Applications of epigenetics Epigenomics Notes Genomics as a
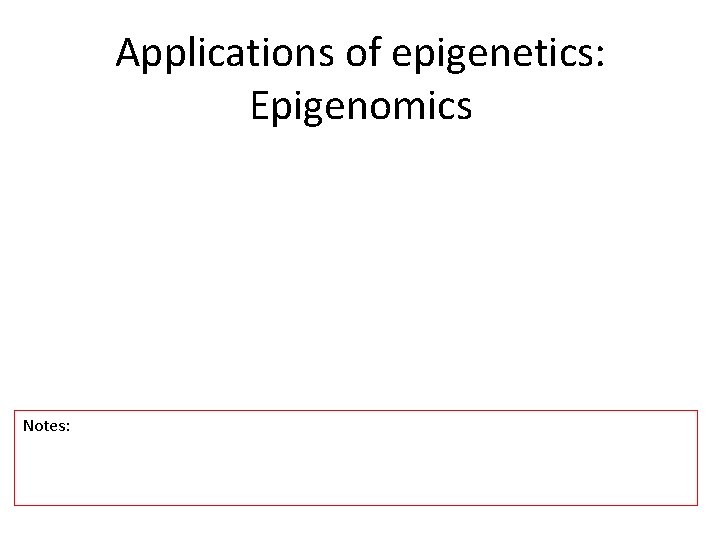
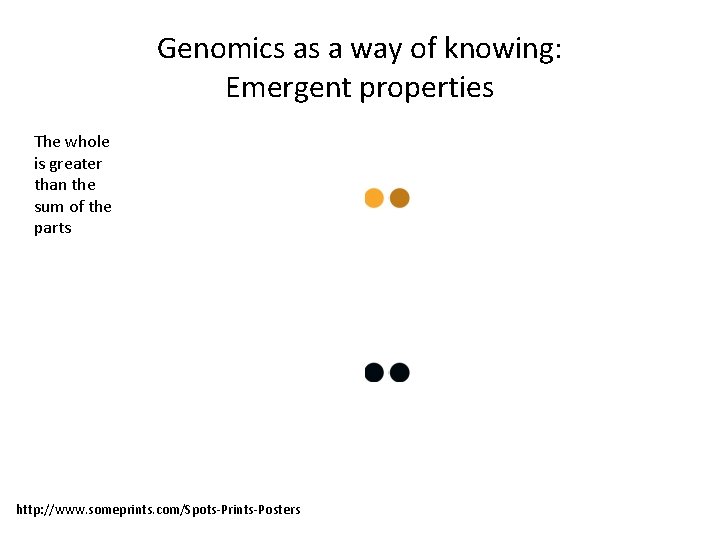
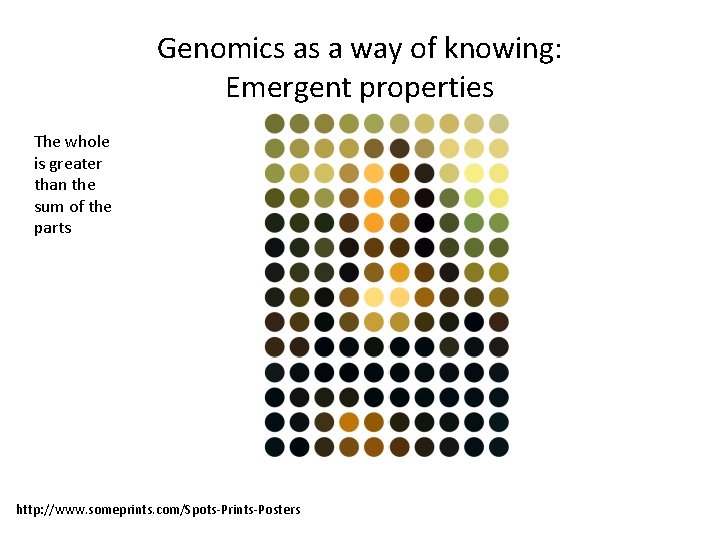
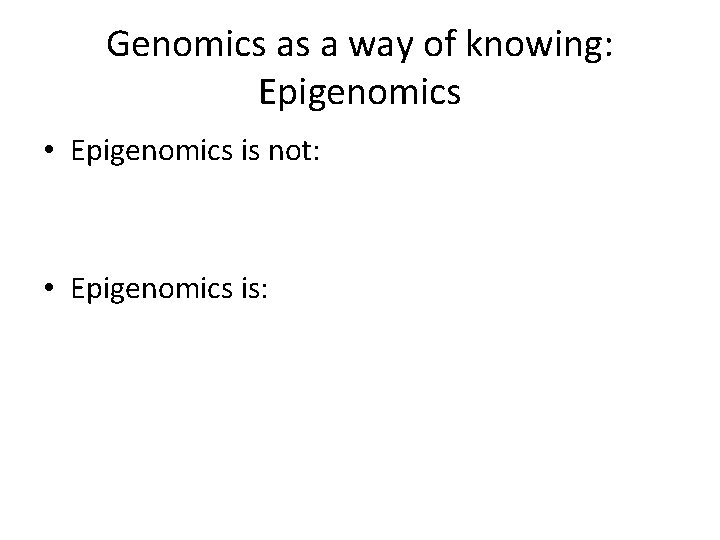
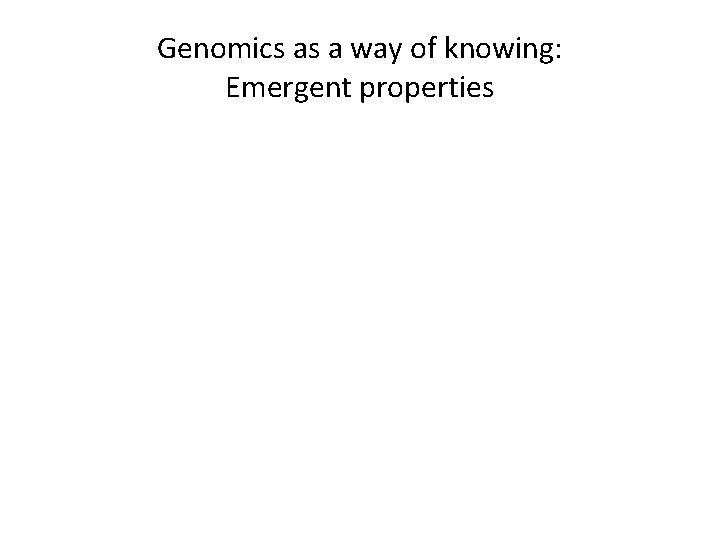
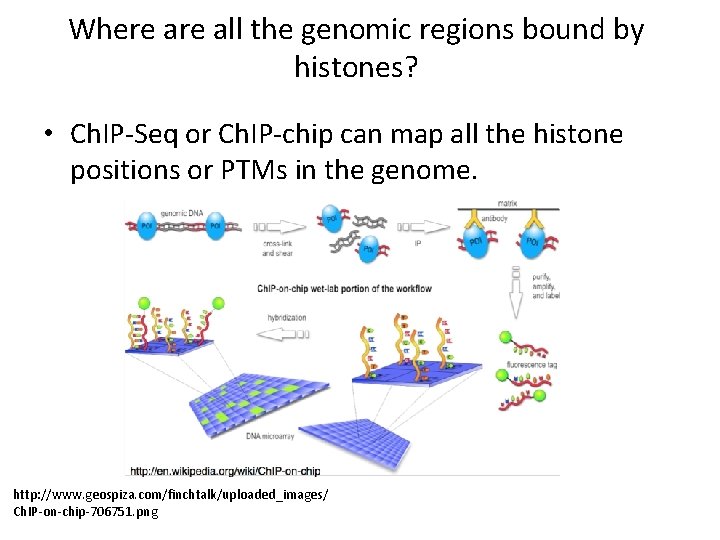
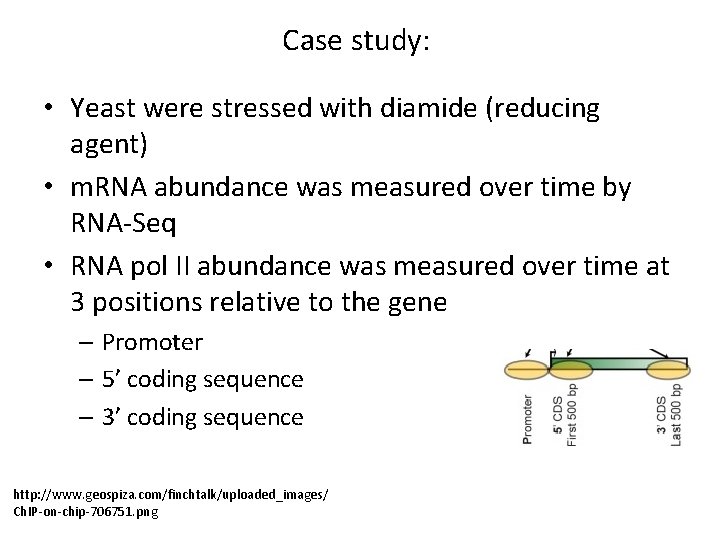
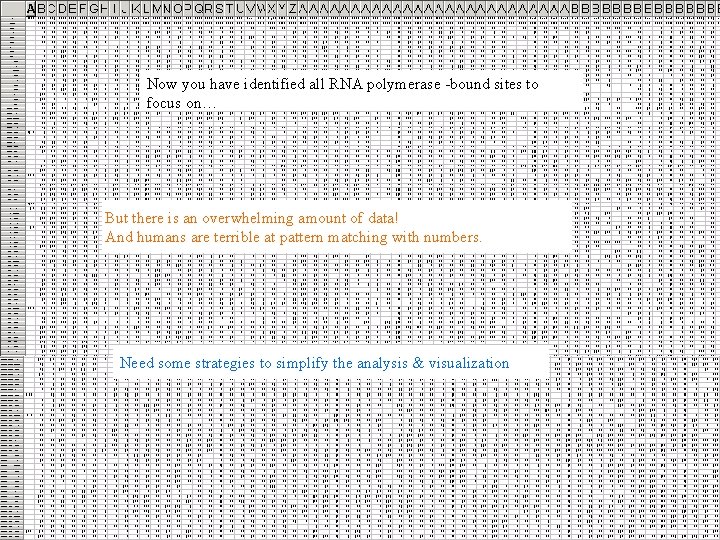
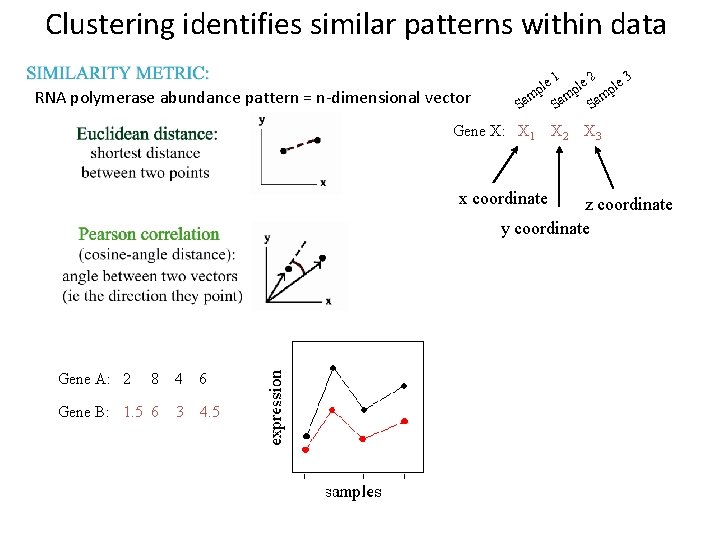
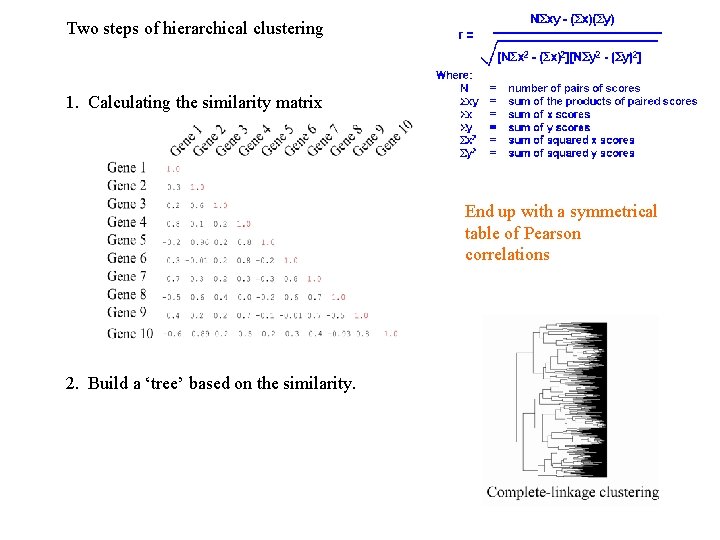
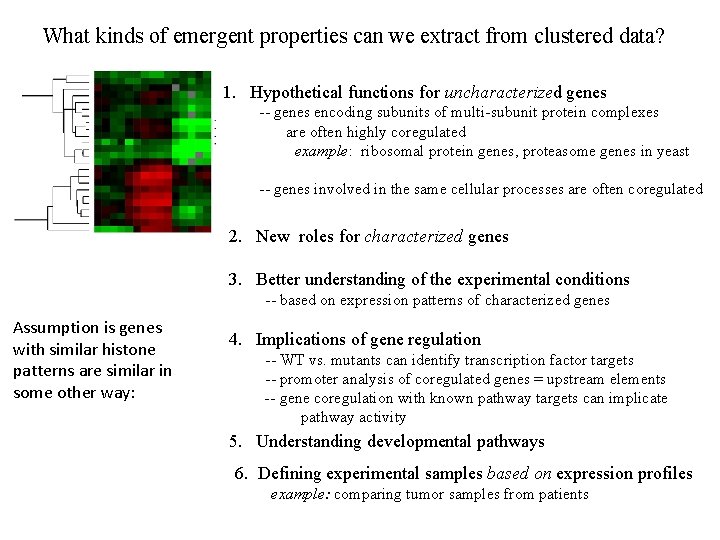
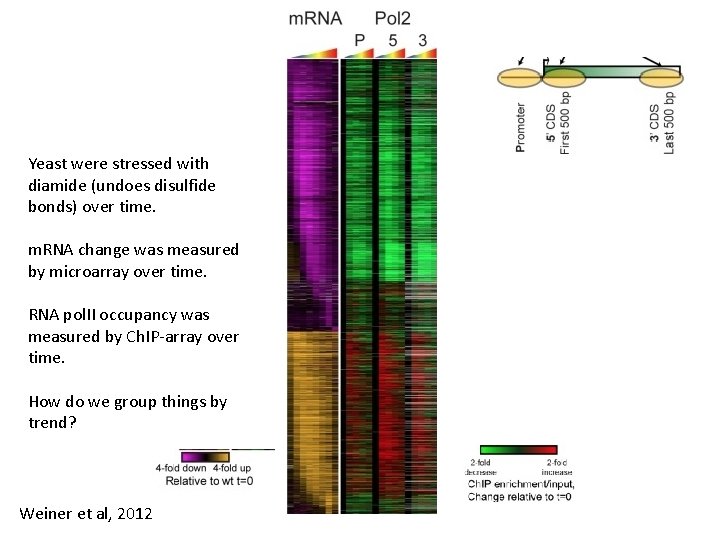
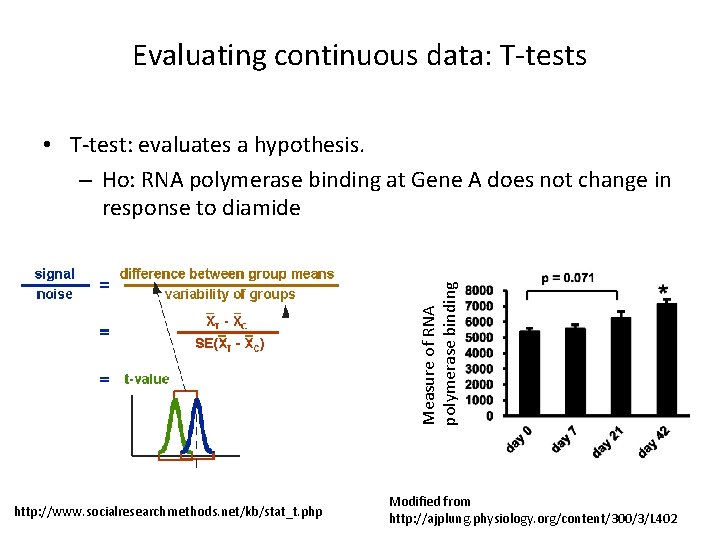
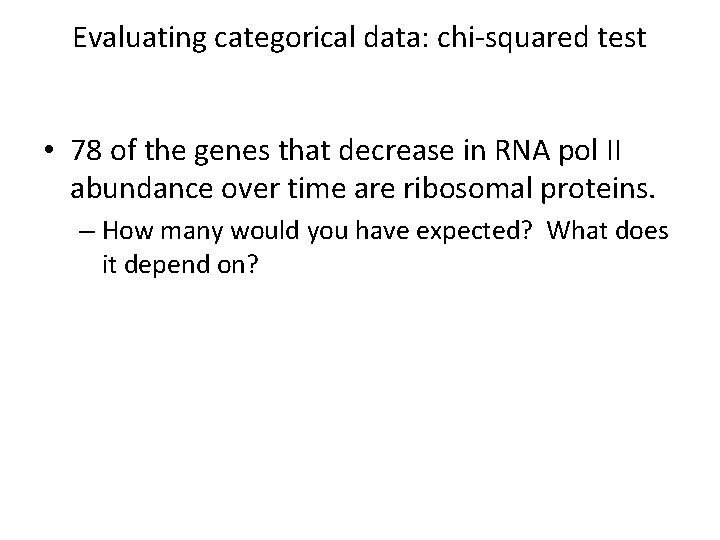
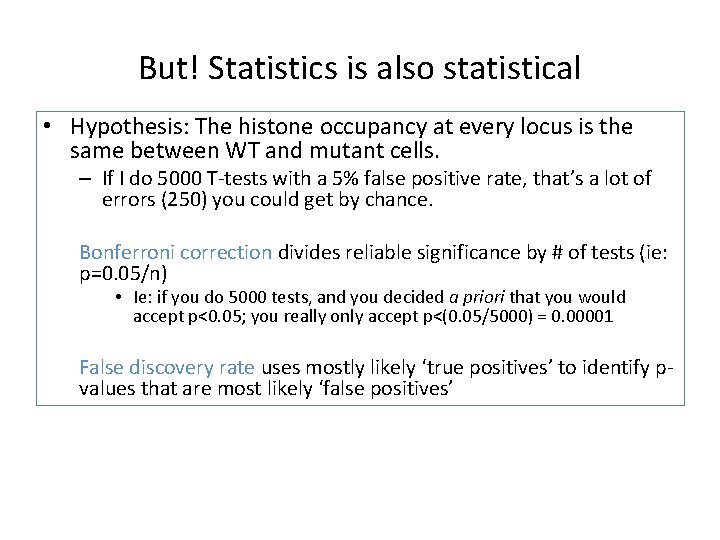
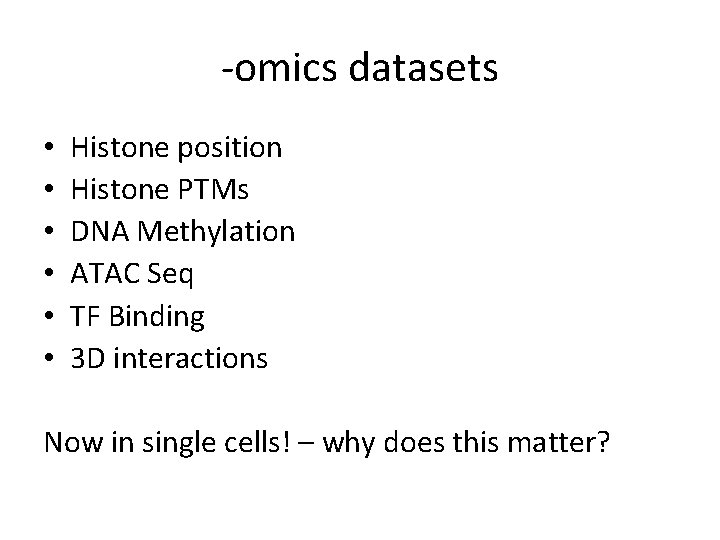
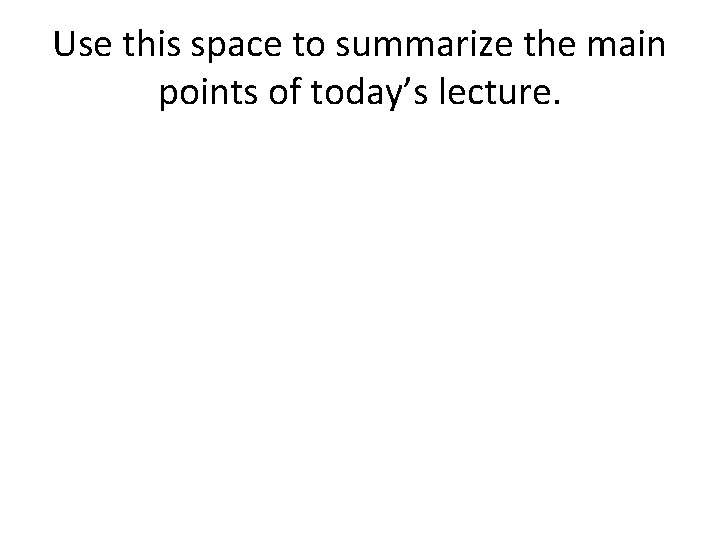
- Slides: 17
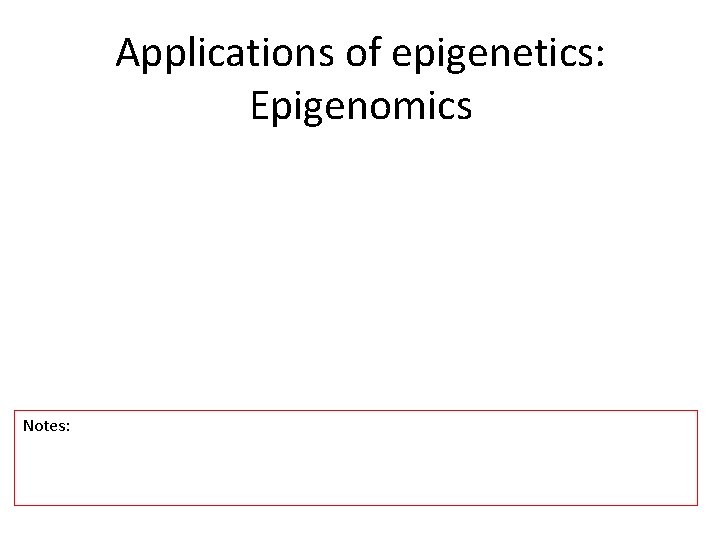
Applications of epigenetics: Epigenomics Notes:
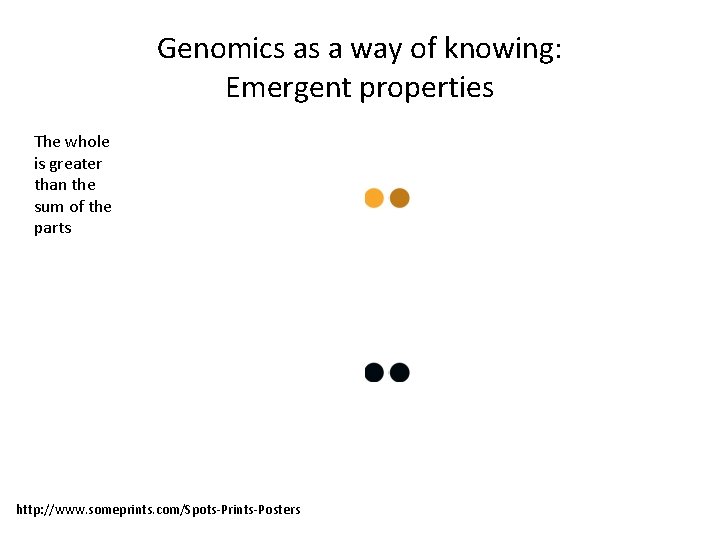
Genomics as a way of knowing: Emergent properties The whole is greater than the sum of the parts http: //www. someprints. com/Spots-Prints-Posters
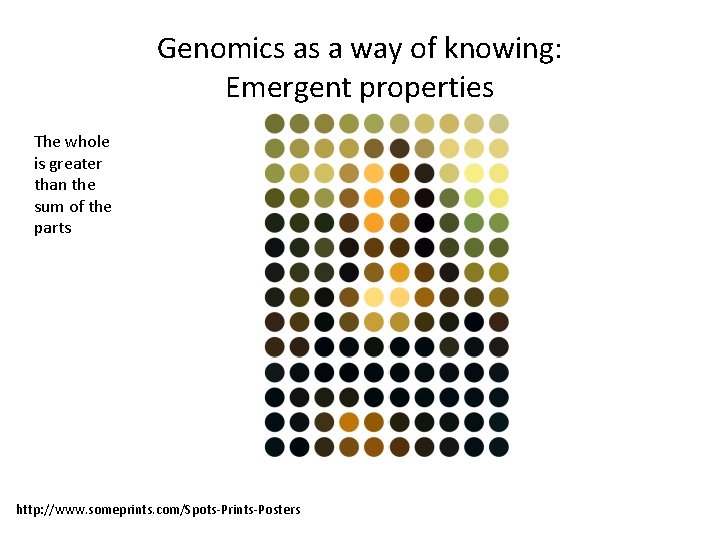
Genomics as a way of knowing: Emergent properties The whole is greater than the sum of the parts http: //www. someprints. com/Spots-Prints-Posters
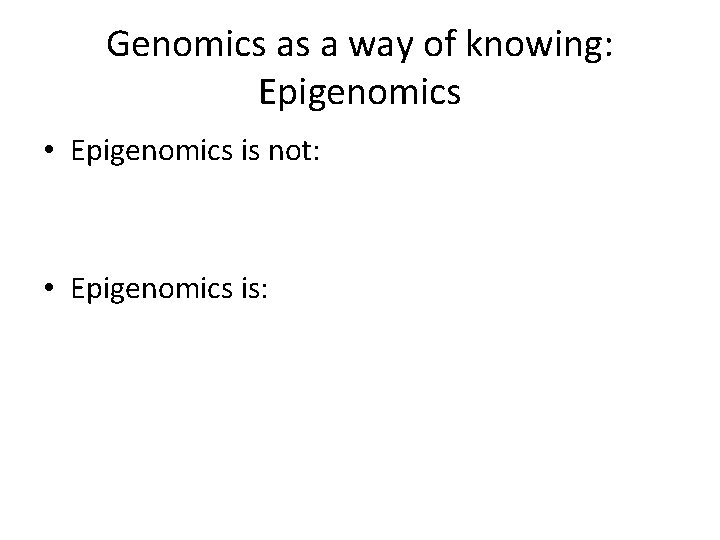
Genomics as a way of knowing: Epigenomics • Epigenomics is not: • Epigenomics is:
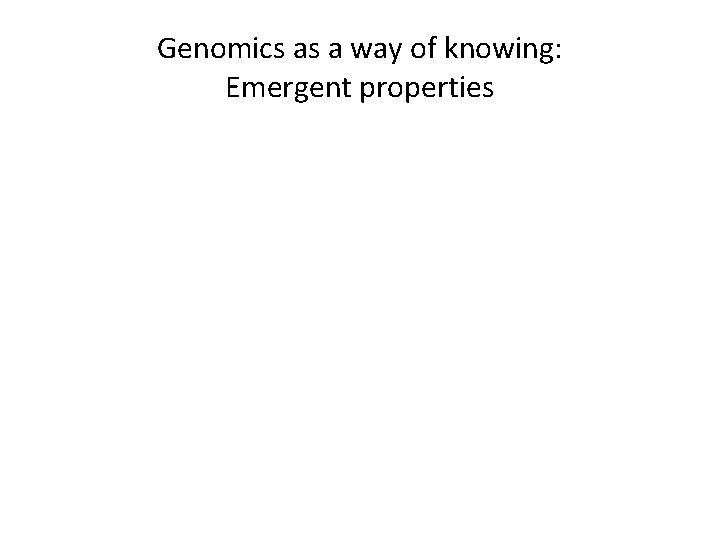
Genomics as a way of knowing: Emergent properties
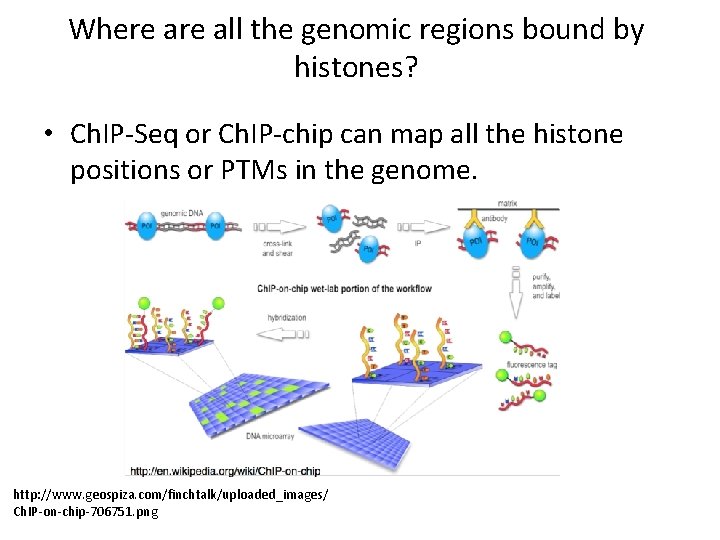
Where all the genomic regions bound by histones? • Ch. IP-Seq or Ch. IP-chip can map all the histone positions or PTMs in the genome. http: //www. geospiza. com/finchtalk/uploaded_images/ Ch. IP-on-chip-706751. png
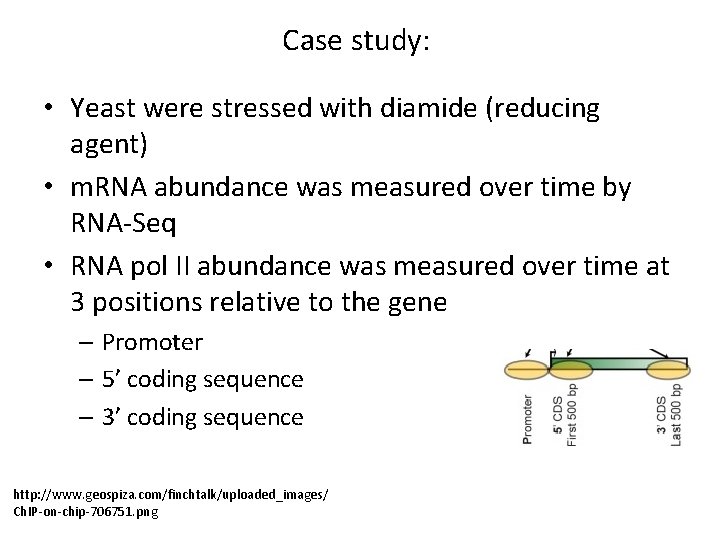
Case study: • Yeast were stressed with diamide (reducing agent) • m. RNA abundance was measured over time by RNA-Seq • RNA pol II abundance was measured over time at 3 positions relative to the gene – Promoter – 5’ coding sequence – 3’ coding sequence http: //www. geospiza. com/finchtalk/uploaded_images/ Ch. IP-on-chip-706751. png
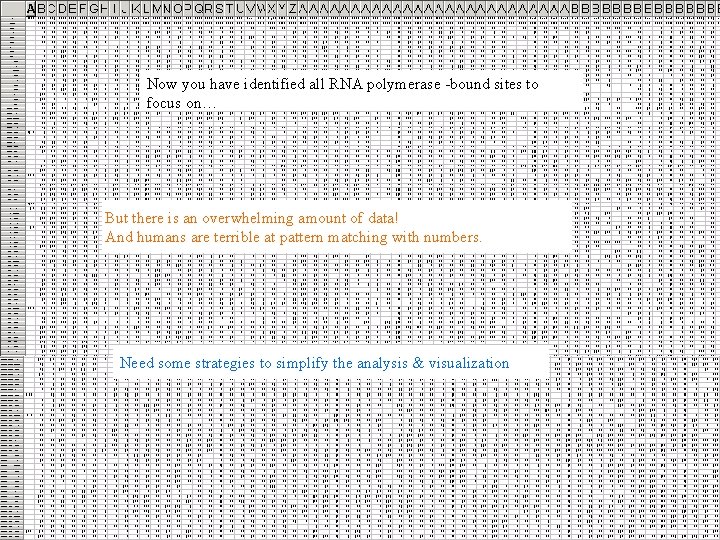
Now you have identified all RNA polymerase -bound sites to focus on… But there is an overwhelming amount of data! And humans are terrible at pattern matching with numbers. Need some strategies to simplify the analysis & visualization 8
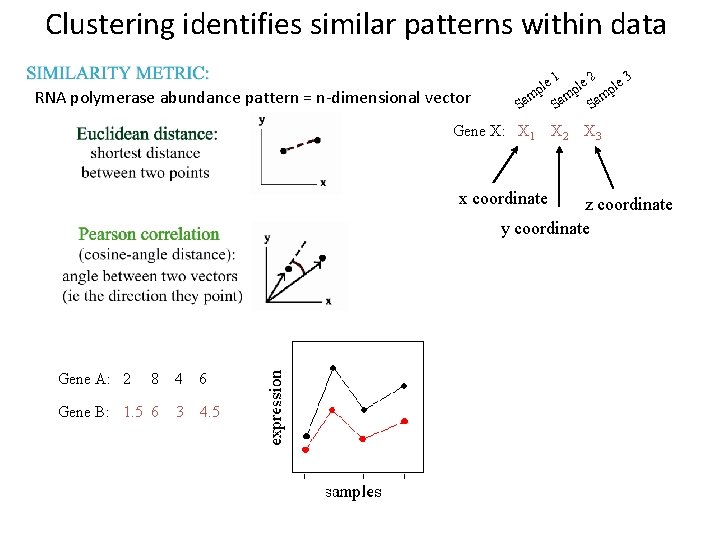
Clustering identifies similar patterns within data RNA polymerase abundance pattern = n-dimensional vector 1 2 3 le le le p p p m m m Sa Sa Sa Gene X: X 1 X 2 X 3 x coordinate z coordinate y coordinate Gene A: 2 8 Gene B: 1. 5 6 4 6 3 4. 5
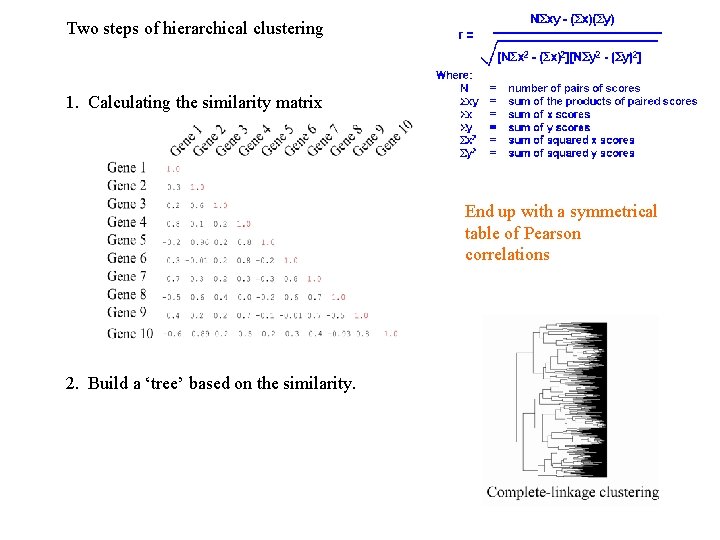
Two steps of hierarchical clustering 1. Calculating the similarity matrix End up with a symmetrical table of Pearson correlations 2. Build a ‘tree’ based on the similarity.
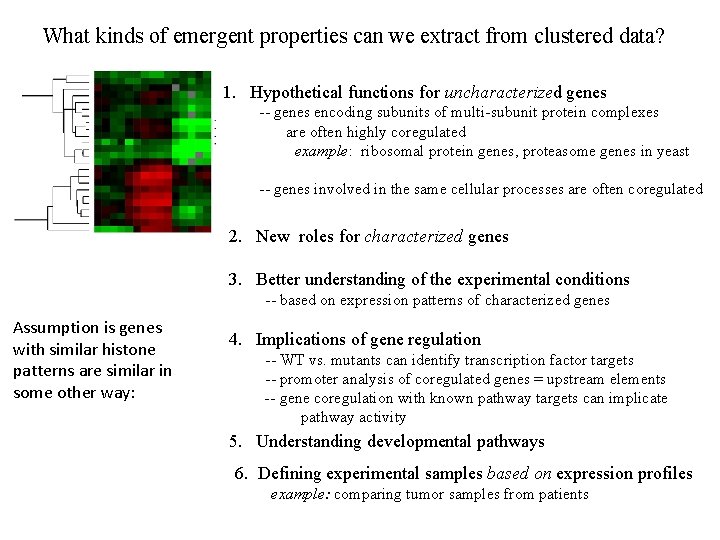
What kinds of emergent properties can we extract from clustered data? 1. Hypothetical functions for uncharacterized genes -- genes encoding subunits of multi-subunit protein complexes are often highly coregulated example: ribosomal protein genes, proteasome genes in yeast -- genes involved in the same cellular processes are often coregulated 2. New roles for characterized genes 3. Better understanding of the experimental conditions -- based on expression patterns of characterized genes Assumption is genes with similar histone patterns are similar in some other way: 4. Implications of gene regulation -- WT vs. mutants can identify transcription factor targets -- promoter analysis of coregulated genes = upstream elements -- gene coregulation with known pathway targets can implicate pathway activity 5. Understanding developmental pathways 6. Defining experimental samples based on expression profiles example: comparing tumor samples from patients
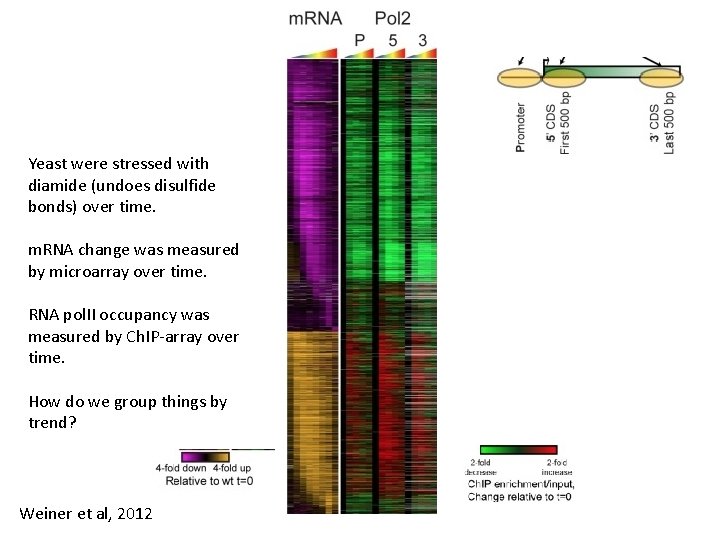
Yeast were stressed with diamide (undoes disulfide bonds) over time. m. RNA change was measured by microarray over time. RNA pol. II occupancy was measured by Ch. IP-array over time. How do we group things by trend? Weiner et al, 2012
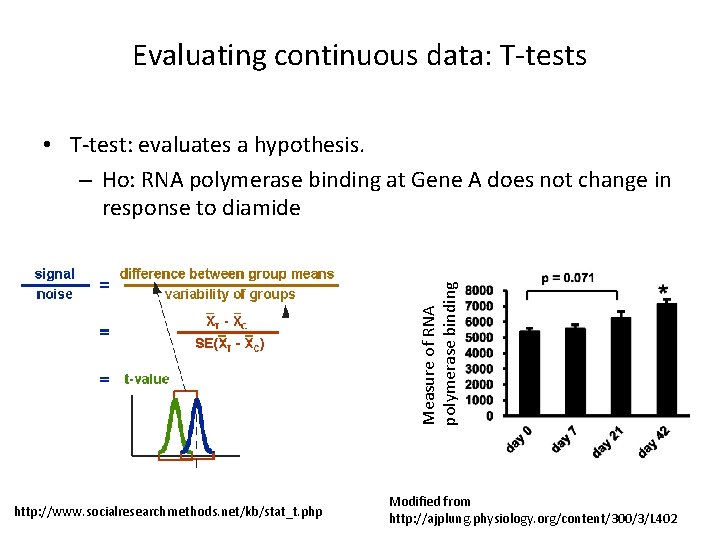
Evaluating continuous data: T-tests Measure of RNA polymerase binding • T-test: evaluates a hypothesis. – Ho: RNA polymerase binding at Gene A does not change in response to diamide http: //www. socialresearchmethods. net/kb/stat_t. php Modified from http: //ajplung. physiology. org/content/300/3/L 402
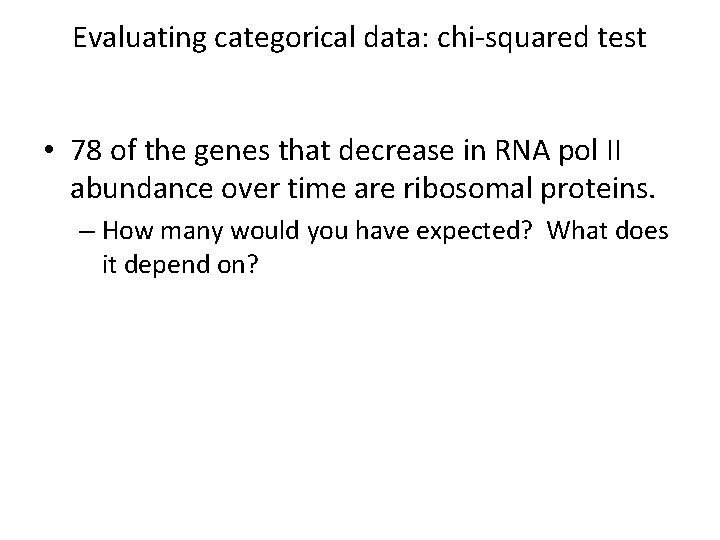
Evaluating categorical data: chi-squared test • 78 of the genes that decrease in RNA pol II abundance over time are ribosomal proteins. – How many would you have expected? What does it depend on?
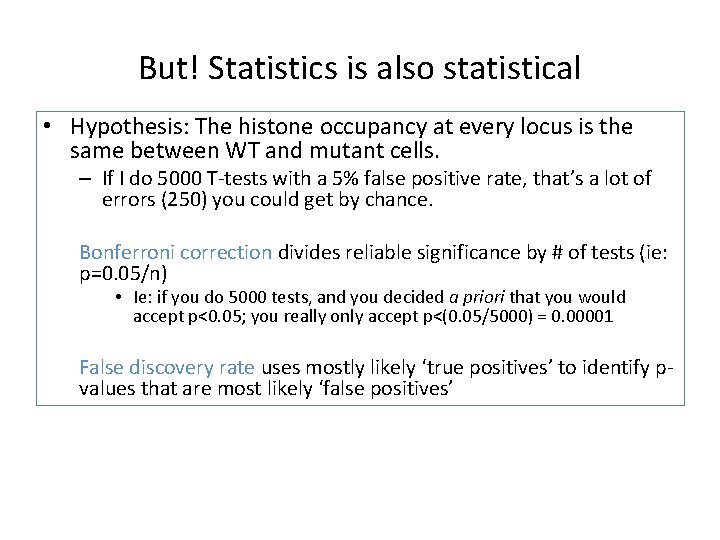
But! Statistics is also statistical • Hypothesis: The histone occupancy at every locus is the same between WT and mutant cells. – If I do 5000 T-tests with a 5% false positive rate, that’s a lot of errors (250) you could get by chance. Bonferroni correction divides reliable significance by # of tests (ie: p=0. 05/n) • Ie: if you do 5000 tests, and you decided a priori that you would accept p<0. 05; you really only accept p<(0. 05/5000) = 0. 00001 False discovery rate uses mostly likely ‘true positives’ to identify pvalues that are most likely ‘false positives’
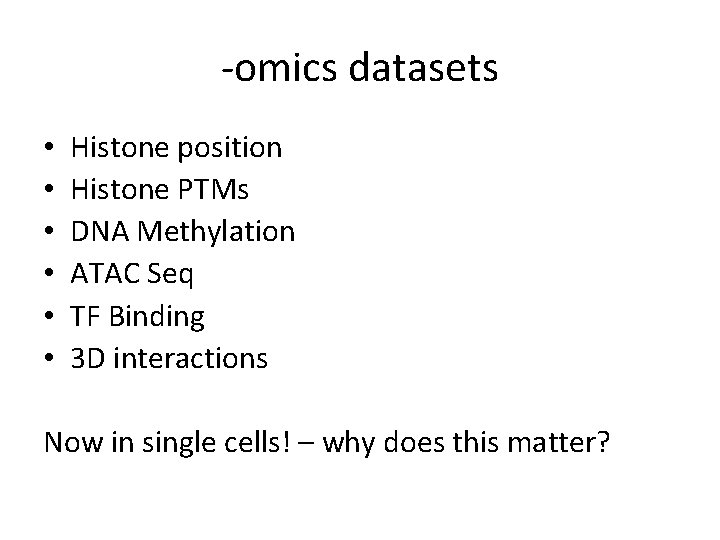
-omics datasets • • • Histone position Histone PTMs DNA Methylation ATAC Seq TF Binding 3 D interactions Now in single cells! – why does this matter?
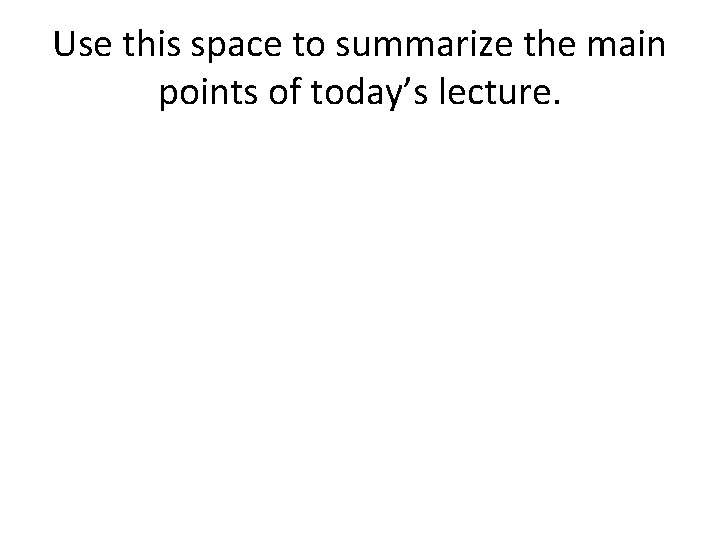
Use this space to summarize the main points of today’s lecture.
Epigenomics n
Early fly
What is epigenetics
Harvest genomics
Difference between structural and functional genomics
Types of genomics
Application of genomics
A vision for the future of genomics research
Genome
Essnet qsr
"encoded genomics" -job
Igv genome
Genomics
Difference between structural and functional genomics
"encoded genomics" -job
Rachel butler genomics
Functional genomics
Integrated genomics viewer