Variance Estimation When Donor Imputation is Used to
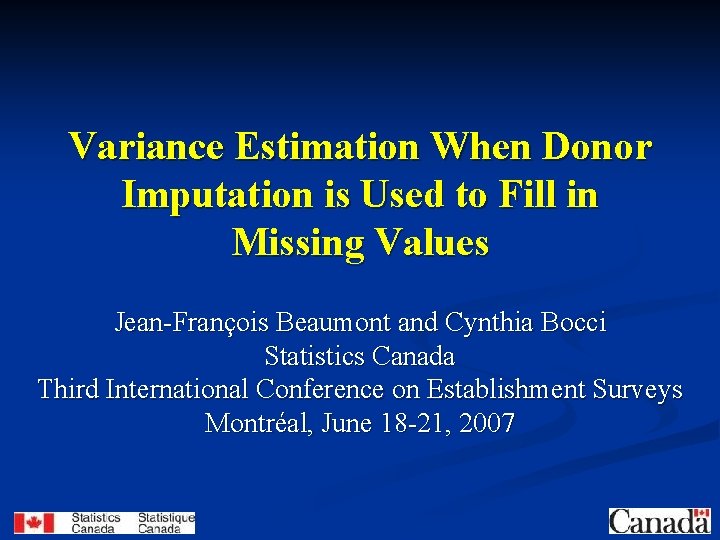
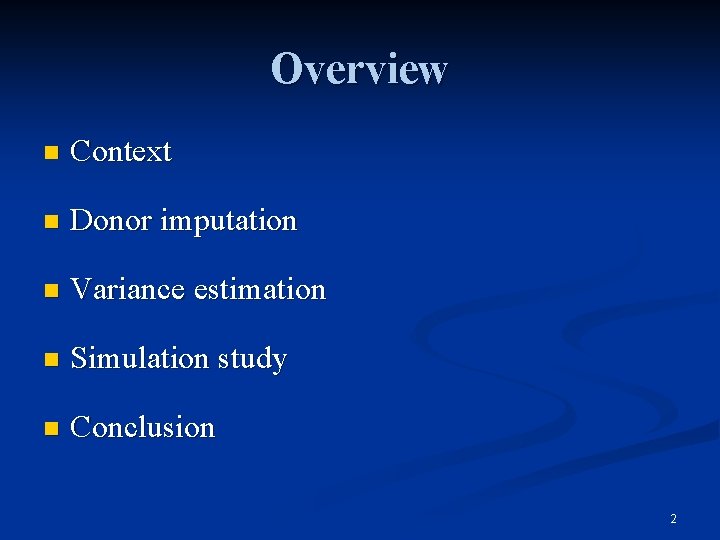
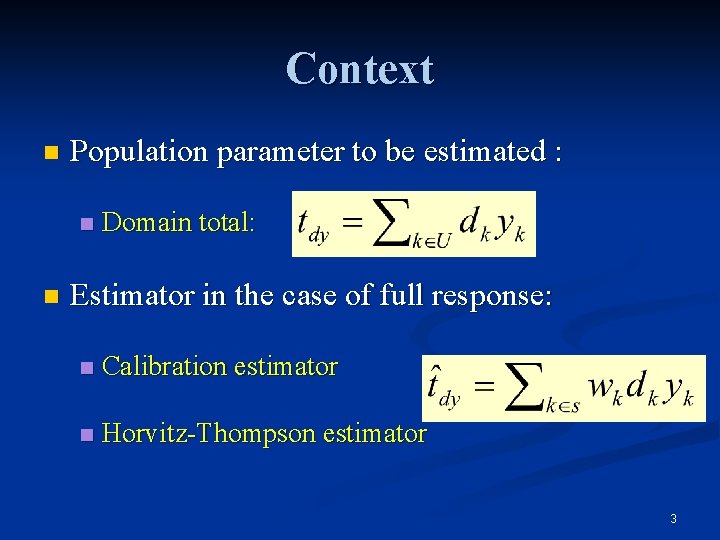
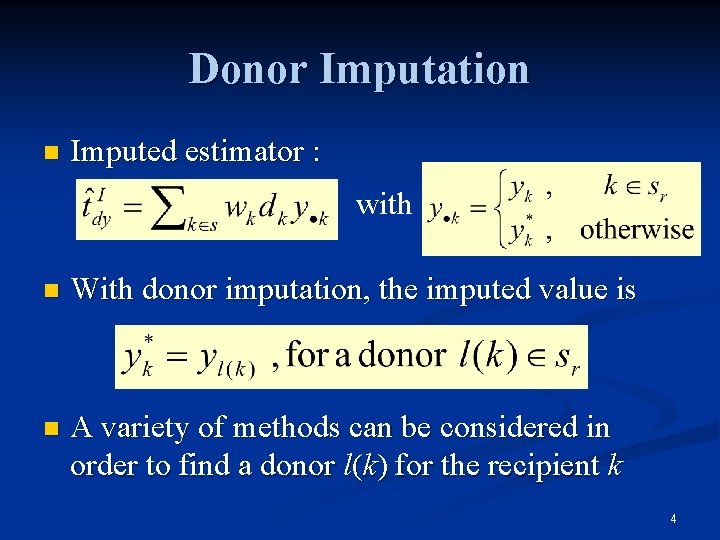
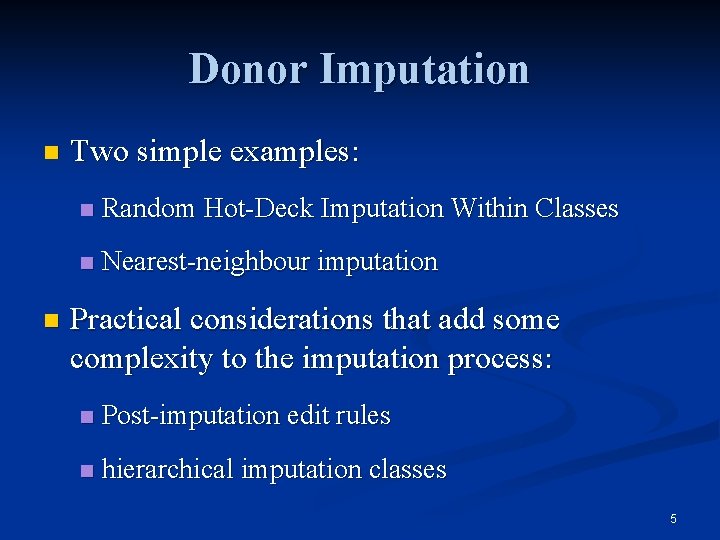
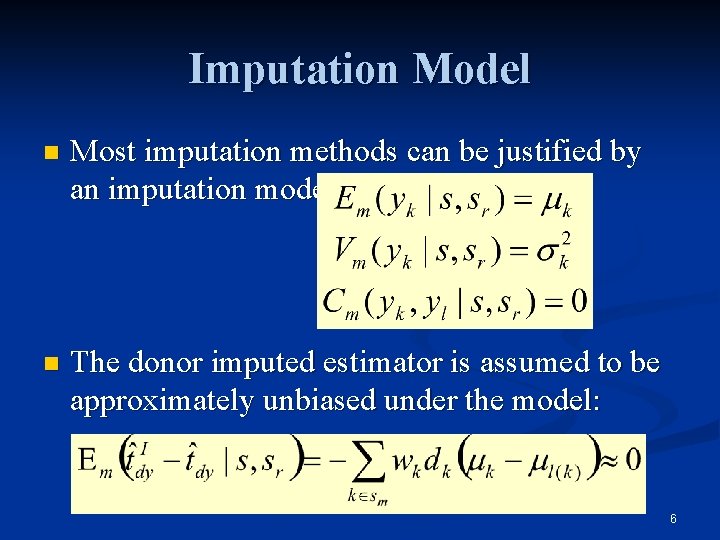
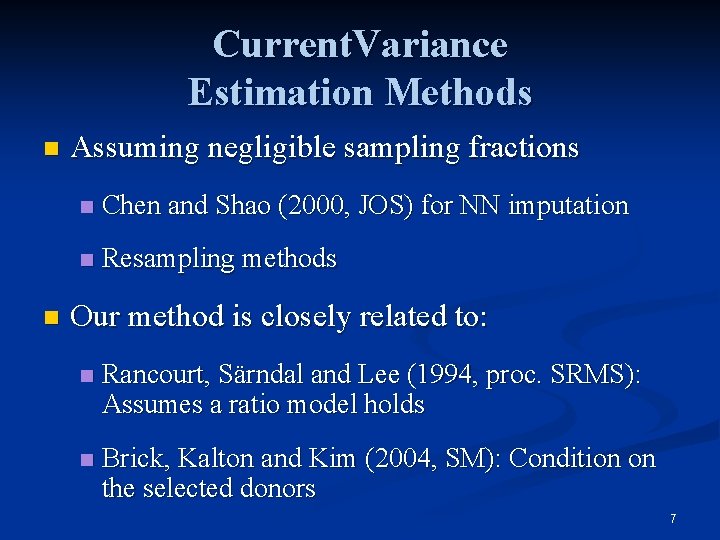
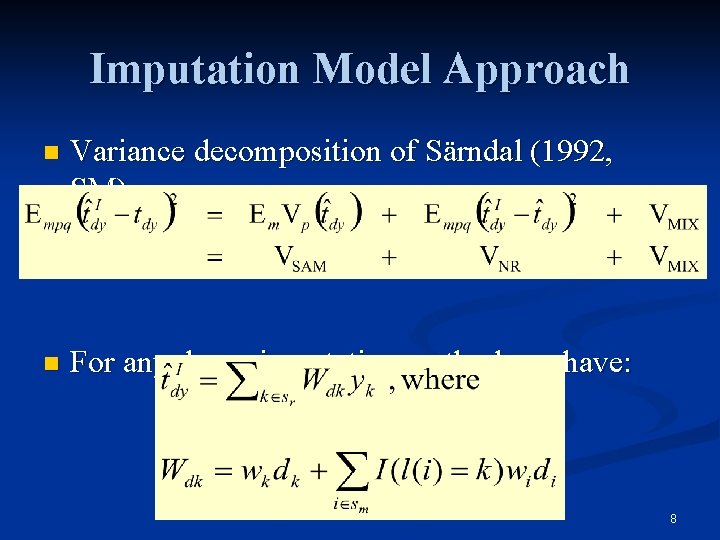
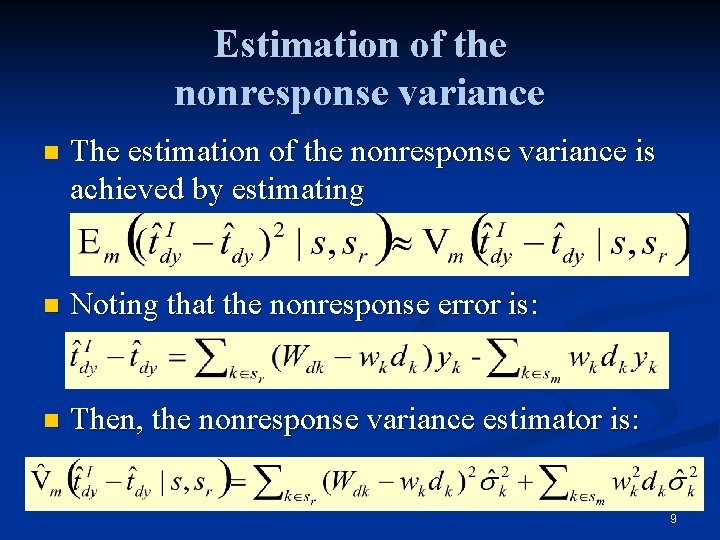
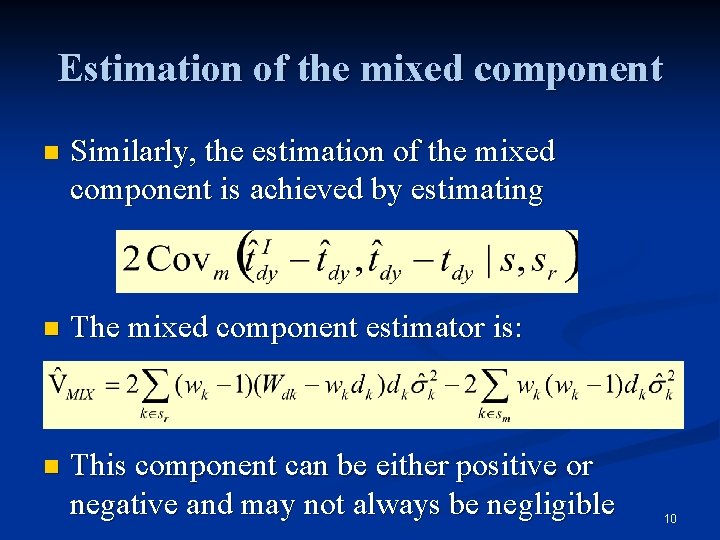
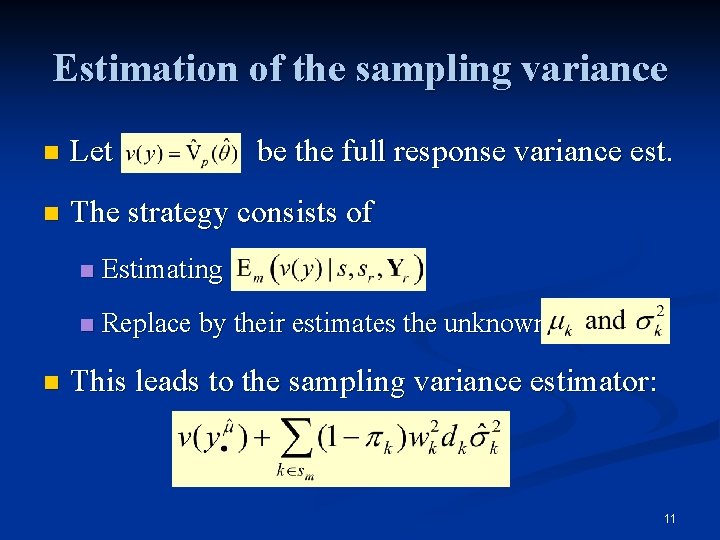
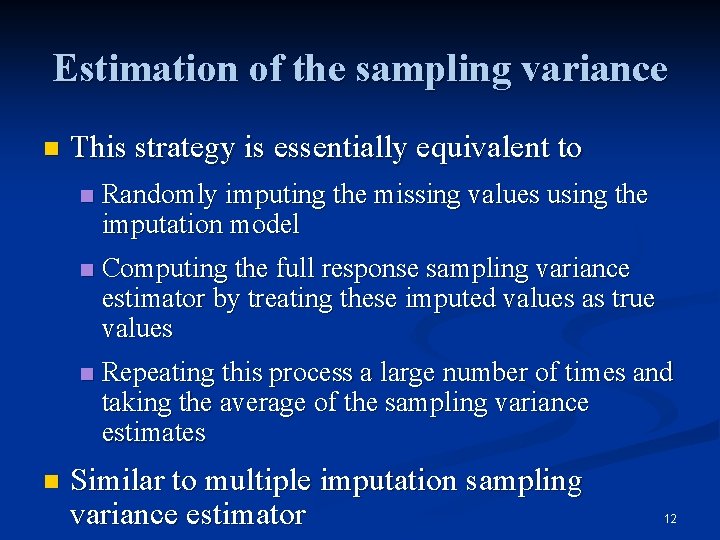
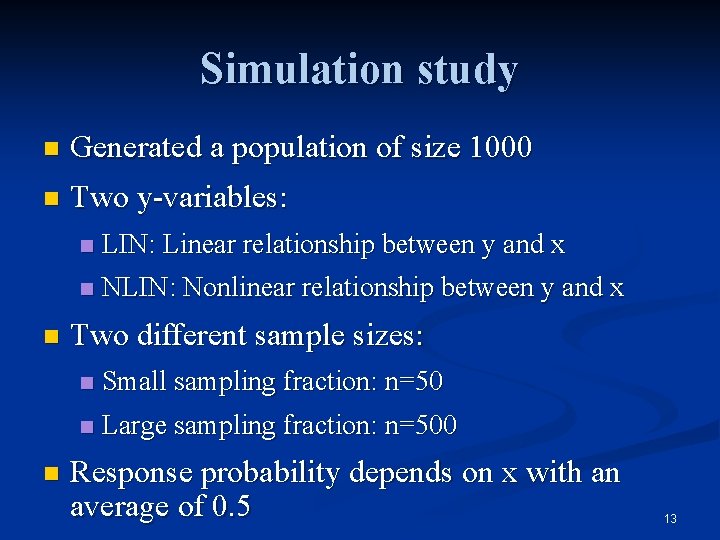
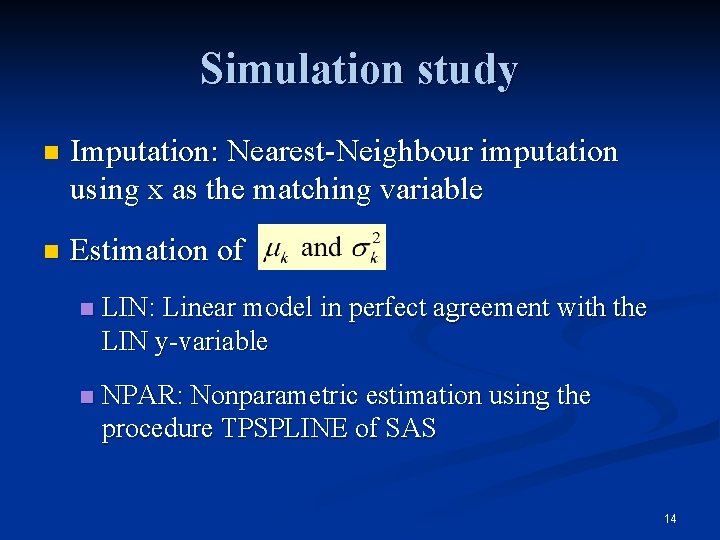
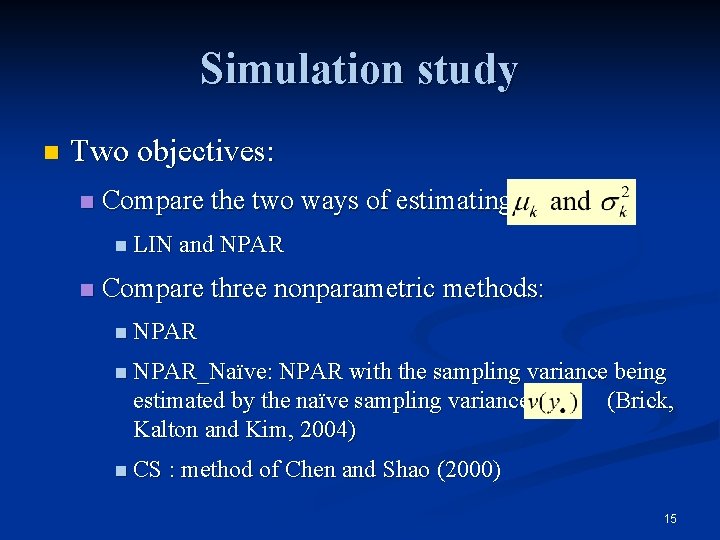
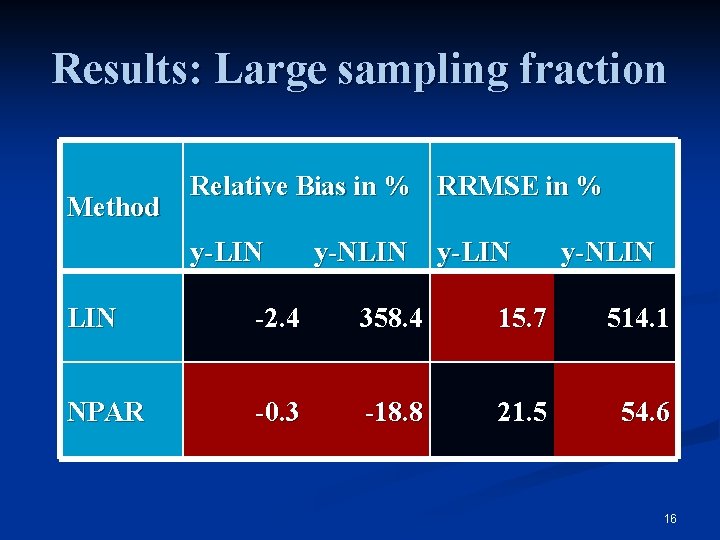
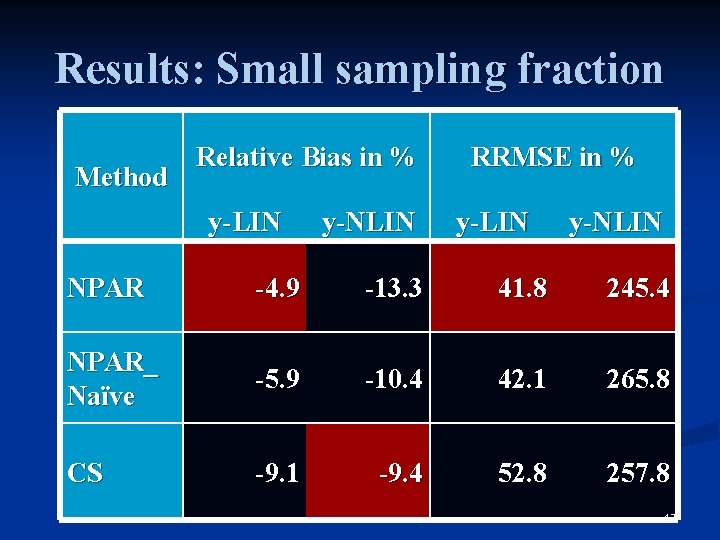
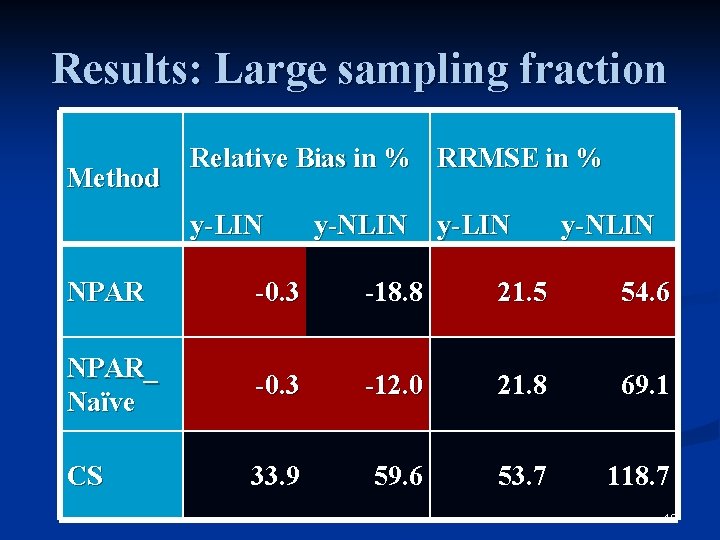
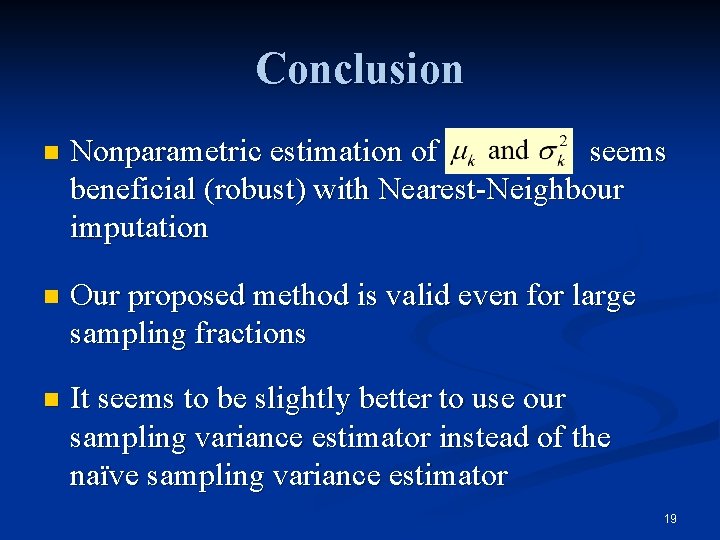
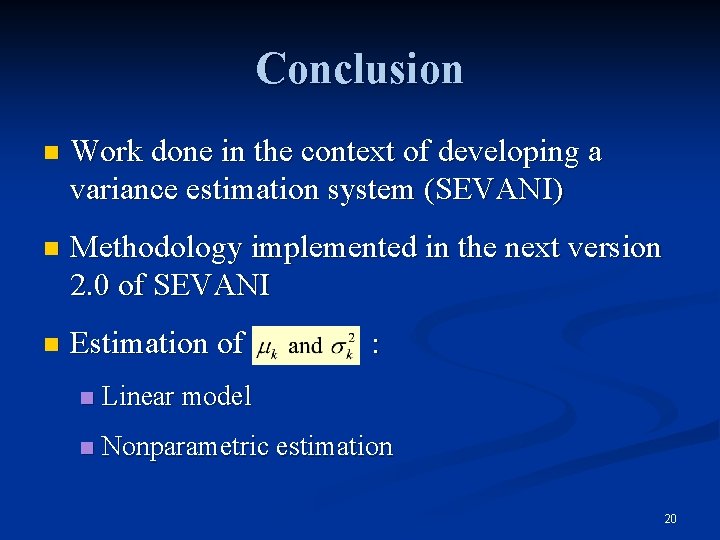
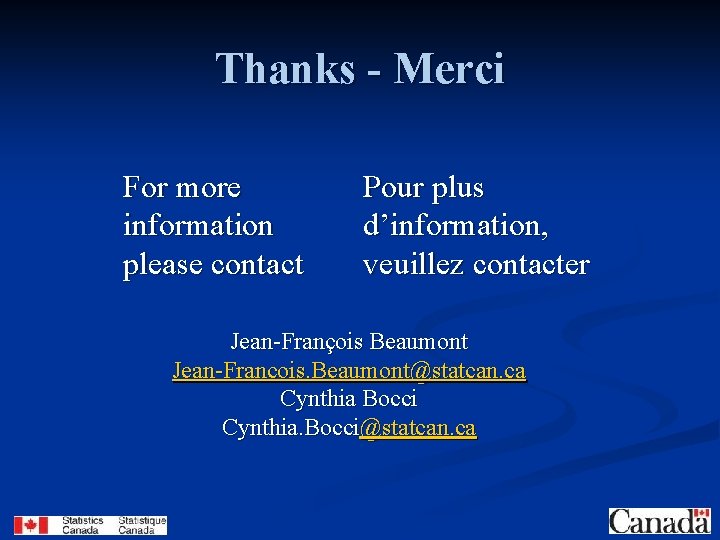
- Slides: 21
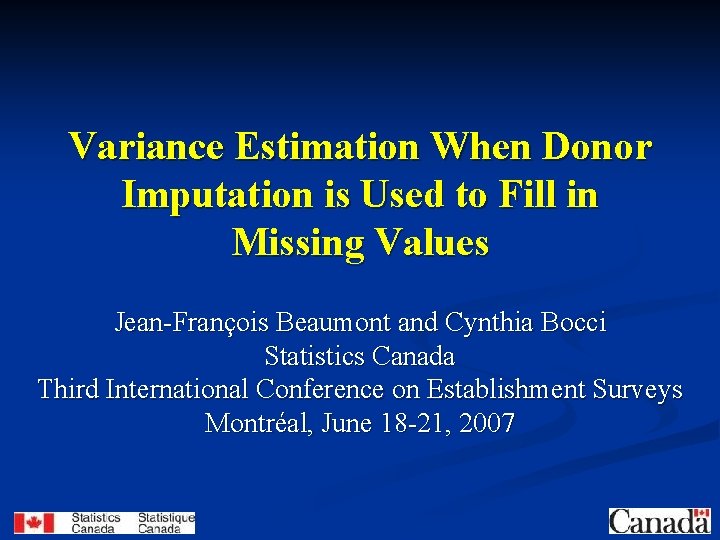
Variance Estimation When Donor Imputation is Used to Fill in Missing Values Jean-François Beaumont and Cynthia Bocci Statistics Canada Third International Conference on Establishment Surveys Montréal, June 18 -21, 2007
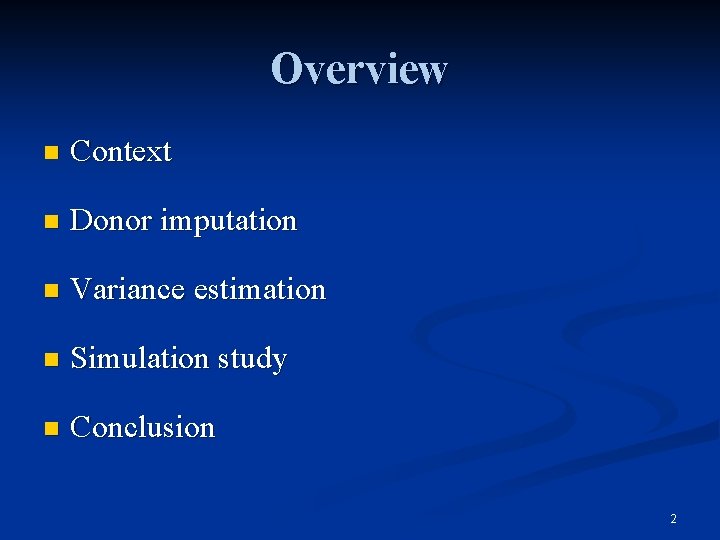
Overview n Context n Donor imputation n Variance estimation n Simulation study n Conclusion 2
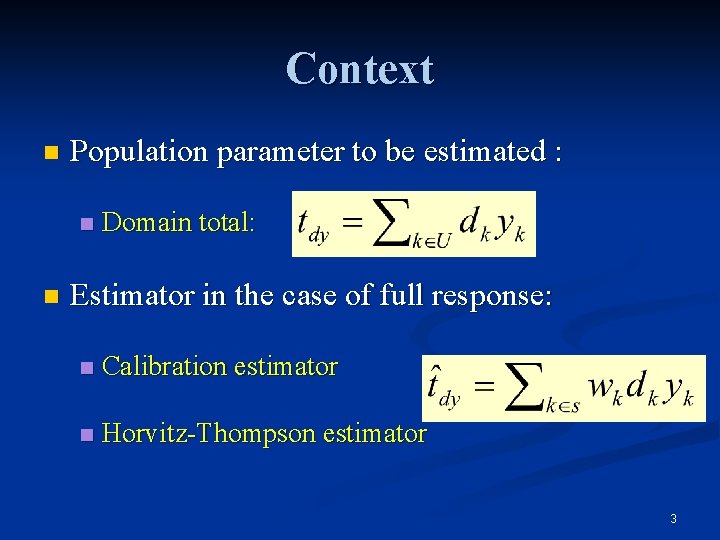
Context n Population parameter to be estimated : n n Domain total: Estimator in the case of full response: n Calibration estimator n Horvitz-Thompson estimator 3
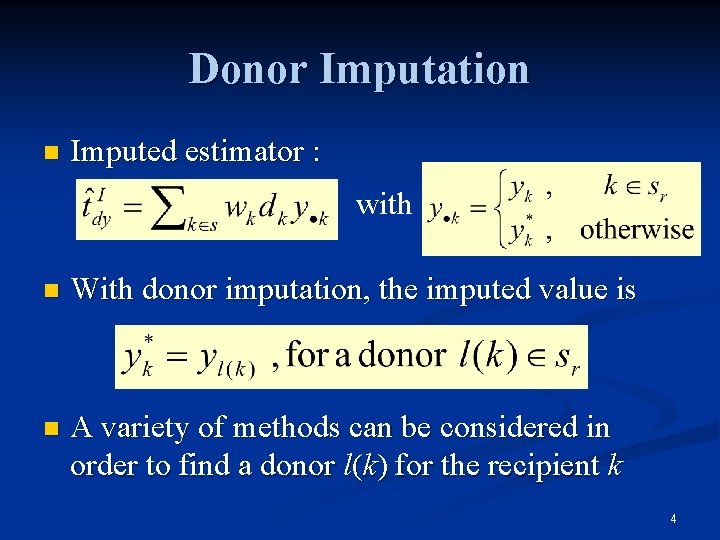
Donor Imputation n Imputed estimator : with n With donor imputation, the imputed value is n A variety of methods can be considered in order to find a donor l(k) for the recipient k 4
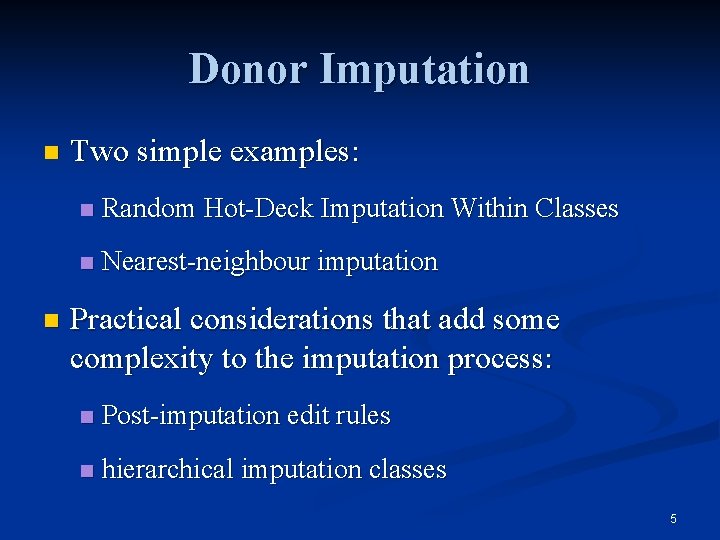
Donor Imputation n n Two simple examples: n Random Hot-Deck Imputation Within Classes n Nearest-neighbour imputation Practical considerations that add some complexity to the imputation process: n Post-imputation edit rules n hierarchical imputation classes 5
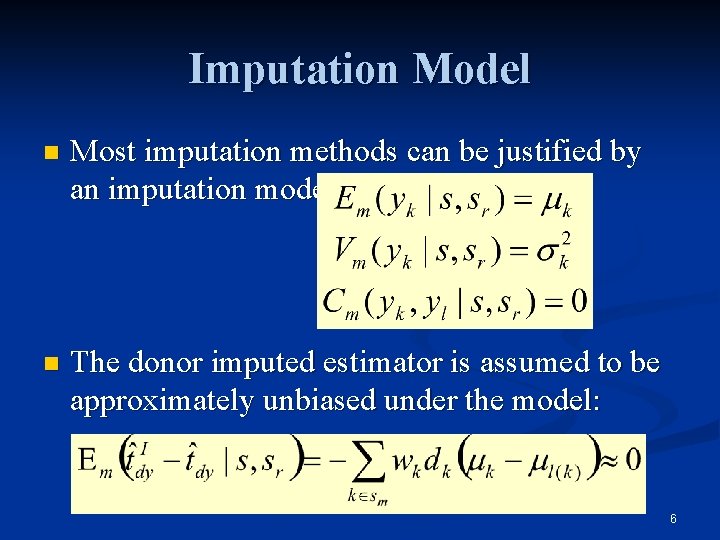
Imputation Model n Most imputation methods can be justified by an imputation model: n The donor imputed estimator is assumed to be approximately unbiased under the model: 6
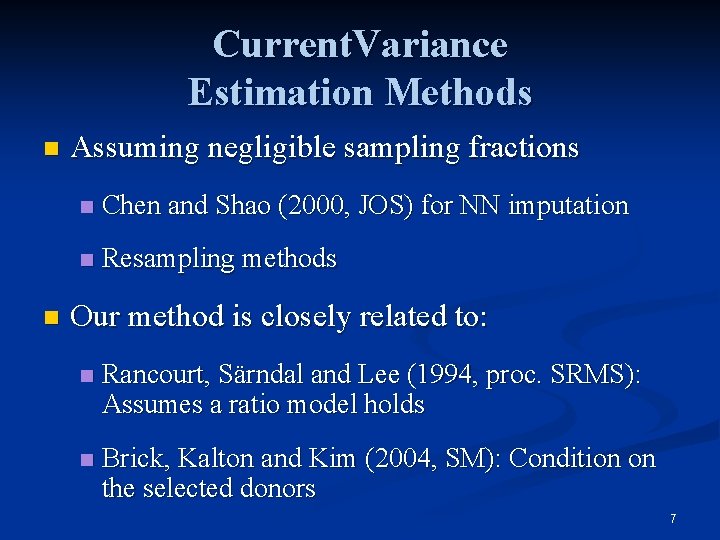
Current. Variance Estimation Methods n n Assuming negligible sampling fractions n Chen and Shao (2000, JOS) for NN imputation n Resampling methods Our method is closely related to: n Rancourt, Särndal and Lee (1994, proc. SRMS): Assumes a ratio model holds n Brick, Kalton and Kim (2004, SM): Condition on the selected donors 7
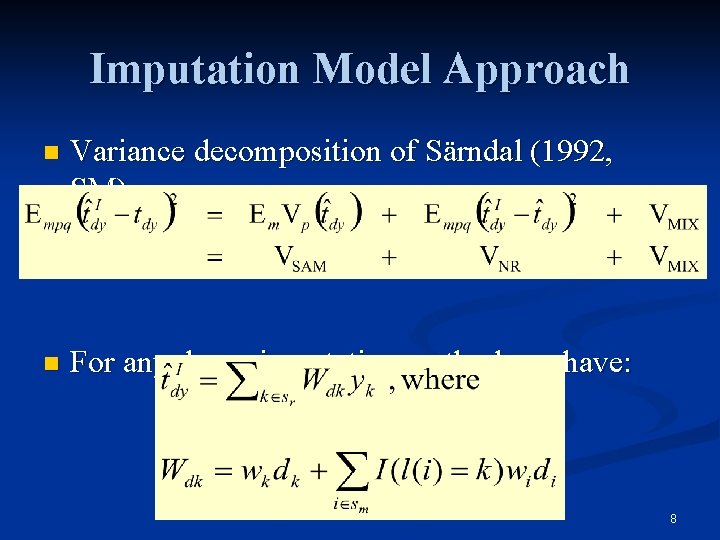
Imputation Model Approach n Variance decomposition of Särndal (1992, SM): n For any donor imputation method, we have: 8
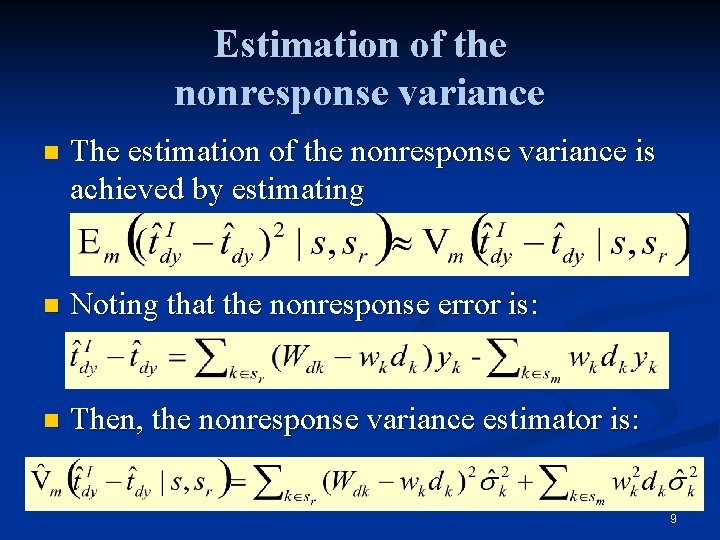
Estimation of the nonresponse variance n The estimation of the nonresponse variance is achieved by estimating n Noting that the nonresponse error is: n Then, the nonresponse variance estimator is: 9
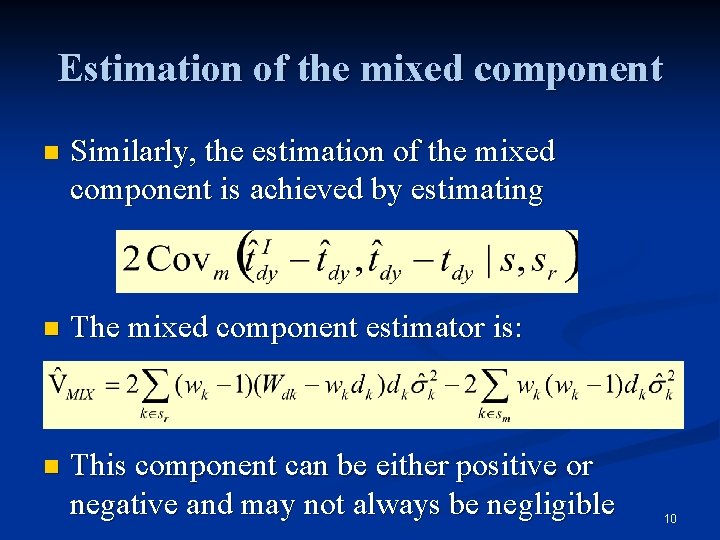
Estimation of the mixed component n Similarly, the estimation of the mixed component is achieved by estimating n The mixed component estimator is: n This component can be either positive or negative and may not always be negligible 10
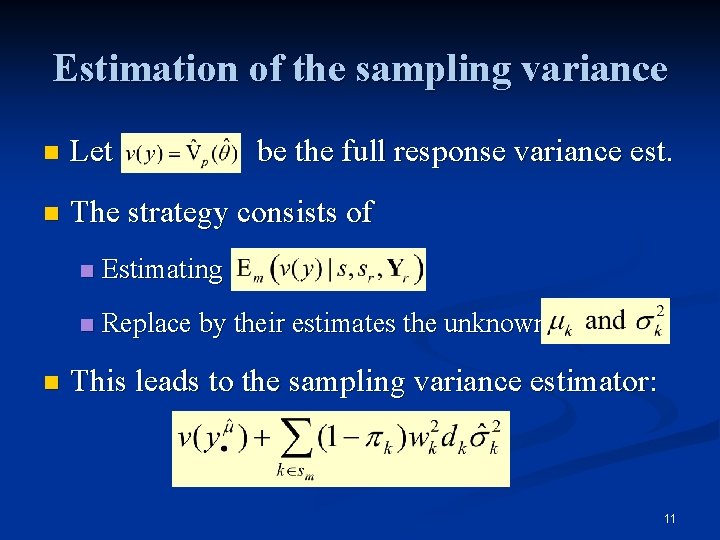
Estimation of the sampling variance n Let n The strategy consists of n be the full response variance est. n Estimating n Replace by their estimates the unknown This leads to the sampling variance estimator: 11
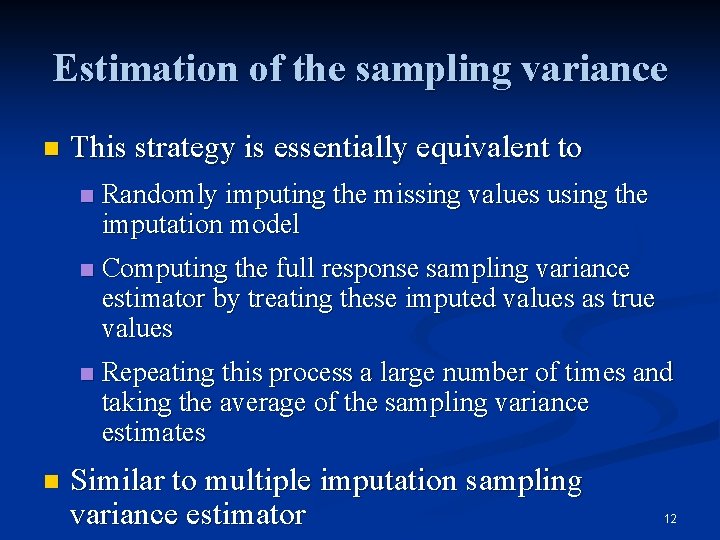
Estimation of the sampling variance n n This strategy is essentially equivalent to n Randomly imputing the missing values using the imputation model n Computing the full response sampling variance estimator by treating these imputed values as true values n Repeating this process a large number of times and taking the average of the sampling variance estimates Similar to multiple imputation sampling variance estimator 12
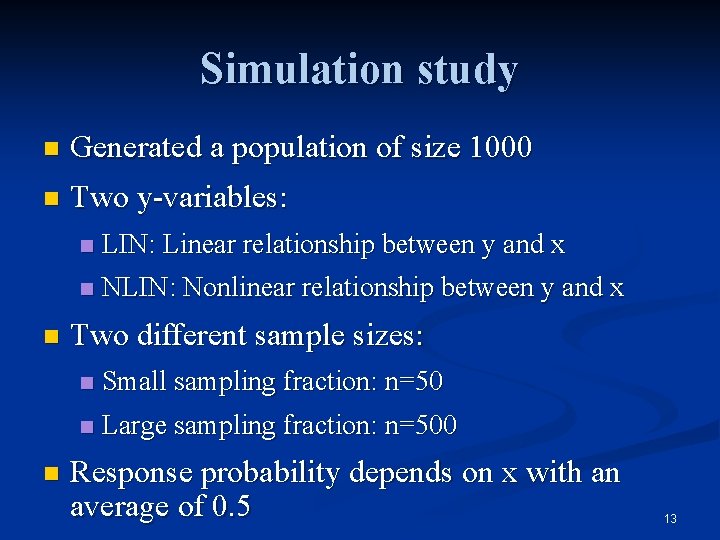
Simulation study n Generated a population of size 1000 n Two y-variables: n n n LIN: Linear relationship between y and x n NLIN: Nonlinear relationship between y and x Two different sample sizes: n Small sampling fraction: n=50 n Large sampling fraction: n=500 Response probability depends on x with an average of 0. 5 13
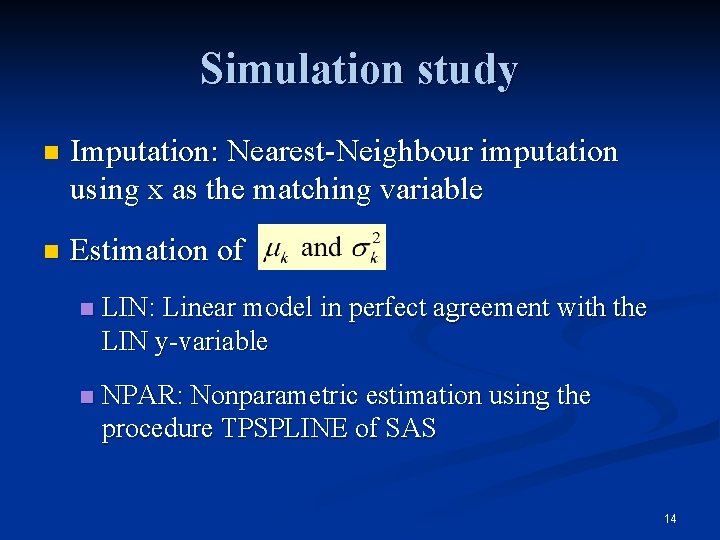
Simulation study n Imputation: Nearest-Neighbour imputation using x as the matching variable n Estimation of n LIN: Linear model in perfect agreement with the LIN y-variable n NPAR: Nonparametric estimation using the procedure TPSPLINE of SAS 14
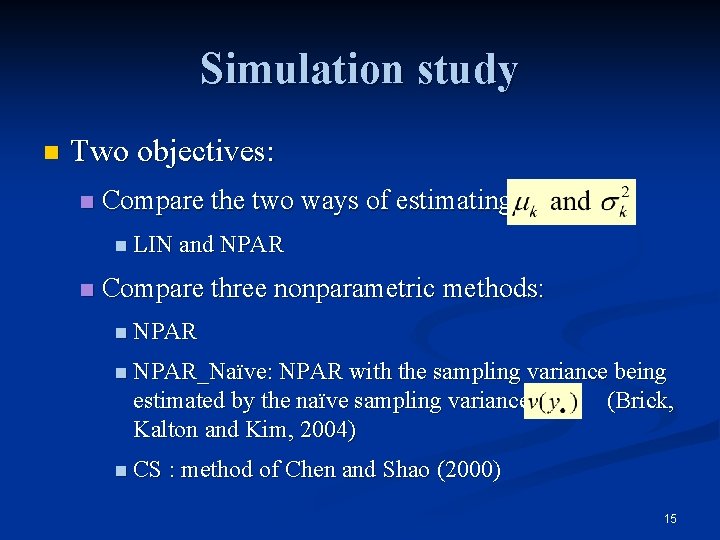
Simulation study n Two objectives: n Compare the two ways of estimating n LIN and NPAR n Compare three nonparametric methods: n NPAR_Naïve: NPAR with the sampling variance being estimated by the naïve sampling variance Kalton and Kim, 2004) n CS : method of Chen and (Brick, Shao (2000) 15
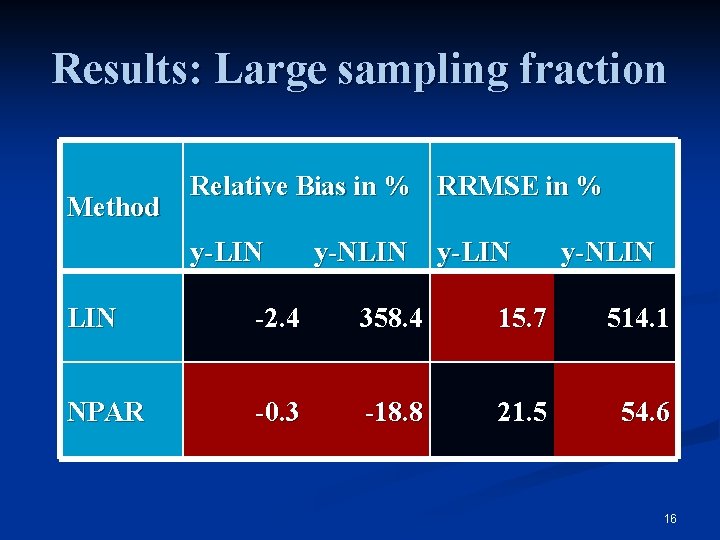
Results: Large sampling fraction Method Relative Bias in % RRMSE in % y-LIN y-NLIN LIN -2. 4 358. 4 15. 7 514. 1 NPAR -0. 3 -18. 8 21. 5 54. 6 16
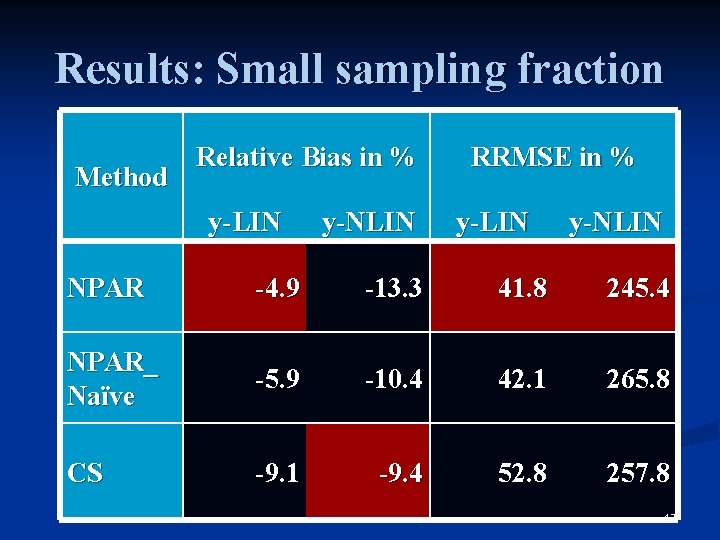
Results: Small sampling fraction Method Relative Bias in % y-LIN y-NLIN RRMSE in % y-LIN y-NLIN NPAR -4. 9 -13. 3 41. 8 245. 4 NPAR_ Naïve -5. 9 -10. 4 42. 1 265. 8 CS -9. 1 -9. 4 52. 8 257. 8 17
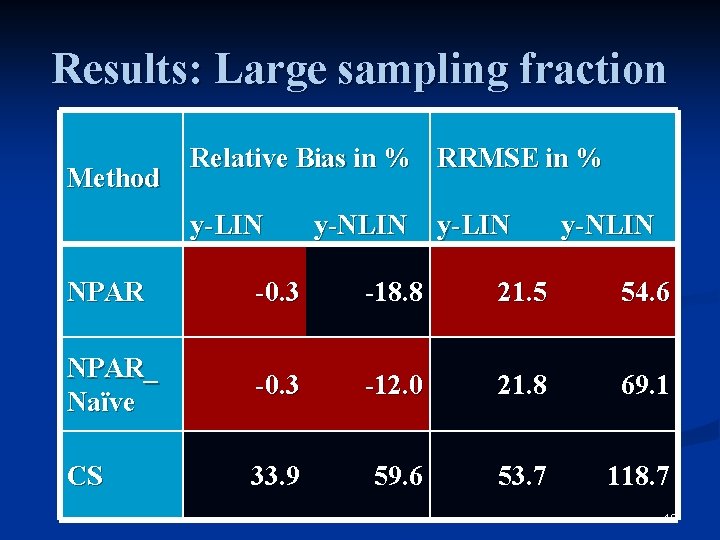
Results: Large sampling fraction Method Relative Bias in % RRMSE in % y-LIN y-NLIN NPAR -0. 3 -18. 8 21. 5 54. 6 NPAR_ Naïve -0. 3 -12. 0 21. 8 69. 1 CS 33. 9 59. 6 53. 7 118. 7 18
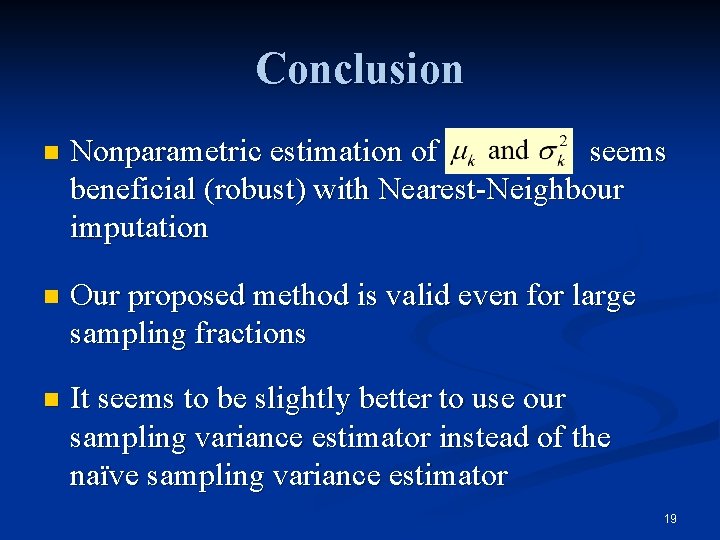
Conclusion n Nonparametric estimation of seems beneficial (robust) with Nearest-Neighbour imputation n Our proposed method is valid even for large sampling fractions n It seems to be slightly better to use our sampling variance estimator instead of the naïve sampling variance estimator 19
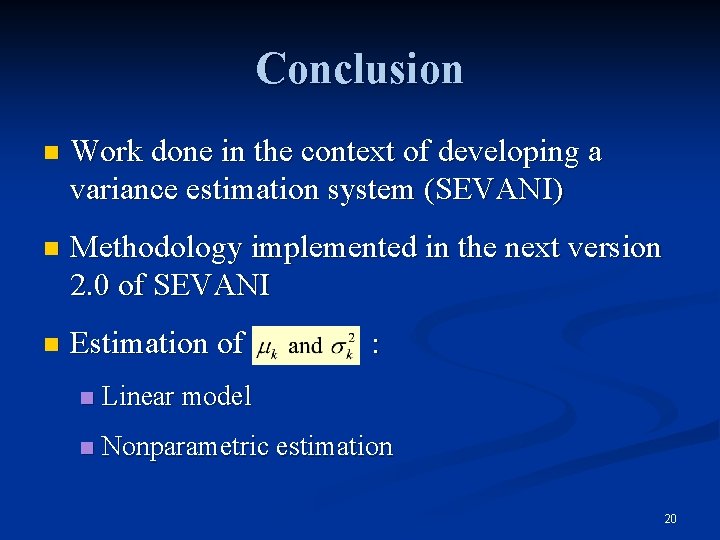
Conclusion n Work done in the context of developing a variance estimation system (SEVANI) n Methodology implemented in the next version 2. 0 of SEVANI n Estimation of : n Linear model n Nonparametric estimation 20
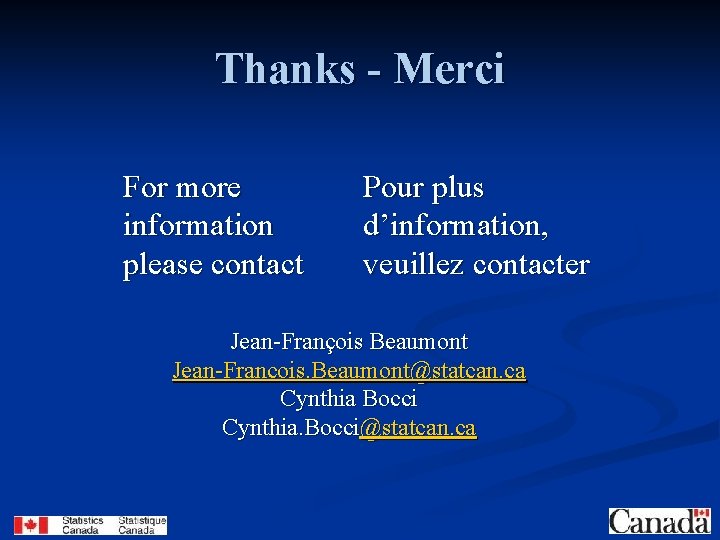
Thanks - Merci For more information please contact Pour plus d’information, veuillez contacter Jean-François Beaumont Jean-Francois. Beaumont@statcan. ca Cynthia Bocci Cynthia. Bocci@statcan. ca 21
Michigan imputation server
Missing data imputation
Boni de suractivité
Mplus missing data
Material usage variance formula
Fluorimetric analysis is used in estimation of *
Refractive index in physical pharmaceutics
Donor recognition levels
Contoh brosur donor darah
"donor alliance"
Konos donor
Rdp and sdp difference
Donor commitment continuum
Donor mapping
Example of donor impurities
Pi donor ligands
Donor manager trapianti
"donor direct"
Donor matrix
Donor centric fundraising
The objectives of report writing
Donor alliance recovery center