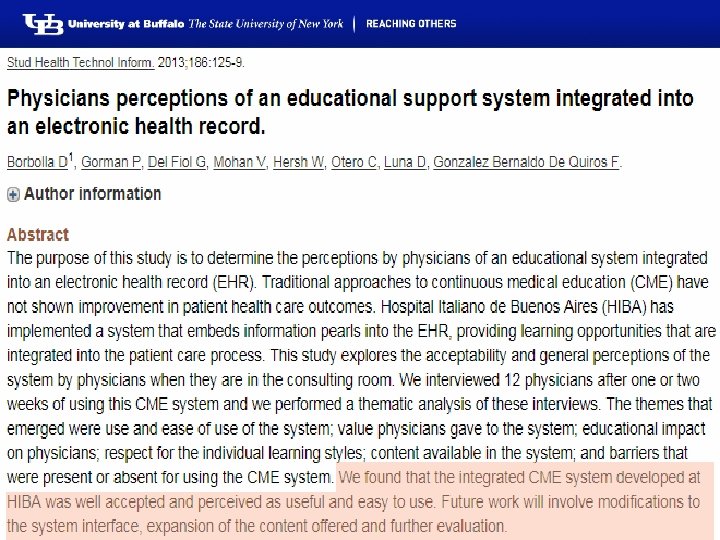
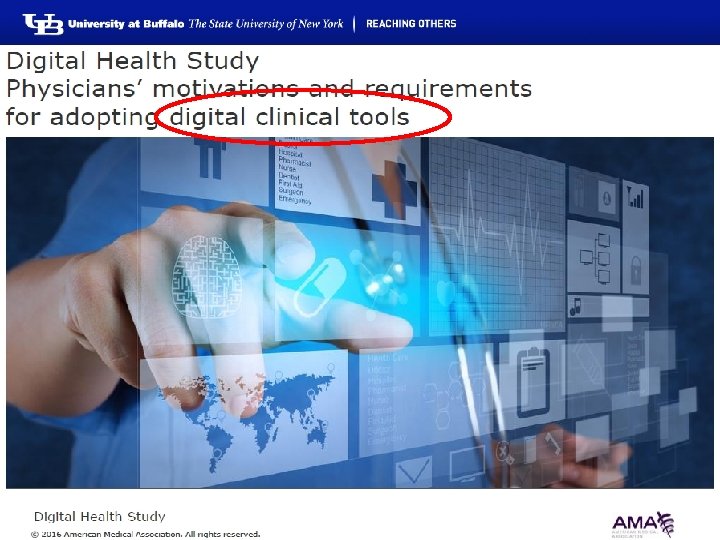
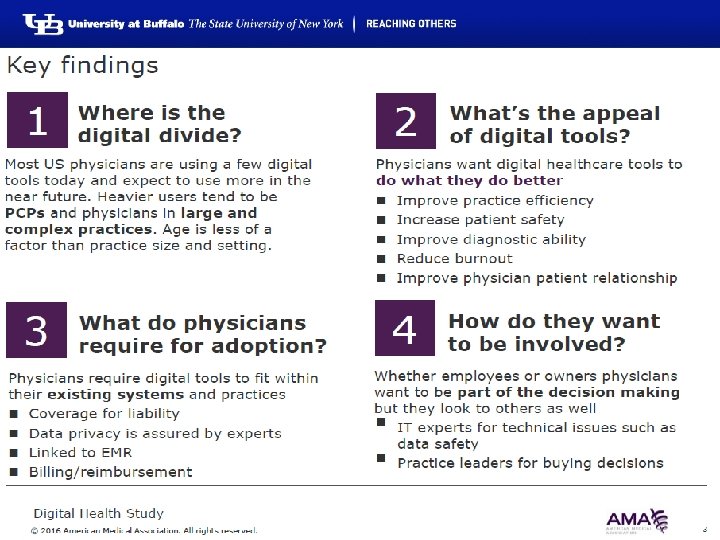
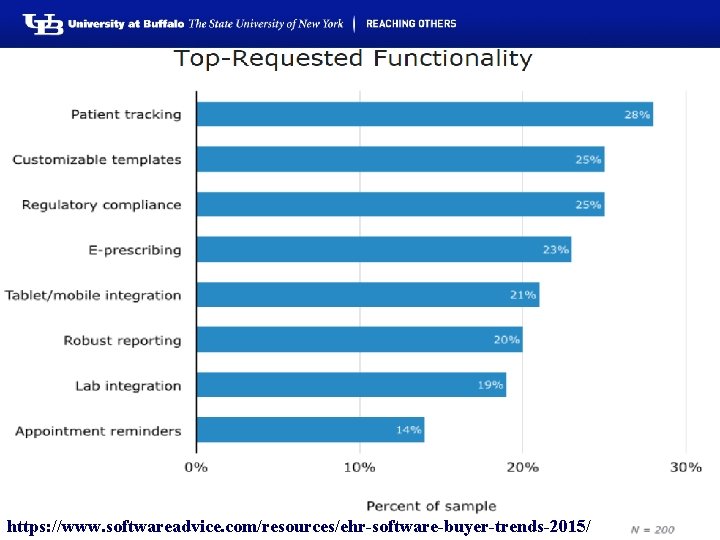
https: //www. softwareadvice. com/resources/ehr-software-buyer-trends-2015/
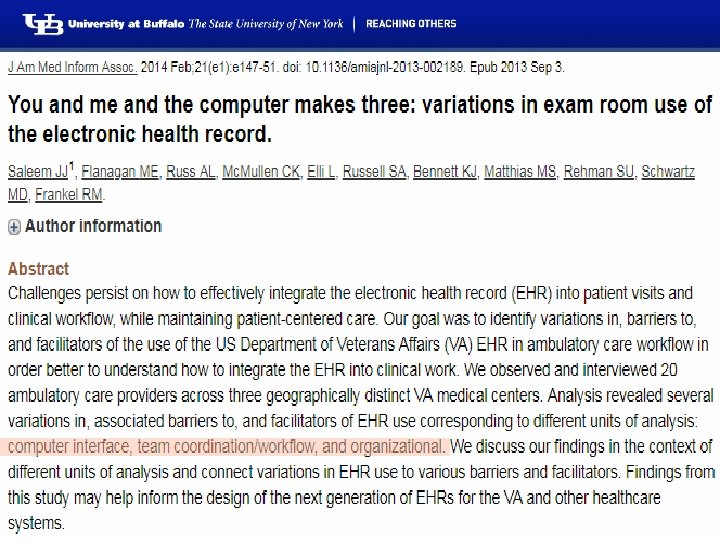
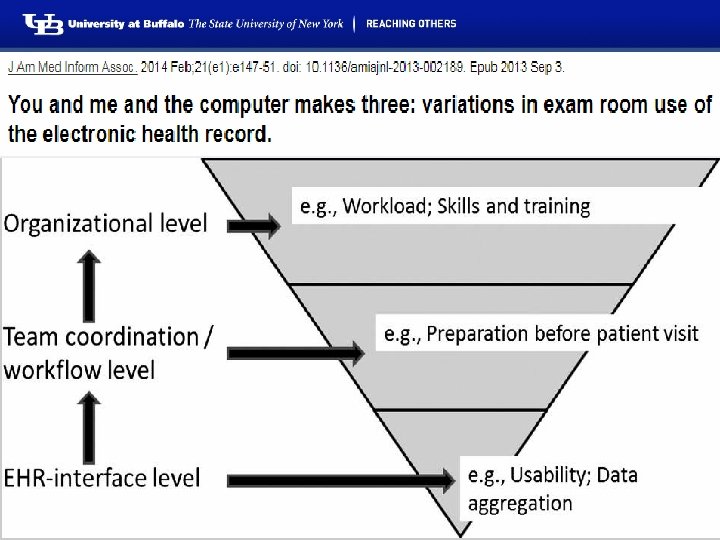
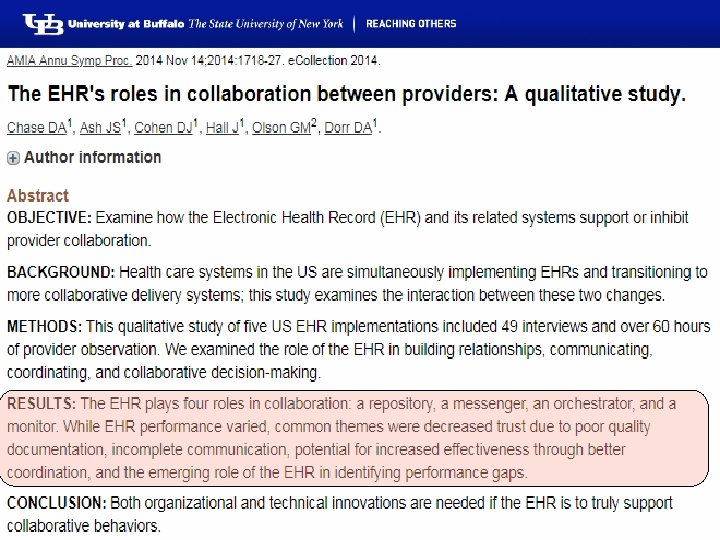
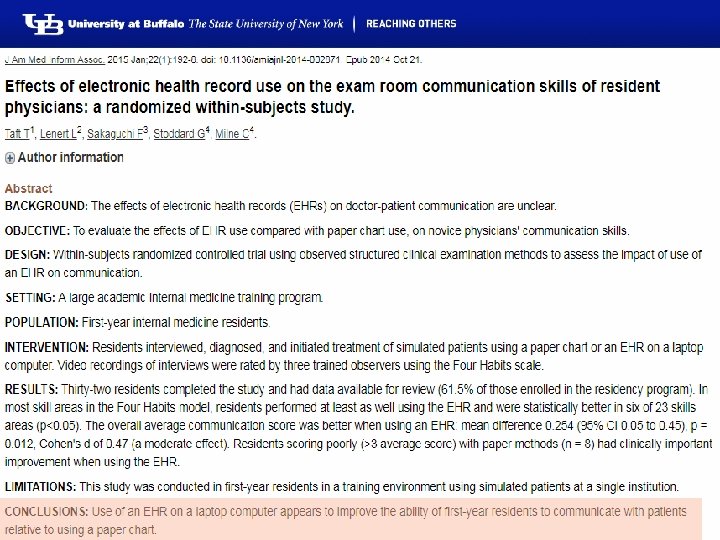
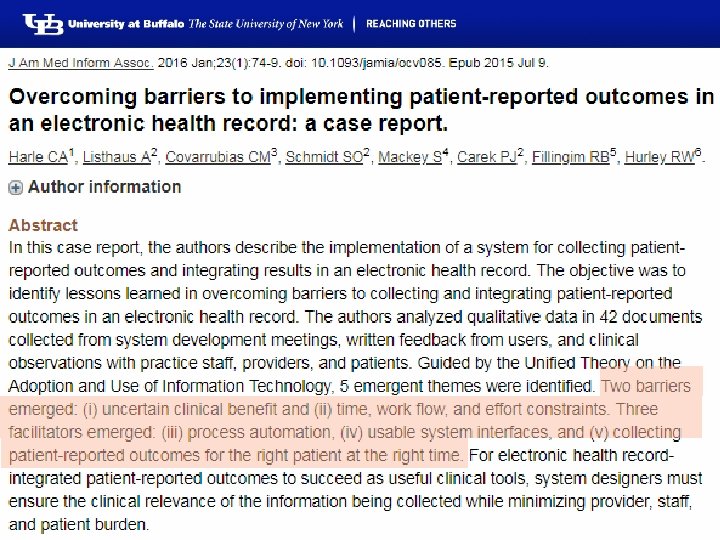
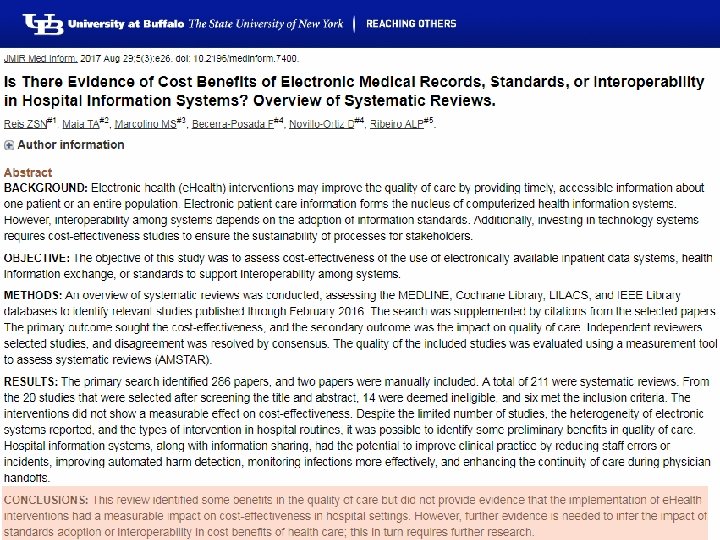
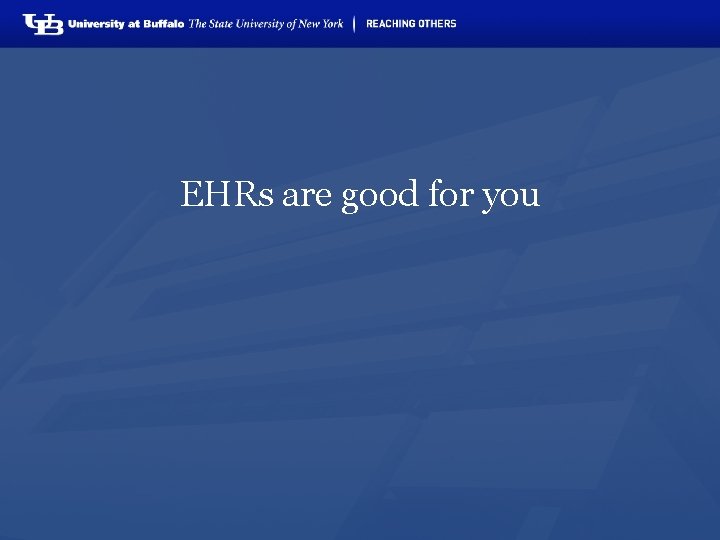
EHRs are good for you
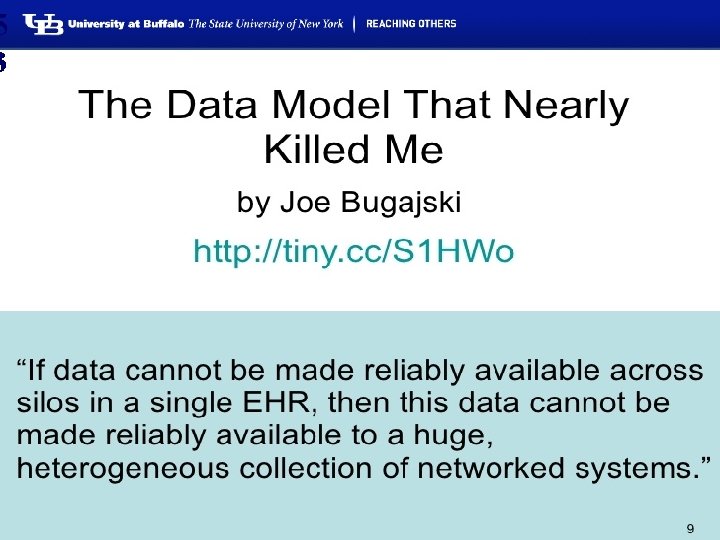
5 3
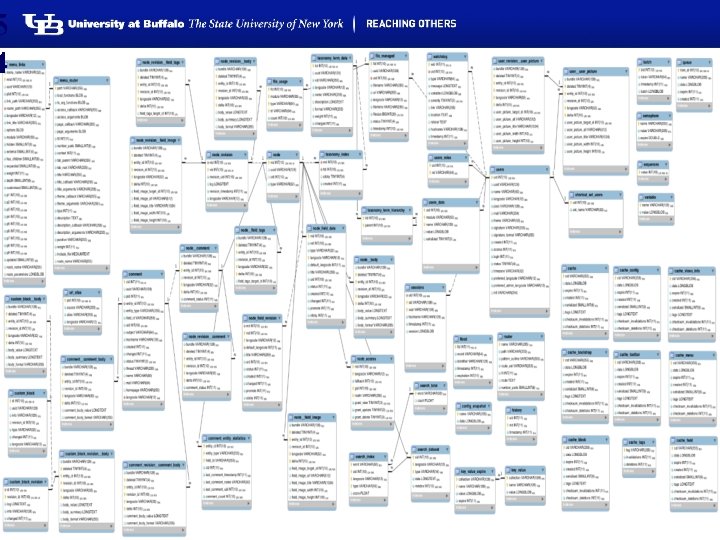
5 4
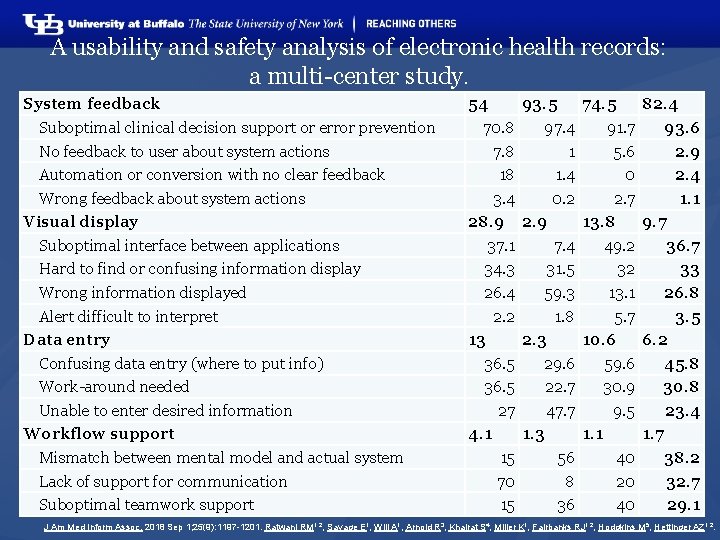
A usability and safety analysis of electronic health records: a multi-center study. System feedback Suboptimal clinical decision support or error prevention No feedback to user about system actions Automation or conversion with no clear feedback Wrong feedback about system actions Visual display Suboptimal interface between applications Hard to find or confusing information display Wrong information displayed Alert difficult to interpret Data entry Confusing data entry (where to put info) Work-around needed Unable to enter desired information Workflow support Mismatch between mental model and actual system Lack of support for communication Suboptimal teamwork support 54 70. 8 7. 8 18 3. 4 28. 9 37. 1 34. 3 26. 4 2. 2 13 36. 5 27 4. 1 15 70 15 93. 5 97. 4 1 1. 4 0. 2 2. 9 7. 4 31. 5 59. 3 1. 8 2. 3 29. 6 22. 7 47. 7 1. 3 56 8 36 74. 5 91. 7 5. 6 0 2. 7 13. 8 49. 2 32 13. 1 5. 7 10. 6 59. 6 30. 9 9. 5 1. 1 40 20 40 82. 4 93. 6 2. 9 2. 4 1. 1 9. 7 36. 7 33 26. 8 3. 5 6. 2 45. 8 30. 8 23. 4 1. 7 38. 2 32. 7 29. 1 J Am Med Inform Assoc. 2018 Sep 1; 25(9): 1197 -1201. Ratwani RM 1, 2, Savage E 1, Will A 1, Arnold R 3, Khairat S 4, Miller K 1, Fairbanks RJ 1, 2, Hodgkins M 5, Hettinger AZ 1, 2.
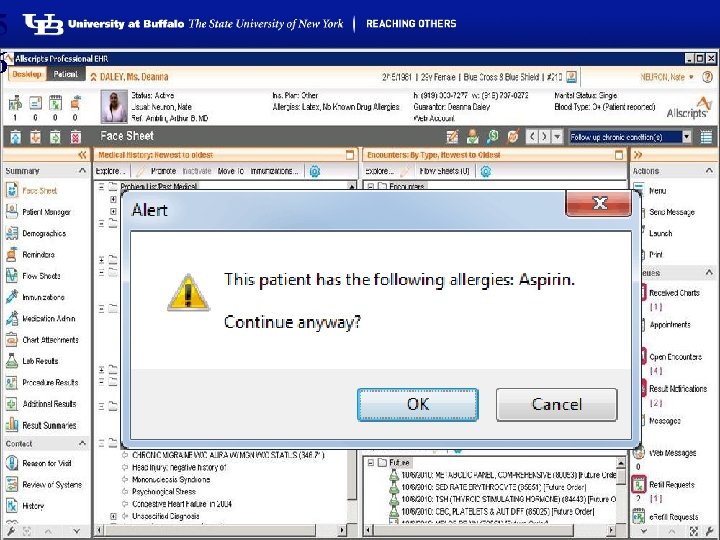
5 6
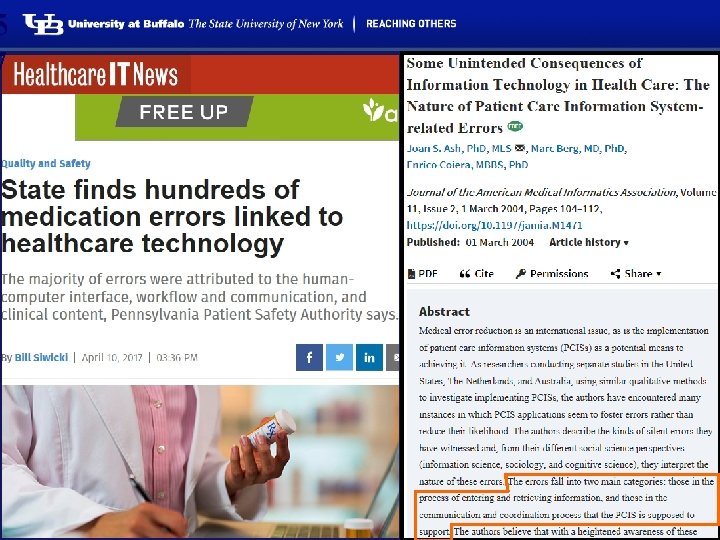
5 7
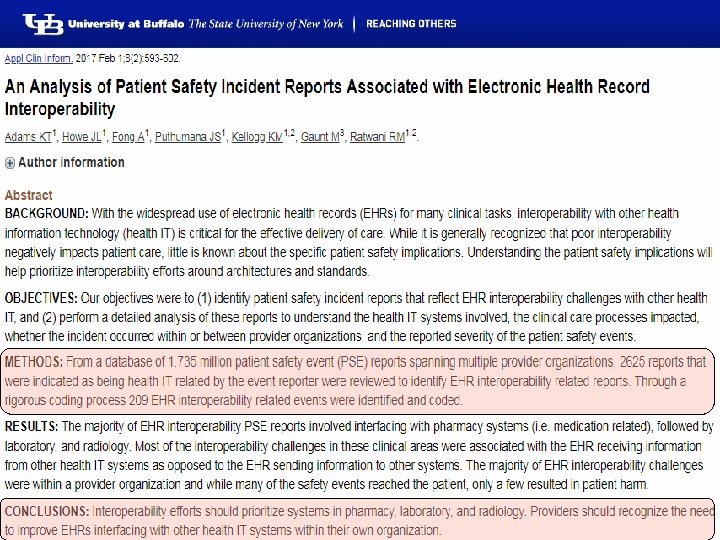
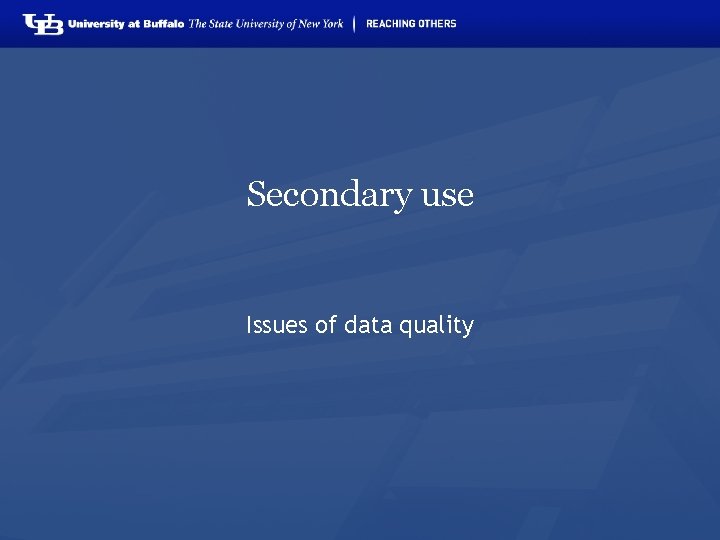
Secondary use Issues of data quality
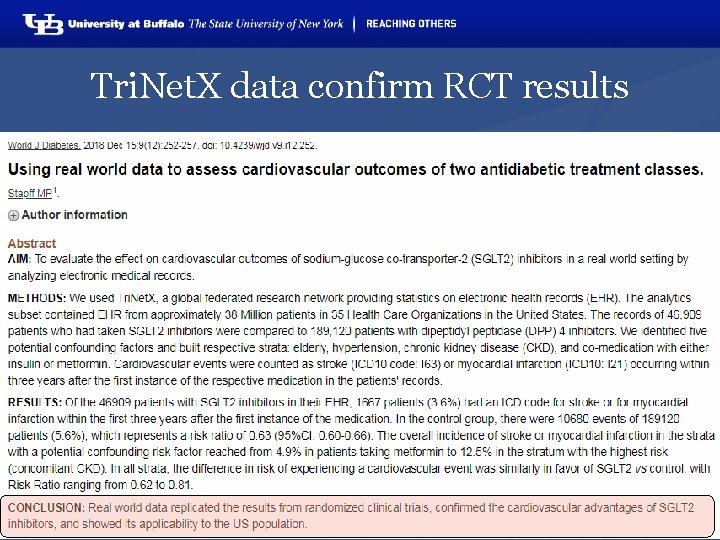
Tri. Net. X data confirm RCT results
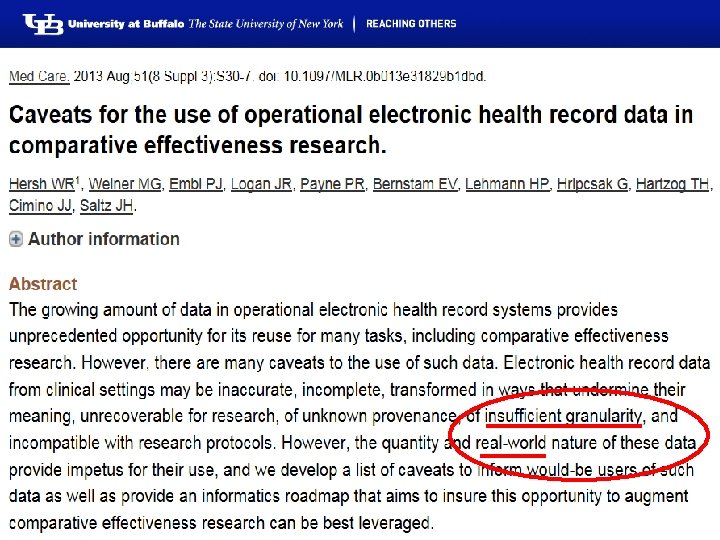
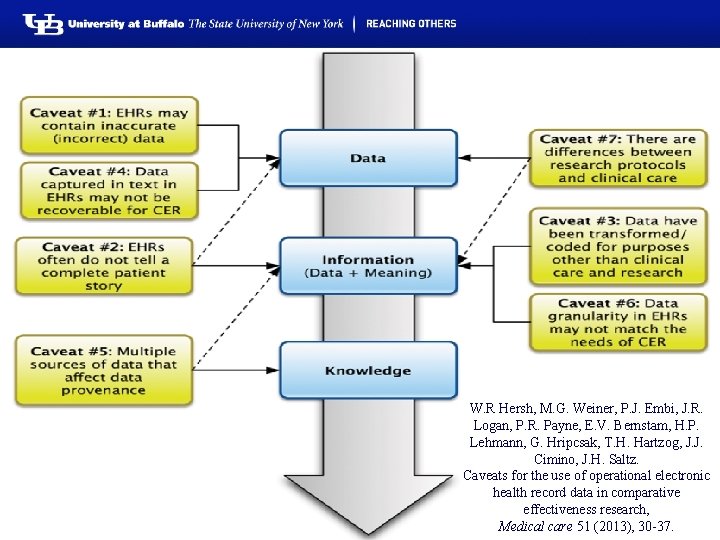
W. R Hersh, M. G. Weiner, P. J. Embi, J. R. Logan, P. R. Payne, E. V. Bernstam, H. P. Lehmann, G. Hripcsak, T. H. Hartzog, J. J. Cimino, J. H. Saltz. Caveats for the use of operational electronic health record data in comparative effectiveness research, Medical care 51 (2013), 30 -37.
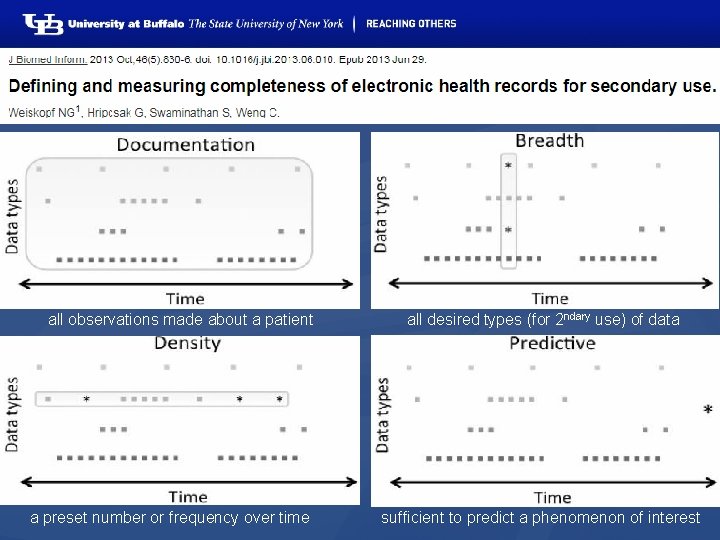
all observations made about a patient a preset number or frequency over time all desired types (for 2 ndary use) of data sufficient to predict a phenomenon of interest
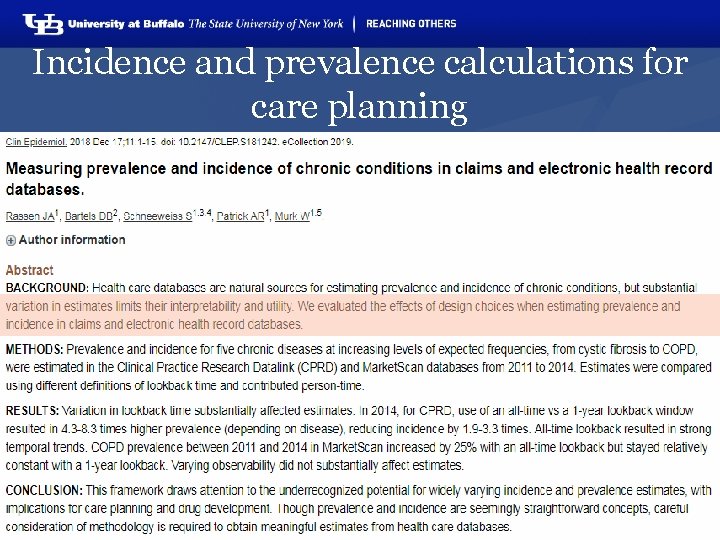
Incidence and prevalence calculations for care planning
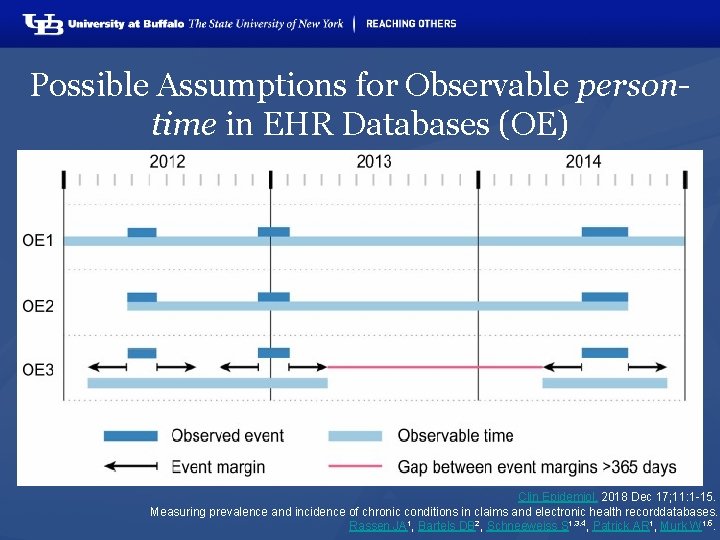
Possible Assumptions for Observable persontime in EHR Databases (OE) Clin Epidemiol. 2018 Dec 17; 11: 1 -15. Measuring prevalence and incidence of chronic conditions in claims and electronic health recorddatabases. Rassen JA 1, Bartels DB 2, Schneeweiss S 1, 3, 4, Patrick AR 1, Murk W 1, 5.
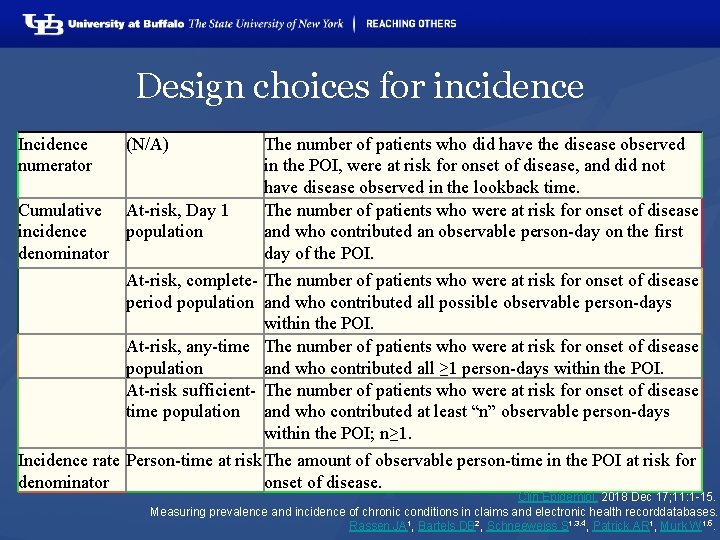
Design choices for incidence Incidence numerator (N/A) Cumulative At-risk, Day 1 incidence population denominator The number of patients who did have the disease observed in the POI, were at risk for onset of disease, and did not have disease observed in the lookback time. The number of patients who were at risk for onset of disease and who contributed an observable person-day on the first day of the POI. At-risk, complete- The number of patients who were at risk for onset of disease period population and who contributed all possible observable person-days within the POI. At-risk, any-time The number of patients who were at risk for onset of disease population and who contributed all ≥ 1 person-days within the POI. At-risk sufficient- The number of patients who were at risk for onset of disease time population and who contributed at least “n” observable person-days within the POI; n≥ 1. Incidence rate Person-time at risk The amount of observable person-time in the POI at risk for denominator onset of disease. Clin Epidemiol. 2018 Dec 17; 11: 1 -15. Measuring prevalence and incidence of chronic conditions in claims and electronic health recorddatabases. Rassen JA 1, Bartels DB 2, Schneeweiss S 1, 3, 4, Patrick AR 1, Murk W 1, 5.
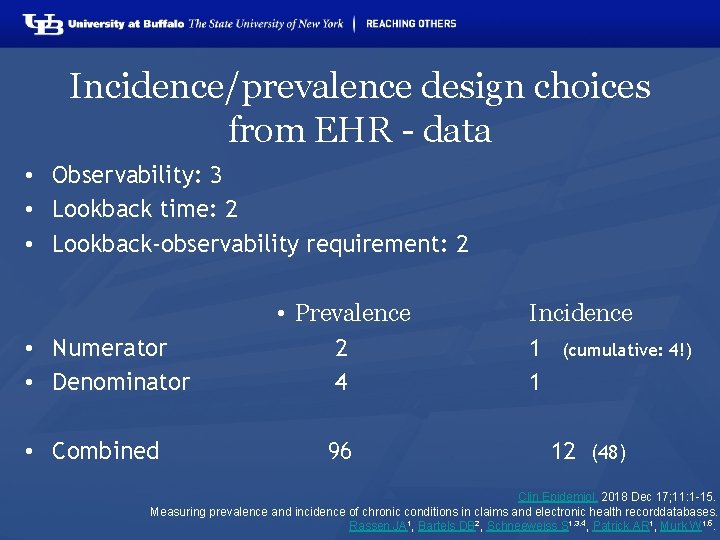
Incidence/prevalence design choices from EHR - data • Observability: 3 • Lookback time: 2 • Lookback-observability requirement: 2 • Numerator • Denominator • Combined • Prevalence 2 4 96 Incidence 1 (cumulative: 4!) 1 12 (48) Clin Epidemiol. 2018 Dec 17; 11: 1 -15. Measuring prevalence and incidence of chronic conditions in claims and electronic health recorddatabases. Rassen JA 1, Bartels DB 2, Schneeweiss S 1, 3, 4, Patrick AR 1, Murk W 1, 5.
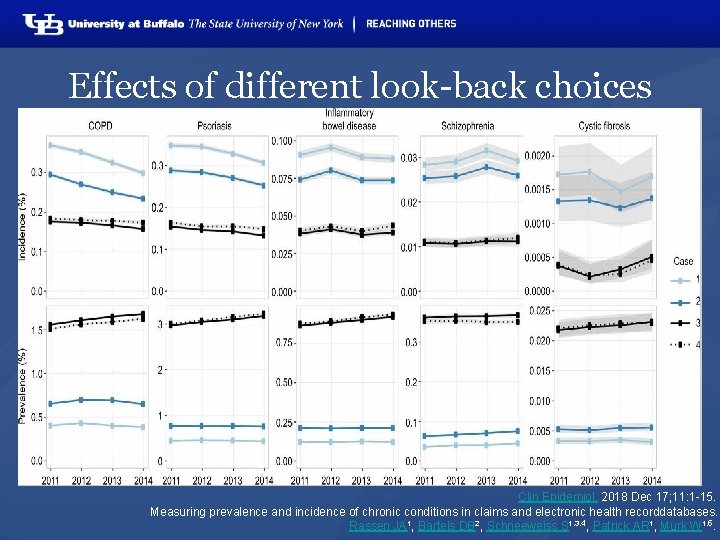
Effects of different look-back choices Clin Epidemiol. 2018 Dec 17; 11: 1 -15. Measuring prevalence and incidence of chronic conditions in claims and electronic health recorddatabases. Rassen JA 1, Bartels DB 2, Schneeweiss S 1, 3, 4, Patrick AR 1, Murk W 1, 5.
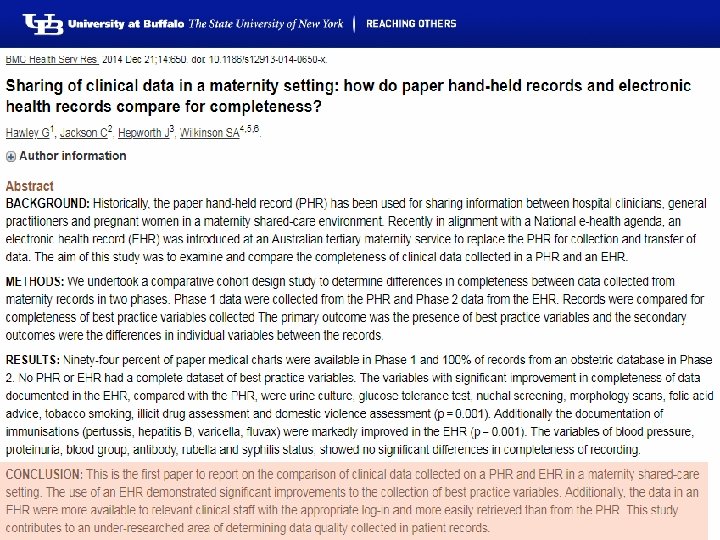
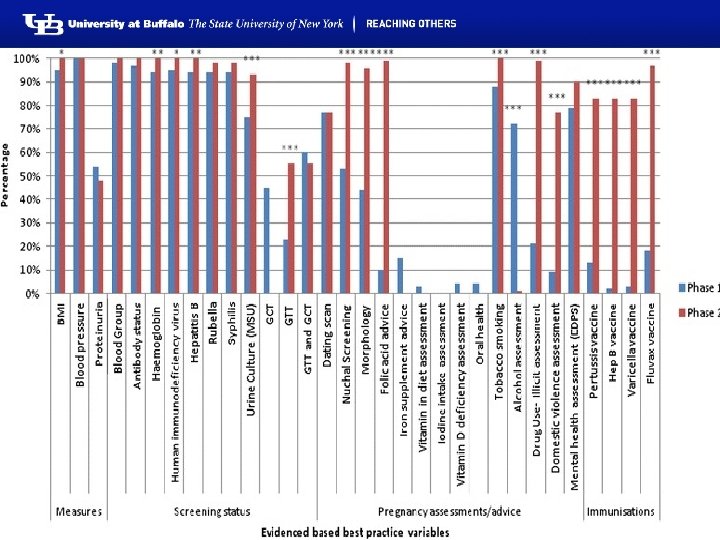
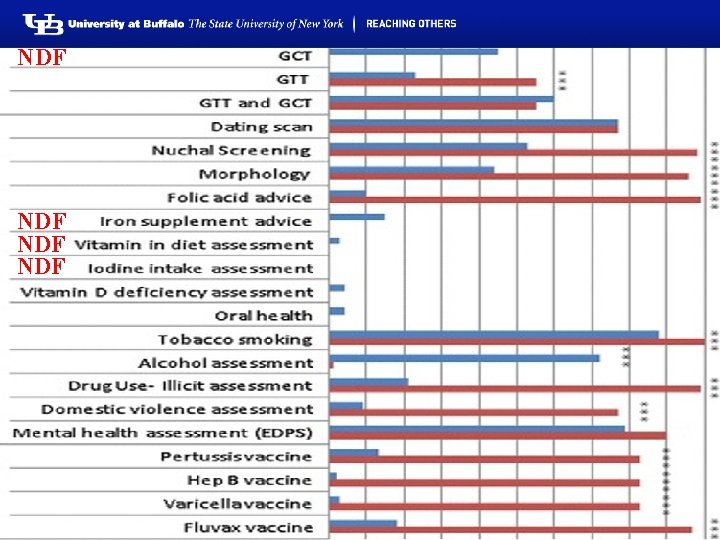
NDF NDF
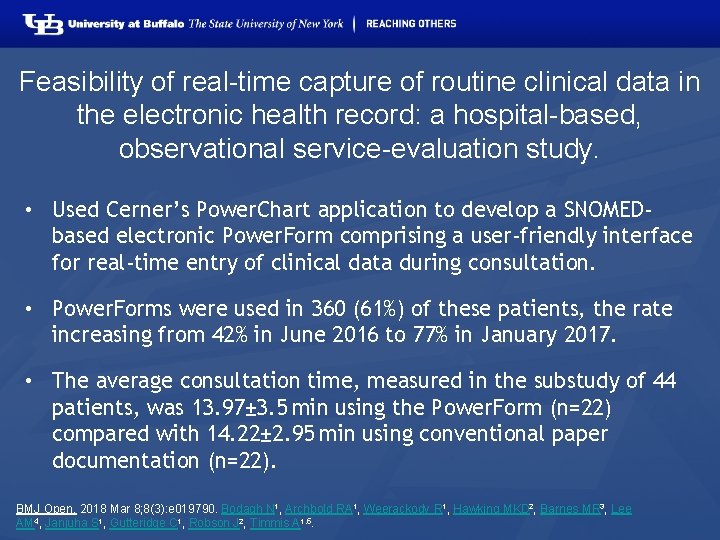
Feasibility of real-time capture of routine clinical data in the electronic health record: a hospital-based, observational service-evaluation study. • Used Cerner’s Power. Chart application to develop a SNOMEDbased electronic Power. Form comprising a user-friendly interface for real-time entry of clinical data during consultation. • Power. Forms were used in 360 (61%) of these patients, the rate increasing from 42% in June 2016 to 77% in January 2017. • The average consultation time, measured in the substudy of 44 patients, was 13. 97± 3. 5 min using the Power. Form (n=22) compared with 14. 22± 2. 95 min using conventional paper documentation (n=22). BMJ Open. 2018 Mar 8; 8(3): e 019790. Bodagh N 1, Archbold RA 1, Weerackody R 1, Hawking MKD 2, Barnes MR 3, Lee AM 4, Janjuha S 1, Gutteridge C 1, Robson J 2, Timmis A 1, 5.
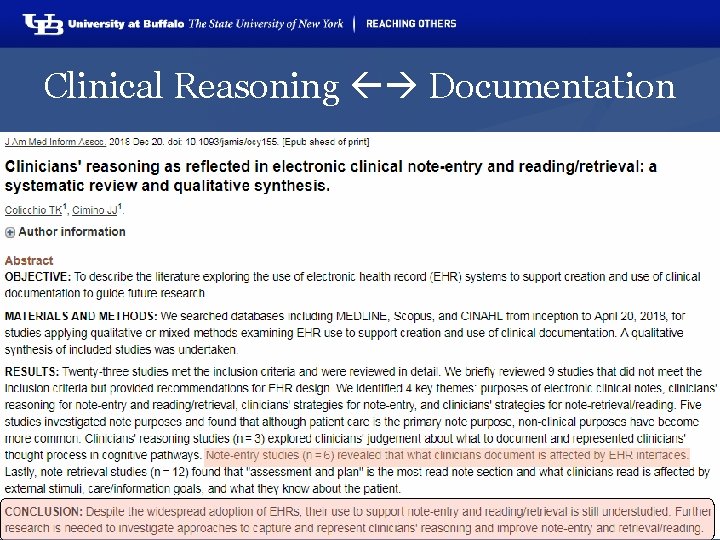
Clinical Reasoning Documentation
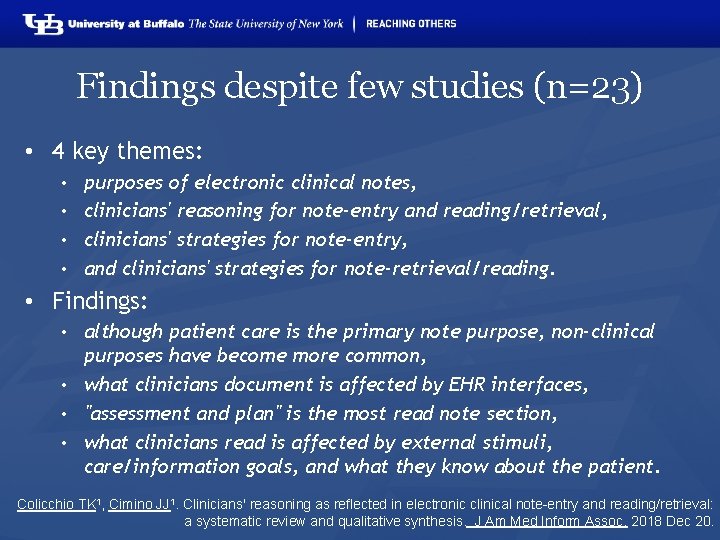
Findings despite few studies (n=23) • 4 key themes: purposes of electronic clinical notes, • clinicians' reasoning for note-entry and reading/retrieval, • clinicians' strategies for note-entry, • and clinicians' strategies for note-retrieval/reading. • • Findings: although patient care is the primary note purpose, non-clinical purposes have become more common, • what clinicians document is affected by EHR interfaces, • "assessment and plan" is the most read note section, • what clinicians read is affected by external stimuli, care/information goals, and what they know about the patient. • Colicchio TK 1, Cimino JJ 1. Clinicians' reasoning as reflected in electronic clinical note-entry and reading/retrieval: a systematic review and qualitative synthesis. J Am Med Inform Assoc. 2018 Dec 20.
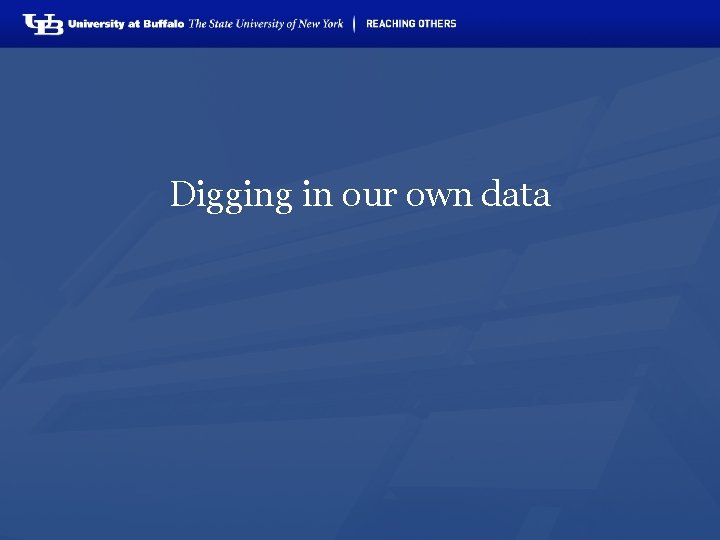
Digging in our own data
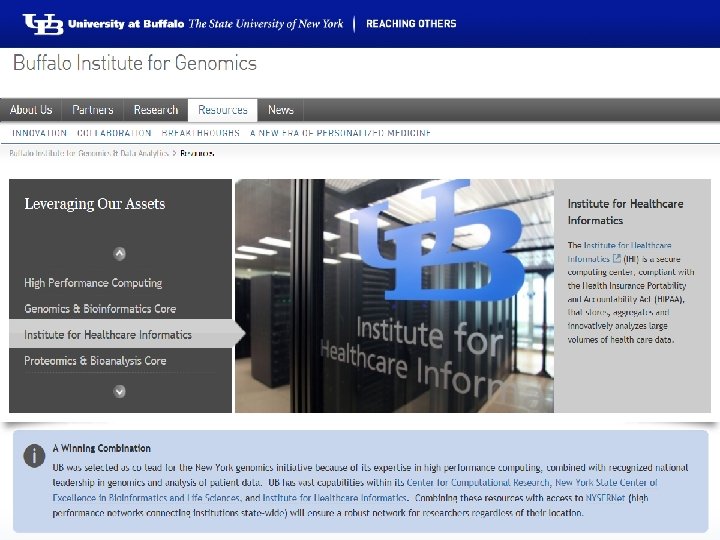
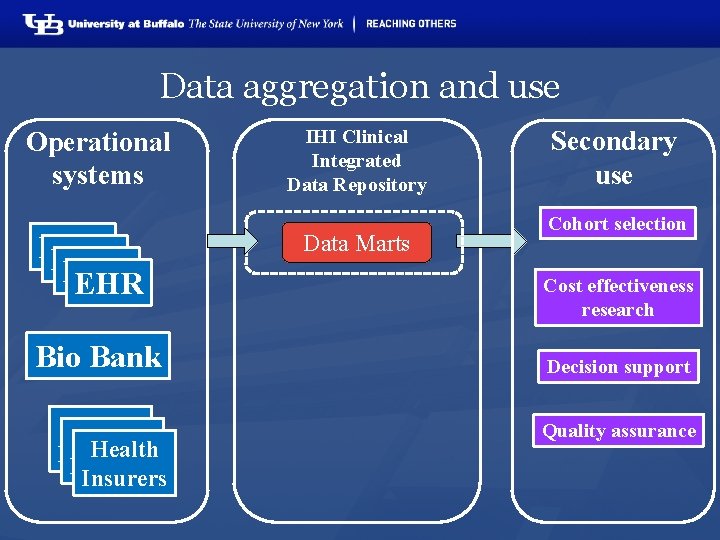
Data aggregation and use Operational systems EHR EHR Bio Bank Health Insurers IHI Clinical Integrated Data Repository Data Marts Secondary use Cohort selection Cost effectiveness research Decision support Quality assurance
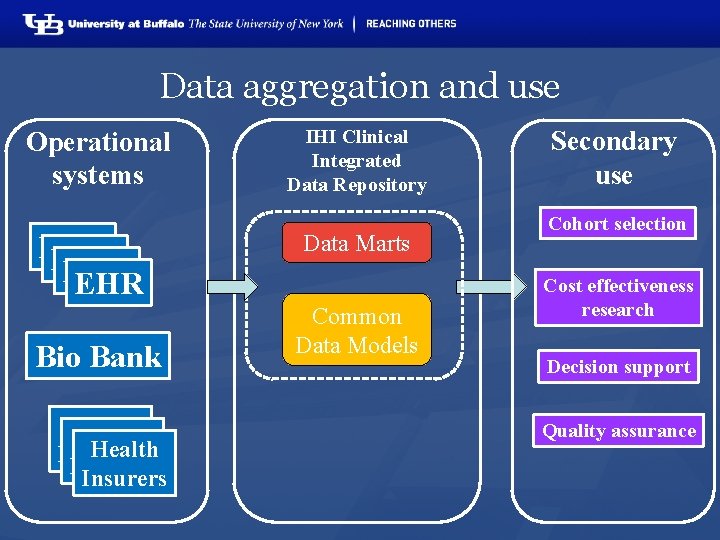
Data aggregation and use Operational systems EHR EHR Bio Bank Health Insurers IHI Clinical Integrated Data Repository Data Marts Common Data Models Secondary use Cohort selection Cost effectiveness research Decision support Quality assurance
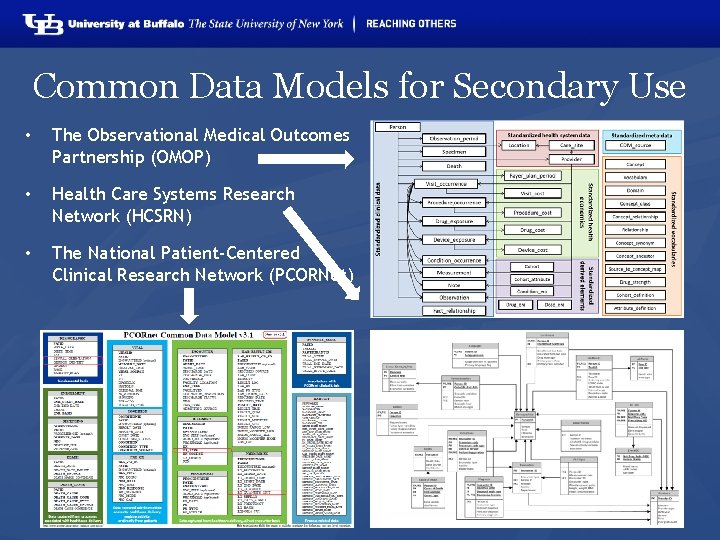
Common Data Models for Secondary Use • The Observational Medical Outcomes Partnership (OMOP) • Health Care Systems Research Network (HCSRN) • The National Patient-Centered Clinical Research Network (PCORNet)
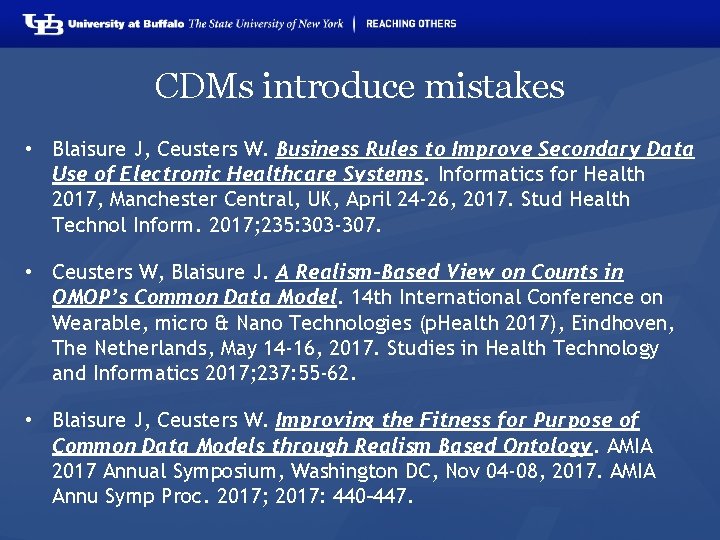
CDMs introduce mistakes • Blaisure J, Ceusters W. Business Rules to Improve Secondary Data Use of Electronic Healthcare Systems. Informatics for Health 2017, Manchester Central, UK, April 24 -26, 2017. Stud Health Technol Inform. 2017; 235: 303 -307. • Ceusters W, Blaisure J. A Realism-Based View on Counts in OMOP’s Common Data Model. 14 th International Conference on Wearable, micro & Nano Technologies (p. Health 2017), Eindhoven, The Netherlands, May 14 -16, 2017. Studies in Health Technology and Informatics 2017; 237: 55 -62. • Blaisure J, Ceusters W. Improving the Fitness for Purpose of Common Data Models through Realism Based Ontology. AMIA 2017 Annual Symposium, Washington DC, Nov 04 -08, 2017. AMIA Annu Symp Proc. 2017; 2017: 440– 447.
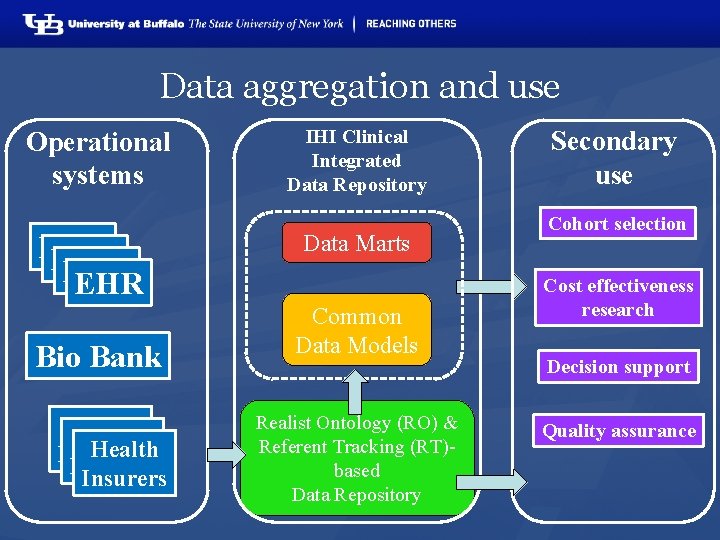
Data aggregation and use Operational systems EHR EHR Bio Bank Health Insurers IHI Clinical Integrated Data Repository Data Marts Common Data Models Realist Ontology (RO) & Referent Tracking (RT)based Data Repository Secondary use Cohort selection Cost effectiveness research Decision support Quality assurance
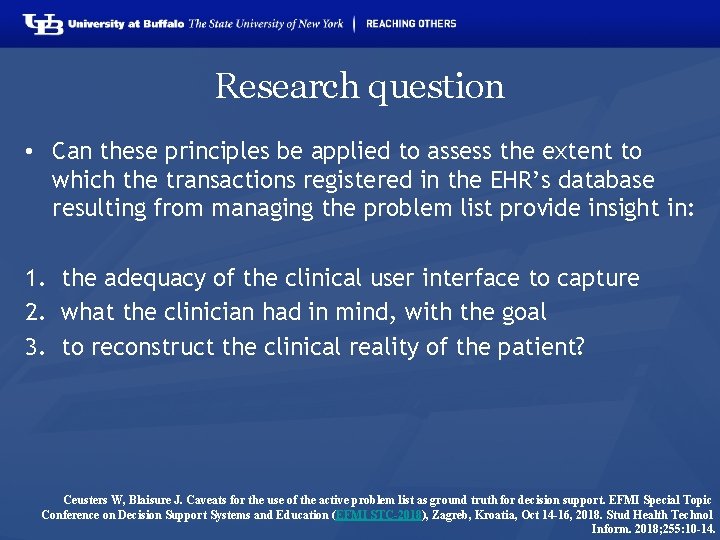
Research question • Can these principles be applied to assess the extent to which the transactions registered in the EHR’s database resulting from managing the problem list provide insight in: 1. the adequacy of the clinical user interface to capture 2. what the clinician had in mind, with the goal 3. to reconstruct the clinical reality of the patient? Ceusters W, Blaisure J. Caveats for the use of the active problem list as ground truth for decision support. EFMI Special Topic Conference on Decision Support Systems and Education (EFMI STC-2018), Zagreb, Kroatia, Oct 14 -16, 2018. Stud Health Technol Inform. 2018; 255: 10 -14.
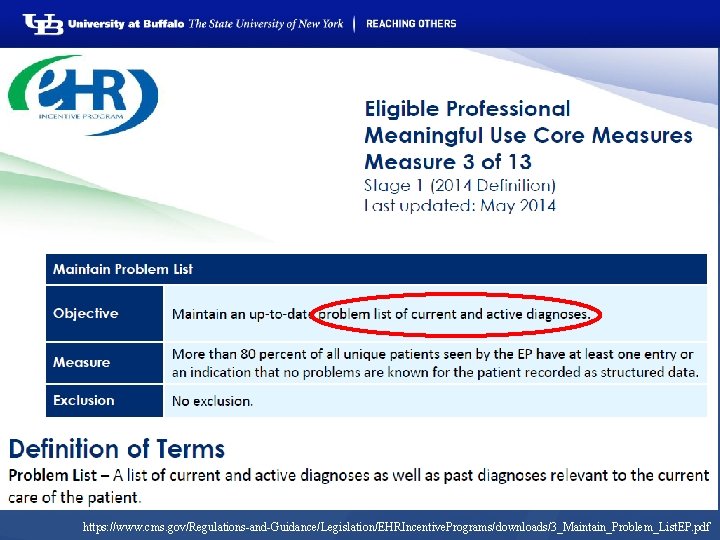
https: //www. cms. gov/Regulations-and-Guidance/Legislation/EHRIncentive. Programs/downloads/3_Maintain_Problem_List. EP. pdf
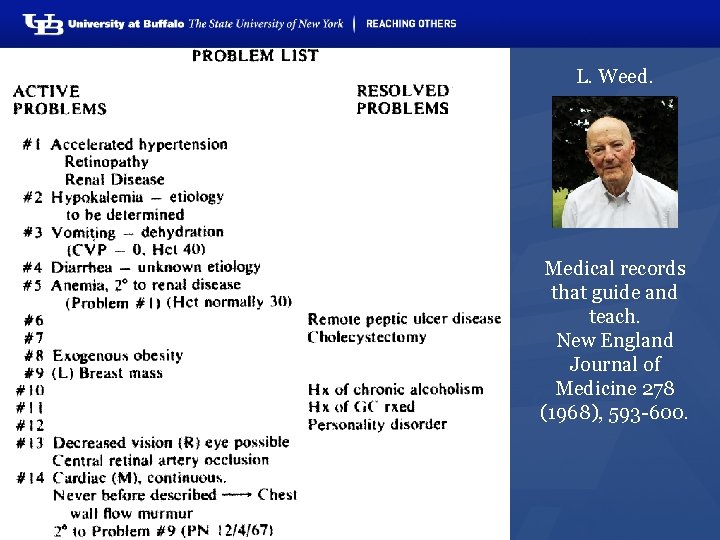
L. Weed. Medical records that guide and teach. New England Journal of Medicine 278 (1968), 593 -600.
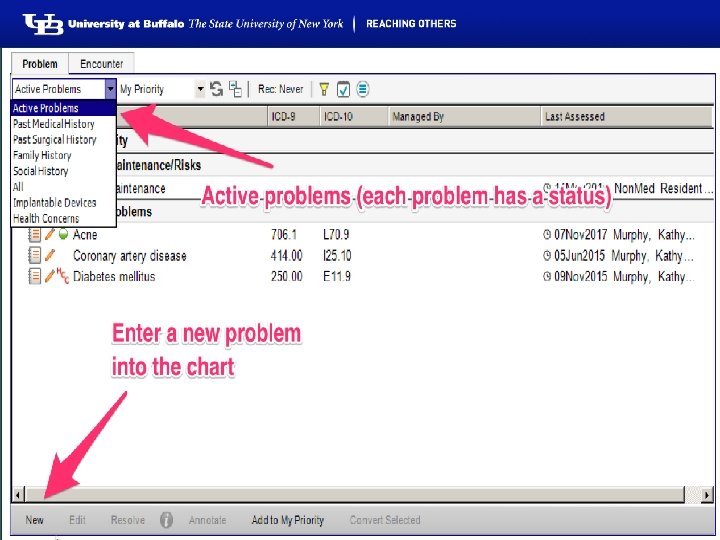
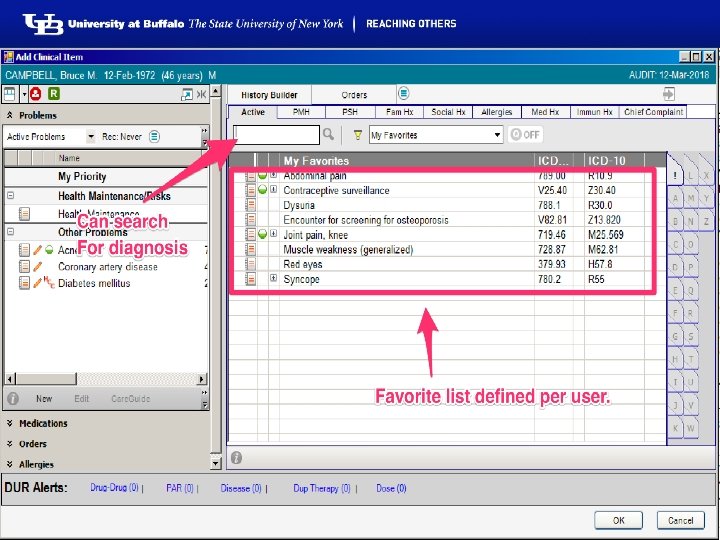
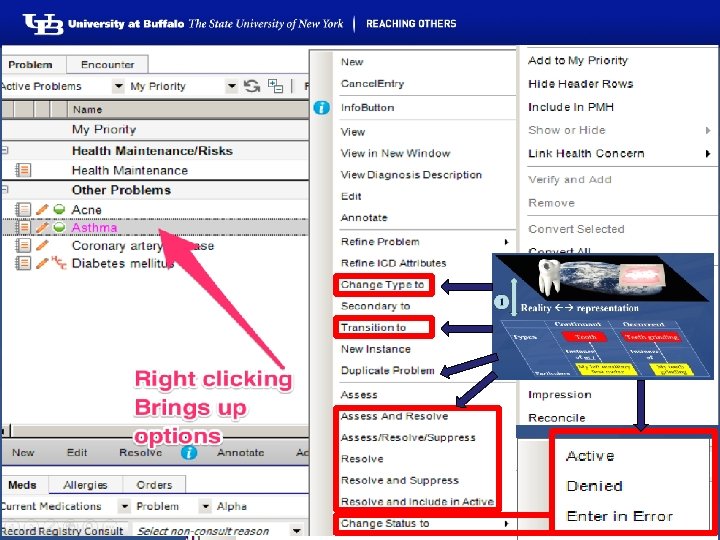
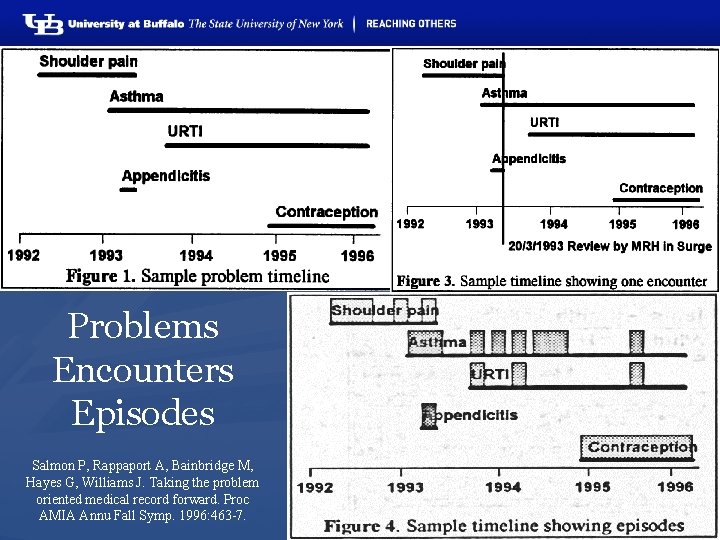
Problems Encounters Episodes Salmon P, Rappaport A, Bainbridge M, Hayes G, Williams J. Taking the problem oriented medical record forward. Proc AMIA Annu Fall Symp. 1996: 463 -7.
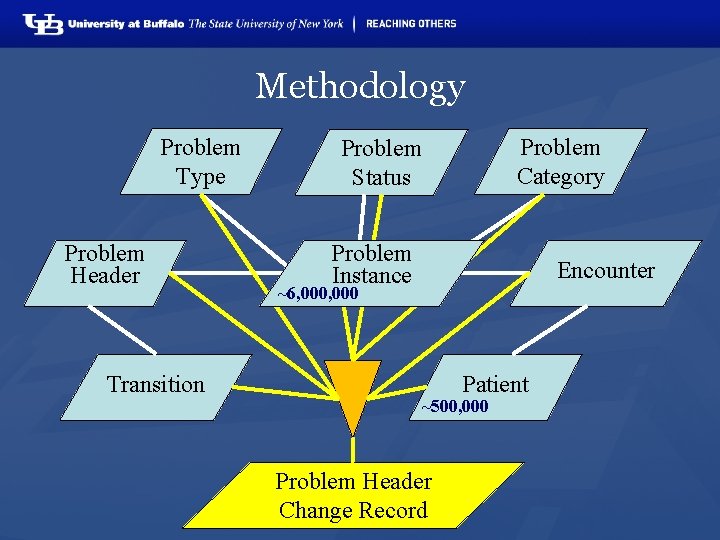
Methodology Problem Type Problem Header Transition Problem Category Problem Status Problem Instance Encounter ~6, 000, 000 Patient ~500, 000 Problem Header Change Record
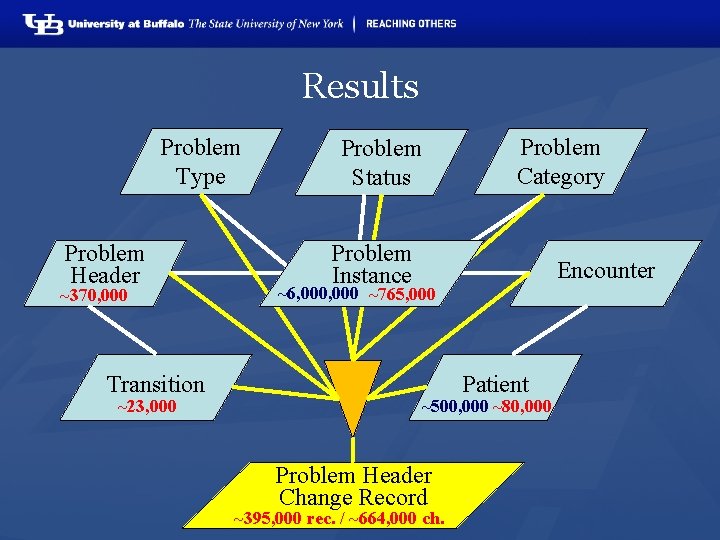
Results Problem Type Problem Header ~370, 000 Transition ~23, 000 Problem Status Problem Category Problem Instance Encounter ~6, 000 ~765, 000 Patient ~500, 000 ~80, 000 Problem Header Change Record ~395, 000 rec. / ~664, 000 ch.
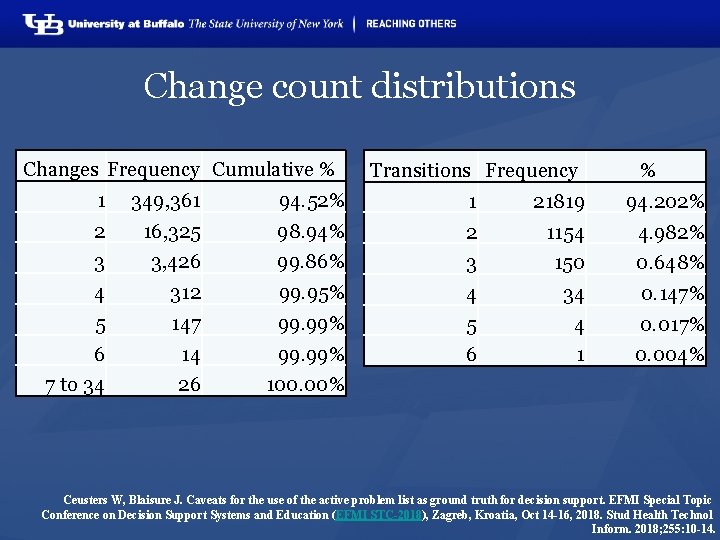
Change count distributions Changes Frequency Cumulative % Transitions Frequency % 1 349, 361 94. 52% 1 21819 94. 202% 2 16, 325 98. 94% 2 1154 4. 982% 3 3, 426 99. 86% 3 150 0. 648% 4 312 99. 95% 4 34 0. 147% 5 147 99. 99% 5 4 0. 017% 6 14 99. 99% 6 1 0. 004% 7 to 34 26 100. 00% Ceusters W, Blaisure J. Caveats for the use of the active problem list as ground truth for decision support. EFMI Special Topic Conference on Decision Support Systems and Education (EFMI STC-2018), Zagreb, Kroatia, Oct 14 -16, 2018. Stud Health Technol Inform. 2018; 255: 10 -14.
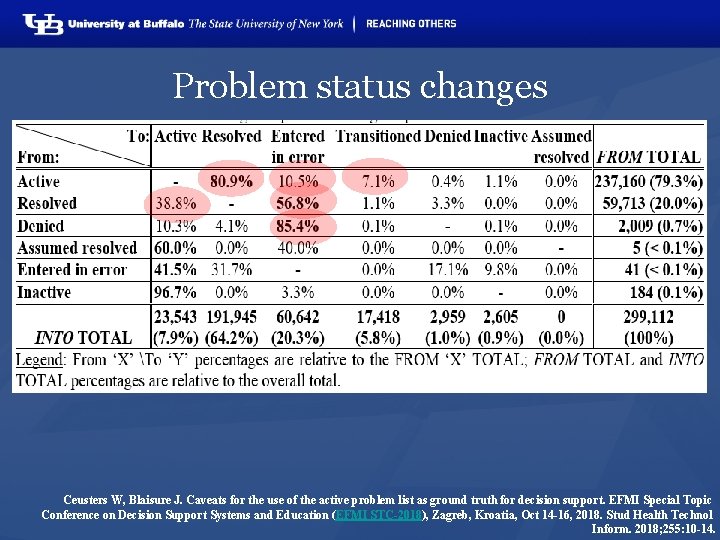
Problem status changes Ceusters W, Blaisure J. Caveats for the use of the active problem list as ground truth for decision support. EFMI Special Topic Conference on Decision Support Systems and Education (EFMI STC-2018), Zagreb, Kroatia, Oct 14 -16, 2018. Stud Health Technol Inform. 2018; 255: 10 -14.
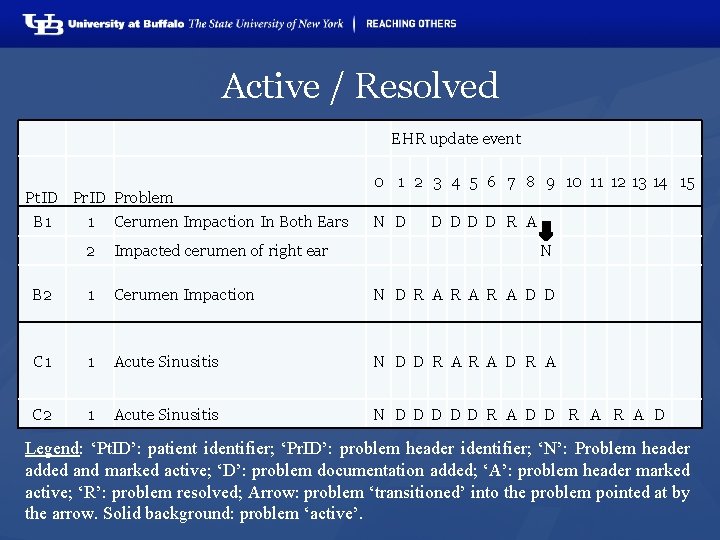
Active / Resolved EHR update event Pt. ID Problem B 1 0 1 2 3 4 5 6 7 8 9 10 11 12 13 14 15 1 Cerumen Impaction In Both Ears N D D DDD R A 2 Impacted cerumen of right ear B 2 1 Cerumen Impaction N D R A R A D D C 1 1 Acute Sinusitis N DD R A C 2 1 Acute Sinusitis N DD D DDR A D D R A D N Legend: ‘Pt. ID’: patient identifier; ‘Pr. ID’: problem header identifier; ‘N’: Problem header added and marked active; ‘D’: problem documentation added; ‘A’: problem header marked active; ‘R’: problem resolved; Arrow: problem ‘transitioned’ into the problem pointed at by the arrow. Solid background: problem ‘active’.
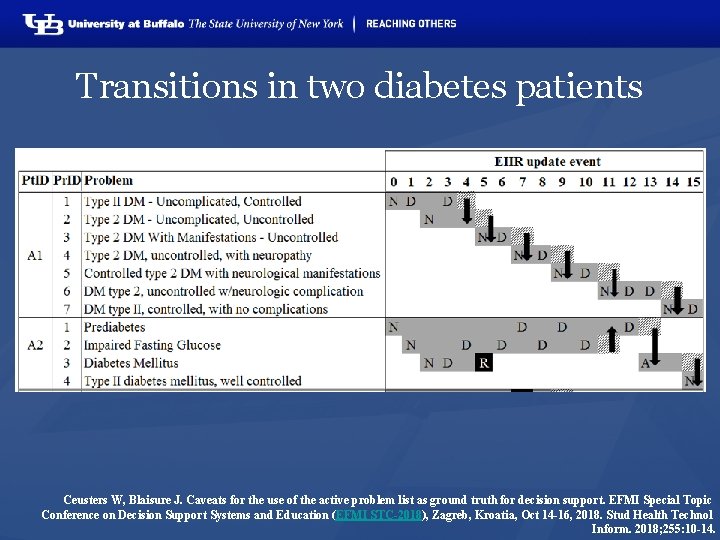
Transitions in two diabetes patients Ceusters W, Blaisure J. Caveats for the use of the active problem list as ground truth for decision support. EFMI Special Topic Conference on Decision Support Systems and Education (EFMI STC-2018), Zagreb, Kroatia, Oct 14 -16, 2018. Stud Health Technol Inform. 2018; 255: 10 -14.
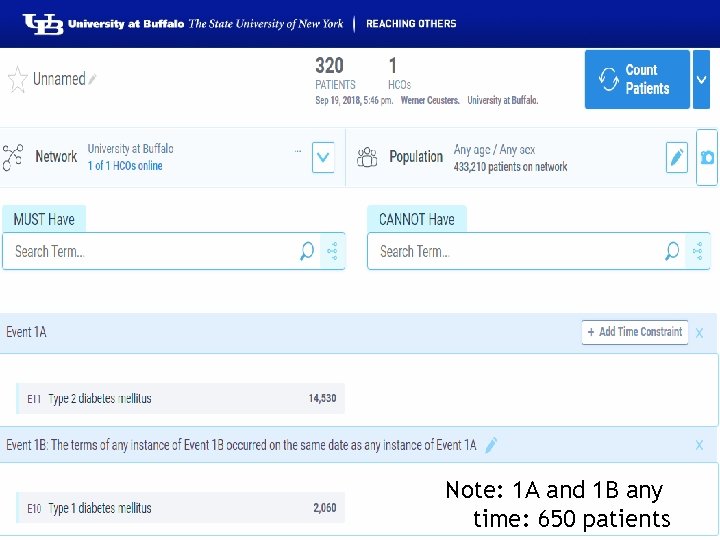
Note: 1 A and 1 B any time: 650 patients
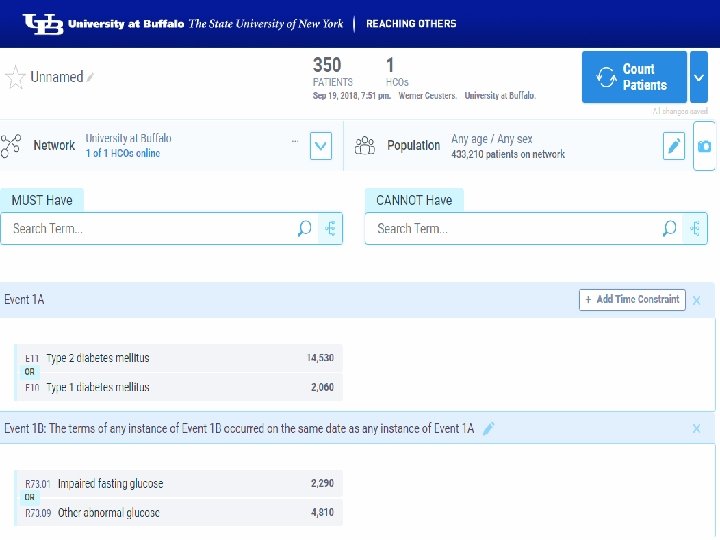
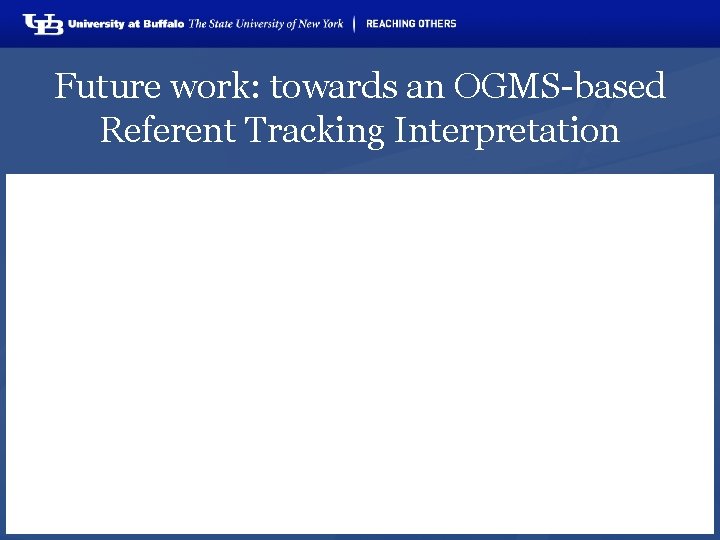
Future work: towards an OGMS-based Referent Tracking Interpretation
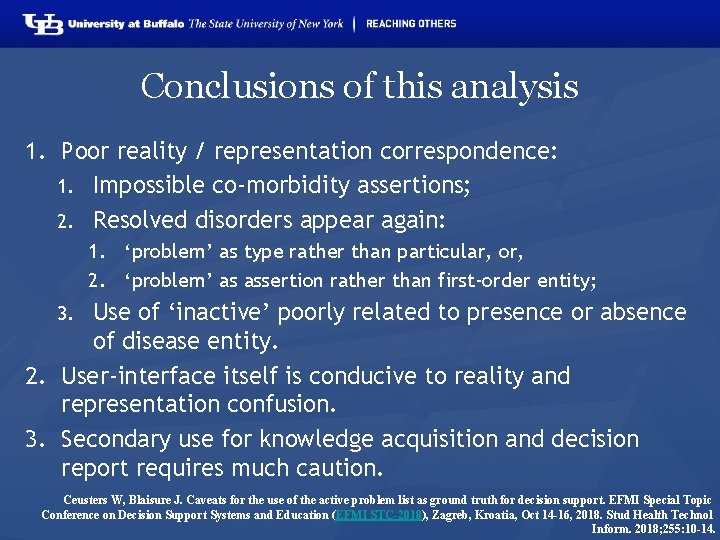
Conclusions of this analysis 1. Poor reality / representation correspondence: 1. Impossible co-morbidity assertions; 2. Resolved disorders appear again: 1. ‘problem’ as type rather than particular, or, 2. ‘problem’ as assertion rather than first-order entity; Use of ‘inactive’ poorly related to presence or absence of disease entity. 2. User-interface itself is conducive to reality and representation confusion. 3. Secondary use for knowledge acquisition and decision report requires much caution. 3. Ceusters W, Blaisure J. Caveats for the use of the active problem list as ground truth for decision support. EFMI Special Topic Conference on Decision Support Systems and Education (EFMI STC-2018), Zagreb, Kroatia, Oct 14 -16, 2018. Stud Health Technol Inform. 2018; 255: 10 -14.
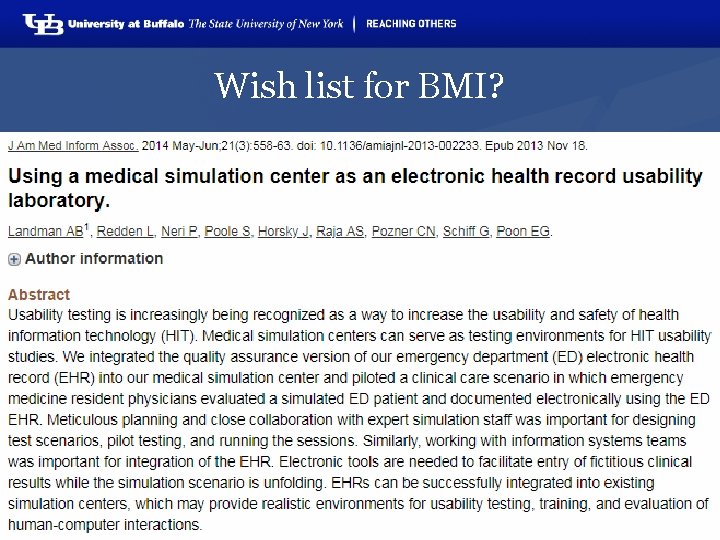
Wish list for BMI?
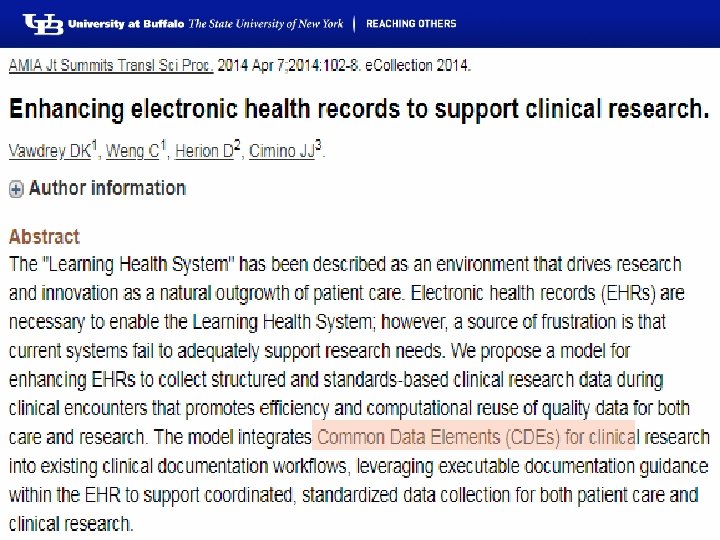
Simple shared healthcare
Healthcare and the healthcare team chapter 2
Sports medicine definition
Specimen record observation
Next generation emr
Integrated electronic medical record
How to convert paper br to electronic batch record
Electronic health record modernization services
Faithful translation example
Hymn 100 sda
Great is thy faithfulness 中文
Besides faithfulness to god what is the strongest value
Thomas obadiah chisholm
Thomas obadiah chisholm great is thy faithfulness lyrics
Types of faithfulness
Great is thy faithfulness 中文
Great is thy faithfulness 中文
Great is thu faithfulness
Brand loyalty synonym
Great is thy faithfulness 中文
Steve jobs adversity
Great is thy faithfulness oh god my father
Faithfulness in adversity
Great is your faithfulness oh god
Is the electronic exchange of money or scrip
Electronic field production
It provides a common framework to allow data to be shared
Interrupts in embedded system
Shared data model
Secondary uses of healthcare data
Late binding data warehouse
Medical internet of things and big data in healthcare
Jenis data kesehatan
Oracle healthcare data model
Healthcare data extraction
Introduction to healthcare data analytics
Healthcare data standards
Predictive prescriptive analytics
Demystifying healthcare data governance
Active record vs data mapper
Active record vs data mapper
Simbol garis atau line pada er model menunjukkan ....
Philip c. ritchey
Yang merupakan pengertian dari rekaman data adalah ?..
Perintah input dbase
Object-based data model
Electronic data sheets
Herds reporting
Edi trends
Electronic data capture rave
Electronic data interchange
A programmable electronic device designed to accept data
Electronic data interchange
Electronic dental data exchange
Edi exchange
Define edi in e commerce
Electronic data capture process flow
Shared inquiry definition
Shared reading vs read aloud
It shared service center
Powervm shared storage pools
Posix shared memory synchronization
Vccs ssc
Shared management model
Shared inquiry
Core practice model
Shared knowledge
It shared services examples
Hhhlh
Hrweb resa
Shared responsibility approach
Google translate english to french
Shared vs distributed memory
Distributed shared memory architecture tutorialspoint
Shared investigator platform login
Model hoy
Section 38-1 review echinoderms answer key
Broken yellow center line
Shared reading vs read aloud
Shared memory in java
Procurement shared services model
Jerome kevin and seth shared a submarine sandwich
Hr shared services model
Personal and shared knowledge
Shared services value proposition
Autoscopic psychosis
Is a shared system of meaning among employees
Nasa shared services center
Nasa shared service center
Share a single centralized memory
Knowledge shared is knowledge squared meaning
Knowledge shared is knowledge multiplied interpretation
Shared situational awareness jesip
Message passing os
Shared reading vs read aloud
Jhu hr shared services
Definition of guided reading
Shared reading vs read aloud