Data Science in Financial Services Michel Kamel Senior
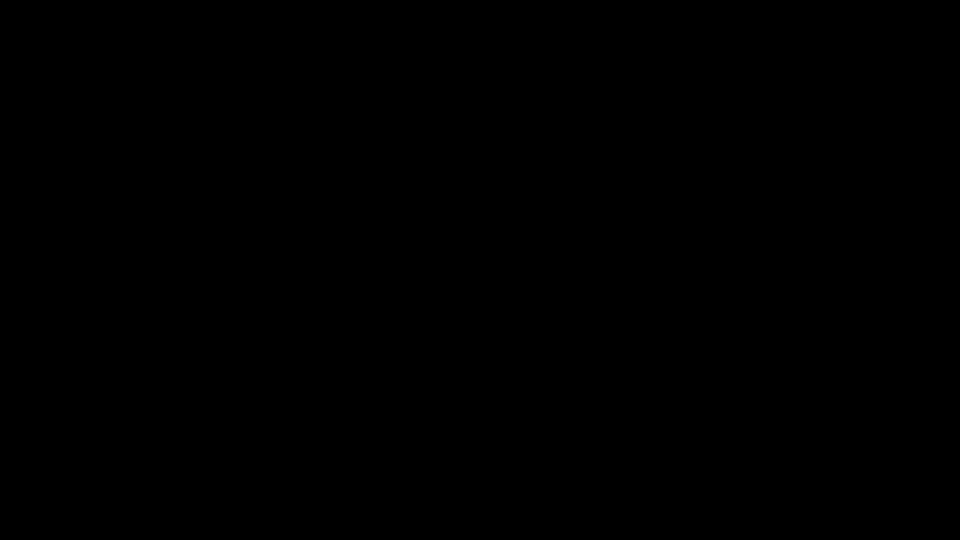
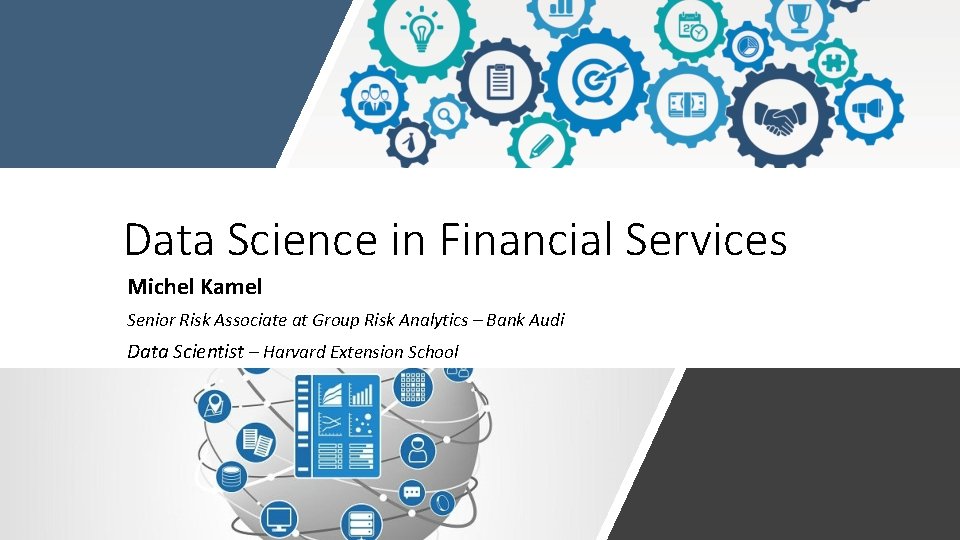
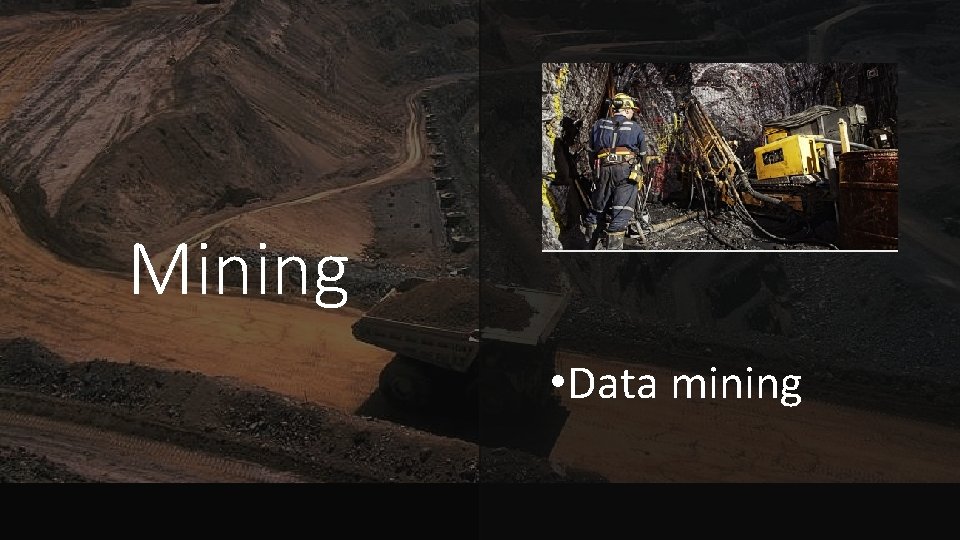
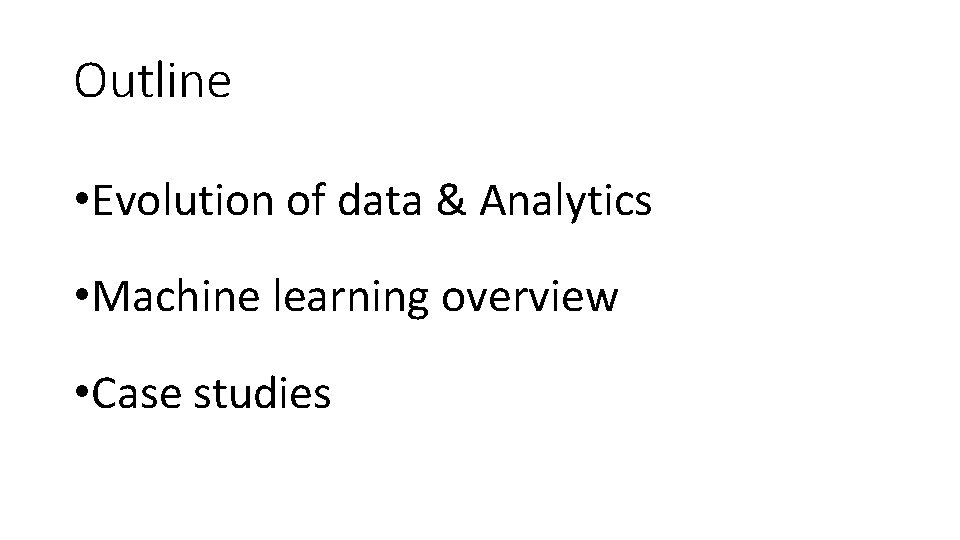
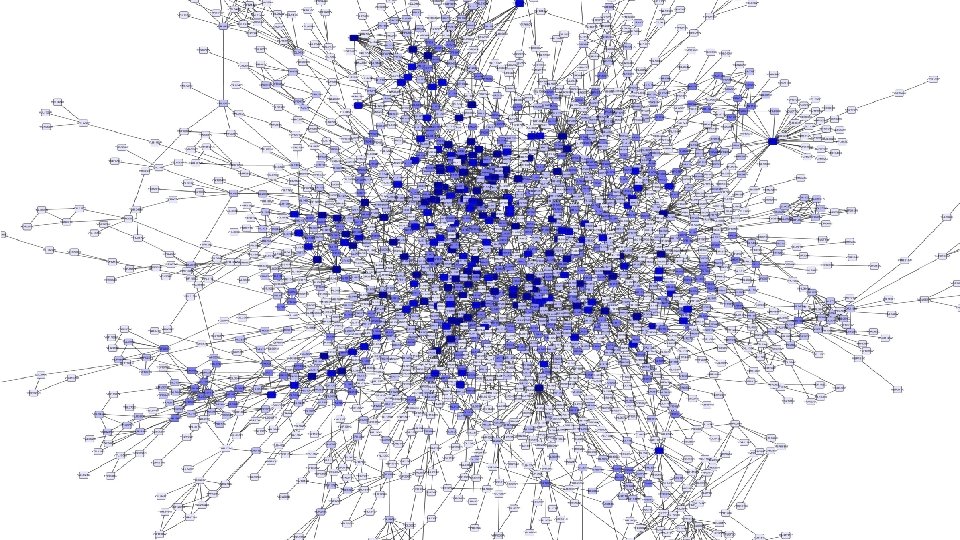
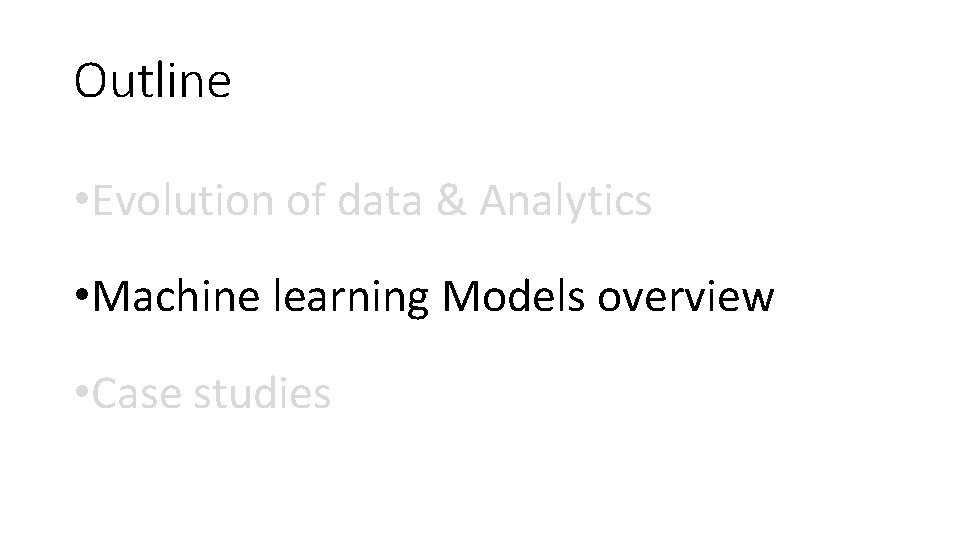
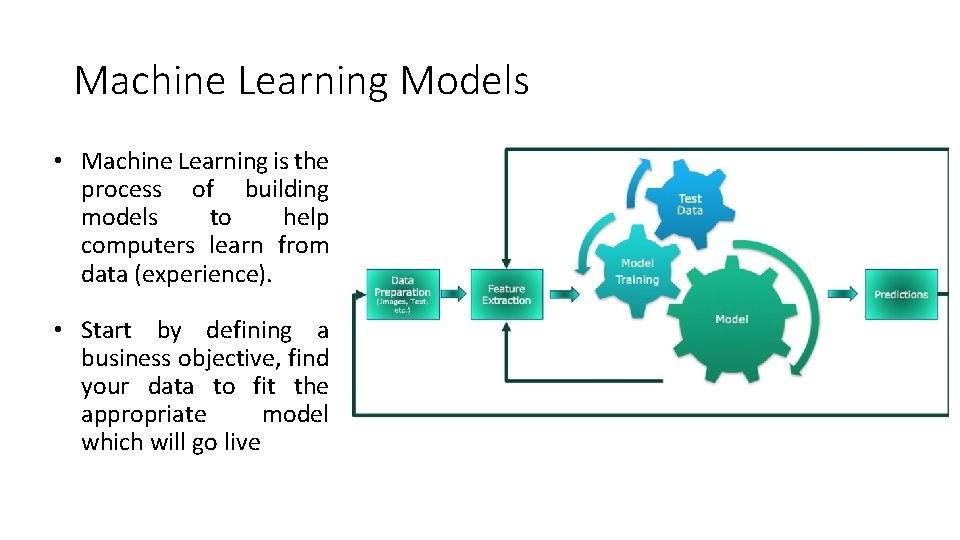
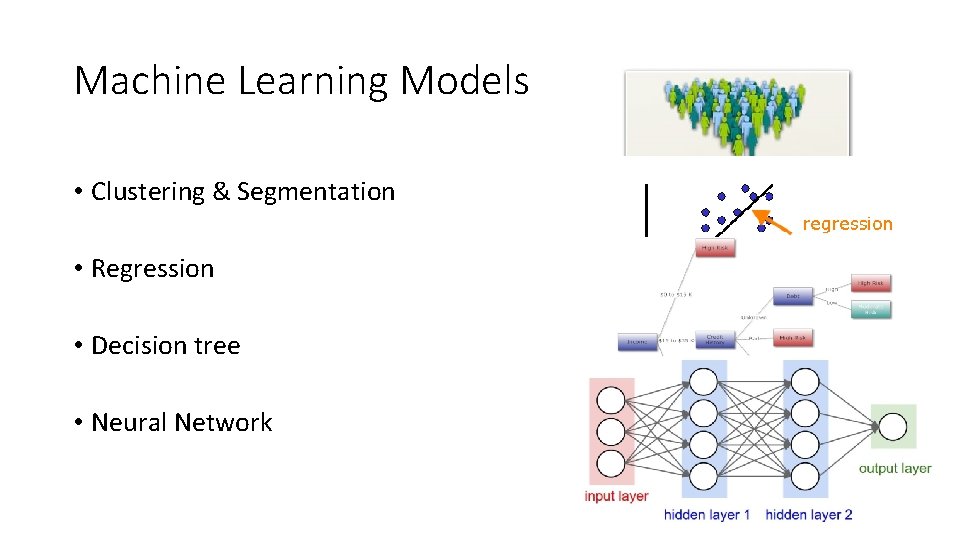
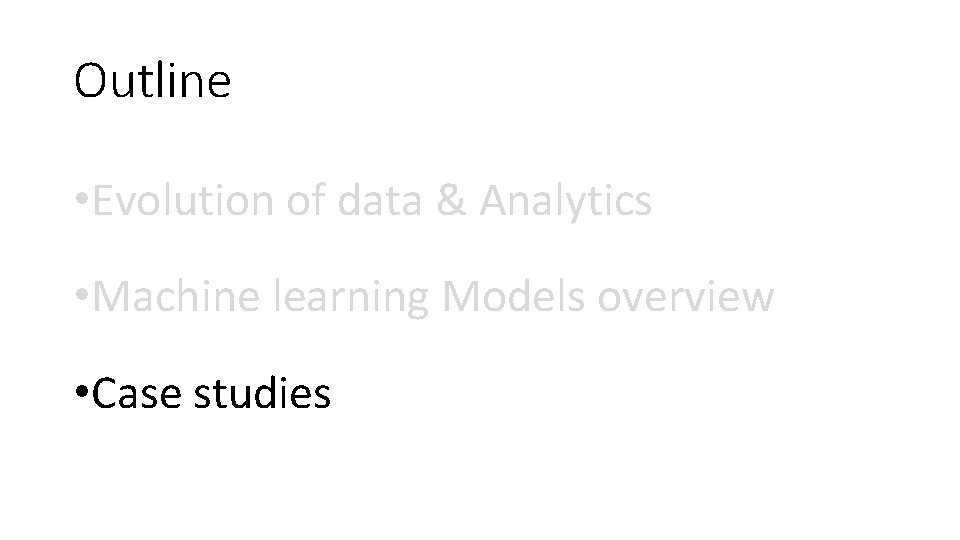
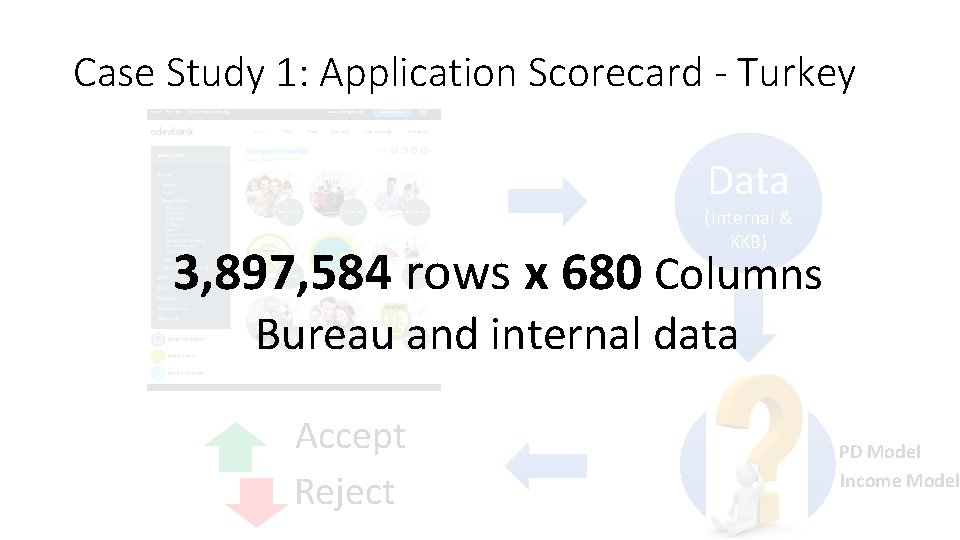
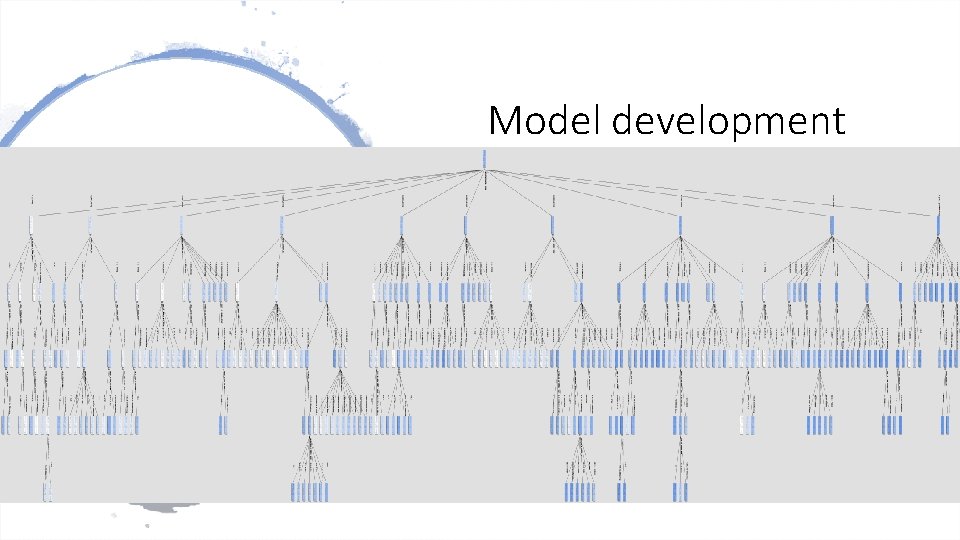
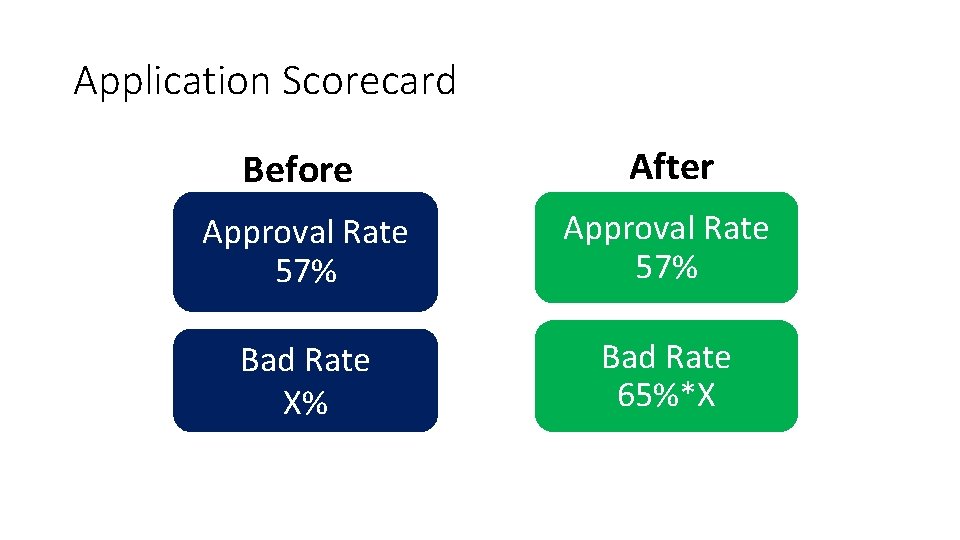
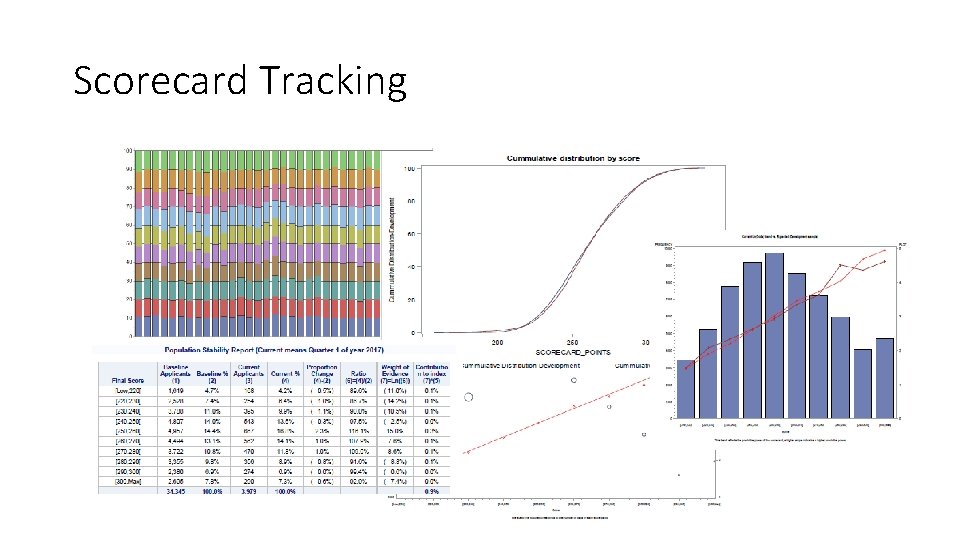
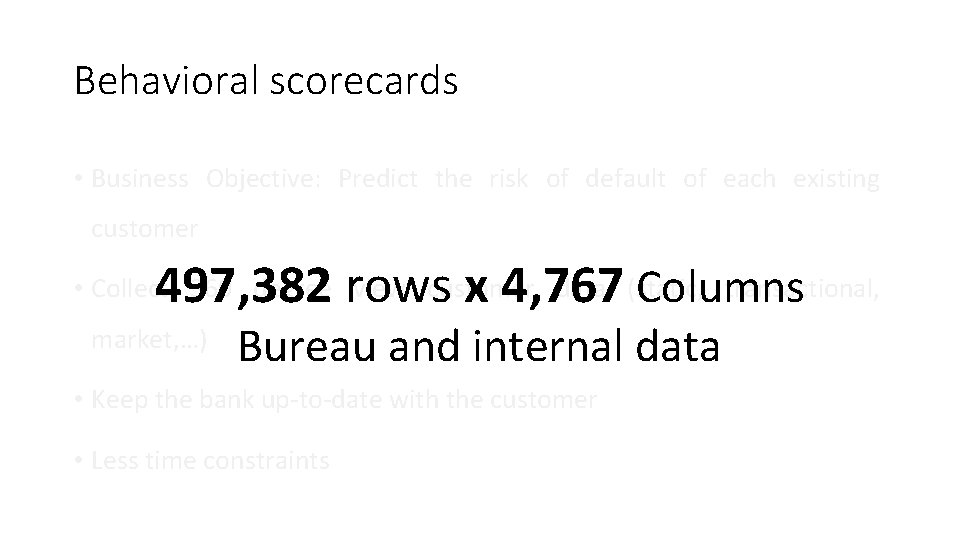
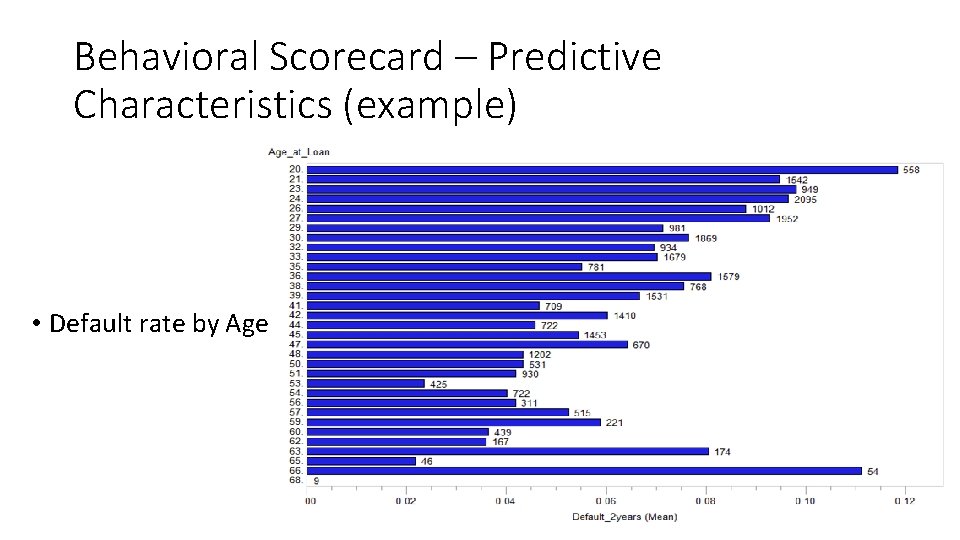
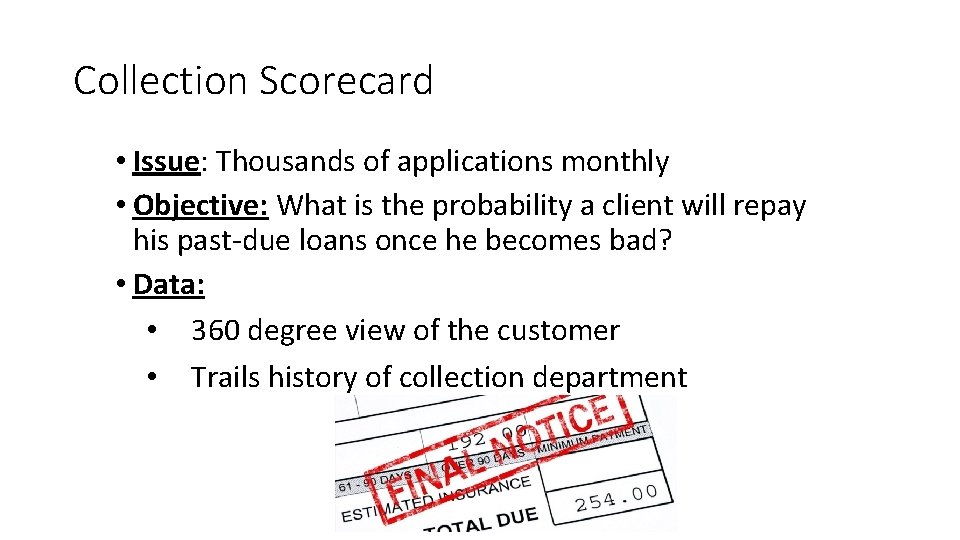
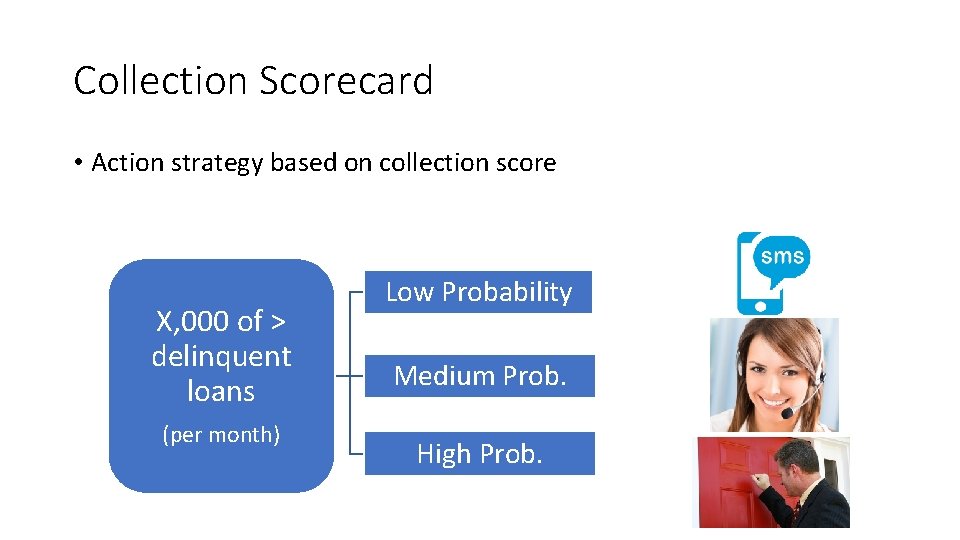
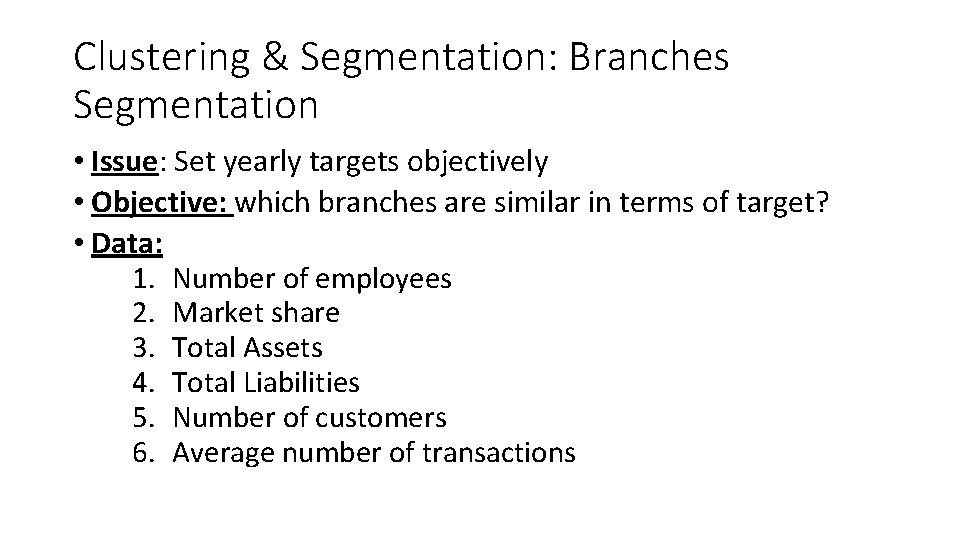
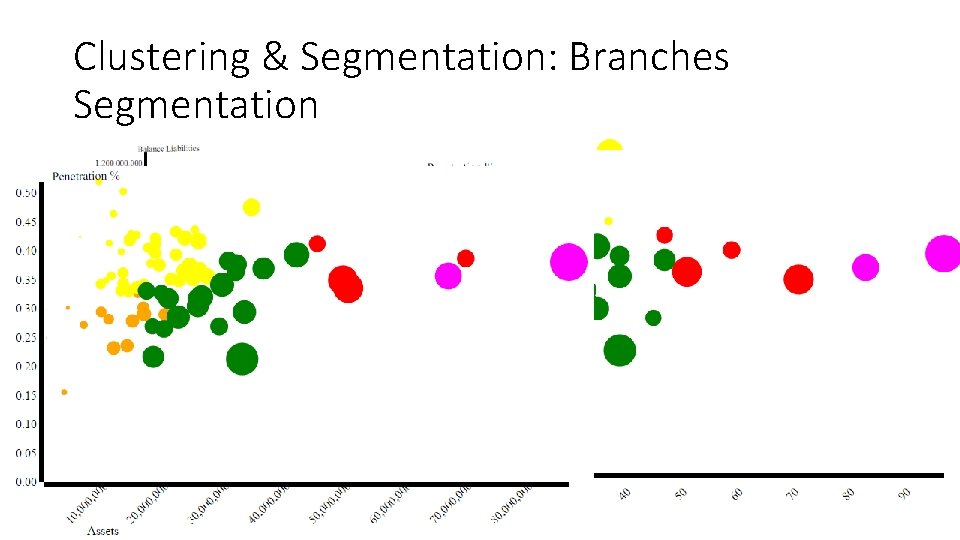
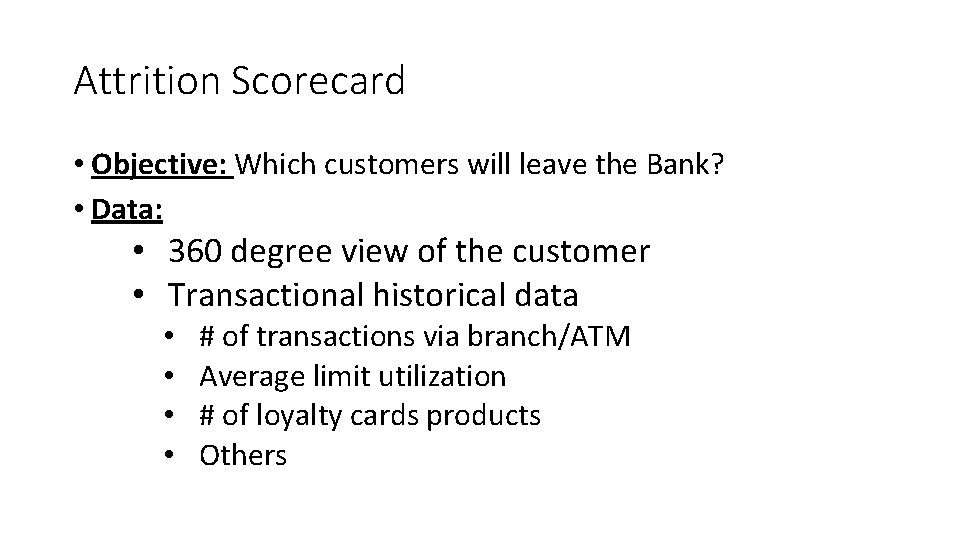
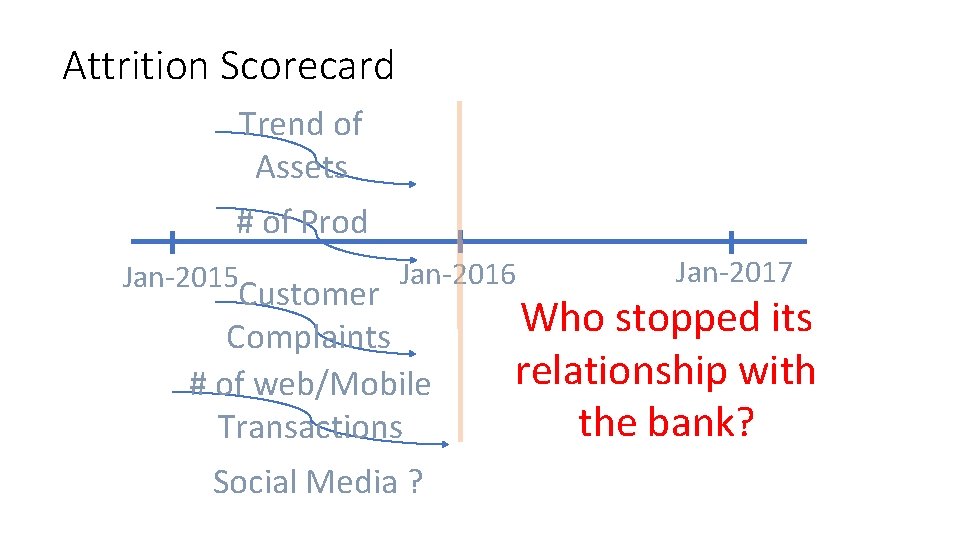
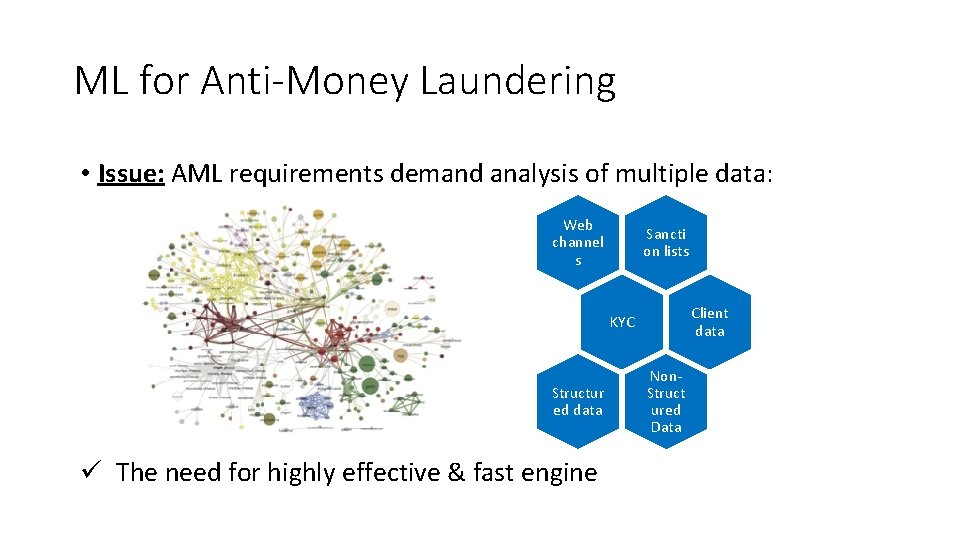
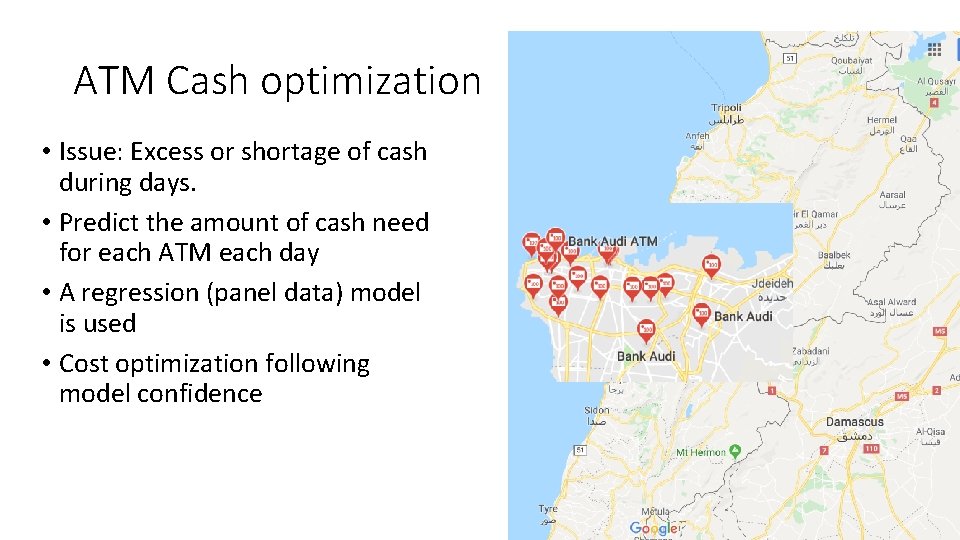
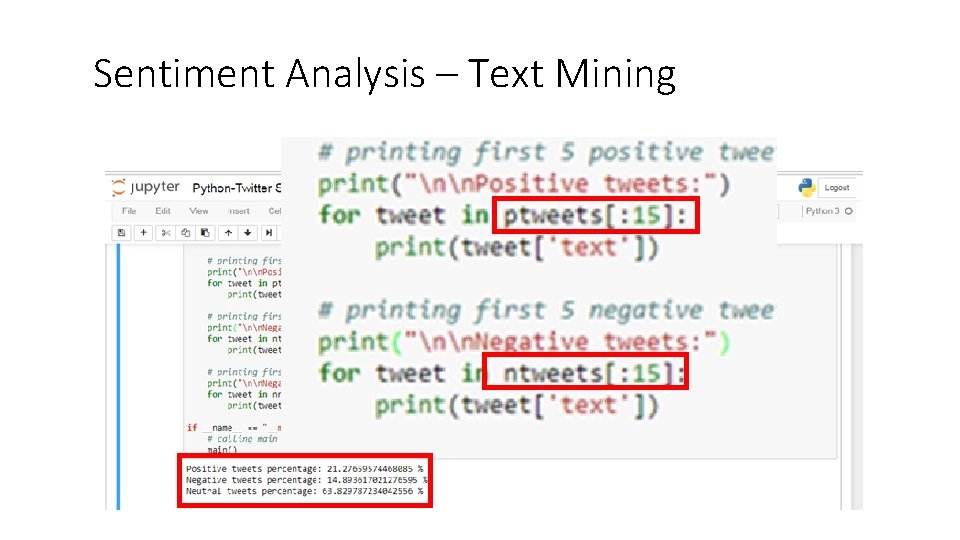
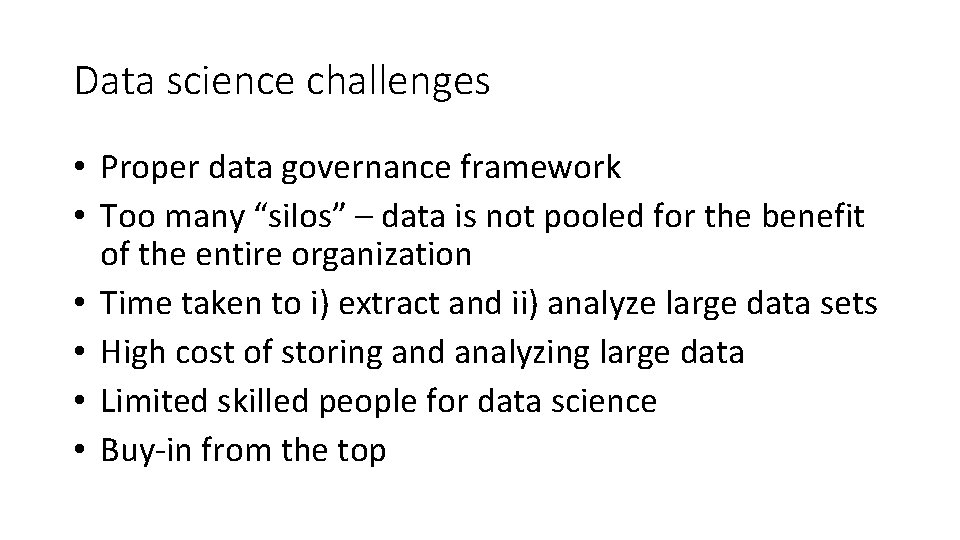
- Slides: 25
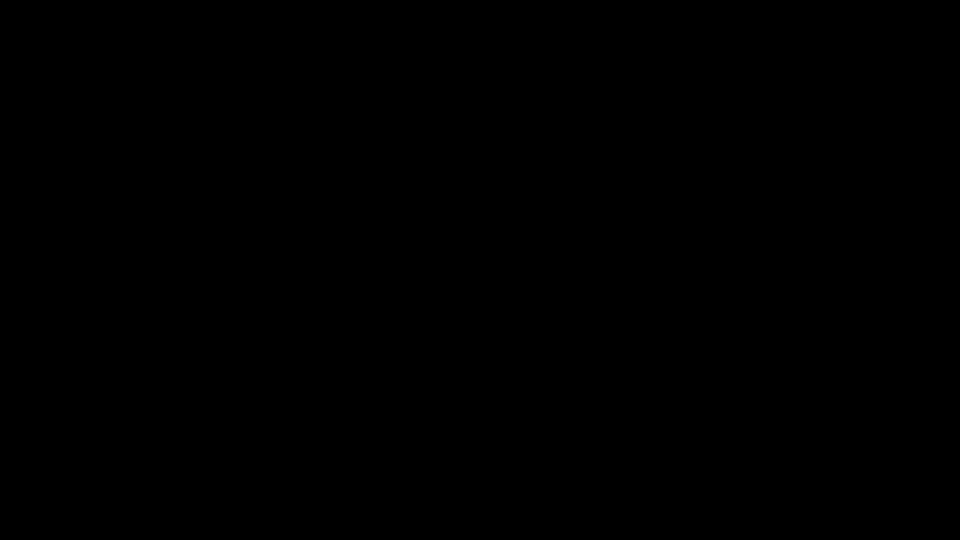
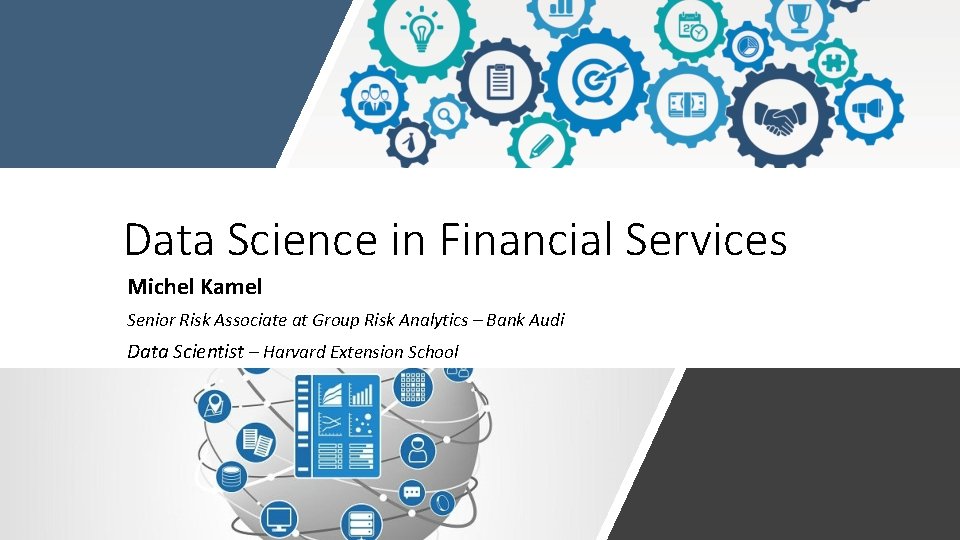
Data Science in Financial Services Michel Kamel Senior Risk Associate at Group Risk Analytics – Bank Audi Data Scientist – Harvard Extension School
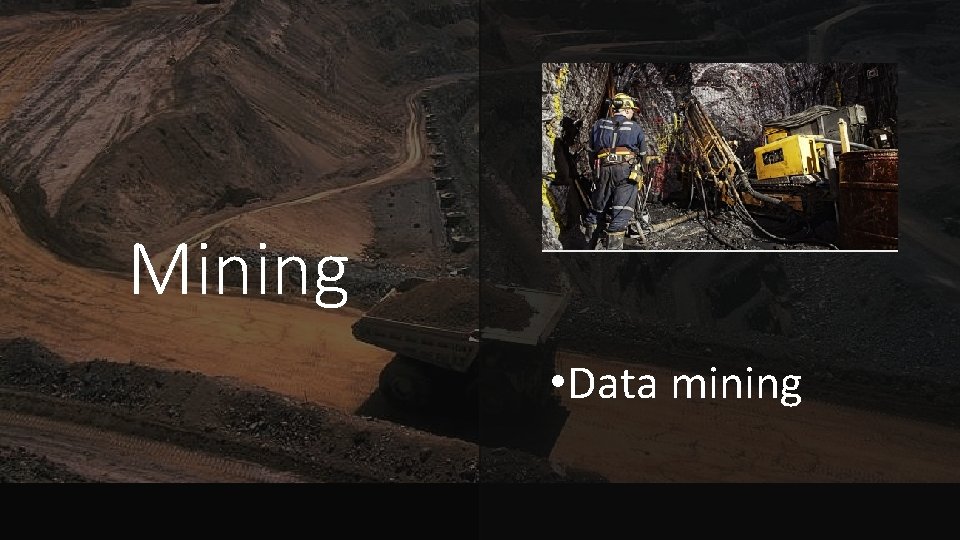
Mining • Data mining
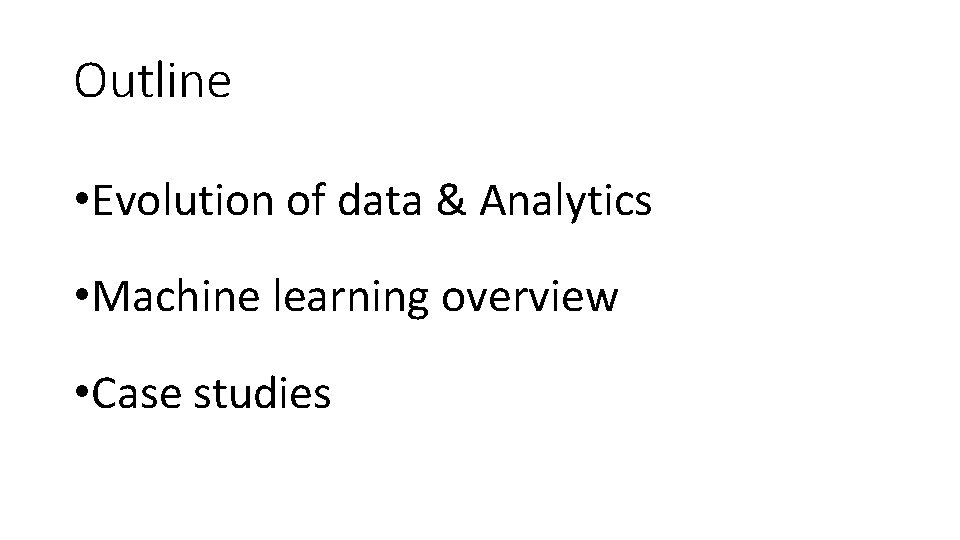
Outline • Evolution of data & Analytics • Machine learning overview • Case studies
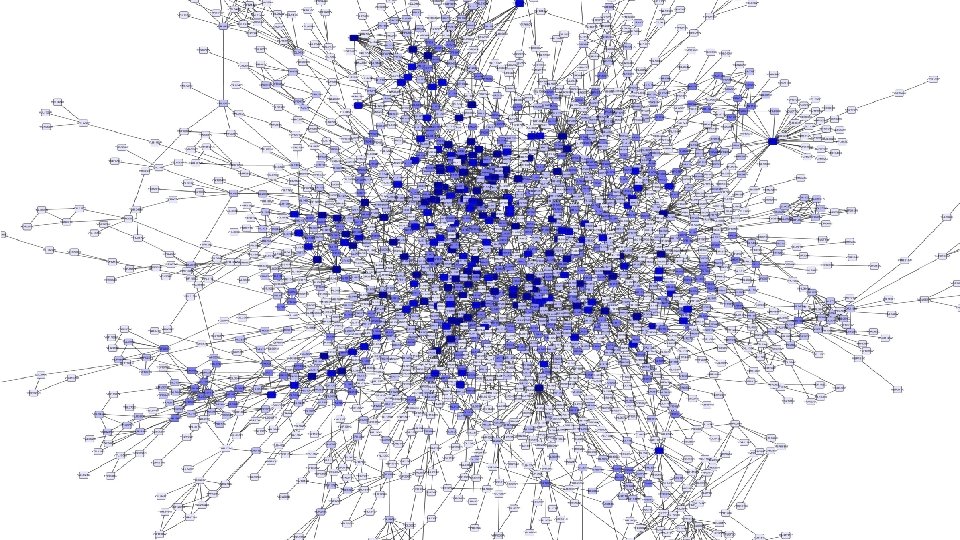
Data Evolution • Banks have data from their first day of 1770 1950 1980 -1990 2005 -2010 2012 -2018 2050
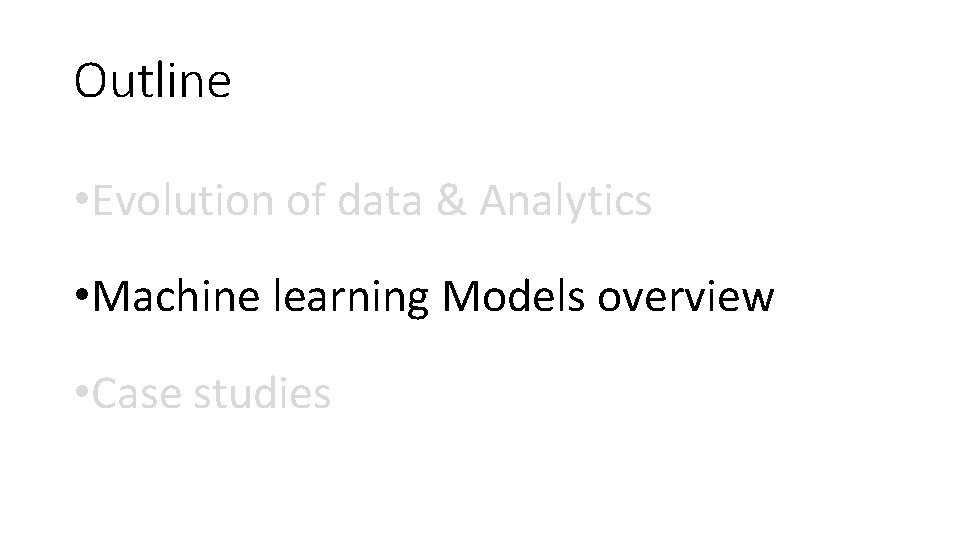
Outline • Evolution of data & Analytics • Machine learning Models overview • Case studies
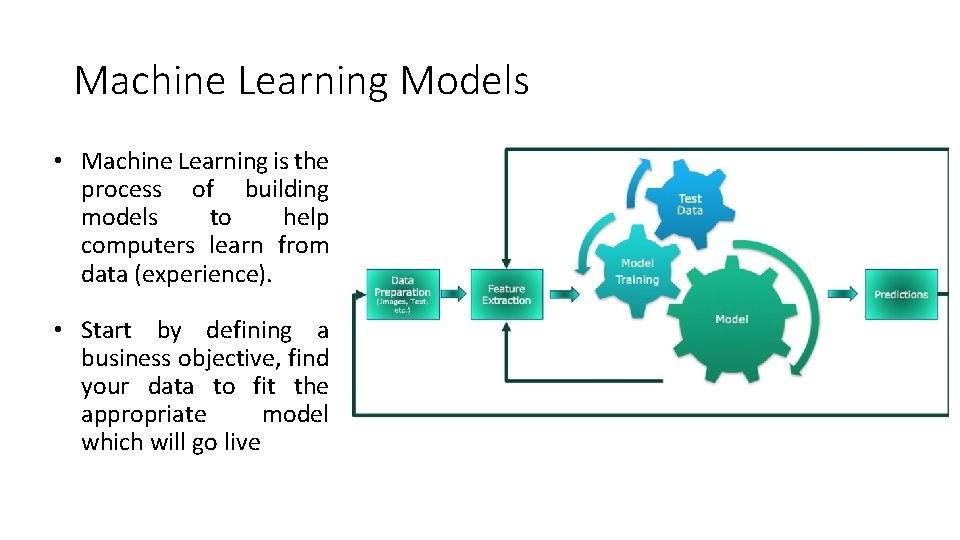
Machine Learning Models • Machine Learning is the process of building models to help computers learn from data (experience). • Start by defining a business objective, find your data to fit the appropriate model which will go live
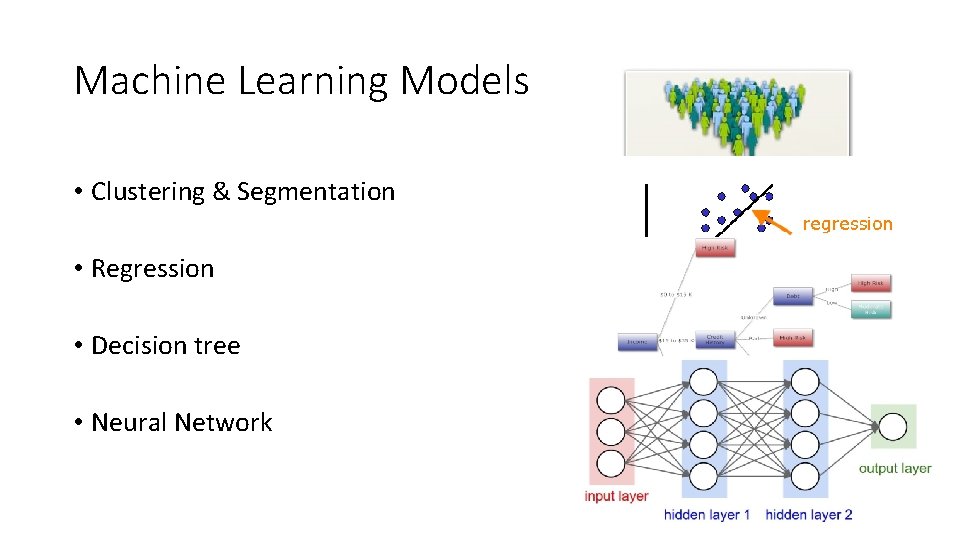
Machine Learning Models • Clustering & Segmentation • Regression • Decision tree • Neural Network
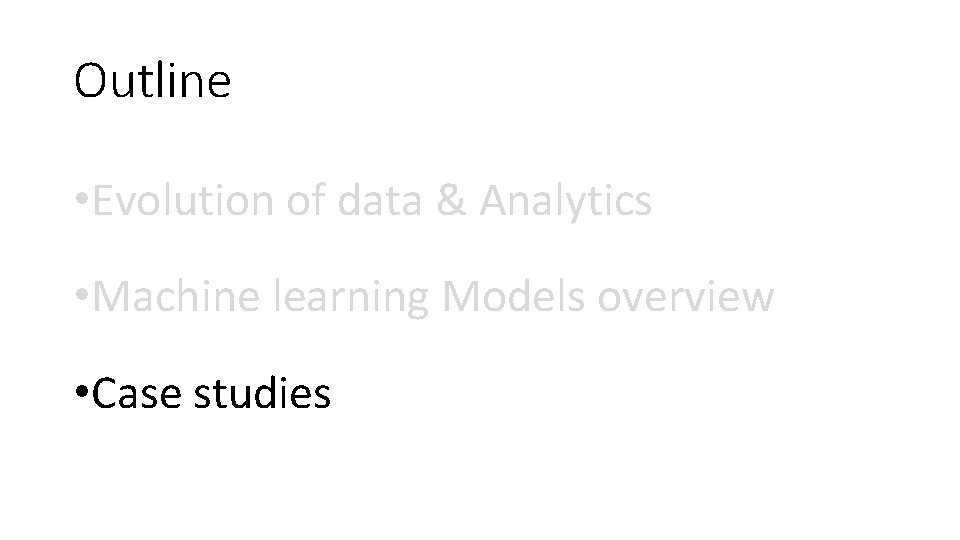
Outline • Evolution of data & Analytics • Machine learning Models overview • Case studies
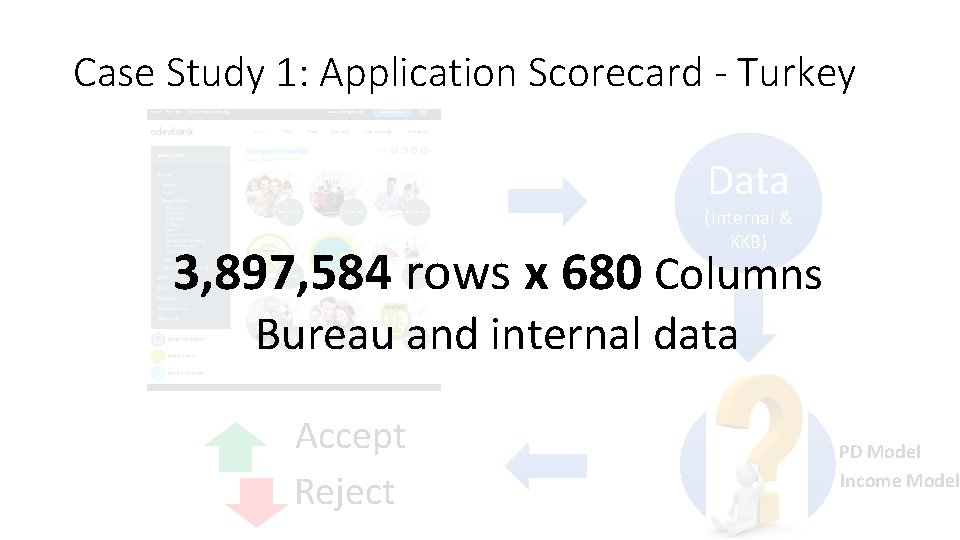
Case Study 1: Application Scorecard - Turkey Data (Internal & KKB) 3, 897, 584 rows x 680 Columns Bureau and internal data Accept Reject Expert Model PD Model Income Model
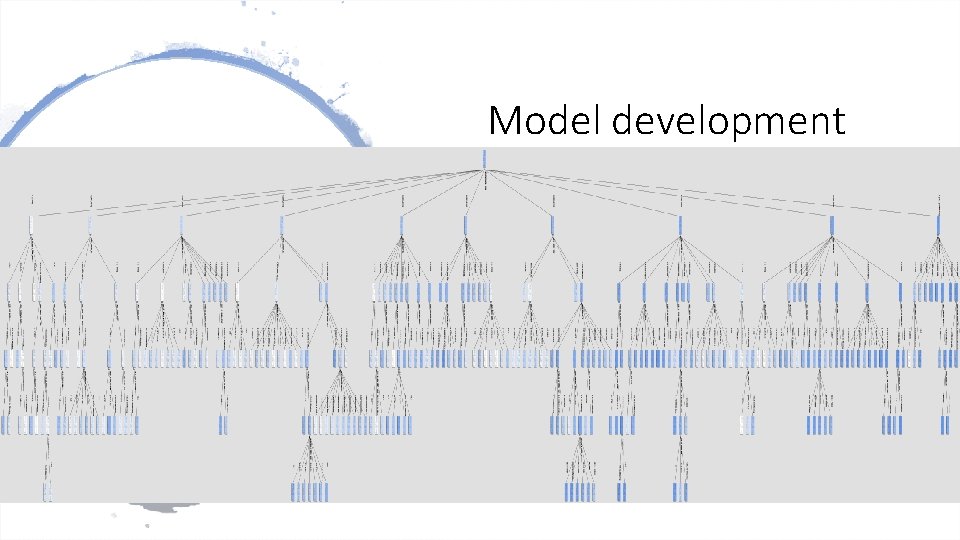
Model development • Comparison of models (logistics, neural network…etc) • Maximize the predictive power of the model
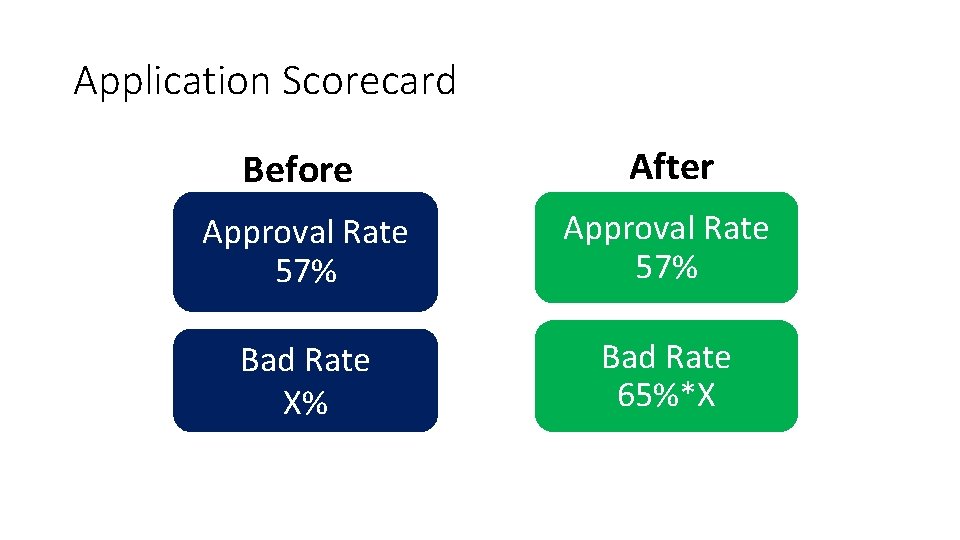
Application Scorecard Before After Approval Rate 57% Bad Rate X% Bad Rate 65%*X
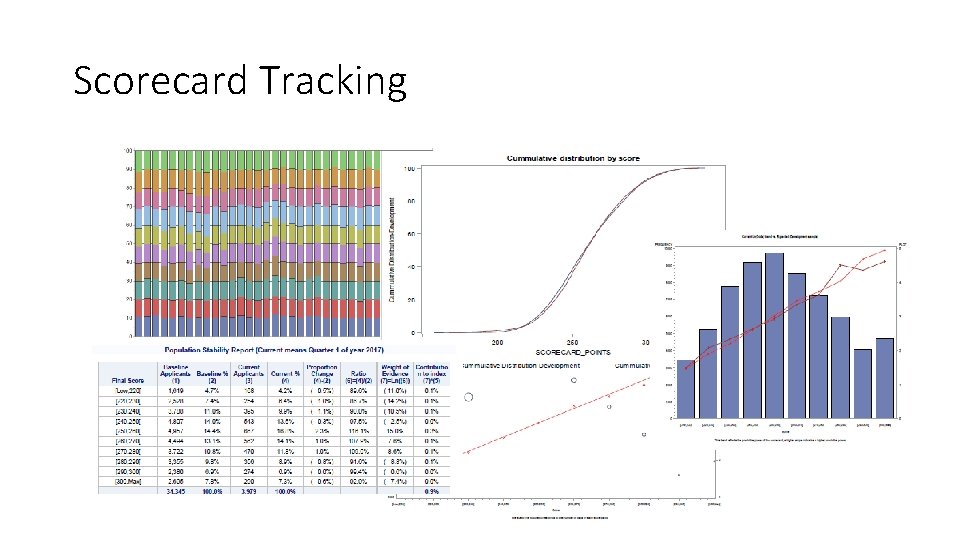
Scorecard Tracking
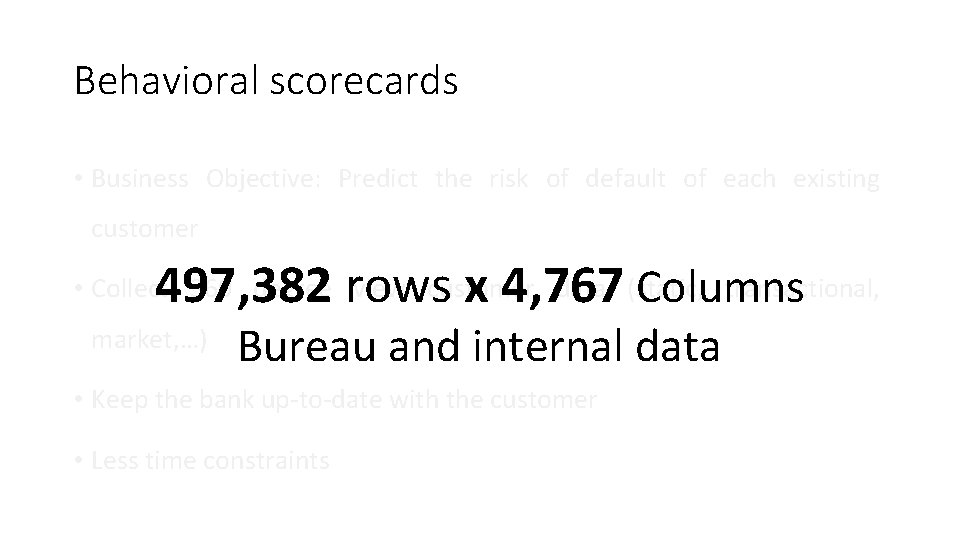
Behavioral scorecards • Business Objective: Predict the risk of default of each existing customer 497, 382 rows x 4, 767 Columns • Collect 360 degree view customer data (static, transactional, market, …) Bureau and internal data • Keep the bank up-to-date with the customer • Less time constraints
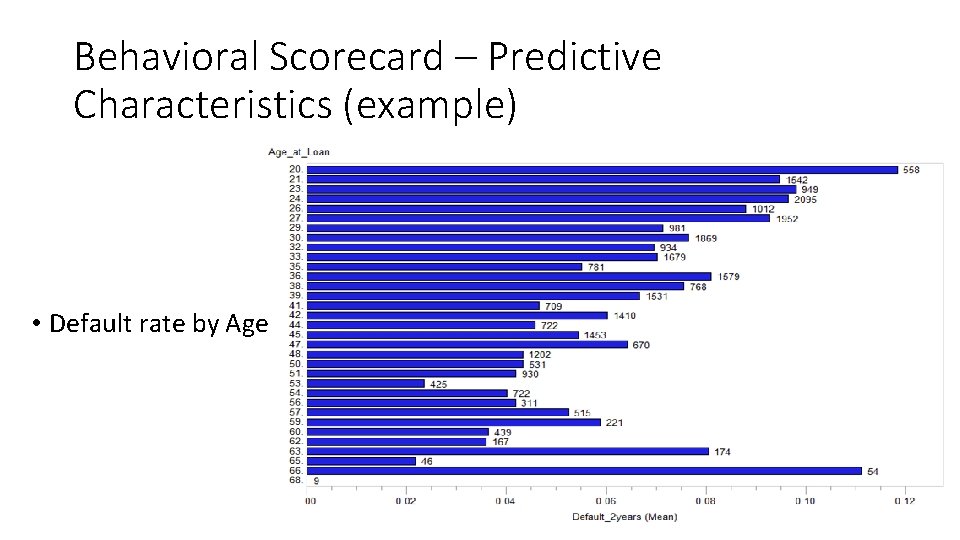
Behavioral Scorecard – Predictive Characteristics (example) • Default rate by Age
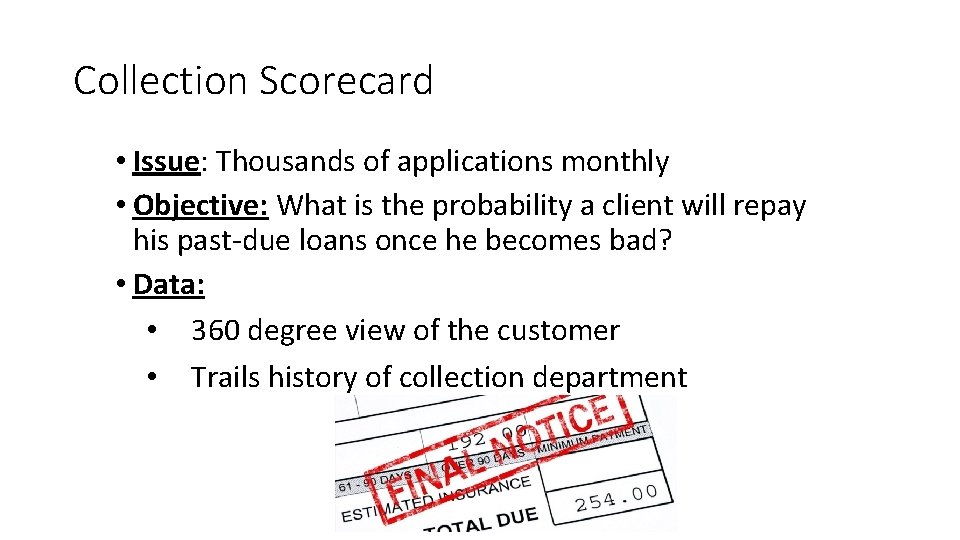
Collection Scorecard • Issue: Thousands of applications monthly • Objective: What is the probability a client will repay his past-due loans once he becomes bad? • Data: • 360 degree view of the customer • Trails history of collection department
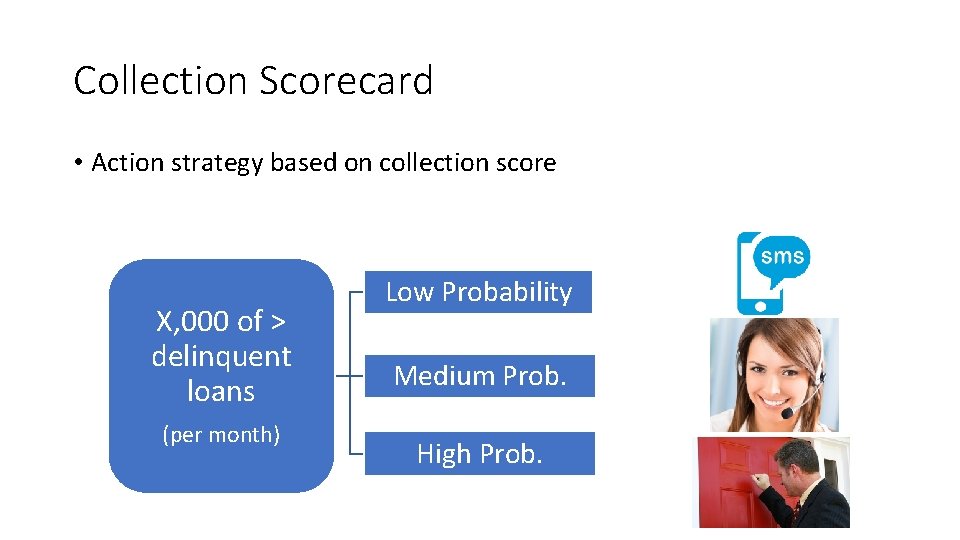
Collection Scorecard • Action strategy based on collection score X, 000 of > delinquent loans (per month) Low Probability Medium Prob. High Prob.
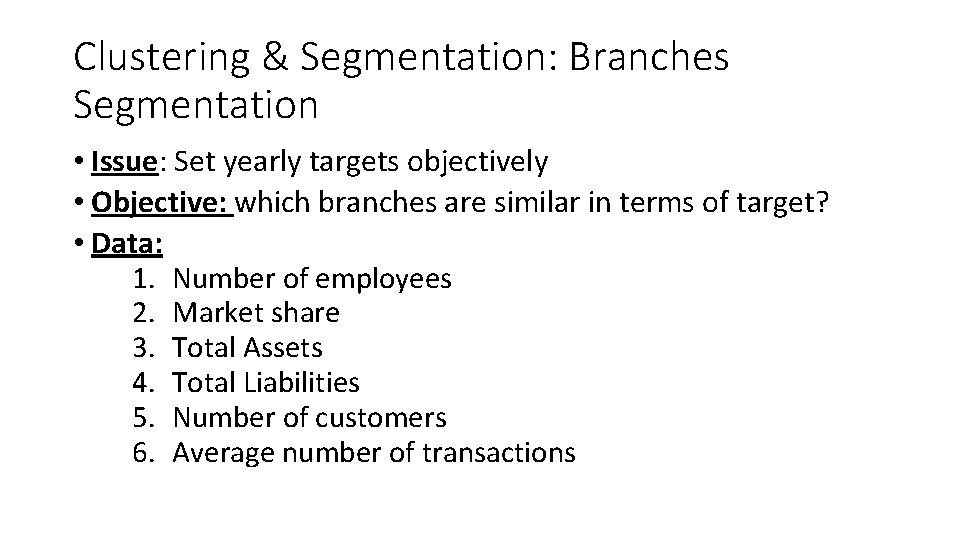
Clustering & Segmentation: Branches Segmentation • Issue: Set yearly targets objectively • Objective: which branches are similar in terms of target? • Data: 1. Number of employees 2. Market share 3. Total Assets 4. Total Liabilities 5. Number of customers 6. Average number of transactions
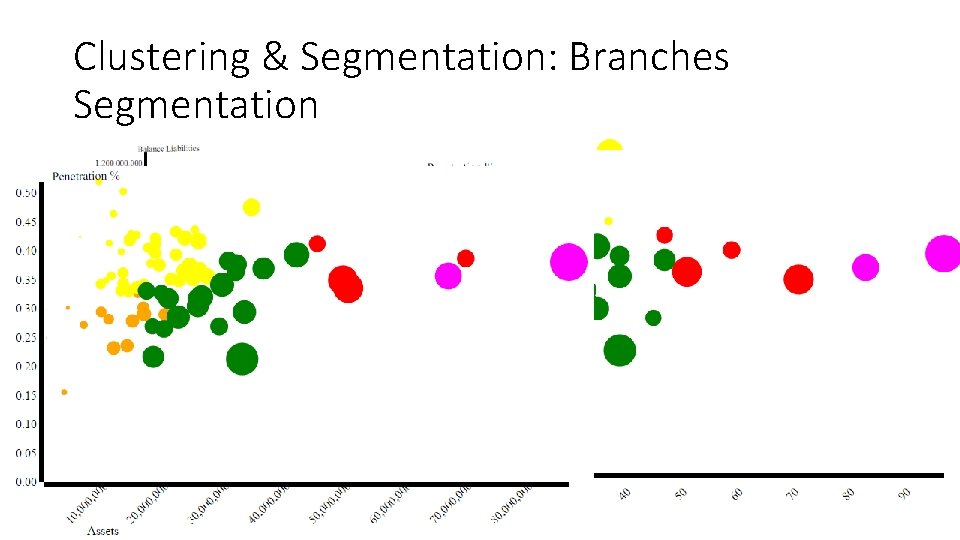
Clustering & Segmentation: Branches Segmentation
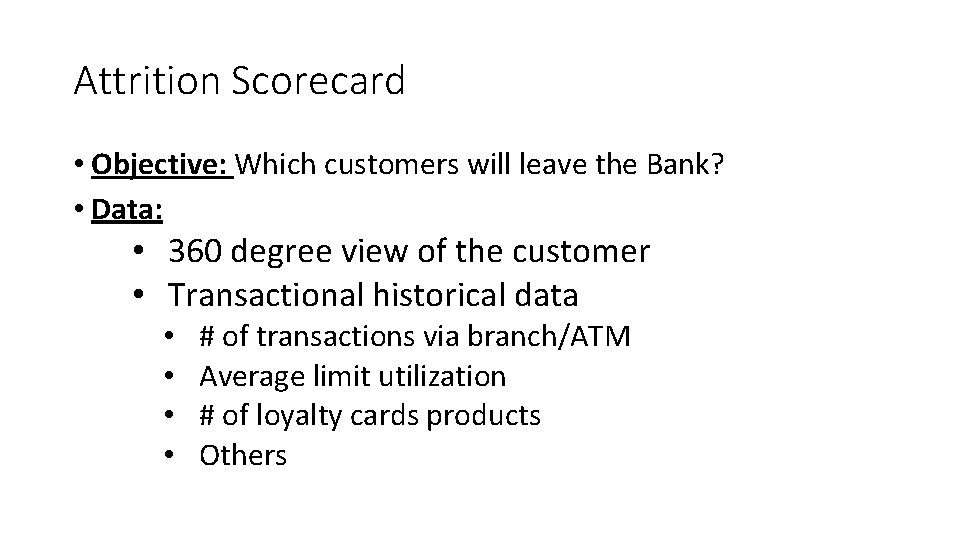
Attrition Scorecard • Objective: Which customers will leave the Bank? • Data: • 360 degree view of the customer • Transactional historical data • • # of transactions via branch/ATM Average limit utilization # of loyalty cards products Others
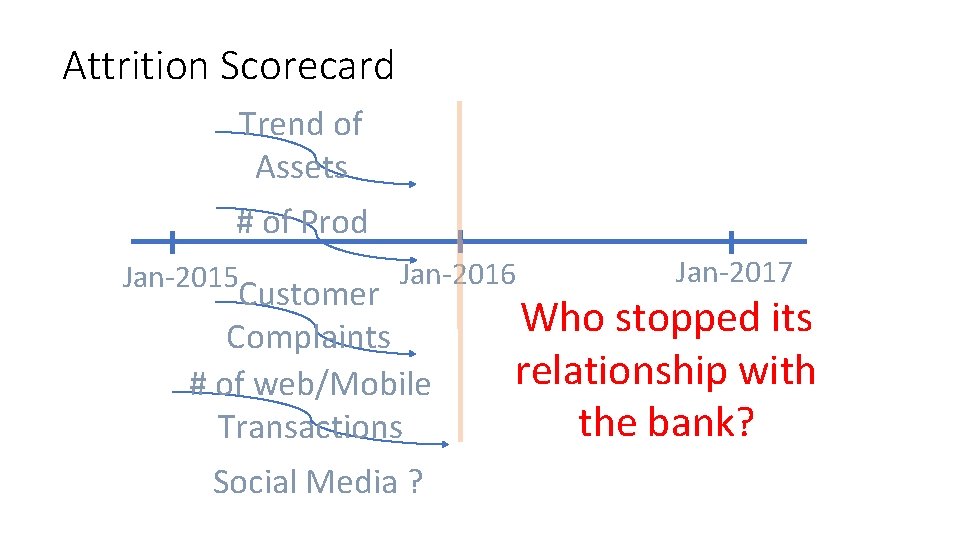
Attrition Scorecard Trend of Assets # of Prod Jan-2015 Jan-2016 Customer Complaints # of web/Mobile Transactions Social Media ? Jan-2017 Who stopped its relationship with the bank?
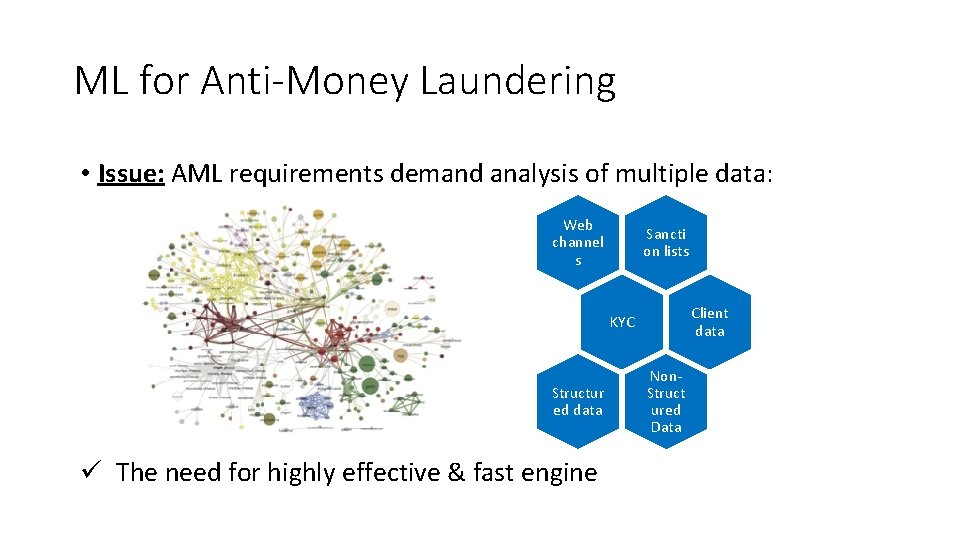
ML for Anti-Money Laundering • Issue: AML requirements demand analysis of multiple data: Web channel s Sancti on lists Client data KYC Structur ed data ü The need for highly effective & fast engine Non. Struct ured Data
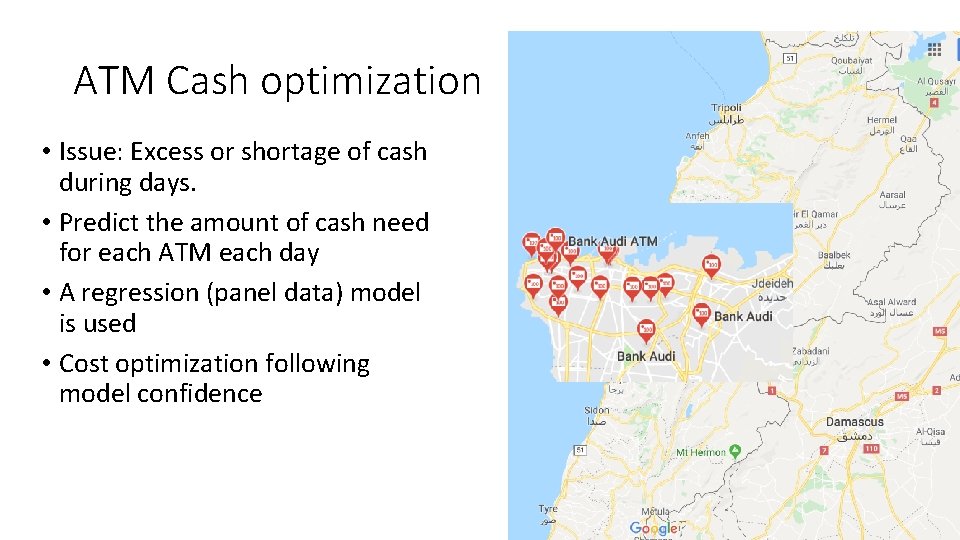
ATM Cash optimization • Issue: Excess or shortage of cash during days. • Predict the amount of cash need for each ATM each day • A regression (panel data) model is used • Cost optimization following model confidence
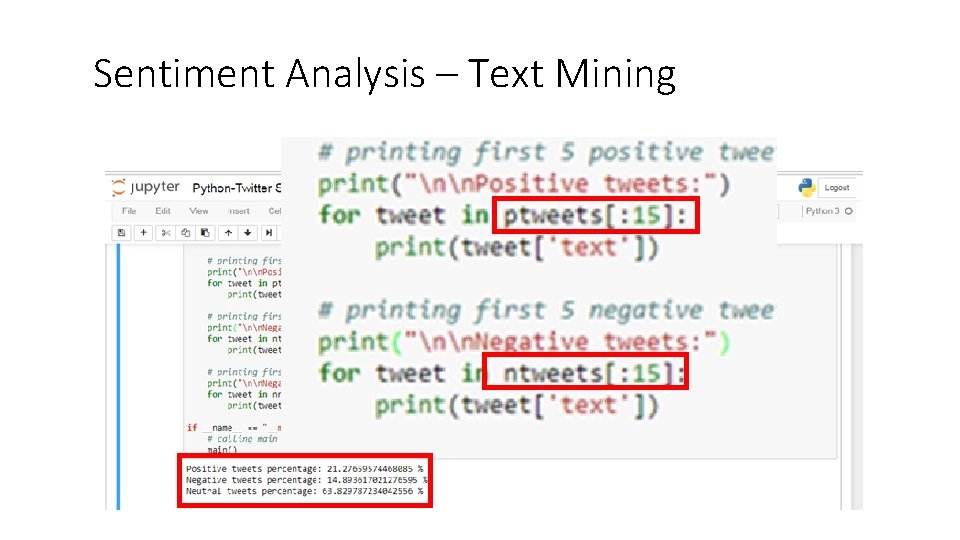
Sentiment Analysis – Text Mining • Twitter
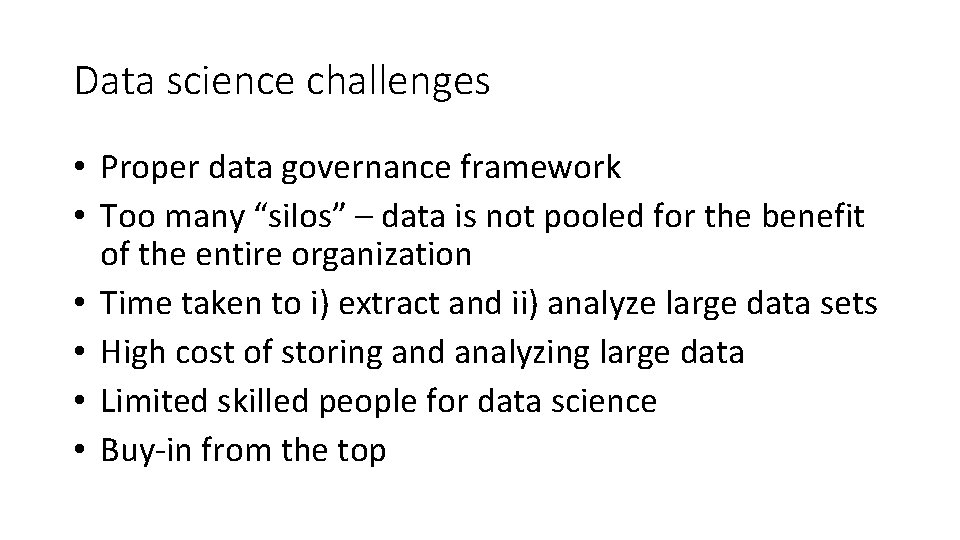
Data science challenges • Proper data governance framework • Too many “silos” – data is not pooled for the benefit of the entire organization • Time taken to i) extract and ii) analyze large data sets • High cost of storing and analyzing large data • Limited skilled people for data science • Buy-in from the top
Michel kamel
Kamel bourenane
Kamel ben-naceur
Kamel bourenane
Kamel bourenane
Dr dalia kamel
Dr sherif kamel
Kamel saleh
Kamel mellahi
Geane kamel
Sherief kamel
His favourite subject is
Elder care services westchester county
Genesee county office for the aging
Bernalillo senior center
Department of health and senior services missouri
Financial and non financial methods of motivation
Financial services technology consortium
Sfs uwaterloo
Regulatory framework of financial services
What is public issue
Personal finance module
Mass marketers such as target and venture stores
Ceb financial services
Financial services marketing environment
Financial education services compensation plan