1 0401 401 Project Management Spring 2006 Risk
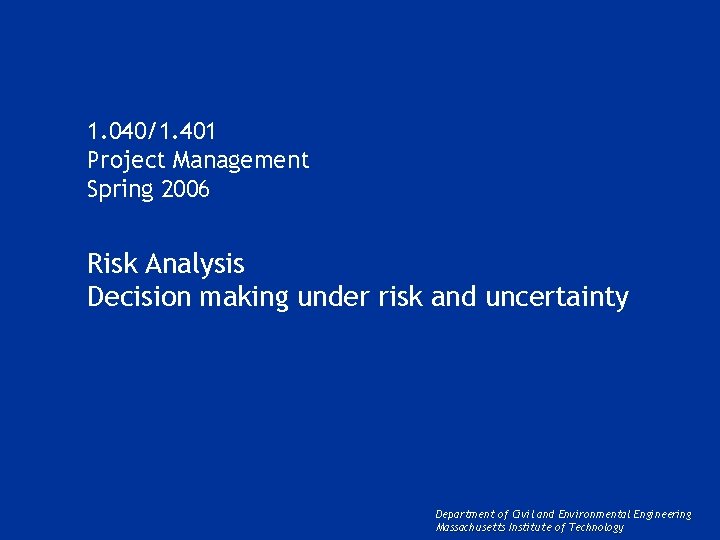
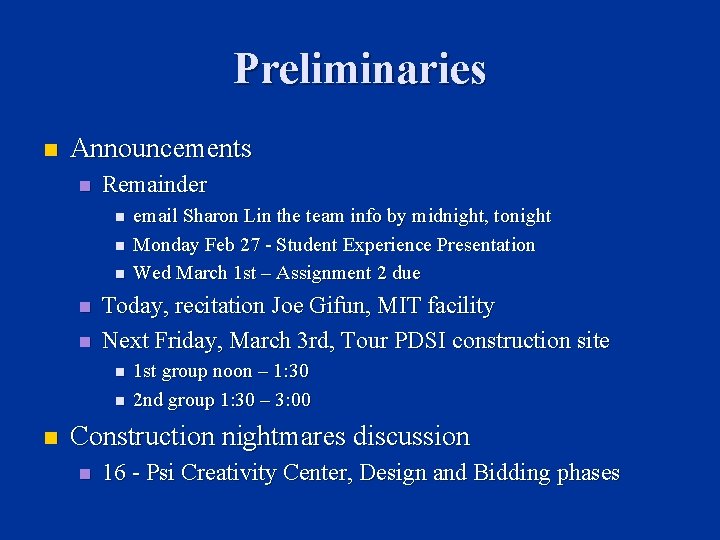
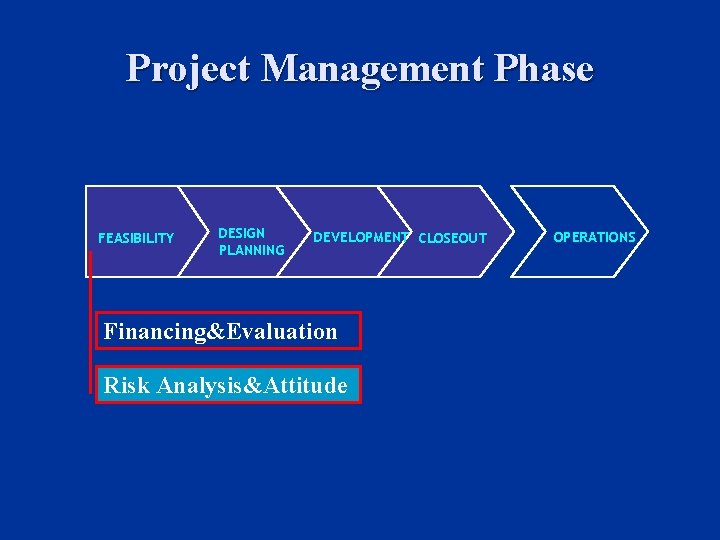
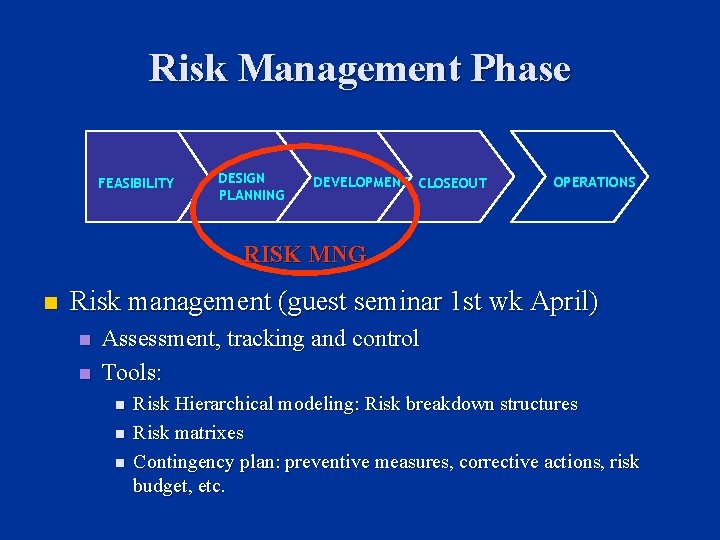
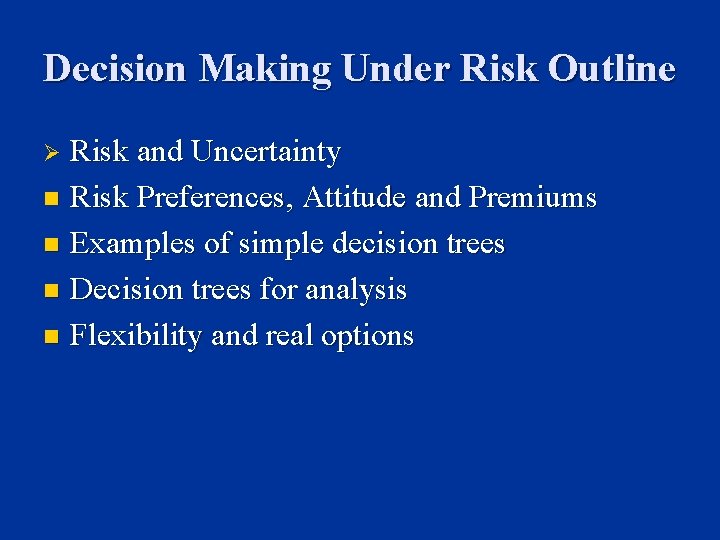
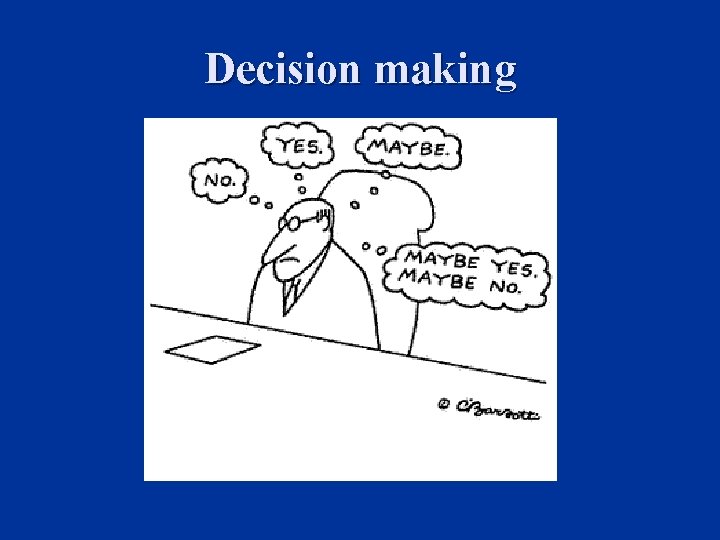
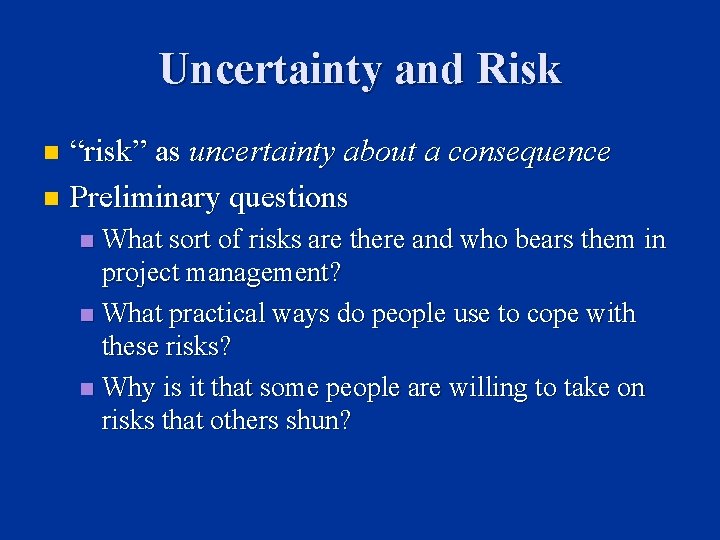
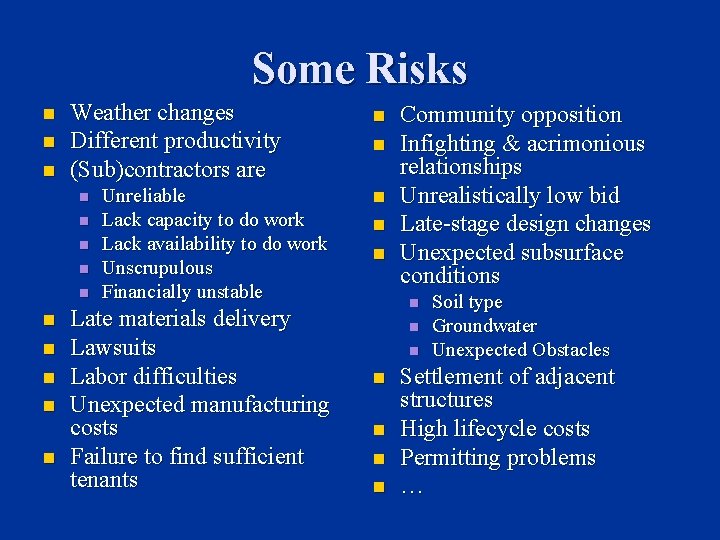
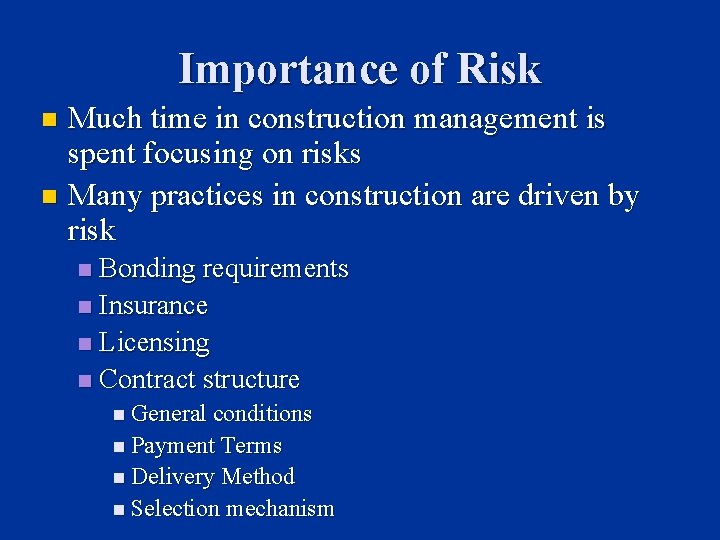
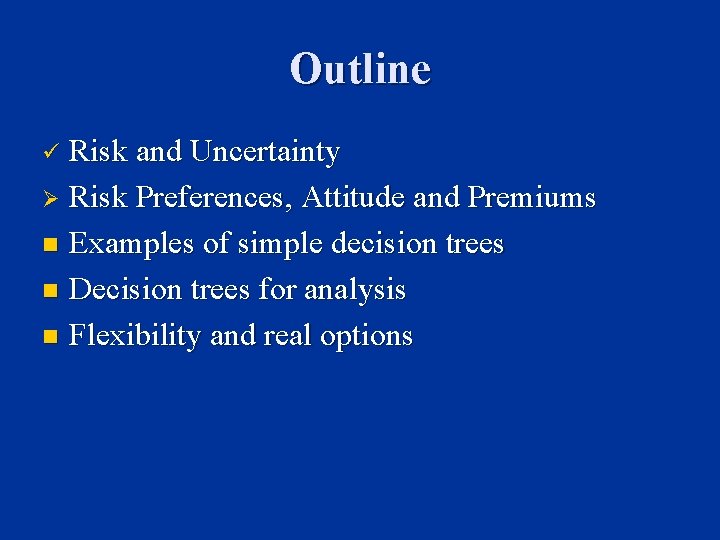
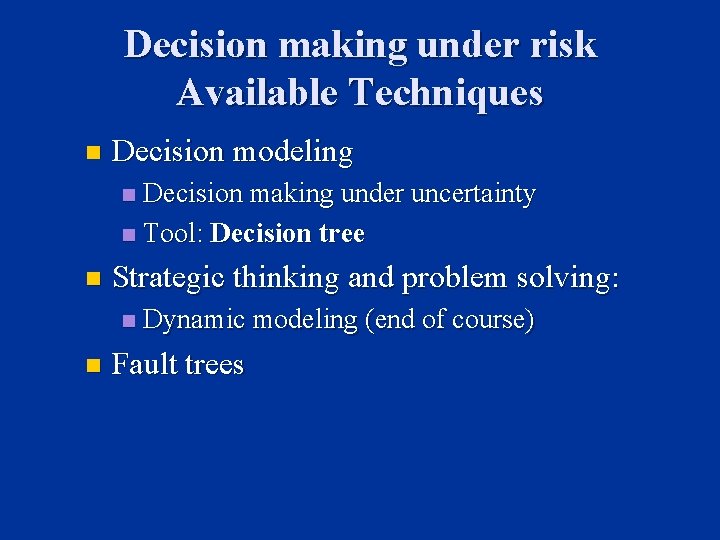
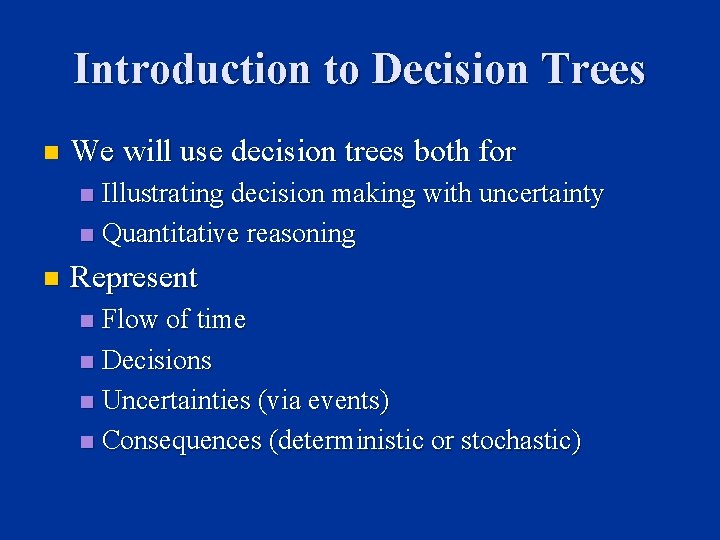
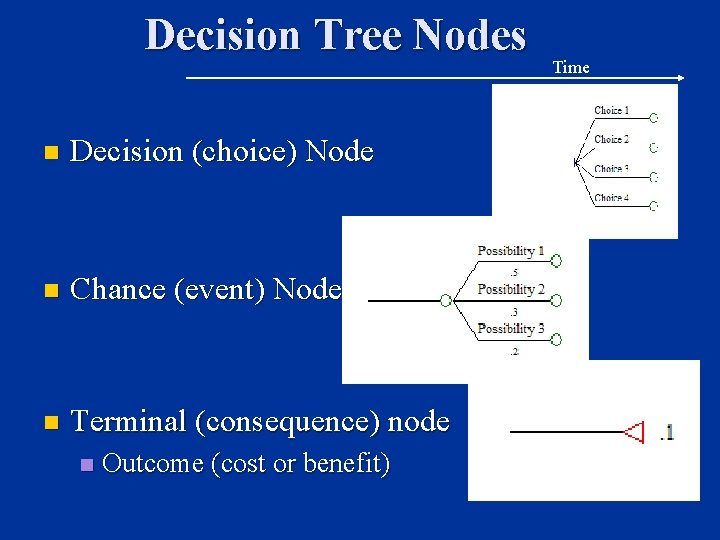
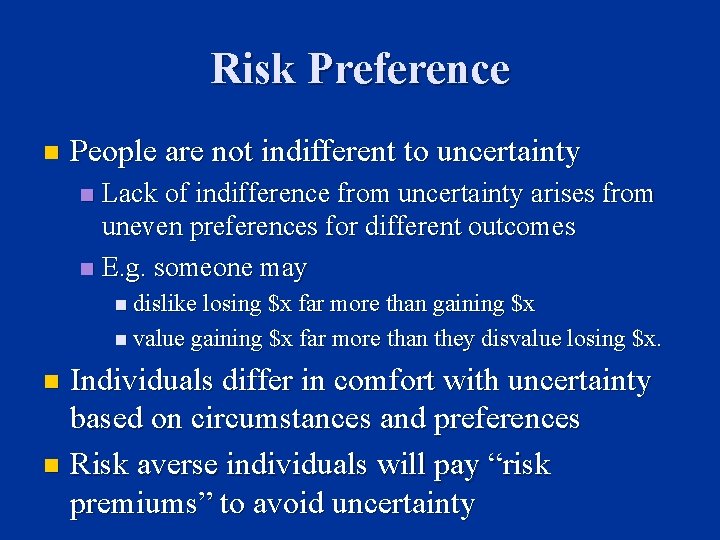
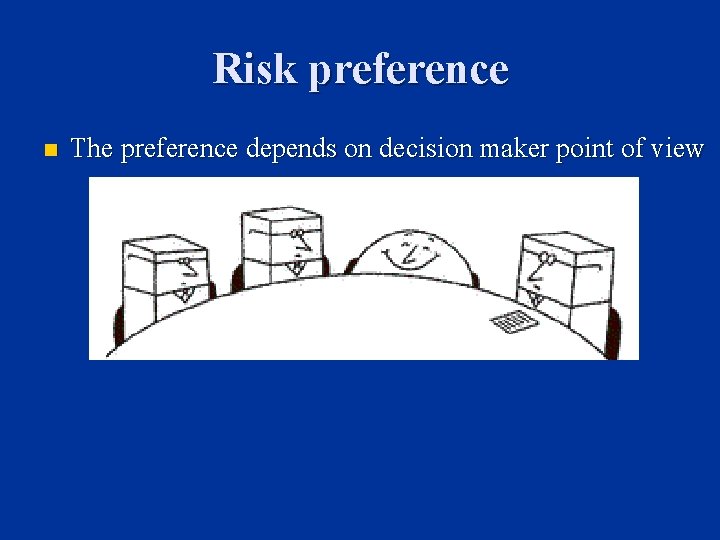
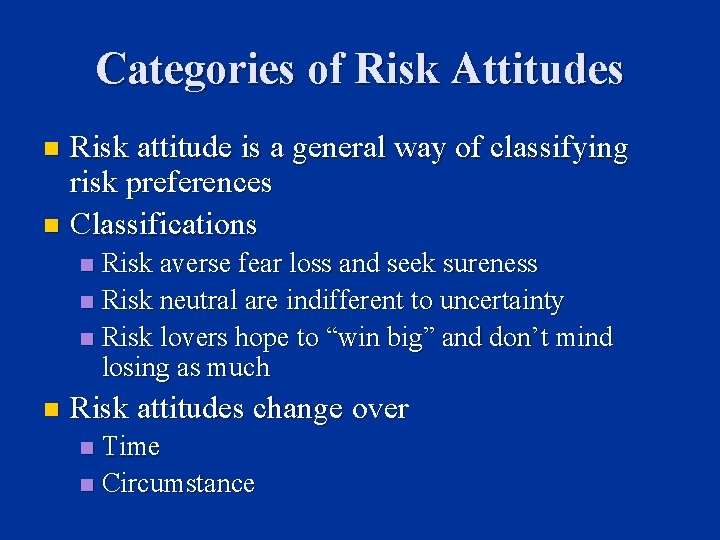
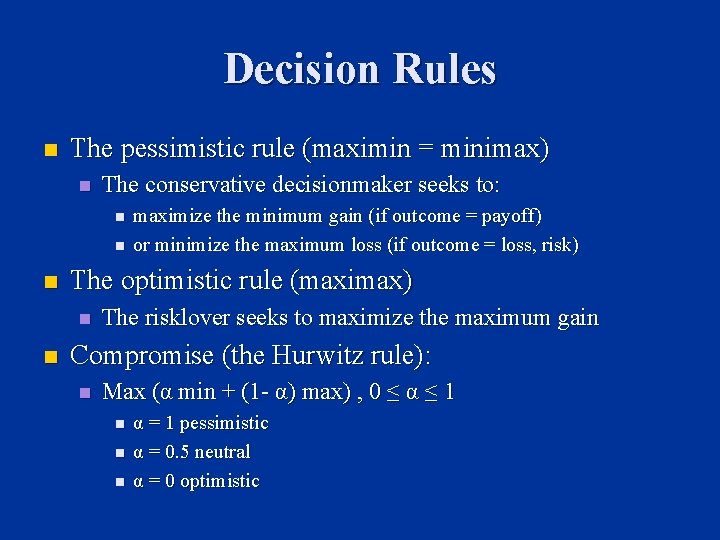
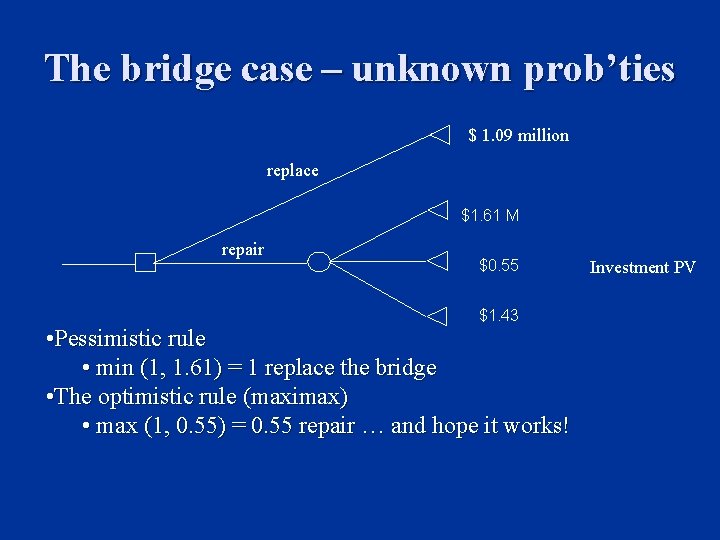
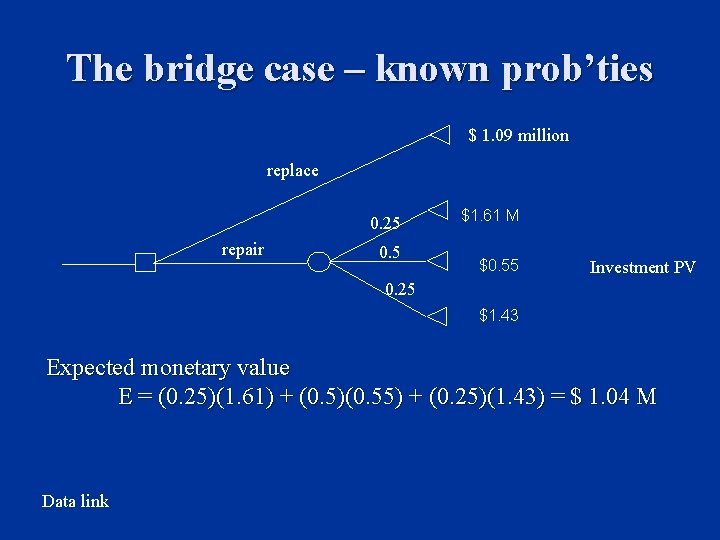
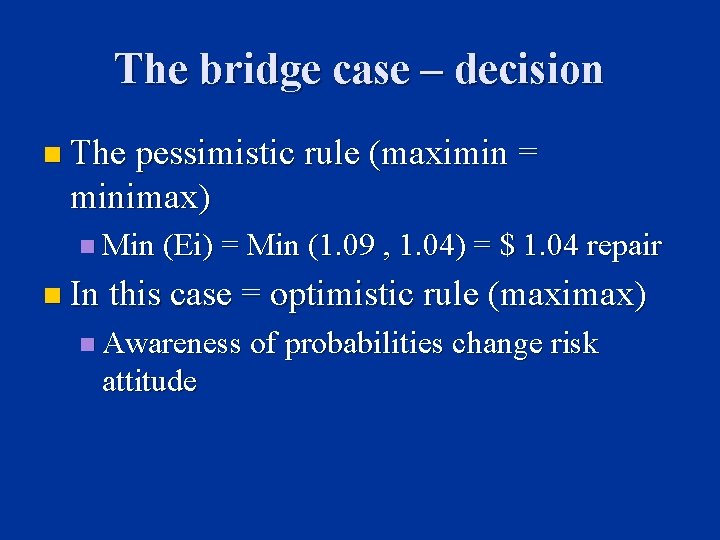
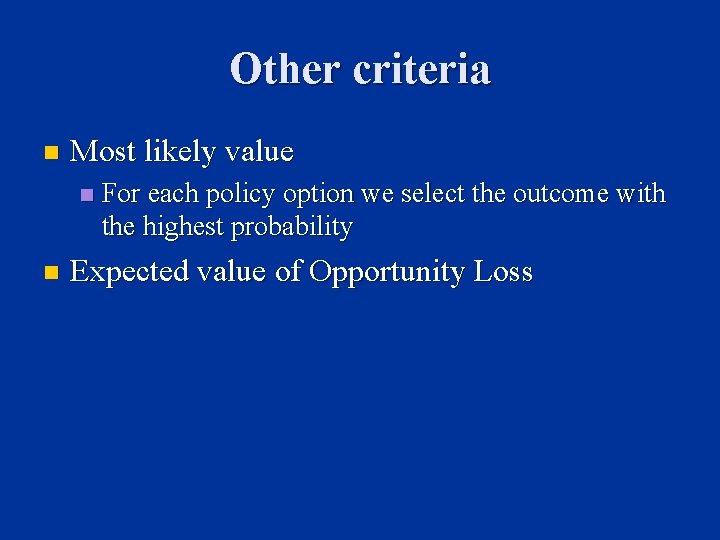
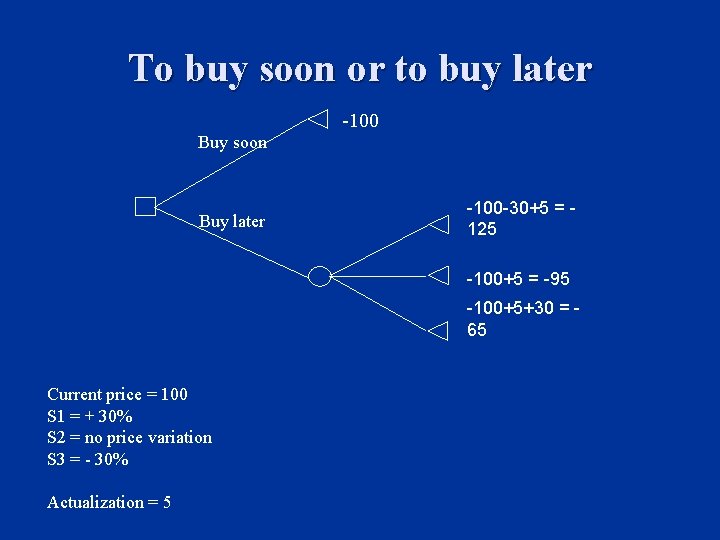
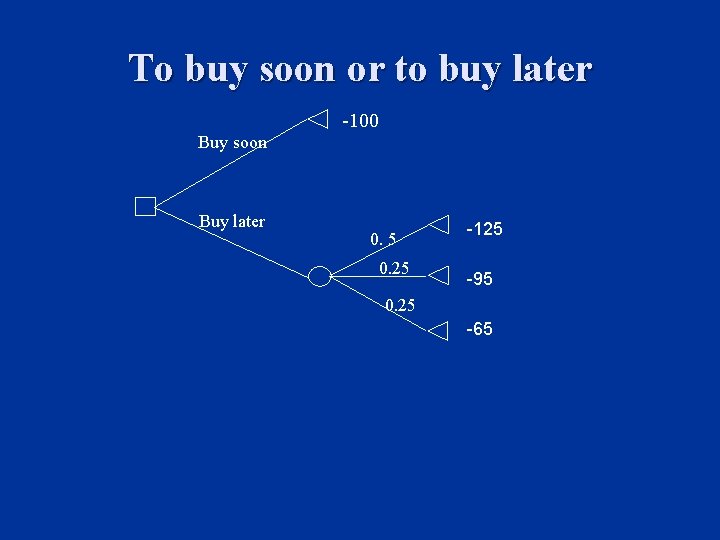
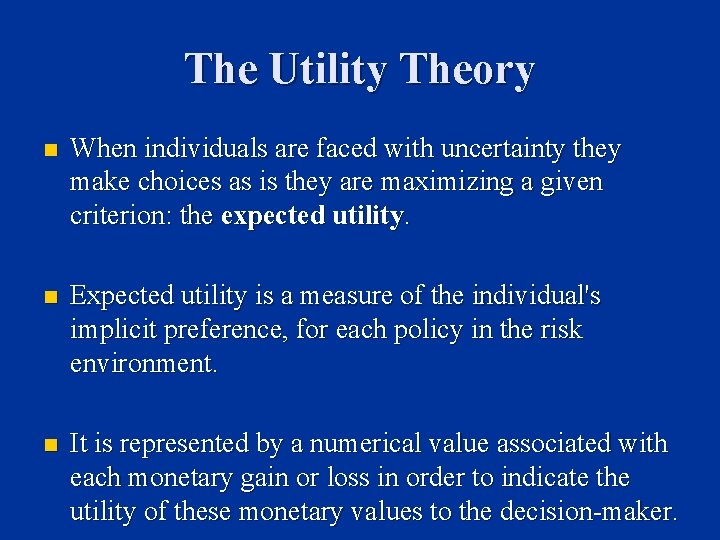
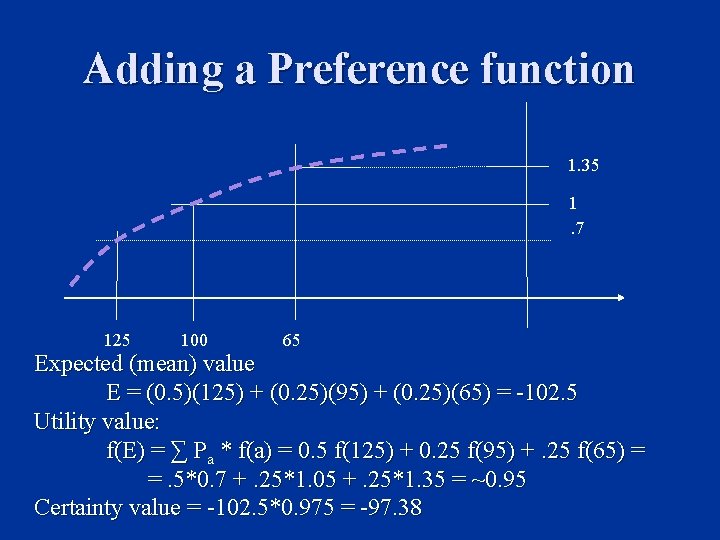
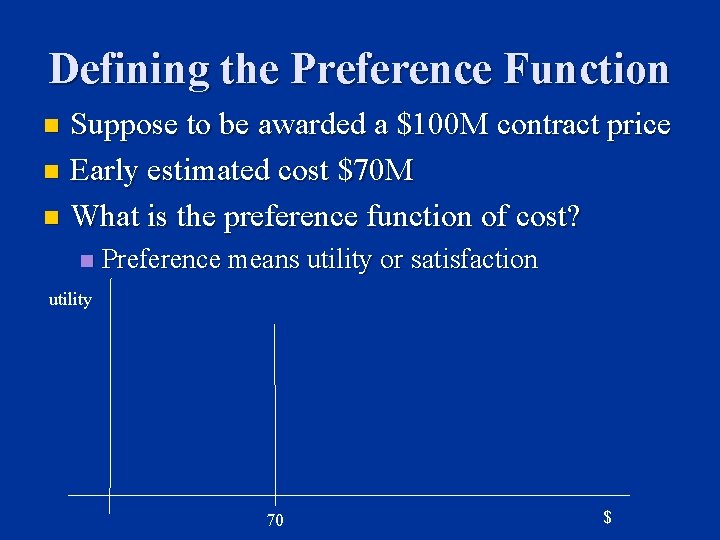
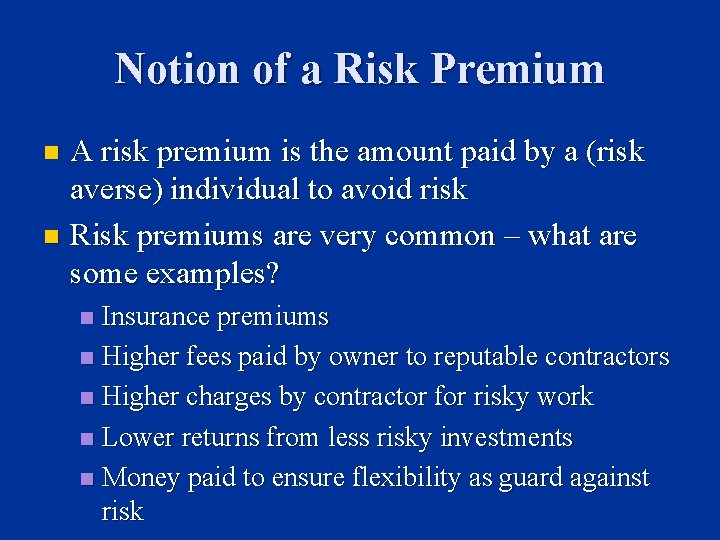
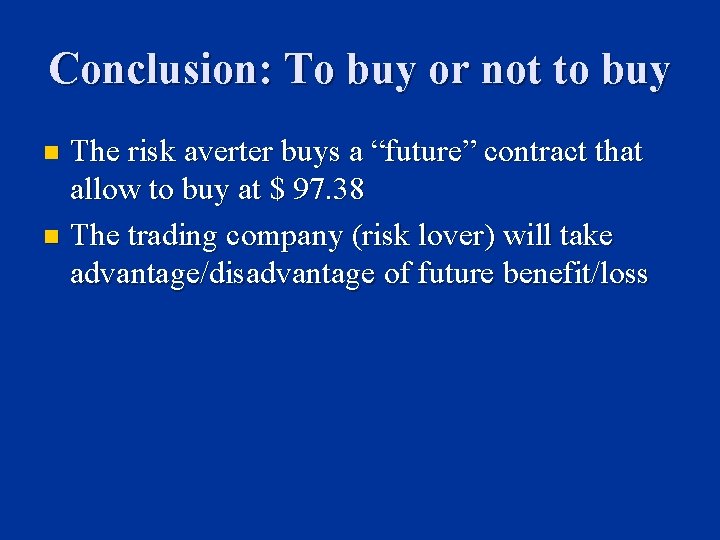
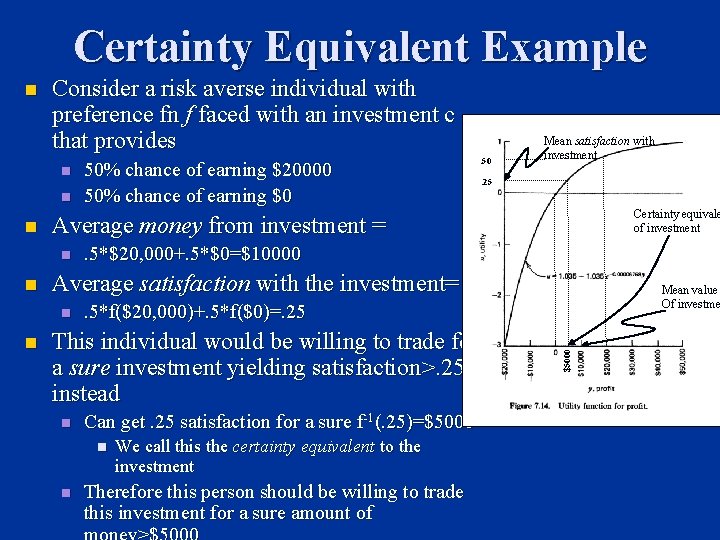
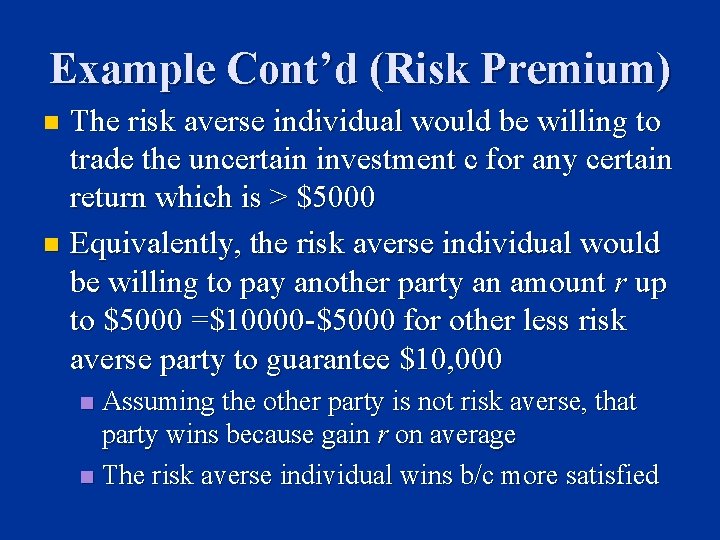
![Certainty Equivalent n More generally, consider situation in which have n n Note: E[X] Certainty Equivalent n More generally, consider situation in which have n n Note: E[X]](https://slidetodoc.com/presentation_image_h/0f0840a5be74ed3abbe338f3a375fd57/image-31.jpg)
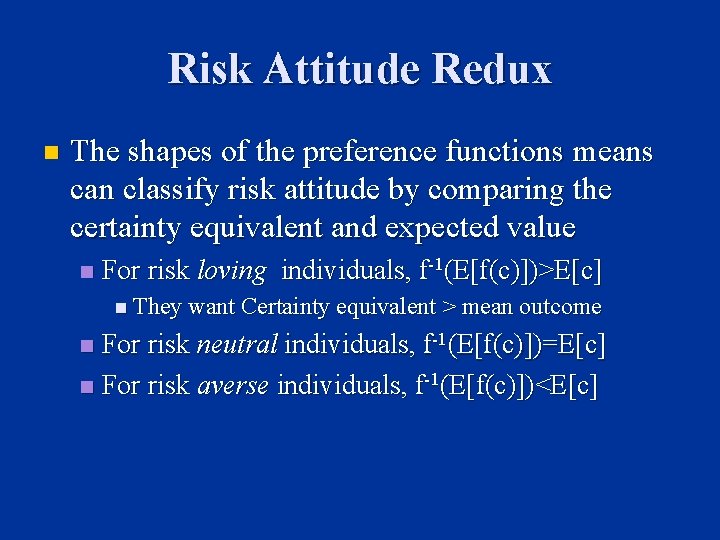
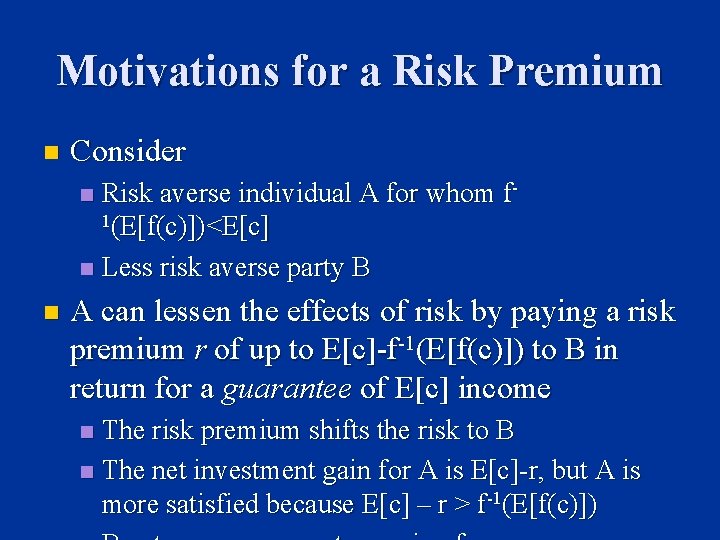
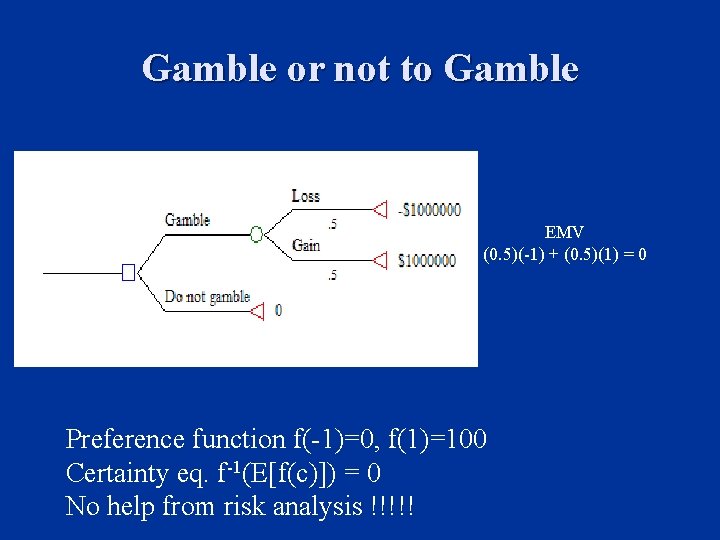
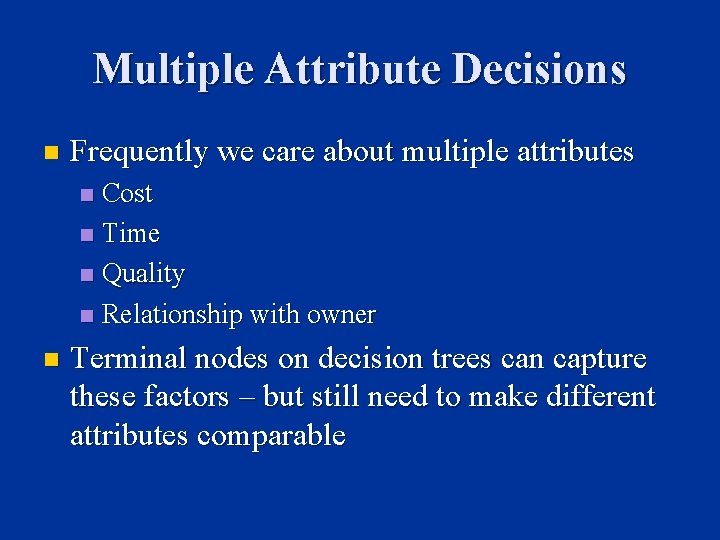
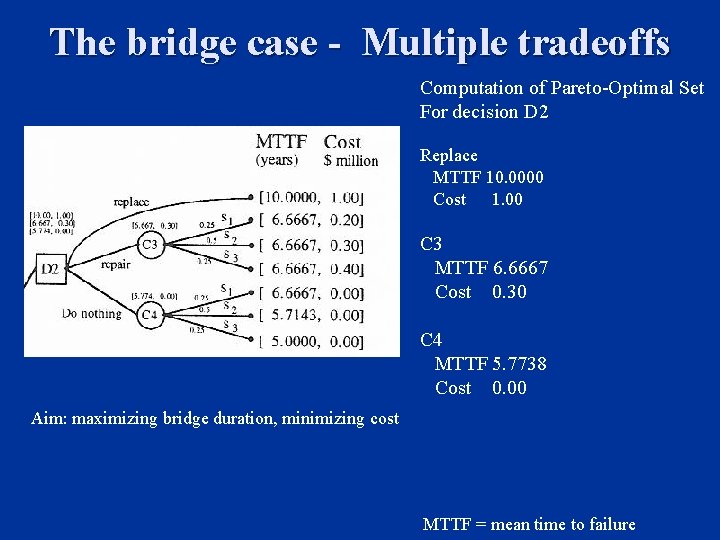
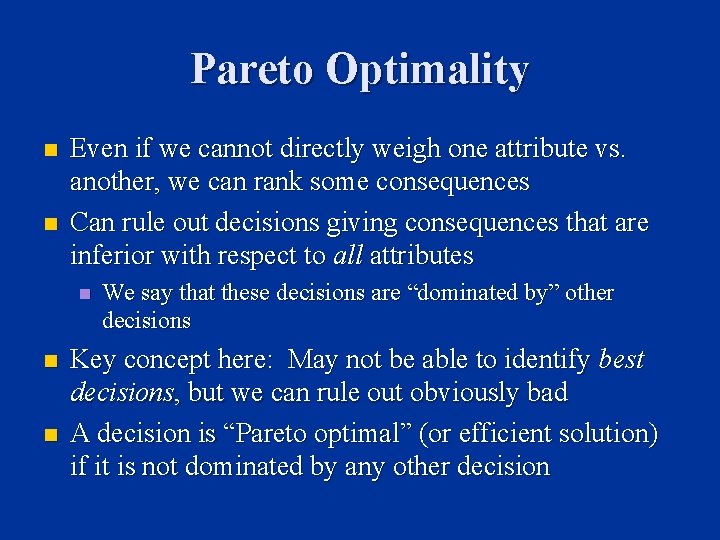
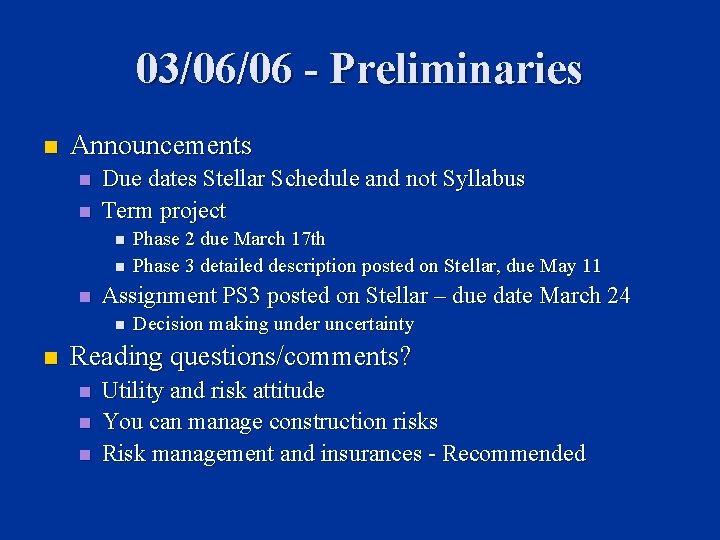
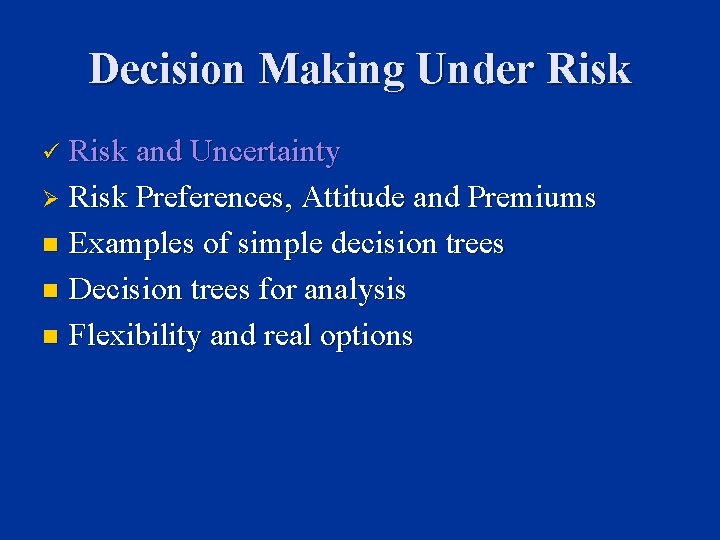
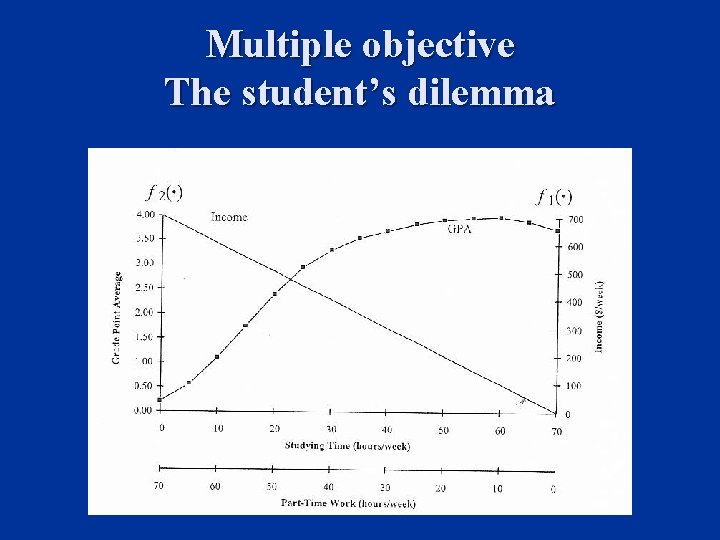
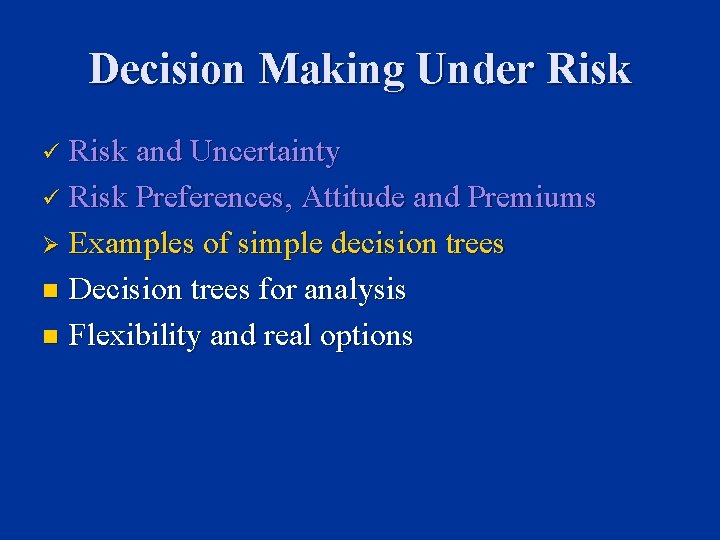
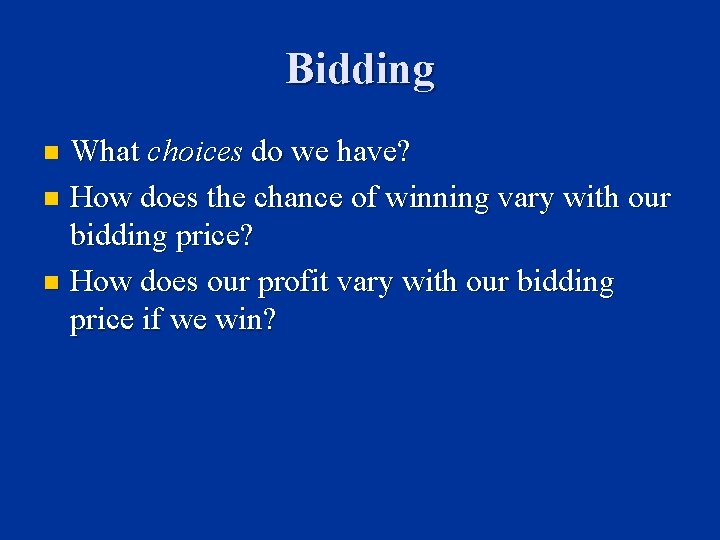
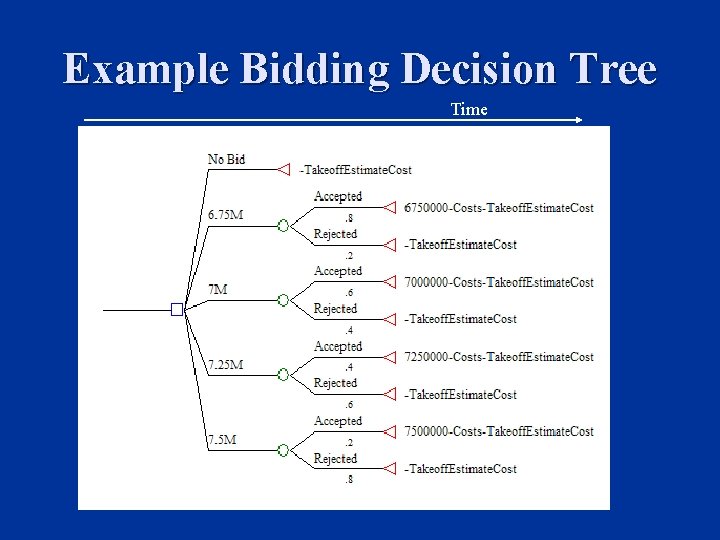
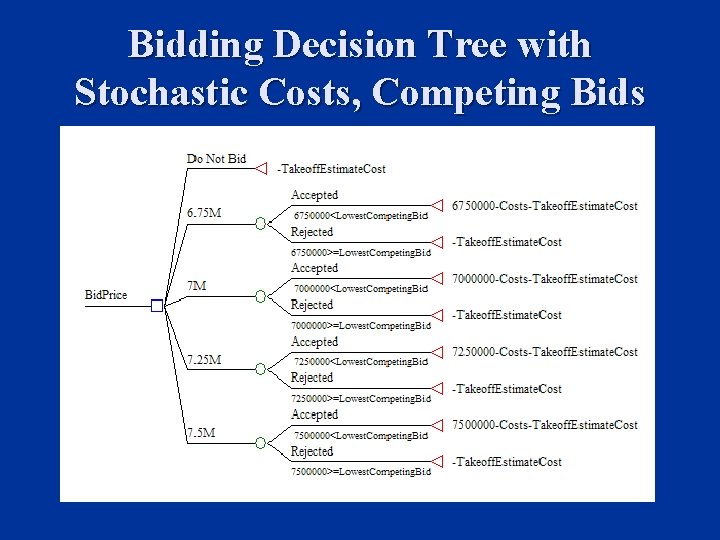
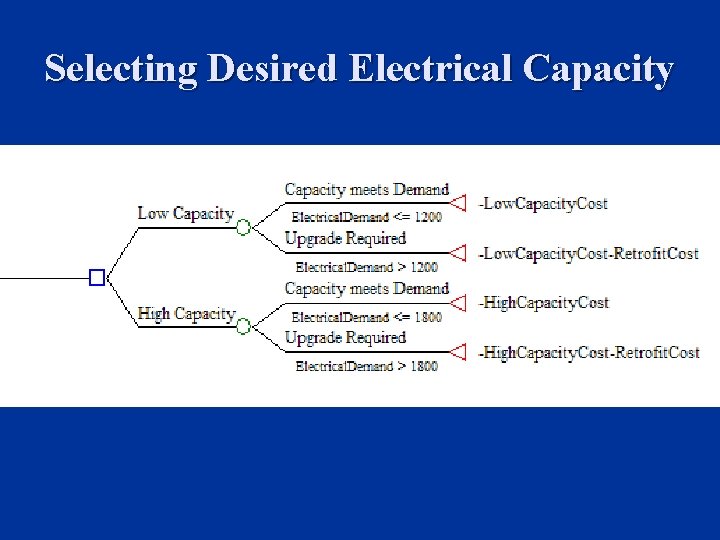
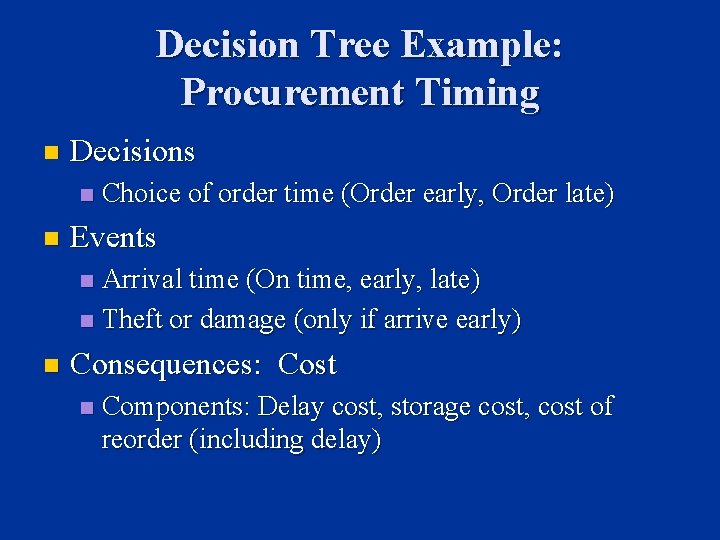
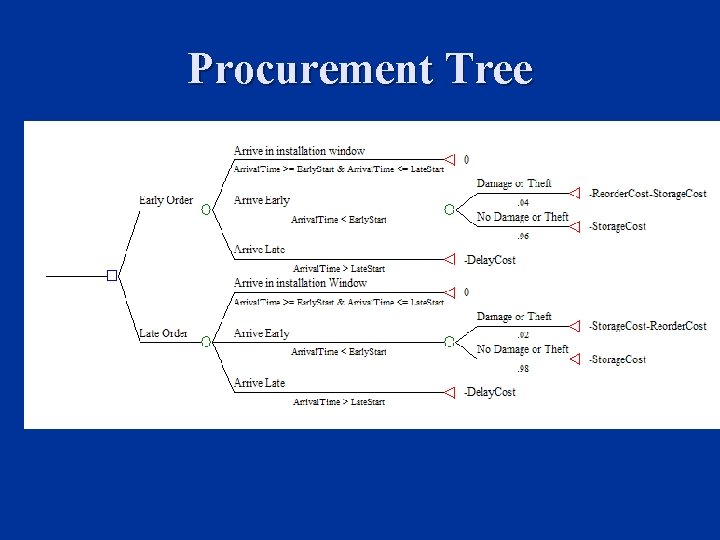
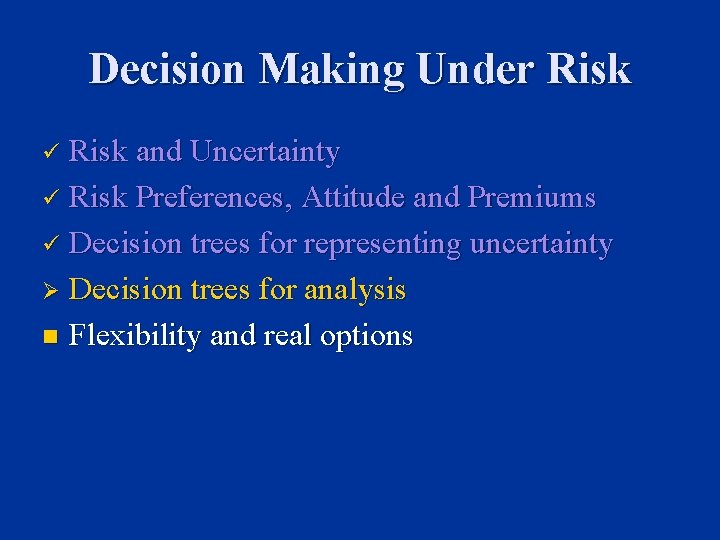
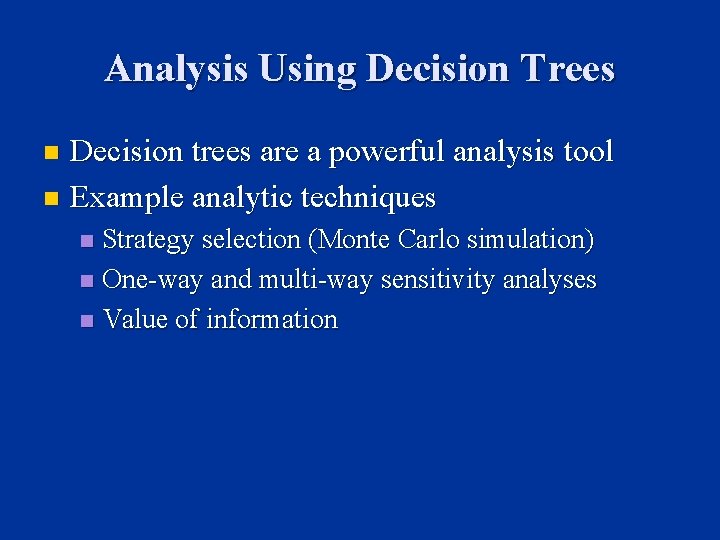
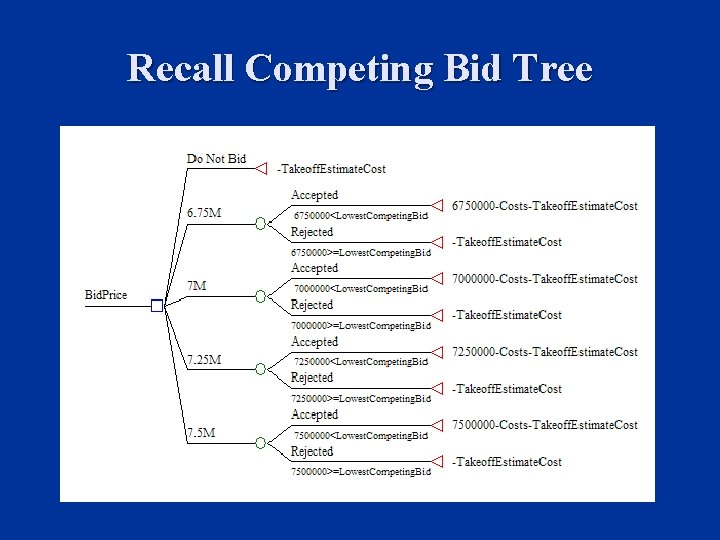
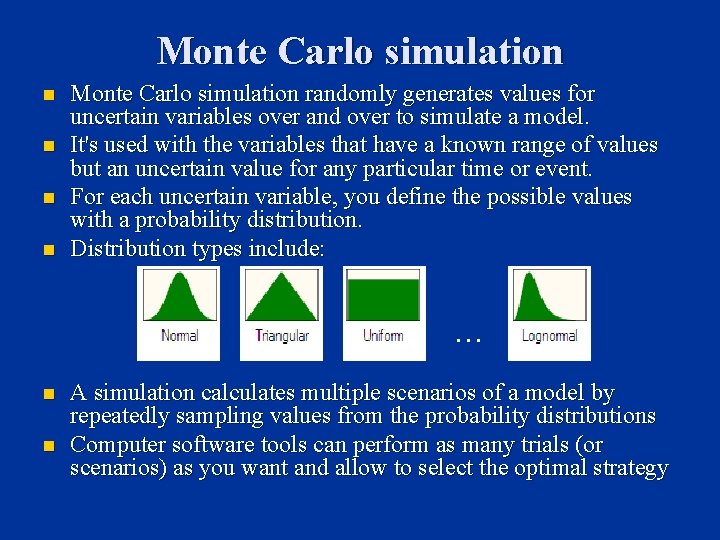
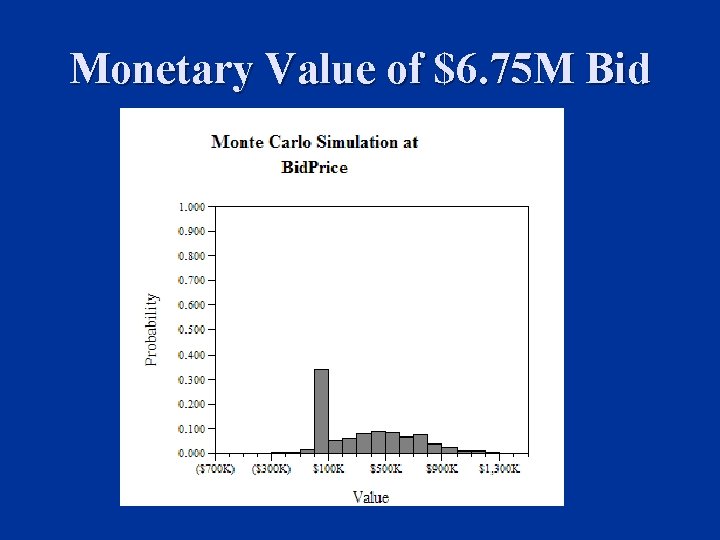
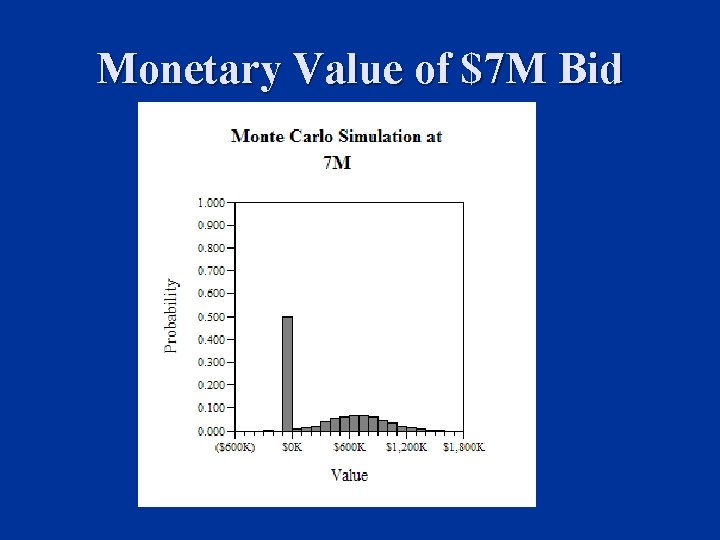
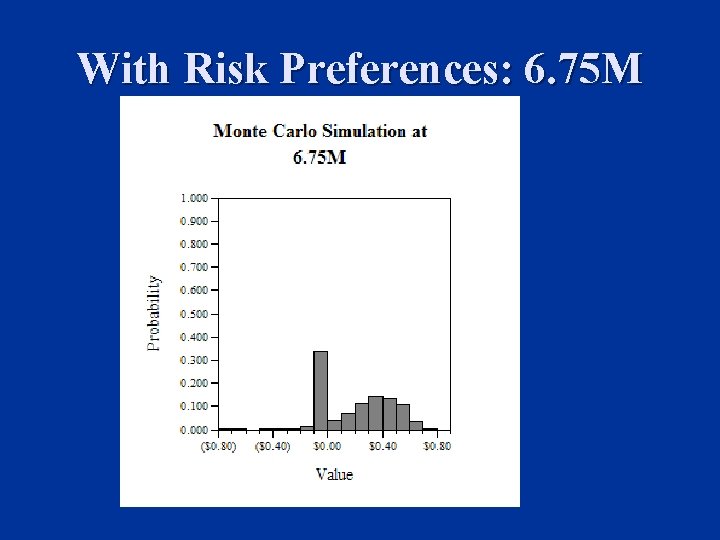
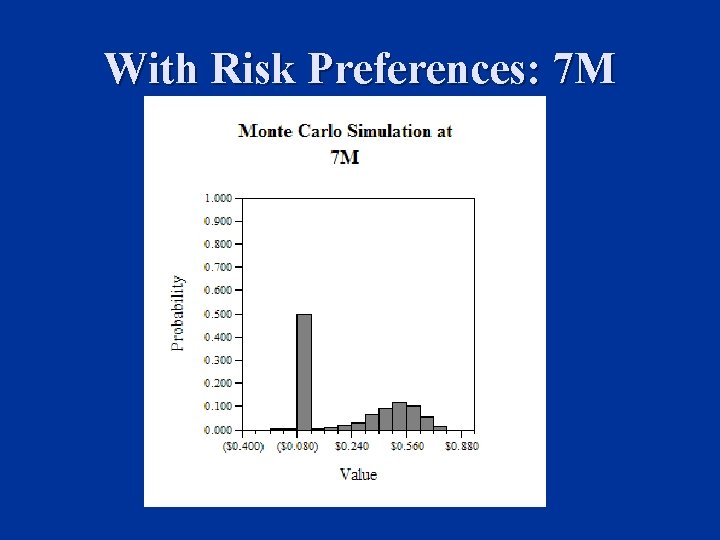
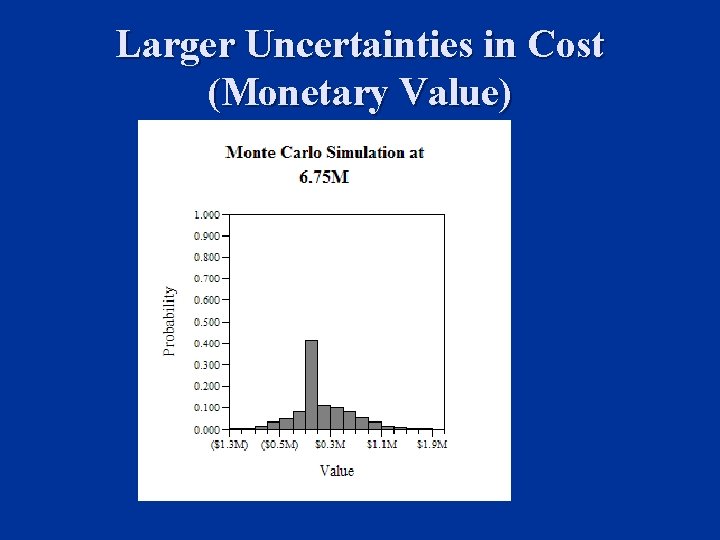
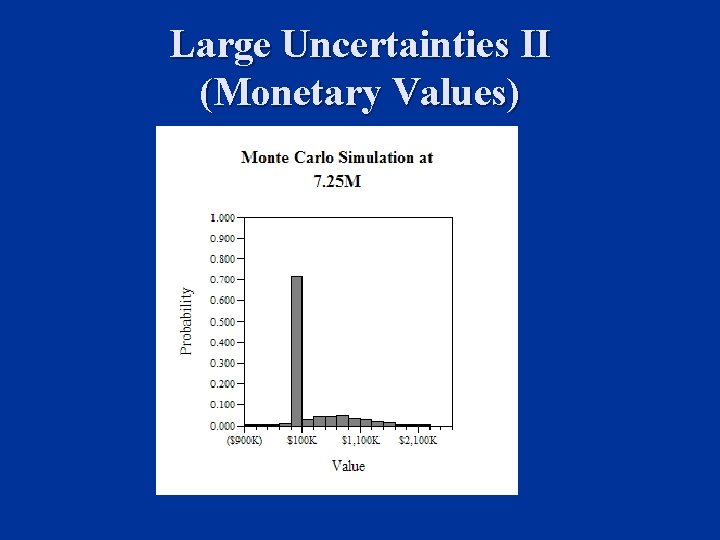
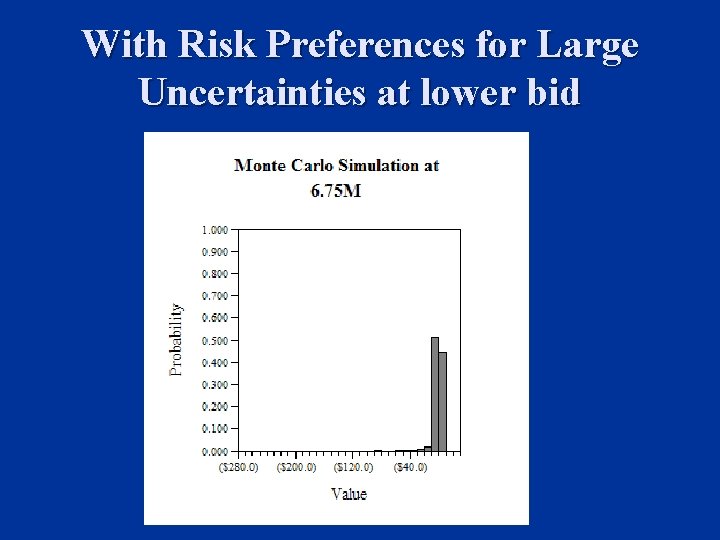
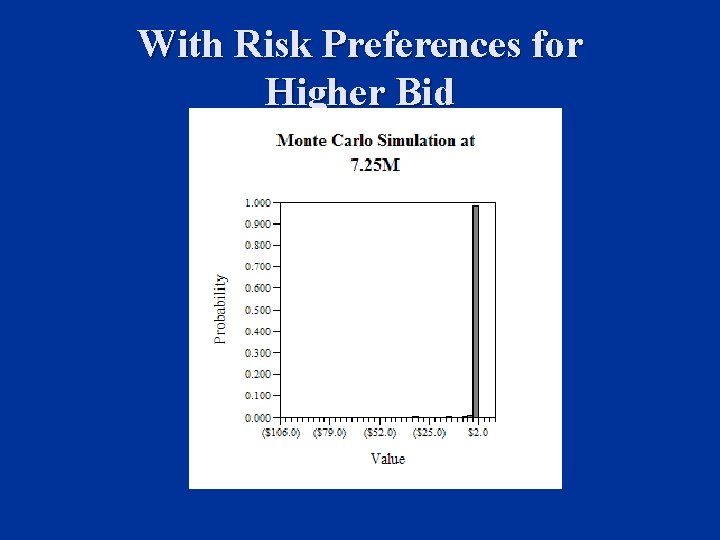
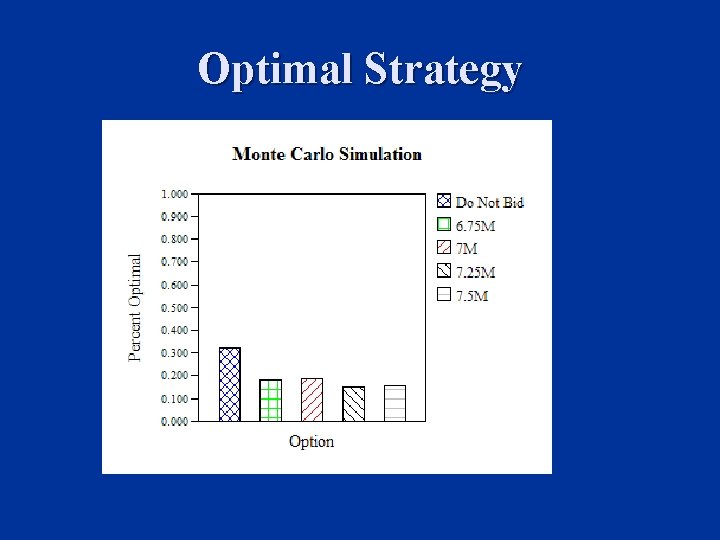
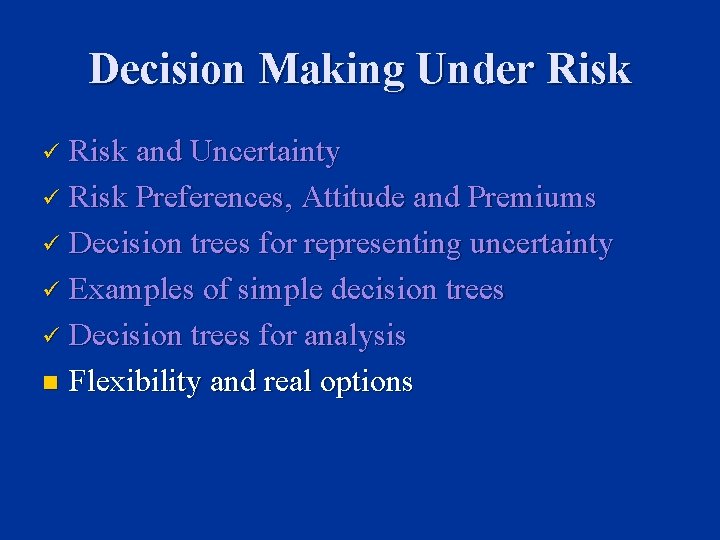
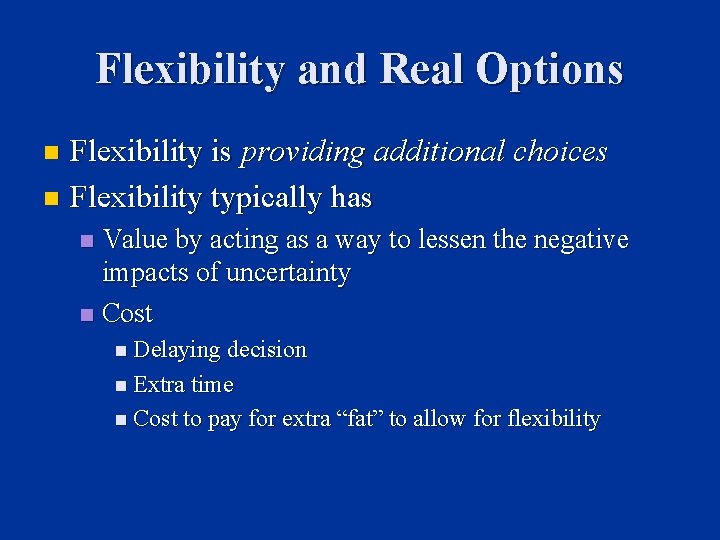
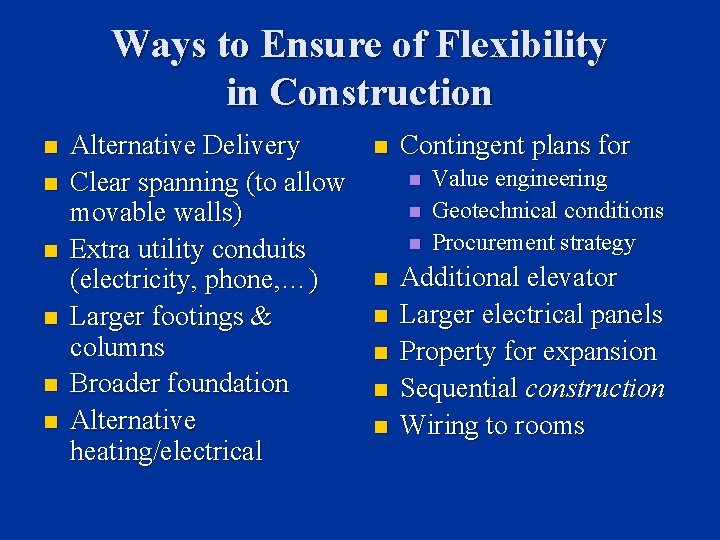
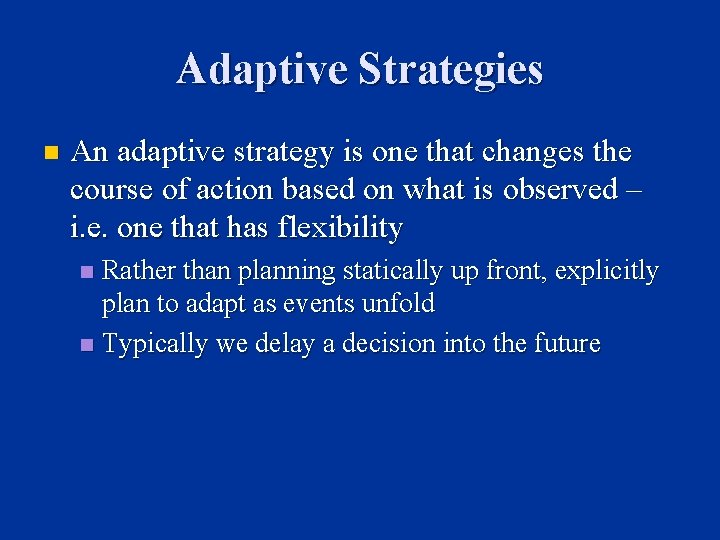
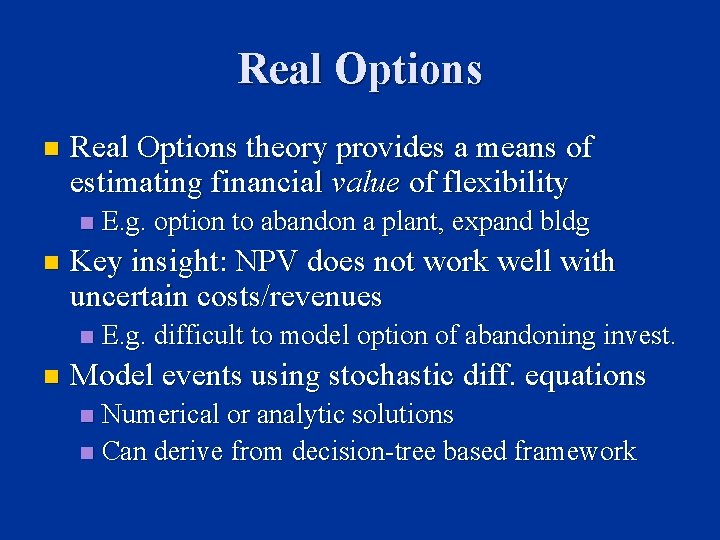
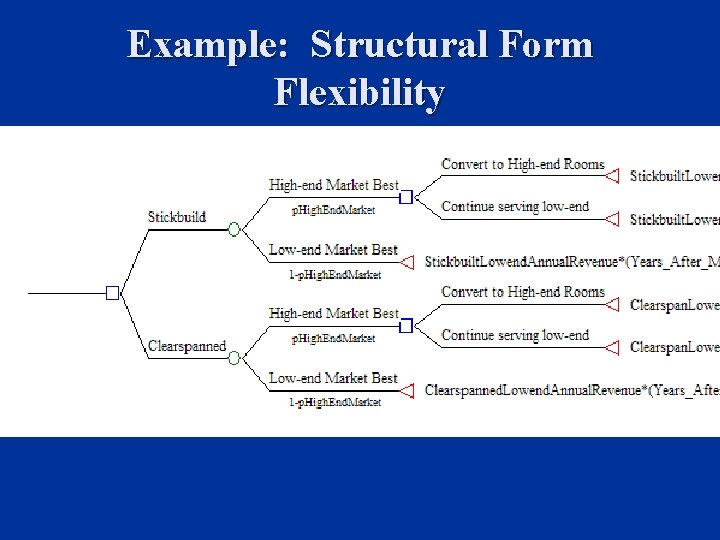
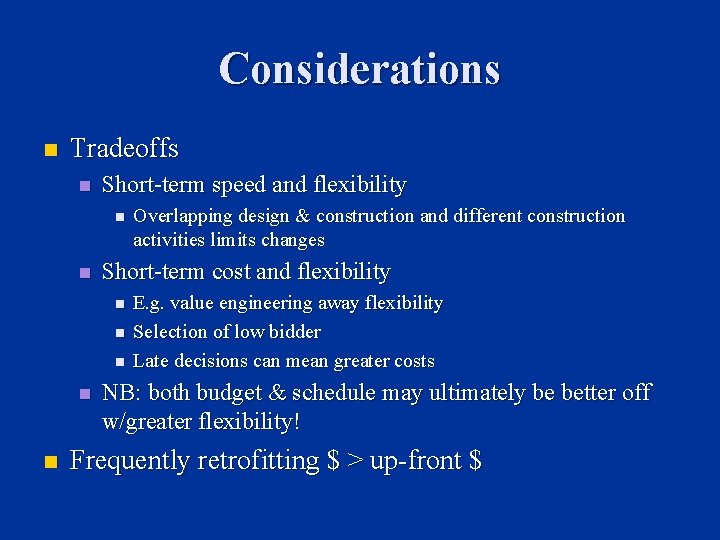
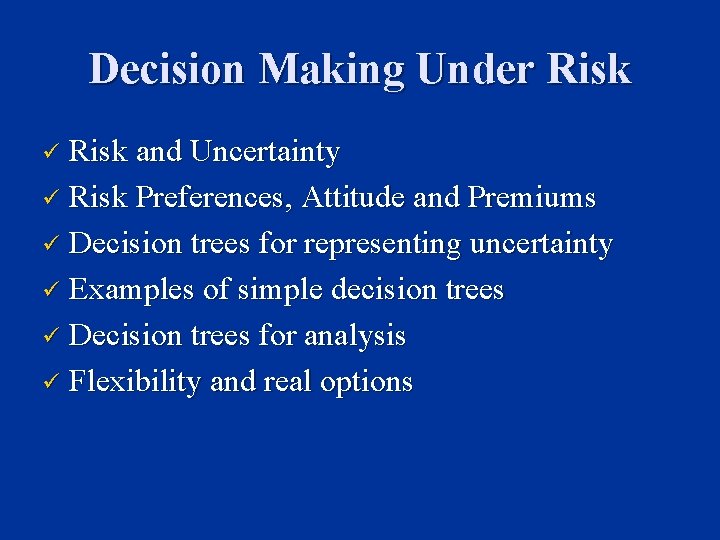
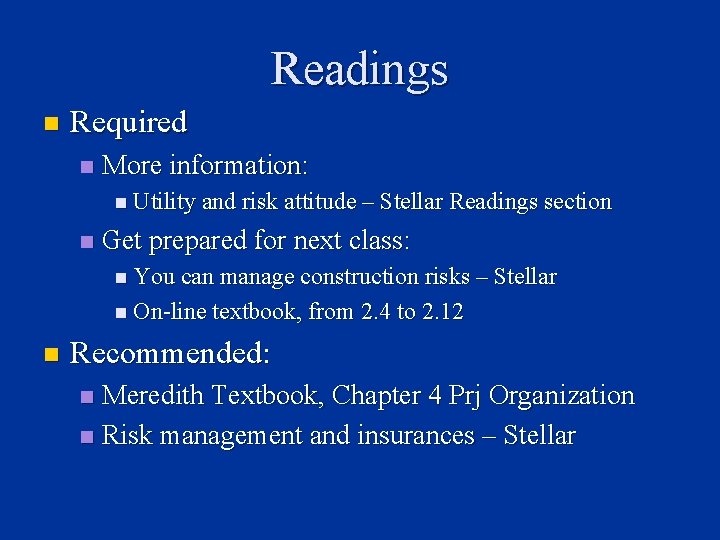
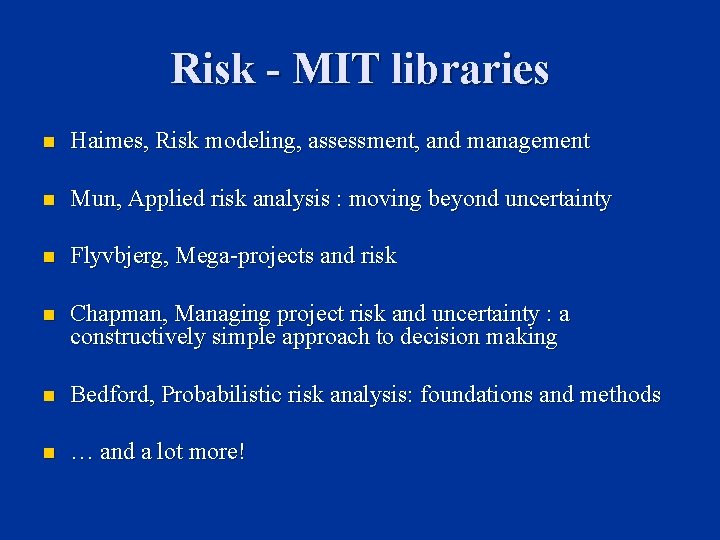
- Slides: 70
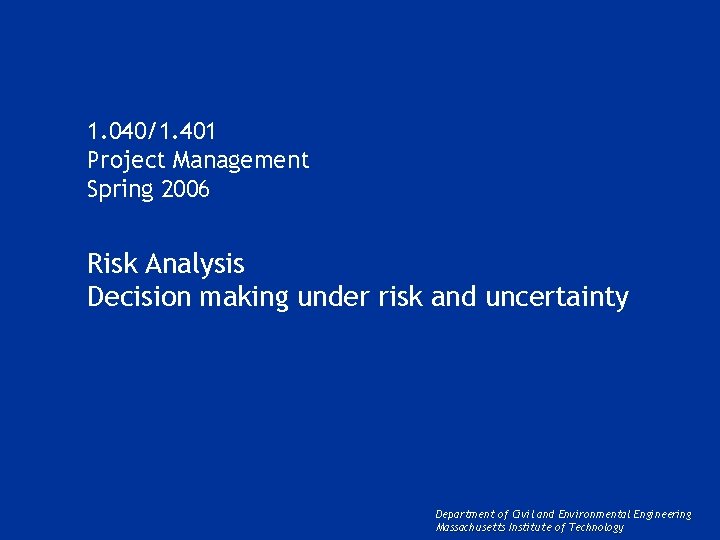
1. 040/1. 401 Project Management Spring 2006 Risk Analysis Decision making under risk and uncertainty Department of Civil and Environmental Engineering Massachusetts Institute of Technology
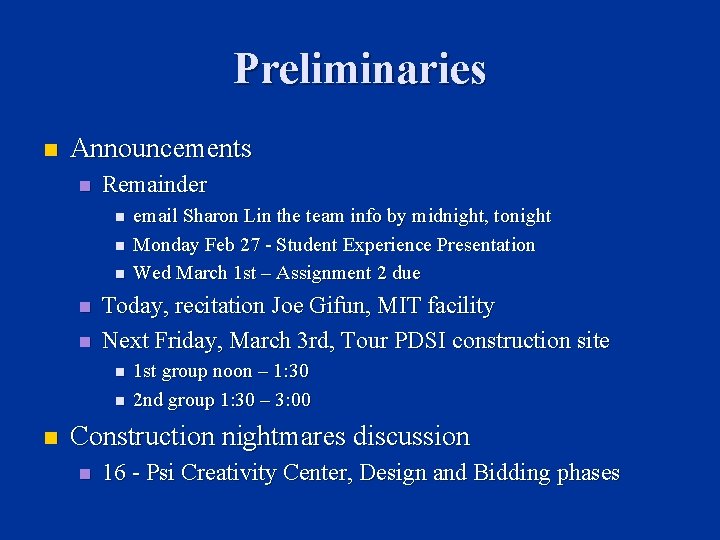
Preliminaries n Announcements n Remainder n n n Today, recitation Joe Gifun, MIT facility Next Friday, March 3 rd, Tour PDSI construction site n n n email Sharon Lin the team info by midnight, tonight Monday Feb 27 - Student Experience Presentation Wed March 1 st – Assignment 2 due 1 st group noon – 1: 30 2 nd group 1: 30 – 3: 00 Construction nightmares discussion n 16 - Psi Creativity Center, Design and Bidding phases
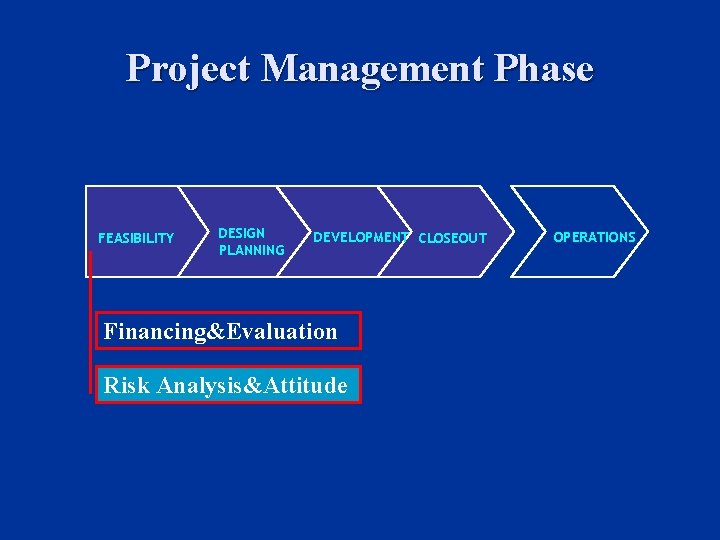
Project Management Phase FEASIBILITY DESIGN PLANNING DEVELOPMENT CLOSEOUT Financing&Evaluation Risk Analysis&Attitude OPERATIONS
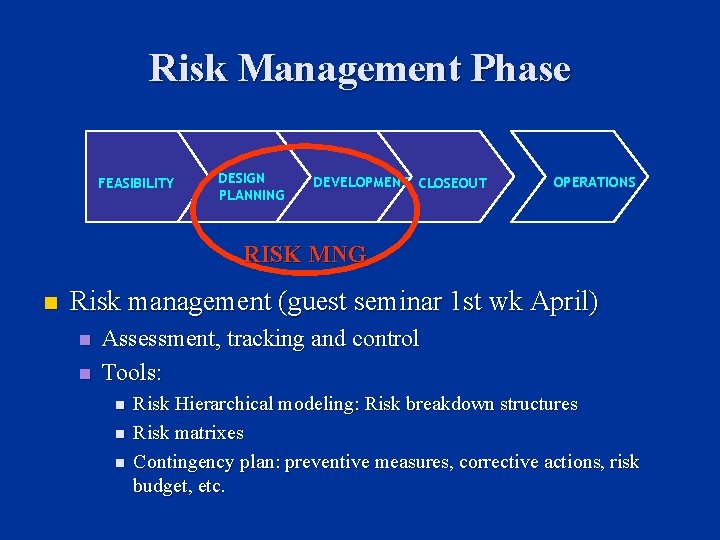
Risk Management Phase FEASIBILITY DESIGN PLANNING DEVELOPMENT CLOSEOUT OPERATIONS RISK MNG n Risk management (guest seminar 1 st wk April) n n Assessment, tracking and control Tools: n n n Risk Hierarchical modeling: Risk breakdown structures Risk matrixes Contingency plan: preventive measures, corrective actions, risk budget, etc.
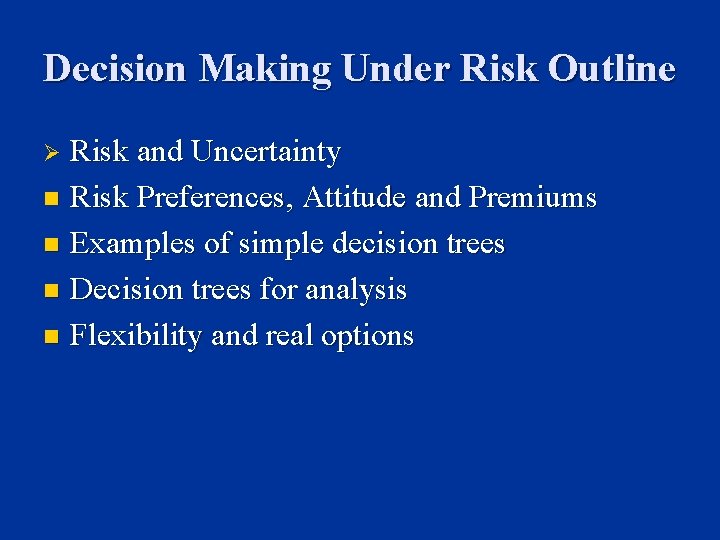
Decision Making Under Risk Outline Risk and Uncertainty n Risk Preferences, Attitude and Premiums n Examples of simple decision trees n Decision trees for analysis n Flexibility and real options Ø
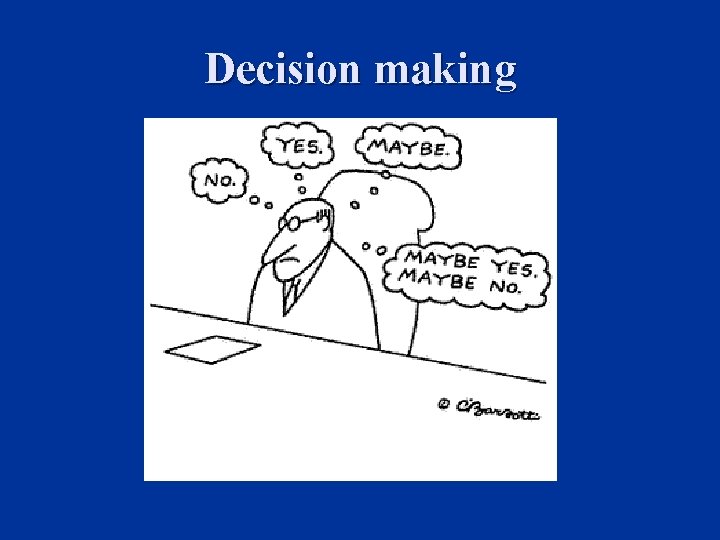
Decision making
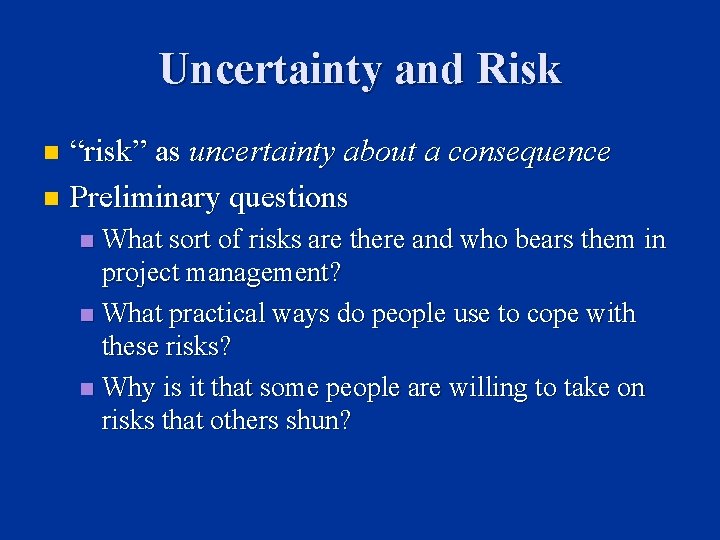
Uncertainty and Risk “risk” as uncertainty about a consequence n Preliminary questions n What sort of risks are there and who bears them in project management? n What practical ways do people use to cope with these risks? n Why is it that some people are willing to take on risks that others shun? n
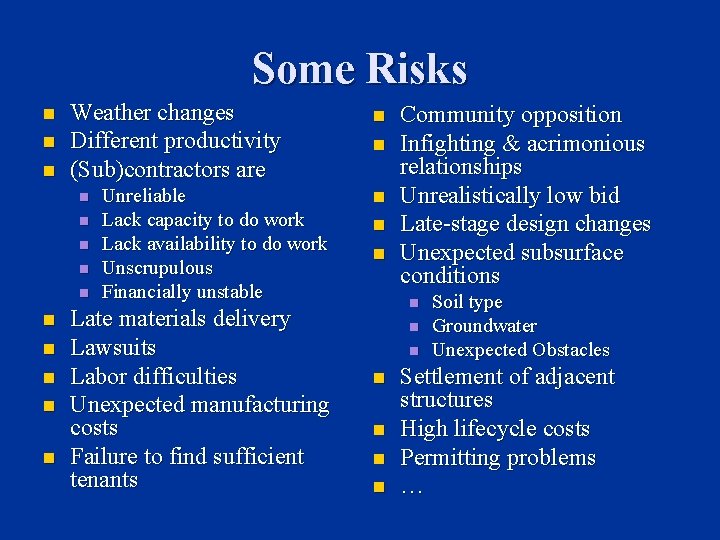
Some Risks n n n Weather changes Different productivity (Sub)contractors are n n n n n Unreliable Lack capacity to do work Lack availability to do work Unscrupulous Financially unstable Late materials delivery Lawsuits Labor difficulties Unexpected manufacturing costs Failure to find sufficient tenants n n n Community opposition Infighting & acrimonious relationships Unrealistically low bid Late-stage design changes Unexpected subsurface conditions n n n n Soil type Groundwater Unexpected Obstacles Settlement of adjacent structures High lifecycle costs Permitting problems …
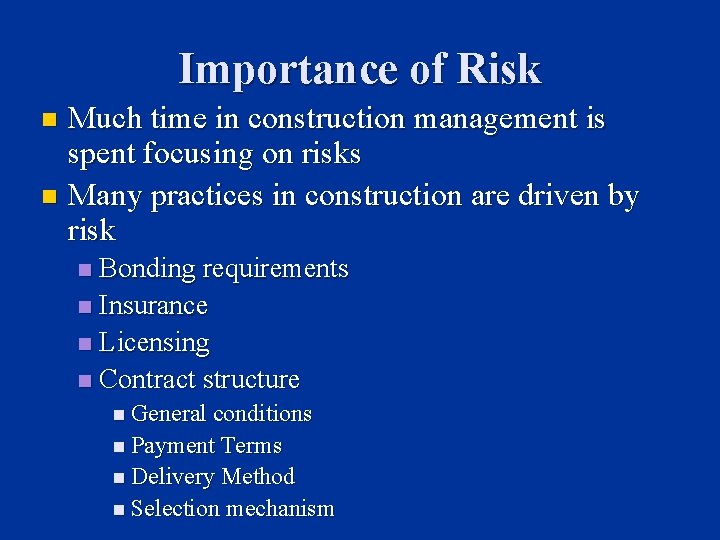
Importance of Risk Much time in construction management is spent focusing on risks n Many practices in construction are driven by risk n Bonding requirements n Insurance n Licensing n Contract structure n n General conditions n Payment Terms n Delivery Method n Selection mechanism
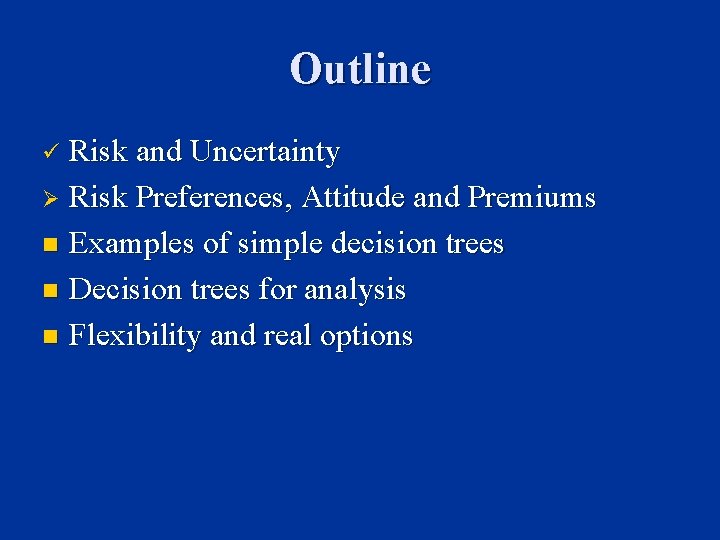
Outline Risk and Uncertainty Ø Risk Preferences, Attitude and Premiums n Examples of simple decision trees n Decision trees for analysis n Flexibility and real options ü
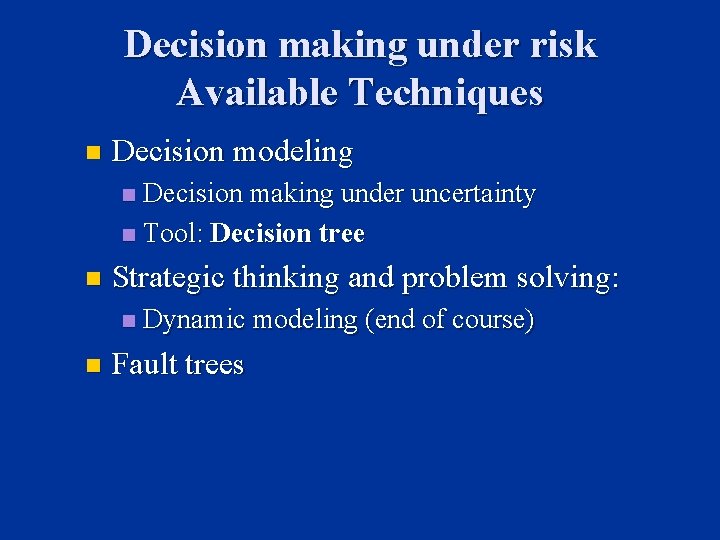
Decision making under risk Available Techniques n Decision modeling Decision making under uncertainty n Tool: Decision tree n n Strategic thinking and problem solving: n n Dynamic modeling (end of course) Fault trees
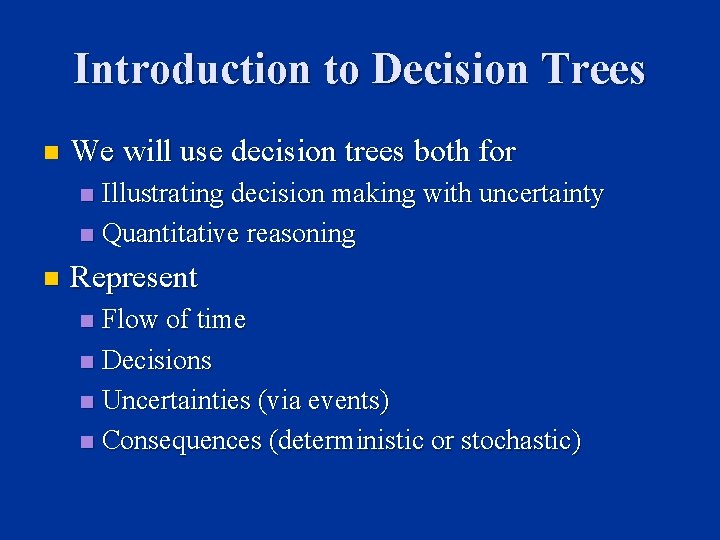
Introduction to Decision Trees n We will use decision trees both for Illustrating decision making with uncertainty n Quantitative reasoning n n Represent Flow of time n Decisions n Uncertainties (via events) n Consequences (deterministic or stochastic) n
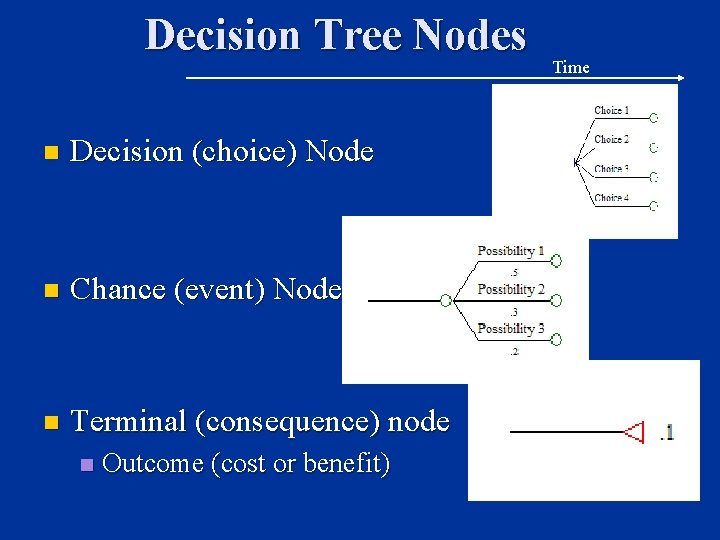
Decision Tree Nodes n Decision (choice) Node n Chance (event) Node n Terminal (consequence) node n Outcome (cost or benefit) Time
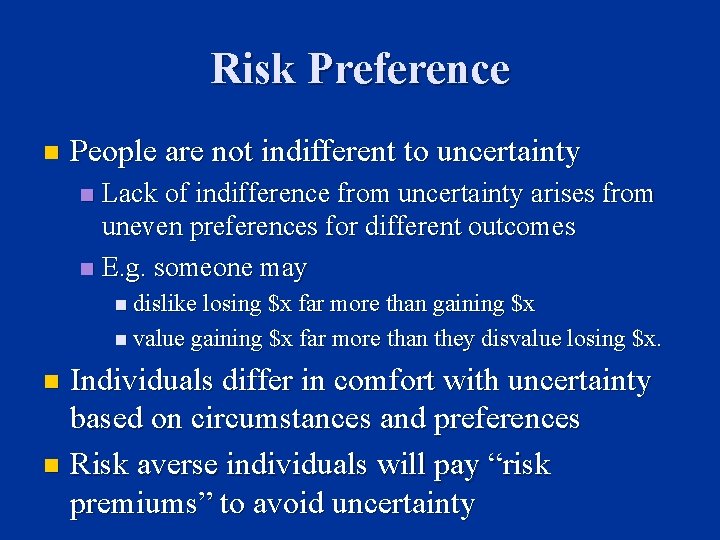
Risk Preference n People are not indifferent to uncertainty Lack of indifference from uncertainty arises from uneven preferences for different outcomes n E. g. someone may n n dislike losing $x far more than gaining $x n value gaining $x far more than they disvalue losing $x. Individuals differ in comfort with uncertainty based on circumstances and preferences n Risk averse individuals will pay “risk premiums” to avoid uncertainty n
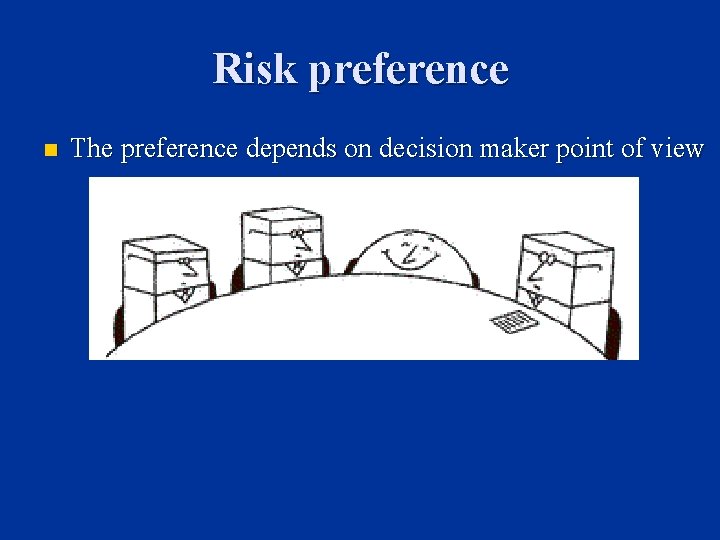
Risk preference n The preference depends on decision maker point of view
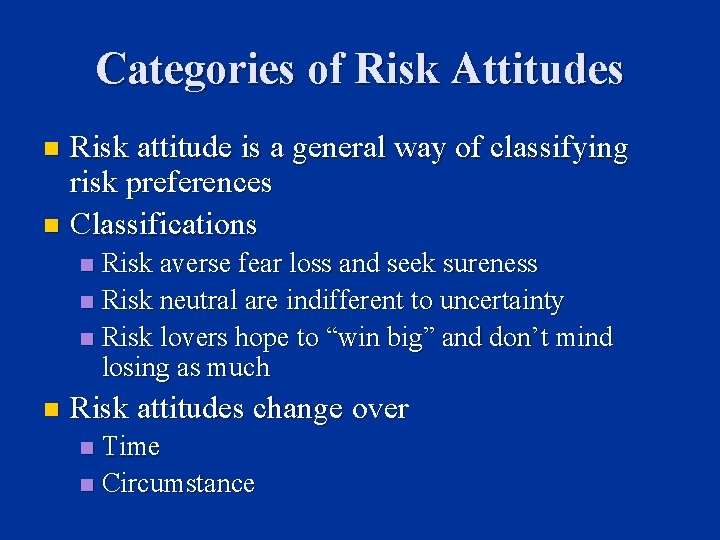
Categories of Risk Attitudes Risk attitude is a general way of classifying risk preferences n Classifications n Risk averse fear loss and seek sureness n Risk neutral are indifferent to uncertainty n Risk lovers hope to “win big” and don’t mind losing as much n n Risk attitudes change over Time n Circumstance n
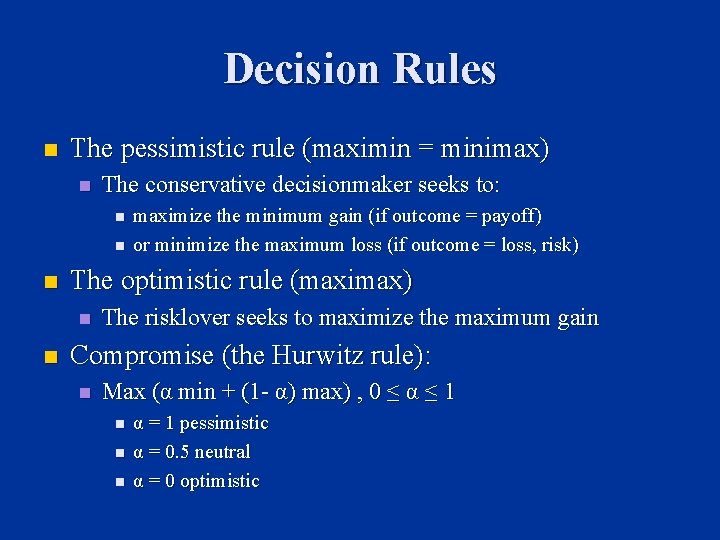
Decision Rules n The pessimistic rule (maximin = minimax) n The conservative decisionmaker seeks to: n n n The optimistic rule (maximax) n n maximize the minimum gain (if outcome = payoff) or minimize the maximum loss (if outcome = loss, risk) The risklover seeks to maximize the maximum gain Compromise (the Hurwitz rule): n Max (α min + (1 - α) max) , 0 ≤ α ≤ 1 n n n α = 1 pessimistic α = 0. 5 neutral α = 0 optimistic
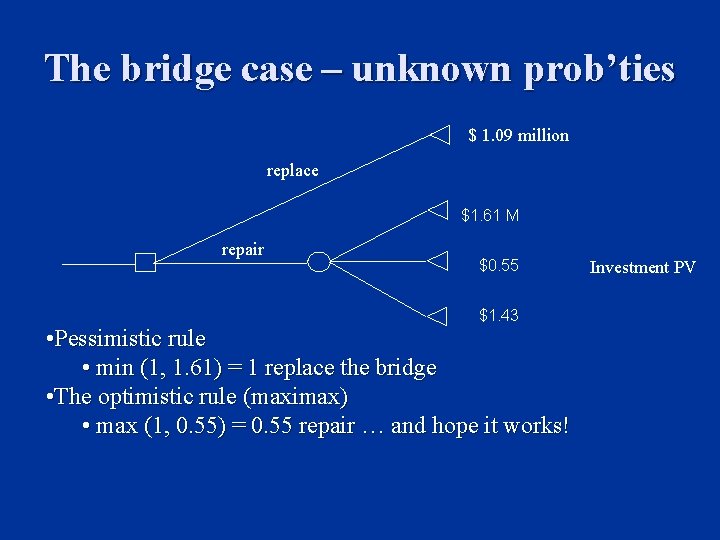
The bridge case – unknown prob’ties $ 1. 09 million replace $1. 61 M repair $0. 55 $1. 43 • Pessimistic rule • min (1, 1. 61) = 1 replace the bridge • The optimistic rule (maximax) • max (1, 0. 55) = 0. 55 repair … and hope it works! Investment PV
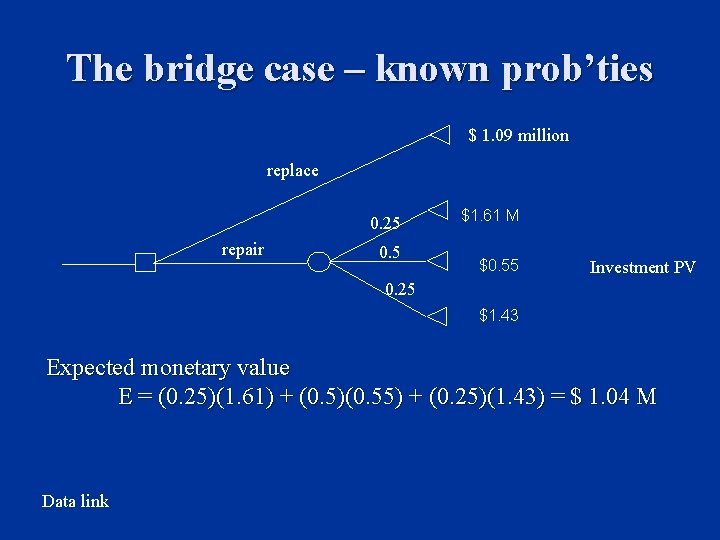
The bridge case – known prob’ties $ 1. 09 million replace 0. 25 repair 0. 5 $1. 61 M $0. 55 Investment PV 0. 25 $1. 43 Expected monetary value E = (0. 25)(1. 61) + (0. 5)(0. 55) + (0. 25)(1. 43) = $ 1. 04 M Data link
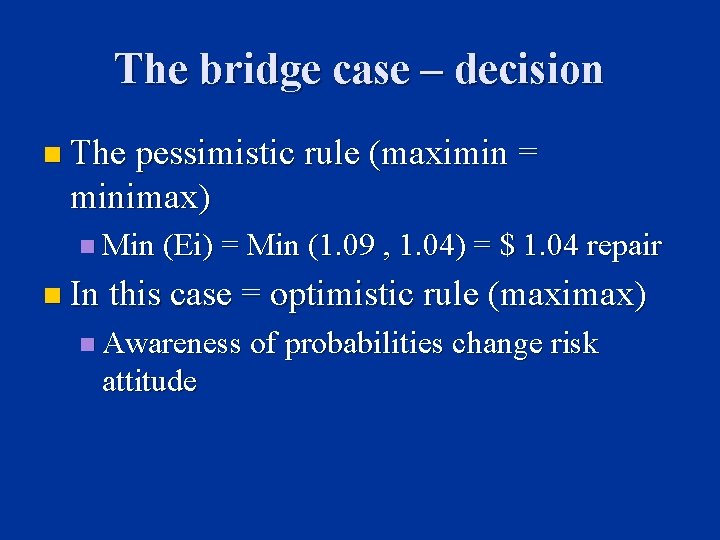
The bridge case – decision n The pessimistic rule (maximin = minimax) n Min (Ei) = Min (1. 09 , 1. 04) = $ 1. 04 repair n In this case = optimistic rule (maximax) n Awareness of probabilities change risk attitude
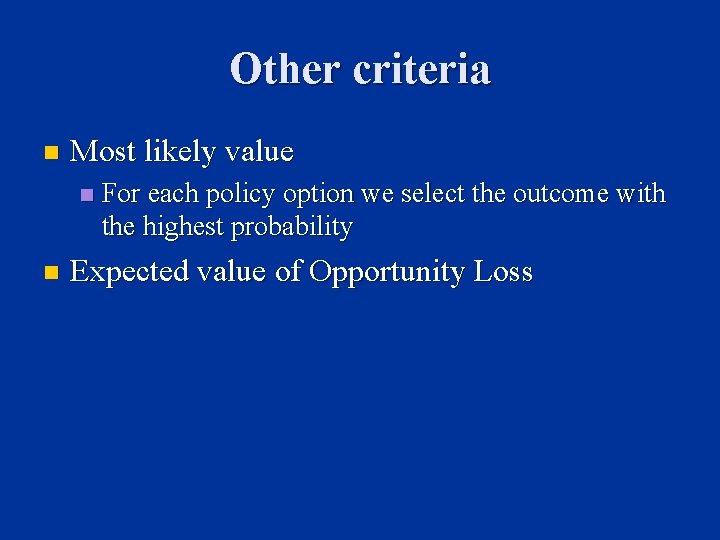
Other criteria n Most likely value n n For each policy option we select the outcome with the highest probability Expected value of Opportunity Loss
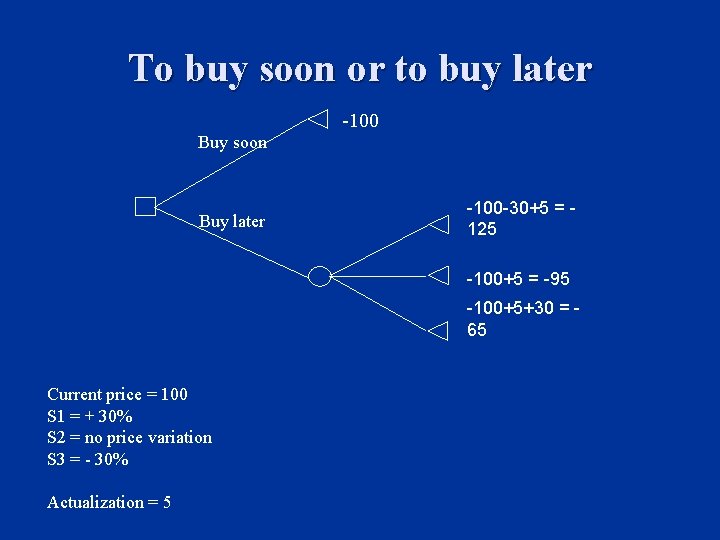
To buy soon or to buy later -100 Buy soon Buy later -100 -30+5 = 125 -100+5 = -95 -100+5+30 = 65 Current price = 100 S 1 = + 30% S 2 = no price variation S 3 = - 30% Actualization = 5
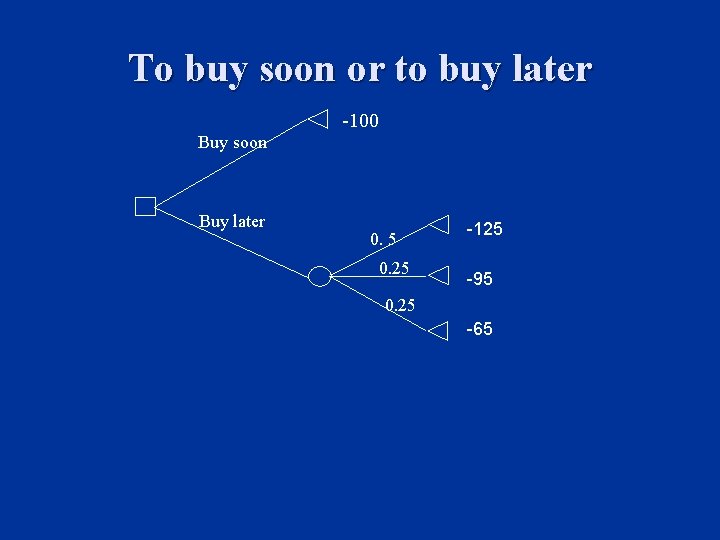
To buy soon or to buy later -100 Buy soon Buy later 0. 5 0. 25 -125 -95 0. 25 -65
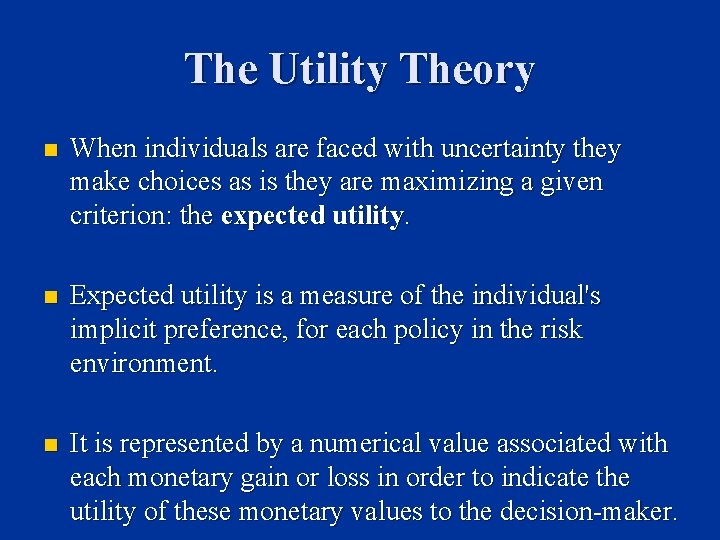
The Utility Theory n When individuals are faced with uncertainty they make choices as is they are maximizing a given criterion: the expected utility. n Expected utility is a measure of the individual's implicit preference, for each policy in the risk environment. n It is represented by a numerical value associated with each monetary gain or loss in order to indicate the utility of these monetary values to the decision-maker.
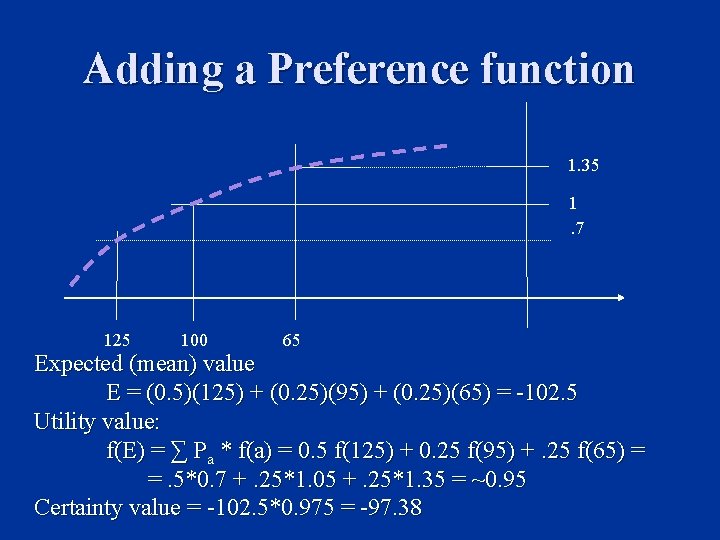
Adding a Preference function 1. 35 1. 7 125 100 65 Expected (mean) value E = (0. 5)(125) + (0. 25)(95) + (0. 25)(65) = -102. 5 Utility value: f(E) = ∑ Pa * f(a) = 0. 5 f(125) + 0. 25 f(95) +. 25 f(65) = =. 5*0. 7 +. 25*1. 05 +. 25*1. 35 = ~0. 95 Certainty value = -102. 5*0. 975 = -97. 38
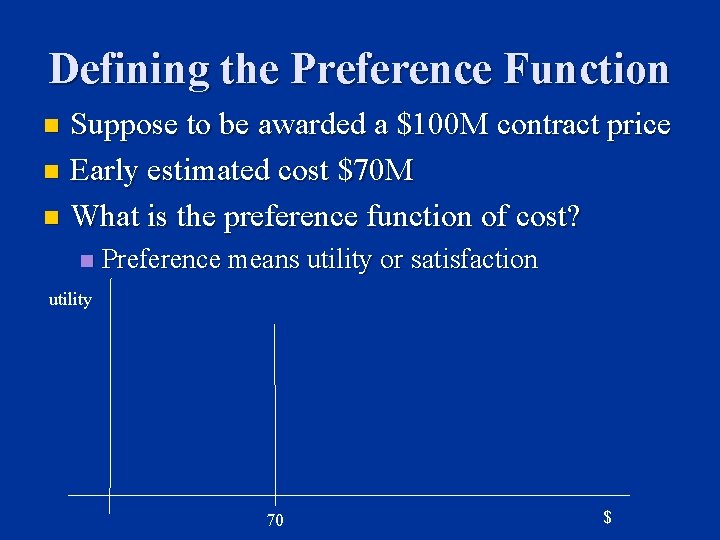
Defining the Preference Function Suppose to be awarded a $100 M contract price n Early estimated cost $70 M n What is the preference function of cost? n n Preference means utility or satisfaction utility 70 $
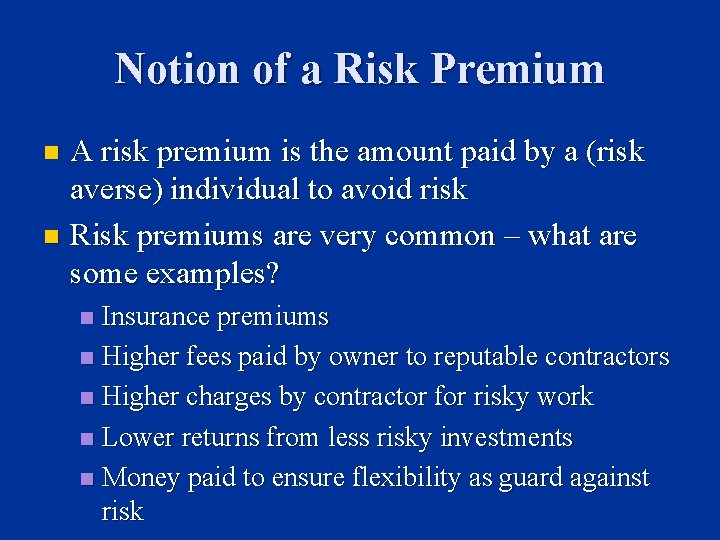
Notion of a Risk Premium A risk premium is the amount paid by a (risk averse) individual to avoid risk n Risk premiums are very common – what are some examples? n Insurance premiums n Higher fees paid by owner to reputable contractors n Higher charges by contractor for risky work n Lower returns from less risky investments n Money paid to ensure flexibility as guard against risk n
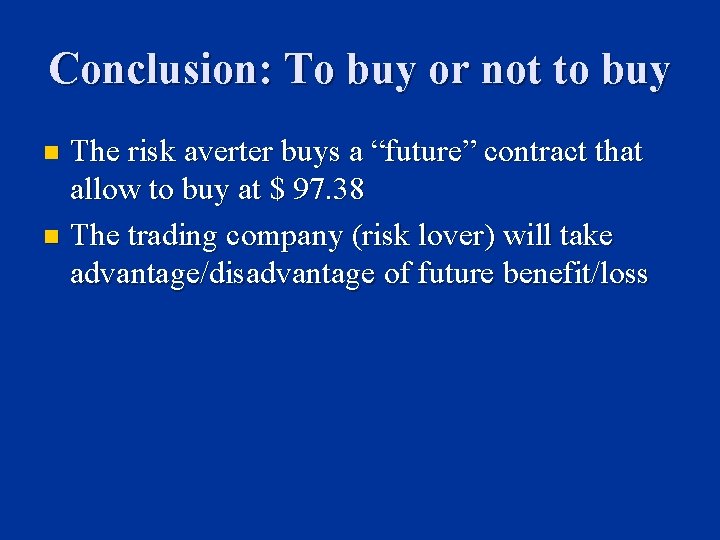
Conclusion: To buy or not to buy The risk averter buys a “future” contract that allow to buy at $ 97. 38 n The trading company (risk lover) will take advantage/disadvantage of future benefit/loss n
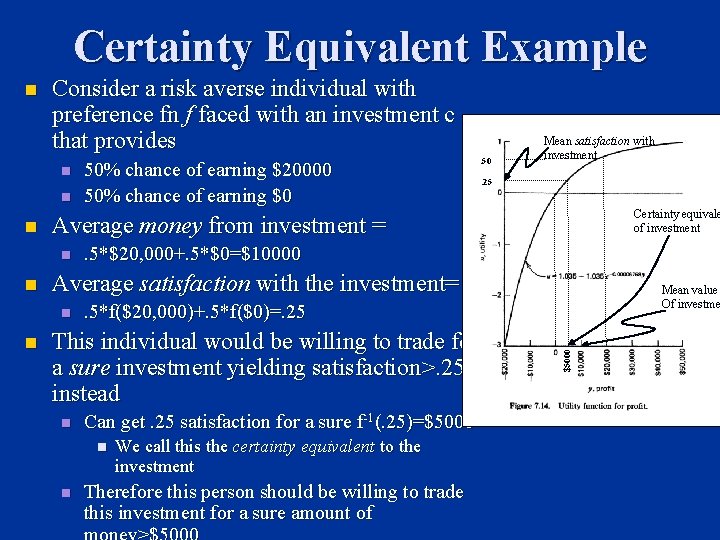
Certainty Equivalent Example Consider a risk averse individual with preference fn f faced with an investment c that provides n n n . 25 Certainty equivale of investment . 5*$20, 000+. 5*$0=$10000 Average satisfaction with the investment= n n . 50 Average money from investment = n n 50% chance of earning $20000 50% chance of earning $0 Mean satisfaction with investment . 5*f($20, 000)+. 5*f($0)=. 25 This individual would be willing to trade for a sure investment yielding satisfaction>. 25 instead n Can get. 25 satisfaction for a sure f-1(. 25)=$5000 n n Mean value Of investme We call this the certainty equivalent to the investment Therefore this person should be willing to trade this investment for a sure amount of $5000 n
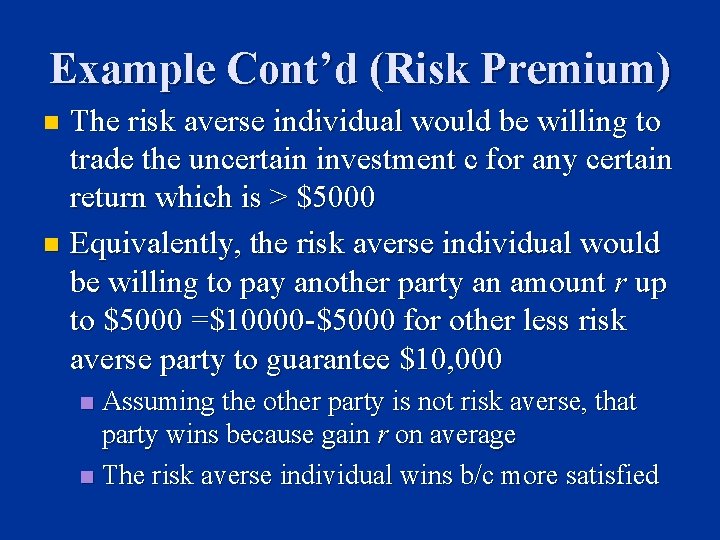
Example Cont’d (Risk Premium) The risk averse individual would be willing to trade the uncertain investment c for any certain return which is > $5000 n Equivalently, the risk averse individual would be willing to pay another party an amount r up to $5000 =$10000 -$5000 for other less risk averse party to guarantee $10, 000 n Assuming the other party is not risk averse, that party wins because gain r on average n The risk averse individual wins b/c more satisfied n
![Certainty Equivalent n More generally consider situation in which have n n Note EX Certainty Equivalent n More generally, consider situation in which have n n Note: E[X]](https://slidetodoc.com/presentation_image_h/0f0840a5be74ed3abbe338f3a375fd57/image-31.jpg)
Certainty Equivalent n More generally, consider situation in which have n n Note: E[X] is the mean (expected value) operator The mean outcome of uncertain investment c is E[c] n n In example, this was. 5*$20, 000+. 5*$0=$10, 000 The mean satisfaction with the investment is E[f(c)] n n Uncertainty with respect to consequence c Non-linear preference function f In example, this was. 5*f($20, 000)+. 5*f($0)=. 25 We call f-1(E[f(c)]) the certainty equivalent of c n n Size of sure return that would give the same satisfaction as c In example, was f-1(. 25)=f-1(. 5*20, 000+. 5*0)=$5, 000
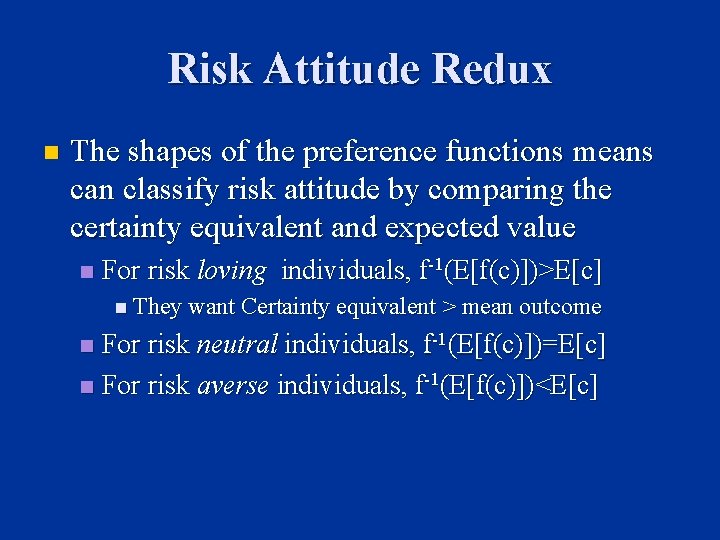
Risk Attitude Redux n The shapes of the preference functions means can classify risk attitude by comparing the certainty equivalent and expected value n For risk loving individuals, f-1(E[f(c)])>E[c] n They want Certainty equivalent > mean outcome For risk neutral individuals, f-1(E[f(c)])=E[c] n For risk averse individuals, f-1(E[f(c)])<E[c] n
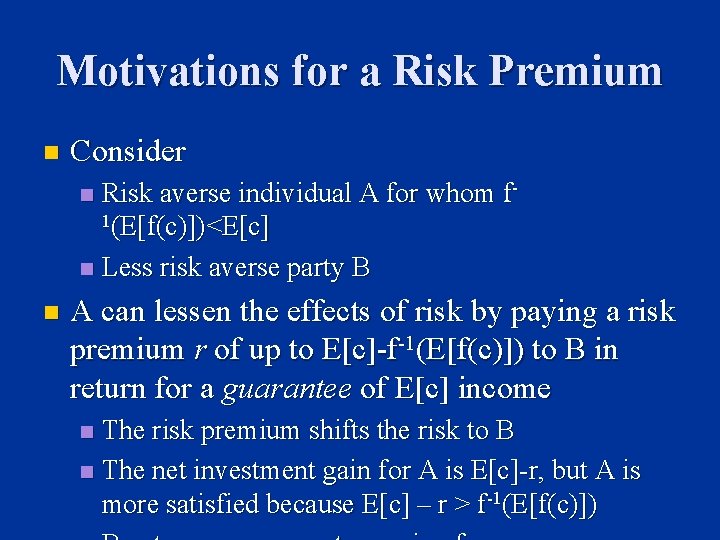
Motivations for a Risk Premium n Consider Risk averse individual A for whom f 1(E[f(c)])<E[c] n Less risk averse party B n n A can lessen the effects of risk by paying a risk premium r of up to E[c]-f-1(E[f(c)]) to B in return for a guarantee of E[c] income The risk premium shifts the risk to B n The net investment gain for A is E[c]-r, but A is more satisfied because E[c] – r > f-1(E[f(c)]) n
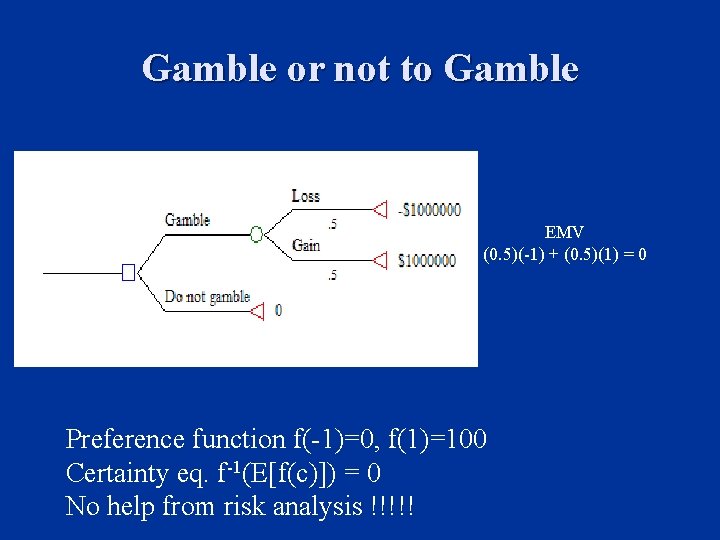
Gamble or not to Gamble EMV (0. 5)(-1) + (0. 5)(1) = 0 Preference function f(-1)=0, f(1)=100 Certainty eq. f-1(E[f(c)]) = 0 No help from risk analysis !!!!!
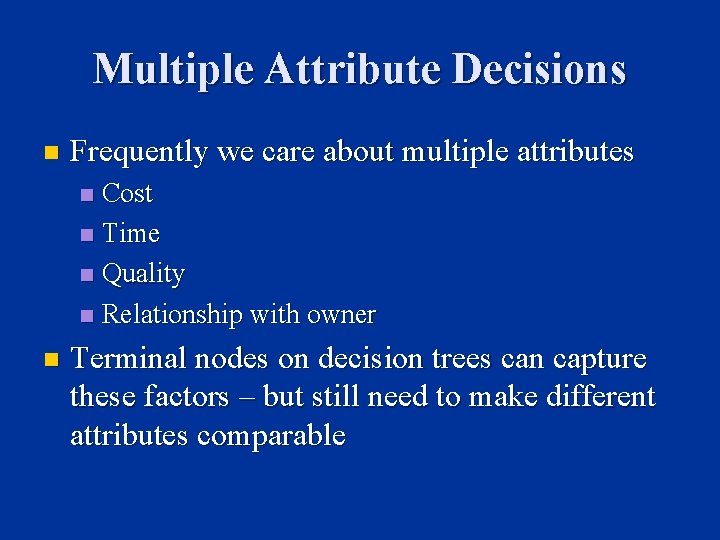
Multiple Attribute Decisions n Frequently we care about multiple attributes Cost n Time n Quality n Relationship with owner n n Terminal nodes on decision trees can capture these factors – but still need to make different attributes comparable
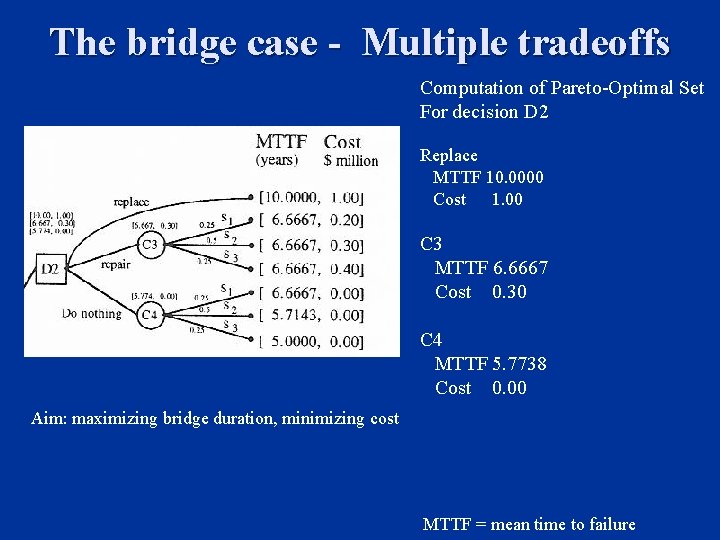
The bridge case - Multiple tradeoffs Computation of Pareto-Optimal Set For decision D 2 Replace MTTF 10. 0000 Cost 1. 00 C 3 MTTF 6. 6667 Cost 0. 30 C 4 MTTF 5. 7738 Cost 0. 00 Aim: maximizing bridge duration, minimizing cost MTTF = mean time to failure
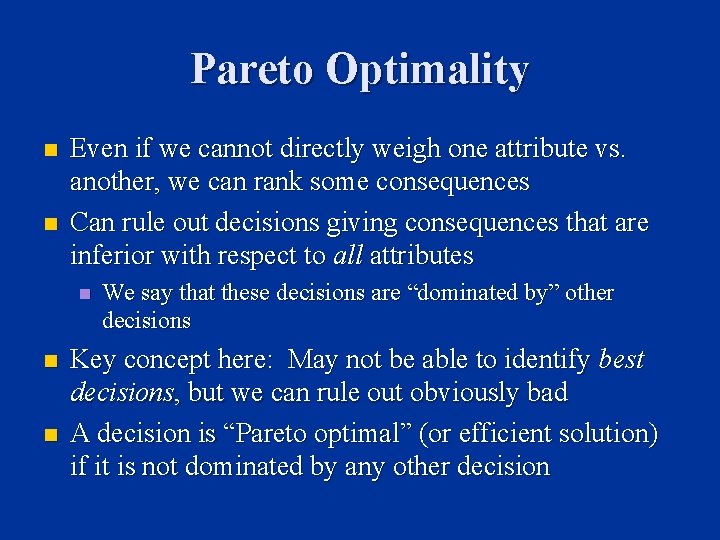
Pareto Optimality n n Even if we cannot directly weigh one attribute vs. another, we can rank some consequences Can rule out decisions giving consequences that are inferior with respect to all attributes n n n We say that these decisions are “dominated by” other decisions Key concept here: May not be able to identify best decisions, but we can rule out obviously bad A decision is “Pareto optimal” (or efficient solution) if it is not dominated by any other decision
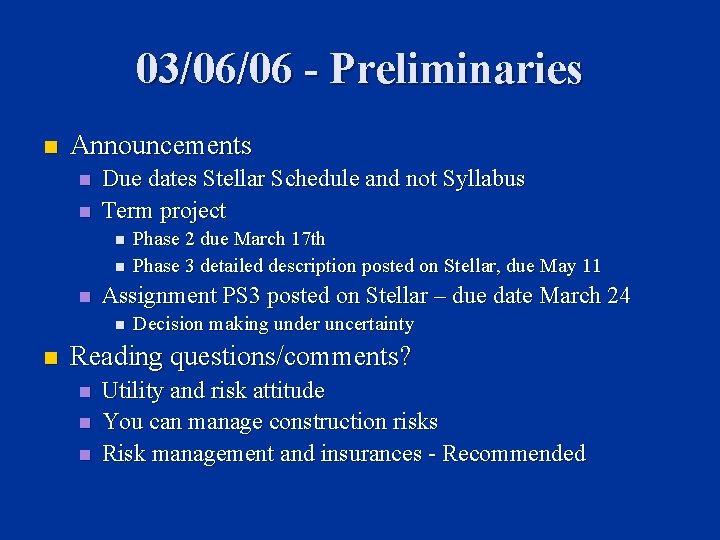
03/06/06 - Preliminaries n Announcements n n Due dates Stellar Schedule and not Syllabus Term project n n n Assignment PS 3 posted on Stellar – due date March 24 n n Phase 2 due March 17 th Phase 3 detailed description posted on Stellar, due May 11 Decision making under uncertainty Reading questions/comments? n n n Utility and risk attitude You can manage construction risks Risk management and insurances - Recommended
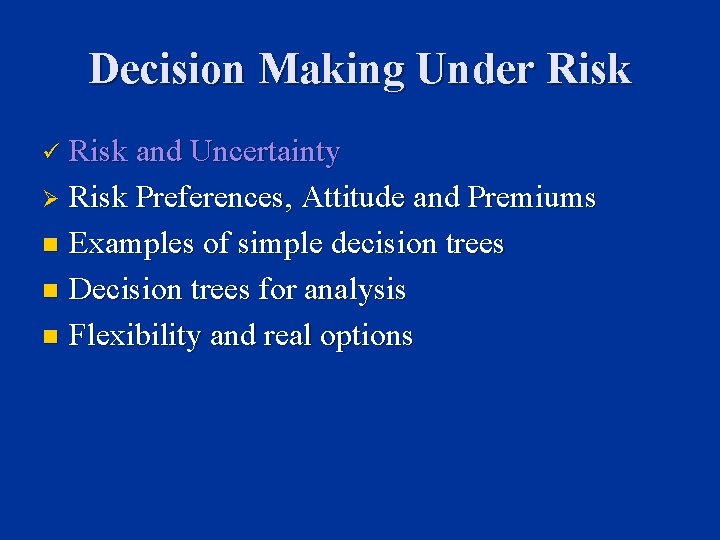
Decision Making Under Risk and Uncertainty Ø Risk Preferences, Attitude and Premiums n Examples of simple decision trees n Decision trees for analysis n Flexibility and real options ü
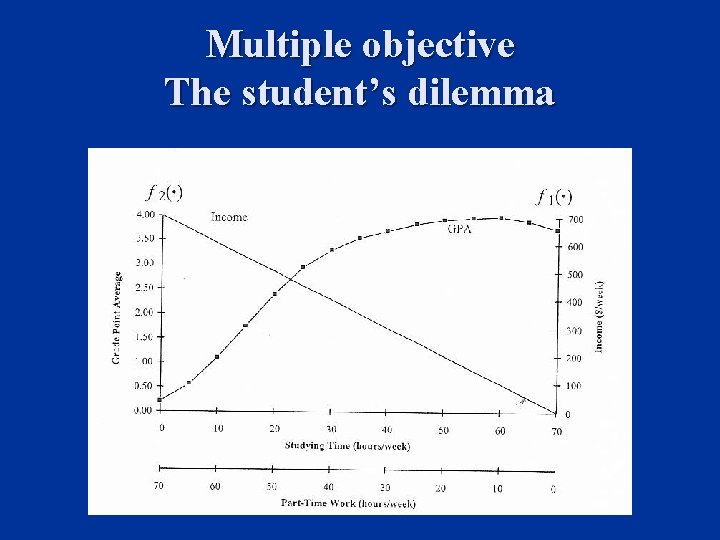
Multiple objective The student’s dilemma
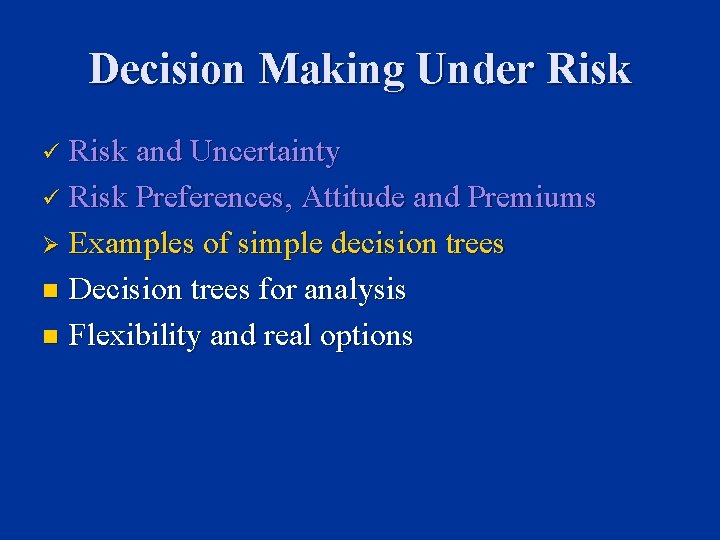
Decision Making Under Risk and Uncertainty ü Risk Preferences, Attitude and Premiums Ø Examples of simple decision trees n Decision trees for analysis n Flexibility and real options ü
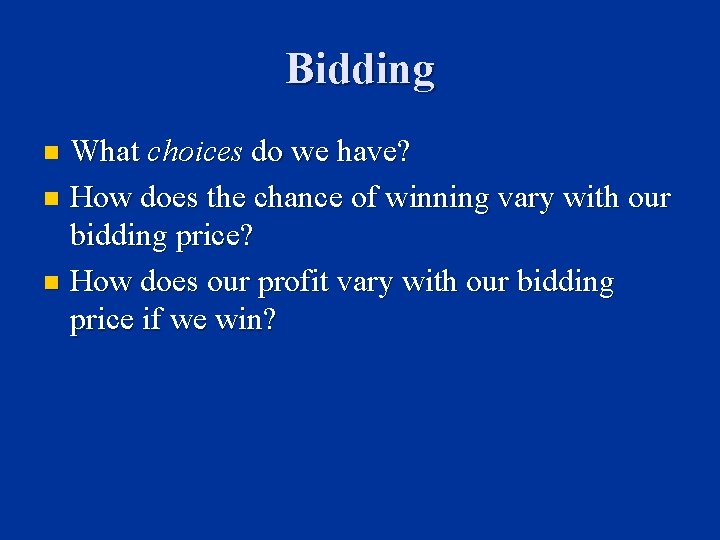
Bidding What choices do we have? n How does the chance of winning vary with our bidding price? n How does our profit vary with our bidding price if we win? n
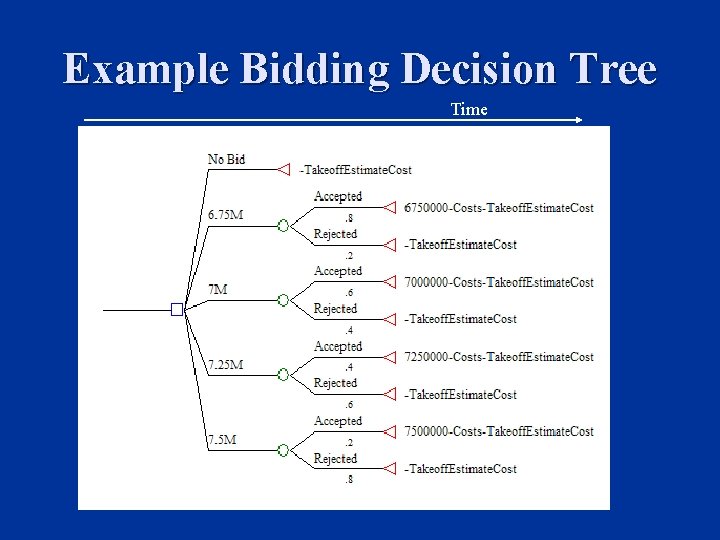
Example Bidding Decision Tree Time
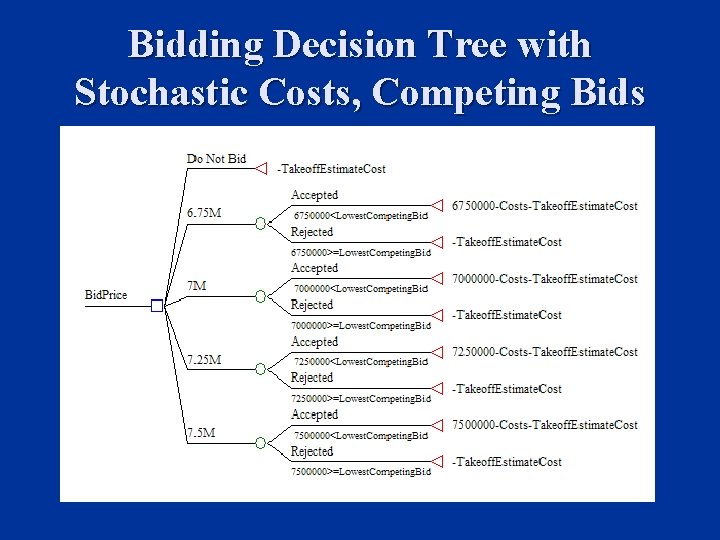
Bidding Decision Tree with Stochastic Costs, Competing Bids
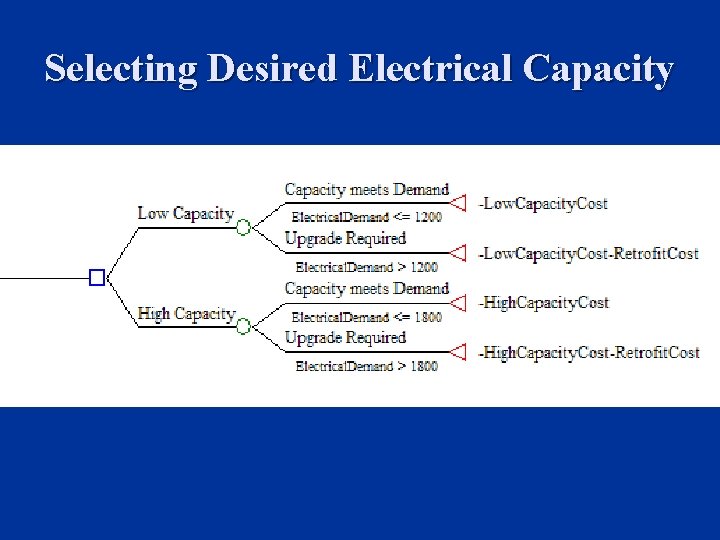
Selecting Desired Electrical Capacity
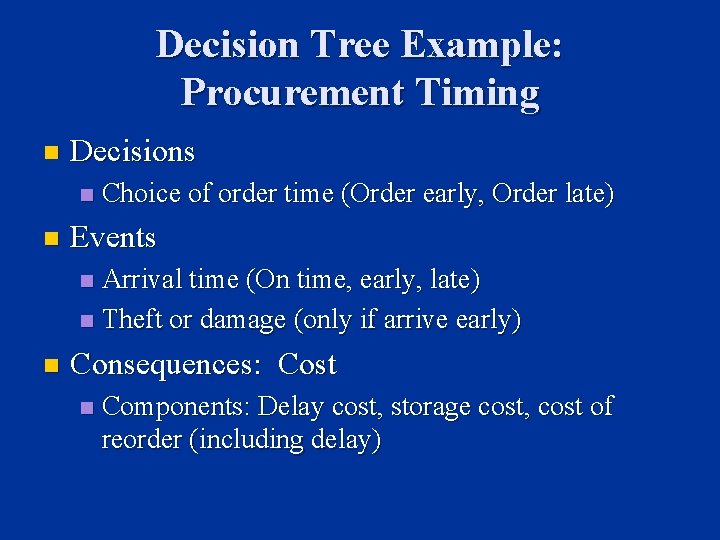
Decision Tree Example: Procurement Timing n Decisions n n Choice of order time (Order early, Order late) Events Arrival time (On time, early, late) n Theft or damage (only if arrive early) n n Consequences: Cost n Components: Delay cost, storage cost, cost of reorder (including delay)
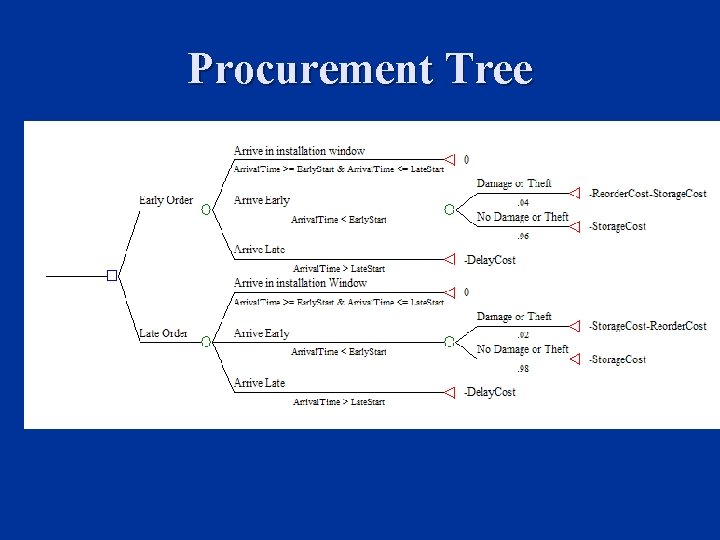
Procurement Tree
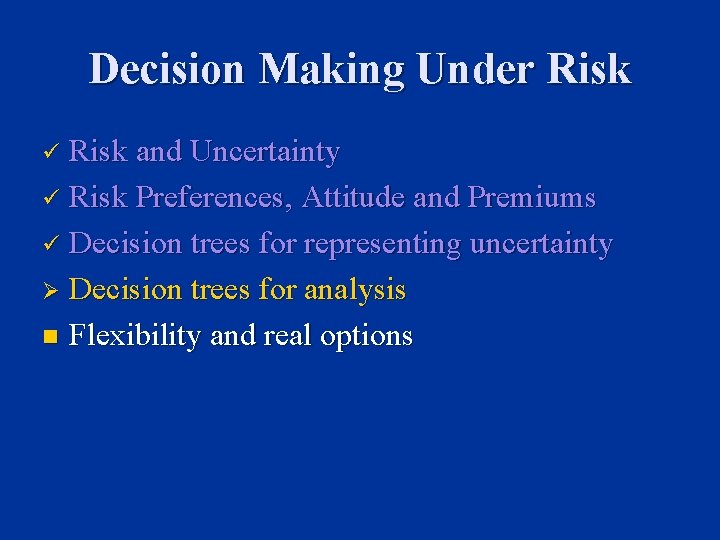
Decision Making Under Risk and Uncertainty ü Risk Preferences, Attitude and Premiums ü Decision trees for representing uncertainty Ø Decision trees for analysis n Flexibility and real options ü
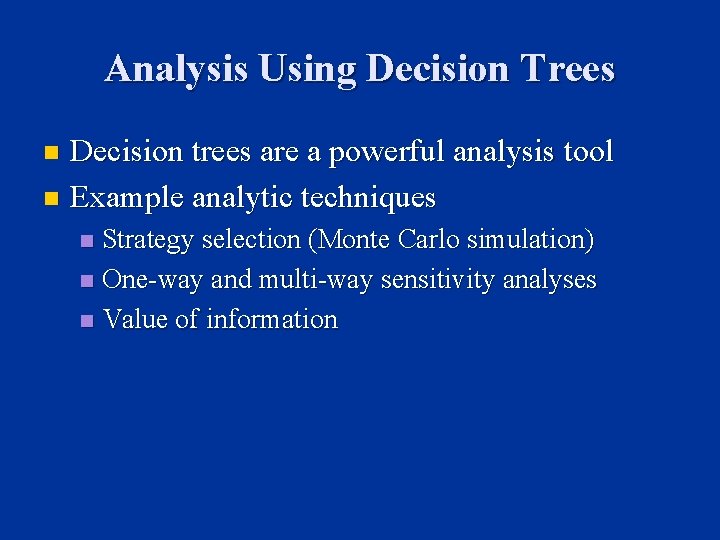
Analysis Using Decision Trees Decision trees are a powerful analysis tool n Example analytic techniques n Strategy selection (Monte Carlo simulation) n One-way and multi-way sensitivity analyses n Value of information n
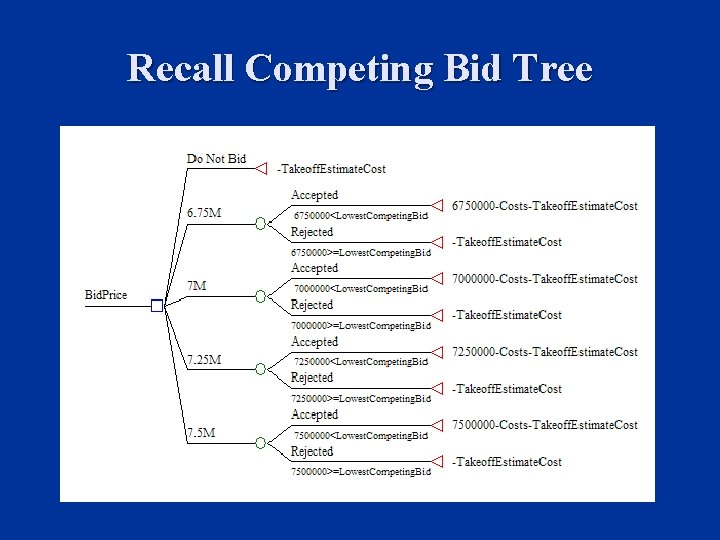
Recall Competing Bid Tree
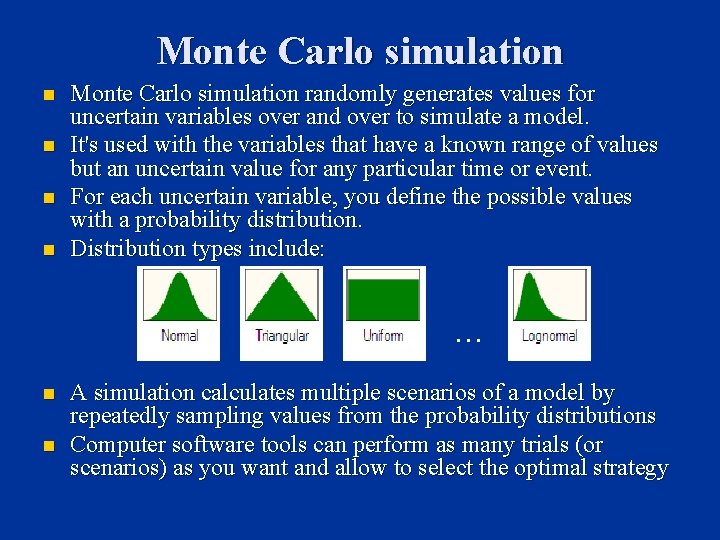
Monte Carlo simulation n n Monte Carlo simulation randomly generates values for uncertain variables over and over to simulate a model. It's used with the variables that have a known range of values but an uncertain value for any particular time or event. For each uncertain variable, you define the possible values with a probability distribution. Distribution types include: … n n A simulation calculates multiple scenarios of a model by repeatedly sampling values from the probability distributions Computer software tools can perform as many trials (or scenarios) as you want and allow to select the optimal strategy
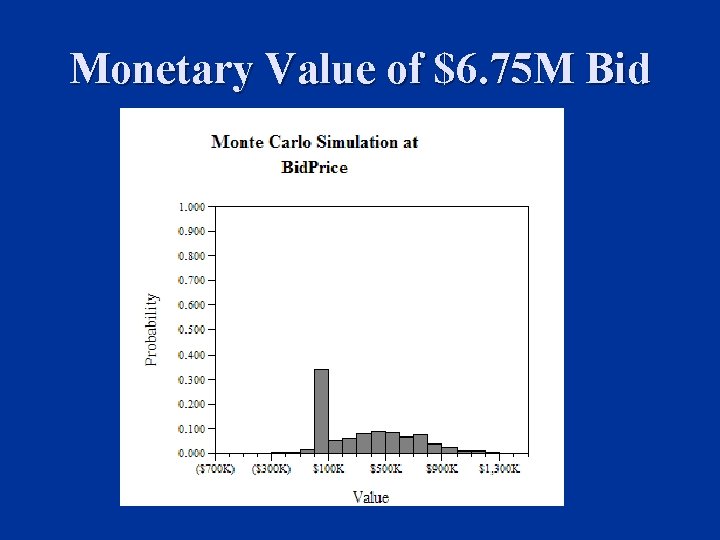
Monetary Value of $6. 75 M Bid
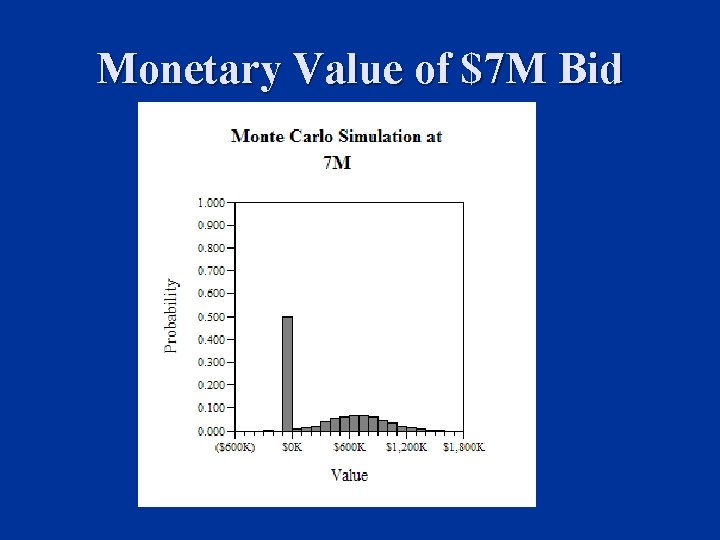
Monetary Value of $7 M Bid
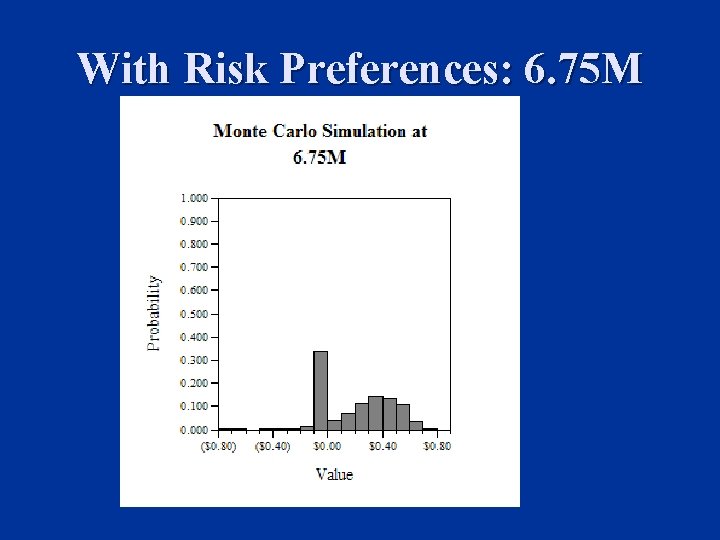
With Risk Preferences: 6. 75 M
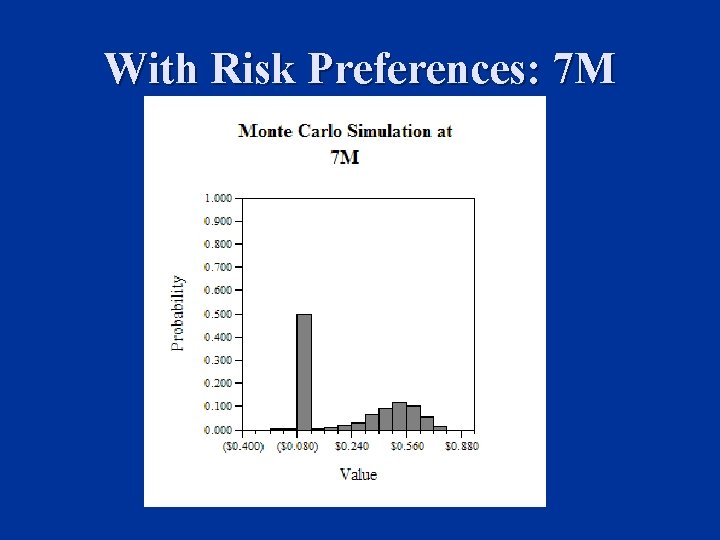
With Risk Preferences: 7 M
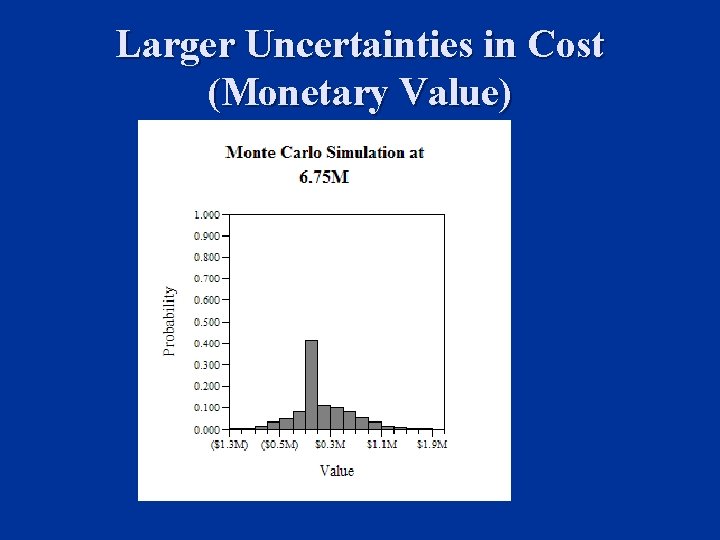
Larger Uncertainties in Cost (Monetary Value)
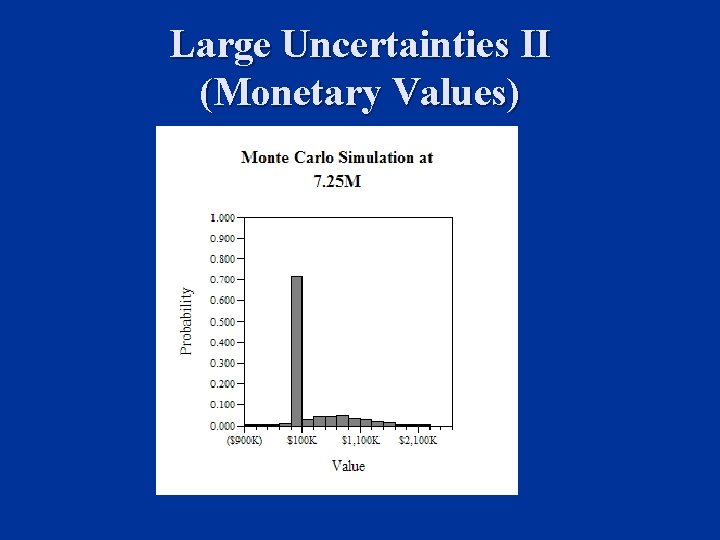
Large Uncertainties II (Monetary Values)
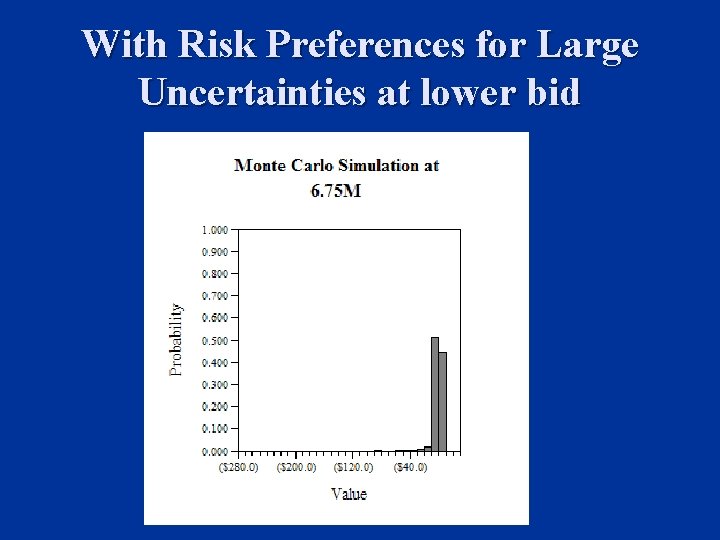
With Risk Preferences for Large Uncertainties at lower bid
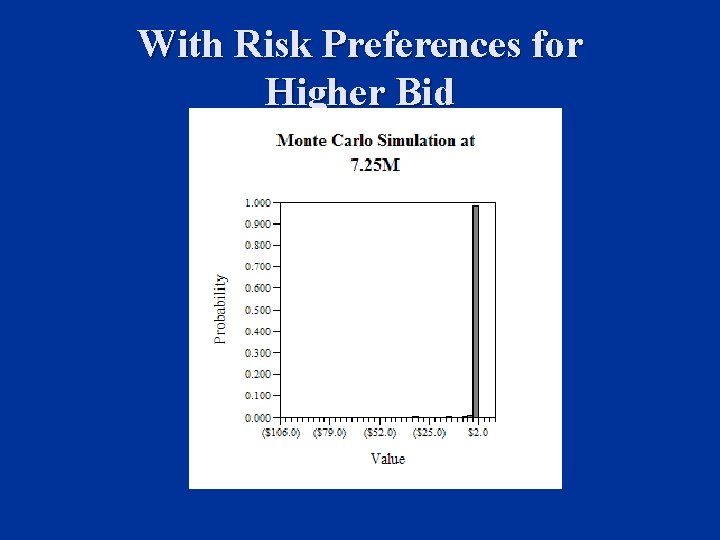
With Risk Preferences for Higher Bid
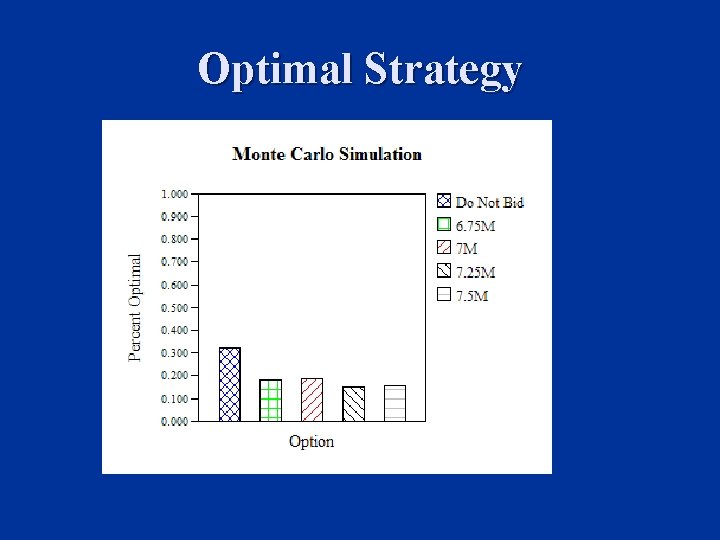
Optimal Strategy
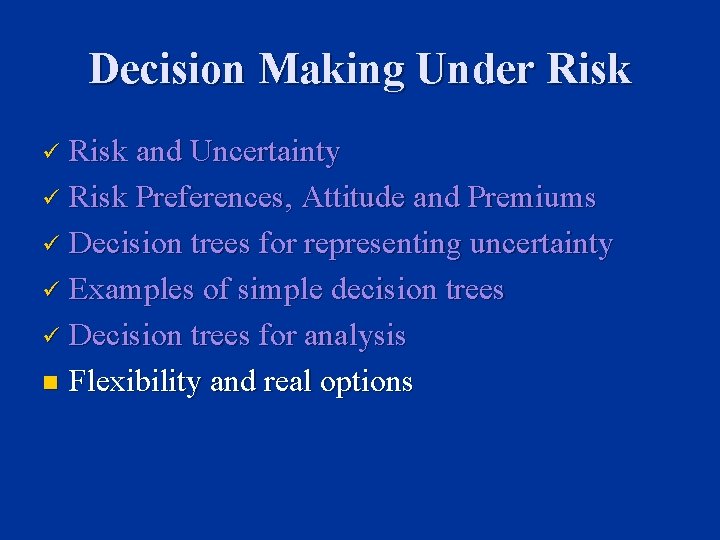
Decision Making Under Risk and Uncertainty ü Risk Preferences, Attitude and Premiums ü Decision trees for representing uncertainty ü Examples of simple decision trees ü Decision trees for analysis n Flexibility and real options ü
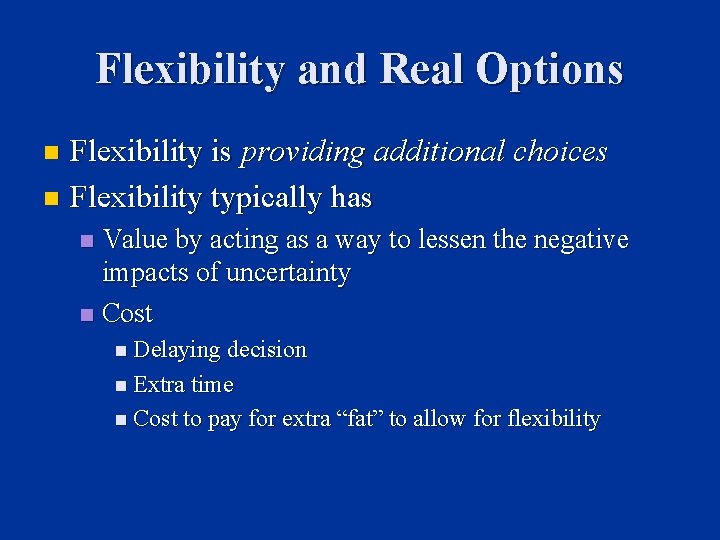
Flexibility and Real Options Flexibility is providing additional choices n Flexibility typically has n Value by acting as a way to lessen the negative impacts of uncertainty n Cost n n Delaying decision n Extra time n Cost to pay for extra “fat” to allow for flexibility
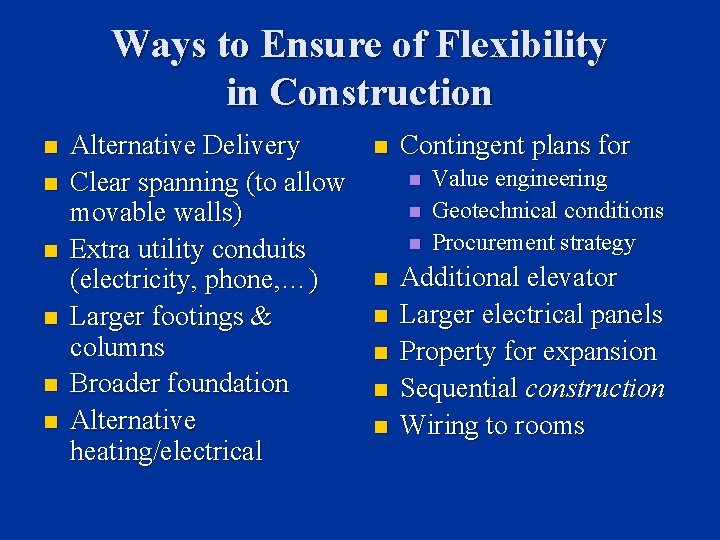
Ways to Ensure of Flexibility in Construction n n n Alternative Delivery Clear spanning (to allow movable walls) Extra utility conduits (electricity, phone, …) Larger footings & columns Broader foundation Alternative heating/electrical n Contingent plans for n n n n Value engineering Geotechnical conditions Procurement strategy Additional elevator Larger electrical panels Property for expansion Sequential construction Wiring to rooms
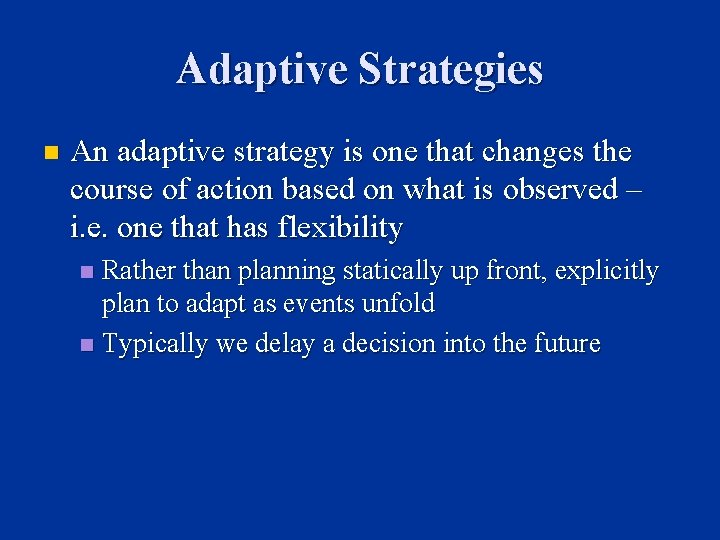
Adaptive Strategies n An adaptive strategy is one that changes the course of action based on what is observed – i. e. one that has flexibility Rather than planning statically up front, explicitly plan to adapt as events unfold n Typically we delay a decision into the future n
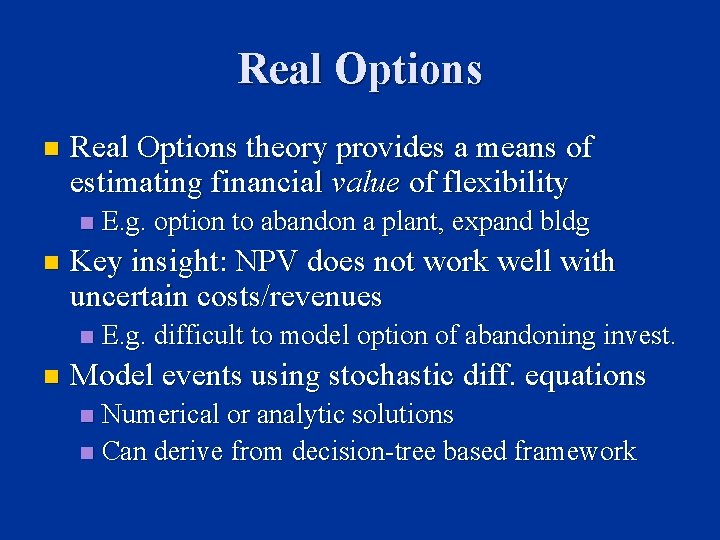
Real Options n Real Options theory provides a means of estimating financial value of flexibility n n Key insight: NPV does not work well with uncertain costs/revenues n n E. g. option to abandon a plant, expand bldg E. g. difficult to model option of abandoning invest. Model events using stochastic diff. equations Numerical or analytic solutions n Can derive from decision-tree based framework n
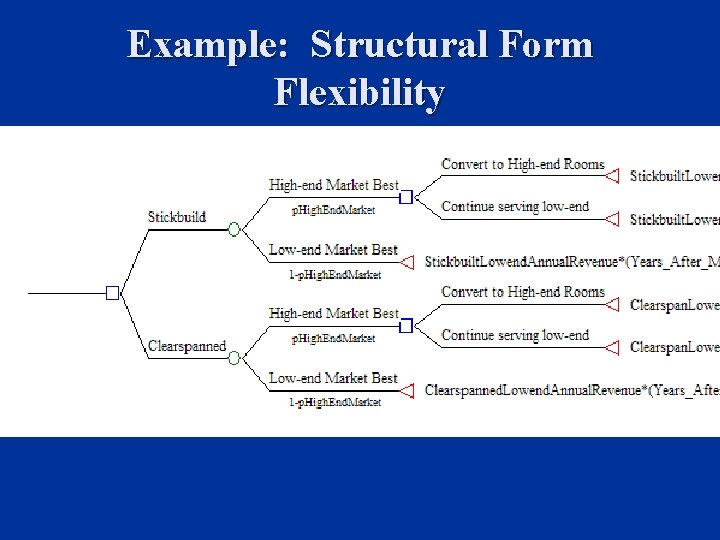
Example: Structural Form Flexibility
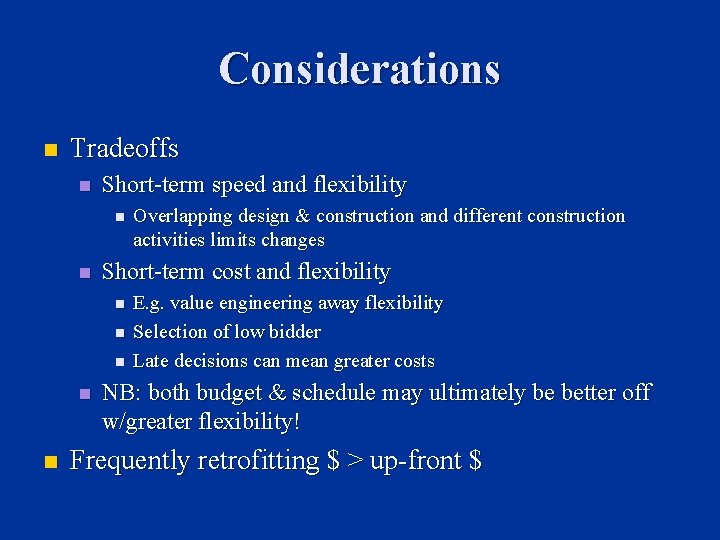
Considerations n Tradeoffs n Short-term speed and flexibility n n Short-term cost and flexibility n n n Overlapping design & construction and different construction activities limits changes E. g. value engineering away flexibility Selection of low bidder Late decisions can mean greater costs NB: both budget & schedule may ultimately be better off w/greater flexibility! Frequently retrofitting $ > up-front $
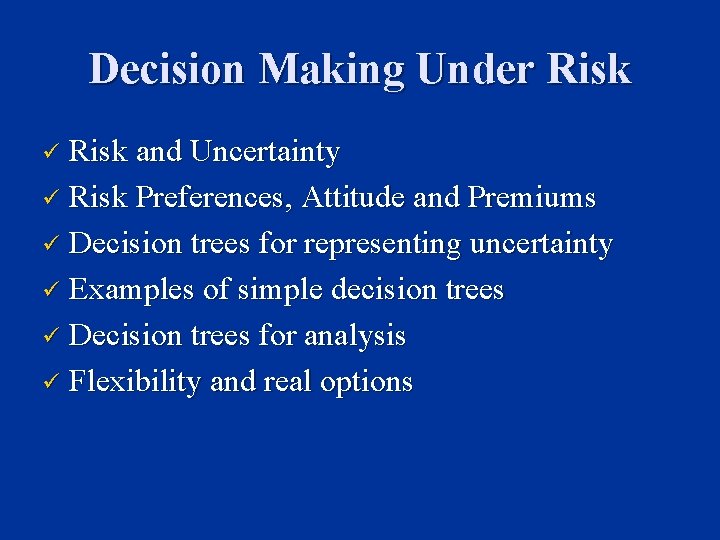
Decision Making Under Risk and Uncertainty ü Risk Preferences, Attitude and Premiums ü Decision trees for representing uncertainty ü Examples of simple decision trees ü Decision trees for analysis ü Flexibility and real options ü
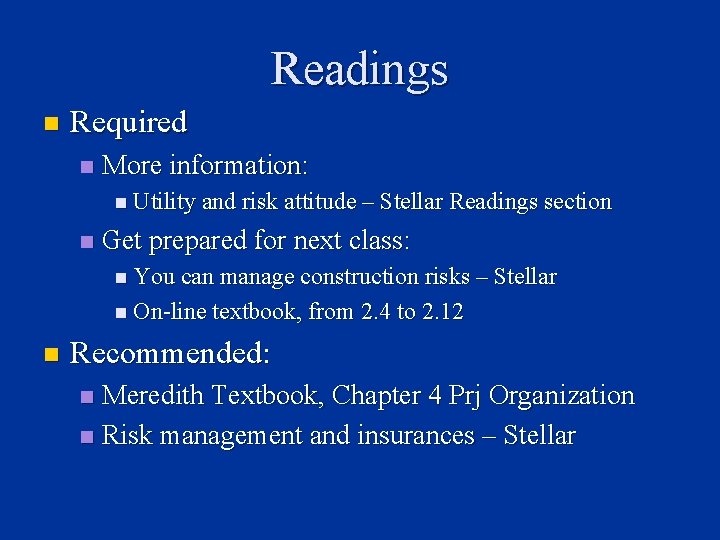
Readings n Required n More information: n Utility and risk attitude – Stellar Readings section n Get prepared for next class: n You can manage construction risks – Stellar n On-line textbook, from 2. 4 to 2. 12 n Recommended: Meredith Textbook, Chapter 4 Prj Organization n Risk management and insurances – Stellar n
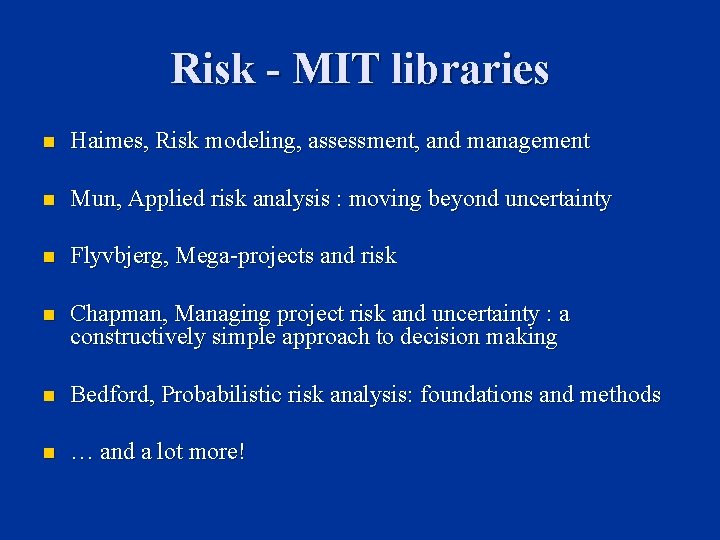
Risk - MIT libraries n Haimes, Risk modeling, assessment, and management n Mun, Applied risk analysis : moving beyond uncertainty n Flyvbjerg, Mega-projects and risk n Chapman, Managing project risk and uncertainty : a constructively simple approach to decision making n Bedford, Probabilistic risk analysis: foundations and methods n … and a lot more!
Market risk assessment
Bae yong-kyun
Spring in months
Importance of software project management
Key risk indicators financial risk management
Risk map
Software project risk
Rmmm table
Project risk management definition pmbok
Risk mitigation techniques in project management
Rmmm table
Figure 11-4
Project risk management adalah
West virginia project risk management
Introduction to project risk management
Project risk management
Four stages of risk management
What is risk management in a project
Pmbok risk
Project portfolio risk management
Lsp401
Svartpilen 401 dyno
Cse401
Ge 401
Where should charts with food code 3-401 be displayed texas
Eng m 401
Clase 401
401 branard st
Bmb 401
Comm 401
401 relevance
As riquezas mundanas nada valem pra mim
Hd-wp-4k-401-c
Quantas prendas faltam para a turma 401 empatar com a 402
Stitch class 600
Program 401 ploiesti
Herpesviridae
Multithread chain stitch
Jul 401 english
Ir401 form
Melinex polyester film
404 asphalt
Csc 401
Concurrent engineering disadvantages
Ce-401
Ce-401
401 west georgia
Nia 580
Comp 401
Worldwide product division structure
Spring dynamic web project
Spring board project
Eclipse folding plugin
The role of project management in achieving project success
Iteration workflows in software project management
Project cost duration graph
Modern project management began with what project
Software project evaluation
Perpetual project closure
Agile ms project template
Types of project termination in project management
Draw rmmm plan
Risk mitigation avoidance
Relative risk calculation
Residual risk and secondary risk pmp
Ar = ir x cr x dr
Absolute risk vs relative risk
Activity sheet 1: conservative, moderate or speculative?
Firm risk scorecard
Pembelanjaan risiko
The biggest risk is not taking any risk