Comparison of different statistical methods to predict Intensive
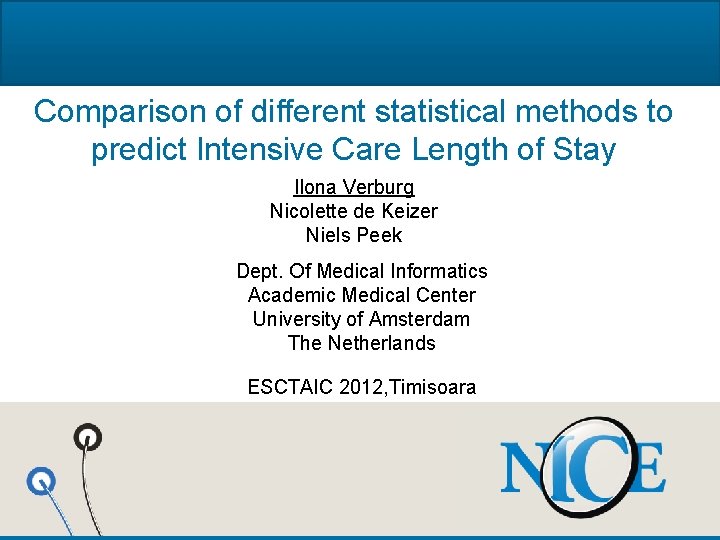
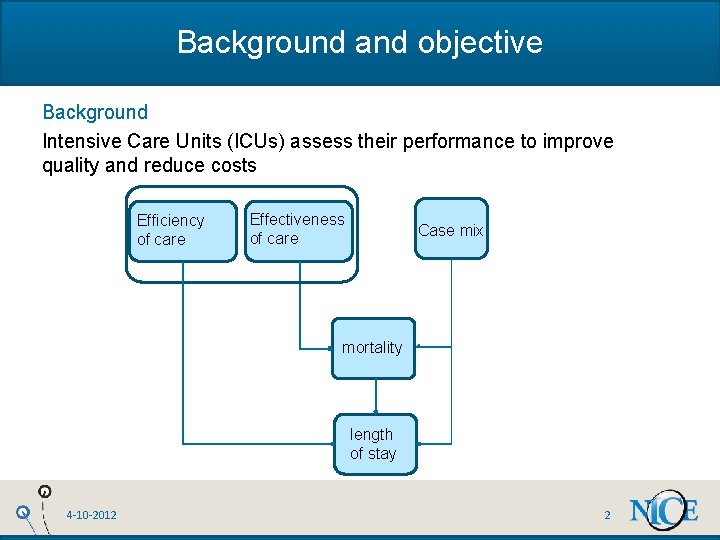
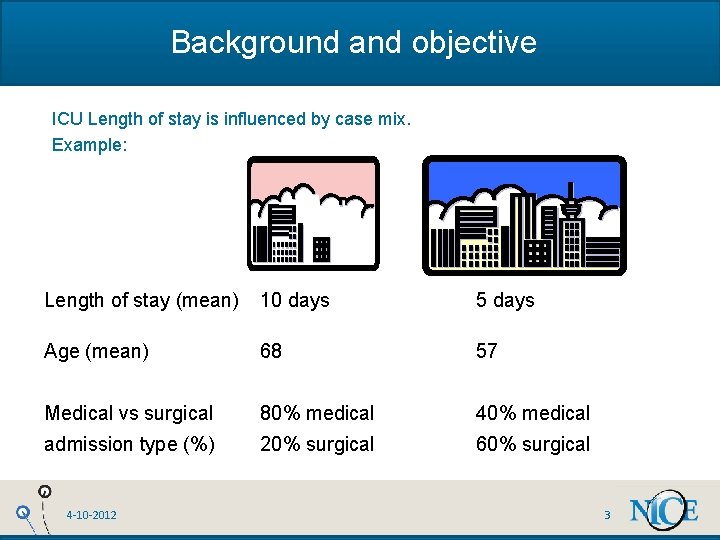
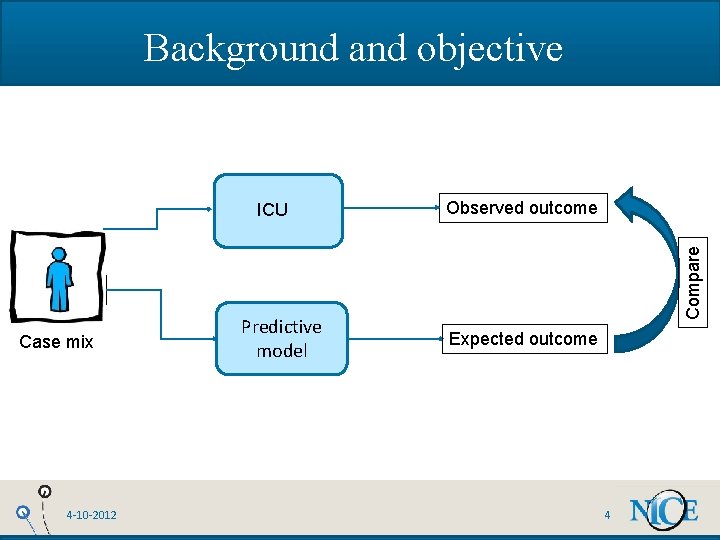
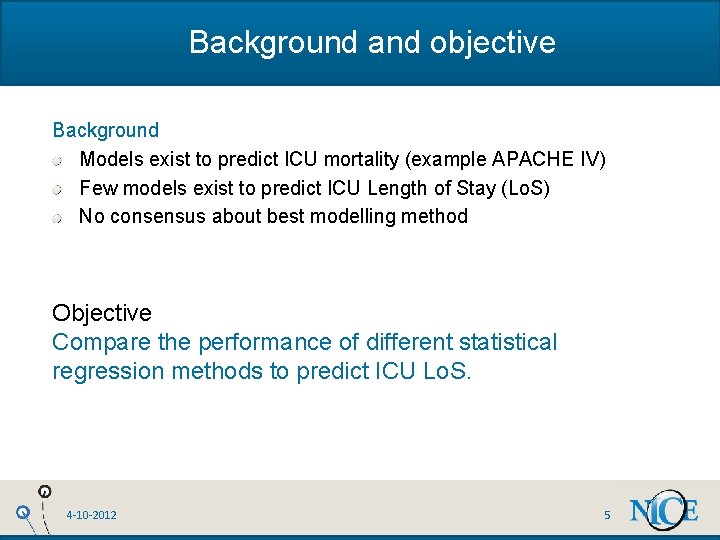
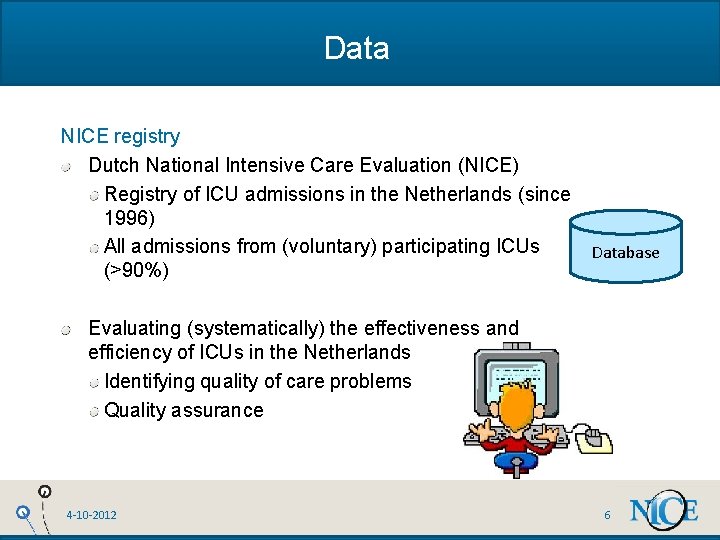
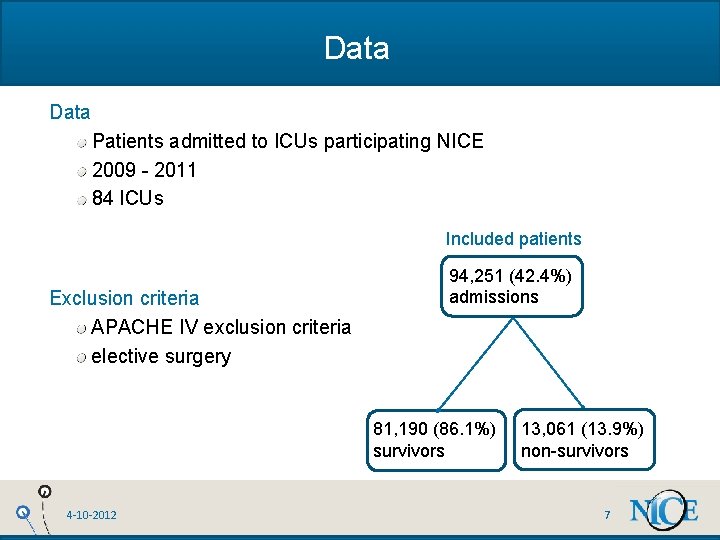
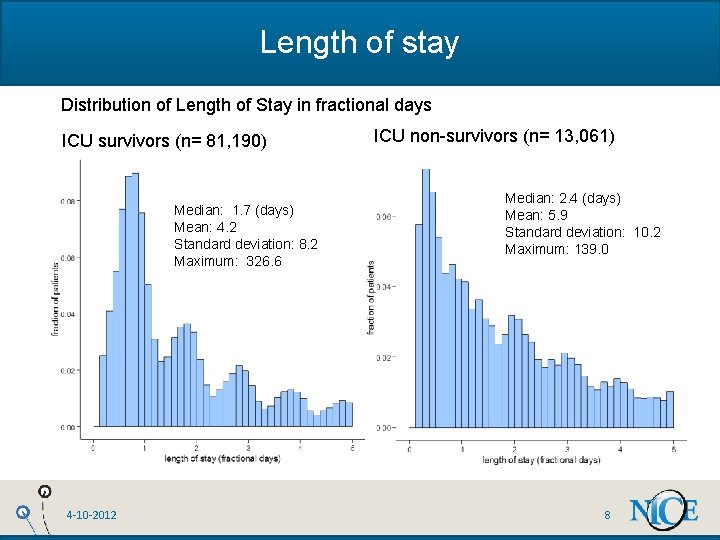
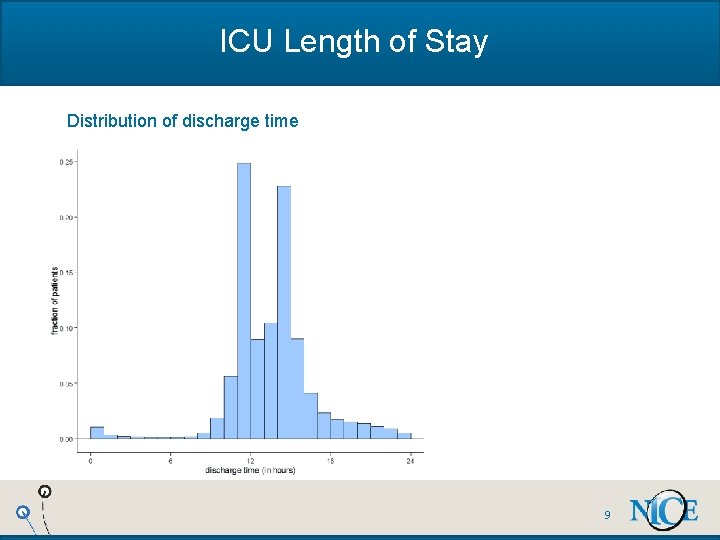
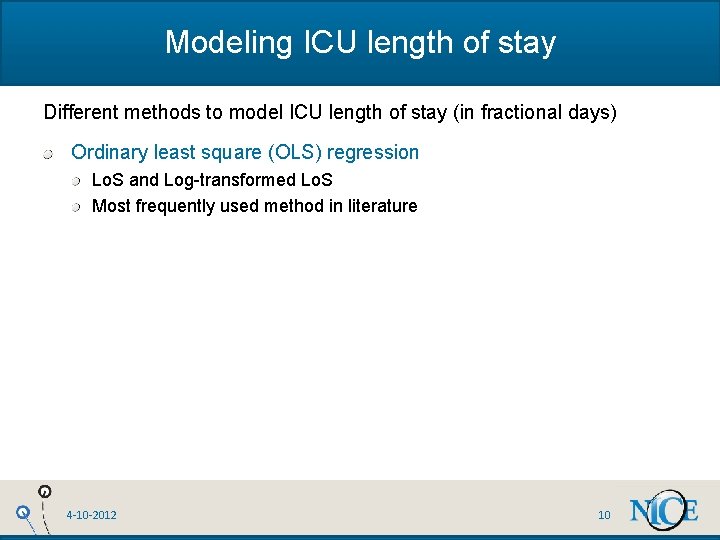
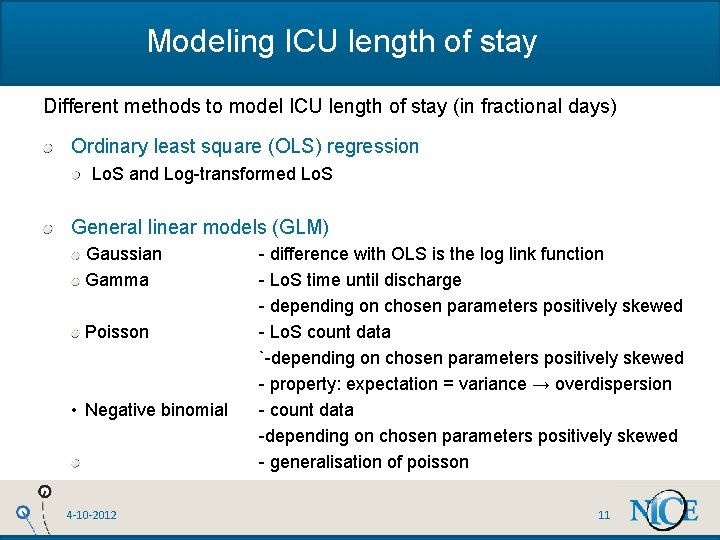
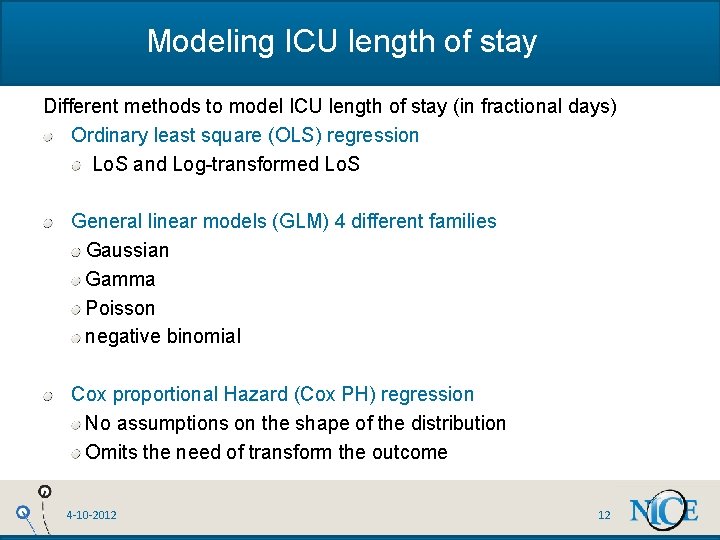
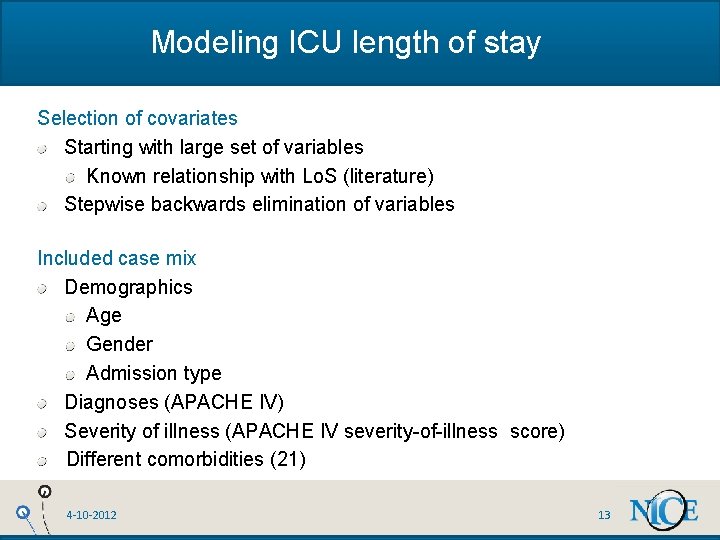
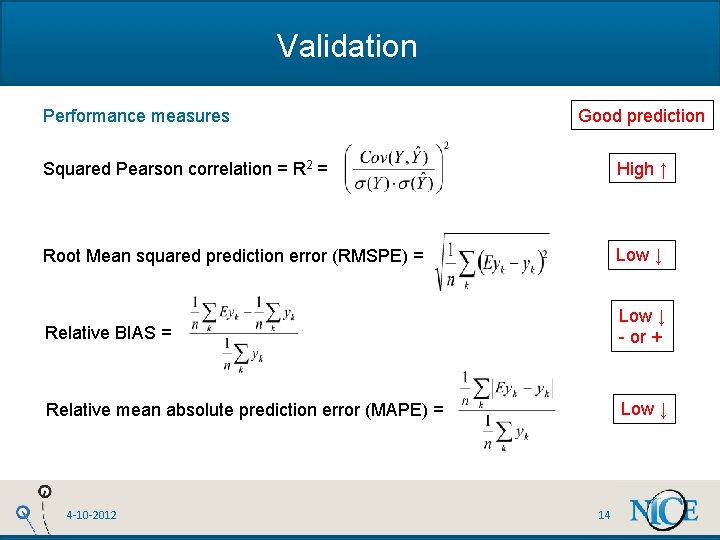
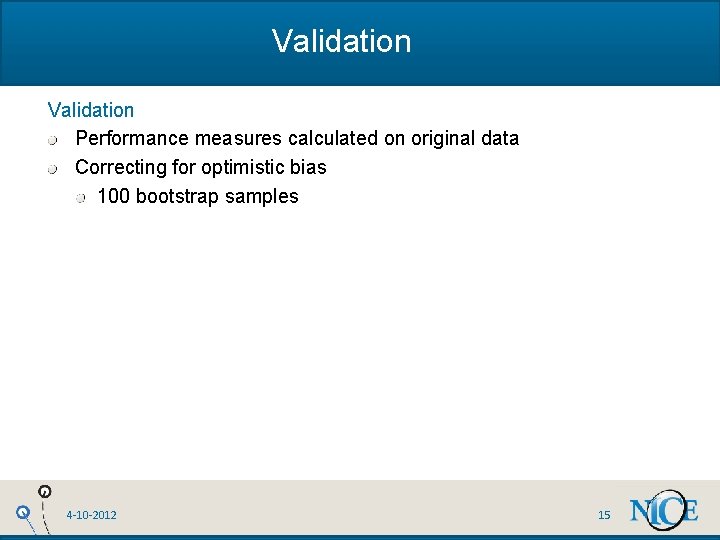
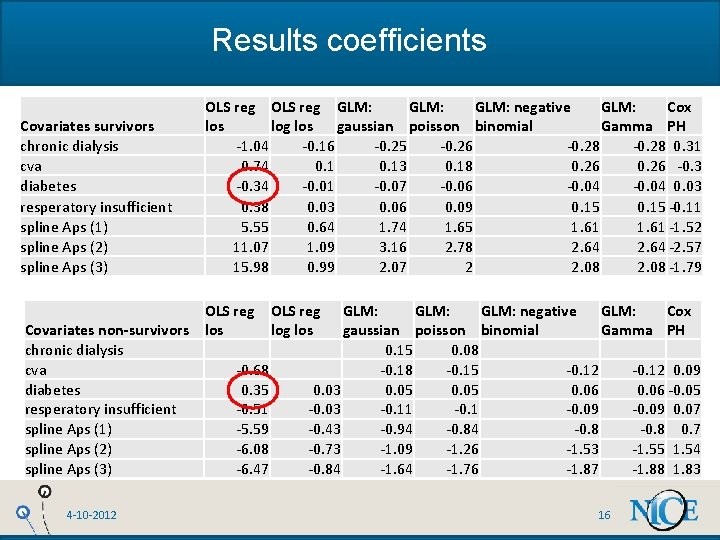
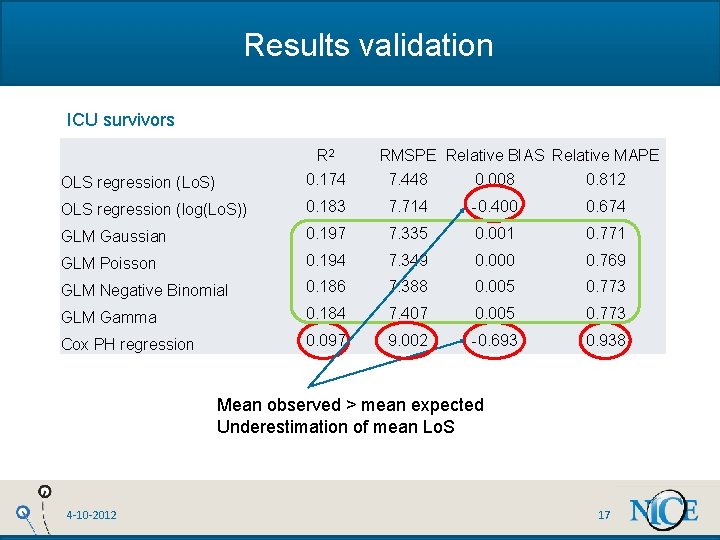
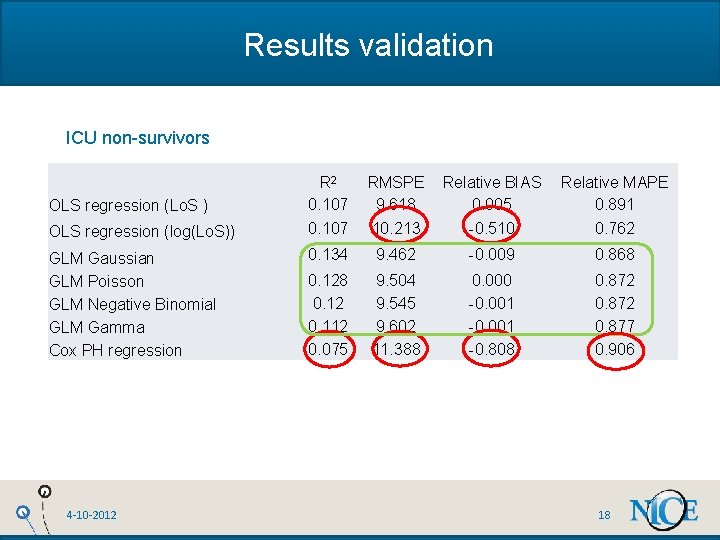
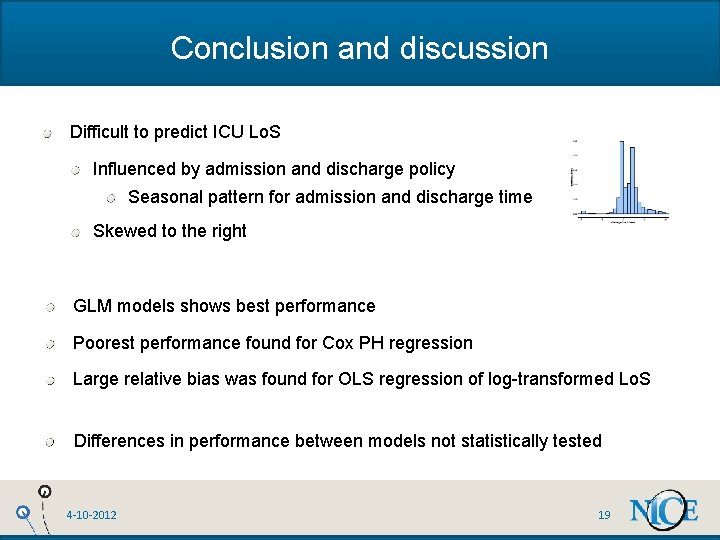
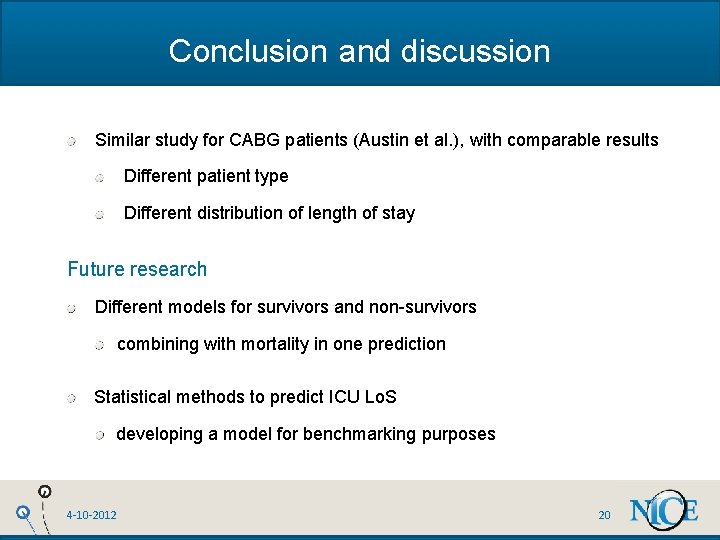
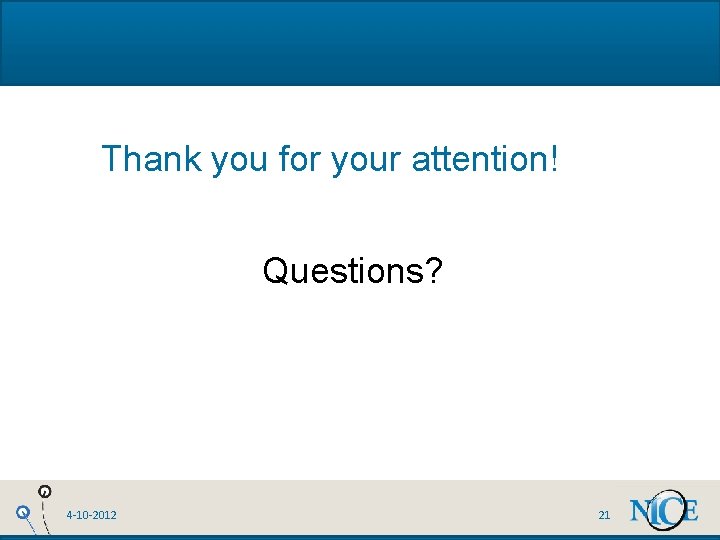
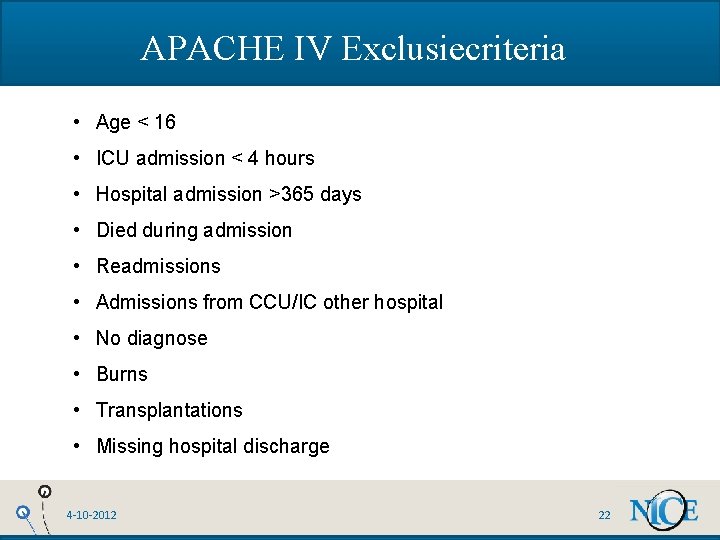
- Slides: 22
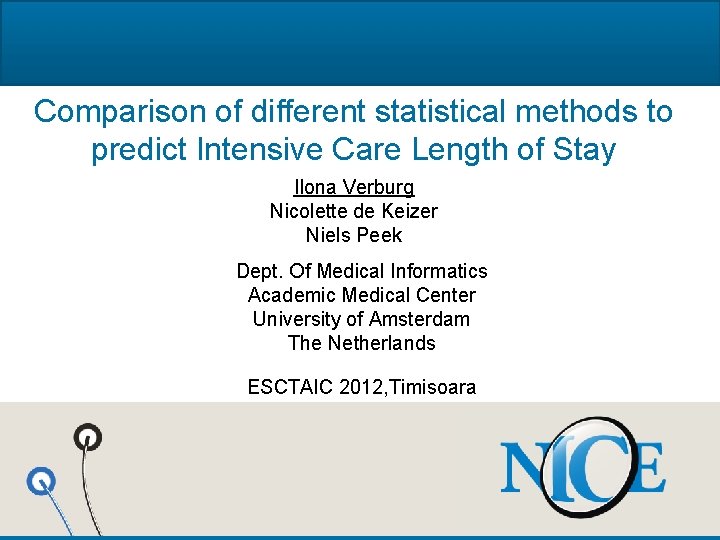
Comparison of different statistical methods to predict Intensive Care Length of Stay Ilona Verburg Nicolette de Keizer Niels Peek Dept. Of Medical Informatics Academic Medical Center University of Amsterdam The Netherlands ESCTAIC 2012, Timisoara
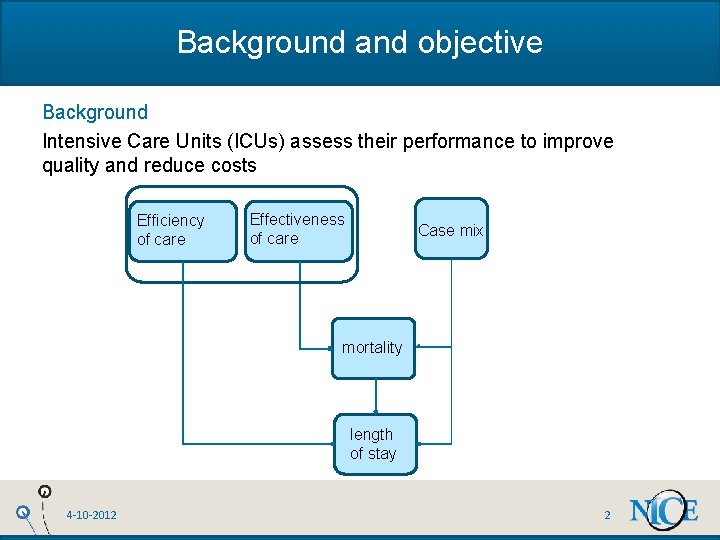
Background and objective Background Intensive Care Units (ICUs) assess their performance to improve quality and reduce costs Background Efficiency of care Effectiveness of care Case mix mortality length of stay 4 -10 -2012 2
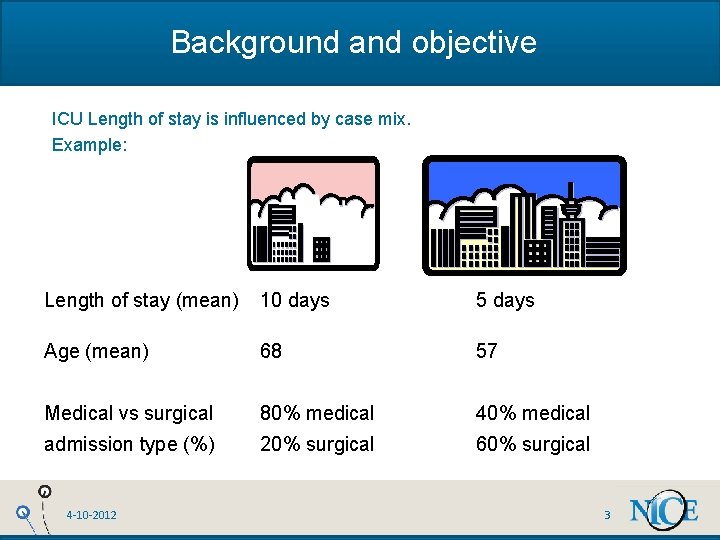
Background and objective ICU Length of stay is influenced by case mix. Example: Length of stay (mean) 10 days 5 days Age (mean) 68 57 Medical vs surgical 80% medical 40% medical admission type (%) 20% surgical 4 -10 -2012 60% surgical 3
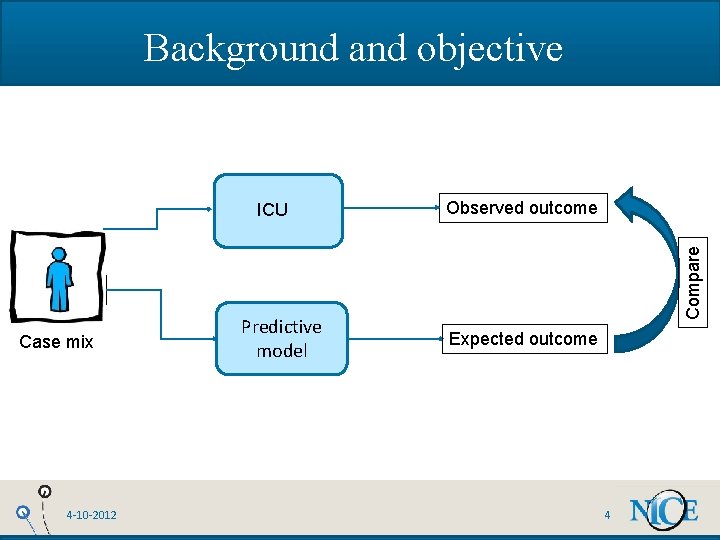
Background and objective Observed outcome Compare ICU Case mix 4 -10 -2012 Predictive model Expected outcome 4
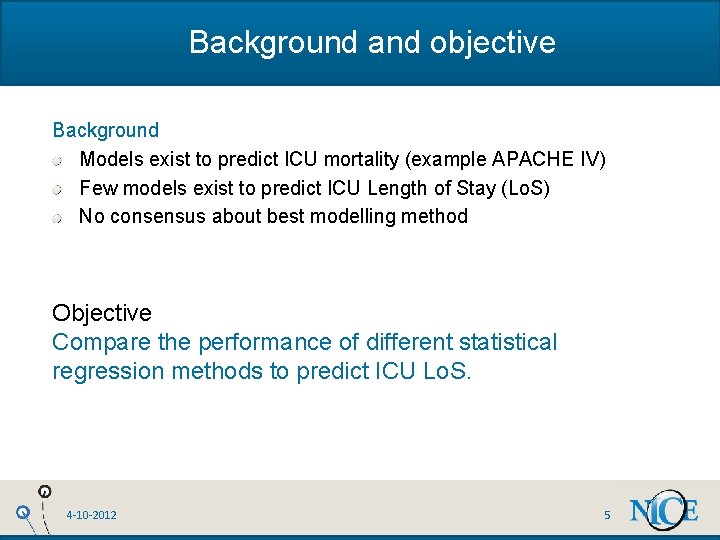
Background and objective Background Models exist to predict ICU mortality (example APACHE IV) Few models exist to predict ICU Length of Stay (Lo. S) No consensus about best modelling method Objective Compare the performance of different statistical regression methods to predict ICU Lo. S. 4 -10 -2012 5
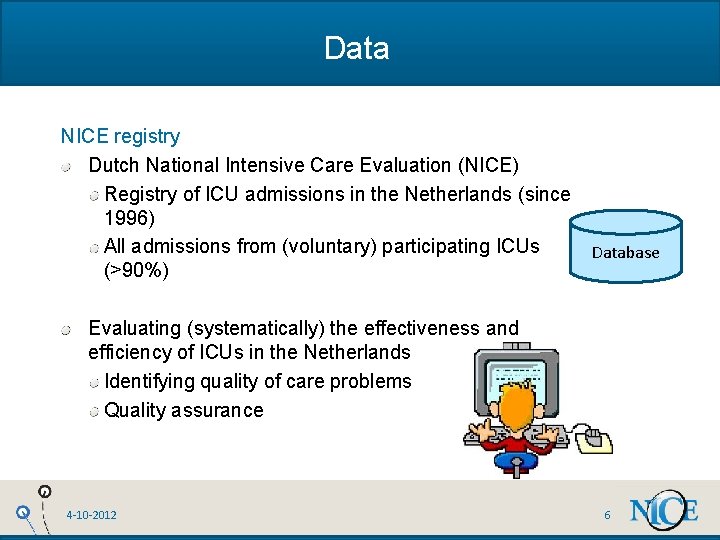
Data NICE registry Dutch National Intensive Care Evaluation (NICE) Registry of ICU admissions in the Netherlands (since 1996) All admissions from (voluntary) participating ICUs Database (>90%) Evaluating (systematically) the effectiveness and efficiency of ICUs in the Netherlands Identifying quality of care problems Quality assurance 4 -10 -2012 6
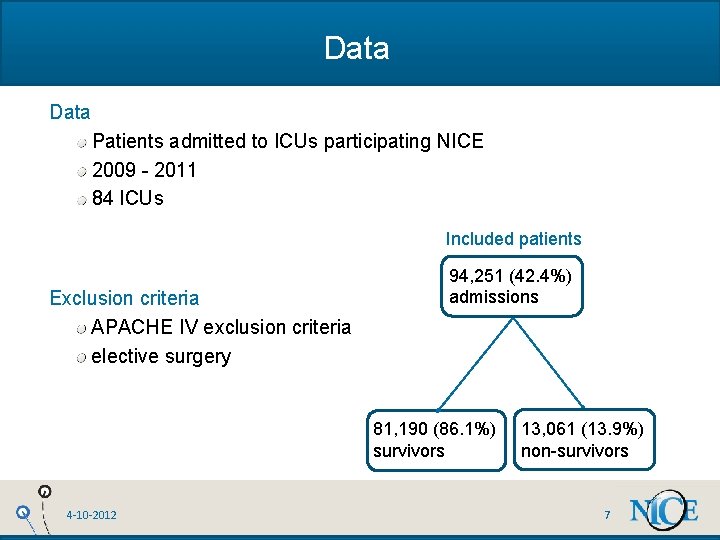
Data Patients admitted to ICUs participating NICE 2009 - 2011 84 ICUs Included patients Exclusion criteria APACHE IV exclusion criteria elective surgery 94, 251 (42. 4%) admissions 81, 190 (86. 1%) survivors 4 -10 -2012 13, 061 (13. 9%) non-survivors 7
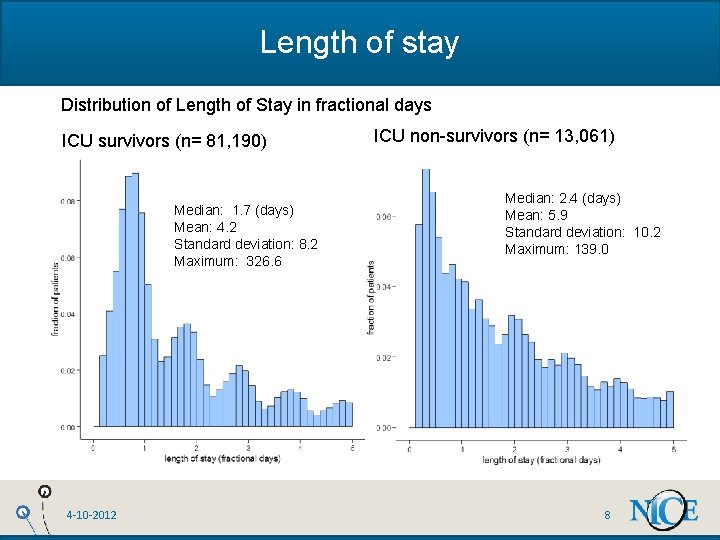
Length of stay Distribution of Length of Stay in fractional days ICU survivors (n= 81, 190) Median: 1. 7 (days) Mean: 4. 2 Standard deviation: 8. 2 Maximum: 326. 6 4 -10 -2012 ICU non-survivors (n= 13, 061) Median: 2. 4 (days) Mean: 5. 9 Standard deviation: 10. 2 Maximum: 139. 0 8
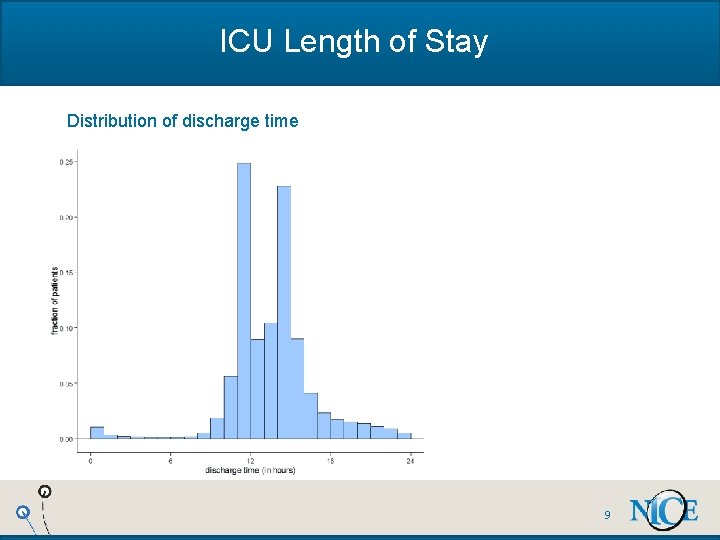
ICU Length of Stay Distribution of discharge time 9
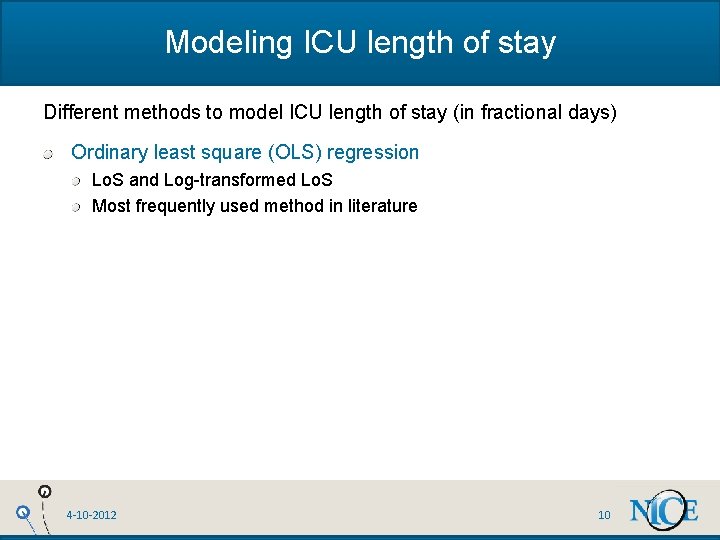
Modeling ICU length of stay Different methods to model ICU length of stay (in fractional days) Ordinary least square (OLS) regression Lo. S and Log-transformed Lo. S Most frequently used method in literature 4 -10 -2012 10
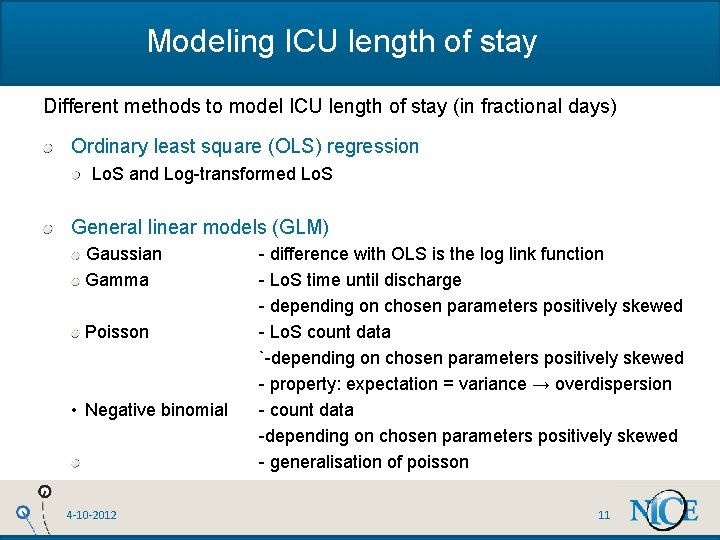
Modeling ICU length of stay Different methods to model ICU length of stay (in fractional days) Ordinary least square (OLS) regression Lo. S and Log-transformed Lo. S General linear models (GLM) Gaussian Gamma Poisson • Negative binomial 4 -10 -2012 - difference with OLS is the log link function - Lo. S time until discharge - depending on chosen parameters positively skewed - Lo. S count data `-depending on chosen parameters positively skewed - property: expectation = variance → overdispersion - count data -depending on chosen parameters positively skewed - generalisation of poisson 11
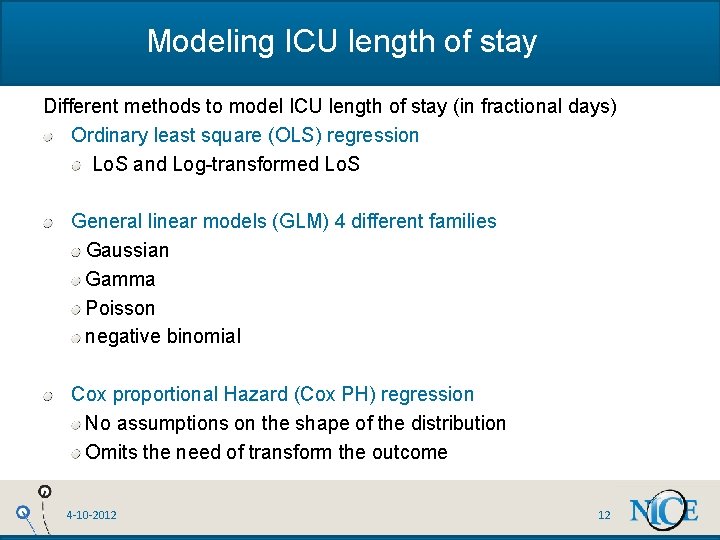
Modeling ICU length of stay Different methods to model ICU length of stay (in fractional days) Ordinary least square (OLS) regression Lo. S and Log-transformed Lo. S General linear models (GLM) 4 different families Gaussian Gamma Poisson negative binomial Cox proportional Hazard (Cox PH) regression No assumptions on the shape of the distribution Omits the need of transform the outcome 4 -10 -2012 12
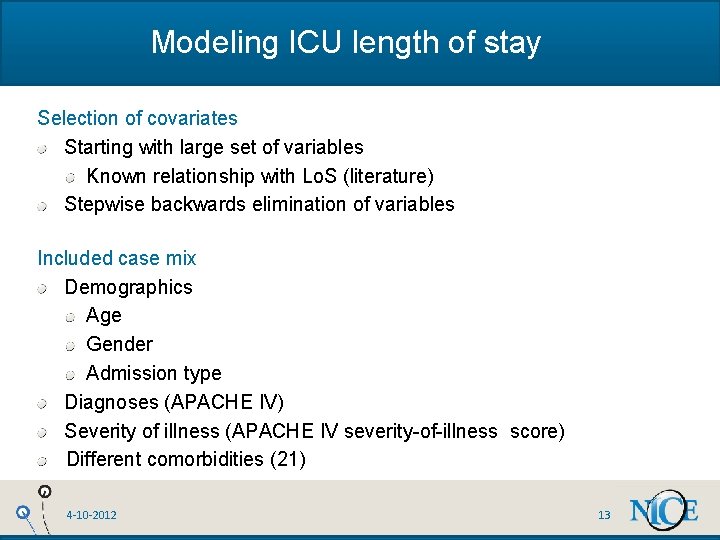
Modeling ICU length of stay Selection of covariates Starting with large set of variables Known relationship with Lo. S (literature) Stepwise backwards elimination of variables Included case mix Demographics Age Gender Admission type Diagnoses (APACHE IV) Severity of illness (APACHE IV severity-of-illness score) Different comorbidities (21) 4 -10 -2012 13
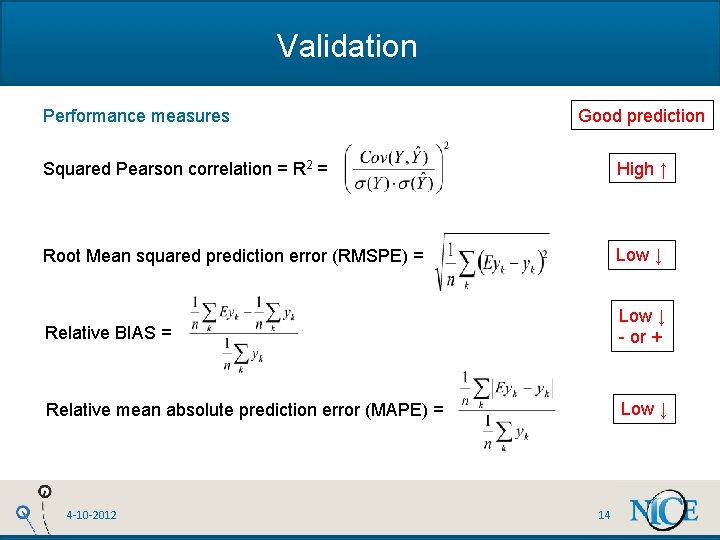
Validation Performance measures Good prediction Squared Pearson correlation = R 2 = High ↑ Root Mean squared prediction error (RMSPE) = Low ↓ Relative BIAS = Low ↓ - or + Relative mean absolute prediction error (MAPE) = Low ↓ 4 -10 -2012 14
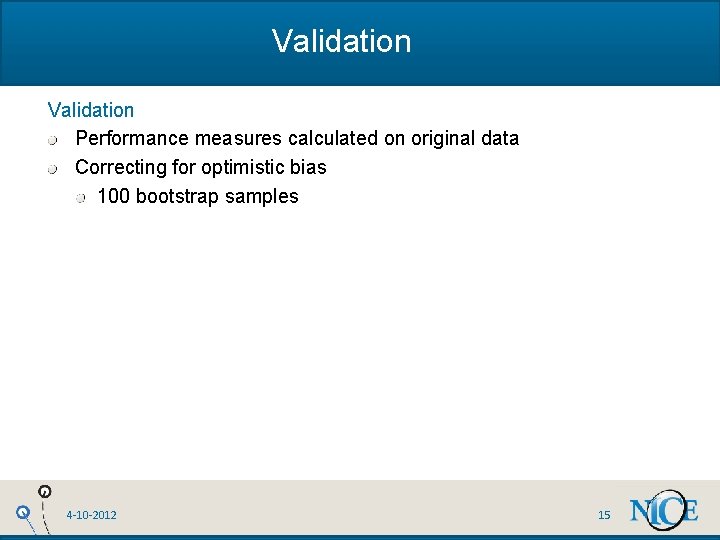
Validation Performance measures calculated on original data Correcting for optimistic bias 100 bootstrap samples 4 -10 -2012 15
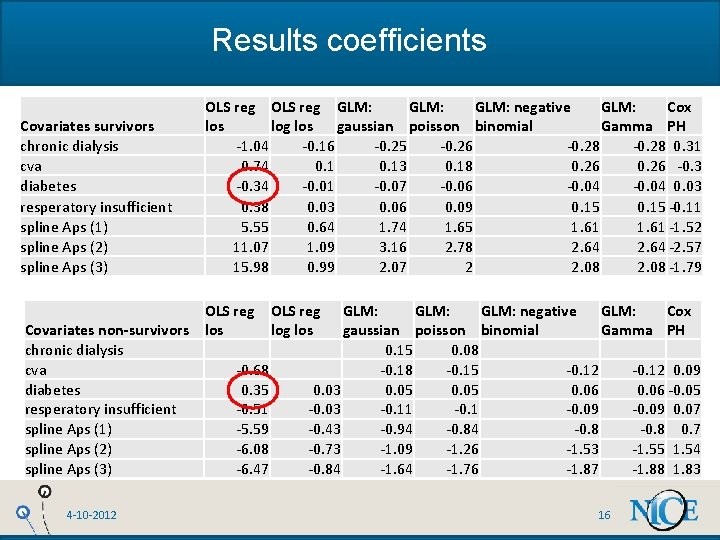
Results coefficients Covariates survivors chronic dialysis cva diabetes resperatory insufficient spline Aps (1) spline Aps (2) spline Aps (3) OLS reg GLM: negative GLM: Cox los log los gaussian poisson binomial Gamma PH -1. 04 -0. 16 -0. 25 -0. 26 -0. 28 0. 31 0. 74 0. 13 0. 18 0. 26 -0. 34 -0. 01 -0. 07 -0. 06 -0. 04 0. 03 0. 38 0. 03 0. 06 0. 09 0. 15 -0. 11 5. 55 0. 64 1. 74 1. 65 1. 61 -1. 52 11. 07 1. 09 3. 16 2. 78 2. 64 -2. 57 15. 98 0. 99 2. 07 2 2. 08 -1. 79 OLS reg GLM: negative GLM: Cox Covariates non-survivors log los gaussian poisson binomial Gamma PH chronic dialysis 0. 15 0. 08 cva -0. 68 -0. 15 -0. 12 0. 09 diabetes 0. 35 0. 03 0. 05 0. 06 -0. 05 resperatory insufficient -0. 51 -0. 03 -0. 11 -0. 09 0. 07 spline Aps (1) -5. 59 -0. 43 -0. 94 -0. 8 0. 7 spline Aps (2) -6. 08 -0. 73 -1. 09 -1. 26 -1. 53 -1. 55 1. 54 spline Aps (3) -6. 47 -0. 84 -1. 64 -1. 76 -1. 87 -1. 88 1. 83 4 -10 -2012 16
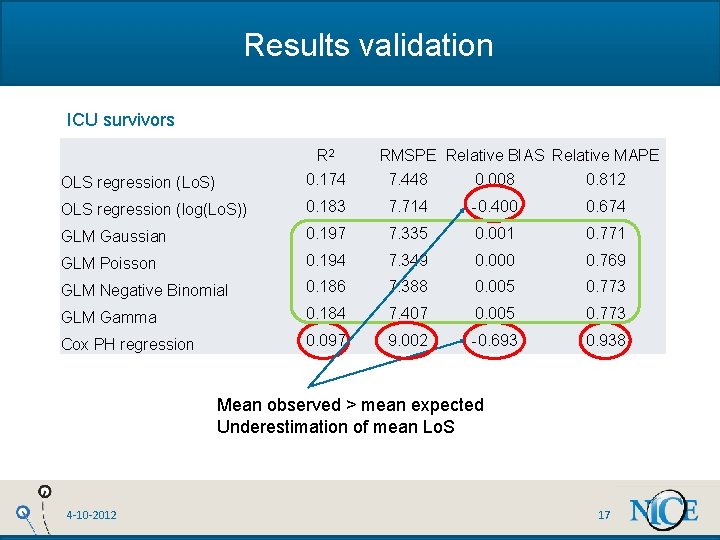
Results validation ICU survivors OLS regression (Lo. S) R 2 0. 174 RMSPE Relative BIAS Relative MAPE 7. 448 0. 008 0. 812 OLS regression (log(Lo. S)) 0. 183 7. 714 -0. 400 0. 674 GLM Gaussian 0. 197 7. 335 0. 001 0. 771 GLM Poisson 0. 194 7. 349 0. 000 0. 769 GLM Negative Binomial 0. 186 7. 388 0. 005 0. 773 GLM Gamma 0. 184 7. 407 0. 005 0. 773 Cox PH regression 0. 097 9. 002 -0. 693 0. 938 Mean observed > mean expected Underestimation of mean Lo. S 4 -10 -2012 17
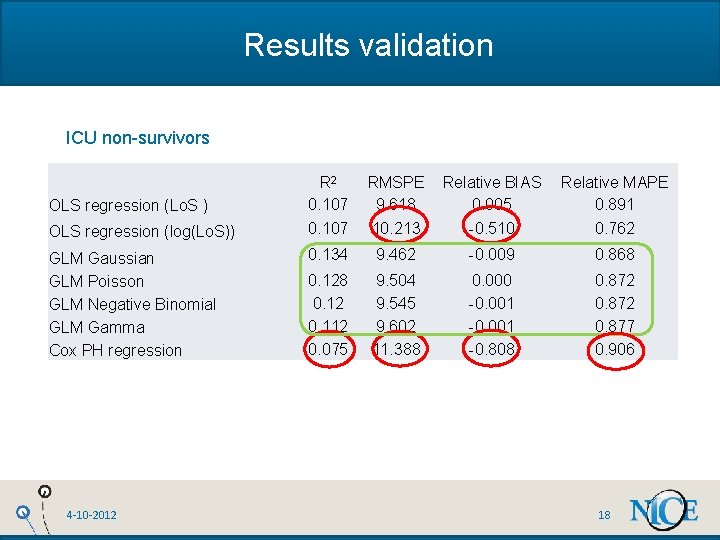
Results validation ICU non-survivors OLS regression (Lo. S ) R 2 0. 107 RMSPE 9. 618 Relative BIAS 0. 005 Relative MAPE 0. 891 OLS regression (log(Lo. S)) 0. 107 10. 213 -0. 510 0. 762 GLM Gaussian GLM Poisson GLM Negative Binomial GLM Gamma Cox PH regression 0. 134 9. 462 -0. 009 0. 868 0. 12 0. 112 0. 075 9. 504 9. 545 9. 602 11. 388 0. 000 -0. 001 -0. 808 0. 872 0. 877 0. 906 4 -10 -2012 18
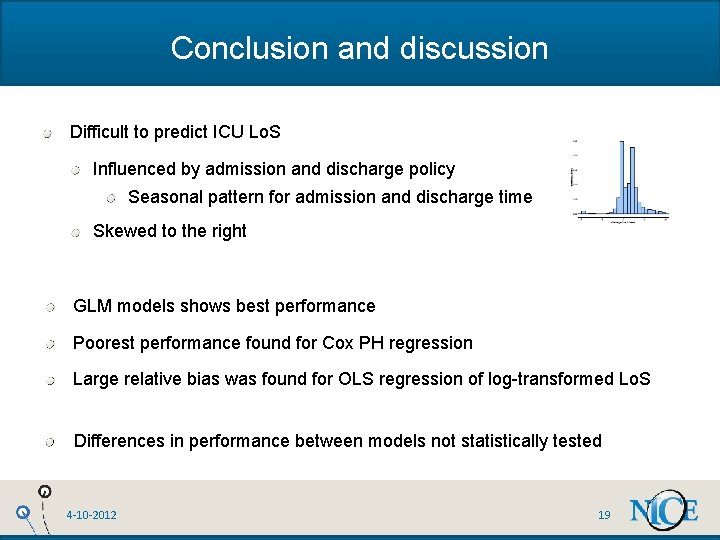
Conclusion and discussion Difficult to predict ICU Lo. S Influenced by admission and discharge policy Seasonal pattern for admission and discharge time Skewed to the right GLM models shows best performance Poorest performance found for Cox PH regression Large relative bias was found for OLS regression of log-transformed Lo. S Differences in performance between models not statistically tested 4 -10 -2012 19
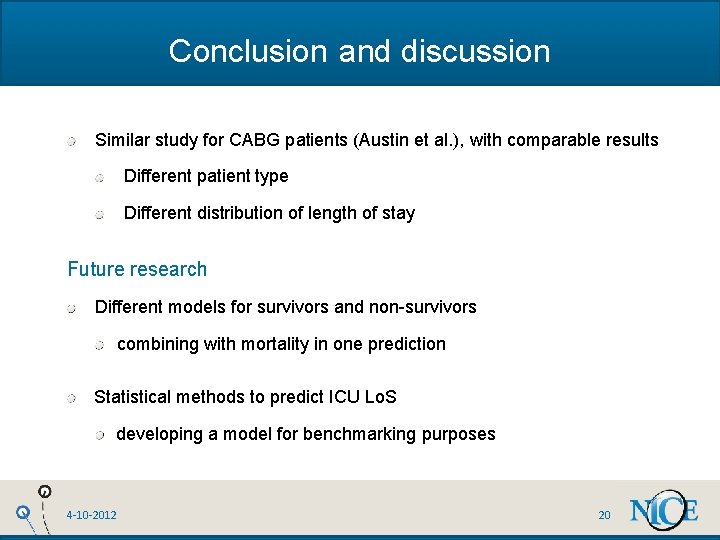
Conclusion and discussion Similar study for CABG patients (Austin et al. ), with comparable results Different patient type Different distribution of length of stay Future research Different models for survivors and non-survivors combining with mortality in one prediction Statistical methods to predict ICU Lo. S developing a model for benchmarking purposes 4 -10 -2012 20
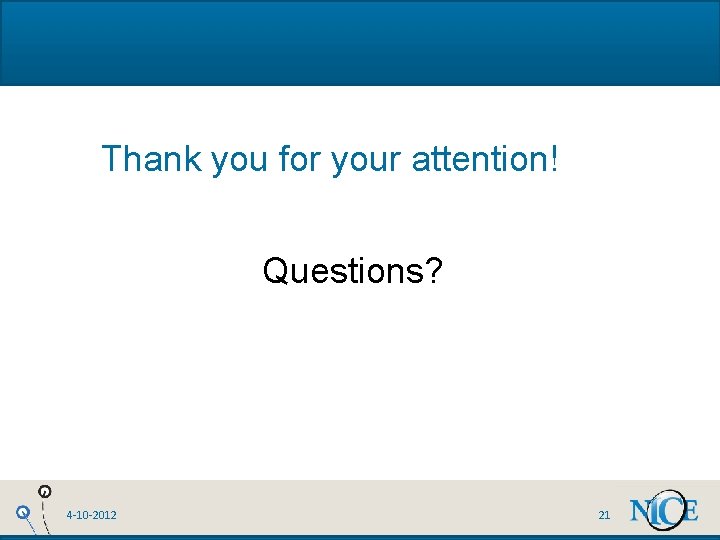
Thank you for your attention! Questions? 4 -10 -2012 21
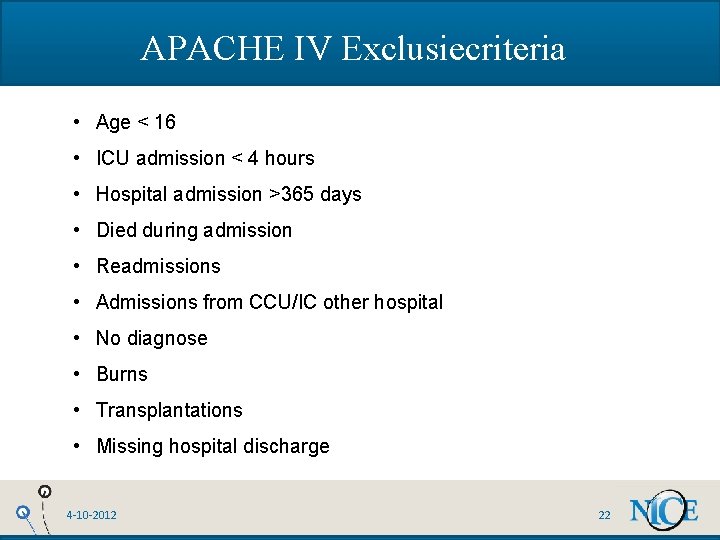
APACHE IV Exclusiecriteria • Age < 16 • ICU admission < 4 hours • Hospital admission >365 days • Died during admission • Readmissions • Admissions from CCU/IC other hospital • No diagnose • Burns • Transplantations • Missing hospital discharge 4 -10 -2012 22
Statistical methods of demand forecasting
Statistical methods of demand forecasting
Two branches of statistics are
Advanced and multivariate statistical methods
Limit comparision test
Direct wax pattern technique
Present progressive tense examples
Advantages and disadvantages of glucose oxidase method
Advantages of size reduction is
Different types of accounting methods
Methods of personal identification
Friedeich
Types of errors in numerical computation
An example of crude drug adulterated with exhausted drug
Different methods of separating mixtures
Approach in teaching
What are the methods of acquiring knowledge
Anatomy of a bullet
Recall the different methods of factoring polynomials
Principles of fish preservation
Presenting information in different ways
Dft perform filtering operation in
Vsepr