Data standards from the Proteomics Standards Initiative Andy
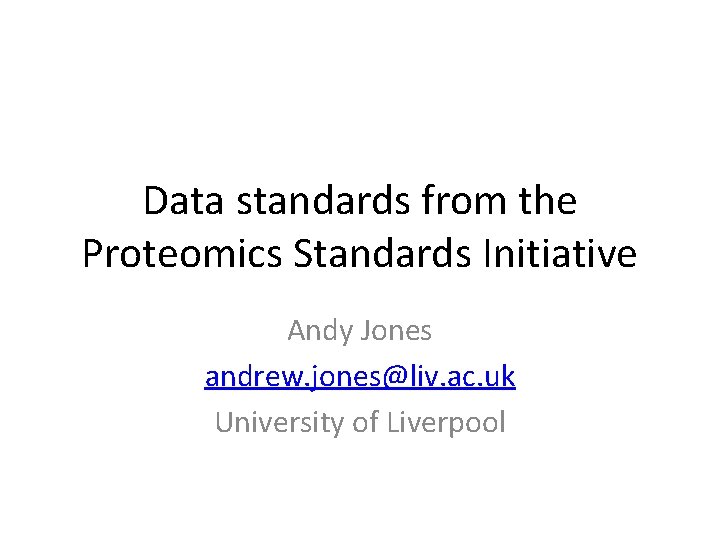
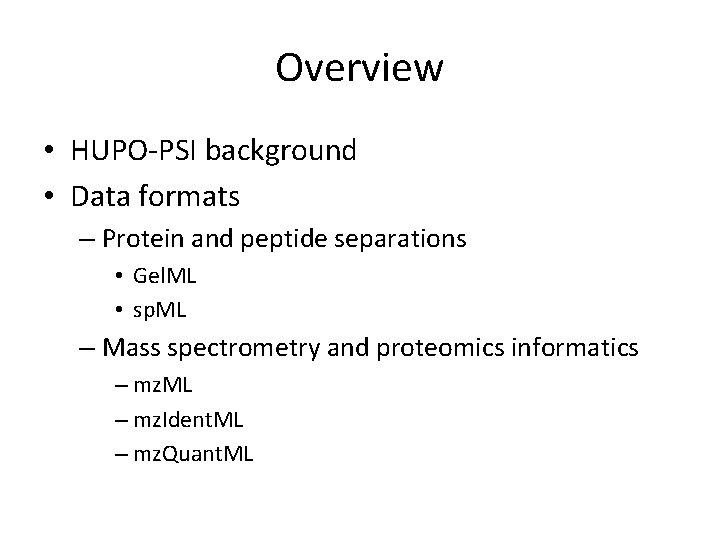
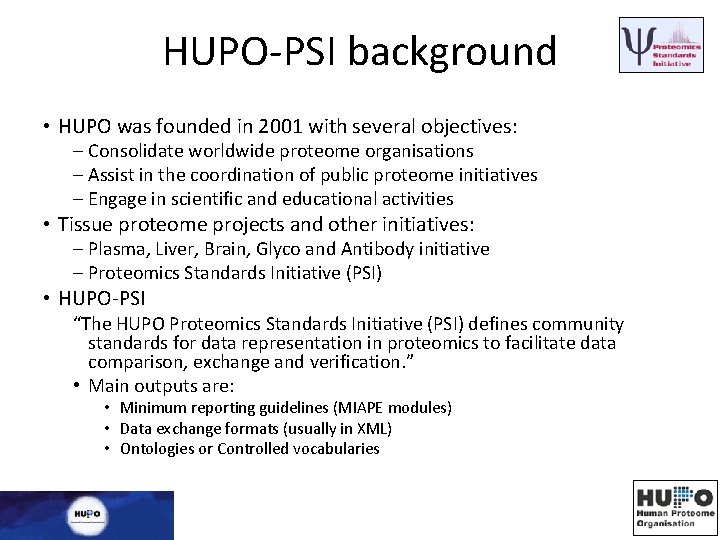
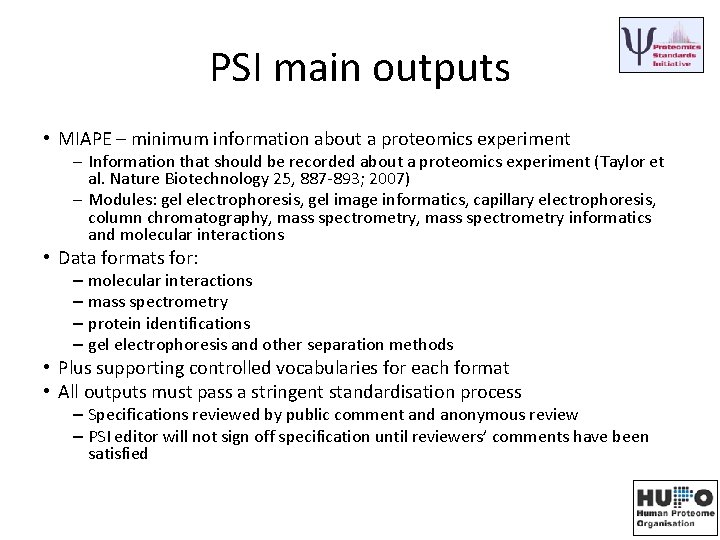
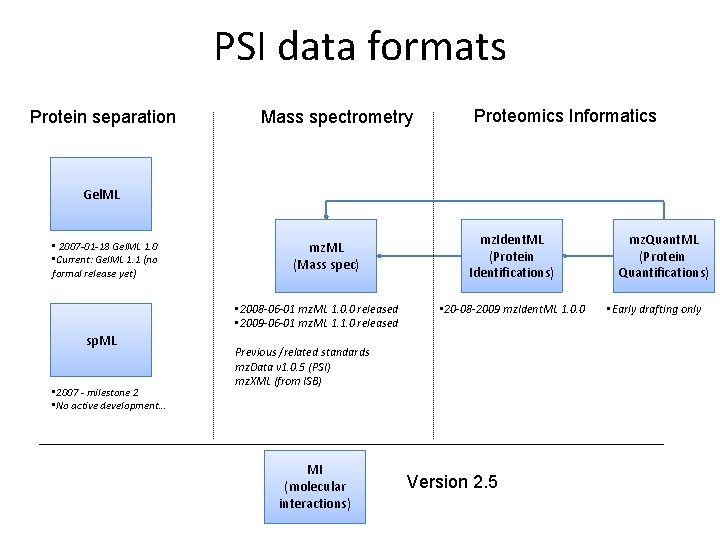
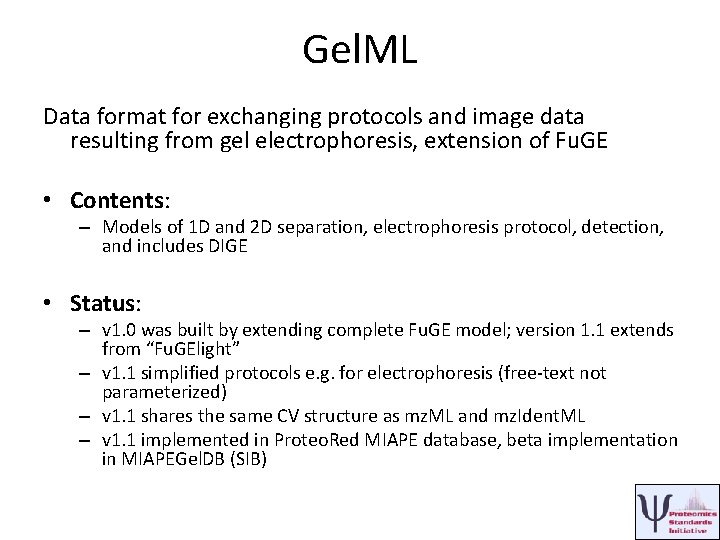
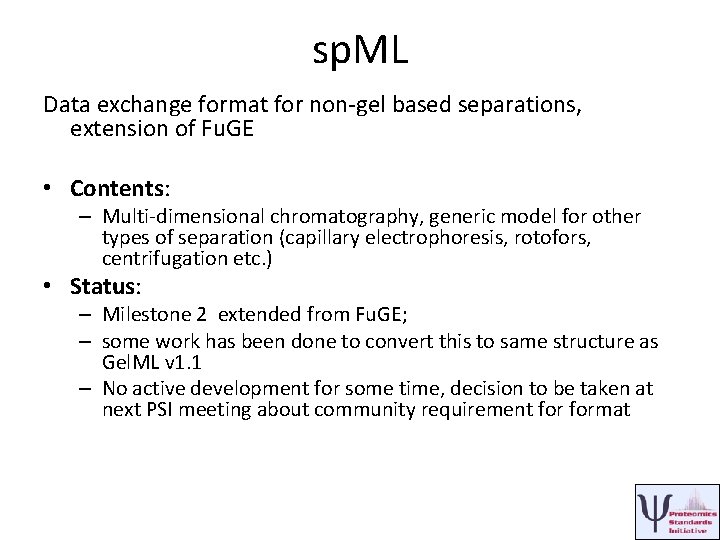
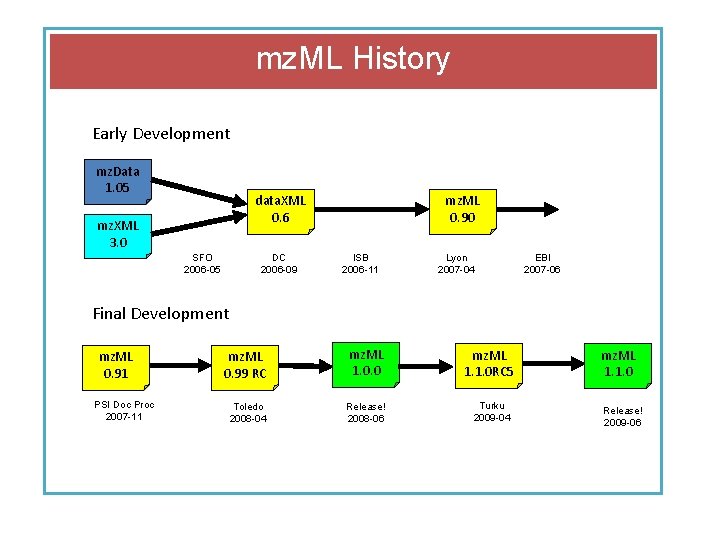
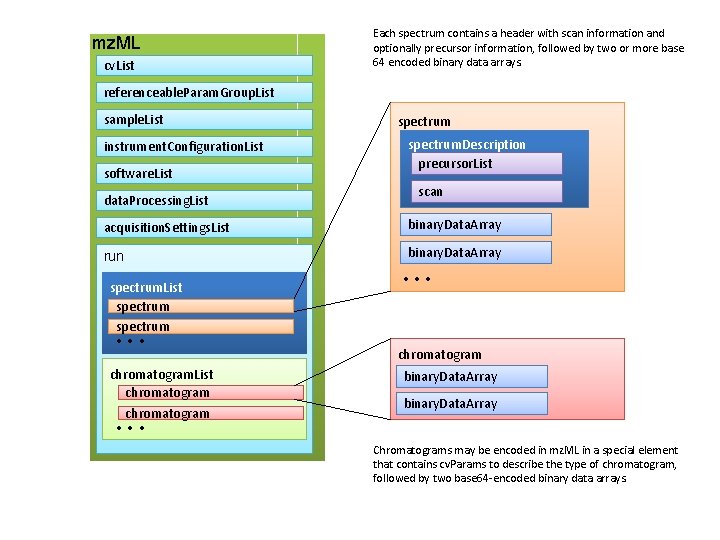
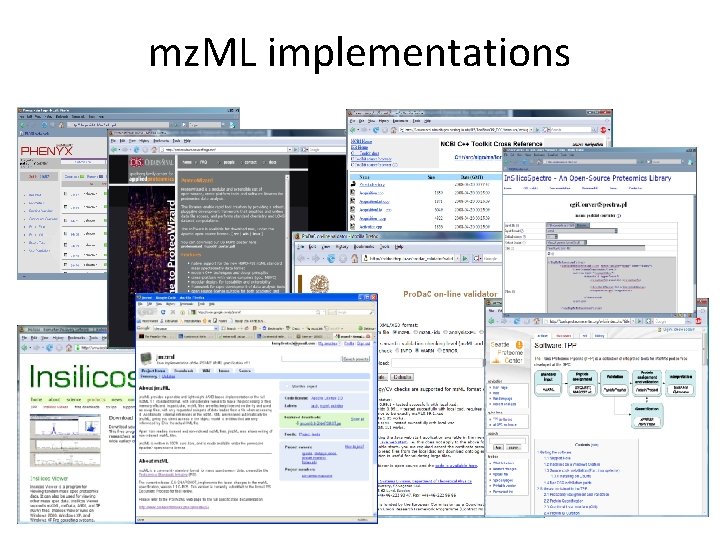
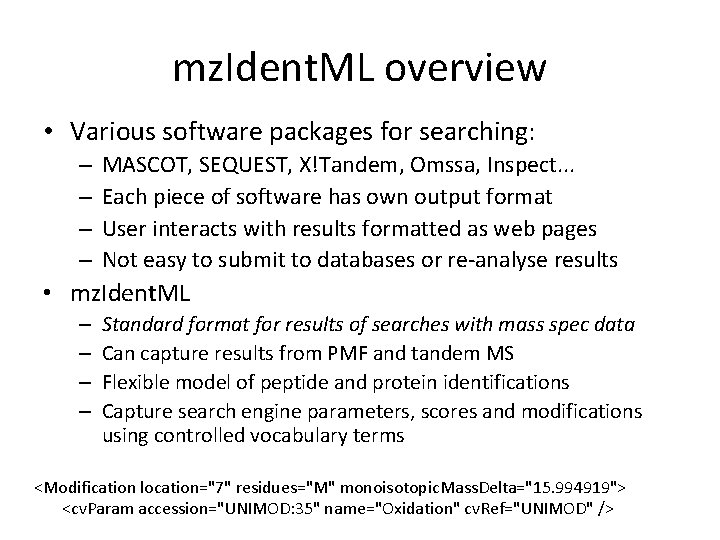
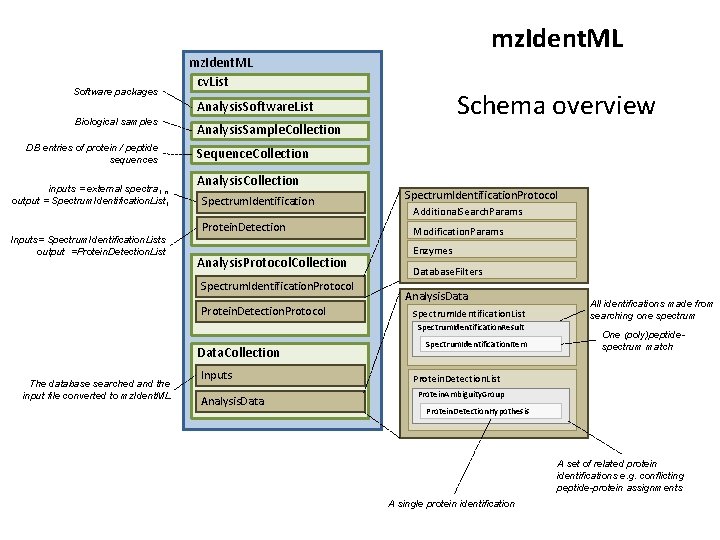
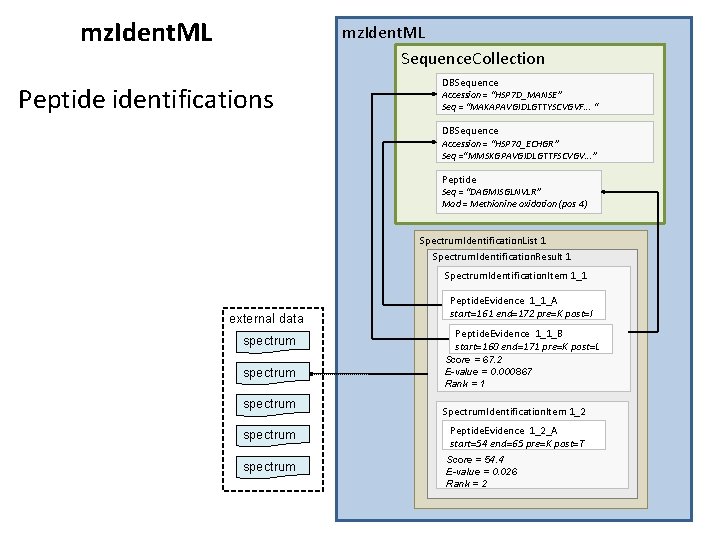
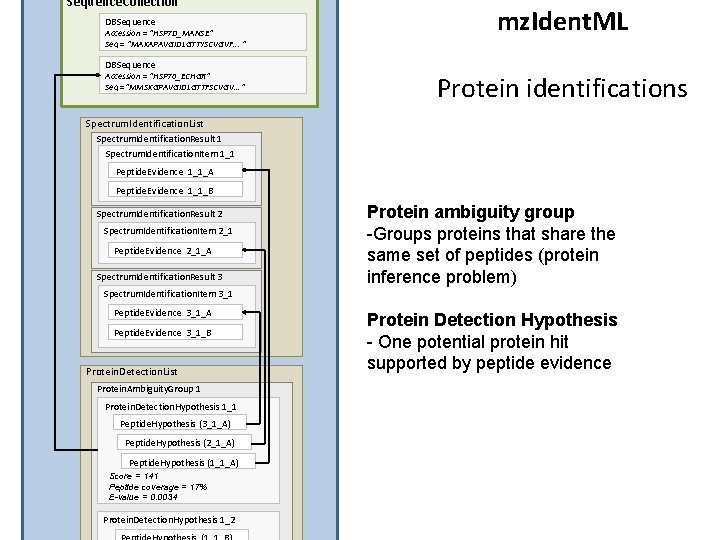
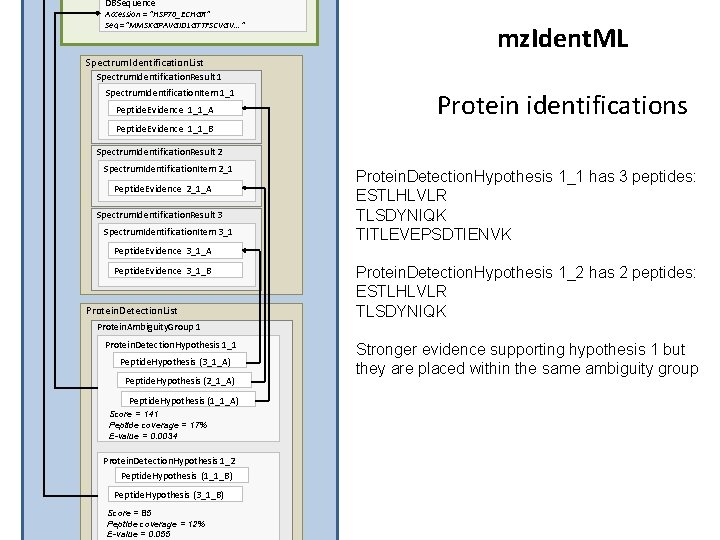
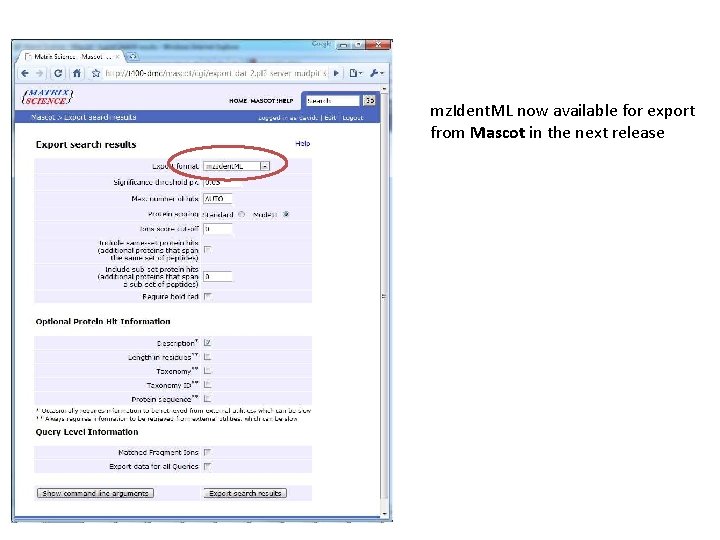
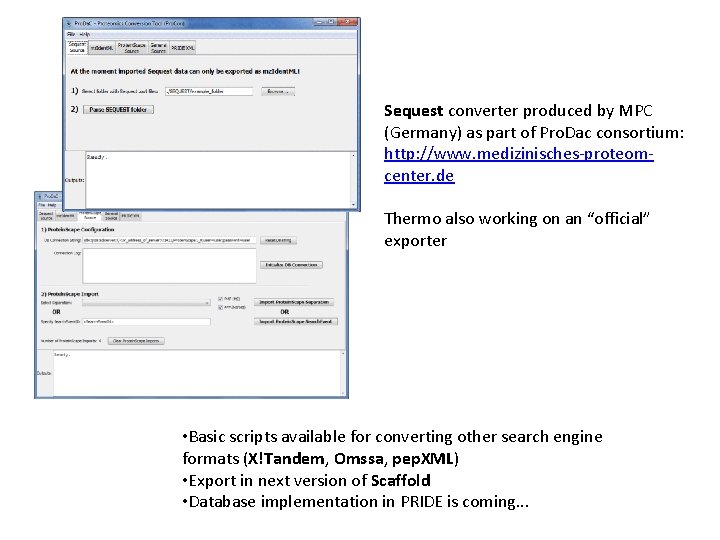
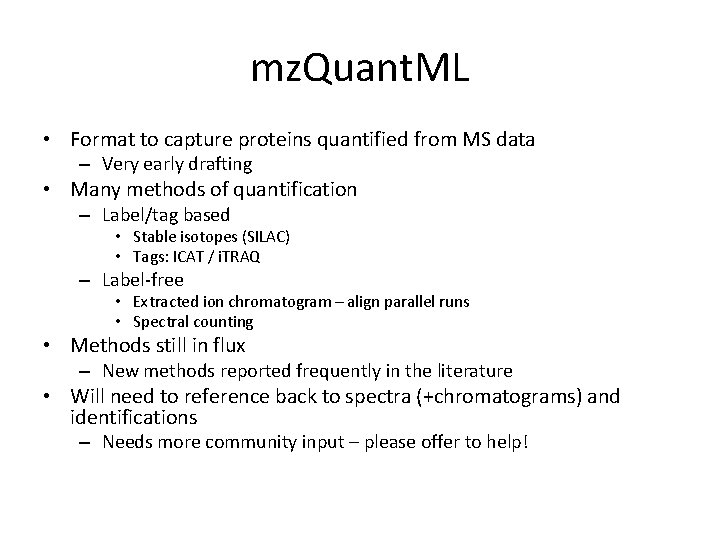
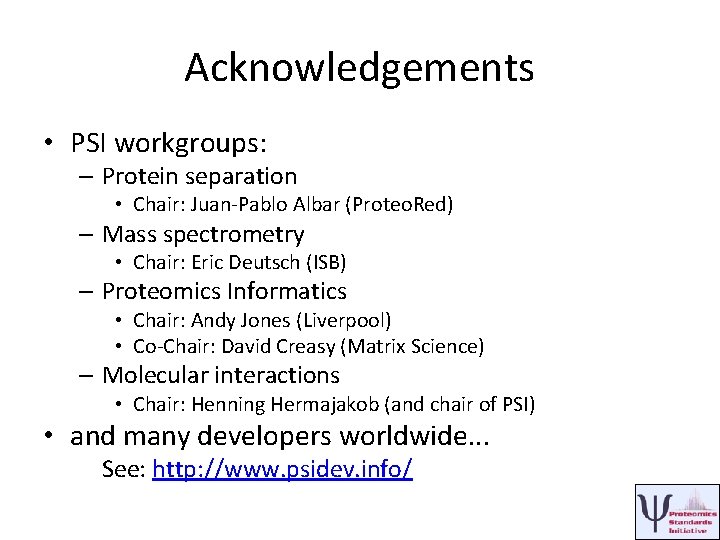
- Slides: 19
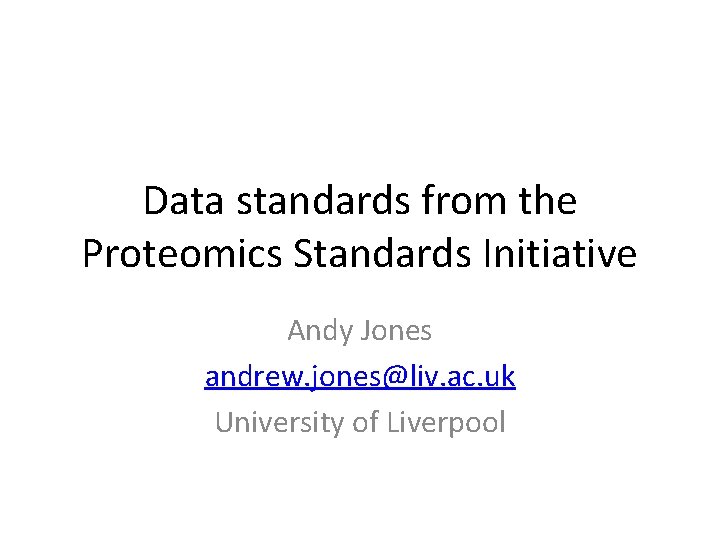
Data standards from the Proteomics Standards Initiative Andy Jones andrew. jones@liv. ac. uk University of Liverpool
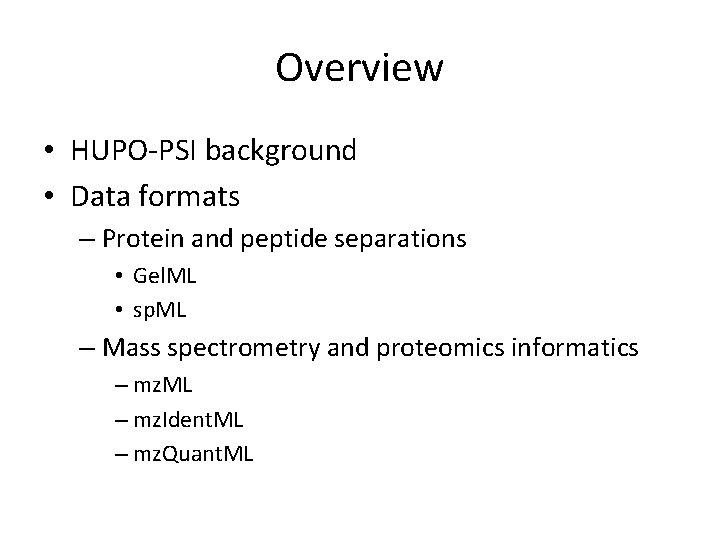
Overview • HUPO-PSI background • Data formats – Protein and peptide separations • Gel. ML • sp. ML – Mass spectrometry and proteomics informatics – mz. ML – mz. Ident. ML – mz. Quant. ML
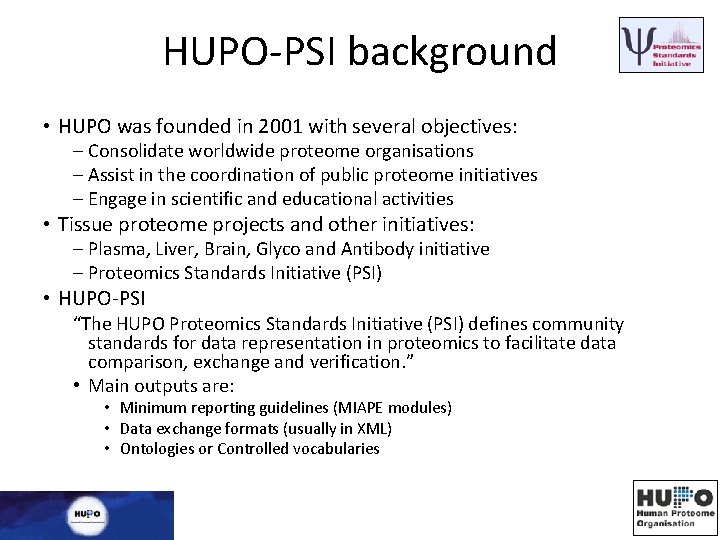
HUPO-PSI background • HUPO was founded in 2001 with several objectives: – Consolidate worldwide proteome organisations – Assist in the coordination of public proteome initiatives – Engage in scientific and educational activities • Tissue proteome projects and other initiatives: – Plasma, Liver, Brain, Glyco and Antibody initiative – Proteomics Standards Initiative (PSI) • HUPO-PSI “The HUPO Proteomics Standards Initiative (PSI) defines community standards for data representation in proteomics to facilitate data comparison, exchange and verification. ” • Main outputs are: • Minimum reporting guidelines (MIAPE modules) • Data exchange formats (usually in XML) • Ontologies or Controlled vocabularies
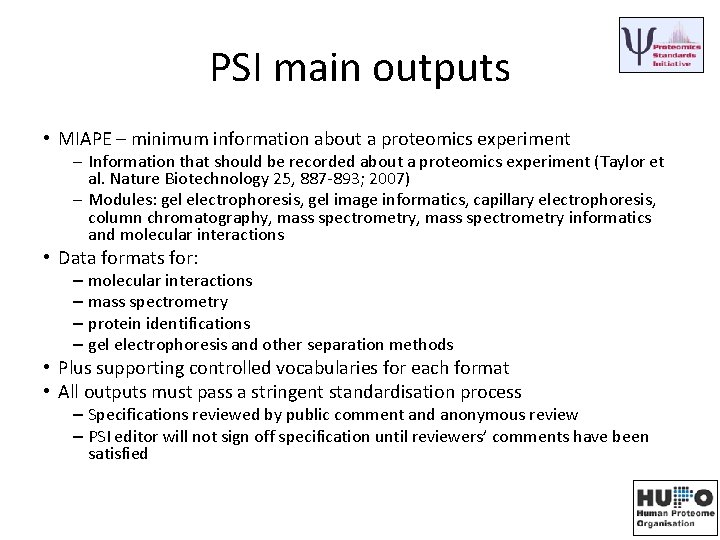
PSI main outputs • MIAPE – minimum information about a proteomics experiment – Information that should be recorded about a proteomics experiment (Taylor et al. Nature Biotechnology 25, 887 -893; 2007) – Modules: gel electrophoresis, gel image informatics, capillary electrophoresis, column chromatography, mass spectrometry informatics and molecular interactions • Data formats for: – molecular interactions – mass spectrometry – protein identifications – gel electrophoresis and other separation methods • Plus supporting controlled vocabularies for each format • All outputs must pass a stringent standardisation process – Specifications reviewed by public comment and anonymous review – PSI editor will not sign off specification until reviewers’ comments have been satisfied
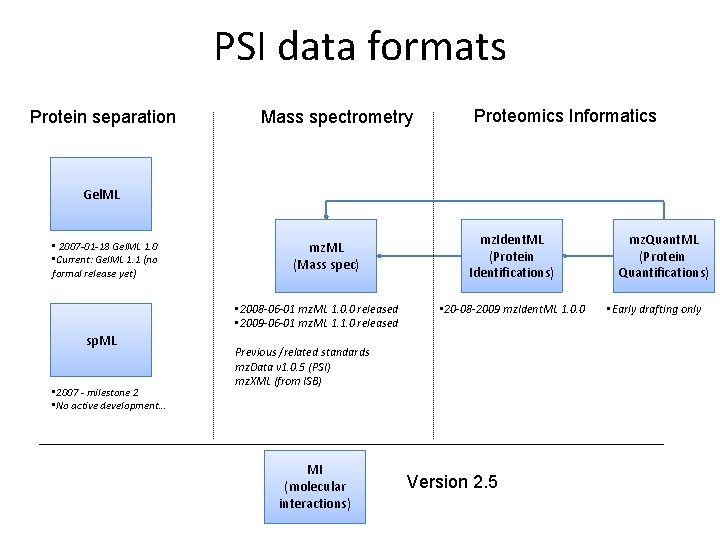
PSI data formats Protein separation Mass spectrometry Proteomics Informatics Gel. ML • 2007 -01 -18 Gel. ML 1. 0 • Current: Gel. ML 1. 1 (no formal release yet) mz. ML (Mass spec) • 2008 -06 -01 mz. ML 1. 0. 0 released • 2009 -06 -01 mz. ML 1. 1. 0 released sp. ML • 2007 - milestone 2 • No active development. . . mz. Ident. ML (Protein Identifications) • 20 -08 -2009 mz. Ident. ML 1. 0. 0 Previous /related standards mz. Data v 1. 0. 5 (PSI) mz. XML (from ISB) MI (molecular interactions) Version 2. 5 mz. Quant. ML (Protein Quantifications) • Early drafting only
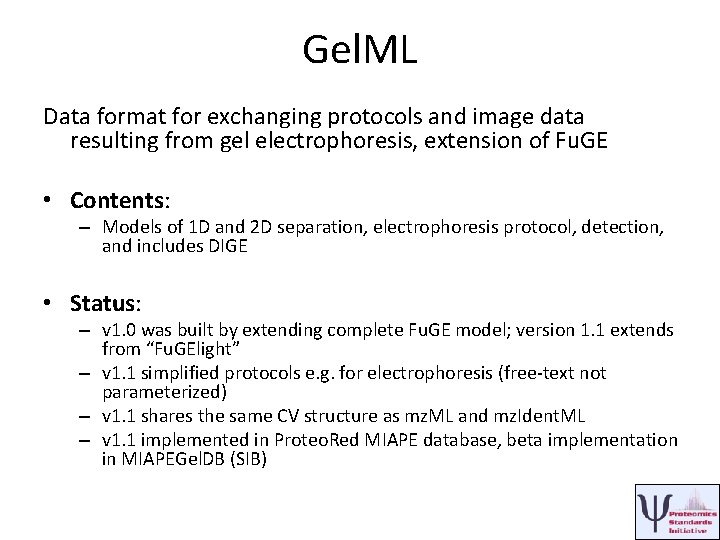
Gel. ML Data format for exchanging protocols and image data resulting from gel electrophoresis, extension of Fu. GE • Contents: – Models of 1 D and 2 D separation, electrophoresis protocol, detection, and includes DIGE • Status: – v 1. 0 was built by extending complete Fu. GE model; version 1. 1 extends from “Fu. GElight” – v 1. 1 simplified protocols e. g. for electrophoresis (free-text not parameterized) – v 1. 1 shares the same CV structure as mz. ML and mz. Ident. ML – v 1. 1 implemented in Proteo. Red MIAPE database, beta implementation in MIAPEGel. DB (SIB)
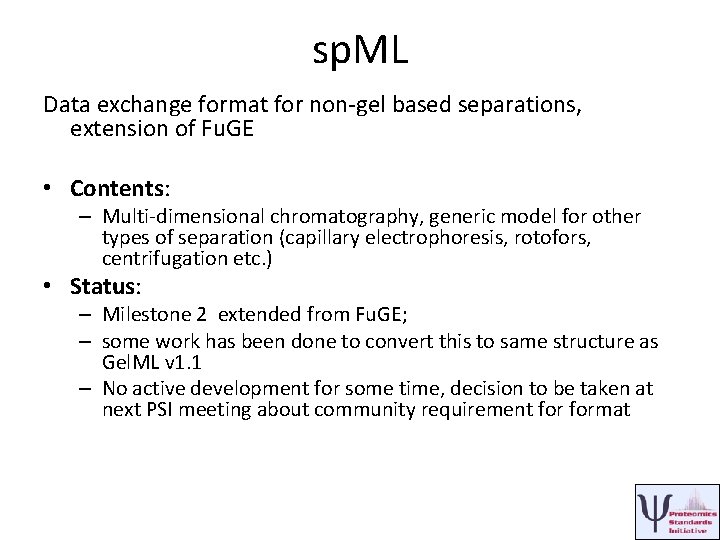
sp. ML Data exchange format for non-gel based separations, extension of Fu. GE • Contents: – Multi-dimensional chromatography, generic model for other types of separation (capillary electrophoresis, rotofors, centrifugation etc. ) • Status: – Milestone 2 extended from Fu. GE; – some work has been done to convert this to same structure as Gel. ML v 1. 1 – No active development for some time, decision to be taken at next PSI meeting about community requirement format
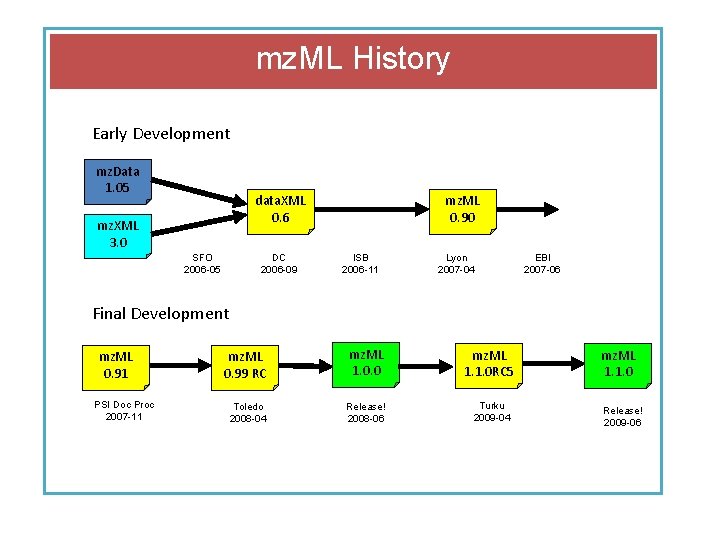
mz. ML History Early Development mz. Data 1. 05 data. XML 0. 6 mz. XML 3. 0 SFO 2006 -05 DC 2006 -09 mz. ML 0. 90 ISB 2006 -11 Lyon 2007 -04 EBI 2007 -06 Final Development mz. ML 0. 91 PSI Doc Proc 2007 -11 mz. ML 0. 99 RC mz. ML 1. 0. 0 mz. ML 1. 1. 0 RC 5 Toledo 2008 -04 Release! 2008 -06 Turku 2009 -04 mz. ML 1. 1. 0 Release! 2009 -06
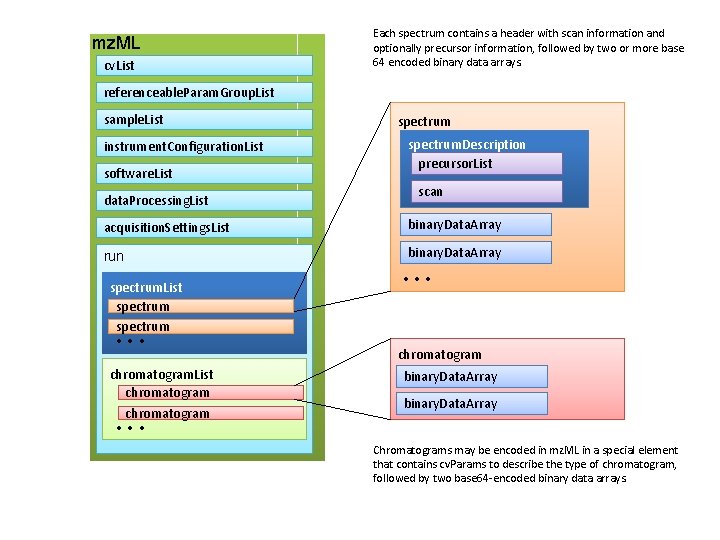
mz. ML cv. List Each spectrum contains a header with scan information and optionally precursor information, followed by two or more base 64 encoded binary data arrays. referenceable. Param. Group. List sample. List instrument. Configuration. List software. List data. Processing. List spectrum. Description precursor. List scan acquisition. Settings. List binary. Data. Array run binary. Data. Array spectrum. List spectrum • • • chromatogram. List chromatogram • • • chromatogram binary. Data. Array • • • Chromatograms may be encoded in mz. ML in a special element that contains cv. Params to describe the type of chromatogram, followed by two base 64 -encoded binary data arrays.
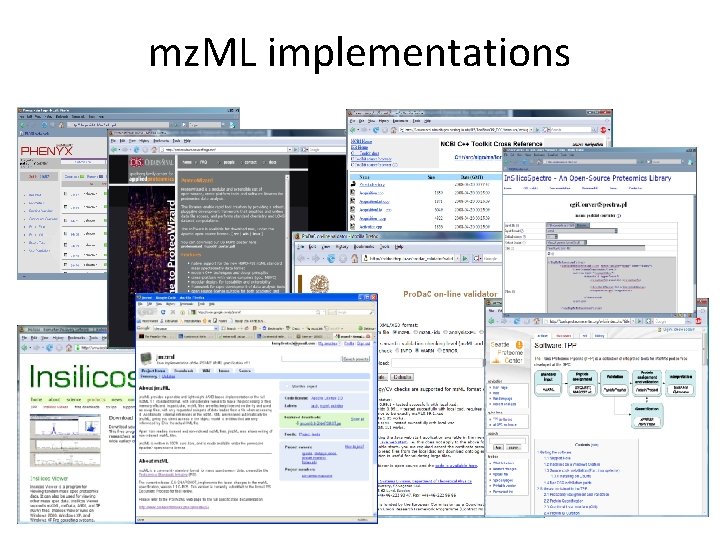
mz. ML implementations
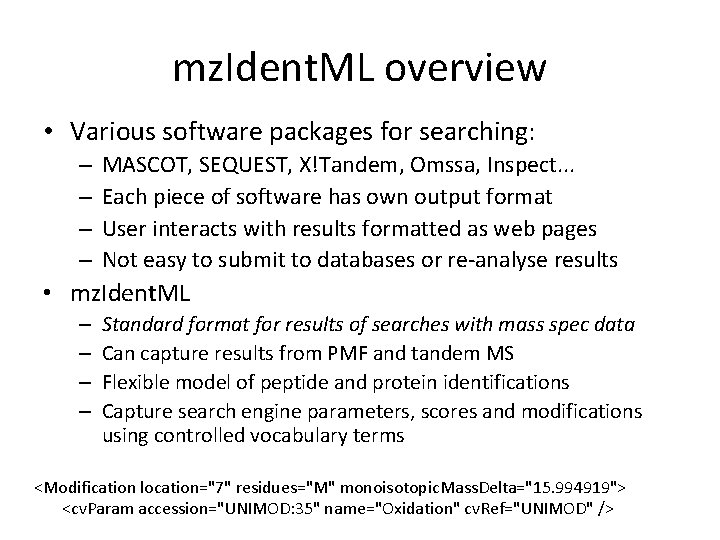
mz. Ident. ML overview • Various software packages for searching: – – MASCOT, SEQUEST, X!Tandem, Omssa, Inspect. . . Each piece of software has own output format User interacts with results formatted as web pages Not easy to submit to databases or re-analyse results • mz. Ident. ML – – Standard format for results of searches with mass spec data Can capture results from PMF and tandem MS Flexible model of peptide and protein identifications Capture search engine parameters, scores and modifications using controlled vocabulary terms <Modification location="7" residues="M" monoisotopic. Mass. Delta="15. 994919"> <cv. Param accession="UNIMOD: 35" name="Oxidation" cv. Ref="UNIMOD" />
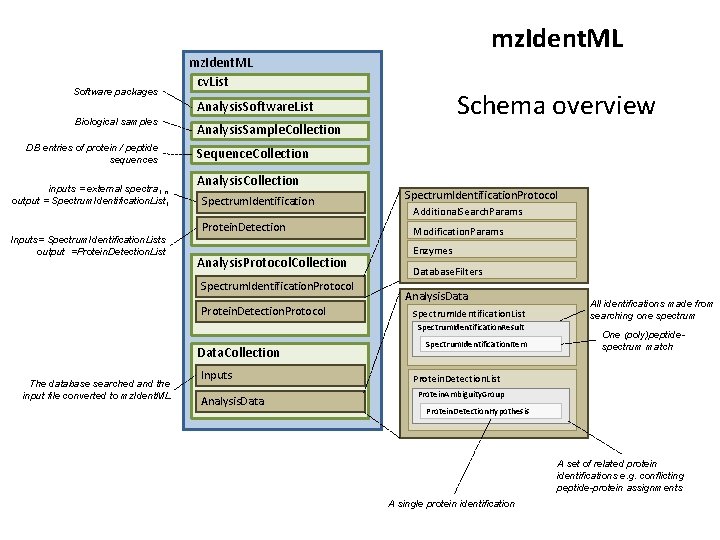
Software packages mz. Ident. ML cv. List Schema overview Analysis. Software. List Biological samples DB entries of protein / peptide sequences inputs = external spectra 1. . n output = Spectrum. Identification. List 1 Inputs= Spectrum. Identification. Lists output =Protein. Detection. List Analysis. Sample. Collection Sequence. Collection Analysis. Collection Spectrum. Identification Protein. Detection Analysis. Protocol. Collection Spectrum. Identification. Protocol Protein. Detection. Protocol Spectrum. Identification. Protocol Additional. Search. Params Modification. Params Enzymes Database. Filters Analysis. Data Spectrum. Identification. List Spectrum. Identification. Result Data. Collection The database searched and the input file converted to mz. Ident. ML Inputs Analysis. Data Spectrum. Identification. Item All identifications made from searching one spectrum One (poly)peptidespectrum match Protein. Detection. List Protein. Ambiguity. Group Protein. Detection. Hypothesis A set of related protein identifications e. g. conflicting peptide-protein assignments A single protein identification
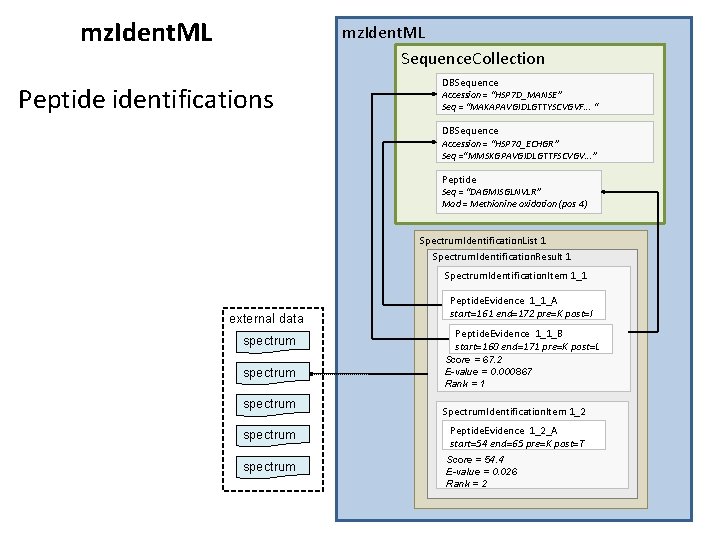
mz. Ident. ML Sequence. Collection Peptide identifications DBSequence Accession = “HSP 7 D_MANSE” Seq = “MAKAPAVGIDLGTTYSCVGVF. . . “ DBSequence Accession = “HSP 70_ECHGR” Seq =“MMSKGPAVGIDLGTTFSCVGV. . . ” Peptide Seq = “DAGMISGLNVLR” Mod = Methionine oxidation (pos 4) Spectrum. Identification. List 1 Spectrum. Identification. Result 1 Spectrum. Identification. Item 1_1 external data spectrum spectrum Peptide. Evidence 1_1_A start=161 end=172 pre=K post=I Peptide. Evidence 1_1_B start=160 end=171 pre=K post=L Score = 67. 2 E-value = 0. 000867 Rank = 1 Spectrum. Identification. Item 1_2 Peptide. Evidence 1_2_A start=54 end=65 pre=K post=T Score = 54. 4 E-value = 0. 026 Rank = 2
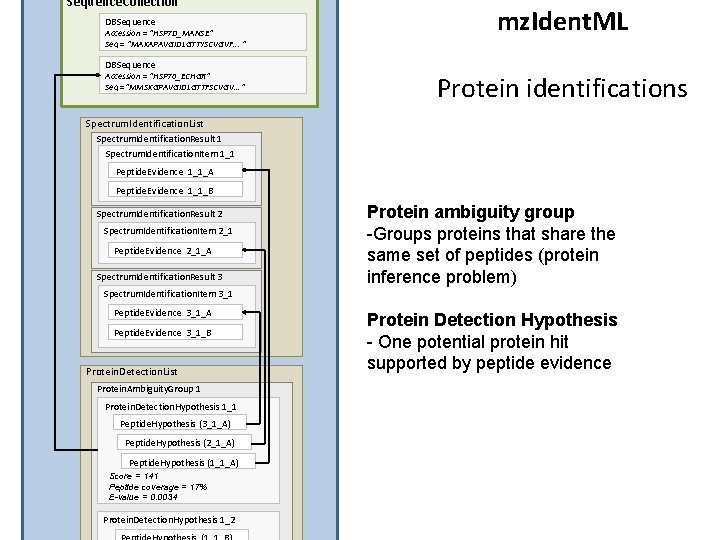
Sequence. Collection DBSequence Accession = “HSP 7 D_MANSE” Seq = “MAKAPAVGIDLGTTYSCVGVF. . . “ DBSequence Accession = “HSP 70_ECHGR” Seq =“MMSKGPAVGIDLGTTFSCVGV. . . ” mz. Ident. ML Protein identifications Spectrum. Identification. List Spectrum. Identification. Result 1 Spectrum. Identification. Item 1_1 Peptide. Evidence 1_1_A Peptide. Evidence 1_1_B Spectrum. Identification. Result 2 Spectrum. Identification. Item 2_1 Peptide. Evidence 2_1_A Spectrum. Identification. Result 3 Protein ambiguity group -Groups proteins that share the same set of peptides (protein inference problem) Spectrum. Identification. Item 3_1 Peptide. Evidence 3_1_A Peptide. Evidence 3_1_B Protein. Detection. List Protein. Ambiguity. Group 1 Protein. Detection. Hypothesis 1_1 Peptide. Hypothesis (3_1_A) Peptide. Hypothesis (2_1_A) Peptide. Hypothesis (1_1_A) Score = 141 Peptide coverage = 17% E-value = 0. 0034 Protein. Detection. Hypothesis 1_2 Protein Detection Hypothesis - One potential protein hit supported by peptide evidence
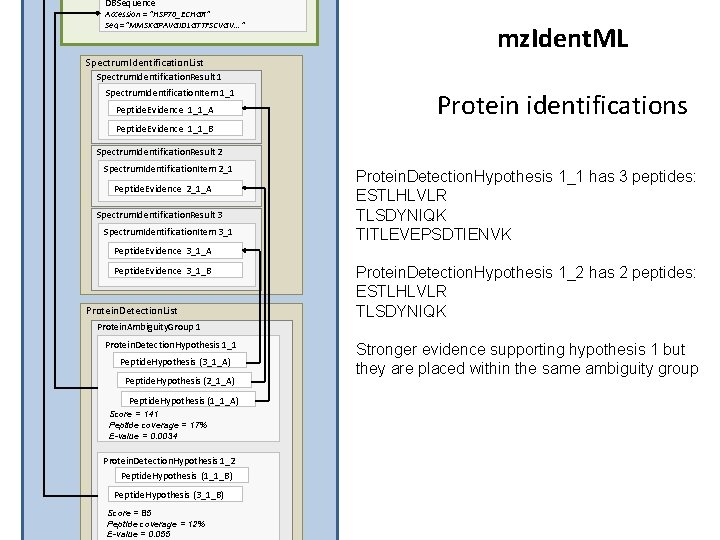
DBSequence Accession = “HSP 70_ECHGR” Seq =“MMSKGPAVGIDLGTTFSCVGV. . . ” mz. Ident. ML Spectrum. Identification. List Spectrum. Identification. Result 1 Spectrum. Identification. Item 1_1 Peptide. Evidence 1_1_A Protein identifications Peptide. Evidence 1_1_B Spectrum. Identification. Result 2 Spectrum. Identification. Item 2_1 Peptide. Evidence 2_1_A Spectrum. Identification. Result 3 Spectrum. Identification. Item 3_1 Protein. Detection. Hypothesis 1_1 has 3 peptides: ESTLHLVLR TLSDYNIQK TITLEVEPSDTIENVK Peptide. Evidence 3_1_A Peptide. Evidence 3_1_B Protein. Detection. List Protein. Detection. Hypothesis 1_2 has 2 peptides: ESTLHLVLR TLSDYNIQK Protein. Ambiguity. Group 1 Protein. Detection. Hypothesis 1_1 Peptide. Hypothesis (3_1_A) Peptide. Hypothesis (2_1_A) Peptide. Hypothesis (1_1_A) Score = 141 Peptide coverage = 17% E-value = 0. 0034 Protein. Detection. Hypothesis 1_2 Peptide. Hypothesis (1_1_B) Peptide. Hypothesis (3_1_B) Score = 85 Peptide coverage = 12% E-value = 0. 055 Stronger evidence supporting hypothesis 1 but they are placed within the same ambiguity group
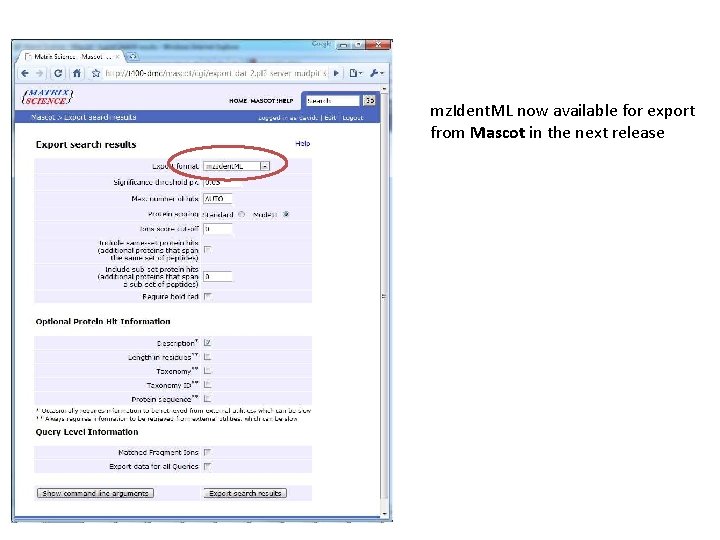
mz. Ident. ML now available for export from Mascot in the next release
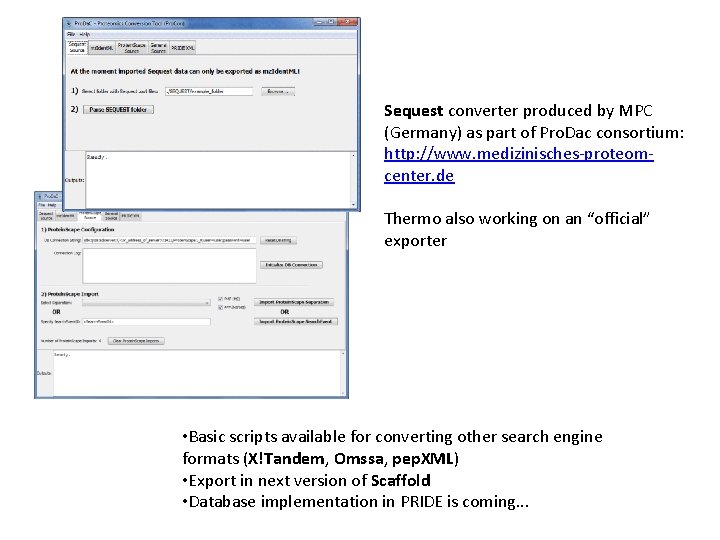
Sequest converter produced by MPC (Germany) as part of Pro. Dac consortium: http: //www. medizinisches-proteomcenter. de Thermo also working on an “official” exporter • Basic scripts available for converting other search engine formats (X!Tandem, Omssa, pep. XML) • Export in next version of Scaffold • Database implementation in PRIDE is coming. . .
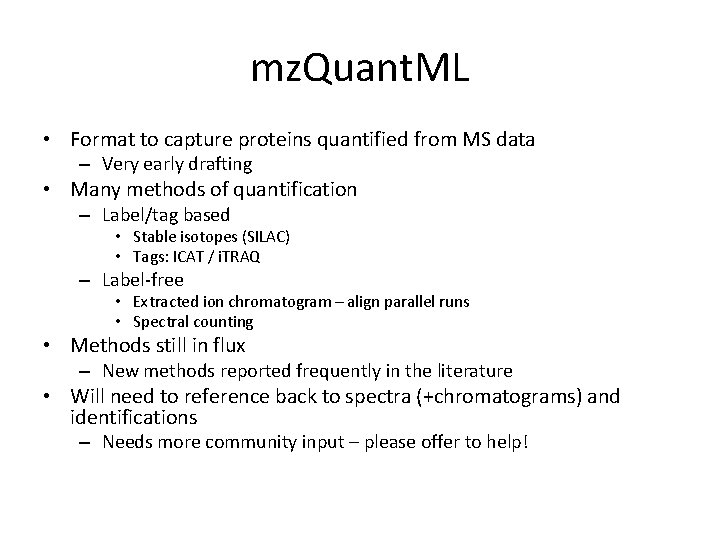
mz. Quant. ML • Format to capture proteins quantified from MS data – Very early drafting • Many methods of quantification – Label/tag based • Stable isotopes (SILAC) • Tags: ICAT / i. TRAQ – Label-free • Extracted ion chromatogram – align parallel runs • Spectral counting • Methods still in flux – New methods reported frequently in the literature • Will need to reference back to spectra (+chromatograms) and identifications – Needs more community input – please offer to help!
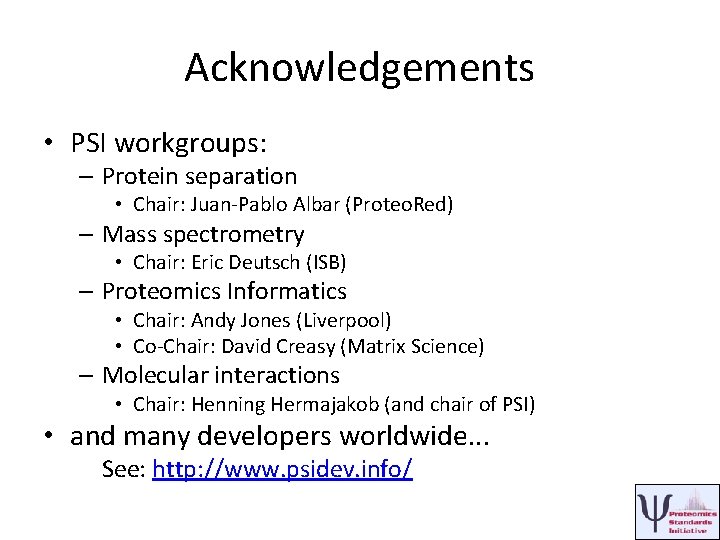
Acknowledgements • PSI workgroups: – Protein separation • Chair: Juan-Pablo Albar (Proteo. Red) – Mass spectrometry • Chair: Eric Deutsch (ISB) – Proteomics Informatics • Chair: Andy Jones (Liverpool) • Co-Chair: David Creasy (Matrix Science) – Molecular interactions • Chair: Henning Hermajakob (and chair of PSI) • and many developers worldwide. . . See: http: //www. psidev. info/
Mini trans-blot module
History of proteomics
Sac spectrogram
Prionproteine
Comparative proteomics kit ii western blot module
Comparative proteomics kit ii western blot module
Comparative proteomics kit ii western blot module
Wdqi
Open data initiative
Hát kết hợp bộ gõ cơ thể
Bổ thể
Tỉ lệ cơ thể trẻ em
Voi kéo gỗ như thế nào
Tư thế worm breton
Alleluia hat len nguoi oi
Các môn thể thao bắt đầu bằng tiếng bóng
Thế nào là hệ số cao nhất
Các châu lục và đại dương trên thế giới
Công thức tính thế năng