1 Model Diagnostics and OLS Assumptions Political Analysis
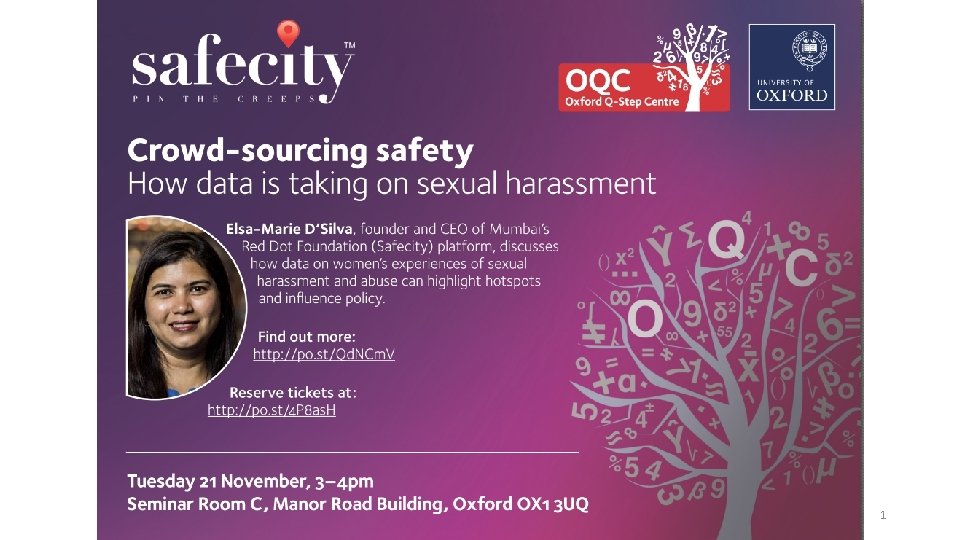
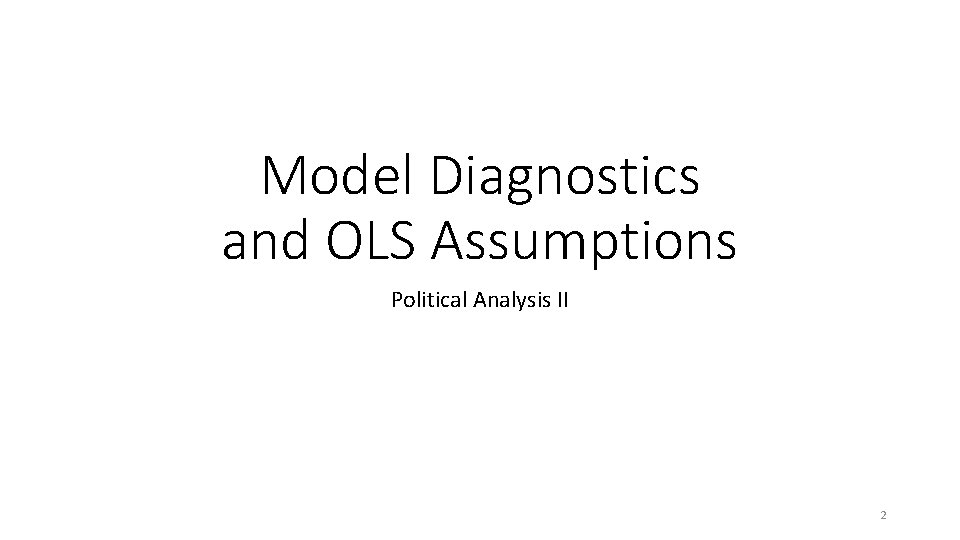
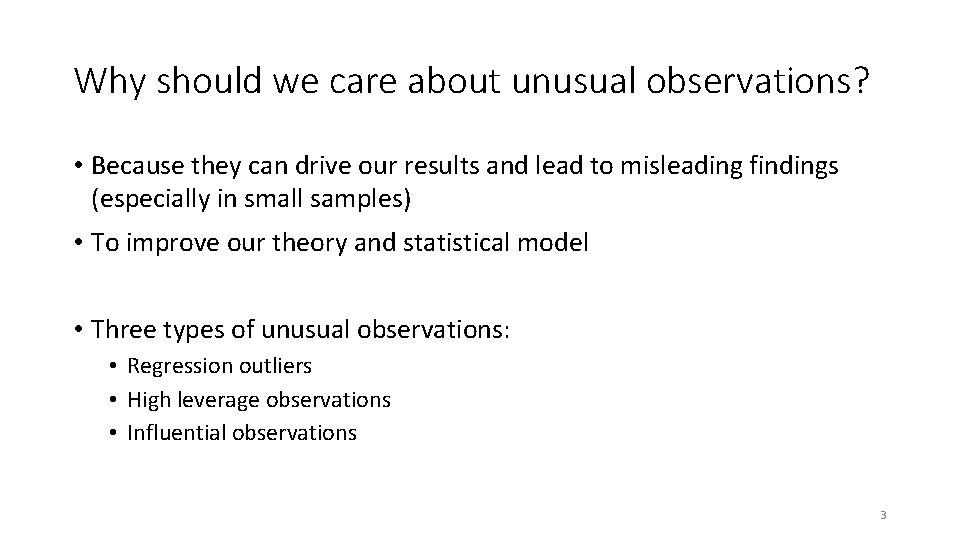
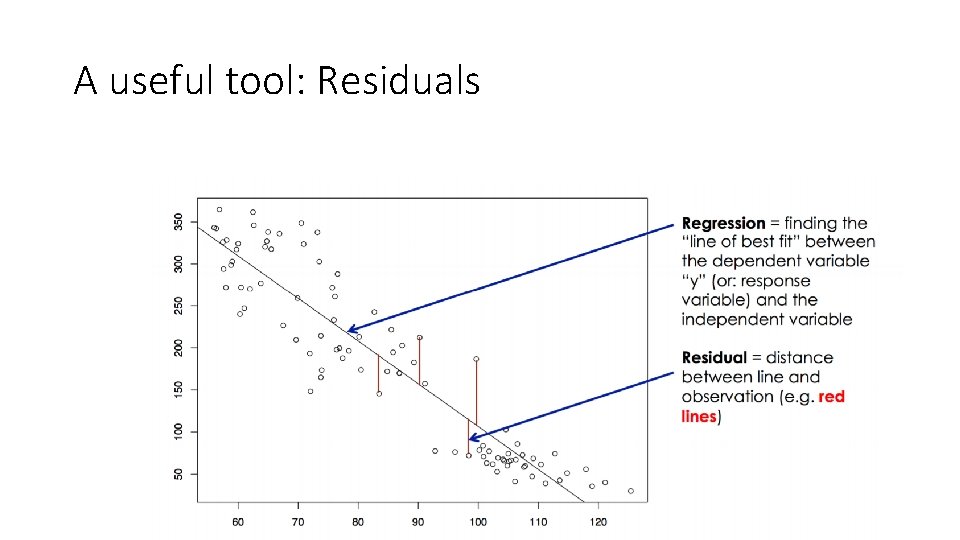
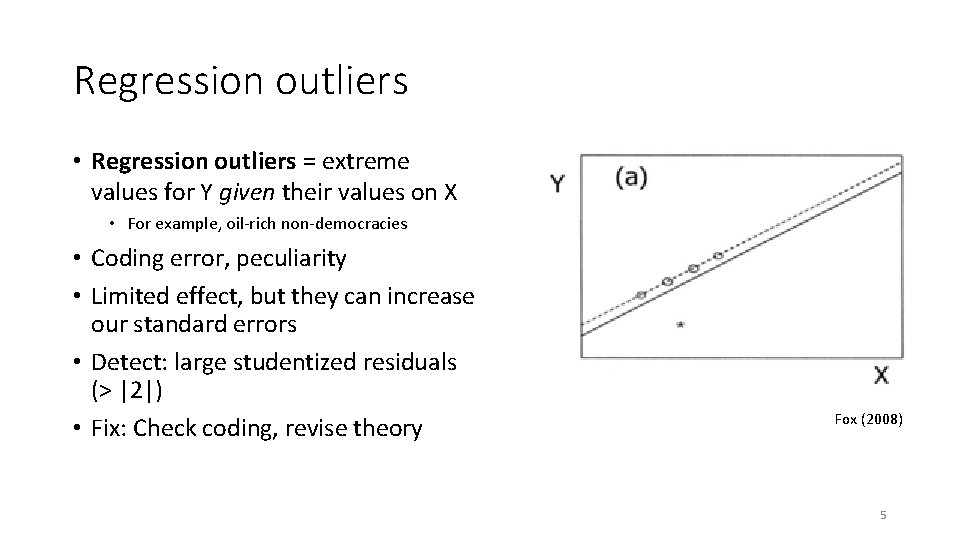
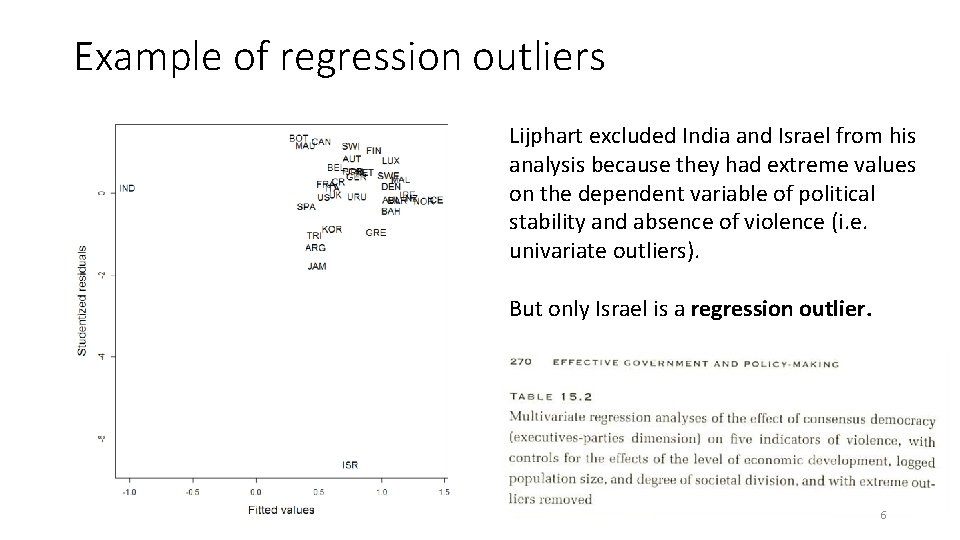
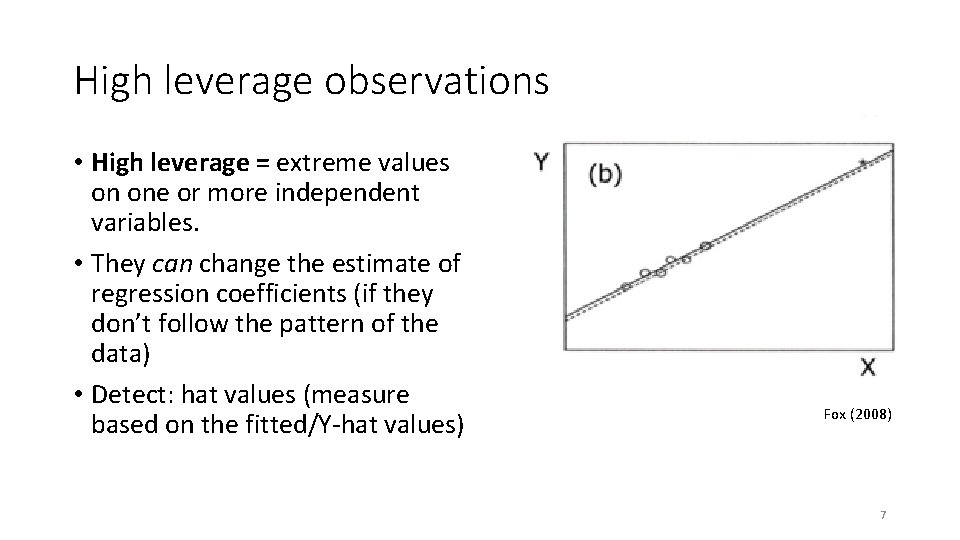
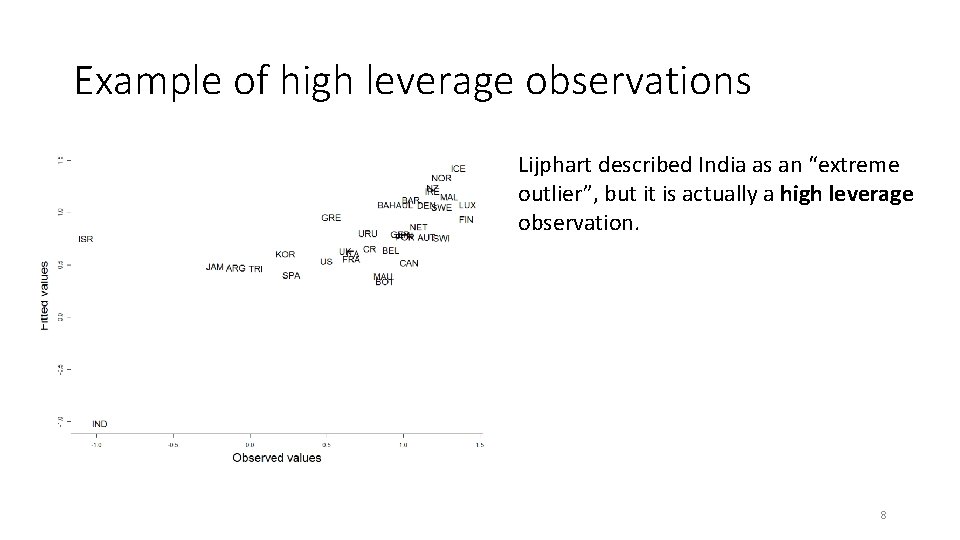
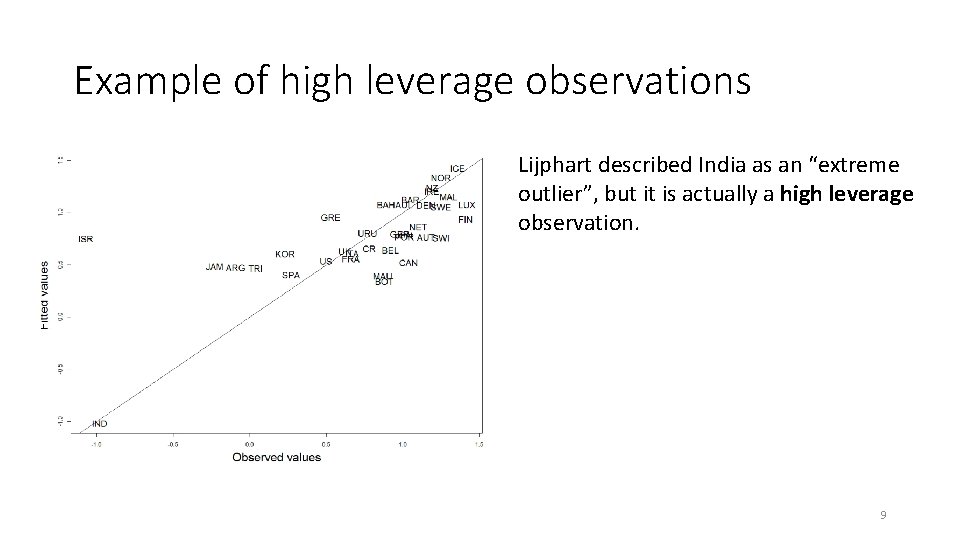
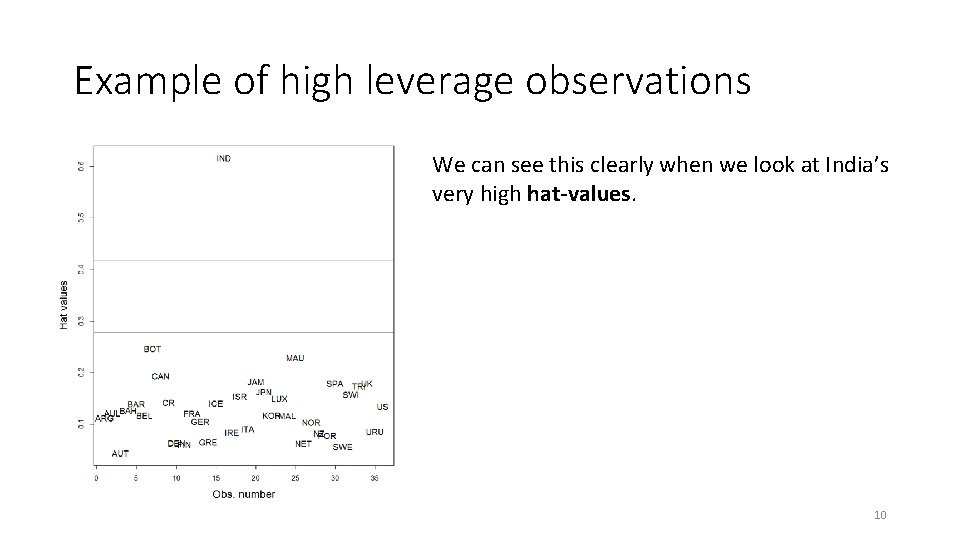
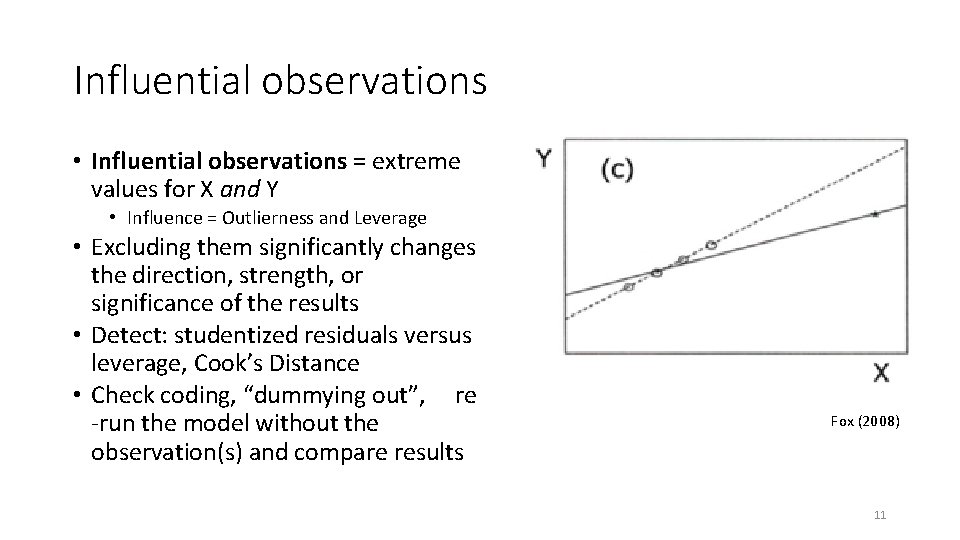
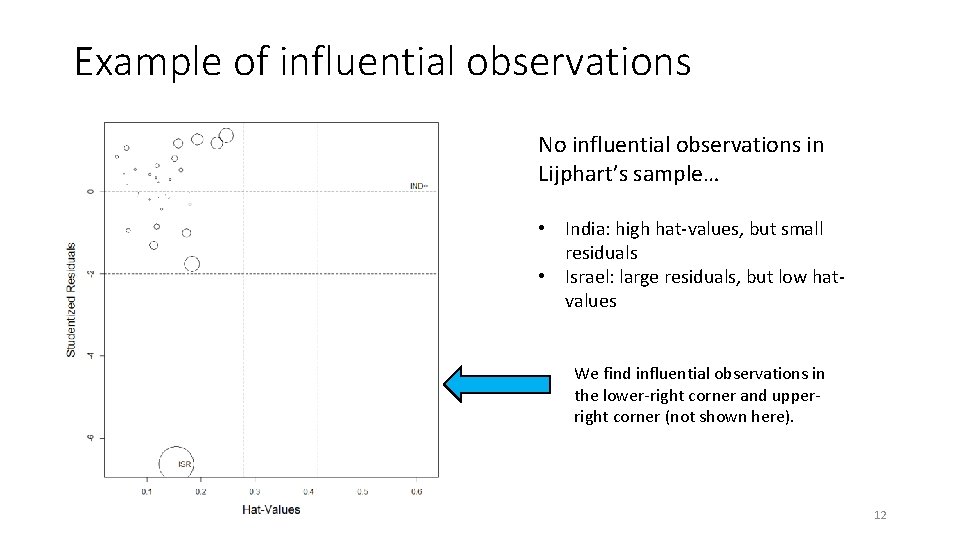
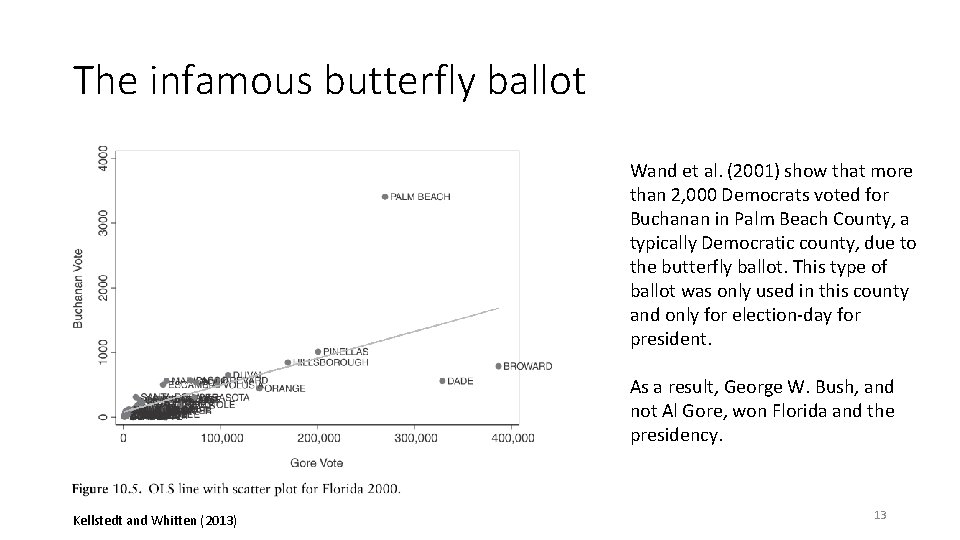
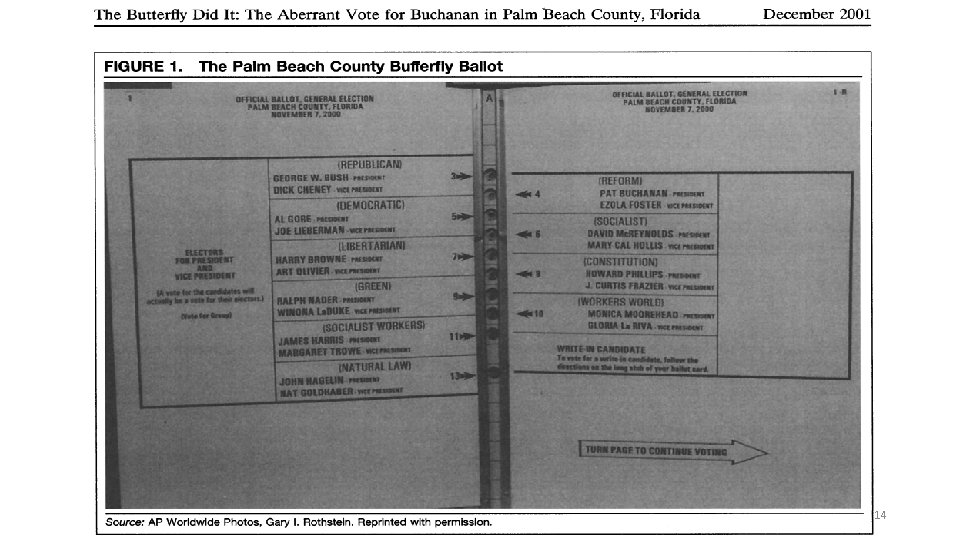
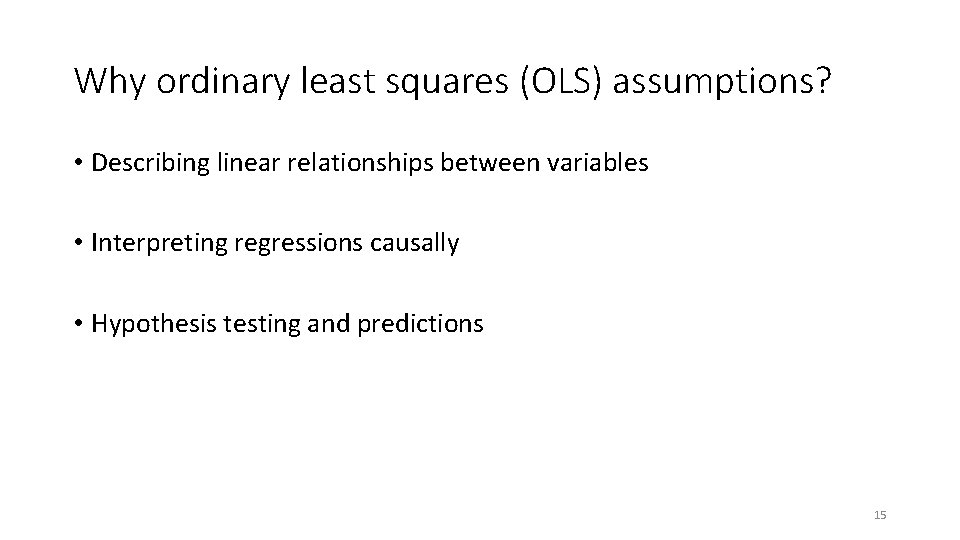
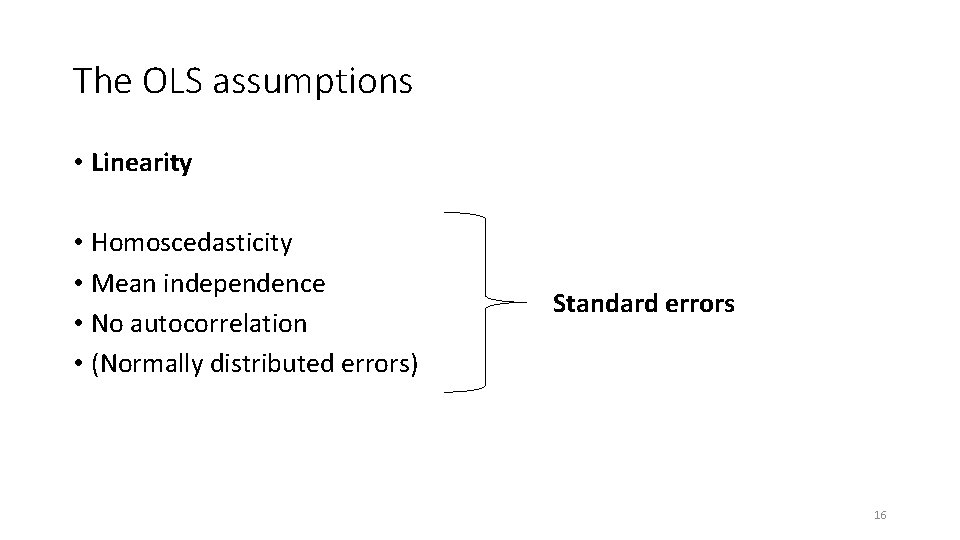
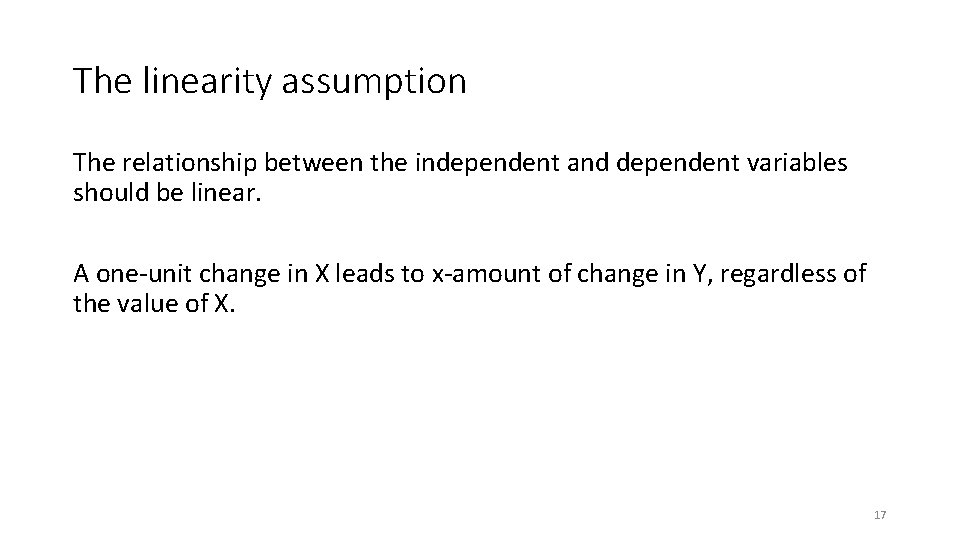
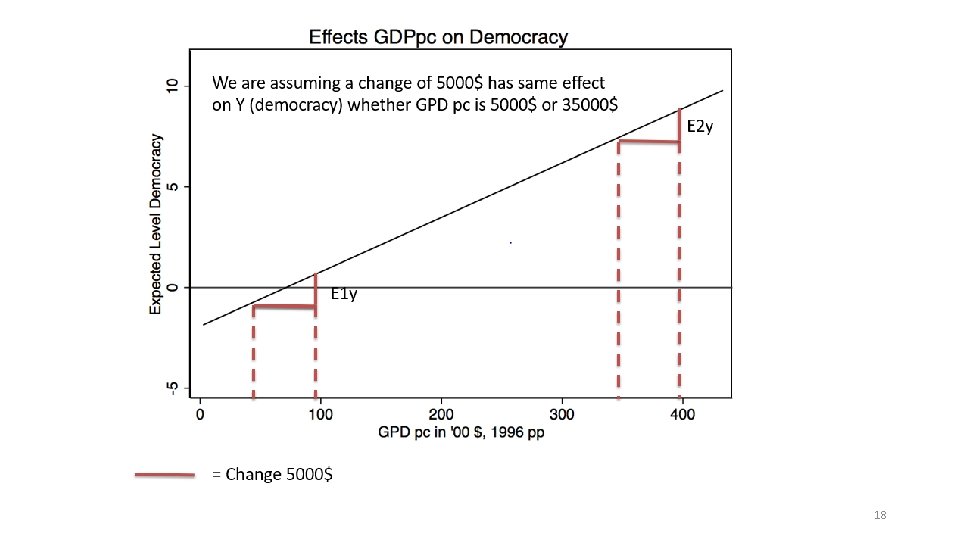
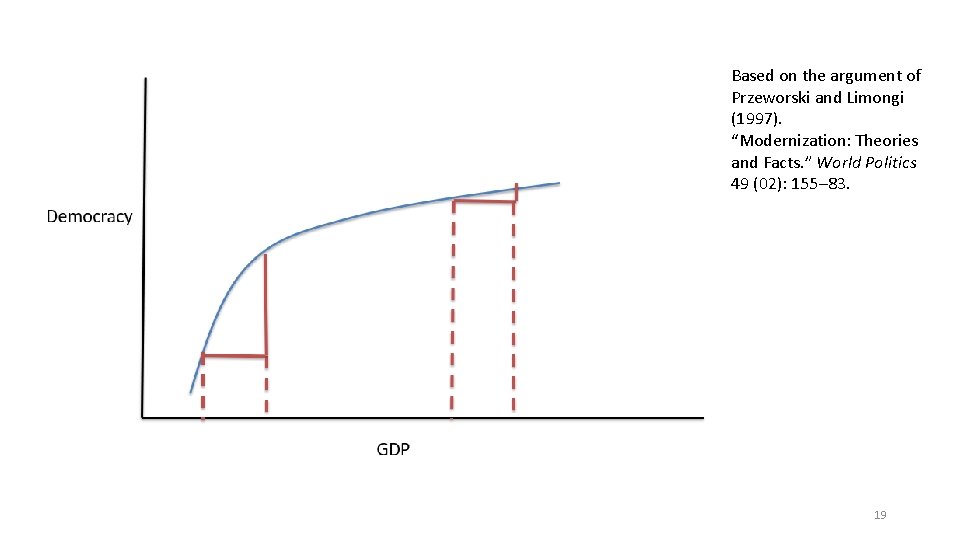
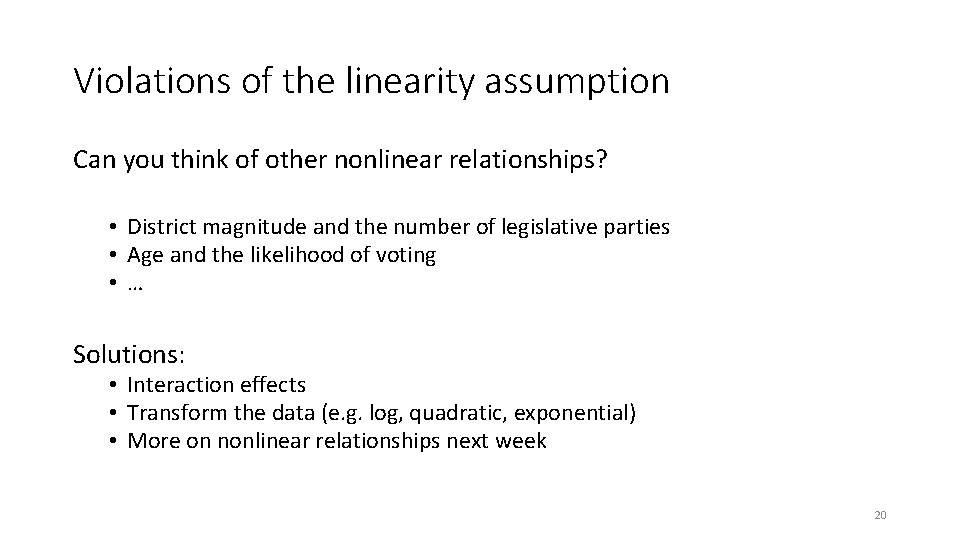
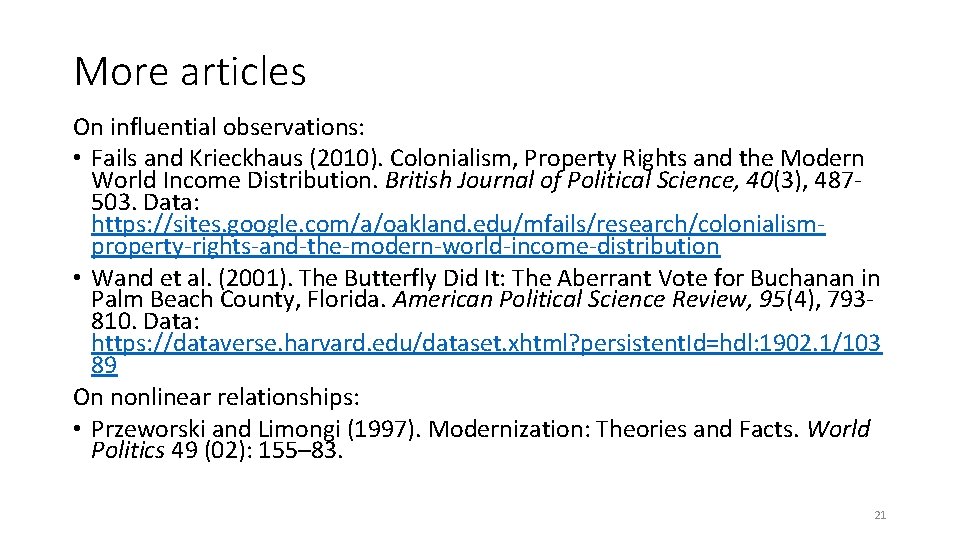
- Slides: 21
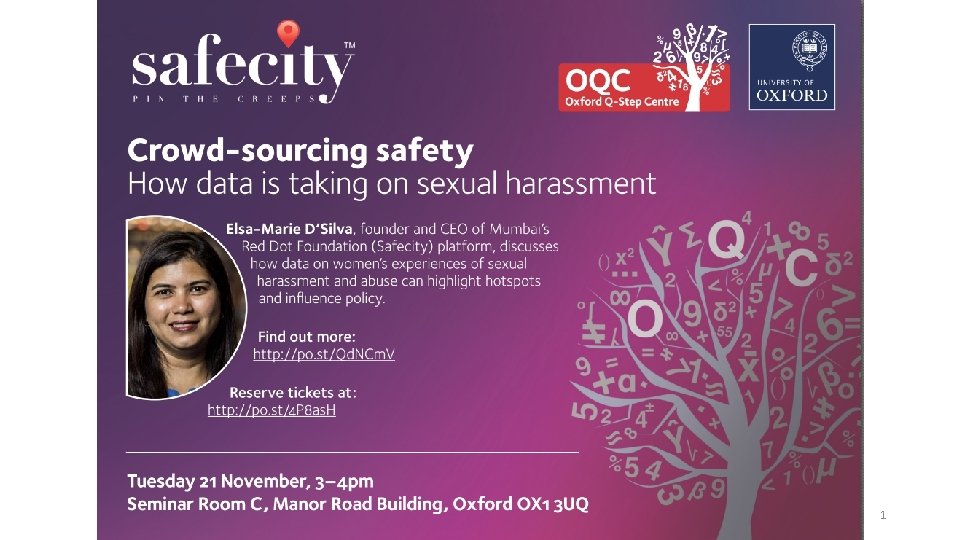
1
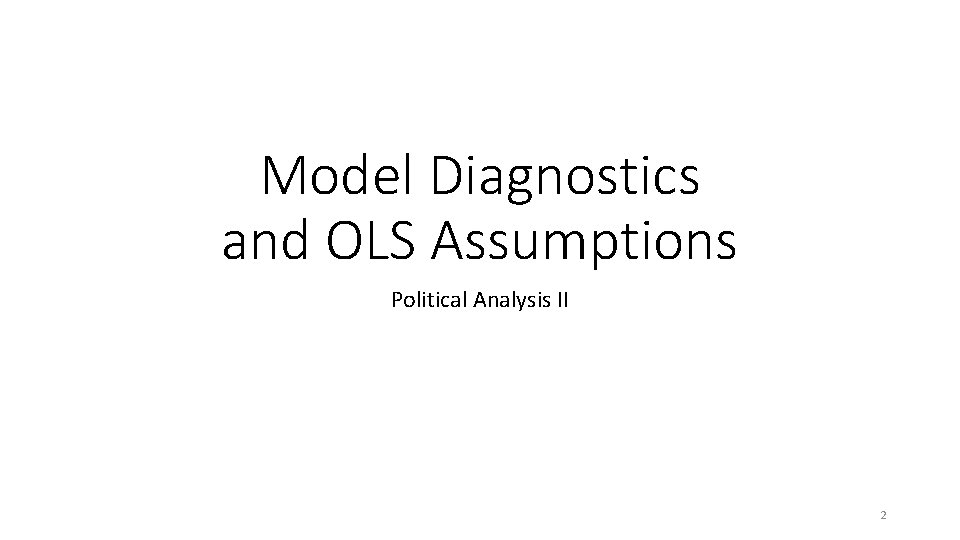
Model Diagnostics and OLS Assumptions Political Analysis II 2
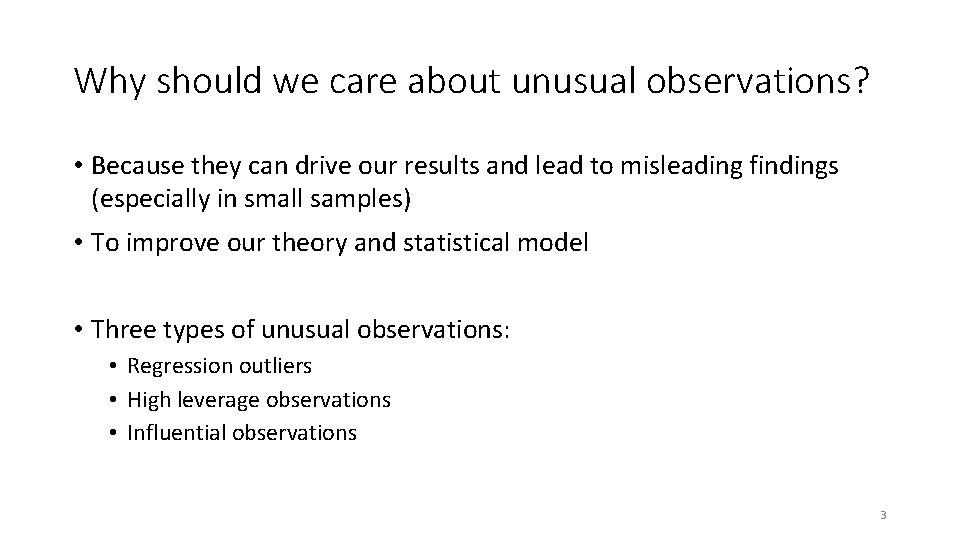
Why should we care about unusual observations? • Because they can drive our results and lead to misleading findings (especially in small samples) • To improve our theory and statistical model • Three types of unusual observations: • Regression outliers • High leverage observations • Influential observations 3
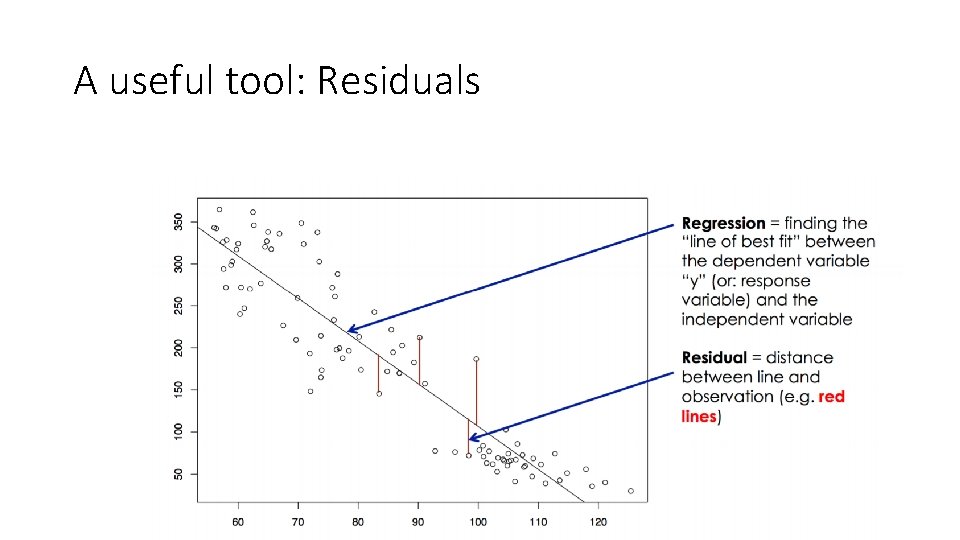
A useful tool: Residuals 4
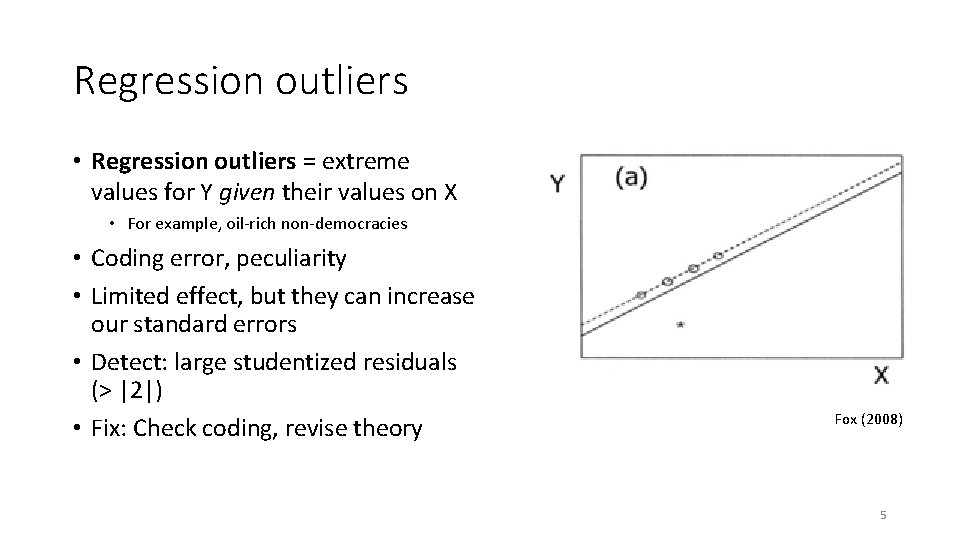
Regression outliers • Regression outliers = extreme values for Y given their values on X • For example, oil-rich non-democracies • Coding error, peculiarity • Limited effect, but they can increase our standard errors • Detect: large studentized residuals (> |2|) • Fix: Check coding, revise theory Fox (2008) 5
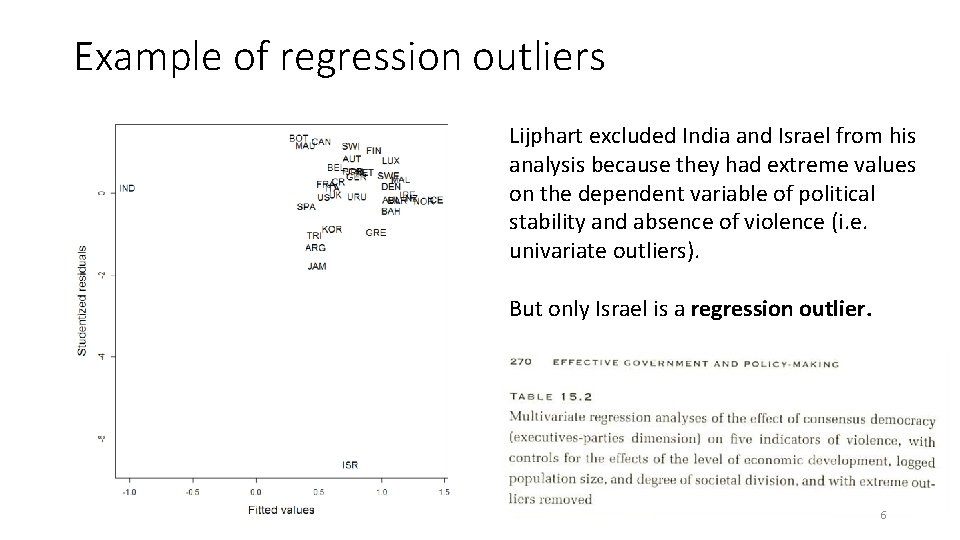
Example of regression outliers Lijphart excluded India and Israel from his analysis because they had extreme values on the dependent variable of political stability and absence of violence (i. e. univariate outliers). But only Israel is a regression outlier. 6
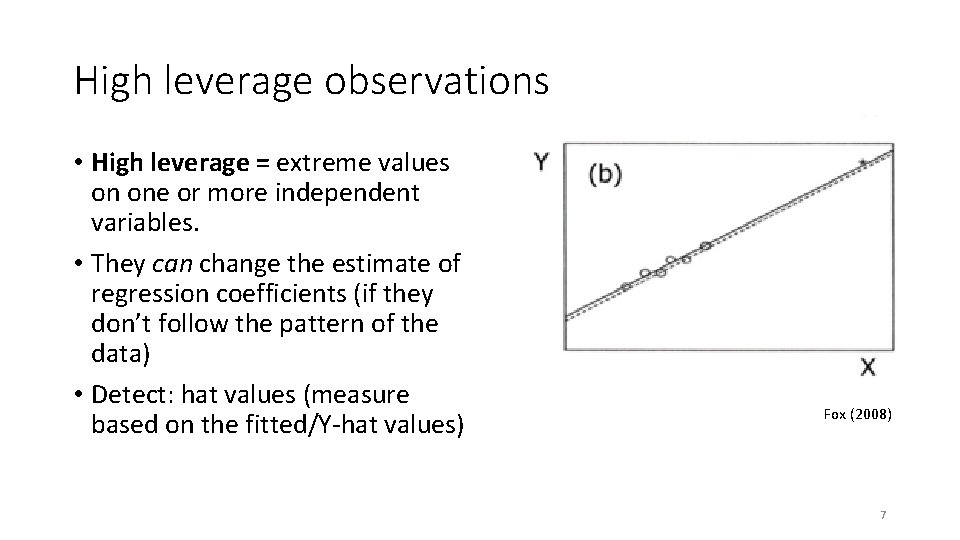
High leverage observations • High leverage = extreme values on one or more independent variables. • They can change the estimate of regression coefficients (if they don’t follow the pattern of the data) • Detect: hat values (measure based on the fitted/Y-hat values) Fox (2008) 7
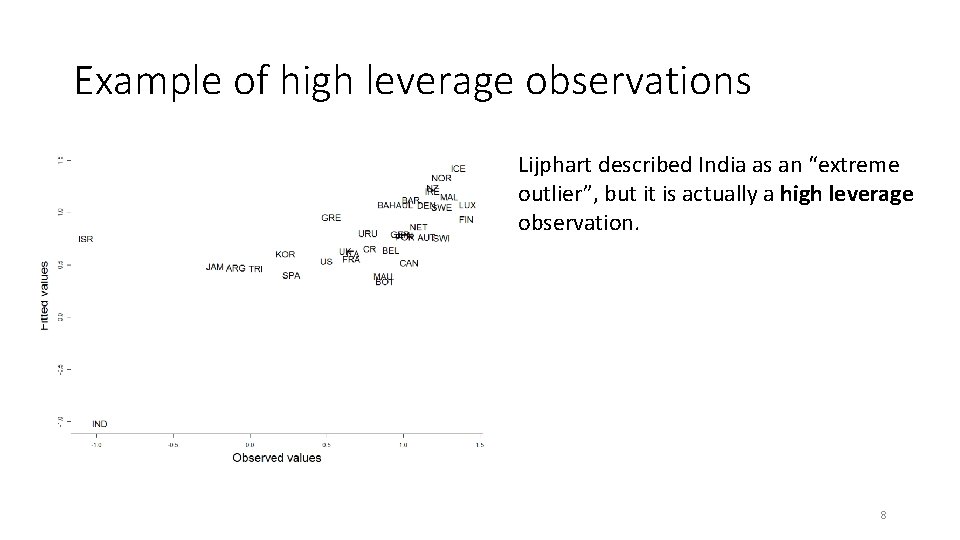
Example of high leverage observations Lijphart described India as an “extreme outlier”, but it is actually a high leverage observation. 8
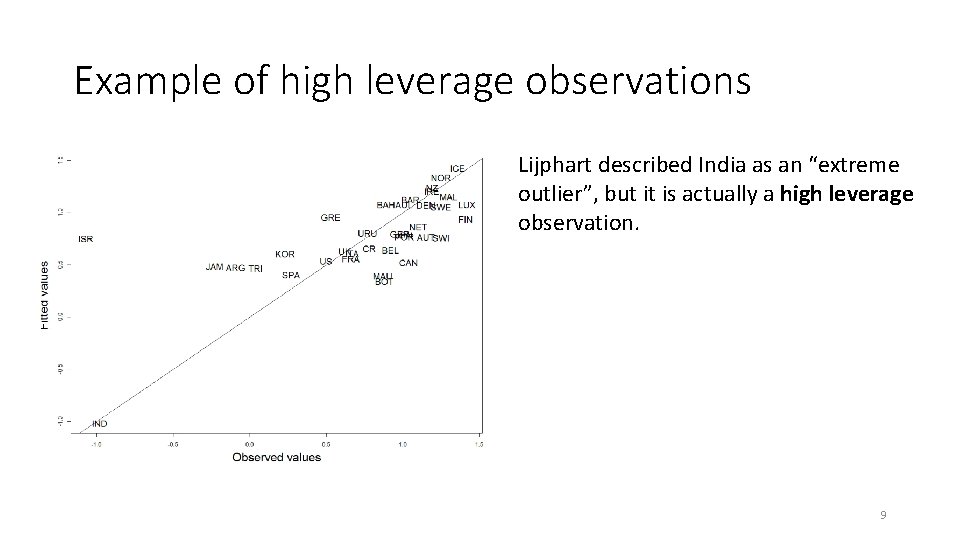
Example of high leverage observations Lijphart described India as an “extreme outlier”, but it is actually a high leverage observation. 9
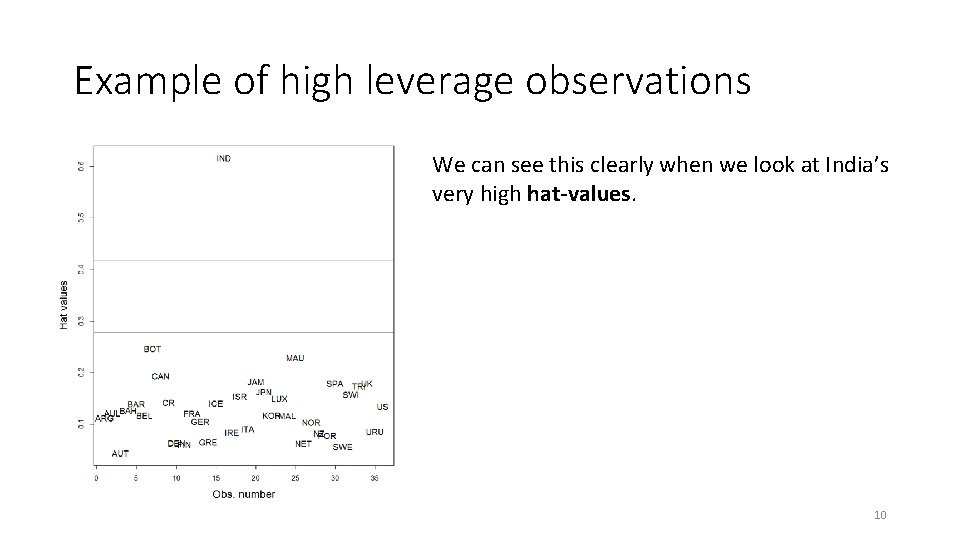
Example of high leverage observations We can see this clearly when we look at India’s very high hat-values. 10
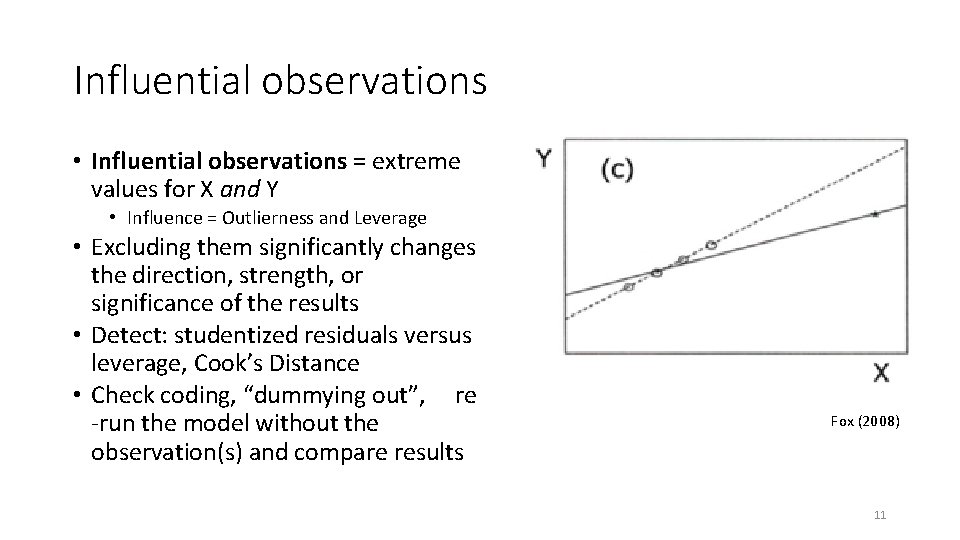
Influential observations • Influential observations = extreme values for X and Y • Influence = Outlierness and Leverage • Excluding them significantly changes the direction, strength, or significance of the results • Detect: studentized residuals versus leverage, Cook’s Distance • Check coding, “dummying out”, re -run the model without the observation(s) and compare results Fox (2008) 11
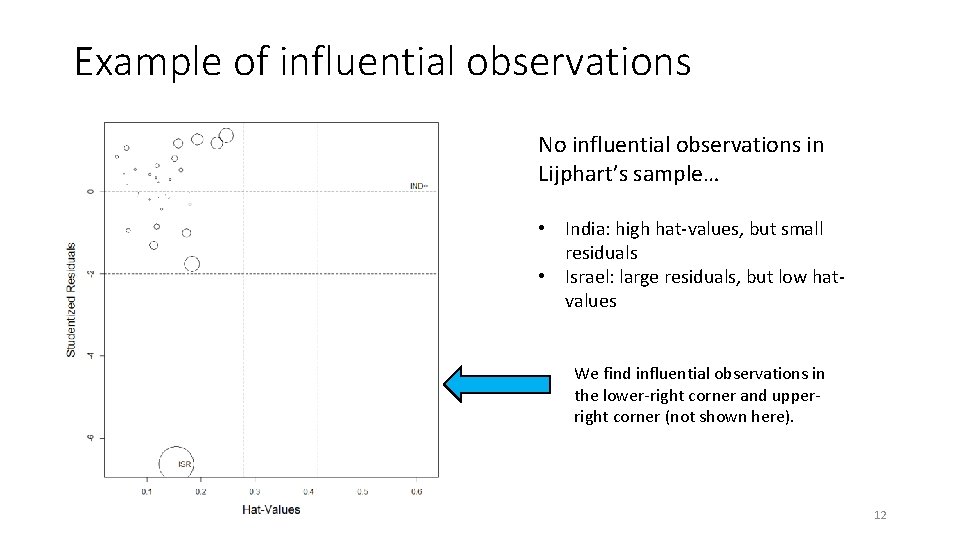
Example of influential observations No influential observations in Lijphart’s sample… • India: high hat-values, but small residuals • Israel: large residuals, but low hatvalues We find influential observations in the lower-right corner and upperright corner (not shown here). 12
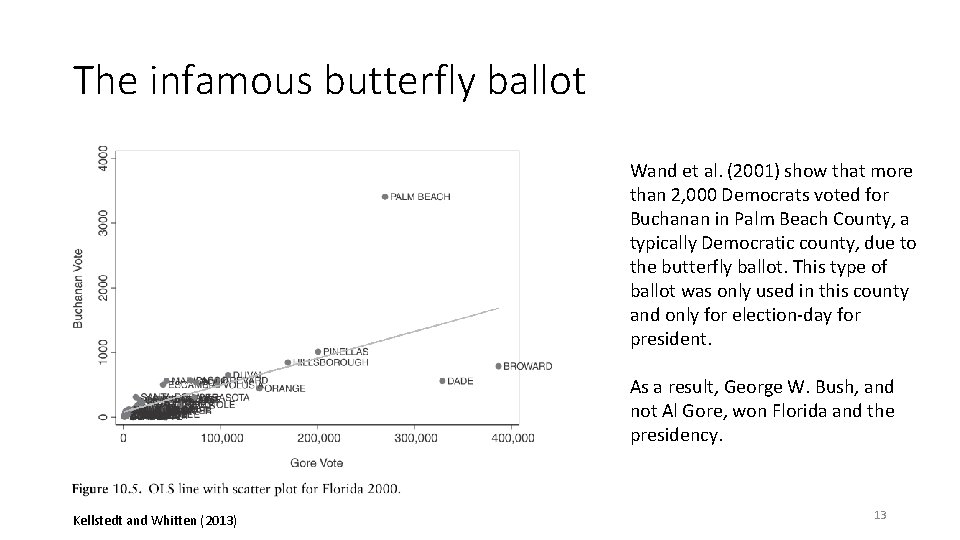
The infamous butterfly ballot Wand et al. (2001) show that more than 2, 000 Democrats voted for Buchanan in Palm Beach County, a typically Democratic county, due to the butterfly ballot. This type of ballot was only used in this county and only for election-day for president. As a result, George W. Bush, and not Al Gore, won Florida and the presidency. Kellstedt and Whitten (2013) 13
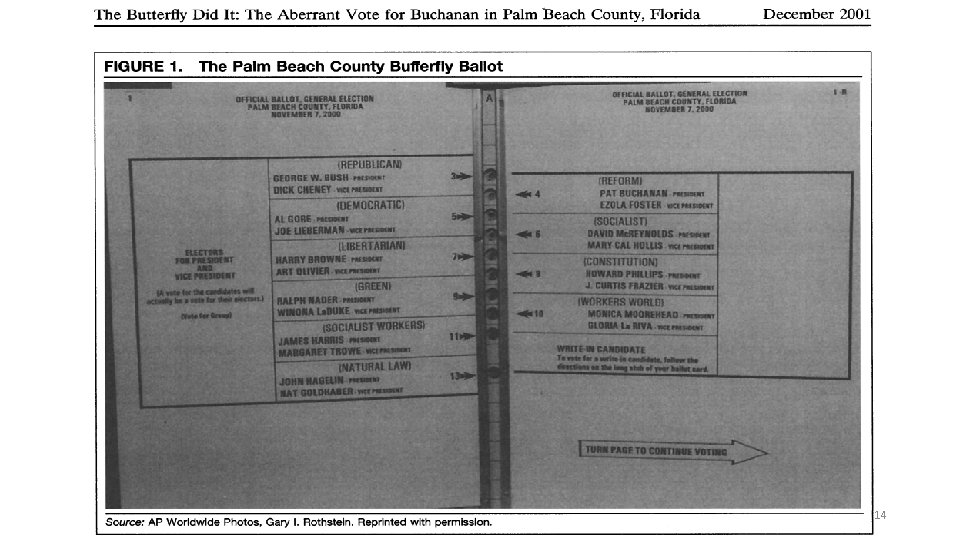
14
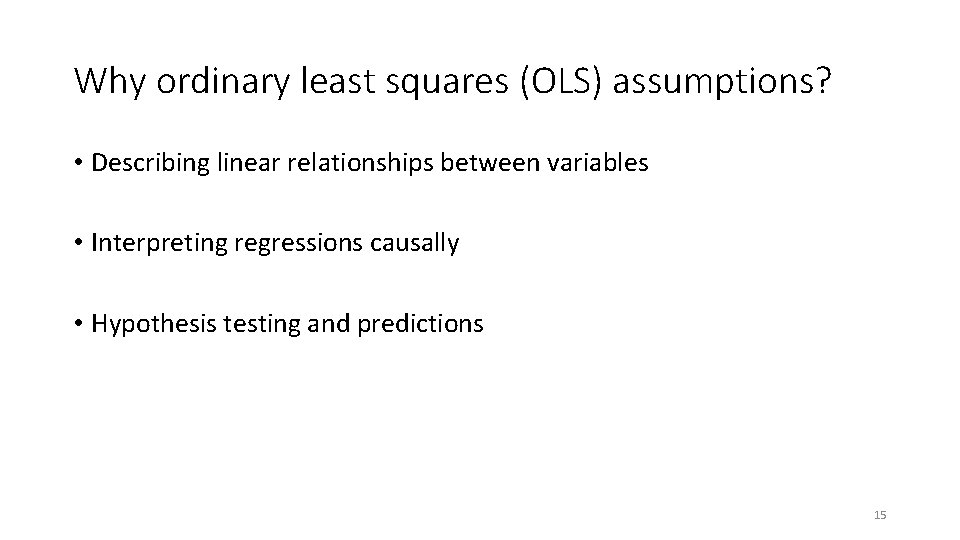
Why ordinary least squares (OLS) assumptions? • Describing linear relationships between variables • Interpreting regressions causally • Hypothesis testing and predictions 15
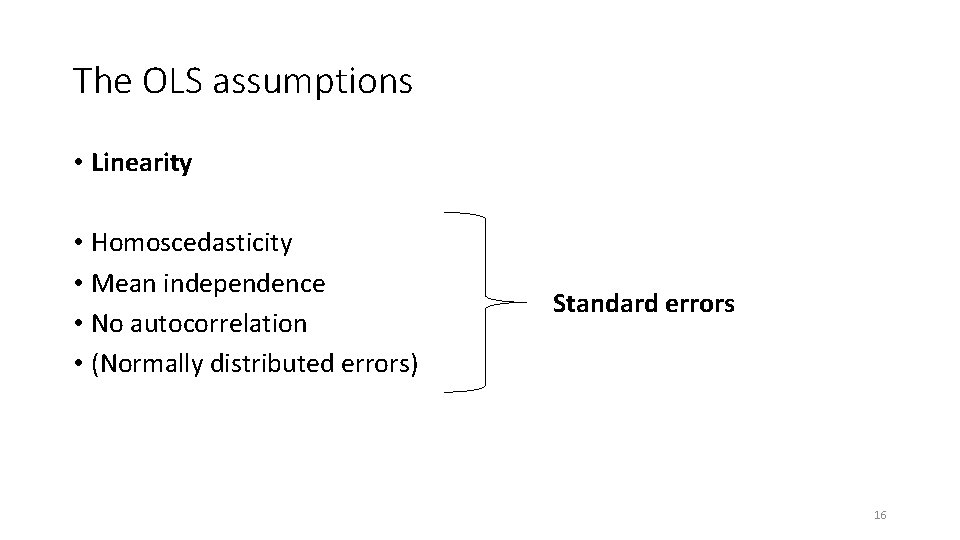
The OLS assumptions • Linearity • Homoscedasticity • Mean independence • No autocorrelation • (Normally distributed errors) Standard errors 16
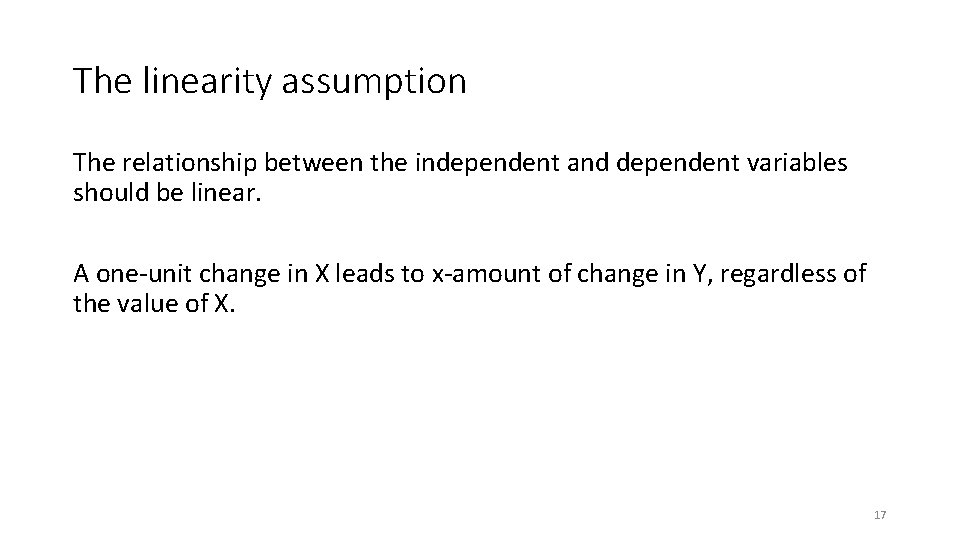
The linearity assumption The relationship between the independent and dependent variables should be linear. A one-unit change in X leads to x-amount of change in Y, regardless of the value of X. 17
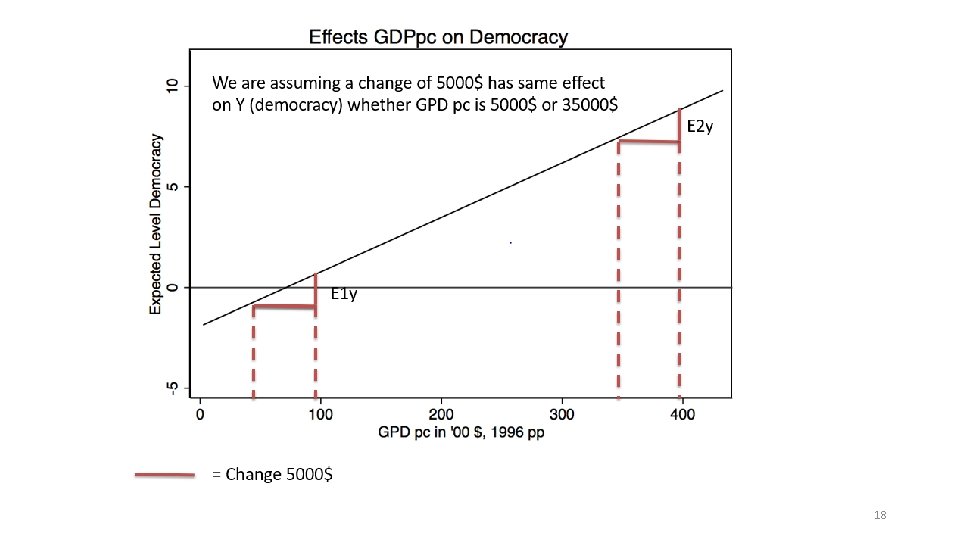
18
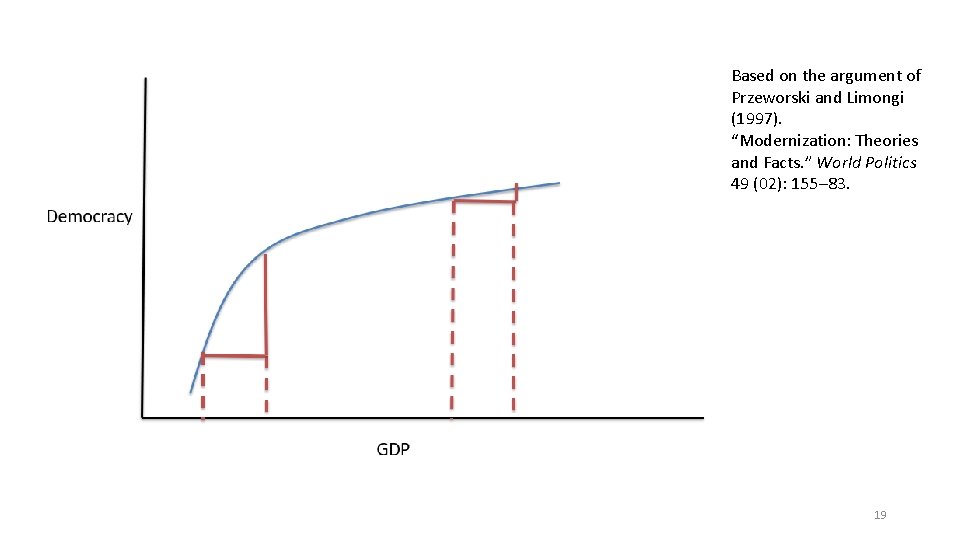
Based on the argument of Przeworski and Limongi (1997). “Modernization: Theories and Facts. ” World Politics 49 (02): 155– 83. 19
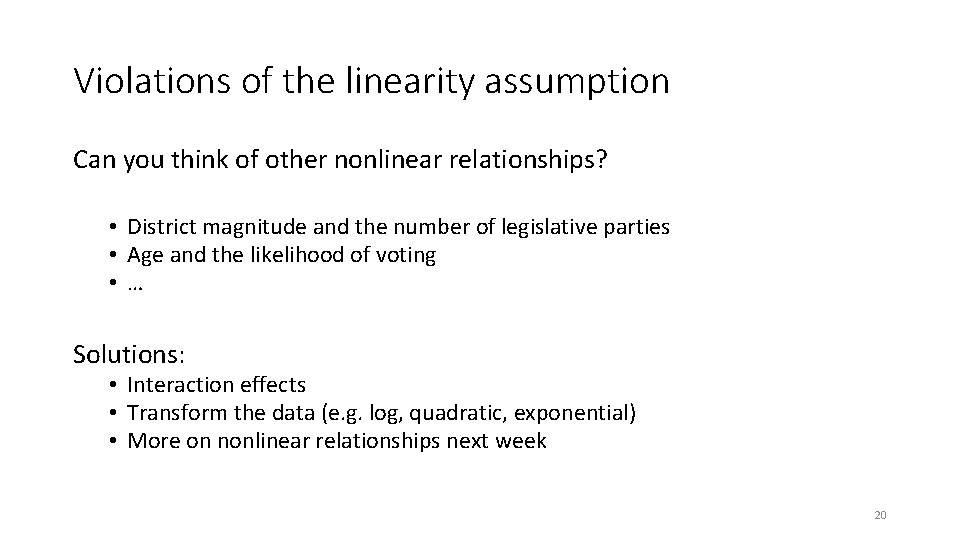
Violations of the linearity assumption Can you think of other nonlinear relationships? • District magnitude and the number of legislative parties • Age and the likelihood of voting • … Solutions: • Interaction effects • Transform the data (e. g. log, quadratic, exponential) • More on nonlinear relationships next week 20
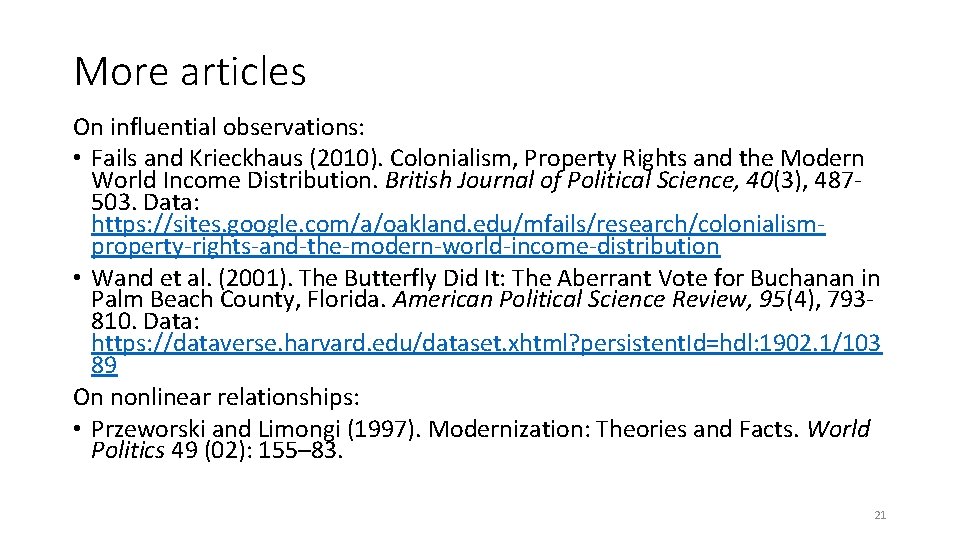
More articles On influential observations: • Fails and Krieckhaus (2010). Colonialism, Property Rights and the Modern World Income Distribution. British Journal of Political Science, 40(3), 487503. Data: https: //sites. google. com/a/oakland. edu/mfails/research/colonialismproperty-rights-and-the-modern-world-income-distribution • Wand et al. (2001). The Butterfly Did It: The Aberrant Vote for Buchanan in Palm Beach County, Florida. American Political Science Review, 95(4), 793810. Data: https: //dataverse. harvard. edu/dataset. xhtml? persistent. Id=hdl: 1902. 1/103 89 On nonlinear relationships: • Przeworski and Limongi (1997). Modernization: Theories and Facts. World Politics 49 (02): 155– 83. 21
Ols assumptions
Ols regression
Estimator
Problems with ols
Hcdsb ols
Explanatory variable definition
Mle vs ols
Ols course
Plataforma ols
Da toolset
Mitel system administration and diagnostics download
Strategy based diagnostics steps
Duct diagnostics
Customer journey diagnostics
Winloader
Standard diagnostics korea
Laser beam diagnostic
Cristina brunet
Birads categories
Cole diagnostics jobs
Spss casewise diagnostics
Sensiringe